the Creative Commons Attribution 4.0 License.
the Creative Commons Attribution 4.0 License.
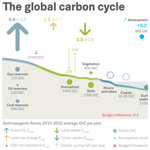
Global Carbon Budget 2023
Pierre Friedlingstein
Michael O'Sullivan
Matthew W. Jones
Robbie M. Andrew
Dorothee C. E. Bakker
Judith Hauck
Peter Landschützer
Corinne Le Quéré
Ingrid T. Luijkx
Glen P. Peters
Wouter Peters
Julia Pongratz
Clemens Schwingshackl
Stephen Sitch
Josep G. Canadell
Philippe Ciais
Robert B. Jackson
Simone R. Alin
Peter Anthoni
Leticia Barbero
Nicholas R. Bates
Meike Becker
Nicolas Bellouin
Bertrand Decharme
Laurent Bopp
Ida Bagus Mandhara Brasika
Patricia Cadule
Matthew A. Chamberlain
Naveen Chandra
Thi-Tuyet-Trang Chau
Frédéric Chevallier
Louise P. Chini
Margot Cronin
Xinyu Dou
Kazutaka Enyo
Wiley Evans
Stefanie Falk
Richard A. Feely
Liang Feng
Daniel J. Ford
Thomas Gasser
Josefine Ghattas
Thanos Gkritzalis
Giacomo Grassi
Luke Gregor
Nicolas Gruber
Özgür Gürses
Ian Harris
Matthew Hefner
Jens Heinke
Richard A. Houghton
George C. Hurtt
Yosuke Iida
Tatiana Ilyina
Andrew R. Jacobson
Atul Jain
Tereza Jarníková
Annika Jersild
Fei Jiang
Zhe Jin
Fortunat Joos
Etsushi Kato
Ralph F. Keeling
Daniel Kennedy
Kees Klein Goldewijk
Jürgen Knauer
Jan Ivar Korsbakken
Arne Körtzinger
Nathalie Lefèvre
Hongmei Li
Junjie Liu
Zhiqiang Liu
Lei Ma
Greg Marland
Nicolas Mayot
Patrick C. McGuire
Galen A. McKinley
Gesa Meyer
Eric J. Morgan
David R. Munro
Shin-Ichiro Nakaoka
Yosuke Niwa
Kevin M. O'Brien
Are Olsen
Abdirahman M. Omar
Tsuneo Ono
Melf Paulsen
Denis Pierrot
Katie Pocock
Benjamin Poulter
Carter M. Powis
Gregor Rehder
Laure Resplandy
Eddy Robertson
Christian Rödenbeck
Thais M. Rosan
Jörg Schwinger
Roland Séférian
T. Luke Smallman
Stephen M. Smith
Reinel Sospedra-Alfonso
Adrienne J. Sutton
Colm Sweeney
Shintaro Takao
Pieter P. Tans
Hanqin Tian
Bronte Tilbrook
Hiroyuki Tsujino
Francesco Tubiello
Guido R. van der Werf
Erik van Ooijen
Rik Wanninkhof
Michio Watanabe
Cathy Wimart-Rousseau
Dongxu Yang
Xiaojuan Yang
Wenping Yuan
Sönke Zaehle
Jiye Zeng
Accurate assessment of anthropogenic carbon dioxide (CO2) emissions and their redistribution among the atmosphere, ocean, and terrestrial biosphere in a changing climate is critical to better understand the global carbon cycle, support the development of climate policies, and project future climate change. Here we describe and synthesize data sets and methodology to quantify the five major components of the global carbon budget and their uncertainties. Fossil CO2 emissions (EFOS) are based on energy statistics and cement production data, while emissions from land-use change (ELUC), mainly deforestation, are based on land-use and land-use change data and bookkeeping models. Atmospheric CO2 concentration is measured directly, and its growth rate (GATM) is computed from the annual changes in concentration. The ocean CO2 sink (SOCEAN) is estimated with global ocean biogeochemistry models and observation-based fCO2 products. The terrestrial CO2 sink (SLAND) is estimated with dynamic global vegetation models. Additional lines of evidence on land and ocean sinks are provided by atmospheric inversions, atmospheric oxygen measurements, and Earth system models. The resulting carbon budget imbalance (BIM), the difference between the estimated total emissions and the estimated changes in the atmosphere, ocean, and terrestrial biosphere, is a measure of imperfect data and incomplete understanding of the contemporary carbon cycle. All uncertainties are reported as ±1σ.
For the year 2022, EFOS increased by 0.9 % relative to 2021, with fossil emissions at 9.9±0.5 Gt C yr−1 (10.2±0.5 Gt C yr−1 when the cement carbonation sink is not included), and ELUC was 1.2±0.7 Gt C yr−1, for a total anthropogenic CO2 emission (including the cement carbonation sink) of 11.1±0.8 Gt C yr−1 (40.7±3.2 Gt CO2 yr−1). Also, for 2022, GATM was 4.6±0.2 Gt C yr−1 (2.18±0.1 ppm yr−1; ppm denotes parts per million), SOCEAN was 2.8±0.4 Gt C yr−1, and SLAND was 3.8±0.8 Gt C yr−1, with a BIM of −0.1 Gt C yr−1 (i.e. total estimated sources marginally too low or sinks marginally too high). The global atmospheric CO2 concentration averaged over 2022 reached 417.1±0.1 ppm. Preliminary data for 2023 suggest an increase in EFOS relative to 2022 of +1.1 % (0.0 % to 2.1 %) globally and atmospheric CO2 concentration reaching 419.3 ppm, 51 % above the pre-industrial level (around 278 ppm in 1750). Overall, the mean of and trend in the components of the global carbon budget are consistently estimated over the period 1959–2022, with a near-zero overall budget imbalance, although discrepancies of up to around 1 Gt C yr−1 persist for the representation of annual to semi-decadal variability in CO2 fluxes. Comparison of estimates from multiple approaches and observations shows the following: (1) a persistent large uncertainty in the estimate of land-use changes emissions, (2) a low agreement between the different methods on the magnitude of the land CO2 flux in the northern extra-tropics, and (3) a discrepancy between the different methods on the strength of the ocean sink over the last decade.
This living-data update documents changes in methods and data sets applied to this most recent global carbon budget as well as evolving community understanding of the global carbon cycle. The data presented in this work are available at https://doi.org/10.18160/GCP-2023 (Friedlingstein et al., 2023).
Global fossil CO2 emissions (including cement carbonation) are expected to further increase in 2023, to 1.4 % above their pre-COVID-19 pandemic 2019 level. The 2022 emission increase was 0.09 Gt C yr−1 (0.33 Gt CO2 yr−1) relative to 2021, bringing 2022 fossil CO2 emissions to 9.9±0.5 Gt C yr−1 (36.4±1.8 Gt CO2 yr−1), virtually equal to the emission level of 2019. Preliminary estimates based on data available suggest fossil CO2 emissions will increase further in 2023, by 1.1 % relative to 2022 (0.0 % to 2.1 %), bringing emissions to 10.0 Gt C yr−1 (36.8 Gt CO2 yr−1), 1.4 % above the 2019 level.
Emissions from coal, oil, and gas in 2023 are all expected to be slightly above their 2022 levels (by 1.1 %, 1.5 %, and 0.5 %, respectively). Regionally, fossil emissions in 2023 are expected to decrease by 7.4 % in the European Union (0.7 Gt C, 2.6 Gt CO2) and by 3.0 % in the United States (1.3 Gt C, 4.9 Gt CO2), but they are expected to increase by 4.0 % in China (3.2 Gt C, 11.9 Gt CO2), 8.2 % in India (0.8 Gt C, 3.1 Gt CO2), and −0.4 % for the rest of the world (3.8 Gt C, 14.0 Gt CO2). International aviation and shipping (IAS) are expected to increase by 11.9 % (0.3 Gt C, 1.2 Gt CO2).
Fossil CO2 emissions decreased in 18 countries during the decade 2013–2022. Altogether, these 18 countries have contributed about 1.9 Gt C yr−1 (7.1 Gt CO2) to fossil fuel CO2 emissions over the last decade, representing about 20 % of world CO2 fossil emissions.
Global CO2 emissions from land use, land-use change, and forestry (LULUCF) averaged 1.3±0.7 Gt C yr−1 (4.7±2.6 Gt CO2 yr−1) for the 2013–2022 period with a preliminary projection for 2023 of 1.1±0.7 Gt C yr−1 (4.0±2.6 Gt CO2 yr−1). A small decrease over the past 2 decades is not robust given the large model uncertainty. Emissions from deforestation, the main driver of global gross sources, remain high at around 1.9 Gt C yr−1 over the 2013–2022 period, highlighting the strong potential of halting deforestation for emissions reductions. Sequestration of 1.3 Gt C yr−1 through re-/afforestation and forestry offsets two-thirds of the deforestation emissions. Emissions from other land-use transitions and from peat drainage and peat fire add further smaller contributions. The highest emitters during 2013–2022 in descending order were Brazil, Indonesia, and the Democratic Republic of the Congo, with these three countries contributing more than half of global land-use CO2 emissions.
Total anthropogenic emissions were 11.1 Gt C yr−1 (40.7 Gt CO2 yr−1) in 2022, with a similar preliminary estimate of 11.1 Gt C yr−1 (40.9 Gt CO2 yr−1) for 2023.
The remaining carbon budget for a 50 % likelihood to limit global warming to 1.5, 1.7, and 2 ∘C has reduced to 75 Gt C (275 Gt CO2), 175 Gt C (625 Gt CO2), and 315 Gt C (1150 Gt CO2), respectively, from the beginning of 2024, equivalent to around 7, 15, and 28 years, assuming 2023 emission levels.
The concentration of CO2 in the atmosphere is set to reach 419.3 parts per million (ppm) in 2023, 51 % above pre-industrial levels. The atmospheric CO2 growth was 5.2±0.02 Gt C yr−1 during the decade 2013–2022 (47 % of total CO2 emissions) with a preliminary 2023 growth rate estimate of around 5.1 Gt C (2.4 ppm).
The ocean CO2 sink resumed a more rapid growth in the past 2 decades after low or no growth during the 1991–2002 period, overlaid with imprints of climate variability. The estimates based on fCO2 products and models diverge with the growth of the ocean CO2 sink in the past decade being larger by a factor of 2.5 compared to in the models. This discrepancy in the trend originates from all latitudes but is largest in the Southern Ocean. The ocean CO2 sink was 2.9±0.4 Gt C yr−1 during the decade 2013–2022 (26 % of total CO2 emissions) and did not grow after 2019 due to a triple La Niña event. A similar value of 2.9 Gt C yr−1 is preliminarily estimated for 2023, which marks an increase in the sink compared to the last 2 years due to the transition from La Niña to El Niño conditions in 2023.
The land CO2 sink continued to increase during the 2013–2022 period primarily in response to increased atmospheric CO2, albeit with large interannual variability. The land CO2 sink was 3.3±0.8 Gt C yr−1 during the 2013–2022 decade (31 % of total CO2 emissions), 0.4 Gt C yr−1 larger than during the previous decade (2000–2009), with a preliminary 2023 estimate of around 2.9 Gt C yr−1, significantly lower than in 2022, and this is attributed to the response of the land biosphere to the emerging El Niño in 2023. Year-to-year variability in the land sink is about 1 Gt C yr−1 and dominates the year-to-year changes in the global atmospheric CO2 concentration, implying that small annual changes in anthropogenic emissions (such as the fossil fuel emission decrease in 2020) are hard to detect in the atmospheric CO2 observations.
The concentration of carbon dioxide (CO2) in the atmosphere has increased from approximately 278 parts per million (ppm) in 1750 (Gulev et al., 2021), the beginning of the industrial era, to 417.1±0.1 ppm in 2022 (Lan et al., 2023; Fig. 1). The atmospheric CO2 increase above pre-industrial levels was, initially, primarily caused by the release of carbon to the atmosphere from deforestation and other land-use change activities (Canadell et al., 2021). While emissions from fossil fuels started before the industrial era, they became the dominant source of anthropogenic emissions to the atmosphere from around 1950 and their relative share has continued to increase until the present. Anthropogenic emissions occur on top of an active natural carbon cycle that circulates carbon between the reservoirs of the atmosphere, ocean, and terrestrial biosphere on timescales from sub-daily to millennia, while exchanges with geologic reservoirs occur on longer timescales (Archer et al., 2009).
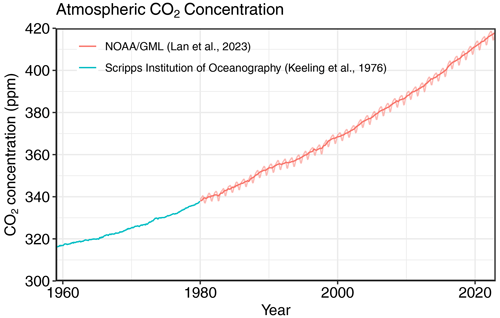
Figure 1Surface average atmospheric CO2 concentration (ppm). From 1980, monthly data are from NOAA/GML (Lan et al., 2023) and are based on an average of direct atmospheric CO2 measurements from multiple stations in the marine boundary layer (Masarie and Tans, 1995). The 1958–1979 monthly data are from the Scripps Institution of Oceanography, based on an average of direct atmospheric CO2 measurements from the Mauna Loa and South Pole stations (Keeling et al., 1976). To account for the difference in mean CO2 and seasonality between the NOAA/GML and the Scripps station networks used here, the Scripps surface average (from two stations) was de-seasonalized and adjusted to match the NOAA/GML surface average (from multiple stations) by adding the mean difference of 0.667 ppm, calculated here from overlapping data during 1980–2012.
The global carbon budget (GCB) presented here refers to the mean of, variations in, and trends in the perturbation of CO2 in the environment, referenced to the beginning of the industrial era (defined here as 1750). This paper describes the components of the global carbon cycle over the historical period with a stronger focus on the recent period (since 1958, onset of robust atmospheric CO2 measurements), the last decade (2013–2022), the last year (2022), and the current year (2023). Finally, it provides cumulative emissions from fossil fuels and land-use change since the year 1750 (the pre-industrial period) and since the year 1850 (the reference year for historical simulations in the Intergovernmental Panel on Climate Change Sixth Assessment Report (IPCC AR6)) (Eyring et al., 2016).
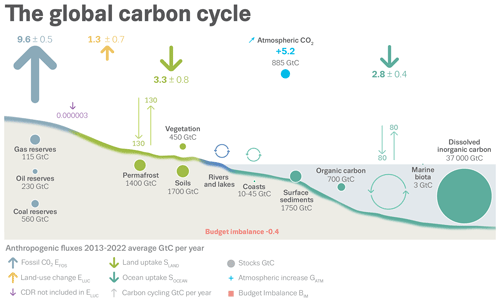
Figure 2Schematic representation of the overall perturbation of the global carbon cycle caused by anthropogenic activities, averaged globally for the decade 2013–2022. See legend for the corresponding arrows. Flux estimates and their 1 standard deviation uncertainty are as reported in Table 7. The uncertainty in the atmospheric CO2 growth rate is very small (±0.02 Gt C yr−1) and is neglected for the figure. The anthropogenic perturbation occurs on top of an active carbon cycle, with fluxes and stocks represented in the background and taken from Canadell et al. (2021) for all numbers, except for the carbon stocks in coasts, which is from a literature review of coastal marine sediments (Price and Warren, 2016). Fluxes are in gigatonnes of carbon per year (Gt C yr−1) and reservoirs in gigatonnes of carbon (Gt C).
We quantify the input of CO2 to the atmosphere by emissions from human activities; the growth rate of atmospheric CO2 concentration; and the resulting changes in the storage of carbon in the land and ocean reservoirs in response to increasing atmospheric CO2 levels, climate change, and variability and other anthropogenic and natural changes (Fig. 2). An understanding of this perturbation budget over time and the underlying variability in and trends of the natural carbon cycle is necessary to understand the response of natural sinks to changes in climate, changes in CO2, and land-use change drivers and to quantify emissions compatible with a given climate stabilization target.
The components of the CO2 budget that are reported annually in this paper include separate and independent estimates for the CO2 emissions from (1) fossil fuel combustion and oxidation from all energy and industrial processes, also including cement production and carbonation (EFOS; Gt C yr−1), and (2) deliberate human activities on land, including those leading to land-use change (ELUC; Gt C yr−1), and their partitioning among (3) the growth rate of atmospheric CO2 concentration (GATM; Gt C yr−1) and the uptake of CO2 (the “CO2 sinks”) (4) in the ocean (SOCEAN; Gt C yr−1) and (5) on land (SLAND; Gt C yr−1). The CO2 sinks as defined here conceptually include the response of the land (including inland waters and estuaries) and ocean (including coastal and marginal seas) to elevated CO2 and changes in climate and other environmental conditions, although in practice not all processes are fully accounted for (see Sect. 2.10). Global emissions and their partitioning among the atmosphere, ocean, and land are in balance in the real world. Due to the combination of imperfect spatial and/or temporal data coverage, errors in each estimate, and smaller terms not included in our budget estimate (discussed in Sect. 2.10), the independent estimates (1) to (5) above do not necessarily add up to zero. We therefore assess a set of additional lines of evidence derived from global atmospheric inversion system results (Sect. 2.7), observed changes in oxygen concentration (Sect. 2.8), and Earth system model (ESM) simulations (Sect. 2.9), all of which close the global carbon balance. We also estimate a budget imbalance (BIM), which is a measure of the mismatch between the estimated emissions and the estimated changes in the atmosphere, land, and ocean, as follows:
GATM is usually reported in parts per million per year (ppm yr−1), which we convert to units of carbon mass per year (Gt C yr−1), using 1 ppm = 2.124 Gt C (Ballantyne et al., 2012; Table 1). All quantities are presented in units of gigatonnes of carbon (Gt C, 1015 g C), which is the same as petagrams of carbon (Pg C; Table 1). Units of gigatonnes of CO2 (or billion tonnes of CO2) used in policy are equal to 3.664 multiplied by the value in units of gigatonnes of carbon (Gt C).
We also quantify EFOS and ELUC by country, including both territorial and consumption-based accounting for EFOS (see Sect. 2), and discuss missing terms from sources other than the combustion of fossil fuels (see Sects. 2.10, S1 and S2).
We now assess carbon dioxide removal (CDR) (see Sect. 2.2 and 2.3). Land-based CDR is significant but already accounted for in ELUC in Eq. (1) (Sect. 3.2.2). Other CDR methods, not based on vegetation, are currently several orders of magnitude smaller than the other components of the budget (Sect. 3.3); hence these are not included in Eq. (1) or in the global carbon budget tables or figures (with the exception of Fig. 2, where CDR is shown primarily for illustrative purpose).
The global CO2 budget has been assessed by the Intergovernmental Panel on Climate Change (IPCC) in all assessment reports (Prentice et al., 2001; Schimel et al., 1995; Watson et al., 1990; Denman et al., 2007; Ciais et al., 2013; Canadell et al., 2021) and by others (e.g. Ballantyne et al., 2012). The Global Carbon Project (GCP; http://www.globalcarbonproject.org, last access: 9 November 2023) has coordinated this cooperative community effort for the annual publication of global carbon budgets for the year 2005 (Raupach et al., 2007; including fossil emissions only), year 2006 (Canadell et al., 2007), year 2007 (GCP, 2007), year 2008 (Le Quéré et al., 2009), year 2009 (Friedlingstein et al., 2010), year 2010 (Peters et al., 2012), year 2012 (Le Quéré et al., 2013; Peters et al., 2013), year 2013 (Le Quéré et al., 2014), year 2014 (Le Quéré et al., 2015a; Friedlingstein et al., 2014), year 2015 (Jackson et al., 2016; Le Quéré et al., 2015b), year 2016 (Le Quéré et al., 2016), year 2017 (Le Quéré et al., 2018a; Peters et al., 2017), year 2018 (Le Quéré et al., 2018b; Jackson et al., 2018), year 2019 (Friedlingstein et al., 2019; Jackson et al., 2019; Peters et al., 2020), year 2020 (Friedlingstein et al., 2020; Le Quéré et al., 2021), year 2021 (Friedlingstein et al., 2022a; Jackson et al., 2022), and most recently year 2022 (Friedlingstein et al., 2022b). Each of these papers updated previous estimates with the latest available information for the entire time series.
We adopt a range of ±1 standard deviation (σ) to report the uncertainties in our global estimates, representing a likelihood of 68 % that the true value will be within the provided range if the errors have a Gaussian distribution, and no bias is assumed. This choice reflects the difficulty of characterizing the uncertainty in the CO2 fluxes between the atmosphere and the ocean and land reservoirs individually, particularly on an annual basis, as well as the difficulty of updating the CO2 emissions from land-use change. A likelihood of 68 % provides an indication of our current capability to quantify each term and its uncertainty given the available information. The uncertainties reported here combine statistical analysis of the underlying data, assessments of uncertainties in the generation of the data sets, and expert judgement of the likelihood of results lying outside this range. The limitations of current information are discussed in the paper and have been examined in detail elsewhere (Ballantyne et al., 2015; Zscheischler et al., 2017). We also use a qualitative assessment of the confidence level to characterize the annual estimates from each term based on the type, amount, quality, and consistency of the different lines of evidence as defined by the IPCC (Stocker et al., 2013).
Table 1Factors used to convert carbon in various units (by convention, Unit 1 = Unit 2 × conversion).

aMeasurements of atmospheric CO2 concentration have units of dry-air mole fraction. “ppm” is an abbreviation for micromoles per mole, dry air. b The use of a factor of 2.124 assumes that all the atmosphere is well mixed within 1 year. In reality, only the troposphere is well mixed and the growth rate of CO2 concentration in the less well mixed stratosphere is not measured by sites from the NOAA network. Using a factor of 2.124 gives an approximation that the growth rate of CO2 concentration in the stratosphere equals that of the troposphere on a yearly basis.
This paper provides a detailed description of the data sets and methodology used to compute the global carbon budget estimates for the industrial period, from 1750 to 2023, and goes into more detail for the period since 1959. This paper is updated every year using the format of “living data” to keep a record of budget versions and the changes in new data, revision of data, and changes in methodology that lead to changes in estimates of the carbon budget. Additional materials associated with the release of each new version will be posted at the Global Carbon Project (GCP) website (http://www.globalcarbonproject.org/carbonbudget, last access: 9 November 2023), with fossil fuel emissions also available through the Global Carbon Atlas (http://www.globalcarbonatlas.org, last access: 9 November 2023). All underlying data used to produce the budget can also be found at https://globalcarbonbudget.org/ (last access: 9 November 2023). With this approach, we aim to provide the highest transparency and traceability in the reporting of CO2, the key driver of climate change.
Multiple organizations and research groups around the world generated the original measurements and data used to complete the global carbon budget. The effort presented here is thus mainly one of synthesis, where results from individual groups are collated, analysed, and evaluated for consistency. We facilitate access to original data with the understanding that primary data sets will be referenced in future work (see Table 2 for how to cite the data sets and the “Data availability” section). Descriptions of the measurements, models, and methodologies follow below, with more detailed descriptions of each component provided in the Supplement (Sects. S1 to S5).
This is the 18th version of the global carbon budget and the 12th revised version in the format of a living-data update in Earth System Science Data. It builds on the latest published global carbon budget of Friedlingstein et al. (2022b). The main changes this year are as follows: the inclusion of (1) data to the year 2022 and a projection for the global carbon budget for the year 2023, (2) CO2 uptake from carbon dioxide removal (CDR), (3) land and ocean net carbon flux estimates from changes in atmospheric oxygen concentration, (4) land and ocean net carbon flux estimates from ESMs, and (5) a revised method to estimate the current-year (2023) atmospheric CO2. The main methodological differences between recent annual carbon budgets (2019 to 2023) are summarized in Table 3, and previous changes since 2006 are provided in Table S8.
Table 3Main methodological changes in the global carbon budget since 2019. Methodological changes introduced in 1 year are kept for the following years unless noted. Empty cells mean there were no methodological changes introduced that year. LUC denotes land-use change; DGVM denotes dynamic global vegetation model; GHG denotes greenhouse gas; NH and SH denote the Northern Hemisphere and Southern Hemisphere, respectively. Table S8 lists methodological changes from the first global carbon budget publication up to 2018.
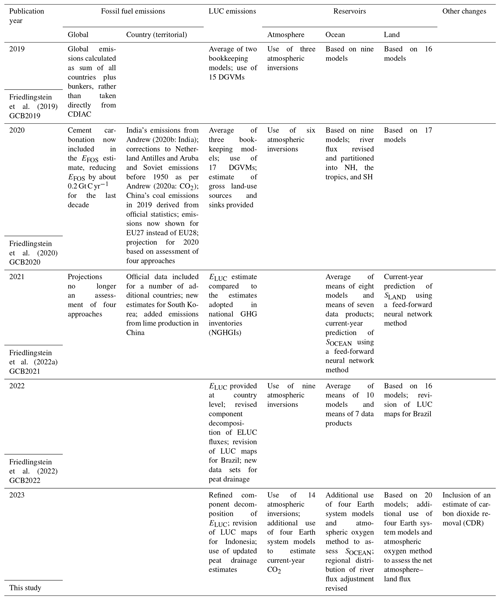
2.1 Fossil CO2 emissions (EFOS)
2.1.1 Historical period 1850–2022
The estimates of global and national fossil CO2 emissions (EFOS) include the oxidation of fossil fuels through both combustion (e.g. transport, heating) and chemical oxidation (e.g. carbon anode decomposition in aluminium refining) activities, as well as the decomposition of carbonates in industrial processes (e.g. the production of cement). We also include CO2 uptake from the cement carbonation process. Several emissions sources are not estimated or not fully covered: coverage of emissions from lime production is not global, and decomposition of carbonates in glass and ceramic production is included only for the “Annex I” countries of the United Nations Framework Convention on Climate Change (UNFCCC) for lack of activity data. These omissions are considered to be minor. Short-cycle carbon emissions – for example from combustion of biomass – are not included here but are accounted for in the CO2 emissions from land use (see Sect. 2.2).
Our estimates of fossil CO2 emissions rely on data collection by many other parties. Our goal is to produce the best estimate of this flux, and we therefore use a prioritization framework to combine data from different sources that have used different methods while being careful to avoid double counting and undercounting of emissions sources. The CDIAC-FF emissions data set, derived largely from UN energy data, forms the foundation, and we extend emissions to year Y−1 using energy growth rates reported by the Energy Institute (a data set formally produced by BP). We then proceed to replace estimates using data from what we consider to be superior sources, for example Annex I countries' official submissions to the UNFCCC. All data points are potentially subject to revision, not just the latest year. For full details see Andrew and Peters (2022).
Other estimates of global fossil CO2 emissions exist, and these are compared by Andrew (2020a). The most common reason for differences in estimates of global fossil CO2 emissions is a difference in which emissions sources are included in the data sets. Data sets such as those published by the energy company BP, the US Energy Information Administration, and the International Energy Agency's “CO2 emissions from fuel combustion” are all generally limited to emissions from combustion of fossil fuels. In contrast, data sets such as PRIMAP-hist, CEDS, EDGAR, and that of GCP's aim to include all sources of fossil CO2 emissions. See Andrew (2020a) for detailed comparisons and discussion.
Cement absorbs CO2 from the atmosphere over its lifetime, a process known as “cement carbonation”. We estimate this CO2 sink from 1931 onwards as the average of two studies in the literature (Cao et al., 2020; Guo et al., 2021). Both studies use the same model, developed by Xi et al. (2016), with different parameterizations and input data, with the estimate of Guo and colleagues being a revision of that of Xi et al. (2016). The trends of the two studies are very similar. Since carbonation is a function of both current and previous cement production, we extend these estimates to 2022 using the growth rate derived from the smoothed cement emissions (10-year smoothing) fitted to the carbonation data. In the present budget, we always include the cement carbonation carbon sink in the fossil CO2 emission component (EFOS).
We use the Kaya identity for a simple decomposition of CO2 emissions into the key drivers (Raupach et al., 2007). While there are variations (Peters et al., 2017), we focus here on a decomposition of CO2 emissions into population, gross domestic product (GDP) per person, energy use per GDP, and CO2 emissions per energy unit. Multiplying these individual components together returns the CO2 emissions. Using the decomposition, it is possible to attribute the change in CO2 emissions to the change in each of the drivers. This method gives a first-order understanding of what causes CO2 emissions to change each year.
2.1.2 Year 2023 projection
We provide a projection of global fossil CO2 emissions in 2022 by combining separate projections for China, the USA, the European Union (EU), India, and all other countries combined. The methods are different for each of these. For China we combine monthly fossil fuel production data from the National Bureau of Statistics and trade data from the customs administration, giving us partial data for the growth rates to date of natural gas, petroleum, and cement and of the apparent consumption itself for raw coal. We then use a regression model to project full-year emissions based on historical observations. For the USA our projection is taken directly from the Energy Information Administration (EIA) Short-Term Energy Outlook (EIA, 2023), combined with the year-to-date growth rate of cement clinker production. For the EU we use monthly energy data from Eurostat to derive estimates of monthly CO2 emissions through July, with coal emissions extended through September using a statistical relationship with reported electricity generation from coal and other factors. For natural gas we use Holt-Winters to project the last 4 months of the year. EU emissions from oil are derived using the EIA's projection of oil consumption for Europe. EU cement emissions are based on available year-to-date data from three of the largest producers, Germany, Poland, and Spain. India's projected emissions are derived from estimates through August (July for coal) using the methods of Andrew (2020b) and are extrapolated assuming seasonal patterns from before 2019. Emissions for the rest of the world are derived using projected growth in economic production from the IMF (2023) combined with extrapolated changes in the emission intensity of economic production. More details on the EFOS methodology and its 2023 projection can be found in the Supplement Sect. S1.
2.2 CO2 emissions from land use, land-use change, and forestry (ELUC)
2.2.1 Historical period 1850–2022
The net CO2 flux from land use, land-use change, and forestry (ELUC, called land-use change emissions in the rest of the text) includes CO2 fluxes from deforestation, afforestation, logging and forest degradation (including harvest activity), shifting cultivation (cycle of cutting forest for agriculture and then abandoning), and regrowth of forests (following wood harvest or agriculture abandonment). Emissions from peat burning and peat drainage are added from external data sets, peat drainage being averaged from three spatially explicit independent data sets (see the Supplement Sect. S2.1).
Three bookkeeping approaches (updated estimates for each of BLUE, Hansis et al., 2015; OSCAR, Gasser et al., 2020; and H&C2023, Houghton and Castanho, 2023) were used to quantify gross emissions and gross removals and the resulting net ELUC. Uncertainty estimates were derived from the dynamic global vegetation model (DGVM) ensemble for the time period prior to 1960 and using for the recent decades an uncertainty range of ±0.7 Gt C yr−1, which is a semi-quantitative measure for annual and decadal emissions and reflects our best value judgement that there is at least a 68 % chance (±1σ) that the true land-use change emission lies within the given range for the range of processes considered here.
Our ELUC estimates follow the definition of global carbon cycle models of CO2 fluxes related to land use and land management and differ from IPCC definitions adopted in national GHG inventories (NGHGIs) for reporting under the UNFCCC, which additionally generally include, through adoption of the IPCC so-called managed-land proxy approach, the terrestrial fluxes occurring on all land that countries define as managed. This partly includes fluxes due to environmental change (e.g. atmospheric CO2 increase), which are part of SLAND in our definition. This causes the global emission estimates to be smaller for NGHGIs than for the global carbon budget definition (Grassi et al., 2018). The same is the case for the Food and Agriculture Organization (FAO) estimates of carbon fluxes on forest land, which include both anthropogenic and natural sources on managed land (Tubiello et al., 2021). We map the two definitions onto each other to provide a comparison of the anthropogenic carbon budget to the official country reporting to the climate convention.
ELUC contains a range of fluxes that are related to carbon dioxide removal (CDR). CDR can be defined as the set of anthropogenic activities that remove CO2 from the atmosphere and store it in durable form, such as in forest biomass and soils, long-lived products, or geological or ocean reservoirs. We quantify vegetation-based CDR that is implicitly or explicitly captured by land-use fluxes consistent with our updated model estimates (CDR not based on vegetation is discussed in Sect. 2.3; IPCC, 2023). We quantify re-/afforestation from the three bookkeeping estimates by separating forest regrowth in shifting cultivation cycles from permanent increases in forest cover (see the Supplement Sect. S.2.1). The latter count as CDR, but it should be noted that the permanence of the storage under climate risks such as fire is increasingly being questioned. Other CDR activities contained in ELUC include the transfer of carbon to harvested wood products (HWPs), which is represented by the bookkeeping models with varying details concerning product usage and their lifetimes, bioenergy with carbon capture and storage (BECCS), and biochar production. Bookkeeping models and DGVMs currently only represent BECCS and biochar with regard to the CO2 removal through photosynthesis and not with regard to the durable storage. HWPs, BECCS, and biochar are typically counted as CDR when the transfer to the durable-storage site occurs and not when the CO2 is removed from the atmosphere, which complicates a direct comparison to the global carbon budget approach to quantify annual fluxes to and from the atmosphere. Estimates for CDR through HWPs, BECCS, and biochar are thus not indicated in this budget, but they can be found elsewhere (see Sect. 3.2.2).
2.2.2 Year 2023 projection
We project the 2023 land-use emissions for BLUE, H&C2023, and OSCAR based on their ELUC estimates for 2022 and adding the change in carbon emissions from peat fires and tropical deforestation and degradation fires (2023 emissions relative to 2022 emissions) estimated using active fire data (MCD14ML; Giglio et al., 2016). Peat drainage is assumed to be unaltered as it has low interannual variability. More details on the ELUC methodology can be found in the Supplement Sect. S.2.
2.3 Carbon dioxide removal (CDR) not based on vegetation
CDR not based on terrestrial vegetation currently relies on enhanced rock weathering and direct air carbon capture and storage (DACCS) projects. The majority of this (58 %) derives from a single project: Climeworks' Orca DACCS plant based in Hellisheiði, Iceland. The remainder is generated by 13 small-scale projects including, for example, 500 t of carbon dioxide sequestered through the spreading of crushed olivine on agricultural areas by Eion Carbon. We use data from the State of Carbon Dioxide Removal report (Smith et al., 2023), which quantifies all currently deployed CDR methods, including the land-use-related activities already covered by Sect. 2.2. The State of Carbon Dioxide Removal report (Smith et al., 2023) combines estimates of carbon storage in managed land derived from NGHGI data with project-by-project storage rates obtained through 20 extant CDR databases and registries (status as of mid-year 2022) by Powis et al. (2023). They assessed the quality of data on existing CDR projects to be poor, suffering from fragmentation, different reporting standards, limited geographical coverage, and the inclusion of a number of pilot plants with uncertain lifespans. As a consequence, these numbers could change substantially from year to year in the near-term future.
2.4 Growth rate of atmospheric CO2 concentration (GATM)
2.4.1 Historical period 1850–2022
The rate of growth of the atmospheric CO2 concentration is provided for the years 1959–2022 by the US National Oceanic and Atmospheric Administration Global Monitoring Laboratory (NOAA/GML; Lan et al., 2023), which includes recent revisions to the calibration scale of atmospheric CO2 measurements (Hall et al., 2021). For the 1959–1979 period, the global growth rate is based on measurements of atmospheric CO2 concentration averaged from the Mauna Loa and South Pole stations, as observed by the CO2 Program at Scripps Institution of Oceanography (Keeling et al., 1976). For the 1980–2021 time period, the global growth rate is based on the average of multiple stations selected from the marine-boundary-layer sites with well-mixed background air (Ballantyne et al., 2012), after fitting a smooth curve through the data for each station as a function of time and averaging by latitude band (Masarie and Tans, 1995). The annual growth rate is estimated by Lan et al. (2023) from atmospheric CO2 concentration by taking the average of the most recent December–January months corrected for the average seasonal cycle and subtracting this same average 1 year earlier. The growth rate in units of parts per million per year (ppm yr−1) is converted to units of gigatonnes of carbon per year (Gt C yr−1) by multiplying by a factor of 2.124 Gt C per ppm, assuming instantaneous mixing of CO2 throughout the atmosphere (Ballantyne et al., 2012; Table 1).
Since 2020, NOAA/GML has provided estimates of atmospheric CO2 concentrations with respect to a new calibration scale, referred to as WMO-CO2-X2019, in line with a recalibration agreed by the World Meteorological Organization (WMO) Global Atmosphere Watch (GAW) community (Hall et al., 2021). The recalibrated data were first used to estimate GATM in the 2021 edition of the global carbon budget (Friedlingstein et al., 2022a). Friedlingstein et al. (2022a) verified that the change of scales from WMO-CO2-X2007 to WMO-CO2-X2019 made a negligible difference to the value of GATM (−0.06 Gt C yr−1 during 2010–2019 and −0.01 Gt C yr−1 during 1959–2019, well within the uncertainty range reported below).
The uncertainty around the atmospheric growth rate is due to four main factors. The first factor is the long-term reproducibility of reference gas standards (around 0.03 ppm for 1σ from the 1980s; Lan et al., 2023). The second comprises small unexplained systematic analytical errors that may have a duration of several months to 2 years. They have been simulated by randomizing both the duration and the magnitude (determined from the existing evidence) in a Monte Carlo procedure. The third factor is the network composition of the marine boundary layer with some sites being added or removed, gaps in the time series at each site, etc. (Lan et al., 2023). The latter uncertainty was estimated by NOAA/GML with a Monte Carlo method by constructing 100 “alternative” networks (Masarie and Tans, 1995; NOAA/GML, 2019). The second and third uncertainties, summed in quadrature, add up to 0.085 ppm on average (Lan et al., 2023). The fourth factor is the uncertainty associated with using the average CO2 concentration from a surface network to approximate the true atmospheric average CO2 concentration (mass-weighted, in three dimensions) as needed to assess the total atmospheric CO2 burden. In reality, CO2 variations measured at the stations will not exactly track changes in total atmospheric burden, with offsets in magnitude and phasing due to vertical and horizontal mixing. This effect must be very small on decadal and longer timescales, when the atmosphere can be considered well mixed. The CO2 increase in the stratosphere lags the increase (meaning lower concentrations) that we observe in the marine boundary layer, while the continental boundary layer (where most of the emissions take place) leads the marine boundary layer with higher concentrations. These effects nearly cancel each other out. In addition the growth rate is nearly the same everywhere (Ballantyne et al., 2012). We therefore maintain an uncertainty around the annual growth rate based on the multiple stations' data set ranges between 0.11 and 0.72 Gt C yr−1, with a mean of 0.61 Gt C yr−1 for 1959–1979 and 0.17 Gt C yr−1 for 1980–2022, when a larger set of stations were available as provided by Lan et al. (2023). We estimate the uncertainty in the decadally averaged growth rate after 1980 at 0.02 Gt C yr−1 based on the calibration and the annual growth rate uncertainty but stretched over a 10-year interval. For the years prior to 1980, we estimate the decadally averaged uncertainty to be 0.07 Gt C yr−1 based on a factor proportional to the annual uncertainty prior to and after 1980 ( Gt C yr−1).
We assign a high confidence to the annual estimates of GATM because they are based on direct measurements from multiple and consistent instruments and stations distributed around the world (Ballantyne et al., 2012; Hall et al., 2021).
To estimate the total carbon accumulated in the atmosphere since 1750 or 1850, we use an atmospheric CO2 concentration of 278.3±3 ppm or 285.1±3 ppm, respectively (Gulev et al., 2021). For the construction of the cumulative budget shown in Fig. 3, we use the fitted estimates of CO2 concentration from Joos and Spahni (2008) to estimate the annual atmospheric growth rate using the conversion factors shown in Table 1. The uncertainty of ±3 ppm (converted to ±1σ) is taken directly from the IPCC's AR5 assessment (Ciais et al., 2013). Typical uncertainties in the growth rate of atmospheric CO2 concentration from ice core data are equivalent to ±0.1–0.15 Gt C yr−1 as evaluated from the Law Dome data (Etheridge et al., 1996) for individual 20-year intervals over the period from 1850 to 1960 (Bruno and Joos, 1997).
2.4.2 Year 2023 projection
We provide an assessment of GATM for 2023 as the average of two methods. The GCB regression method models monthly global-average atmospheric CO2 concentrations and derives the increment and annual average from these. The model uses lagged observations of concentration (Lan et al., 2023): both a 12-month lag and the lowest lag that will allow model prediction to produce an estimate for the following January, recalling that the GATM increment is derived from December–January pairs. The largest driver of interannual changes is the El Niño–Southern Oscillation (ENSO) signal (Betts et al., 2016), so the monthly Nino 3.4 index (Huang et al., 2017) is included in the model. Given the natural lag between sea surface temperatures and effects on the biosphere and in turn effects on globally mixed atmospheric CO2 concentration, a lagged ENSO index is used, and we use both a 5-month and a 6-month lag. The combination of the two lagged ENSO values helps reduce possible effects of noise in a single month. To help characterize the seasonal variation, we add month as a categorical variable. Finally, we flag the period affected by the Pinatubo eruption (August 1991–November 1993) as a categorical variable. Note that while emissions of CO2 are the largest driver of the trend in atmospheric CO2 concentration, our goal here is to predict divergence from that trend. Because changes in emissions from year to year are relatively minor, this has little effect on the variation in concentration from the trend line. Even the relatively large drop in emissions in 2020 due to the COVID-19 pandemic does not cause any problems for the model.
For the first time this year, we also use the multi-model mean of and uncertainty in the 2023 GATM estimated by the ESM prediction system (see Sect. 2.9). We then take the average of the GCB regression and ESM GATM estimates, with their respective uncertainty combined quadratically.
Similarly, the projection of the 2023 global-average CO2 concentration (in ppm) is calculated as the average of the estimates from the two methods. For the GCB regression method, it is the annual average of global concentration over the 12 months of 2023; for the ESMs, it is the observed global-average CO2 concentration for 2022 plus the annual increase in 2023 of the global-average CO2 concentration predicted by the ESM multi-model mean.
2.5 Ocean CO2 sink
2.5.1 Historical period 1850–2022
The reported estimate of the global ocean anthropogenic CO2 sink SOCEAN is derived as the average of two estimates. The first estimate is derived as the mean over an ensemble of 10 global ocean biogeochemistry models (GOBMs; Tables 4 and S2). The second estimate is obtained as the mean over an ensemble of seven surface ocean fCO2-observation-based (fugacity of CO2, which equals pCO2 corrected for the non-ideal behaviour of the gas; Pfeil et al., 2013) data products (Tables 4 and S3). An eighth fCO2 product (Watson et al., 2020) is shown, but it is not included in the ensemble average as it differs from the other products by adjusting the flux to a cool, salty ocean surface skin (see the Supplement Sect. S.3.1 for a discussion of the Watson product). The GOBMs simulate both the natural and the anthropogenic CO2 cycles in the ocean. They constrain the anthropogenic air–sea CO2 flux (the dominant component of SOCEAN) by the transport of carbon into the ocean interior, which is also the controlling factor of present-day ocean carbon uptake in the real world. They cover the full globe and all seasons and were recently evaluated against surface ocean carbon observations, suggesting they are suitable for estimating the annual ocean carbon sink (Hauck et al., 2020). The fCO2 products are tightly linked to observations of fCO2, which carry imprints of temporal and spatial variability but are also sensitive to uncertainties in gas-exchange parameterizations and data sparsity (Gloege et al., 2021; Hauck et al., 2023). Their asset is the assessment of the mean spatial pattern of variability and its seasonality (Hauck et al., 2020; Gloege et al., 2021; Hauck et al., 2023). We further use two diagnostic ocean models to estimate SOCEAN over the industrial era (1781–1958).
The global fCO2-based flux estimates were adjusted to remove the pre-industrial ocean source of CO2 to the atmosphere of 0.65±0.3 Gt C yr−1 from river input to the ocean (Regnier et al., 2022) and satisfy our definition of SOCEAN (Hauck et al., 2020). The river flux adjustment was distributed over the latitudinal bands using the regional distribution of Lacroix et al. (2020; north: 0.14 Gt C yr−1; tropics: 0.42 Gt C yr−1; south: 0.09 Gt C yr−1). Acknowledging that this distribution is based on only one model, the advantage is that a gridded field is available and the river flux adjustment can be calculated for the three latitudinal bands and the RECCAP regions (REgional Carbon Cycle Assessment and Processes (RECCAP-2); Ciais et al., 2022; Poulter et al., 2022; DeVries et al., 2023). This data set suggests that more of the riverine outgassing is located in the tropics than in the Southern Ocean and is thus opposed to the previously used data set of Aumont et al. (2001). Accordingly, the regional distribution is associated with a major uncertainty in addition to the large uncertainty around the global estimate (Crisp et al., 2022; Gruber et al., 2023). Anthropogenic perturbations of river carbon and nutrient transport to the ocean are not considered (see Sects. 2.10 and S.6.3).
We derive SOCEAN from GOBMs using a simulation (sim A) with historical forcing of climate and atmospheric CO2, accounting for model biases and drift from a control simulation (sim B) with constant atmospheric CO2 and normal-year climate forcing. A third simulation (sim C) with historical atmospheric CO2 increase and normal-year climate forcing is used to attribute the ocean sink to CO2 (sim C minus sim B) and climate (sim A minus sim C) effects. A fourth simulation (sim D; historical climate forcing and constant atmospheric CO2) is used to compare the change in the anthropogenic carbon inventory in the interior ocean (sim A minus sim D) to the observational estimate of Gruber et al. (2019) with the same flux components (steady-state and non-steady-state anthropogenic carbon flux). The fCO2 products are adjusted with respect to their original publications to represent the full ice-free ocean area, including coastal zones and marginal seas, when the area coverage is below 99 %. This is done by either area filling following Fay et al. (2021) or a simple scaling approach. GOBMs and fCO2 products fall within the observational constraints over the 1990s (2.2±0.7 Gt C yr−1; Ciais et al., 2013) after applying adjustments.
SOCEAN is calculated as the average of the GOBM ensemble mean and the fCO2-product ensemble mean from 1990 onwards. Prior to 1990, it is calculated as the GOBM ensemble mean plus half of the offset between GOBMs and fCO2-product ensemble means over 1990–2001.
We assign an uncertainty of ±0.4 Gt C yr−1 to the ocean sink based on a combination of random uncertainties (ensemble standard deviation) and systematic uncertainties (GOBM bias in anthropogenic carbon accumulation, previously reported uncertainties in fCO2 products; see Sect. S.3.4). We assign a medium confidence level to the annual ocean CO2 sink and its uncertainty because it is based on multiple lines of evidence, it is consistent with ocean interior carbon estimates (Gruber et al., 2019; see Sect. 3.6.5), and the interannual variability in the GOBMs and data-based estimates is largely consistent and can be explained by climate variability. We refrain from assigning a high confidence because of the systematic deviation between the GOBM and fCO2-product trends since around 2002. More details on the SOCEAN methodology can be found in Sect. S.3.
2.5.2 Year 2023 projection
The ocean CO2 sink forecast for the year 2023 is based on the annual historical (Lan et al., 2023) and our estimated 2023 atmospheric CO2 concentration growth rate, the historical and our estimated 2023 annual global fossil fuel emissions from this year's carbon budget, and the spring (March, April, May) Oceanic Niño Index (ONI) (NCEP, 2023). Using a non-linear regression approach, i.e. a feed-forward neural network, atmospheric CO2, ONI, and the fossil fuel emissions are used as training data to best match the annual ocean CO2 sink (i.e. combined SOCEAN estimate from GOBMs and data products) from 1959 through 2022 from this year's carbon budget. Using this relationship, the 2023 SOCEAN can then be estimated from the projected 2022 input data using the non-linear relationship established during the network training. To avoid overfitting, the neural network was trained with a variable number of hidden neurons (varying between 2–5), and 20 % of the randomly selected training data were withheld for independent internal testing. Based on the best output performance (tested using the 20 % withheld input data), the best-performing number of neurons was selected. In a second step, we trained the network 10 times using the best number of neurons identified in step 1 and different sets of randomly selected training data. The mean of the 10 training runs is considered our best forecast, whereas the standard deviation of the 10 ensembles provides a first-order estimate of the forecast uncertainty. This uncertainty is then combined with the SOCEAN uncertainty (0.4 Gt C yr−1) to estimate the overall uncertainty in the 2023 projection. As an additional line of evidence, we also assess the 2023 atmosphere–ocean carbon flux from the Earth system model (ESM) prediction system (see Sect. 2.9).
2.6 Land CO2 sink
2.6.1 Historical period 1850–2022
The terrestrial land sink (SLAND) is thought to be due to the combined effects of fertilization by rising atmospheric CO2 and N inputs on plant growth, as well as the effects of climate change such as the lengthening of the growing season in northern temperate and boreal areas. SLAND does not include land sinks directly resulting from land use and land-use change (e.g. regrowth of vegetation) as these are part of the land-use flux (ELUC), although system boundaries make it difficult to exactly attribute CO2 fluxes on land to SLAND and ELUC (Erb et al., 2013).
SLAND is estimated from the multi-model mean of 20 DGVMs (Table S1) with an additional comparison of DGVMs with a data-driven, carbon data model framework (CARDAMOM) (Bloom and Williams, 2015; Bloom et al., 2016); see Sect. S4. DGVM simulations include all climate variability and CO2 effects over land. In addition to the carbon cycle represented in all DGVMs, 14 models also account for the nitrogen cycle and hence can include the effect of N inputs on SLAND. The DGVM estimate of SLAND does not include the export of carbon to aquatic systems or its historical perturbation, which is discussed in Sect. S.6.3. DGVMs need to meet several criteria to be included in this assessment. In addition, we use the International Land Model Benchmarking (ILAMB) system (Collier et al., 2018) for the DGVM evaluation (see Sect. S.4.2). The uncertainty in SLAND is taken from the DGVMs' standard deviation (see Sect. S.4.3). More details on the SLAND methodology can be found in Sect. S.4.
2.6.2 Year 2023 projection
Like for the ocean forecast, the land CO2 sink (SLAND) forecast is based on the annual historical (Lan et al., 2023) and our estimated 2023 atmospheric CO2 concentration, historical and our estimated 2023 annual global fossil fuel emissions from this year's carbon budget, and the summer (June, July, August) ONI (NCEP, 2023). All training data are again used to best match SLAND from 1959 through 2022 from this year's carbon budget using a feed-forward neural network. To avoid overfitting, the neural network was trained with a variable number of hidden neurons (varying between 2–15), larger than for SOCEAN prediction due to the stronger land carbon interannual variability. As done for SOCEAN, pre-training selects the optimal number of hidden neurons based on 20 % withheld input data, and in a second step, an ensemble of 10 forecasts is produced to provide the mean forecast plus uncertainty. This uncertainty is then combined with the SLAND uncertainty for 2022 (0.9 Gt C yr−1) to estimate the overall uncertainty in the 2023 projection.
2.7 Atmospheric inversion estimate
The worldwide network of in situ atmospheric measurements and satellite-derived atmospheric CO2 column (xCO2) observations puts a strong constraint on changes in the atmospheric abundance of CO2. This is true not only globally (hence our large confidence in GATM) but also regionally in regions with sufficient observational density, found mostly in the extra-tropics. This allows atmospheric inversion methods to constrain the magnitude and location of the combined total surface CO2 fluxes from all sources, including fossil and land-use change emissions and land and ocean CO2 fluxes. The inversions assume EFOS to be well known, and they solve for the spatial and temporal distribution of land and ocean fluxes from the residual gradients of CO2 between stations that are not explained by fossil fuel emissions. By design, such systems thus close the carbon balance (BIM=0) and provide an additional perspective on the independent estimates of the ocean and land fluxes.
This year's release includes 14 inversion systems that are described in Table S4, of which 13 are included in the ensemble of inverse estimates presented in the text and figures. Each system is rooted in Bayesian inversion principles but uses different methodologies. These differences concern the selection of atmospheric CO2 data or xCO2 and the choice of a priori fluxes to refine. They also differ in spatial and temporal resolution, assumed correlation structures, and the mathematical approach of the models (see references in Table S4 for details). Importantly, the systems use a variety of transport models, which was demonstrated to be a driving factor behind differences in atmospheric-inversion-based flux estimates and specifically their distribution across latitudinal bands (Gaubert et al., 2019; Schuh et al., 2019). Six inversion systems (CAMS-FT23r1, CMS-Flux, GONGGA, THU, COLA, GCASv2) used satellite xCO2 retrievals from GOSAT and/or OCO-2, scaled to the WMO 2019 calibration scale. Two inversions this year (CMS-Flux, COLA) used these xCO2 data sets in addition to the in situ observational CO2 mole fraction records.
The original products delivered by the inverse modellers were modified to facilitate the comparison to the other elements of the budget, specifically on two accounts: (1) global total fossil fuel emissions including cement carbonation CO2 uptake and (2) riverine CO2 transport. Details are given below. We note that with these adjustments, the inverse results no longer represent the net atmosphere–surface exchange over land/ocean areas as sensed by atmospheric observations. Instead, for land, they become the net uptake of CO2 by vegetation and soils that is not exported by fluvial systems, which is similar to the DGVM estimates. For oceans, they become the net uptake of anthropogenic CO2, which is similar to the GOBM estimates.
The inversion systems prescribe global fossil fuel emissions based on, for example, GCP's Gridded Fossil Emissions Dataset version 2023.1 (GCP-GridFED; Jones et al., 2023), which is an update to GCP-GridFEDv2021 presented by M. W. Jones et al. (2021). GCP-GridFEDv2023 scales gridded estimates of CO2 emissions from EDGAR v4.3.2 (Janssens-Maenhout et al., 2019) within national territories to match national emission estimates provided by the GCB for the years 1959–2022, which were compiled following the methodology described in Sect. 2.1. Small differences between the systems due to for instance regridding to the transport model resolution or use of different fossil fuel emissions are adjusted in the latitudinal partitioning we present to ensure agreement with the estimate of EFOS in this budget. We also note that the ocean fluxes used as prior by 8 out of 14 inversions are part of the suite of the ocean process model or fCO2 products listed in Sect. 2.5. Although these fluxes are further adjusted by the atmospheric inversions, it makes the inversion estimates of the ocean fluxes not completely independent of SOCEAN assessed here.
To facilitate comparisons to the independent SOCEAN and SLAND, we used the same corrections for transport and outgassing of carbon transported from land to ocean, as done for the observation-based estimates of SOCEAN (see Sect. S.3).
The atmospheric inversions are evaluated using vertical profiles of atmospheric CO2 concentrations (Fig. S4). More than 30 aircraft programmes over the globe, either regular programmes or repeated surveys over at least 9 months (except for SH programmes), have been used to assess system performance (with space–time observational coverage sparse in the SH and tropics and denser in NH mid-latitudes; Table S7). The 14 systems are compared to the independent aircraft CO2 measurements between 2 and 7 km above sea level between 2001 and 2022. Results are shown in Fig. S4 and discussed in Sect. S.5.2. One inversion was flagged for concerns after quality control with these observations, as well as assessment of their global growth rate. This makes the number of systems included in the ensemble N=13.
With a relatively small ensemble of systems that cover at least 1 full decade (N=9) and which moreover share some a priori fluxes used with one another, or with the process-based models, it is difficult to justify using their mean and standard deviation as metrics for uncertainty across the ensemble. We therefore report their full range (min–max) without their mean. More details on the atmospheric inversion methodology can be found in Sect. S.5.
2.8 Atmospheric-oxygen-based estimate
Long-term atmospheric O2 and CO2 observations allow estimation of the global ocean and land carbon sinks due to the coupling of O2 and CO2 with distinct exchange ratios for fossil fuel emissions and land uptake and due to uncoupled O2 and CO2 ocean exchange (Keeling and Manning, 2014). The global ocean and net land carbon sinks were calculated following methods and constants used in Keeling and Manning (2014) but modified to also include the effective O2 source from metal refining (Battle et al., 2023), using a value of 1.05 for the exchange ratio of the net land sink following Resplandy et al. (2018). Atmospheric O2 is observed as δ() and combined with CO2 mole fraction observations into atmospheric potential oxygen (APO; Stephens et al., 1998). The APO observations from 1990 to 2022 were taken from a weighted average of flask records from the three stations in the Scripps O2 Program network (Alert, Canada (ALT); La Jolla, California (LJO); and Cape Grim, Australia (CGO), weighted as per Keeling and Manning, 2014). Observed CO2 was taken from the globally averaged marine surface annual mean growth rate from the NOAA/GML Global Greenhouse Gas Reference Network (Lan et al., 2023). The O2 source from ocean warming is based on ocean heat content from updated data from NOAA (Levitus et al., 2012). The effective O2 source from metal refining is based on production data from Bray (2020), Flanagan (2021), and Tuck (2022). Uncertainty was determined through a Monte Carlo approach with 5000 iterations, using uncertainties prescribed in Keeling and Manning (2014), including observational uncertainties from Keeling et al. (2007) and autoregressive errors in fossil fuel emissions (Ballantyne et al., 2015). The reported uncertainty is 1 standard deviation of the ensemble.
2.9 Earth system models' estimate
Reconstructions and predictions from decadal prediction systems based on Earth system models (ESMs) provide a novel line of evidence in assessing the atmosphere–land and atmosphere–ocean carbon fluxes in the past decades and predicting their changes for the current year. The decadal prediction systems based on ESMs used here consist of three sets of simulations: (i) uninitialized freely evolving historical simulations (1850–2014); (ii) assimilation reconstruction incorporating observational data into the model (1980–2022); and (iii) initialized prediction simulations for the 1981–2023 period, starting every year from initial states obtained from the above assimilation simulations. The assimilations are designed to reconstruct the actual evolution of the Earth system by assimilating essential fields from data products. The assimilations' states, which are expected to be close to observations, are used to start the initialized prediction simulations used for the current-year (2023) global carbon budget. Similar initialized prediction simulations starting every year (1 November or 1 January) over the 1981–2022 period (i.e. hindcasts) are also performed for predictive skill quantification and for bias correction. More details on the illustration of a decadal prediction system based on an ESM can be found in Fig. 1 of Li et al. (2023).
By assimilating physical atmospheric and oceanic data products into the ESMs, the models are able to reproduce the historical variations in the atmosphere–sea CO2 fluxes, atmosphere–land CO2 fluxes, and atmospheric CO2 growth rate (Li et al., 2016, 2019; Lovenduski et al., 2019a, b; Ilyina et al., 2021; Li et al., 2023). Furthermore, the ESM-based predictions have proven their skill in predicting the air–sea CO2 fluxes for up to 6 years and the air–land CO2 fluxes and atmospheric CO2 growth for 2 years (Lovenduski et al., 2019a, b; Ilyina et al., 2021; Li et al., 2023). The reconstructions from the fully coupled model simulations ensure a closed budget within the Earth system, i.e. no budget imbalance term.
Four ESMs, i.e. CanESM5 (Swart et al., 2019; Sospedra-Alfonso et al., 2021), IPSL-CM6A-CO2-LR (Boucher et al., 2020), MIROC-ES2L (Watanabe et al., 2020), and MPI-ESM1-2-LR (Mauritsen et al., 2019; Li et al., 2023), have been used to perform the set of prediction simulations. Each ESM uses a different assimilation method and combination of data products incorporated into the system; more details on the models' configuration can be found in Table 4. The ESMs use external forcings from the Coupled Model Intercomparison Project Phase 6 (CMIP6) historical (1980–2014) plus the Shared Socioeconomic Pathway SSP2-4.5 baseline and CovidMIP 2-year blip scenario (2015–2023) (Eyring et al., 2016; C. D. Jones et al., 2021). The CO2 emissions forcing from 2015–2023 is substituted by GCP-GridFED (v2023.1; Jones et al., 2023) to provide a more realistic forcing. Reconstructions of atmosphere–ocean CO2 fluxes (SOCEAN) and atmosphere–land CO2 fluxes (SLAND−ELUC) for the time period from 1980–2022 are assessed here. Predictions of the atmosphere–ocean CO2 flux, atmosphere–land CO2 flux, and atmospheric CO2 growth for 2023 are calculated based on the predictions at a lead time of 1 year. The predictions are bias-corrected using the 1985–2014 climatology mean of GCB2022 (Friedlingstein et al., 2022b); more details on methods can be found in Boer et al. (2016) and Li et al. (2023). The ensemble size of initialized prediction simulations is 10, and the ensemble mean for each individual model is used here. The ESMs are used here to support the assessment of SOCEAN and net atmosphere–land CO2 flux (SLAND−ELUC) over the 1980–2022 period and to provide an estimate of the 2023 projection of GATM.
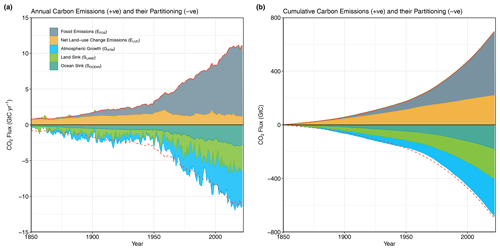
Figure 3Combined components of the global carbon budget as a function of time, for fossil CO2 emissions (EFOS, including a small sink from cement carbonation; grey) and emissions from land-use change (ELUC; brown), as well as their partitioning among the atmosphere (GATM; cyan), ocean (SOCEAN; blue), and land (SLAND; green). Panel (a) shows annual estimates of each flux (in Gt C yr−1) and panel (b) the cumulative flux (the sum of all prior annual fluxes, in Gt C) since the year 1850. The partitioning is based on nearly independent estimates from observations (for GATM) and from process model ensembles constrained by data (for SOCEAN and SLAND) and does not exactly add up to the sum of the emissions, resulting in a budget imbalance (BIM) which is represented by the difference between the bottom red line (mirroring total emissions) and the sum of carbon fluxes in the ocean, land, and atmosphere reservoirs. All data are in gigatonnes of carbon per year (Gt C yr−1) (a) and gigatonnes of carbon (Gt C) (b). The EFOS estimate is based on a mosaic of different data sets, and has an uncertainty of ±5 % (±1σ). The ELUC estimate is from three bookkeeping models (Table 4) with an uncertainty of ±0.7 Gt C yr−1. The GATM estimates prior to 1959 are from Joos and Spahni (2008) with uncertainties equivalent to about ±0.1–0.15 Gt C yr−1 and from Lan et al. (2023) since 1959 with uncertainties of about ±0.07 Gt C yr−1 during 1959–1979 and ±0.02 Gt C yr−1 since 1980. The SOCEAN estimate is the average from Khatiwala et al. (2013) and DeVries (2014) with an uncertainty of about ±30 % prior to 1959 and the average of an ensemble of models and an ensemble of fCO2 products (Table 4) with uncertainties of about ±0.4 Gt C yr−1 since 1959. The SLAND estimate is the average of an ensemble of models (Table 4) with uncertainties of about ±1 Gt C yr−1. See the text for more details of each component and its uncertainties.
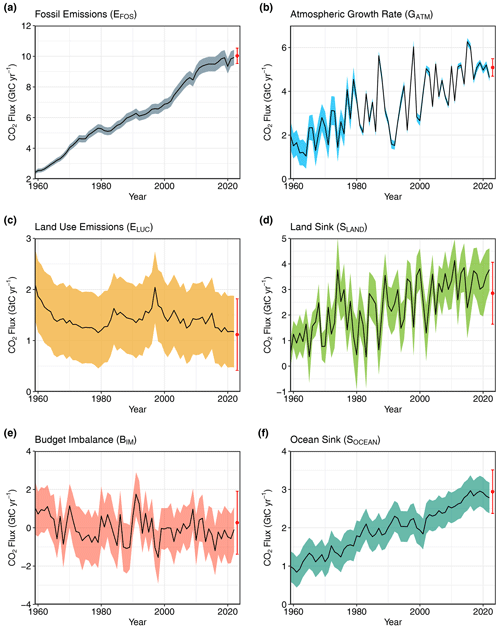
Figure 4Components of the global carbon budget and their uncertainties as a function of time, presented individually for (a) fossil CO2 and cement carbonation emissions (EFOS), (b) the growth rate of atmospheric CO2 concentration (GATM), (c) emissions from land-use change (ELUC), (d) the land CO2 sink (SLAND), (e) the budget imbalance that is not accounted for by the other terms, and (f) the ocean CO2 sink (SOCEAN). Positive values of SLAND and SOCEAN represent a flux from the atmosphere to land or the ocean. All data are in gigatonnes of carbon per year (Gt C yr−1) with the uncertainty bounds representing ±1 standard deviation in shaded colour. Data sources are as in Fig. 3. The red dots indicate our projections for the year 2023 and the red error bars the uncertainty in the projections (see Methods).
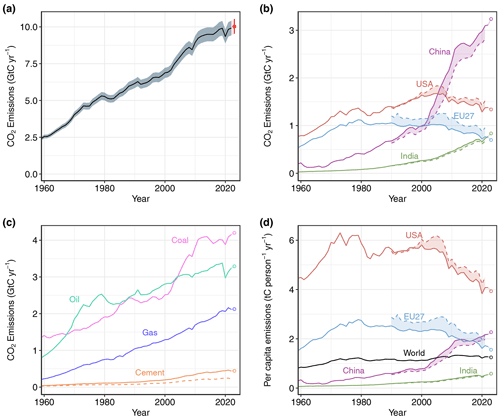
Figure 5(a) Fossil CO2 emissions for the globe, including an uncertainty of ±5 % (grey shading) and a projection through the year 2023 (red dot and uncertainty range); (b) territorial (solid lines) and consumption (dashed lines) emissions for the top three country emitters (USA, China, India) and for the European Union (EU27); (c) global emissions by fuel type, including coal, oil, gas, cement, and cement minus cement carbonation (dashed); (d) per capita emissions for the world and for the large emitters as in panel (b). Territorial emissions are primarily from a draft update of Gilfillan and Marland (2021) except for national data for Annex I countries for 1990–2021, which are reported to the UNFCCC as detailed in the text, as well as some improvements in individual countries, and they are extrapolated forward to 2022 using data from the Energy Institute. Consumption-based emissions are updated from Peters et al. (2011). See Sects. 2.1 and S.1 for details of the calculations and data sources.
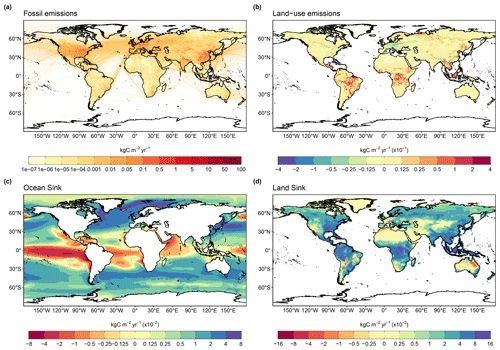
Figure 6The 2013–2022 decadal mean components of the global carbon budget, presented for (a) fossil CO2 emissions (EFOS), (b) land-use change emissions (ELUC), (c) the ocean CO2 sink (SOCEAN), and (d) the land CO2 sink (SLAND). Positive values for EFOS and ELUC represent a flux to the atmosphere, whereas positive values of SOCEAN and SLAND represent a flux from the atmosphere to the ocean or the land (carbon sink). In all panels, yellow and red colours represent a source (flux from the land or ocean to the atmosphere) and green and blue colours represent a sink (flux from the atmosphere into the land or ocean). All units are in kilograms of carbon per square metre per year (kg C m−2 yr−1). Note the different scales in each panel. EFOS data shown are from GCP-GridFEDv2023.1 and do not include cement carbonation. The ELUC map shows the average ELUC from the three bookkeeping models plus emissions from peat drainage and peat fires. Gridded ELUC estimates for H&C2023 and OSCAR are derived by spatially distributing their national data based on the spatial patterns of BLUE gross fluxes in each country (see Schwingshackl et al., 2022, for more details about the methodology). SOCEAN data shown are the average of global ocean biogeochemistry models (GOBMs) and data-product means, using GOBM simulation A, no adjustment for bias and drift applied to the gridded fields (see Sect. 2.5). SLAND data shown are the average of the DGVMs for simulation S2 (see Sect. 2.6).
2.10 Processes not included in the global carbon budget
The contribution of anthropogenic CO and CH4 to the global carbon budget is not fully accounted for in Eq. (1) and is described in Sect. S.6.1. The contributions to CO2 emissions of decomposition of carbonates not accounted for are described in Sect. S.6.2. The contribution of anthropogenic changes in river fluxes is conceptually included in Eq. (1) in SOCEAN and in SLAND, but it is not represented in the process models used to quantify these fluxes. This effect is discussed in Sect. S.6.3. Similarly, the loss of additional sink capacity from reduced forest cover is missing in the combination of approaches used here to estimate both land fluxes (ELUC and SLAND), and its potential effect is discussed and quantified in Sect. S.6.4.
For each component of the global carbon budget, we present results for three different time periods – the full historical period, from 1850 to 2022; the decades in which we have atmospheric concentration records from Mauna Loa (1960–2022); and a specific focus on last year (2022) – and the projection for the current year (2023). Subsequently, we assess the estimates of the budget components of the last few decades against the top-down constraints from inverse modelling of atmospheric observations, the land/ocean partitioning derived from the atmospheric O2 measurements, and the budget component estimates from the ESM assimilation simulations. Atmospheric inversions further allow for an assessment of the budget components with a regional breakdown of land and ocean sinks.
3.1 Fossil CO2 emissions
3.1.1 Historical period 1850–2022
Cumulative fossil CO2 emissions for 1850–2022 were 477±25 Gt C, including the cement carbonation sink (Fig. 3, Table 8, with all cumulative numbers rounded to the nearest 5 Gt C). In this period, 46 % of global fossil CO2 emissions came from coal, 35 % from oil, 15 % from natural gas, 3 % from decomposition of carbonates, and 1 % from flaring. In 1850, the UK contributed 62 % of global fossil CO2 emissions. In 1891 the combined cumulative emissions of the current members of the European Union reached and subsequently surpassed the level of the UK. Since 1917 US cumulative emissions have been the largest. Over the entire period 1850–2022, US cumulative emissions amounted to 115 Gt C (24 % of world total), the EU's to 80 Gt C (17 %), China's to 70 Gt C (15 %), and India's to 15 Gt C (3 %).
In addition to the estimates of fossil CO2 emissions that we provide here (see Methods), there are three global data sets with long time series that include all sources of fossil CO2 emissions: CDIAC-FF (Gilfillan and Marland, 2021), CEDS version v_2021_04_21 (Hoesly et al., 2018; O'Rourke et al., 2021), and PRIMAP-hist version 2.4.2 (Gütschow et al., 2016; Gütschow and Pflüger, 2023), although these data sets are not entirely independent of each other (Andrew, 2020a). CDIAC-FF has the lowest cumulative emissions over 1750–2018 at 440 Gt C; GCP has 444 Gt C, CEDS 445 Gt C, PRIMAP-hist 453 Gt C, and PRIMAP-hist CR 452 Gt C. CDIAC-FF excludes emissions from lime production. CEDS has higher emissions from international shipping in recent years, while PRIMAP-hist has higher fugitive emissions than the other data sets. However, in general these four data sets are in relative agreement as to total historical global emissions of fossil CO2.
3.1.2 Recent period 1960–2022
Global fossil CO2 emissions, EFOS (including the cement carbonation sink), have increased every decade from an average of 3.0±0.2 Gt C yr−1 for the decade of the 1960s to an average of 9.6±0.5 Gt C yr−1 during 2013–2022 (Table 7, Figs. 2 and 5). The growth rate of these emissions decreased between the 1960s and the 1990s, from 4.3 % yr−1 in the 1960s (1960–1969), 3.2 % yr−1 in the 1970s (1970-1979), 1.6 % yr−1 in the 1980s (1980–1989) to 1.0 % yr−1 in the 1990s (1990–1999). After this period, the growth rate began increasing again in the 2000s at an average growth rate of 2.8 % yr−1, decreasing to 0.5 % yr−1 for the last decade (2013–2022). China's emissions increased by +1.6 % yr−1 on average over the last 10 years, dominating the global trend, and India's emissions increased by +3.5 % yr−1, while emissions decreased in EU27 by −1.7 % yr−1 and in the USA by −1.0 % yr−1. Figure 6 illustrates the spatial distribution of fossil fuel emissions for the 2013–2022 period.
EFOS reported here includes the uptake of CO2 by cement via carbonation, which has increased with increasing stocks of cement products, from an average of 18 Mt C yr−1 (0.018 Gt C yr−1) in the 1960s to an average of 197 Mt C yr−1 (0.197 Gt C yr−1) during 2013–2022 (Fig. 5).
3.1.3 Final year 2022
Global fossil CO2 emissions were slightly higher, 0.9 %, in 2022 than in 2021, with an increase of less than 0.1 Gt C to reach 9.9±0.5 Gt C (including the 0.2 Gt C cement carbonation sink) in 2022 (Fig. 5), distributed among coal (41 %), oil (32 %), natural gas (21 %), cement (4 %), flaring (1 %), and others (<1 %). Compared to the previous year, 2022 emissions from coal and oil increased by 1.6 % and 3.2 %, respectively, while emissions from gas and cement decreased by 2.2 % and 5.1 %, respectively. All growth rates presented are adjusted for the leap year, unless stated otherwise.
In 2022, the largest absolute contributions to global fossil CO2 emissions were from China (31 %), the USA (14 %), India (8 %), and the EU27 (7 %). These four regions account for 59 % of global fossil CO2 emissions, while the rest of the world contributed 41 %, including international aviation and marine bunker fuels (2.6 % of the total). Growth rates for these countries from 2021 to 2022 were 0.5 % (China), 0.5 % (USA), −1.6 % (EU27), and 5.8 % (India), with +0.9 % for the rest of the world. The per capita fossil CO2 emissions in 2022 were 1.3 t C per person per year for the globe and were 4.1 (USA), 2.2 (China), 1.7 (EU27), and 0.5 (India) t C per person per year for the four highest emitters (Fig. 5).
3.1.4 Year 2023 projection
Globally, we estimate that global fossil CO2 emissions (including cement carbonation, −0.21 Gt C) will grow by 1.1 % in 2023 (0.0 % to 2.1 %) to 10.0 Gt C (36.8 Gt CO2), exceeding the pre-COVID-19 2019 emission levels of 9.9 Gt C (36.3 Gt CO2). Global increases in 2023 emissions per fuel type are projected to be +1.1 % (range −0.1 % to 2.4 %) for coal, +1.5 % (range 0.6 % to 2.3 %) for oil, +0.5 % (range −0.9 % to 1.8 %) for natural gas, and 0.8 % (range −0.7 % to 2.4 %) for cement.
For China, projected fossil emissions in 2023 are expected to increase by 4 % (range 1.9 % to 6.1 %) compared with 2022 emissions, bringing 2023 emissions for China to around 3.2 Gt C yr−1 (11.9 Gt CO2 yr−1). Changes in fuel specific projections for China are 3.3 % for coal, 9.9 % for oil, 6.5 % for natural gas, and −0.9 % for cement.
For the USA, the Energy Information Administration (EIA) emissions projection for 2023 combined with cement clinker data from USGS gives a decrease of 3.0 % (range −5.0 % to −1.0 %) compared to 2022, bringing 2023 US emissions to around 1.3 Gt C yr−1 (4.9 Gt CO2 yr−1). This is based on separate projections of −18.3 % for coal, −0.3 % for oil, +1.4 % for natural gas, and −4.0 % for cement.
For the European Union, our projection for 2023 is for a decrease of 7.4 % (range −9.9 % to −4.9 %) over 2022, with 2023 emissions at around 0.7 Gt C yr−1 (2.6 Gt CO2 yr−1). This is based on separate projections of −18.8 % for coal, −1.5 % for oil, −6.6 % for natural gas, and −8.7 % for cement.
For India, our projection for 2023 is an increase of 8.2 % (range of 6.7 % to 9.7 %) over 2022, with 2023 emissions at around 0.8 Gt C yr−1 (3.1 Gt CO2 yr−1). This is based on separate projections of +9.5 % for coal, +5.3 % for oil, +5.6 % for natural gas, and +8.8 % for cement.
International aviation and shipping (2.8 % of global emissions) are projected to increase by 11.9 % in 2023, with international aviation projected to be up 28 % on 2022, continuing to recover from pandemic lows, and international shipping projected to rise by 1 %.
For the rest of the world, the expected change for 2023 is a decrease of 0.4 % (range −2.4 % to 1.6 %) with 2023 emissions at around 3.8 Gt C yr−1 (14.0 Gt CO2 yr−1). The fuel-specific projected 2023 growth rates for the rest of the world are as follows: +0.8 % for coal, −2.0 % for oil, 0.0 % for natural gas, +2.4 % for cement.
3.2 Emissions from land-use changes
3.2.1 Historical period 1850–2022
Cumulative CO2 emissions from land-use changes (ELUC) for 1850–2022 were 220±65 Gt C (Table 8; Figs. 3, 15). The cumulative emissions from ELUC show a large spread among individual estimates of 150 Gt C (H&C2023), 290 Gt C (BLUE), and 215 Gt C (OSCAR) for the three bookkeeping models and a similarly wide estimate of 210±65 Gt C for the DGVMs (all cumulative numbers are rounded to the nearest 5 Gt C). These estimates are broadly consistent with indirect constraints from vegetation biomass observations, giving cumulative emissions of 155±50 Gt C over the 1901–2012 period (Li et al., 2017). However, given the large spread, a best estimate is difficult to ascertain.
3.2.2 Recent period 1960–2022
In contrast to growing fossil emissions, CO2 emissions from land use, land-use change, and forestry remained relatively constant over the 1960–1999 period. Since then, they have shown a slight decrease of about 0.1 Gt C per decade, reaching 1.3±0.7 Gt C yr−1 for the 2013–2022 period (Table 7) but with a large spread across estimates (Table 5, Fig. 7). Differently from the bookkeeping average, the DGVM average grows slightly larger over the 1970–2022 period and shows no sign of decreasing emissions in recent decades (Table 5, Fig. 7). This is, however, expected as DGVM-based estimates include the loss of additional sink capacity, which grows with time, while the bookkeeping estimates do not (Sect. S.6.4).
Table 5Comparison of results from the bookkeeping method and budget residuals with results from the DGVMs, as well as additional estimates from atmospheric oxygen, atmospheric inversions, and Earth system models (ESMs) for different periods, the last decade, and the last year available. All values are in gigatonnes of carbon per year (Gt C yr−1). See Fig. 7 for explanation of the bookkeeping component fluxes. The DGVM uncertainties represent ±1σ of the decadal or annual (for 2022) estimates from the individual DGVMs: for the inverse systems the mean and range of available results are given. All values are rounded to the nearest 0.1 Gt C, and therefore columns do not necessarily add to zero.
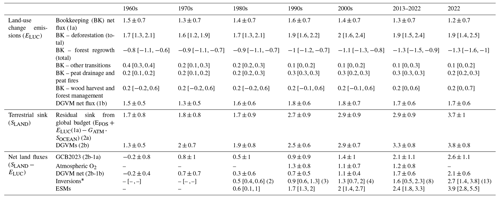
* Estimates are adjusted for the pre-industrial influence of river fluxes and for the cement carbonation sink and are adjusted to a common EFOS (Sect. 2.7). The ranges given include varying numbers (in parentheses) of inversions in each decade (Table S4).
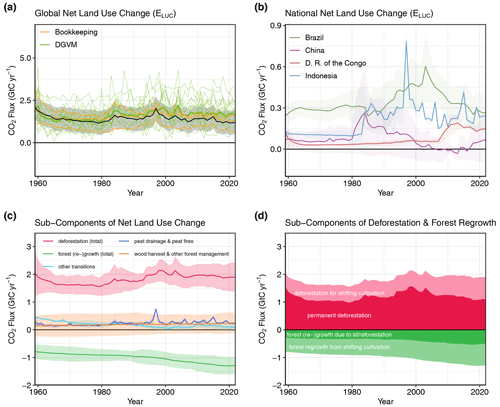
Figure 7Net CO2 exchanges between the atmosphere and the terrestrial biosphere related to land-use change. (a) Net CO2 emissions from land-use change (ELUC) with estimates from the three bookkeeping models (yellow lines) and the budget estimate (black with ±1σ uncertainty), which is the average of the three bookkeeping models. Estimates from individual DGVMs (narrow green lines) and the DGVM ensemble mean (thick green line) are also shown. (b) Net CO2 emissions from land-use change from the four countries with the largest cumulative emissions since 1959. Values shown are the average of the three bookkeeping models, with shaded regions as ±1σ uncertainty. (c) Sub-components of ELUC: (i) emissions from deforestation (including permanent deforestation and deforestation in shifting cultivation cycles), (ii) emissions from peat drainage and peat fires, (iii) removals from forest (re)growth (including forest (re)growth due to afforestation and reforestation and forest regrowth in shifting cultivation cycles), (iv) fluxes from wood harvest and other forest management (comprising slash and product decay following wood harvest, regrowth after wood harvest, and fire suppression), and (v) emissions and removals related to other land-use transitions. The sum of the five components is ELUC shown in panel (a). (d) Sub-components of “deforestation (total)” and of “forest (re)growth (total)”: (i) deforestation in shifting cultivation cycles, (ii) permanent deforestation, (iii) forest (re)growth due to afforestation and/or reforestation, and (iv) forest regrowth in shifting cultivation cycles.
We separate net ELUC into five component fluxes to gain further insight into the drivers of net emissions: deforestation, forest (re)growth, wood harvest and other forest management, peat drainage and peat fires, and all other transitions (Fig. 7c; Sect. S.2.1). We further decompose the deforestation and the forest (re)growth term into contributions from shifting cultivation vs. permanent forest cover changes (Fig. 7d). Averaged over the 2013–2022 period and over the three bookkeeping estimates, fluxes from deforestation amount to 1.9 [1.5, 2.4] Gt C yr−1 (Table 5), of which 1.1 [1.0, 1.2] Gt C yr−1 is from permanent deforestation. Fluxes from forest (re)growth amount to −1.3 [−1.5, −0.9] Gt C yr−1 (Table 5), of which −0.5 [−0.8, −0.2] Gt C yr−1 is from re-/afforestation and the remainder is from forest regrowth in shifting cultivation cycles. Emissions from wood harvest and other forest management (0.2 [0.0, 0.6] Gt C yr−1), emissions from peat drainage and peat fires (0.3 [0.3, 0.3] Gt C yr−1), and the net flux from other transitions (0.1 [0.0, 0.3] Gt C yr−1) are substantially less important globally (Table 5). However, the small net flux from wood harvest and other forest management contains substantial gross fluxes that largely compensate for each other (see Fig. S7): 1.3 [0.9, 2.0] Gt C yr−1 emissions result from the decomposition of slash and the decay of wood products and −1.1 [−1.3, −0.8] Gt C yr−1 removals result from regrowth after wood harvesting. This split into component fluxes clarifies the potential for emissions reduction and carbon dioxide removal: the emissions from permanent deforestation – the largest of our component fluxes – could be halted (largely) without compromising carbon uptake by forests, contributing substantially to emissions reduction. By contrast, reducing wood harvesting would have limited potential to reduce emissions as it would be associated with less forest regrowth; removals and emissions cannot be decoupled here on long timescales. A similar conclusion applies to removals and emissions from shifting cultivation, which we have therefore separated out. Carbon dioxide removal (CDR) in forests could instead be increased by permanently increasing the forest cover through re-/afforestation. Our estimate of about −0.5 [−0.8, −0.2] Gt C yr−1 (of which about two-thirds is located in non-Annex I countries, in particular in China) removed on average each year during 2013–2022 by re-/afforestation is very similar to independent estimates that were derived from NGHGIs for 2022. Re-/afforestation constitutes the vast majority of all current CDR (Powis et al., 2023). Though it cannot be compared directly to annual fluxes from the atmosphere, CDR through transfers between non-atmospheric reservoirs such as in durable HWPs, biochar, or BECCS comprises much smaller amounts of carbon. A total of 61 Mt C yr−1 has been estimated to be transferred to HWPs in 2022, and BECCS projects have been estimated to store 0.5 Mt C yr−1 in geological projects worldwide (Powis et al., 2023). “Blue carbon”, i.e. coastal wetland management such as restoration of mangrove forests, salt marshes, and seagrass meadows, though at the interface of land and ocean carbon fluxes, is counted towards the land-use sector as well. Currently, bookkeeping models do not include blue carbon; however, current CDR deployment in coastal wetlands is small globally, less than 0.003 Mt C yr−1 (Powis et al., 2023).
The small declining trend of ELUC over the last 3 decades is a result of total deforestation emissions showing no clear trend, while forest regrowth has provided steadily increasing removals. Since the processes behind gross removals, foremost forest regrowth and soil recovery, are all slow, while gross emissions include a large instantaneous component, short-term changes in land-use dynamics, such as a temporary decrease in deforestation, influence gross emissions dynamics more than gross removal dynamics, which rather are a response to longer-term dynamics. Component fluxes often differ more than the net flux across the three bookkeeping estimates, which is expected due to different process representation; in particular, treatment of shifting cultivation, which increases both gross emissions and removals, differs across models, but net and gross wood harvest fluxes also show high uncertainty. By contrast, models agree relatively well for emissions from permanent deforestation and removals by re-/afforestation.
Overall, the highest land-use emissions occur in the tropical regions of all three continents. The top three emitters (both cumulatively over 1959–2022 and on average over 2013–2022) are Brazil (in particular the Amazon arc of deforestation), Indonesia, and the Democratic Republic of the Congo, with these three countries contributing 0.7 Gt C yr−1 or 55 % of the global net land-use emissions (average over 2013–2022) (Fig. 6b). This is related to massive expansion of cropland, particularly in the last few decades in Latin America, Southeast Asia, and sub-Saharan Africa (Hong et al., 2021), to a substantial part for export of agricultural products (Pendrill et al., 2019). Emission intensity is high in many tropical countries, particularly in Southeast Asia, due to high rates of land conversion in regions of carbon-dense, and often still pristine, undegraded natural forests (Hong et al., 2021). Emissions are further increased by peat fires in equatorial Asia (GFED4s; van der Werf et al., 2017). Uptake due to land-use change occurs, particularly in Europe, partly related to expanding forest area as a consequence of the forest transition in the 19th and 20th centuries and subsequent regrowth of forest (Fig. 6b) (Mather, 2001; McGrath et al., 2015).
While the mentioned patterns are robust and supported by independent literature, we acknowledge that the model spread is substantially larger on regional than on global levels, as has been shown for bookkeeping models (Bastos et al., 2021) as well as DGVMs (Obermeier et al., 2021). Assessments for individual regions will be performed as part of REgional Carbon Cycle Assessment and Processes (RECCAP-2; Ciais et al., 2022; Poulter et al., 2022) or already exist for selected regions (e.g. for Europe by Petrescu et al., 2020; for Brazil by Rosan et al., 2021; for eight selected countries/regions in comparison to inventory data by Schwingshackl et al., 2022).
As mentioned before, the NGHGI data under the LULUCF sector or data submitted by countries to FAOSTAT differ from the global models' definition of ELUC. In the NGHGI reporting, the natural fluxes (SLAND) are counted towards ELUC when they occur on managed land (Grassi et al., 2018). To compare our results to the NGHGI approach, we perform a translation of our ELUC estimates by subtracting SLAND in managed forest derived from the DGVM simulations (following the methodology described in Grassi et al., 2023) from the bookkeeping ELUC estimate (see Sect. S.2.3). For the 2013–2022 period, we estimate that 2.0 Gt C yr−1 of SLAND occurred in managed forests. Subtracting this value from ELUC changes ELUC from being a source of 1.3 Gt C yr−1 to a sink of 0.8 Gt C yr−1, which is very similar to the NGHGI estimate that yields a sink of 0.7 Gt C yr−1 (Table 9). The translation approach has been shown to be generally applicable also at the country level (Grassi et al., 2023; Schwingshackl et al., 2022). Country-level analysis suggests, for example, that the bookkeeping method estimates higher deforestation emissions than the national report in Indonesia but less CO2 removal by afforestation than the national report in China. The fraction of the natural CO2 sinks that the NGHGI estimates include differs substantially across countries, related to varying proportions of managed vs. total forest areas (Schwingshackl et al., 2022). By comparing ELUC and NGHGI on the basis of the component fluxes used above, we find that our estimates reproduce very closely the NGHGI estimates for emissions from permanent deforestation (1.1 Gt C yr−1 averaged over 2013–2022). Forest fluxes, that is, (re)growth from re-/afforestation plus the net flux from wood harvesting and other forest management, constitute a large sink in the NGHGI (−1.9 Gt C yr−1 averaged over 2013–2022), since they also include SLAND in managed forests. Summing up the bookkeeping estimates of (re)growth from re-/afforestation and the net flux from wood harvesting and other forest management and adding SLAND in managed forests yields a flux of −2.3 Gt C yr−1 (averaged over 2013–2022), which compares well with the NGHGI estimate. Emissions from organic soils in NGHGI are similar to the estimates based on the bookkeeping approach and the external peat drainage and burning data sets. The net flux from other transitions is small in both NGHGI and bookkeeping estimates, but a difference in sign (small source in bookkeeping estimates, small sink in NGHGI) creates a notable difference between NGHGI and bookkeeping estimates. Though estimates between NGHGI, FAOSTAT, and the translated budget estimates still differ in value and need further analysis, the approach suggested by Grassi et al. (2023), which we adopt here, provides a feasible way to relate the global models' and NGHGI approach to each other and thus link the anthropogenic carbon budget estimates of land CO2 fluxes directly to the Global Stocktake, as part of the UNFCCC Paris Agreement.
3.2.3 Final year 2022
The global CO2 emissions from land-use change are estimated as 1.2±0.7 Gt C in 2022, which is similar to the 2020 and 2021 estimates. However, confidence in the annual change remains low. Effects of the COVID-19 pandemic on land-use change have turned out to be country-specific as global market mechanisms, national economics, and changes in household income all could act to curb or enhance deforestation (Wunder et al., 2021). Concerns about enhanced deforestation due to weakened environmental protection and monitoring in tropical countries (Brancalion et al., 2020; Vale et al., 2021) have been confirmed only for some countries (Céspedes et al., 2023). For example, a recent study suggests slightly increased deforestation rates for the Democratic Republic of Congo linked in particular to post-pandemic economic recovery in the mining sector, while deforestation trends in Brazil seem to have been unaffected. Land-use dynamics may be further altered by the Russian invasion of Ukraine, but scientific evidence related to international dependencies (like a shift to tropical palm oil to alleviate dependencies on sunflower oil) has so far been very limited and recent changes will not be reflected by the land-use forcing applied in the global models. High food prices, which preceded but were exacerbated by the war in Ukraine (FAO, 2023), are generally linked to higher deforestation (Angelsen and Kaimowitz, 1999). A new wave of cropland abandonment in the conflict region may increase the substantial eastern European carbon sink due to land-use changes, but sanctions being placed on trade may also incentivize domestic agricultural production and thus lead to the recultivation of abandoned areas in Russia (Winkler et al., 2023).
3.2.4 Year 2023 projection
In Indonesia, peat fire emissions are below average (12 Tg C through 29 September 2023) despite El Niño conditions, which in general lead to more fires. Tropical deforestation and degradation fires in Indonesia are around average (13 Tg C through 29 September 2023) but higher than in the previous year, which had a relatively wet dry season (GFED4.1s; van der Werf et al., 2017; see also https://www.geo.vu.nl/~gwerf/GFED/GFED4/tables/GFED4.1s_C.txt, last access: 9 November 2023). In South America, emissions from tropical deforestation and degradation fires are among the lowest over the last few decades (64 Tg C through 29 September 2023). Effects of the El Niño in the Amazon, such as droughts, are not expected before 2024. Disentangling the degree to which interannual variability in rainfall patterns and stronger environmental protection measures in both Indonesia after their 2015 high-fire-activity season and Brazil after a change in government there play a role in this is an important research topic. Cumulative fire emission estimates through 29 September 2023 are 155 Tg C for global deforestation and degradation fires and 12 Tg C for peatland fires in Indonesia (https://www.geo.vu.nl/~gwerf/GFED/GFED4/tables/GFED4.1s_C.txt, last access: 9 November 2023).
Based on these estimates, we expect ELUC emissions of around 1.1 Gt C (4.1 Gt CO2) in 2023. Our preliminary estimate of ELUC for 2023 is substantially lower than the 2013–2022 average, which saw years of anomalously dry conditions in Indonesia and high-deforestation fires in South America (Friedlingstein et al., 2022b). Note that although our extrapolation includes tropical deforestation and degradation fires, degradation attributable to selective logging, edge effects or fragmentation is not captured. Further, deforestation and fires in deforestation zones may become more disconnected, partly due to changes in legislation in some regions. For example, Van Wees et al. (2021) found that the contribution from fires to forest loss decreased in the Amazon and in Indonesia over the period of 2003–2018.
3.3 CDR not based on vegetation
Besides the CDR through land use (Sect. 3.2), the atmosphere-to-geosphere flux of carbon resulting from carbon dioxide removal (CDR) activity is currently 0.003 Mt C yr−1, with 0.002 Mt C yr−1 of DACCS and 0.001 Mt C yr−1 of enhanced weathering projects. This is more than a million times smaller than current fossil CO2 emissions.
3.4 Total anthropogenic emissions
Cumulative anthropogenic CO2 emissions for 1850–2022 totalled 695±70 Gt C (2550±260 Gt CO2), of which 70 % (485 Gt C) has occurred since 1960 and 33 % (235 Gt C) since 2000 (Tables 7 and 8). Total anthropogenic emissions more than doubled over the last 60 years, from 4.6±0.7 Gt C yr−1 for the decade of the 1960s to an average of 10.9±0.8 Gt C yr−1 during 2013–2022, and reached 11.1±0.9 Gt C (40.7±3.3 Gt CO2) in 2022. For 2023, we project global total anthropogenic CO2 emissions from fossil and land-use changes to also be around 11.2 Gt C (40.9 Gt CO2). All values here include the cement carbonation sink (currently about 0.2 Gt C yr−1).
During the historical period 1850–2022, 31 % of historical emissions were from land-use change and 69 % from fossil emissions. However, fossil emissions have grown significantly since 1960 while land-use changes have not, and consequently the contributions of land-use change to total anthropogenic emissions were smaller during recent periods (18 % during the period 1960–2022 and down to 12 % over the 2013–2022 period).
3.5 Atmospheric CO2
3.5.1 Historical period 1850–2022
Atmospheric CO2 concentration was approximately 278 parts per million (ppm) in 1750, reaching 300 ppm in the 1910s, 350 ppm in the late 1980s, and 417.07±0.1 ppm in 2022 (Lan et al., 2023; Fig. 1). The mass of carbon in the atmosphere increased by 48 % from 590 Gt C in 1750 to 886 Gt C in 2022. Current CO2 concentrations in the atmosphere are unprecedented for the last 2 million years, and the current rate of atmospheric CO2 increase is at least 10 times faster than at any other time during the last 800 000 years (Canadell et al., 2021).
Table 6Comparison of results for the ocean sink from the fCO2 products, global ocean biogeochemistry models (GOBMs), and the best estimate for GCB2023 as calculated from fCO2 products and GOBMs that is used in the budget (Table 7), as well as additional estimates from atmospheric oxygen, atmospheric inversions, and Earth system models (ESMs) for different periods, the last decade, and the last year available. All values are in gigatonnes of carbon per year (Gt C yr−1). Uncertainties represent ±1σ of the estimates from the GOBMs (N>10), and the range of ensemble members is given for ensembles with N<10 (fCO2 products, inversions, ESMs). The ranges given include varying numbers (in parentheses) of inversions in each decade (Table S4). The uncertainty in the GCB2023 estimate is based on expert judgement (Sects. 2 and S1 to S4), and for oxygen it is the standard deviation of a Monte Carlo ensemble (Sect. 2.8).

3.5.2 Recent period 1960–2022
The growth rate of the atmospheric CO2 level increased from 1.7±0.07 Gt C yr−1 in the 1960s to 5.2±0.02 Gt C yr−1 during 2013–2022 with important decadal variations (Table 7, Figs. 3 and 4). During the last decade (2013–2022), the growth rate of atmospheric CO2 concentration continued to increase, albeit with large interannual variability (Fig. 4).
The airborne fraction (AF), defined as the ratio of atmospheric CO2 growth rate to total anthropogenic emissions,
provides a diagnostic of the relative strength of the land and ocean carbon sinks in removing part of the anthropogenic CO2 perturbation. The evolution of AF over the last 60 years shows no significant trend, remaining at around 44 %, albeit showing a large interannual and decadal variability driven by the year-to-year variability in GATM (Fig. 9). The observed stability of the airborne fraction over the 1960–2020 period indicates that the ocean and land CO2 sinks have been removing on average about 56 % of the anthropogenic emissions (see Sect. 3.6.2 and 3.7.2).
3.5.3 Final year 2022
The growth rate of atmospheric CO2 concentration was 4.6±0.2 Gt C (2.18±0.08 ppm) in 2022 (Fig. 4; Lan et al., 2023), below the 2021 growth rate (5.2±0.2 Gt C) and the 2013–2022 average (5.2±0.02 Gt C).
3.5.4 Year 2023 projection
The 2023 growth in atmospheric CO2 concentration (GATM) is projected to be about 5.1 Gt C (2.4 ppm). This is the average of the GCB regression method (5.07 Gt C, 2.39 ppm) and ESM multi-model mean (5.11 Gt C, 2.41 ppm). The 2023 atmospheric CO2 concentration, averaged over the year, is expected to reach the level of 419.3 ppm, 51 % above the pre-industrial level.
Table 7Decadal mean in the five components of the anthropogenic CO2 budget for different periods and the last year available. All values are in gigatonnes of carbon per year (Gt C yr−1), and uncertainties are reported as ±1σ. Fossil CO2 emissions include cement carbonation. The table also shows the budget imbalance (BIM), which provides a measure of the discrepancies among the nearly independent estimates. A positive imbalance means the emissions are overestimated and/or the sinks are too small. All values are rounded to the nearest 0.1 Gt C and therefore columns do not necessarily add to zero.
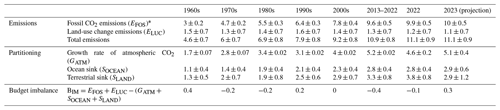
∗ Fossil emissions excluding the cement carbonation sink amount to 3±0.2, 4.7±0.2, 5.5±0.3, 6.4±0.3, 7.9±0.4, and 9.8±0.5 Gt C yr−1 for the decades of the 1960s to 2010s, respectively; 10.1±0.5 Gt C yr−1 for 2022; and 10.2±0.5 Gt C yr−1 for 2023.
3.6 Ocean sink
3.6.1 Historical period 1850–2022
Cumulated since 1850, the ocean sink adds up to 180±35 Gt C, with more than two-thirds of this amount (125 Gt C) having been taken up by the global ocean since 1960. Over the historical period, the ocean sink increased in pace with the anthropogenic emissions' exponential increase (Fig. 3). Since 1850, the ocean has removed 26 % of total anthropogenic emissions.
3.6.2 Recent period 1960–2022
The ocean CO2 sink increased from 1.1±0.4 Gt C yr−1 in the 1960s to 2.8±0.4 Gt C yr−1 during 2013–2022 (Table 7), with interannual variations of the order of a few tenths of gigatonnes per carbon per year (Gt C yr−1) (Fig. 10). The ocean-borne fraction () has been remarkably constant of around 25 % on average (Fig. 9c), with variations around this mean illustrating the decadal variability in the ocean carbon sink. So far, there has been no indication of a decrease in the ocean-borne fraction from 1960 to 2022. The increase in the ocean sink is primarily driven by the increased atmospheric CO2 concentration, with the strongest CO2-induced signal in the North Atlantic and the Southern Ocean (Fig. 11a). The effect of climate change is much weaker, reducing the ocean sink globally by 0.16±0.04 Gt C yr−1 (−6.7 % of SOCEAN) during 2013–2022 (all models simulate a weakening of the ocean sink by climate change, range −4.3 % to −10.3 %), and does not show clear spatial patterns across the GOBM ensemble (Fig. 11b). This is the combined effect of change and variability in all atmospheric forcing fields, previously attributed, in one model, to wind and temperature changes (Le Quéré et al., 2010).
The global net air–sea CO2 flux is a residual of large natural and anthropogenic CO2 fluxes into and out of the ocean with distinct regional and seasonal variations (Figs. 6 and B1). Natural fluxes dominate on regional scales but largely cancel each other out when integrated globally (Gruber et al., 2009). Mid-latitudes in all basins and the high-latitude North Atlantic dominate the ocean CO2 uptake where low temperatures and high wind speeds facilitate CO2 uptake at the surface (Takahashi et al., 2009). In these regions, the formation of mode, intermediate, and deep water masses transports anthropogenic carbon into the ocean interior, thus allowing for continued CO2 uptake at the surface. Outgassing of natural CO2 occurs mostly in the tropics, especially in the equatorial upwelling region, and to a lesser extent in the North Pacific and polar Southern Ocean, mirroring a well-established understanding of regional patterns of air–sea CO2 exchange (e.g. Takahashi et al., 2009; Gruber et al., 2009). These patterns are also noticeable in the Surface Ocean CO2 Atlas (SOCAT) data set, where an ocean fCO2 value above the atmospheric level indicates outgassing (Fig. S1). This map further illustrates the data sparsity in the Indian Ocean and the Southern Hemisphere in general.
Interannual variability in the ocean carbon sink is driven by climate variability with a first-order effect from a stronger ocean sink during large El Niño events (e.g. 1997–1998) (Fig. 10; Rödenbeck et al., 2014; Hauck et al., 2020; McKinley et al., 2017). The GOBMs show the same patterns of decadal variability as the mean of the fCO2 products, with a stagnation of the ocean sink in the 1990s and a strengthening since the early 2000s (Fig. 10; Le Quéré et al., 2007; Landschützer et al., 2015, 2016; DeVries et al., 2017; Hauck et al., 2020; McKinley et al., 2020; Gruber et al., 2023). Different explanations have been proposed for this decadal variability, ranging from the ocean's response to changes in atmospheric wind and pressure systems (e.g. Le Quéré et al., 2007; Keppler and Landschützer, 2019), including variations in upper-ocean overturning circulation (DeVries et al., 2017), to the eruption of Mount Pinatubo and its effects on sea surface temperature and a slowed atmospheric CO2 growth rate in the 1990s (McKinley et al., 2020). The main origin of the decadal variability is a matter of debate, with a number of studies initially pointing to the Southern Ocean (see review in Canadell et al., 2021), but contributions from the North Atlantic and North Pacific (Landschützer et al., 2016; DeVries et al., 2019) or a global signal (McKinley et al., 2020) were also proposed.
Although all individual GOBMs and fCO2 products fall within the observational constraint, the ensemble means of GOBMs and fCO2 products adjusted for the riverine flux diverge over time, with a mean offset increasing from 0.30 Gt C yr−1 in the 1990s to 0.57 Gt C yr−1 in the decade 2013–2022 and reaching 0.61 Gt C yr−1 in 2022. The SOCEAN positive trend over time has diverged by a factor of 2 since 2002 (GOBMs: 0.24±0.07 Gt C yr−1 per decade, fCO2 products: 0.48±0.11 Gt C yr−1 per decade; SOCEAN: 0.36 Gt C yr−1 per decade) and by a factor of 2.5 since 2010 (GOBMs: 0.16±0.15 Gt C yr−1 per decade; fCO2 products: 0.42±0.18 Gt C yr−1 per decade; SOCEAN: 0.29 Gt C yr−1 per decade). The fCO2-product estimate is slightly different compared to Friedlingstein et al. (2022b) as a result of an updated submission of the NIES-ML3 product (previously NIES-NN); however the difference in the integrated mean flux is small.
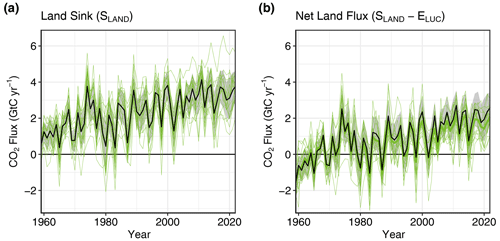
Figure 8(a) The land CO2 sink (SLAND) estimated by individual DGVMs (green), as well as the budget estimate (black with ±1σ uncertainty), which is the average of all DGVMs. (b) Net atmosphere–land CO2 fluxes (SLAND−ELUC). The budget estimate of the net land flux (black with ±1σ uncertainty) combines the DGVM estimate of SLAND from panel (a) with the bookkeeping estimate of ELUC from Fig. 7a. Uncertainties are similarly propagated in quadrature. DGVMs also provide estimates of ELUC (see Fig. 7a), which can be combined with their own estimates of the land sink. Hence panel (b) also includes an estimate for the net land flux for individual DGVMs (thin green lines) and their multi-model mean (thick green line).
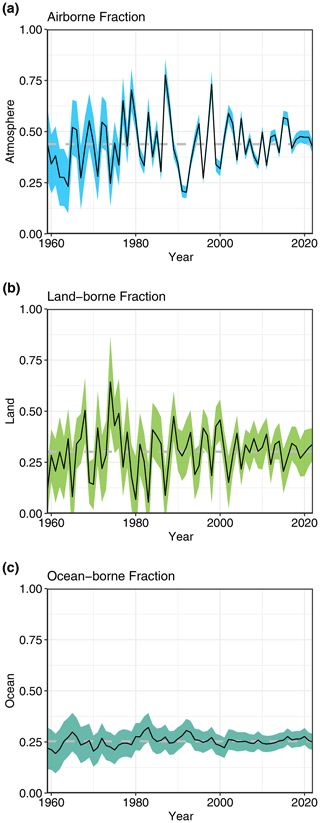
Figure 9The partitioning of total anthropogenic CO2 emissions (EFOS+ELUC) across the (a) atmosphere (airborne fraction), (b) land (land-borne fraction), and (c) ocean (ocean-borne fraction). Black lines represent the central estimate, and the coloured shading represents the uncertainty. The dashed grey lines represent the long-term average of the airborne (44 %), land-borne (30 %), and ocean-borne (25 %) fractions during 1960–2022 (with a BIM of 1 %).
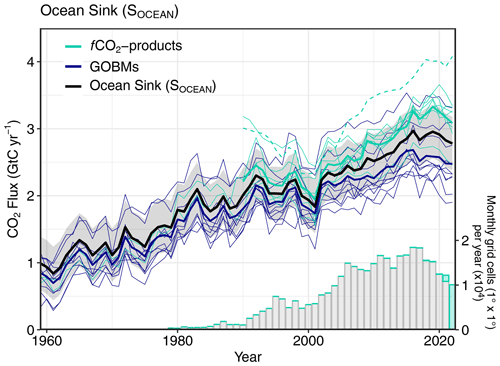
Figure 10Comparison of the anthropogenic atmosphere–ocean CO2 flux showing the budget values of SOCEAN (black, with the uncertainty in grey shading), individual ocean models (royal blue), and the ocean fCO2 products (cyan, with Watson et al., 2020, represented by a dashed line as not used for ensemble mean). Only one fCO2 product (Jena-MLS) extends back to 1959 (Rödenbeck et al., 2022). The fCO2 products were adjusted for the pre-industrial ocean source of CO2 from river input to the ocean by subtracting a source of 0.65 Gt C yr−1 to make them comparable to SOCEAN (see Sect. 2.5). The bar plot on the lower right illustrates the number of fCO2 observations in the SOCATv2023 database (Bakker et al., 2023). Grey bars indicate the number of data points in SOCATv2022 and coloured bars the newly added observations in v2023.
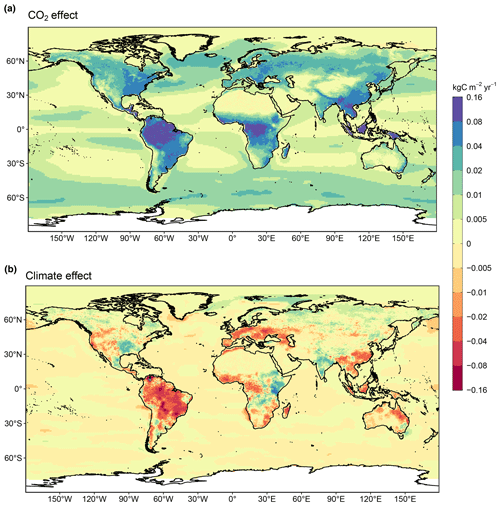
Figure 11Attribution of the atmosphere–ocean (SOCEAN) and atmosphere–land (SLAND) CO2 fluxes to (a) increasing atmospheric CO2 concentrations and (b) changes in climate, averaged over the previous decade 2013–2022. All data shown are from the processed-based GOBMs and DGVMs. Note that the sum of ocean CO2 and climate effects shown here will not equal the ocean sink shown in Fig. 6, which includes the fCO2 products. See Sects. S.3.2 and S.4.1 for attribution methodology. Units are in kilograms of carbon per square metre per year (kg C m−2 yr−1) (note the non-linear colour scale).
The discrepancy between the two types of estimates stems from a larger SOCEAN trend in the northern and southern extra-tropics since around 2002 (Fig. 13). Note that the discrepancy in the mean flux, which was located in the Southern Ocean in previous versions of the GCB, has been reduced due to the choice of the regional river flux adjustment (Lacroix et al., 2020, instead of Aumont et al., 2001). This comes at the expense of a new discrepancy in the mean SOCEAN of about 0.2 Gt C yr−1 in the tropics. Likely explanations for the discrepancy in the trends in the high latitudes are data sparsity and uneven data distribution (Bushinsky et al., 2019; Gloege et al., 2021; Hauck et al., 2023). In particular, two fCO2 products that are part of the GCB ensemble were shown to overestimate the Southern Ocean CO2 flux trend by 50 % and 130 % based on current sampling in a model subsampling experiment (Hauck et al., 2023). Another likely contributor to the discrepancy between GOBMs and fCO2 products comprises model biases (as indicated by the large model spread in the south, Fig. 13, and the larger model–data fCO2 mismatch, Fig. S2).
In previous GCB releases, the ocean sink 1959–1989 was only estimated by GOBMs due to the absence of fCO2 observations. Now, the first data-based estimates extending back to 1957–1958 are becoming available (Jena-MLS, Rödenbeck et al., 2022; LDEO-HPD, Bennington et al., 2022; Gloege et al., 2022). These are based on a multi-linear regression of pCO2 with environmental predictors (Rödenbeck et al., 2022) or on model–data pCO2 misfits and their relation to environmental predictors (Bennington et al., 2022). The Jena-MLS and LDEO-HPD estimates fall well within the range of GOBM estimates and have a correlation of 0.99 and 0.98, respectively, with SOCEAN for the period 1959–2022 (and 0.98 and 0.97 for the 1959–1989 period). They agree well on the mean SOCEAN estimate since 1977 with a slightly higher amplitude of variability (Fig. 10). Until 1976, Jena-MLS and LDEO-HPD are about 0.25 Gt C yr−1 and about 0.1 Gt C yr−1, respectively, below the central SOCEAN estimate. The agreement especially on phasing of variability is impressive in both products, and the discrepancies in the mean flux 1959–1976 could be explained by an overestimated trend of Jena-MLS (Rödenbeck et al., 2022). Bennington et al. (2022) report a larger flux into the pre-1990 ocean than in Jena-MLS, although it is lower than SOCEAN.
Table 8Cumulative CO2 for different time periods in gigatonnes of carbon (Gt C). Fossil CO2 emissions include cement carbonation. The budget imbalance (BIM) provides a measure of the discrepancies among the nearly independent estimates. All values are rounded to the nearest 5 Gt C, and therefore columns do not necessarily add to zero. Uncertainties are reported as follows: EFOS is 5 % of cumulative emissions, ELUC prior to 1959 is 1σ spread from the DGVMs and ELUC post-1959 is 0.7 times the number of years (where 0.7 Gt C yr−1 is the uncertainty in the annual ELUC flux estimate), GATM uncertainty is held constant at 5 Gt C for all time periods, SOCEAN uncertainty is 20 % of the cumulative sink (20 % relates to the annual uncertainty of 0.4 Gt C yr−1, which is ∼20 % of the current ocean sink), and SLAND is the 1σ spread from the DGVM estimates.
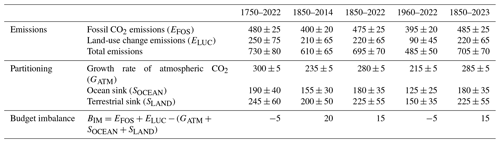
Table 9Translation of global carbon cycle models' land flux definitions to the definition of the LULUCF net flux used in national greenhouse gas inventories reported to the UNFCCC. See Sect. S2.3 and Table S9 for detail on methodology and comparison to other data sets. Units are gigatonnes of carbon per year (Gt C yr−1).
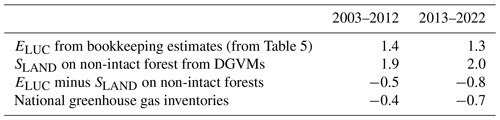
The reported SOCEAN estimate from GOBMs and fCO2 products is 2.2±0.4 Gt C yr−1 over the period 1994 to 2007, which is in excellent agreement with the ocean interior estimate of 2.2±0.4 Gt C yr−1 and accounts for the climate effect on the natural CO2 flux of Gt C yr−1 (Gruber et al., 2019) to match the definition of SOCEAN used here (Hauck et al., 2020). This comparison depends critically on the estimate of the climate effect on the natural CO2 flux, which is smaller from the GOBMs (−0.1 Gt C yr−1) than in Gruber et al. (2019). Uncertainties in these two estimates would also overlap when using the GOBM estimate of the climate effect on the natural CO2 flux.
During 2010–2016, the ocean CO2 sink appears to have intensified in line with the expected increase from atmospheric CO2 (McKinley et al., 2020). This effect is slightly stronger in the fCO2 products (Fig. 10, ocean sink 2016 minus 2010; GOBMs: Gt C yr−1; fCO2 products: Gt C yr−1). The reduction of −0.14 Gt C yr−1 (range: −0.39 to +0.01 Gt C yr−1) in the ocean CO2 sink in 2017 is consistent with the return to normal conditions after the El Niño in 2015–2016, which caused an enhanced sink in previous years. After an increasing SOCEAN in 2018 and 2019, the GOBM and fCO2-product ensemble means suggest a decrease in SOCEAN, related to the triple La Niña event of 2020–2023.
3.6.3 Final year 2022
The estimated ocean CO2 sink is 2.8±0.4 Gt C for 2022. This is a small decrease of 0.05 Gt C compared to 2021, in line with the expected sink weakening from persistent La Niña conditions. GOBM and fCO2-product estimates consistently result in a near stagnation of SOCEAN (GOBMs: Gt C; fCO2 products: Gt C). Four models and six fCO2 products show a decrease in SOCEAN (GOBMs down to −0.09 Gt C, fCO2 products down to −0.25 Gt C), while one model shows no change and five models and two fCO2 products show an increase in SOCEAN (GOBMs up to 0.07 Gt C, fCO2 products up to 0.15 Gt C; Fig. 10). The fCO2 products have a larger uncertainty at the end of the reconstructed time series (tail effect, e.g. Watson et al., 2020). Specifically, the fCO2 products' estimate of the last year is regularly adjusted in the following release owing to the tail effect and incrementally increasing data availability. While the monthly grid cells covered may have a lag of only about a year (Fig. 10 inset), the values within grid cells may change with a 1- to 5-year lag (see absolute number of observations plotted in previous GCB releases).
3.6.4 Year 2023 projection
Using a feed-forward neural network method (see Sect. 2.5.2), we project an ocean sink of 2.9 Gt C for 2023. This is slightly higher than for the year 2022 (2.8 Gt C) and could mark a reversal of the slight decrease in the SOCEAN sink since 2019, due to the transition from persisting La Niña conditions to emerging El Niño conditions in 2023. The new set of ESM predictions support this estimate with a 2023 ocean sink of around 3.1 [2.9, 3.2] Gt C.
3.6.5 Ocean models' evaluation
The process-based model evaluation draws a generally positive picture with GOBMs scattered around the observational values for Southern Ocean sea surface salinity, the Southern Ocean stratification index, and the surface ocean Revelle factor (Sect. S3.3 and Table S10). However, the Atlantic Meridional Overturning Circulation at 26∘ N is underestimated by 8 out of 10 GOBMs. It is planned to derive skill scores for the GOBMs in future releases based on these metrics.
The model simulations allow us to separate the anthropogenic carbon component (steady state and non-steady state, sim D − sim A) and to compare the model flux and dissolved inorganic carbon (DIC) inventory change directly to the interior ocean estimate of Gruber et al. (2019) without further assumptions (Table S10). The GOBM ensemble average of anthropogenic carbon inventory changes over 1994–2007 amounts to 2.4 Gt C yr−1 and is thus lower than the 2.6±0.3 Gt C yr−1 estimated by Gruber et al. (2019) although within the uncertainty. Only four models with the highest sink estimate fall within the range reported by Gruber et al. (2019). This suggests that the majority of the GOBMs underestimate anthropogenic carbon uptake by 10 %–20 %. Analysis of Earth system models indicates that an underestimation by about 10 % may be due to biases in ocean carbon transport and mixing from the surface mixed layer to the ocean interior (Goris et al., 2018; Terhaar et al., 2021; Bourgeois et al., 2022; Terhaar et al., 2022), biases in the chemical buffer capacity (Revelle factor) of the ocean (Vaittinada Ayar et al., 2022; Terhaar et al., 2022), and partly a late starting date of the simulations (mirrored in atmospheric CO2 chosen for the pre-industrial control simulation, Table S2; Bronselaer et al., 2017; Terhaar et al., 2022). Interestingly, and in contrast to the uncertainties in the surface CO2 flux, we find the largest mismatch in interior ocean carbon accumulation in the tropics (96 % of the mismatch), with minor contributions from the north (3 %) and the south (<1 %). These numbers deviate slightly from GCB2021 because of submission of the ACCESS model with a high anthropogenic carbon accumulation, particularly in the Southern Ocean. The large discrepancy in accumulation in the tropics highlights the role of interior ocean carbon redistribution for those inventories (Khatiwala et al., 2009; DeVries et al., 2023).
The evaluation of the ocean estimates with the fCO2 observations from the SOCATv2023 data set for the period 1990–2022 shows an RMSE from annually detrended data of 0.4 to 2.4 µatm for the seven fCO2 products over the globe (Fig. S2). The GOBM RMSEs are larger and range from 2.9 to 5.4 µatm. The RMSEs are generally larger at high latitudes compared to the tropics, for both the fCO2 products and the GOBMs. The fCO2 products have RMSEs of 0.3 to 2.8 µatm in the tropics, 0.7 to 2.3 µatm in the north, and 0.7 to 2.8 µatm in the south. Note that the fCO2 products are based on the SOCATv2023 database; hence the SOCAT is not an independent data set for the evaluation of the fCO2 products. The GOBM RMSEs are more spread across regions, ranging from 2.5 to 5.0 µatm in the tropics, 3.0 to 7.2 µatm in the north, and 3.7 to 8.5 µatm in the south. The higher RMSEs occur in regions with stronger climate variability, such as the northern and southern high latitudes (poleward of the subtropical gyres). The upper range of the model RMSEs have increased somewhat relative to Friedlingstein et al. (2022b).
3.7 Land sink
3.7.1 Historical period 1850–2022
Cumulated since 1850, the terrestrial CO2 sink amounts to 225±55 Gt C, 32 % of total anthropogenic emissions. Over the historical period, the sink increased in pace with the anthropogenic emissions' exponential increase (Fig. 3).
3.7.2 Recent period 1960–2022
The terrestrial CO2 sink SLAND increased from 1.3±0.5 Gt C yr−1 in the 1960s to 3.3±0.8 Gt C yr−1 during 2013–2022, with important interannual variations of up to 2 Gt C yr−1 generally showing a decreased land sink during El Niño events (Fig. 8), responsible for the corresponding enhanced growth rate of atmospheric CO2 concentration. The larger land CO2 sink during 2013–2022 compared to the 1960s is reproduced by all the DGVMs in response to the increase in both atmospheric CO2 and nitrogen deposition and to the changes in climate, and it is consistent with constraints from the other budget terms (Table 5).
Over the period 1960 to the present, the increase in the global terrestrial CO2 sink is largely attributed to the CO2 fertilization effect (Prentice et al., 2001; Piao et al., 2009; Schimel et al., 2015) and increased nitrogen deposition (Huntzinger et al., 2017; O'Sullivan et al., 2019), directly stimulating plant photosynthesis and increased plant water use in water-limited systems, with a small negative contribution of climate change (Fig. 11). There is a range of evidence to support a positive terrestrial carbon sink in response to increasing atmospheric CO2, albeit with uncertain magnitude (Walker et al., 2021). As expected from theory, the greatest CO2 effect is simulated in the tropical forest regions, associated with warm temperatures and long growing seasons (Hickler et al., 2008) (Fig. 11a). However, evidence from tropical intact-forest plots indicates an overall decline in the land sink across Amazonia (1985–2011), attributed to enhanced mortality offsetting productivity gains (Brienen et al., 2015; Hubau et al., 2020). During 2013-2022 the land sink is positive in all regions (Fig. 6) with the exception of eastern Brazil, Bolivia, Paraguay, northern Venezuela, the southwest USA, central Europe and central Asia, northern and southern Africa, and eastern Australia, where the negative effects of climate variability and change (i.e. reduced rainfall and/or increased temperature) counterbalance CO2 effects. This is clearly visible in Fig. 11, where the effects of CO2 (Fig. 11a) and climate (Fig. 11b) as simulated by the DGVMs are isolated. The negative effect of climate is the strongest in most of South America, Central America, the southwest USA, central Europe, the western Sahel, southern Africa, Southeast Asia and southern China, and eastern Australia (Fig. 11b). Globally, over the 2013–2022 period, climate change reduces the land sink by 0.68±0.62 Gt C yr−1 (20 % of SLAND).
Most DGVMs have similar SLAND values averaged over 2013–2022, and 14 out of 20 models fall within the 1σ range of the residual land sink [2.0–3.8 Gt C yr−1] (see Table 5), and all but one model are within the 2σ range [1.1–4.7 Gt C yr−1]. The ED model is an outlier, with a land sink estimate of 5.7 Gt C yr−1, driven by a strong CO2 fertilization effect (6.6 Gt C yr−1 in the CO2-only (S1 simulations; see Sect. S4.1) that is offset by correspondingly high land-use emissions. There are no direct global observations of the land sink or the CO2 fertilization effect, and so we are not yet in a position to rule out models based on component fluxes if the net land sink (SLAND−ELUC) is within the observational uncertainty provided by atmospheric O2 measurements (Table 5). Overall, therefore, the spread among models for the estimate of SLAND over the last decade has increased this year (0.8 Gt C yr−1) compared to GCB2022 (0.6 Gt C yr−1).
Furthermore, DGVMs were compared against a data-constrained intermediate-complexity model of the land carbon cycle (CARDAMOM) (Bloom and Williams, 2015; Bloom et al., 2016). Results suggest good correspondence between approaches at the interannual timescales but divergence in the recent trend with CARDAMOM, simulating a stronger trend than the DGVMs (Fig. S8).
Since 2020 the globe has experienced La Niña conditions, which would be expected to lead to an increased land carbon sink. A clear peak in the global land sink is not evident in SLAND, and we find that a La Niña-driven increase in the tropical land sink is offset by a reduced high-latitude extra-tropical land sink, which may be linked to the land response to recent climate extremes. A notable difference from GCB2022 (2012–2021 SLAND mean) is the reduced carbon losses across tropical drylands. Further, central Europe has switched from a sink of carbon to a source, with the summer heatwave of 2022 (and associated drought and wildfire) causing widespread losses (van der Woude et al., 2023). In the past years several regions experienced record-setting fire events. While the global burned area has declined over the past decades mostly due to declining fire activity in savannas (Andela et al., 2017), forest fire emissions are rising and have the potential to counter the negative fire trend in savannas (Zheng et al., 2021). Noteworthy events include the 2019–2020 Black Summer event in Australia (emissions of roughly 0.2 Gt C; van der Velde et al., 2021) and fires in Siberia in 2021 when emissions approached 0.4 Gt C or 3 times the 1997–2020 average according to GFED4s. While other regions, including the western USA and Mediterranean Europe, also experienced intense fire seasons in 2021, their emissions are substantially lower.
Despite these regional negative effects of climate change on SLAND, the efficiency of land to remove anthropogenic CO2 emissions has remained broadly constant over the last 6 decades, with a land-borne fraction () of around 30 % (Fig. 9b).
3.7.3 Final year 2022
The terrestrial CO2 sink from the DGVM ensemble was 3.8±0.8 Gt C in 2022, above the decadal average of 3.3±0.8 Gt C yr−1 (Fig. 4, Table 7) and slightly above the 2021 sink of 3.5±1.0 Gt C, likely driven by the persistent La Niña conditions. We note that the DGVM estimate for 2022 is similar to the 3.7±1.0 Gt C yr−1 estimate from the residual sink from the global budget () (Table 5).
3.7.4 Year 2023 projection
Using a feed-forward neural network method, we project a land sink of 2.9 Gt C for 2023, 0.9 Gt C smaller than the 2022 estimate. As for the ocean sink, we attribute this to the emerging El Niño conditions in 2023, leading to a reduced land sink. The ESMs do not provide an additional estimate of SLAND as they only simulate the net atmosphere–land carbon flux (SLAND−ELUC).
3.7.5 Land models' evaluation
The evaluation of the DGVMs shows generally high skill scores across models for runoff and to a lesser extent for vegetation biomass, gross primary production (GPP), and ecosystem respiration. These conclusions are supported by a more comprehensive analysis of DGVM performance in comparison with benchmark data (Seiler et al., 2022). A relative comparison of DGVM performance (Fig. S3) suggests several DGVMs (CABLE-POP, CLASSIC, OCN, ORCHIDEE) may outperform others in terms of multiple carbon and water cycle benchmarks. However, results from Seiler et al. (2022) also show how DGVM differences are often of similar magnitude compared with the range across observational data sets. All models score high enough over the metrics tests to support their use here. There are a few anomalously low scores for individual metrics from a single model, and these can direct the effort to improve models for use in future budgets.
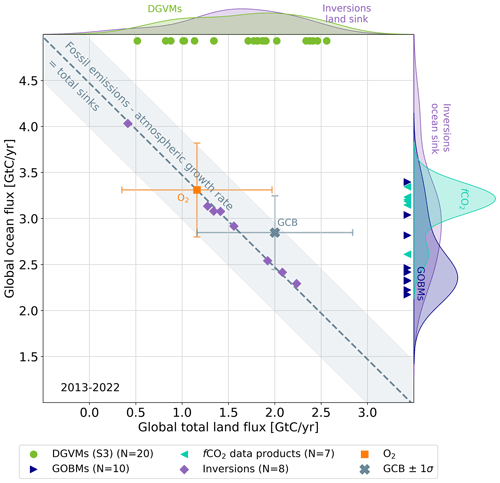
Figure 12The 2013–2022 decadal mean global net atmosphere–ocean and atmosphere–land fluxes derived from the ocean models and fCO2 products (y axis, right- and left-pointing blue triangles, respectively) and from the DGVMs (x axis, green symbols) and the same fluxes estimated from the atmospheric inversions (purple symbols). The shaded distributions show the densities of the ensembles of individual estimates. The grey central cross is the mean (±1σ) of SOCEAN and (SLAND−ELUC) as assessed in this budget. The grey diagonal line represents the constraint on the global land + ocean net flux, i.e. global fossil fuel emissions minus the atmospheric growth rate from this budget (EFOS−GATM). The orange square represents the same global net atmosphere–ocean and atmosphere–land fluxes as estimated from the atmospheric O2 constraint (±1σ for each of the fluxes although caution is needed to interpret these error bars, since solutions outside the grey band are unlikely, being outside of the 1σ uncertainty). Positive values are CO2 sinks. Note that the inverse estimates have been scaled for a minor difference between EFOS and GridFEDv2023.1 (Jones et al., 2023).
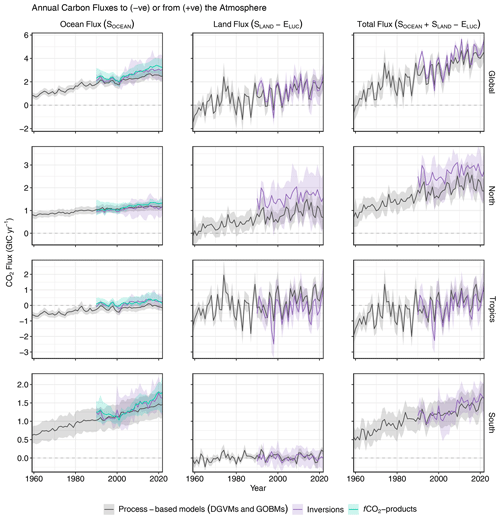
Figure 13CO2 fluxes between the atmosphere and the Earth's surface separated between land and oceans, globally and in three latitude bands. The ocean flux is SOCEAN, and the land flux is the net atmosphere–land fluxes from the DGVMs. The latitude bands are (top row) global, (second row) north (>30∘ N), (third row) tropics (30∘ S–30∘ N), and (bottom row) south (<30∘ S). The fluxes are over ocean (left column), over land (middle column), and total (right column). Estimates are shown for the following: process-based models (DGVMs for land, GOBMs for oceans), inversion systems (land and ocean), and fCO2 products (ocean only). Positive values are CO2 sinks. Mean estimates from the combination of the process models for the land and oceans are shown (black line) with ±1σ of the model ensemble (grey shading). For the total uncertainty in the process-based estimate of the total sink, uncertainties are summed in quadrature. Mean estimates from the atmospheric inversions are shown (purple lines) with their full spread (purple shading). Mean estimates from the fCO2 products are shown for the ocean domain (light-blue lines) with their full model spread (light-blue shading). The global SOCEAN (upper left) and the sum of SOCEAN in all three regions represent the anthropogenic atmosphere-to-ocean flux based on the assumption that the pre-industrial ocean sink was 0 Gt C yr−1 when riverine fluxes are not considered. This assumption does not hold at the regional level, where pre-industrial fluxes can be significantly different from zero. Hence, the regional panels for SOCEAN represent a combination of natural and anthropogenic fluxes. Bias correction and area weighting were only applied to global SOCEAN; hence the sum of the regions is slightly different from the global estimate (<0.05 Gt C yr−1).
3.8 Partitioning the carbon sinks
3.8.1 Global sinks and spread of estimates
In the period 2013–2022, the bottom-up view of global net ocean and land carbon sinks provided by the GCB, SOCEAN for the ocean and SLAND−ELUC for the land, agrees closely with the top-down global carbon sinks delivered by the atmospheric inversions. This is shown in Fig. 12, which visualizes the individual decadal mean atmosphere–land and atmosphere–ocean fluxes from each, along with the constraints on their sum offered by the global fossil CO2 emission flux minus the atmospheric growth rate (EFOS−GATM, 4.5±0.5 Gt C yr−1, Table 7, shown as diagonal line in Fig. 12). The GCB estimate for net atmosphere-to-surface flux () during 2013–2022 is 4.9±1.2 Gt C yr−1 (Table 7), with the difference from the diagonal representing the budget imbalance (BIM) of 0.4 Gt C yr−1 discussed in Sect. 3.9. By virtue of the inversion methodology, the imbalance of the top-down estimates is <0.1 Gt C yr−1, and thus they scatter across the diagonal, inverse models trading land for ocean fluxes in their solution. The independent constraint on the net atmosphere-to-surface flux based on atmospheric O2 is 4.5±1.0 Gt C yr−1 over the 2013–2022 period (orange symbol in Fig. 12), while the ESM estimate for the net atmosphere-to-surface flux over that period is 5.0 [4.2, 5.5] Gt C yr−1, consistent with the GCB estimate (Tables 5 and 6).
The distributions based on the individual models and data products reveal a substantial spread but converge near the decadal means quoted in Tables 5 to 7. Sink estimates for SOCEAN and from inverse systems are mostly non-Gaussian, while the ensemble of DGVMs appears more normally distributed, justifying the use of a multi-model mean and standard deviation for their errors in the budget. Noteworthy is that the tails of the distributions provided by the land and ocean bottom-up estimates would not agree with the global constraint provided by the fossil fuel emissions and the observed atmospheric CO2 growth rate. This illustrates the power of the atmospheric joint constraint from GATM and the global CO2 observation network it derives from.
Net atmosphere-to-land fluxes
The GCB net atmosphere-to-land fluxes (SLAND−ELUC), calculated as the difference between SLAND from the DGVMs and ELUC from the bookkeeping models, amount to a 2.1±1.1 Gt C yr−1 sink during 2013–2022 (Table 5). Estimates of net atmosphere-to-land fluxes (SLAND−ELUC) from the DGVMs alone (1.7±0.6 Gt C yr−1, Table 5, green symbols in Fig. 12) are slightly lower, within the uncertainty in the GCB estimate and also with the global carbon budget constraint from the ocean sink (, 1.6±0.6 Gt C yr−1; Table 7). For the last decade (2013–2022), the inversions estimate the net atmosphere-to-land uptake to be 1.6 [0.5, 2.3] Gt C yr−1, which is similar to the DGVM estimates (purple symbols in Fig. 12). The ESM estimate for the net atmosphere-to-land uptake during 2013–2022 is 2.4 [1.8, 3.3] Gt C yr−1, consistent with the GCB and DGVM estimates of SLAND−ELUC (Fig. 13 top row). The independent constraint based on atmospheric O2 is significantly lower, 1.2±0.8 Gt C yr−1, although its relatively high uncertainty range overlaps with the central estimates from other approaches.
Net atmosphere-to-ocean fluxes
For the 2013–2022 period, the GOBMs (2.6±0.4 Gt C yr−1) produce a lower estimate for the ocean sink than the fCO2 products (3.1 [2.6, 3.3] Gt C yr−1), which shows up in Fig. 12 as separate peaks in the distribution from the GOBMs (dark-blue symbols) and from the fCO2 products (light-blue symbols). Atmospheric inversions (3.0 [2.4, 4.1] Gt C yr−1) suggest an ocean uptake more in line with the fCO2 products for the recent decade (Table 7), although the inversion range includes both the GOBMs and the fCO2-product estimates (Fig. 13 top row). The ESM estimate of 2.6 [2.2, 3.4] Gt C yr−1 suggests a moderate estimate for the ocean carbon sink, comparable to the GOBM estimate with regard to mean and spread. Conversely, the independent constraint based on atmospheric O2 suggests a larger ocean sink (3.5±0.6 Gt C yr−1), more consistent with the fCO2 products and atmospheric inversions. We caution that the riverine transport of carbon taken up on land and outgassing from the ocean comprise a substantial (0.65±0.3 Gt C yr−1) and uncertain term (Crisp et al., 2022; Gruber et al., 2023; DeVries et al., 2023) that separates the GOBM-, ESM-, and oxygen-based estimates on the one hand from the fCO2 products and atmospheric inversions on the other hand. However, the high ocean sink estimate based on atmospheric oxygen that is not subject to river flux adjustment provides another line of evidence that most GOBMs and ESMs underestimate the ocean sink.
3.8.2 Regional partitioning
Figure 13 shows the latitudinal partitioning of the global atmosphere-to-ocean flux (SOCEAN) and atmosphere-to-land flux (SLAND−ELUC) and their sum () according to the estimates from GOBMs and ocean fCO2 products (SOCEAN), DGVMs (SLAND−ELUC), and atmospheric inversions (SOCEAN and SLAND−ELUC).
North
Despite being one of the most densely observed and studied regions of our globe, annual mean carbon sink estimates in the northern extra-tropics (north of 30∘ N) continue to differ. The atmospheric inversions suggest an atmosphere-to-surface sink () for 2013–2022 of 2.8 [1.7, 3.3] Gt C yr−1, which is higher than the process models' estimate of 2.2±0.4 Gt C yr−1 (Fig. 13). The GOBMs (1.2±0.2 Gt C yr−1), fCO2 products (1.3 [1.2, 1.4] Gt C yr−1), and inversion systems (1.2 [0.7, 1.4] Gt C yr−1) produce consistent estimates of the ocean sink. Thus, the difference mainly arises from the net land flux (SLAND−ELUC) estimate, which is 1.0±0.4 Gt C yr−1 in the DGVMs compared to 1.6 [0.4, 2.6] Gt C yr−1 in the atmospheric inversions (Fig. 13, second row). We note that the range among inversions driven by OCO-2 satellite data is smaller though (1.6–2.2 Gt C yr−1, N=6), supporting the notion that northern extra-tropics land uptake was larger than suggested by the DGVMs at least in the 2015–2022 period covered by this data product.
Discrepancies in the northern land fluxes conform with persistent issues surrounding the quantification of the drivers of the global net land CO2 flux (Arneth et al., 2017; Huntzinger et al., 2017; O'Sullivan et al., 2022) and the distribution of atmosphere-to-land fluxes between the tropics and high northern latitudes (Baccini et al., 2017; Schimel et al., 2015; Stephens et al., 2007; Ciais et al., 2019; Gaubert et al., 2019).
In the northern extra-tropics, the process models, inversions, and fCO2 products consistently suggest that most of the variability stems from the land (Fig. 13). Inversions generally estimate similar interannual variations (IAVs) over land to DGVMs (0.28–0.35 vs. 0.8–0.64 Gt C yr−1, averaged over 1990–2022), and they have higher IAVs in ocean fluxes (0.05–0.10 Gt C yr−1) relative to GOBMs (0.02–0.06 Gt C yr−1, Fig. S2) and fCO2 products (0.03–0.10 Gt C yr−1).
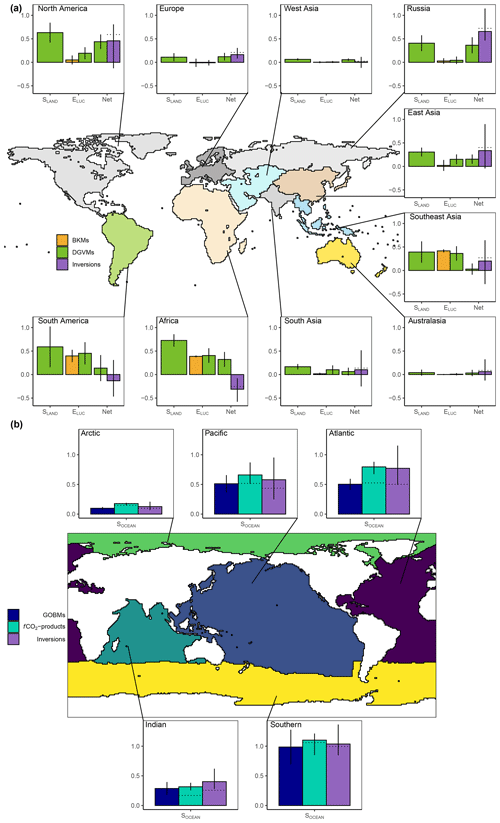
Figure 14Decadal mean (a) land and (b) ocean fluxes for RECCAP-2 regions over 2013–2022. For land fluxes, SLAND is estimated by the DGVMs (green bars), with the error bar as ±1σ spread among models. A positive SLAND value is a net transfer of carbon from the atmosphere to the land. ELUC fluxes are shown for both DGVMs (green) and bookkeeping models (BKMs; orange), again with the uncertainty calculated as the ±1σ spread. Note that a positive ELUC flux indicates a loss of carbon from the land. The net land flux is shown for both DGVMs (green) and atmospheric inversions (purple), including the full model spread for inversions. The net ocean sink (SOCEAN) is estimated by GOBMs (royal blue), fCO2 products (cyan), and atmospheric inversions (purple). Uncertainty is estimated as the ±1σ spread for GOBMs and the full model spread for the other two products. The dotted lines show the fCO2 products and inversion results without river flux adjustment. Positive values are CO2 sinks.
Tropics
In the tropics (30∘ S–30∘ N), both the atmospheric inversions and process models estimate a net carbon balance () that is close to neutral over the past decade. The GOBMs ( Gt C yr−1), fCO2 products (0.2 [0.2, 0.3] Gt C yr−1), and inversion systems (−0.3 [−0.1, 0.8] Gt C yr−1) all indicate an approximately neutral tropical ocean flux (see Fig. S1 for spatial patterns). DGVMs indicate a net land sink (SLAND−ELUC) of 0.6±0.4 Gt C yr−1, whereas the inversion systems indicate a net land flux of 0.03 [−0.8, 1.1] Gt C yr−1, though with high uncertainty (Fig. 13, third row).
The tropical lands are the origin of most of the atmospheric CO2 interannual variability (Ahlström et al., 2015), consistently among the process models and inversions (Fig. 13). The interannual variability in the tropics is similar among the ocean fCO2 products (0.07–0.16 Gt C yr−1) and the GOBMs (0.07–0.16 Gt C yr−1, Fig. S2), which is the highest ocean sink variability of all regions. The DGVMs and inversions indicate that atmosphere-to-land CO2 fluxes are more variable than atmosphere-to-ocean CO2 fluxes in the tropics, with interannual variability of 0.35–1.61 and 0.77–0.92 Gt C yr−1 for DGVMs and inversions, respectively.
South
In the southern extra-tropics (south of 30∘ S), the atmospheric inversions suggest a net atmosphere-to-surface sink () for 2013–2022 of 1.5 [1.2, 1.9] Gt C yr−1, slightly higher than the process models' estimate of 1.5±0.4 Gt C yr−1 (Fig. 13). An approximately neutral net land flux (SLAND−ELUC) for the southern extra-tropics is estimated by both the DGVMs (0.05±0.07 Gt C yr−1) and the inversion systems (sink of 0.02 [−0.2, 0.2] Gt C yr−1). This means nearly all carbon uptake is due to oceanic sinks south of 30∘ S. The Southern Ocean flux in the fCO2 products (1.6 [1.3, 1.7 Gt C] yr−1) and inversion estimates (1.5 [1.3, 1.9] Gt C yr−1) is slightly higher than in the GOBMs (1.4±0.3 Gt C yr−1) (Fig. 13, bottom row). This discrepancy in the mean flux is smaller this year than in previous releases due to the change of the data set of the regional distribution of the river flux adjustment applied to fCO2 products and inverse systems to isolate the anthropogenic SOCEAN flux. The data set used (Lacroix et al., 2020) has less river-induced carbon outgassing in the Southern Ocean than the previously used data set (Aumont et al., 2001). Nevertheless, the time series of atmospheric inversions and fCO2 products diverge from the GOBMs. A substantial overestimation of the trends in the fCO2 products could be explained by sparse and unevenly distributed observations, especially in wintertime (Fig. S1; Hauck et al., 2023; Gloege et al., 2021). Model biases may contribute as well, with biases in mode water formation, stratification, and the chemical buffer capacity known to play a role in Earth system models (Terhaar et al., 2021; Bourgeois et al., 2022; Terhaar et al., 2022).
The interannual variability in the southern extra-tropics is low because of the dominance of ocean areas with low variability compared to land areas. The split between land (SLAND−ELUC) and ocean (SOCEAN) shows a substantial contribution to variability in the south coming from the land, with no consistency between the DGVMs and the inversions or among inversions. This is expected due to the difficulty of precisely separating the land and oceanic fluxes when viewed from atmospheric observations alone. The SOCEAN interannual variability was found to be higher in the fCO2 products (0.04–0.18 Gt C yr−1) compared to GOBMs (0.03 to 0.06 Gt C yr−1) in 1990–2022 (Fig. S2). Model subsampling experiments recently illustrated that fCO2 products may overestimate decadal variability in the Southern Ocean carbon sink by 30 % and the trend since 2000 by 50 %–130 % due to data sparsity, based on a couple of fCO2 products with strong variability (Gloege et al., 2021; Hauck et al., 2023).
RECCAP-2 regions
Aligning with the RECCAP-2 initiative (Ciais et al., 2022; Poulter et al., 2022; DeVries et al., 2023), we provide a breakdown of this GCB paper estimate of the ELUC, SLAND, net land (SLAND−ELUC), and SOCEAN fluxes over the 10 land and 5 ocean RECCAP-2 regions, averaged over the period 2013–2022. The DGVMs and inversions suggest a positive net land sink in all regions, except for South America and Africa, where the inversions indicate a small net source of −0.1 [−0.5, 0.3] Gt C yr−1 and −0.3 [−0.6, −0.1] Gt C yr−1, respectively, compared to a small sink of 0.1±0.3 Gt C yr−1 and 0.3±0.2 Gt C yr−1 for the DGVMs. However, for South America, there is substantial uncertainty in both products (ensembles span zero). For the DGVMs, this is driven by uncertainty in both SLAND (0.6±0.5 Gt C yr−1) and ELUC (0.4±0.2 Gt C yr−1). The bookkeeping models also suggest an ELUC source of around 0.4 Gt C yr−1 in South America and Africa, in line with the DGVM estimates. Bookkeeping models and DGVMs similarly estimate a source of 0.4 Gt C yr−1 in Southeast Asia, with DGVMs suggesting a near-neutral net land sink (0.03±0.12 Gt C yr−1). This contrasts with the inversion estimate of a 0.2 [−0.3, 0.6] Gt C yr−1 sink, although the inversion spread is substantial. The inversions suggest the largest net land sinks are located in North America (0.5 [−0.1, 0.8] Gt C yr−1), Russia (0.7 [0.5, 1.1] Gt C yr−1), and East Asia (0.3 [0.0, 0.9] Gt C yr−1). This agrees well with the DGVMs in North America (0.4±0.2 Gt C yr−1), which indicate a large natural land sink (SLAND) of 0.6±0.2 Gt C yr−1, being slightly reduced by land-use-related carbon losses (0.2±0.1 Gt C yr−1). The DGVMs suggest a smaller net land sink in Russia compared to inversions (0.4±0.2 Gt C yr−1) and a similar net sink in East Asia (0.2±0.1 Gt C yr−1).
There is generally a higher level of agreement in the estimates of regional SOCEAN between the different data streams (GOBMs, fCO2 products, and atmospheric inversions) on a decadal scale, compared to the agreement between the different land flux estimates. All data streams agree that the largest contribution to SOCEAN stems from the Southern Ocean due to a combination of high flux density and a large surface area but with important contributions also from the Atlantic (high flux density) and Pacific (large area) basins. In the Southern Ocean, GOBMs suggest a sink of 1.0±0.3 Gt C yr−1, in line with the fCO2 products (1.1 [0.9, 1.2] Gt C yr−1) and atmospheric inversions (1.0 [0.8, 1.4] Gt C yr−1). There is similar agreement in the Pacific Ocean, with GOBMs, fCO2 products, and atmospheric inversions indicating a sink of 0.5±0.1 Gt C yr−1, 0.7 [0.5, 0.9] Gt C yr−1, and 0.6 [0.2, 1.0] Gt C yr−1, respectively. However, in the Atlantic Ocean, GOBMs simulate a sink of 0.5±0.1 Gt C yr−1, noticeably lower than both the fCO2 products (0.8 [0.7, 0.9] Gt C yr−1) and atmospheric inversions (0.8 [0.5, 1.2] Gt C yr−1). It is important to note the fCO2 products and atmospheric inversions have a substantial and uncertain river flux adjustment in the Atlantic Ocean (0.3 Gt C yr−1) that also leads to a mean offset between GOBMs and fCO2 products/inversions in the latitude band of the tropics (Fig. 13). The Indian Ocean due to its smaller size and the Arctic Ocean due to its size and sea-ice cover that prevents air–sea gas exchange are responsible for smaller but non-negligible SOCEAN fluxes (Indian Ocean: 0.3 [0.2, 0.4] Gt C yr−1, 0.3 [0.3, 0.4] Gt C yr−1, and 0.4 [0.3, 0.6] Gt C yr−1 for GOBMs, fCO2 products, and atmospheric inversions, respectively; Arctic Ocean: 0.1 [0.1, 0.1] Gt C yr−1, 0.2 [0.2, 0.2] Gt C yr−1, and 0.1 [0.1, 0.1] Gt C yr−1 for GOBMs, fCO2 products, and atmospheric inversions, respectively). Note that the SOCEAN numbers presented here deviate from numbers reported in RECCAP-2, where the net air–sea CO2 flux is reported (i.e. without river flux adjustment for fCO2 products and inversions and with river flux adjustment subtracted from GOBMs in most chapters or comparing unadjusted data sets with discussion of uncertain regional riverine fluxes as major uncertainty, e.g. Sarma et al., 2023; DeVries et al., 2023).
Tropical vs. northern land uptake
A continuing conundrum is the partitioning of the global atmosphere–land flux between the Northern Hemisphere land and the tropical land (Stephens et al., 2007; Pan et al., 2011; Gaubert et al., 2019). It is of importance because each region has its own history of land-use change, climate drivers, and impact of increasing atmospheric CO2 and nitrogen deposition. Quantifying the magnitude of each sink is a prerequisite to understanding how each individual driver impacts the tropical and middle-/high-latitude carbon balance.
We define the north–south (N–S) difference as net atmosphere–land flux north of 30∘ N minus the net atmosphere–land flux south of 30∘ N. For the inversions, the N–S difference ranges from −0.5 Gt C yr−1 to +3.0 Gt C yr−1 across this year's inversion ensemble but with a clear cluster of solutions driven by the OCO-2 satellite product with a NH land sink of 1.6–2.2 Gt C yr−1, along with a tropical land flux of −0.6 to +0.2 Gt C yr−1 and a dipole between +1.4 and +2.8 Gt C yr−1 for the period 2015–2022. Whether this tighter clustering relative to the surface-observation-based inversions is driven by (a) additional information on tropical fluxes delivered by tropical retrievals contained in OCO-2, (b) a tighter constraint on the NH land sink from that same product, or (c) a reduced sensitivity to vertical transport differences between models when using CO2 column integrals requires further investigation.
In the ensemble of DGVMs, the N–S difference is 0.5±0.6 Gt C yr−1, a much narrower range than the one from atmospheric inversions. Five DGVMs have a N–S difference larger than 1.0 Gt C yr−1, compared to only two from last year's ensemble. This is still only 25 % of DGVMs, compared to most inversion systems simulating a difference at least this large. The smaller spread across DGVMs than across inversions is to be expected as there is no correlation between northern and tropical land sinks in the DGVMs as opposed to the inversions where the sum of the two regions being well constrained by atmospheric observations leads to an anti-correlation between these two regions. This atmospheric N–S gradient could be used as an additional way to evaluate tropical and NH uptake in DGVMs if their fluxes were combined with multiple transport models. Vice versa, the much smaller spread in the N–S difference between the DGVMs could help to scrutinize the inverse systems further. For example, a large northern land sink and a tropical land source in an inversion would suggest a large sensitivity to CO2 fertilization (the dominant factor driving the land sinks) for northern ecosystems, which would not be mirrored by tropical ecosystems. Such a combination could be hard to reconcile with the process understanding gained from the DGVM ensembles and independent measurements (e.g. free-air CO2 enrichment experiments).
3.8.3 Fire emissions in 2023
Fire emissions so far in 2023 have been above the average of recent decades due to an extreme wildfire season in North America. Figure S9 shows global and regional emission estimates for the period 1 January–30 September in each year 2003–2023. Estimates derive from two global fire emission products: the Global Fire Emissions Database (GFED, version 4.1s; van der Werf et al., 2017) and the Global Fire Assimilation System (GFAS, operated by the Copernicus Atmosphere Monitoring Service; Di Giuseppe et al., 2018). The two products estimate that global emissions from fires were 1.9–2.3 Gt C yr−1 during January–October 2023. These estimates are 19 %–33 % above the 2013–2022 average for the same months (1.6–1.7 Gt C yr−1) and 10 %–28 % above the 2003–2022 average (1.8 Gt C yr−1 in both products).
The above-average global fire emissions during January–October 2023 occurred despite below-average fire emissions from major source regions. On average during 2013–2022, 75 %–80 % of global fire emissions through October occurred in the tropics (1.2–1.4 Gt C yr−1) and around 41 %–48 % of global fire emissions through October occurred in Africa (0.7–0.8 Gt C yr−1). This year, through October, fire emissions in the tropics were approximately equal to the 2013–2022 average and 7 %–9 % below the 2003–2022 average, while in Africa the fire emissions were approximately equal to the 2013–2022 average and 4 %–13 % below the 2003–2022 average.
In contrast, fire emissions from the northern extra-tropics so far in 2023 have exceeded the values of all previous years. Northern extra-tropical fire emissions during January–October 2023 (0.7–0.9 Gt C yr−1) were 84 %–183 % above the average for the same months in 2013–2022 (0.3–0.4 Gt C yr−1) and 76 %–190 % above the average for the same months in 2003–2022 (0.3–0.4 Gt C yr−1). Fire emissions in North America alone (0.5–0.8 Gt C yr−1) were 239 %–438 % above the average of 2013–2022 (0.2 Gt C yr−1 for both products) and 215 %–410 % above the average for 2003–2022 (0.2 Gt C yr−1 for both products). Extreme fires in Canada were the largest contributor to the anomaly in 2023, with emissions reaching 0.5–0.8 Gt C yr−1 or 527 %–874 % above the 2013–2022 average (0.1 Gt C yr−1 in both products) and 450 %–709 % above the 2003–2022 average (0.1 Gt C yr−1 in both products).
While the fire emission fluxes presented above point towards a highly unusual Northern Hemisphere fire season so far in 2023, we caution that the fluxes presented should not be compared directly with other fluxes of the budget (e.g. SLAND or ELUC) due to incompatibilities between the observable fire emission fluxes and what is quantified in the SLAND and ELUC components of the budget. The fire emission estimates from global fire products relate to all fire types that can be observed in Earth observations (Giglio et al., 2018; Randerson et al., 2012; Kaiser et al., 2012), including (i) fires occurring as part of natural disturbance–recovery cycles that would also have occurred in the pre-industrial period (Yue et al., 2016; Keeley and Pausas, 2019; Zou et al., 2019); (ii) fires occurring above and beyond natural disturbance–recovery cycles due to changes in climate, CO2, and N fertilization and to an increased frequency of extreme drought and heatwave events (Abatzoglou et al., 2019; Jones et al., 2022; Zheng et al., 2021; Burton et al., 2023); and (iii) fires occurring in relation to land use and land-use change, such as deforestation fires and agricultural fires (van der Werf et al., 2010; Magi et al., 2012). In the context of the global carbon budget, only the portion of fire emissions associated with (ii) should be included in the SLAND component, and fire emissions associated with (iii) should already be accounted for in the ELUC component. Emissions associated with (i) should not be included in the global carbon budget. It is not currently possible to derive specific estimates for fluxes (i), (ii), and (iii) using global fire emission products such as GFED or GFAS. In addition, the fire emission estimates from global fire emission products represent a gross flux of carbon to the atmosphere, whereas the SLAND component of the budget is a net flux that should also include post-fire recovery fluxes. Even if emissions from fires of type (ii) could be separated from those of type (i), these fluxes may be partially or wholly offset in subsequent years by post-fire fluxes as vegetation recovers, sequestering carbon from the atmosphere in the terrestrial biosphere (Yue et al., 2016).
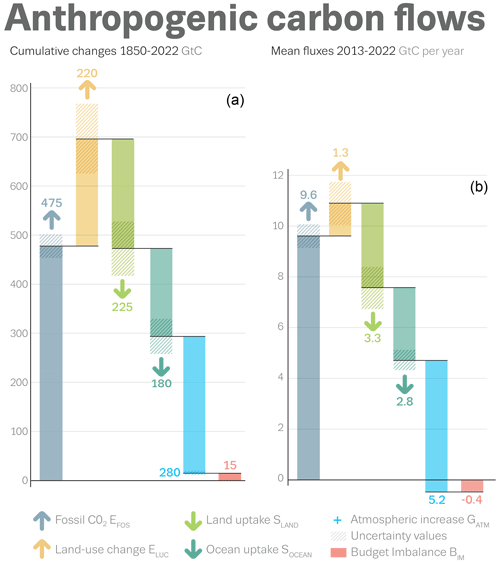
Figure 15Cumulative changes over the 1850–2022 period (a) and average fluxes over the 2013–2022 period (b) for the anthropogenic perturbation of the global carbon cycle. See the caption of Fig. 3 for key information and Methods in the text for full details.
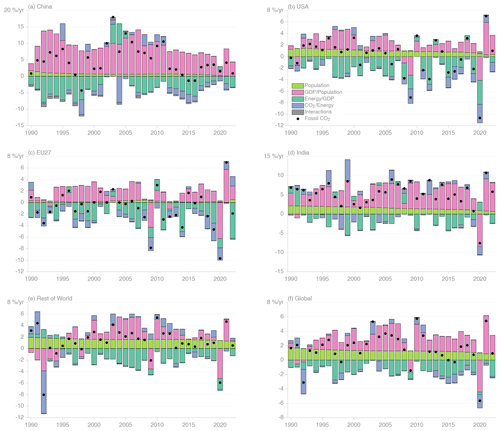
Figure 16Kaya decomposition of the main drivers of fossil CO2 emissions, considering population, GDP per person, energy per GDP, and CO2 emissions per energy unit, for China (a), the USA (b), EU27 (c), India (d), the rest of the world (e), and globally (f). Black dots are the annual fossil CO2 emissions growth rate; coloured bars are the contributions from the different drivers to this growth rate. A general trend is that population and GDP growth put upward pressure on emissions (positive values), while energy per GDP and, more recently, CO2 emissions per energy unit put downward pressure on emissions (negative values). Both the COVID-19-induced drop during 2020 and the recovery in 2021 led to a stark contrast to previous years, with different drivers in each region. The EU27 had strong energy per GDP improvement in 2022.
3.9 Closing the global carbon cycle
3.9.1 Partitioning of cumulative emissions and sink fluxes
The global carbon budget over the historical period (1850–2021) is shown in Fig. 3.
Emissions during the period 1850–2022 amounted to 695±70 Gt C and were partitioned among the atmosphere (280±5 Gt C; 40 %), ocean (180±35 Gt C; 26 %), and land (225±55 Gt C; 32 %). The cumulative land sink is almost equal to the cumulative land-use emissions (220±70 Gt C), making the global land nearly neutral over the whole 1850–2022 period.
The use of nearly independent estimates for the individual terms of the global carbon budget shows a cumulative budget imbalance of 15 Gt C (2 % of total emissions) during 1850–2022 (Fig. 3, Table 8), which, if correct, suggests that emissions could be slightly too high by the same proportion (2 %) or that the combined land and ocean sinks are slightly underestimated (by about 3 %), although these are well within the uncertainty range of each component of the budget. Nevertheless, part of the imbalance could originate from the estimation of a significant increase in EFOS and ELUC between the mid-1920s and the mid-1960s which is unmatched by a similar growth in atmospheric CO2 concentration as recorded in ice cores (Fig. 3). However, the known loss of additional sink capacity of 30–40 Gt C (over the 1850–2020 period) due to reduced forest cover has not been accounted for in our method and would exacerbate the budget imbalance (see Sects. 2.10 and S.6.4).
For the more recent 1960–2022 period where direct atmospheric CO2 measurements are available, total emissions (EFOS+ELUC) amounted to 485±50 Gt C, of which 395±20 Gt C (82 %) was caused by fossil CO2 emissions and 90±45 Gt C (18 %) by land-use change (Table 8). The total emissions were partitioned among the atmosphere (215±5 Gt C; 44 %), ocean (125±25 Gt C; 25 %), and land (150±35 Gt C; 31 %), with a near-zero (−5 Gt C) unattributed budget imbalance. All components except land-use change emissions have significantly grown since 1960, with important interannual variability in the growth rate of atmospheric CO2 concentration and in the land CO2 sink (Fig. 4) and some decadal variability in all terms (Table 7). Differences with previous budget releases are documented in Fig. S5.
The global carbon budget averaged over the last decade (2013–2022) is shown in Figs. 2 and 15 (right panel) and Table 7. For this period, 88 % of the total emissions (EFOS+ELUC) were from fossil CO2 emissions (EFOS) and 12 % from land-use change (ELUC). The total emissions were partitioned among the atmosphere (47 %), ocean (26 %), and land (31 %), with a small unattributed budget imbalance (∼4 %). For single years, the budget imbalance can be larger (Fig. 4). For 2022, the combination of our estimated sources (11.1±0.9 Gt C yr−1) and sinks (11.2±0.9 Gt C yr−1) leads to a BIM of −0.09 Gt C, suggesting a near closure of the global carbon budget, although there is relatively high uncertainty in BIM (±1.3 Gt C for 2022) as this is calculated as the residual of the five budget terms.
3.9.2 Trend and variability in the carbon budget imbalance
The carbon budget imbalance (BIM; Eq. 1, Fig. 4) quantifies the mismatch between the estimated total emissions and the estimated changes in the atmosphere, land, and ocean reservoirs. The budget imbalance from 1960 to 2022 is very small (−3.0 Gt C over the period, i.e. average of 0.05 Gt C yr−1) and shows no trend over the full time series (Fig. 4e). The process models (GOBMs and DGVMs) and data products have been selected to match observational constraints in the 1990s, but no further constraints have been applied to their representation of trend and variability. Therefore, the near-zero mean of and trend in the budget imbalance are seen as evidence of a coherent community understanding of the emissions and their partitioning on those timescales (Fig. 4). However, the budget imbalance shows substantial variability of the order of ±1 Gt C yr−1, particularly over semi-decadal timescales, although most of the variability is within the uncertainty of the estimates. The positive carbon imbalance during the 1960s, and early 1990s, indicates that either the emissions were overestimated or the sinks were underestimated during these periods. The reverse is true for the 1970s and to a lesser extent for the 1980s and 2013–2022 period (Fig. 4, Table 7).
We cannot attribute the cause of the variability in the budget imbalance with our analysis; we only note that the budget imbalance is unlikely to be explained by errors or biases in the emissions alone because of its component of large semi-decadal variability, a variability that is atypical of emissions and has not changed in the past 60 years despite a near tripling in emissions (Fig. 4). Errors in SLAND and SOCEAN are more likely to be the main cause for the budget imbalance, especially on interannual to semi-decadal timescales. For example, underestimation of the SLAND by DGVMs has been reported following the eruption of Mount Pinatubo in 1991, possibly due to missing responses to changes in diffuse radiation (Mercado et al., 2009). Although since GCB2021 we have accounted for aerosol effects on solar radiation quantity and quality (diffuse vs. direct), most DGVMs have only used the former as input (i.e. total solar radiation) (Table S1). Thus, the ensemble mean may not capture the full effects of volcanic eruptions, i.e. associated with high loads of light-scattering sulfate aerosols, on the land carbon sink (O'Sullivan et al., 2021). DGVMs are suspected to overestimate the land sink in response to the wet decade of the 1970s (Sitch et al., 2008). Quasi-decadal variability in the ocean sink has also been reported, with all methods agreeing on a smaller-than-expected ocean CO2 sink in the 1990s and a larger-than-expected sink in the 2000s (Fig. 10; Landschützer et al., 2016; DeVries et al., 2019; Hauck et al., 2020; McKinley et al., 2020; Gruber et al., 2023), and the climate-driven variability could be substantial but is not well constrained (DeVries et al., 2023; Müller et al., 2023). Errors in sink estimates could also be driven by errors in the climatic forcing data, particularly precipitation for SLAND and wind for SOCEAN. Also, the BIM shows substantial departure from zero on yearly timescales (Fig. 4e), highlighting unresolved variability in the carbon cycle, likely in the land sink (SLAND) given its large year-to-year variability (Figs. 4d and 8).
Both the budget imbalance (BIM, Table 7) and the residual land sink from the global budget (, Table 5) include an error term due to the inconsistencies that arise from combining ELUC from bookkeeping models with SLAND from DGVMs, most notably the loss of additional sink capacity (see Sects. 2.10 and S.6.4). Other differences include a better accounting of land-use-change practices and processes in bookkeeping models than in DGVMs and the bookkeeping models' error of having present-day-observed carbon densities fixed in the past. That the budget imbalance shows no clear trend towards larger values over time is an indication that these inconsistencies probably play a minor role compared to other errors in SLAND or SOCEAN.
Although the budget imbalance is near zero for the recent decades, this could be due to compensation of errors. We cannot exclude an overestimation of CO2 emissions, particularly from land-use change, given their large uncertainty, as has been suggested elsewhere (Piao et al., 2018), and/or an underestimate of the sinks. A larger DGVM estimate of the atmosphere–land CO2 flux (SLAND−ELUC) over the extra-tropics would reconcile model results with inversion estimates for fluxes in the total land during the past decade (Fig. 13; Table 5). Likewise, a larger SOCEAN value is also possible given the higher estimates from the fCO2 products (see Sect. 3.6.2, Figs. 10 and 13); the underestimation of interior ocean anthropogenic carbon accumulation in the GOBMs (Sect. 3.6.5); and the recently suggested upward adjustments of the ocean carbon sink in Earth system models (Terhaar et al., 2022) and in fCO2 products, here related to a potential temperature bias and skin effects (Watson et al., 2020; Dong et al., 2022; Fig. 10). If SOCEAN were to be based on fCO2 products alone, with all fCO2 products including this adjustment, this would result in a 2013–2022 SOCEAN value of 3.7 Gt C yr−1 (Dong et al., 2022) or >3.9 Gt C yr−1 (Watson et al., 2020), i.e. outside of the range supported by the atmospheric inversions and with an implied negative BIM of more than −1 Gt C yr−1, indicating that a closure of the budget could only be achieved with anthropogenic emissions being significantly larger and/or the net land sink being substantially smaller than estimated here. A recent model study suggests that the skin effect is smaller (about 0.1 Gt C yr−1 or 5 %) due to feedbacks with surface carbon concentration (Bellenger et al., 2023), which would nevertheless lead to a larger SOCEAN value even in the GOBMs. More integrated use of observations in the global carbon budget, either on their own or for further constraining model results, should help resolve some of the budget imbalance (Peters et al., 2017).
The average growth in global fossil CO2 emissions peaked at nearly +3 % yr−1 during the 2000s, driven by the rapid growth in emissions in China. In the last decade, however, the global growth rate has slowly declined, reaching a low of +0.5 % yr−1 over 2013–2022. While this slowdown in global fossil CO2 emissions growth is welcome, global fossil CO2 emissions continue to grow and are far from the rapid emission decreases needed to be consistent with the temperature goals of the Paris Agreement.
Since the 1990s, the average growth rate of fossil CO2 emissions has continuously declined across the group of developed countries of the Organisation for Economic Co-operation and Development (OECD), with emissions peaking in around 2005 and now declining at around 1 % yr−1 (Le Quéré et al., 2021). In the decade 2013–2022, territorial fossil CO2 emissions decreased significantly (at the 95 % confidence level) in 26 countries/economic entities whose economies grew significantly: Belgium, Brazil, Czechia, Denmark, Estonia, Finland, France, Germany, Greece, Hong Kong SAR, Israel, Italy, Jamaica, Japan, Luxembourg, the Netherlands, Norway, Portugal, Romania, Slovenia, South Africa, Sweden, Switzerland, the United Kingdom, the USA, and Zimbabwe (updated from Le Quéré et al., 2019). Altogether, these 26 countries emitted 2.7 Gt C yr−1 (10.0 Gt CO2 yr−1) on average over the last decade, about 28 % of world CO2 fossil emissions. For comparison, 22 countries showed a significant decrease in territorial fossil CO2 emissions over the previous decade (2003–2012). Figure 16 shows that the emission declines in the USA and the EU27 are primarily driven by slightly weaker economic growth in the last decade compared to the 1990s, sustained declines in energy per GDP (though weakening in the USA), and sustained declines in CO2 emissions per energy unit (decarbonization) with a slight acceleration in the USA in the last decade.
In contrast, fossil CO2 emissions continue to grow in non-OECD countries, although the growth rate has slowed from more than 6 % yr−1 during the 2000s to less than 2 % yr−1 in the last decade. Representing 47 % of non-OECD emissions in 2022, a large part of this slowdown is due to China, which has seen emissions growth decline from 9 % yr−1 in the 2000s to 1.6 % yr−1 in the last decade. Excluding China, non-OECD emissions grew at 3.1 % yr−1 in the 2000s compared to 1.5 % yr−1 in the last decade. China had weaker economic growth in the 2000s compared to the 2010s and a higher decarbonization rate from 2005 to 2015 that is comparable to the highs in the 1990s, though the decarbonization rate has slowed considerably since 2016 (Fig. 16). India and the rest of the world have had strong economic growth that is not offset by decarbonization or declines in energy per GDP, driving up fossil CO2 emissions. Despite the high deployment of renewables in some countries (e.g. India), fossil energy sources continue to grow to meet growing energy demand (Le Quéré et al., 2019).
Globally, fossil CO2 emissions growth is slowing, and this is due in part to the emergence of climate policy (Eskander and Fankhauser, 2020; Le Quéré et al., 2019) and technological change, which are leading to a shift from coal to gas and growth in renewable energies, as well as reduced expansion of coal capacity. At the aggregated global level, decarbonization shows a strong and growing signal in the last decade, with smaller contributions due to lower economic growth and declines in energy per GDP. Altogether, global emissions are still growing (average of 0.5 % yr−1 over the 2013–2022 decade) and are far from the reductions needed to meet the ambitious climate goals of the UNFCCC Paris Agreement.
This year we updated the remaining carbon budget (RCB) based on two studies, the IPCC AR6 (Canadell et al., 2021) as used in GCB2022 and a recent revision of the IPCC AR6 estimates (Forster et al., 2023; Lamboll et al., 2023). We update the RCB assessed by the IPCC AR6 (Canadell et al., 2021), accounting for the 2020–2023 estimated emissions from fossil fuel combustion (EFOS) and land-use changes (ELUC). From January 2024, the IPCC AR6 RCB (50 % likelihood) for limiting global warming to 1.5, 1.7, and 2 ∘C is estimated to amount to 95, 190, and 325 Gt C (340, 690, 1190 Gt CO2). The Forster et al. (2023) study proposed a significantly lower RCB than the IPCC AR6, with the largest reduction being due to an update of the climate emulator (MAGICC) used to estimate the warming contribution of non-CO2 agents and due to the warming (i.e. emissions) that occurred over the 2020–2022 period. We update the Forster et al. (2023) budget accounting for the 2023 estimated emissions from fossil fuel combustion (EFOS) and land-use changes (ELUC). From January 2024, the Forster et al. (2023) RCB (50 % likelihood) for limiting global warming to 1.5, 1.7, and 2 ∘C is estimated to amount to 55, 155, and 305 Gt C (210, 560, 1110 Gt CO2), significantly smaller than the updated IPCC AR6 estimate. Both the original IPCC AR6 and the Forster et al. (2023) estimates include an uncertainty due to the climate response to cumulative CO2 emissions, which is reflected through the percent likelihood of exceeding the given temperature threshold, an additional uncertainty of 220 Gt CO2 due to alternative non-CO2 emission scenarios, and other sources of uncertainties (see Canadell et al., 2021). The two sets of estimates overlap when considering all uncertainties. The IPCC AR6 estimates have the advantage of a consensus-building approach, while the Forster et al. (2023) estimates include significant update estimates but without the backing of the IPCC yet. Here, we take the average of our update of both IPCC AR6 and Forster et al. (2023) estimates, giving a remaining carbon level (50 % likelihood) for limiting global warming to 1.5, 1.7, and 2 ∘C of 75, 175, and 315 Gt C (275, 625, 1150 Gt CO2), respectively, starting from January 2024. We emphasize the large uncertainties, particularly when close to the global warming limit of 1.5 ∘C. These 1.5, 1.7, and 2 ∘C average remaining carbon budgets correspond to about 7, 15, and 28 years, respectively, from the beginning of 2024, at the 2023 level of total anthropogenic CO2 emissions. Reaching net-zero CO2 emissions by 2050 entails cutting total anthropogenic CO2 emissions by about 0.4 Gt C (1.5 Gt CO2) each year on average, comparable to the decrease in EFOS observed in 2020 during the COVID-19 pandemic. However, this would lead to cumulative emissions over 2024–2050 of 150 Gt C (550 Gt CO2), which is well above the remaining carbon budget of 75 Gt C to limit global warming to 1.5 ∘C but still below the remaining budget of 175 Gt C to limit warming to 1.7 ∘C (in phase with the “well below 2 ∘C” ambition of the Paris Agreement). Even reaching net-zero CO2 globally by 2040, which would require annual emissions cuts of 0.7 Gt C (2.4 Gt CO2) on average, would still exceed the remaining carbon budget, with 95 Gt C (350 Gt CO2) cumulative emissions over 2024–2050, unless the global emissions trajectory becomes net negative (i.e. more anthropogenic CO2 sinks than emissions) after 2040.
Table 10Major known sources of uncertainties in each component of the global carbon budget, defined as input data or processes that have a demonstrated effect of at least ±0.3 Gt C yr−1.
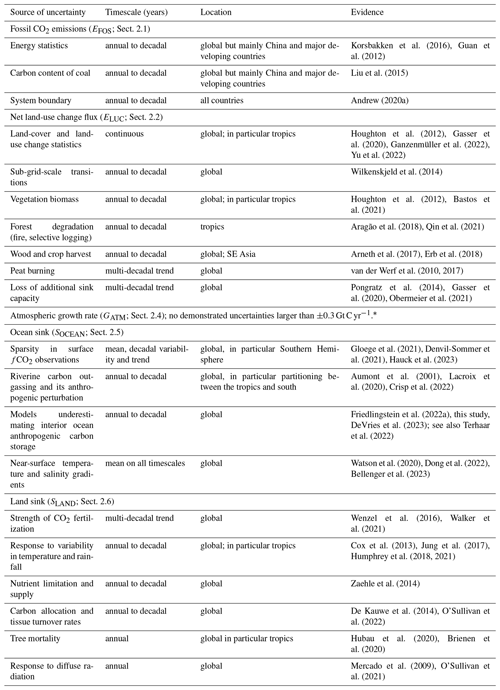
* The uncertainties in GATM have been estimated as ±0.2 Gt C yr−1, although the conversion of the growth rate into a global annual flux assuming instantaneous mixing throughout the atmosphere introduces additional errors that have not yet been quantified.
Each year when the global carbon budget is published, each flux component is updated for all previous years to consider corrections that are the result of further scrutiny and verification of the underlying data in the primary-input data sets. Annual estimates may be updated with improvements in data quality and timeliness (e.g. to eliminate the need for extrapolation of forcing data such as land use). Of all terms in the global budget, only the fossil CO2 emissions and the growth rate of atmospheric CO2 concentration are based primarily on empirical inputs supporting annual estimates in this carbon budget. The carbon budget imbalance, although an imperfect measure, provides a strong indication of the limitations in observations, in understanding and representing processes in models, and/or in the integration of the carbon budget components.
The persistent unexplained variability in the carbon budget imbalance limits our ability to verify reported emissions (Peters et al., 2017) and suggests we do not yet have a complete understanding of the underlying carbon cycle dynamics on annual to decadal timescales. Resolving most of this unexplained variability should be possible through different and complementary approaches. First, as intended with our annual updates, the imbalance as an error term should be reduced by improvements of individual components of the global carbon budget that follow from improving the underlying data and statistics and by improving the models through the resolution of some of the key uncertainties detailed in Table 10. Second, additional clues to the origin of and processes responsible for the variability in the budget imbalance could be obtained through a closer scrutiny of carbon variability in light of other Earth system data (e.g. heat balance, water balance) and the use of a wider range of biogeochemical observations to better understand the land–ocean partitioning of the carbon imbalance, such as the constraint from atmospheric oxygen included this year. Finally, additional information could also be obtained through better inclusion of process knowledge at the regional level and through the introduction of inferred fluxes such as those based on satellite xCO2 retrievals. The limit of the resolution of the carbon budget imbalance is still unclear but has most certainly not yet been reached given the possibilities for improvements that lie ahead.
Estimates of global fossil CO2 emissions from different data sets are in relatively good agreement when the different system boundaries of these data sets are considered (Andrew, 2020a). But while estimates of EFOS are derived from reported activity data requiring far fewer complex transformations than some other components of the budget, uncertainties remain, and one reason for the apparently low variation between data sets is precisely the reliance on the same underlying reported energy data. The budget excludes some sources of fossil CO2 emissions, which available evidence suggests are relatively small (<1 %). We have added emissions from lime production in China and the USA, but these are still absent in most other non-Annex I countries and before 1990 in other Annex I countries.
Estimates of ELUC suffer from a range of intertwined issues, including the poor quality of historical land-cover and land-use change maps, the rudimentary representation of management processes in most models, and the confusion in methodologies and boundary conditions used across methods (e.g. Arneth et al., 2017; Pongratz et al., 2014; see also Sect. S.6.4 on the loss of sink capacity; Bastos et al., 2021). Uncertainties in current and historical carbon stocks in soils and vegetation also add uncertainty into the ELUC estimates. Unless a major effort to resolve these issues is made, little progress is expected in the resolution of ELUC. This is particularly concerning given the growing importance of ELUC for climate mitigation strategies and the large issues in the quantification of the cumulative emissions over the historical period that arise from large uncertainties in ELUC.
By adding the DGVM estimates of CO2 fluxes due to environmental change from countries' managed forest areas (part of SLAND in this budget) to the budget ELUC estimate, we successfully reconciled the large gap between our ELUC estimate and the land-use flux from NGHGIs using the approach described in Grassi et al. (2021) for future scenarios and in Grassi et al. (2023) with data from the Global Carbon Budget 2021. The updated data presented here can be used for potential adjustments in the policy context, e.g. to help assess the collective countries' progress towards the goal of the Paris Agreement and to avoid double accounting for the sink in managed forests. In the absence of this adjustment, collective progress would hence appear better than it is (Grassi et al., 2021). The application of this adjustment is also recommended in the UNFCCC synthesis report for the first Global Stocktake (UNFCCC, 2022) whenever a comparison between LULUCF fluxes reported by countries and the global emission estimates of the IPCC is conducted. However, this adjustment should be seen as a short-term and pragmatic fix based on existing data, rather than a definitive solution to bridge the differences between global models and national inventories. Additional steps are needed to understand and reconcile the remaining differences, some of which are relevant at the country level (Grassi et al., 2023; Schwingshackl et al., 2022).
The comparison of GOBMs, fCO2 products, and inversions highlights a substantial discrepancy in the temporal evolution of SOCEAN in the Southern Ocean and northern high latitudes (Fig. 13; Hauck et al., 2023) and in the mean SOCEAN in the tropics. A large part of the uncertainty in the mean fluxes stems from the regional distribution of the river flux adjustment term. The current distribution simulates the largest share of the outgassing occurring in the tropics (Lacroix et al., 2020) in contrast to the regional distribution previously used with the largest riverine outgassing flux south of 20∘ S (Aumont et al., 2001). The long-standing sparse data coverage of fCO2 observations in the Southern Hemisphere compared to the Northern Hemisphere (e.g. Takahashi et al., 2009) continues to exist (Bakker et al., 2016, 2023, Fig. S1) and to lead to substantially higher uncertainty in the SOCEAN estimate for the Southern Hemisphere (Watson et al., 2020; Gloege et al., 2021; Hauck et al., 2023). This discrepancy, which also hampers model improvement, points to the need for increased high-quality fCO2 observations especially in the Southern Ocean. At the same time, model uncertainty is illustrated by the large spread of individual GOBM estimates (indicated by shading in Fig. 13) and highlights the need for model improvement. The diverging trends in SOCEAN from different methods are a matter of concern. Recent and ongoing work suggests that the fCO2 products may overestimate the trend (Hauck et al., 2023), though many products remain to be tested, whereas evidence is accumulating that GOBMs likely underestimate the mean flux (Sect. 3.6.2; Terhaar et al., 2022; DeVries et al., 2023; Müller et al., 2023). The independent constraint from atmospheric oxygen measurements is consistent within errors with the relatively large ocean sink in the fCO2 products. The assessment of the net land–atmosphere exchange from DGVMs and atmospheric inversions also shows substantial discrepancy, particularly for the estimate of the net land flux over the northern extra-tropics. This discrepancy highlights the difficulty of quantifying complex processes (CO2 fertilization, nitrogen deposition and fertilizers, climate change and variability, land management, etc.) that collectively determine the net land CO2 flux. Resolving the differences in the Northern Hemisphere land sink will require the consideration and inclusion of larger volumes of observations.
We provide metrics for the evaluation of the ocean and land models and the atmospheric inversions (Figs. S2 to S4, Table S10). These metrics expand the use of observations in the global carbon budget, helping (1) to support improvements in the ocean and land carbon models that produce the sink estimates and (2) to constrain the representation of key underlying processes in the models and to allocate the regional partitioning of the CO2 fluxes. The introduction of process-based metrics targeted to evaluate the simulation of SOCEAN in the ocean biogeochemistry models is an important addition to the evaluation based on ocean carbon observations. This is an initial step towards the introduction of a broader range of observations and more stringent model evaluation that we hope will support continued improvements in the annual estimates of the global carbon budget.
We have previously established that a sustained decrease of −1 % in global emissions could be detected at the 66 % likelihood level after a decade only (Peters et al., 2017). Similarly, a change in behaviour of the land and/or ocean carbon sink would take as long to detect and much longer if it emerges more slowly. To continue reducing the carbon imbalance on annual to decadal timescales, regionalizing the carbon budget and integrating multiple variables are powerful ways to shorten the detection limit and ensure the research community can rapidly identify issues of concern in the evolution of the global carbon cycle under the current rapid and unprecedented changing environmental conditions.
The data presented here are made available in the belief that their wide dissemination will lead to greater understanding of and new scientific insights into how the carbon cycle works, how humans are altering it, and how we can mitigate the resulting human-driven climate change. Full contact details and information on how to cite the data shown here are given at the top of each page in the accompanying database and are summarized in Table 2.
The accompanying database includes three Excel files organized in the following spreadsheets.
The file Global_Carbon_Budget_2023v1.0.xlsx includes the following:
-
a summary
-
the global carbon budget (1959–2022),
-
the historical global carbon budget (1750–2022),
-
global CO2 emissions from fossil fuels and cement production by fuel type and the per capita emissions (1850–2022),
-
CO2 emissions from land-use change from the individual bookkeeping models (1959–2022);
-
the ocean CO2 sink from the individual global ocean biogeochemistry models and fCO2 products (1959–2022),
-
the terrestrial CO2 sink from the individual DGVMs (1959–2022),
-
the cement carbonation CO2 sink (1959–2022).
The file National_Fossil_Carbon_Emissions_2023v1.0.xlsx includes the following:
-
a summary,
-
territorial country CO2 emissions from fossil fuels and cement production (1850–2022),
-
consumption country CO2 emissions from fossil fuels and cement production and emissions transfer from the international trade of goods and services (1990–2020) using CDIAC/UNFCCC data as reference,
-
emissions transfers (consumption minus territorial emissions, 1990–2020),
-
country definitions.
The file National_LandUseChange_Carbon_Emissions_2023v1.0.xlsx includes the following:
-
a summary
-
territorial country CO2 emissions from land use change (1850–2022) from three bookkeeping models.
All three spreadsheets are published by the Integrated Carbon Observation System (ICOS) Carbon Portal and are available at https://doi.org/10.18160/GCP-2023 (Friedlingstein et al., 2023). National emissions data are also available on Zenodo (Andrew and Peters, 2022, https://doi.org/10.5281/zenodo.7215364), from the Global Carbon Atlas (http://www.globalcarbonatlas.org/, last access: 9 November 2023, Global Carbon Project, 2023), and from Our World in Data (https://ourworldindata.org/co2-emissions, last access: 9 November 2023, Our World in Data, 2023).
The estimation of global CO2 emissions and sinks is a major effort by the carbon cycle research community that requires a careful compilation and synthesis of measurements, statistical estimates, and model results. The delivery of an annual carbon budget serves two purposes. First, there is a large demand for up-to-date information on the state of the anthropogenic perturbation of the climate system and its underpinning causes. A broad stakeholder community relies on the data sets associated with the annual carbon budget including scientists, policy-makers, businesses, journalists, and non-governmental organizations engaged in adapting to and mitigating human-driven climate change. Second, over the last few decades we have seen unprecedented changes in human and biophysical environments (e.g. changes in the growth of fossil fuel emissions, impact of COVID-19 pandemic, Earth's warming, and strength of the carbon sinks), which call for frequent assessments of the state of the planet, a better quantification of the causes of changes in the contemporary global carbon cycle, and an improved capacity to anticipate its evolution in the future. Building scientific understanding to meet this extraordinary climate mitigation challenge requires frequent, robust, transparent, and traceable data sets and methods that can be scrutinized and replicated. This paper via living data helps to keep track of new budget updates.
The supplement related to this article is available online at: https://doi.org/10.5194/essd-15-5301-2023-supplement.
PF, MO'S, MWJ, RMA, DCEB, JH, PL, CLQ, ITL, GPP, WP, JP, CSc, and SSi designed the study, conducted the analysis, and wrote the paper with input from JGC, PCi, and RBJ. RMA, GPP, and JIK produced the fossil CO2 emissions and their uncertainties and analysed the emissions data. MH and GMa provided fossil fuel emissions data. JP, TGa, CSc, and RAH provided the bookkeeping land-use change emissions with synthesis by JP and CSc. FJo provided peat drainage emission estimates. SSm and CMP provided the estimates of non-vegetation CDR fluxes. LBo, MCh, ÖG, NG, TI, TJ, LR, JS, RS, and HiT provided an update of the global ocean biogeochemical models, and TTTC, DF, LG, YI, AJ, GMc, ChR, and JZ provided an update of the ocean fCO2 data products, with synthesis on both streams by JH, PL, and NMa. SRA, LBa, NRB, MB, MCr, KE, WE, RAF, TGk, AK, NL, DRM, SN, AO, AMO, TO, MEP, DP, KP, GR, AJS, CSw, ST, BT, EvO, RW, and CWR provided ocean fCO2 measurements for the year 2022, with synthesis by DCEB and KMO. PA, DB, SF, JG, HJ, AKJ, EK, DK, JK, GMe, LM, PM, MO, BP, TLS, QS, HTi, WY, XYua, XYue, and SZ provided an update of the dynamic global vegetation models, with synthesis by SSi and MO. HL, RSA, MW, and PCa provided estimates of land and ocean sinks from Earth system models, as well as a projection of the atmospheric growth rate for 2023. FC, ITL, NC, LF, ARJ, FJi, JL, ZJin, ZLiu, YN, CR, DY, and BZ provided an updated atmospheric inversion. WP, FC, and ITL developed the protocol and produced the synthesis and evaluation of the atmospheric inversions. RMA provided projections of the 2023 fossil emissions and atmospheric CO2 growth rate. PL provided the predictions of the 2023 ocean and land sinks. IBMB, LPC, GCH, KKG, TMR, and GRvdW provided forcing data for land-use change. FT and GG provided data for the land-use change NGHGI harmonization. RK provided key atmospheric CO2 data. EJM and RFK provided the atmospheric oxygen constraint on surface net carbon sinks. XL, PPT, and MWJ provided the historical atmospheric CO2 concentration and growth rate. MO and NB produced the aerosol diffuse radiative forcing for the DGVMs. IH provided the climate forcing data for the DGVMs. ER provided the evaluation of the DGVMs. MWJ provided the emission prior for use in the inversion systems. XD provided seasonal emissions data for the most recent years for the emission prior. PF, MO, and MWJ coordinated the effort and revised all figures, tables, text, and numbers to ensure the update was clear from the 2022 edition and in line with https://globalcarbonatlas.org/ (last access: 9 November 2023).
At least one of the (co-)authors is a member of the editorial board of Earth System Science Data. The peer-review process was guided by an independent editor, and the authors also have no other competing interests to declare.
Publisher's note: Copernicus Publications remains neutral with regard to jurisdictional claims made in the text, published maps, institutional affiliations, or any other geographical representation in this paper. While Copernicus Publications makes every effort to include appropriate place names, the final responsibility lies with the authors.
We thank all people and institutions who provided the data used in this Global Carbon Budget 2023 and the Global Carbon Project members for their input throughout the development of this publication. We thank Nigel Hawtin for producing Figs. 2 and 15. We thank Alex Vermeulen and Hanna Ritchie for hosting the global carbon budget data sets on the ICOS portal and the Our World in Data website, respectively. We thank Ian G. C. Ashton, Sebastian Brune, Fatemeh Cheginig, Sam Ditkovsky, Christian Ethé, Amanda R. Fay, Lonneke Goddijn-Murphy, T. Holding, Yawen Kong, Fabrice Lacroix, Yi Liu, Damian Loher, Naiqing Pan, Paridhi Rustogi, Shijie Shu, Jamie Shutler, Richard Sims, Phillip Townsend, Jing Wang, Andrew J. Watson, and David K. Woolf for their involvement in the development, use, and analysis of the models and data products used here. We thank Toste Tanhua, Marcos Fontela, Claire Lo Monaco, and Nicolas Metzl, who contributed to the provision of surface ocean CO2 observations for the year 2022 (see Table S6). We also thank Stephen D. Jones of the Ocean Thematic Centre of the EU Integrated Carbon Observation System (ICOS) Research Infrastructure, Eugene Burger of NOAA's Pacific Marine Environmental Laboratory, and Alex Kozyr of NOAA's National Centers for Environmental Information for their contribution to surface ocean CO2 data and metadata management. This is PMEL contribution 5550. We thank the scientists, institutions, and funding agencies responsible for the collection and quality control of the data in SOCAT as well as the International Ocean Carbon Coordination Project (IOCCP), the Surface Ocean–Lower Atmosphere Study (SOLAS), and the Integrated Marine Biosphere Research (IMBeR) programme for their support. We thank Nadine Goris and Lavinia Patara for support in calculating observational ocean evaluation metrics. We thank Fortunat Joos, Samar Khatiwala, and Timothy DeVries for providing historical atmospheric and ocean data. We thank data providers ObsPack GLOBALVIEWplus v8.0 and NRT v8.1 for atmospheric CO2 observations. Ingrid T. Luijkx and Wouter Peters thank the CarbonTracker Europe team at Wageningen University, including Remco de Kok, Joram Hooghiem, Linda Kooijmans, and Auke van der Woude. Daniel Kennedy thanks all the scientists, software engineers, and administrators who contributed to the development of CESM2. Josefine Ghattas thanks the whole ORCHIDEE group. Ian Harris thanks the Japan Meteorological Agency (JMA) for producing the Japanese 55-year Reanalysis (JRA-55). Reinel Sospedra-Alfonso thanks Barbara Winter, Woosung Lee, and William J. Merryfield for their contribution to the preparation and production of CanESM5 runs. Patricia Cadule thanks Olivier Torres, Juliette Mignot, Didier Swingedouw, and Laurent Bopp for contributions to the IPSL-CM6A-CO2-LR simulations. Yosuke Niwa thanks CSIRO, EC, Empa, FMI, IPEN, JMA, LSCE, NCAR, NIES, NILU, NIWA, NOAA, SIO, and TU/NIPR for providing data for NISMON-CO2. Zhe Jin thanks Xiangjun Tian, Yilong Wang, Hongqin Zhang, Min Zhao, Tao Wang, Jinzhi Ding and Shilong Piao for their contributions to the GONGGA inversion system. Bo Zheng thanks Yawen Kong for running the THU inversion system. Frédéric Chevallier thanks Zoé Lloret, who maintained the atmospheric transport model for the CAMS inversions. Frédéric Chevallier and Thi-Tuyet-Trang Chau thank Marion Gehlen for her contribution to the CMEMS-LSCE-FFNNv2 product. Lian Fang thanks Paul I. Palmer and acknowledges ongoing support from the National Centre for Earth Observation. Junjie Liu thanks the Jet Propulsion Laboratory, California Institute of Technology. Zhiqiang Liu thanks Ning Zeng, Yun Liu, Eugenia Kalnay, and Gassem Asrar for their contributions to the COLA system. Fei Jiang acknowledges ongoing support from the Frontiers Science Center for Critical Earth Material Cycling, Nanjing University. Andy Jacobson thanks the team at NOAA GML, Boulder, Colorado, USA, who provided the CarbonTracker CT2022 and CT-NRT.v2023-3 results from the website at http://carbontracker.noaa.gov (last access: 9 November 2023). Meike Becker and Are Olsen thank Sparebanken Vest/Agenda Vestlandet for their support for the observations on the Statsraad Lehmkuhl. Margot Cronin thanks Anthony English, Clynt Gregory, and Gordon Furey (P&O Maritime Services) and Tobias Steinhoff and Aodhan Fitzgerald (Marine Institute) for their support. Wiley Evans and Katie Pocock thank the Tula Foundation for funding support. Thanos Gkritzalis and the VLIZ ICOS team are thankful to the crew of the research vessel Simon Stevin for all the support and help they provide. Data providers Nicolas Metzl and Claire LoMonaco thank the French Institut National des Sciences de l'Univers (INSU), Institut Polaire français Paul-Émile Victor (IPEV), Observatoire des sciences de l'univers Ecce Terra (OSU at Sorbonne Université), Institut Français de Recherche pour l'Exploitation de la Mer (IFREMER), and French Oceanographic Fleet (FOF) for the Marion Dufresne data set (https://doi.org/10.17600/18001858, Lo Monaco and Metzl, 2022). Bronte Tilbrook and Erik van Ooijen thank Australia's Integrated Marine Observing System (IMOS) for sourcing of CO2 data. IMOS is enabled by the National Collaborative Research Infrastructure Strategy (NCRIS). FAOSTAT is funded by FAO member states through their contributions to the FAO Regular Programme; data contributions by national experts are greatly acknowledged. The views expressed in this paper are the authors' only and do not necessarily reflect those of FAO. Finally, we thank all funders who have supported the individual and joint contributions to this work (see details below), as well as the two anonymous reviewers of this paper and the many researchers who have provided feedback.
This research was supported by the following sources of funding: the Integrated Marine Observing System (IMOS) (Australia); ICOS Flanders (Belgium); the Research Foundation – Flanders (grant no. I001821N) (Belgium); the Tula Foundation (Canada); the Chinese Academy of Science Project for Young Scientists in Basic Research (grant no. YSBR-037) (China); the National Key R&D Program of China (grant no. 2020YFA0607504); the National Natural Science Foundation (grant nos. 42141020, 42275128, 42275128, 41921005) (China); Scientific Research Start-up Funds (grant no. QD2021024C) from Tsinghua Shenzhen International Graduate School (China); the Second Tibetan Plateau Scientific Expedition and Research Program (grant no. 2022QZKK0101) (China); the Young Elite Scientists Sponsorship Program by CAST (grant no. YESS20200135) (China); Fundamental Research Funds for the Central Universities (grant no. 090414380031) (China); the Copernicus Atmosphere Monitoring Service, implemented by ECMWF (grant no. CAMS2 55) (European Commission, EC); Copernicus Marine Environment Monitoring Service, implemented by MOi (grant no. CAMS2 55) (EC); H2020 (Horizon 2020) 4C (grant no. 821003) (EC); H2020 ESM2025 – Earth System Models for the Future (grant no. 101003536) (EC); H2020 EuroSea (grant no. 862626) (EC); H2020 GEORGE (grant no. 101094716) (EC); H2020 JERICO-S3 (grant no. 871153) (EC); ICOS France (France); the Institut de Recherche pour le Développement (IRD) (France); the Federal Ministry of Education and Research (BMBF) (grant no. 03F0885AL1) (Germany); the Federal Ministry of Education and Research, collaborative project C-SCOPE (Towards Marine Carbon Observations 2.0: Socializing, COnnecting, Perfecting and Expanding, project no. 03F0877A) (Germany); the Helmholtz Association ATMO programme (Germany); the Helmholtz Association of German Research Centres (project MOSES; Modular Observation Solutions for Earth Systems) (Germany); ICOS (Integrated Carbon Observation System) Germany (Germany); the Ludwig Maximilians University of Munich, Department of Geography (Germany); the Marine Institute (Ireland); the Arctic Challenge for Sustainability phase II project (ArCS II; grant no. JP-MXD1420318865) (Japan); the Environment Research and Technology Development Fund (grant no. JP-MEERF21S20800) (Japan); the Global Environmental Research Coordination System, Ministry of the Environment (grant no. E2252) (Japan); the Japan Meteorological Agency (Japan); the Ministry of Education, Culture, Sports, Science and Technology, MEXT Program for the Advanced Studies of Climate Change Projection (SENTAN) (grant nos. JPMXD0722680395, JPMXD0722681344) (Japan); the Ministry of Environment, Environmental Restoration and Conservation Agency, Environment Research and Technology Development Fund (grant no. JPMEERF21S20810) (Japan); the National Institute for Environmental Studies (Japan); the Research Council of Norway (N-ICOS-2; grant no. 296012) (Norway); the Swiss National Science Foundation (grant no. 200020-200511) (Switzerland); the National Centre for Atmospheric Science (UK); NERC (Natural Environment Research Council) Independent Research Fellowship (NE/V01417X/1) (UK); NERC (NE/R016518/1) (UK); the Royal Society (grant no. RP\R1\191063) (UK); UK Research and Innovation (UKRI) for Horizon Europe (GreenFeedBack; grant no. 10040851) (UK); the NASA Carbon Monitoring System programme (80NSSC21K1059) (USA); the NASA OCO Science Team programme (grant no. 80NM0018F0583) (USA); a National Center for Atmospheric Research (NCAR) cooperative agreement (NSF no. 1852977) (USA); a National Oceanic and Atmospheric Administration (NOAA) cooperative agreement (grant no. NA22OAR4320151) (USA); the National Science Foundation (grant nos. NSF-831361857, OPP-1922922) (USA); NOAA (grant no. NA20OAR4320278) (USA); a NOAA cooperative agreement, the Cooperative Institute for Climate, Ocean, & Ecosystem Studies (CIOCES; grant no. NA20OAR4320271) (USA); a NOAA cooperative agreement, the Cooperative Institute for Marine and Atmospheric Studies (CIMAS), University of Miami (grant no. NA20OAR4320472) (USA); the NOAA Global Ocean Monitoring and Observing Program (grant nos. 100018302, 100007298, NA-03-AR4320179) (USA); and the NOAA Ocean Acidification Program (USA). We also acknowledge support from the following computing facilities: the Adapter Allocation Scheme from the National Computational Infrastructure (NCI) (Australia); the High-Performance Computing Center (HPCC) of Nanjing University (China); GENCI-TGCC (grant nos. A0130102201, A0130106328, A0140107732, A0130107403) (France); CCRT awarded by CEA DRF (grant no. CCRT2023-p24cheva) (France); the HPC cluster Aether at the University of Bremen, financed by DFG within the scope of the Excellence Initiative (Germany); the state of Baden-Württemberg, through bwHPC (Germany); Earth Simulator (ES4) at JAMSTEC (Japan); JAMSTEC's supercomputer system (Japan); the NIES supercomputer system (Japan); NIES (SX-Aurora) and MRI (Fujitsu server PRIMERGY CX2550M5) (Japan); the ADA HPC cluster at the University of East Anglia (UK); the UK CEDA JASMIN supercomputer (UK); and Cheyenne NCAR HPC resources managed by CISL (https://doi.org/10.5065/D6RX99HX, Hart, 2021) (USA).
This paper was edited by Kirsten Elger and reviewed by two anonymous referees.
Abatzoglou, J. T., Williams, A. P., and Barbero, R.: Global Emergence of Anthropogenic Climate Change in Fire Weather Indices, Geophys. Res. Lett., 46, 326–336, https://doi.org/10.1029/2018GL080959, 2019.
Ahlström, A., Raupach, M. R., Schurgers, G., Smith, B., Arneth, A., Jung, M., Reichstein, M., Canadell, J. G., Friedlingstein, P., Jain, A. K., Kato, E., Poulter, B., Sitch, S., Stocker, B. D., Viovy, N., Wang, Y. P., Wiltshire, A., Zaehle, S., and Zeng, N.: The dominant role of semi-arid ecosystems in the trend and variability of the land CO2 sink, 348, 895–899, https://doi.org/10.1126/science.aaa1668, 2015.
Andela, N., Morton, D. C., Giglio, L., Chen, Y., van der Werf, G. R., Kasibhatla, P. S., DeFries, R. S., Collatz, G. J., Hantson, S., Kloster, S., Bachelet, D., Forrest, M., Lasslop, G., Li, F., Mangeon, S., Melton, J. R., Yue, C., and Randerson, J. T.: A human-driven decline in global burned area, Science, 356, 1356–1362, https://doi.org/10.1126/science.aal4108, 2017.
Andrew, R. M.: A comparison of estimates of global carbon dioxide emissions from fossil carbon sources, Earth Syst. Sci. Data, 12, 1437–1465, https://doi.org/10.5194/essd-12-1437-2020, 2020a.
Andrew, R. M.: Timely estimates of India's annual and monthly fossil CO2 emissions, Earth Syst. Sci. Data, 12, 2411–2421, https://doi.org/10.5194/essd-12-2411-2020, 2020b.
Andrew, R. M. and Peters, G. P.: The Global Carbon Project's fossil CO2 emissions dataset (2022v27), Zenodo [data set], https://doi.org/10.5281/zenodo.7215364, 2022.
Angelsen, A. and Kaimowitz, D.: Rethinking the Causes of Deforestation: Lessons from Economic Models, World Bank Res. Obs., 14, 73–98, https://doi.org/10.1093/wbro/14.1.73, 1999.
Aragão, L. E. O. C., Anderson, L. O., Fonseca, M. G., Rosan, T. M., Vedovato, L. B., Wagner, F. H., Silva, C. V. J., Silva Junior, C. H. L., Arai, E., Aguiar, A. P., Barlow, J., Berenguer, E., Deeter, M. N., Domingues, L. G., Gatti, L., Gloor, M., Malhi, Y., Marengo, J. A., Miller, J. B., Phillips, O. L., and Saatchi, S.: 21st Century drought-related fires counteract the decline of Amazon deforestation carbon emissions, Nat. Commun., 9, 536, https://doi.org/10.1038/s41467-017-02771-y, 2018.
Archer, D., Eby, M., Brovkin, V., Ridgwell, A., Cao, L., Mikolajewicz, U., Caldeira, K., Matsumoto, K., Munhoven, G., Montenegro, A., and Tokos, K.: Atmospheric Lifetime of Fossil Fuel Carbon Dioxide, Annu. Rev. Earth Planet. Sci., 37, 117–134, https://doi.org/10.1146/annurev.earth.031208.100206, 2009.
Arneth, A., Sitch, S., Pongratz, J., Stocker, B. D., Ciais, P., Poulter, B., Bayer, A. D., Bondeau, A., Calle, L., Chini, L. P., Gasser, T., Fader, M., Friedlingstein, P., Kato, E., Li, W., Lindeskog, M., Nabel, J. E. M. S., Pugh, T. A. M., Robertson, E., Viovy, N., Yue, C., and Zaehle, S.: Historical carbon dioxide emissions caused by land-use changes are possibly larger than assumed, Nat. Geosci., 10, 79–84, https://doi.org/10.1038/ngeo2882, 2017.
Asaadi, A., Arora, V. K., Melton, J. R., and Bartlett, P.: An improved parameterization of leaf area index (LAI) seasonality in the Canadian Land Surface Scheme (CLASS) and Canadian Terrestrial Ecosystem Model (CTEM) modelling framework, Biogeosciences, 15, 6885–6907, https://doi.org/10.5194/bg-15-6885-2018, 2018.
Aumont, O., Orr, J. C., Monfray, P., Ludwig, W., Amiotte-Suchet, P., and Probst, J.-L.: Riverine-driven interhemispheric transport of carbon, Global Biogeochem. Cycles, 15, 393–405, https://doi.org/10.1029/1999GB001238, 2001.
Aumont, O., Ethé, C., Tagliabue, A., Bopp, L., and Gehlen, M.: PISCES-v2: an ocean biogeochemical model for carbon and ecosystem studies, Geosci. Model Dev., 8, 2465–2513, https://doi.org/10.5194/gmd-8-2465-2015, 2015.
Baccini, A., Walker, W., Carvalho, L., Farina, M., Sulla-Menashe, D., and Houghton, R. A.: Tropical forests are a net carbon source based on aboveground measurements of gain and loss, Science, 358, 230–234, https://doi.org/10.1126/science.aam5962, 2017.
Bakker, D. C. E., Pfeil, B., Landa, C. S., Metzl, N., O'Brien, K. M., Olsen, A., Smith, K., Cosca, C., Harasawa, S., Jones, S. D., Nakaoka, S., Nojiri, Y., Schuster, U., Steinhoff, T., Sweeney, C., Takahashi, T., Tilbrook, B., Wada, C., Wanninkhof, R., Alin, S. R., Balestrini, C. F., Barbero, L., Bates, N. R., Bianchi, A. A., Bonou, F., Boutin, J., Bozec, Y., Burger, E. F., Cai, W.-J., Castle, R. D., Chen, L., Chierici, M., Currie, K., Evans, W., Featherstone, C., Feely, R. A., Fransson, A., Goyet, C., Greenwood, N., Gregor, L., Hankin, S., Hardman-Mountford, N. J., Harlay, J., Hauck, J., Hoppema, M., Humphreys, M. P., Hunt, C. W., Huss, B., Ibánhez, J. S. P., Johannessen, T., Keeling, R., Kitidis, V., Körtzinger, A., Kozyr, A., Krasakopoulou, E., Kuwata, A., Landschützer, P., Lauvset, S. K., Lefèvre, N., Lo Monaco, C., Manke, A., Mathis, J. T., Merlivat, L., Millero, F. J., Monteiro, P. M. S., Munro, D. R., Murata, A., Newberger, T., Omar, A. M., Ono, T., Paterson, K., Pearce, D., Pierrot, D., Robbins, L. L., Saito, S., Salisbury, J., Schlitzer, R., Schneider, B., Schweitzer, R., Sieger, R., Skjelvan, I., Sullivan, K. F., Sutherland, S. C., Sutton, A. J., Tadokoro, K., Telszewski, M., Tuma, M., van Heuven, S. M. A. C., Vandemark, D., Ward, B., Watson, A. J., and Xu, S.: A multi-decade record of high-quality fCO2 data in version 3 of the Surface Ocean CO2 Atlas (SOCAT), Earth Syst. Sci. Data, 8, 383–413, https://doi.org/10.5194/essd-8-383-2016, 2016.
Bakker, D. C. E., Alin, S. R., Bates, N., Becker, M., Feely, R. A., Gkritzalis, T., Jones, S. D., Kozyr, A., Lauvset, S, K., Metzl, N., Munro, D. R., Nakaoka, S., Nojiri, Y., O'Brien, K. M., Olsen, A., Pierrot, D., Rehder, G., Steinhoff, T., Sutton, A. J., Sweeney, C., Tilbrook, B., Wada, C., Wanninkhof, R., Akl, J., Barbero, L., Beatty, C. M., Berghoff, C. F., Bittig, H. C., Bott, R., Burger, E. F., Cai, W.-J., Castaño-Primo, R., Corredor, J. E., Cronin, M., De Carlo, E. H., DeGrandpre, M. D., Dietrich, C., Drennan, W. M., Emerson, S. R., Enochs, I. C., Enyo, K., Epherra, L., Evans, W., Fiedler, B., Fontela, M., Frangoulis, C., Gehrung, M., Giannoudi, L., Glockzin, M., Hales, B., Howden, S. D., Ibánhez, J., Severino, P., Kamb, L., Körtzinger, A., Lefèvre, N., Lo Monaco, C., Lutz, V. A., Macovei, V. A., Maenner Jones, S., Manalang, D., Manzello, D. P., Metzl, N., Mickett, J., Millero, F. J., Monacci, N. M., Morell, J. M., Musielewicz, S., Neill, C., Newberger, T., Newton, J., Noakes, S., Ólafsdóttir, S. R., Ono, T., Osborne, J., Padín, X. A., Paulsen, M., Perivoliotis, L., Petersen, W., Petihakis, G., Plueddemann, A. J., Rodriguez, C., Rutgersson, A., Sabine, C. L., Salisbury, J. E., Schlitzer, R., Skjelvan, I., Stamataki, N., Sullivan, K. F., Sutherland, S. C., T'Jampens, M., Tadokoro, K., Tanhua, T., Telszewski, M., Theetaert, H., Tomlinson, M., Vandemark, D., Velo, A., Voynova, Y. G., Weller, R. A., and Whitehead, C., Wimart-Rousseau, C.: Surface Ocean CO2 Atlas Database Version 2023 (SOCATv2023) (NCEI Accession 0278913), NOAA National Centers for Environmental Information [data set], https://doi.org/10.25921/r7xa-bt92, 2023.
Ballantyne, A. P., Alden, C. B., Miller, J. B., Tans, P. P., and White, J. W. C.: Increase in observed net carbon dioxide uptake by land and oceans during the past 50 years, Nature, 488, 70–72, https://doi.org/10.1038/nature11299, 2012.
Ballantyne, A. P., Andres, R., Houghton, R., Stocker, B. D., Wanninkhof, R., Anderegg, W., Cooper, L. A., DeGrandpre, M., Tans, P. P., Miller, J. B., Alden, C., and White, J. W. C.: Audit of the global carbon budget: estimate errors and their impact on uptake uncertainty, Biogeosciences, 12, 2565–2584, https://doi.org/10.5194/bg-12-2565-2015, 2015.
Bastos, A., Hartung, K., Nützel, T. B., Nabel, J. E. M. S., Houghton, R. A., and Pongratz, J.: Comparison of uncertainties in land-use change fluxes from bookkeeping model parameterisation, Earth Syst. Dynam., 12, 745–762, https://doi.org/10.5194/esd-12-745-2021, 2021.
Battle, M. O., Raynor, R., Kesler, S., and Keeling, R.: Technical Note: The impact of industrial activity on the amount of atmospheric O2, Atmos. Chem. Phys. Discuss. [preprint], https://doi.org/10.5194/acp-2022-765, 2023.
Bellenger, H., Bopp, L., Ethé, C., Ho, D., Duvel, J. P., Flavoni, S., Guez, L., Kataoka, T., Perrot, X., Parc, L., and Watanabe, M.: Sensitivity of the Global Ocean Carbon Sink to the Ocean Skin in a Climate Model, J. Geophys. Res.-Oceans, 128, e2022JC019479, https://doi.org/10.1029/2022JC019479, 2023.
Bennington, V., Gloege, L., and McKinley, G. A.: Variability in the Global Ocean Carbon Sink From 1959 to 2020 by Correcting Models with Observations, Geophys. Res. Lett., 49, e2022GL098632, https://doi.org/10.1029/2022GL098632, 2022.
Berthet, S., Séférian, R., Bricaud, C., Chevallier, M., Voldoire, A., and Ethé, C.: Evaluation of an Online Grid-Coarsening Algorithm in a Global Eddy-Admitting Ocean Biogeochemical Model, J. Adv. Model Earth Sy., 11, 1759–1783, https://doi.org/10.1029/2019MS001644, 2019.
Betts, R. A., Jones, C. D., Knight, J. R., Keeling, R. F., and Kennedy, J. J.: El Niño and a record CO2 rise, Nat. Clim. Change, 6, 806–810, https://doi.org/10.1038/nclimate3063, 2016.
Bloom, A. A. and Williams, M.: Constraining ecosystem carbon dynamics in a data-limited world: integrating ecological “common sense” in a model–data fusion framework, Biogeosciences, 12, 1299–1315, https://doi.org/10.5194/bg-12-1299-2015, 2015.
Bloom, A. A., Exbrayat, J.-F., van der Velde, I. R., Feng, L., and Williams, M.: The decadal state of the terrestrial carbon cycle: Global retrievals of terrestrial carbon allocation, pools, and residence times, P. Natl. Acad. Sci. USA, 113, 1285–1290, https://doi.org/10.1073/pnas.1515160113, 2016.
Boer, G. J., Smith, D. M., Cassou, C., Doblas-Reyes, F., Danabasoglu, G., Kirtman, B., Kushnir, Y., Kimoto, M., Meehl, G. A., Msadek, R., Mueller, W. A., Taylor, K. E., Zwiers, F., Rixen, M., Ruprich-Robert, Y., and Eade, R.: The Decadal Climate Prediction Project (DCPP) contribution to CMIP6, Geosci. Model Dev., 9, 3751–3777, https://doi.org/10.5194/gmd-9-3751-2016, 2016.
Boucher, O., Servonnat, J., Albright, A. L., Aumont, O., Balkanski, Y., Bastrikov, V., Bekki, S., Bonnet, R., Bony, S., Bopp, L., Braconnot, P., Brockmann, P., Cadule, P., Caubel, A., Cheruy, F., Codron, F., Cozic, A., Cugnet, D., D'Andrea, F., Davini, P., de Lavergne, C., Denvil, S., Deshayes, J., Devilliers, M., Ducharne, A., Dufresne, J.-L., Dupont, E., Éthé, C., Fairhead, L., Falletti, L., Flavoni, S., Foujols, M.-A., Gardoll, S., Gastineau, G., Ghattas, J., Grandpeix, J.-Y., Guenet, B., Guez, E., Lionel, Guilyardi, E., Guimberteau, M., Hauglustaine, D., Hourdin, F., Idelkadi, A., Joussaume, S., Kageyama, M., Khodri, M., Krinner, G., Lebas, N., Levavasseur, G., Lévy, C., Li, L., Lott, F., Lurton, T., Luyssaert, S., Madec, G., Madeleine, J.-B., Maignan, F., Marchand, M., Marti, O., Mellul, L., Meurdesoif, Y., Mignot, J., Musat, I., Ottlé, C., Peylin, P., Planton, Y., Polcher, J., Rio, C., Rochetin, N., Rousset, C., Sepulchre, P., Sima, A., Swingedouw, D., Thiéblemont, R., Traore, A. K., Vancoppenolle, M., Vial, J., Vialard, J., Viovy, N., and Vuichard, N.: Presentation and Evaluation of the IPSL-CM6A-LR Climate Model, J. Adv. Model. Earth Sy., 12, e2019MS002010, https://doi.org/10.1029/2019MS002010, 2020.
Bourgeois, T., Goris, N., Schwinger, J., and Tjiputra, J. F.: Stratification constrains future heat and carbon uptake in the Southern Ocean between 30∘ S and 55∘ S, Nat. Commun., 13, 340, https://doi.org/10.1038/s41467-022-27979-5, 2022.
Brancalion, P. H. S., Broadbent, E. N., de-Miguel, S., Cardil, A., Rosa, M. R., Almeida, C. T., Almeida, D. R. A., Chakravarty, S., Zhou, M., Gamarra, J. G. P., Liang, J., Crouzeilles, R., Hérault, B., Aragão, L. E. O. C., Silva, C. A., and Almeyda-Zambrano, A. M.: Emerging threats linking tropical deforestation and the COVID-19 pandemic, Persp. Ecol. Conserv., 18, 243–246, https://doi.org/10.1016/j.pecon.2020.09.006, 2020.
Bray, E.: 2017 Minerals Yearbook: Aluminum [Advance Release], Tech. rep., U.S. Geological Survey, https://d9-wret.s3-us-west-2.amazonaws.com/assets/palladium/production/atoms/files/myb1-2017-alumi.pdf (last access: 9 November 2023), 2020.
Brienen, R. J. W., Phillips, O. L., Feldpausch, T. R., Gloor, E., Baker, T. R., Lloyd, J., Lopez-Gonzalez, G., Monteagudo-Mendoza, A., Malhi, Y., Lewis, S. L., Vásquez Martinez, R., Alexiades, M., Álvarez Dávila, E., Alvarez-Loayza, P., Andrade, A., Aragão, L. E. O. C., Araujo-Murakami, A., Arets, E. J. M. M., Arroyo, L., Aymard C., G. A., Bánki, O. S., Baraloto, C., Barroso, J., Bonal, D., Boot, R. G. A., Camargo, J. L. C., Castilho, C. V., Chama, V., Chao, K. J., Chave, J., Comiskey, J. A., Cornejo Valverde, F., da Costa, L., de Oliveira, E. A., Di Fiore, A., Erwin, T. L., Fauset, S., Forsthofer, M., Galbraith, D. R., Grahame, E. S., Groot, N., Hérault, B., Higuchi, N., Honorio Coronado, E. N., Keeling, H., Killeen, T. J., Laurance, W. F., Laurance, S., Licona, J., Magnussen, W. E., Marimon, B. S., Marimon-Junior, B. H., Mendoza, C., Neill, D. A., Nogueira, E. M., Núñez, P., Pallqui Camacho, N. C., Parada, A., Pardo-Molina, G., Peacock, J., Peña-Claros, M., Pickavance, G. C., Pitman, N. C. A., Poorter, L., Prieto, A., Quesada, C. A., Ramírez, F., Ramírez-Angulo, H., Restrepo, Z., Roopsind, A., Rudas, A., Salomão, R. P., Schwarz, M., Silva, N., Silva-Espejo, J. E., Silveira, M., Stropp, J., Talbot, J., ter Steege, H., Teran-Aguilar, J., Terborgh, J., Thomas-Caesar, R., Toledo, M., Torello-Raventos, M., Umetsu, R. K., van der Heijden, G. M. F., van der Hout, P., Guimarães Vieira, I. C., Vieira, S. A., Vilanova, E., Vos, V. A., and Zagt, R. J.: Long-term decline of the Amazon carbon sink, Nature, 519, 344–348, https://doi.org/10.1038/nature14283, 2015.
Brienen, R. J. W., Caldwell, L., Duchesne, L., Voelker, S., Barichivich, J., Baliva, M., Ceccantini, G., Di Filippo, A., Helama, S., Locosselli, G. M., Lopez, L., Piovesan, G., Schöngart, J., Villalba, R., and Gloor, E.: Forest carbon sink neutralized by pervasive growth-lifespan trade-offs, Nat. Commun., 11, 4241, https://doi.org/10.1038/s41467-020-17966-z, 2020.
Bronselaer, B., Winton, M., Russell, J., Sabine, C. L., and Khatiwala, S.: Agreement of CMIP5 Simulated and Observed Ocean Anthropogenic CO2 Uptake, Geophys. Res. Lett., 44, 12298–12305, https://doi.org/10.1002/2017GL074435, 2017.
Bruno, M. and Joos, F.: Terrestrial carbon storage during the past 200 years: A Monte Carlo Analysis of CO2 data from ice core and atmospheric measurements, Global Biogeochem. Cycles, 11, 111–124, https://doi.org/10.1029/96GB03611, 1997.
Burrows, S. M., Maltrud, M., Yang, X., Zhu, Q., Jeffery, N., Shi, X., Ricciuto, D., Wang, S., Bisht, G., Tang, J., Wolfe, J., Harrop, B. E., Singh, B., Brent, L., Baldwin, S., Zhou, T., Cameron-Smith, P., Keen, N., Collier, N., Xu, M., Hunke, E. C., Elliott, S. M., Turner, A. K., Li, H., Wang, H., Golaz, J.-C., Bond-Lamberty, B., Hoffman, F. M., Riley, W. J., Thornton, P. E., Calvin, K., and Leung, L. R.: The DOE E3SM v1.1 Biogeochemistry Configuration: Description and Simulated Ecosystem-Climate Responses to Historical Changes in Forcing, J. Adv. Model. Earth Sy., 12, e2019MS001766, https://doi.org/10.1029/2019MS001766, 2020.
Burton, C., Betts, R., Cardoso, M., Feldpausch, T. R., Harper, A., Jones, C. D., Kelley, D. I., Robertson, E., and Wiltshire, A.: Representation of fire, land-use change and vegetation dynamics in the Joint UK Land Environment Simulator vn4.9 (JULES), Geosci. Model Dev., 12, 179–193, https://doi.org/10.5194/gmd-12-179-2019, 2019.
Burton, C., Lampe, S., Kelley, D., Thiery, W., Hantson, S., Christidis, N., Gudmundsson, L., Forrest, M., Burke, E., Chang, J., Huang, H., Ito, A., Kou-Giesbrecht, S., Lasslop, G., Li, W., Nieradzik, L., Li, F., Chen, Y., Randerson, J., Reyer, C., and Mengel, M.: Global burned area increasingly explained by climate change, Nature Portfolio, in review, https://doi.org/10.21203/rs.3.rs-3168150/v1, 2023.
Bushinsky, S. M., Landschützer, P., Rödenbeck, C., Gray, A. R., Baker, D., Mazloff, M. R., Resplandy, L., Johnson, K. S., and Sarmiento, J. L.: Reassessing Southern Ocean Air-Sea CO2 Flux Estimates With the Addition of Biogeochemical Float Observations, Global Biogeochem. Cycles, 33, 1370–1388, https://doi.org/10.1029/2019GB006176, 2019.
Canadell, J. G., Le Quere, C., Raupach, M. R., Field, C. B., Buitenhuis, E. T., Ciais, P., Conway, T. J., Gillett, N. P., Houghton, R. A., and Marland, G.: Contributions to accelerating atmospheric CO2 growth from economic activity, carbon intensity, and efficiency of natural sinks, P. Natl. Acad. Sci. USA, 104, 18866–18870, https://doi.org/10.1073/pnas.0702737104, 2007.
Canadell, J. G., Monteiro, P. M. S., Costa, M. H., Cotrim da Cunha, L., Cox, P. M., Eliseev, A. V., Henson, S., Ishii, M., Jaccard, S., Koven, C., Lohila, A., Patra, P. K., Piao, S., Rogelj, J., Syampungani, S., Zaehle, S., and Zickfeld, K.: Global Carbon and other Biogeochemical Cycles and Feedbacks. In: Climate Change 2021: The Physical Science Basis, Contribution of Working Group I to the Sixth Assessment Report of the Intergovernmental Panel on Climate Change, edited by: Masson-Delmotte, V., Zhai, P., Pirani, A., Connors, S. L., Péan, C., Berger, S., Caud, N., Chen, Y., Goldfarb, L., Gomis, M. I., Huang, M., Leitzell, K., Lonnoy, E., Matthews, J. B. R., Maycock, T. K., Waterfield, T., Yelekçi, O., Yu, R., and Zhou, B., Cambridge University Press, Cambridge, United Kingdom and New York, NY, USA, 673–816, https://doi.org/10.1017/9781009157896.007, 2021.
Cao, Z., Myers, R. J., Lupton, R. C., Duan, H., Sacchi, R., Zhou, N., Reed Miller, T., Cullen, J. M., Ge, Q., and Liu, G.: The sponge effect and carbon emission mitigation potentials of the global cement cycle, Nat. Commun., 11, 3777, https://doi.org/10.1038/s41467-020-17583-w, 2020.
Céspedes, J., Sylvester, J. M., Pérez-Marulanda, L., Paz-Garcia, P., Reymondin, L., Khodadadi, M., Tello, J. J., and Castro-Nunez, A.: Has global deforestation accelerated due to the COVID-19 pandemic?, J. For. Res., 34, 1153–1165, https://doi.org/10.1007/s11676-022-01561-7, 2023.
Chandra, N., Patra, P. K., Niwa, Y., Ito, A., Iida, Y., Goto, D., Morimoto, S., Kondo, M., Takigawa, M., Hajima, T., and Watanabe, M.: Estimated regional CO2 flux and uncertainty based on an ensemble of atmospheric CO2 inversions, Atmos. Chem. Phys., 22, 9215–9243, https://doi.org/10.5194/acp-22-9215-2022, 2022.
Chau, T. T. T., Gehlen, M., and Chevallier, F.: A seamless ensemble-based reconstruction of surface ocean pCO2 and air–sea CO2 fluxes over the global coastal and open oceans, Biogeosciences, 19, 1087–1109, https://doi.org/10.5194/bg-19-1087-2022, 2022.
Chevallier, F., Fisher, M., Peylin, P., Serrar, S., Bousquet, P., Bréon, F.-M., Chédin, A., and Ciais, P.: Inferring CO2 sources and sinks from satellite observations: Method and application to TOVS data, J. Geophys. Res., 110, D24309, https://doi.org/10.1029/2005JD006390, 2005.
Ciais, P., Sabine, C., Bala, G., Bopp, L., Brovkin, V., Canadell, J. G., Chhabra, A., DeFries, R., Galloway, J., Heimann, M., Jones, C., Le Quéré, C., Myneni, R., Piao, S., Thornton, P., Willem, J., Friedlingstein, P., and Munhoven, G.: Carbon and Other Biogeochemical Cycles, in Climate Change 2013: The Physical Science Basis, Contribution of Working Group I to the Fifth Assessment Report of the Intergovernmental Panel on Climate Change, edited by: Intergovernmental Panel on Climate Change, Cambridge University Press, Cambridge, United Kingdom and New York, NY, USA, https://doi.org/10.1017/CBO9781107415324.015, 2013.
Ciais, P., Tan, J., Wang, X., Roedenbeck, C., Chevallier, F., Piao, S.-L., Moriarty, R., Broquet, G., Le Quéré, C., Canadell, J. G., Peng, S., Poulter, B., Liu, Z., and Tans, P.: Five decades of northern land carbon uptake revealed by the interhemispheric CO2 gradient, Nature, 568, 221–225, https://doi.org/10.1038/s41586-019-1078-6, 2019.
Ciais, P., Bastos, A., Chevallier, F., Lauerwald, R., Poulter, B., Canadell, J. G., Hugelius, G., Jackson, R. B., Jain, A., Jones, M., Kondo, M., Luijkx, I. T., Patra, P. K., Peters, W., Pongratz, J., Petrescu, A. M. R., Piao, S., Qiu, C., Von Randow, C., Regnier, P., Saunois, M., Scholes, R., Shvidenko, A., Tian, H., Yang, H., Wang, X., and Zheng, B.: Definitions and methods to estimate regional land carbon fluxes for the second phase of the REgional Carbon Cycle Assessment and Processes Project (RECCAP-2), Geosci. Model Dev., 15, 1289–1316, https://doi.org/10.5194/gmd-15-1289-2022, 2022.
Collier, N., Hoffman, F. M., Lawrence, D. M., Keppel-Aleks, G., Koven, C. D., Riley, W. J., Mu, M., and Randerson, J. T.: The International Land Model Benchmarking (ILAMB) System: Design, Theory, and Implementation, J. Adv. Model. Earth Sy., 10, 2731–2754, https://doi.org/10.1029/2018MS001354, 2018.
Cox, P. M., Pearson, D., Booth, B. B., Friedlingstein, P., Huntingford, C., Jones, C. D., and Luke, C. M.: Sensitivity of tropical carbon to climate change constrained by carbon dioxide variability, Nature, 494, 341–344, https://doi.org/10.1038/nature11882, 2013.
Crisp, D., Dolman, H., Tanhua, T., McKinley, G. A., Hauck, J., Bastos, A., Sitch, S., Eggleston, S., and Aich, V.: How Well Do We Understand the Land-Ocean-Atmosphere Carbon Cycle?, Rev. Geophys., 60, e2021RG000736, https://doi.org/10.1029/2021RG000736, 2022.
De Kauwe, M. G., Medlyn, B. E., Zaehle, S., Walker, A. P., Dietze, M. C., Wang, Y.-P., Luo, Y., Jain, A. K., El-Masri, B., Hickler, T., Wårlind, D., Weng, E., Parton, W. J., Thornton, P. E., Wang, S., Prentice, I. C., Asao, S., Smith, B., McCarthy, H. R., Iversen, C. M., Hanson, P. J., Warren, J. M., Oren, R., and Norby, R. J.: Where does the carbon go? A model–data intercomparison of vegetation carbon allocation and turnover processes at two temperate forest free-air CO2 enrichment sites, New Phytol., 203, 883–899, https://doi.org/10.1111/nph.12847, 2014.
Delire, C., Séférian, R., Decharme, B., Alkama, R., Calvet, J.-C., Carrer, D., Gibelin, A.-L., Joetzjer, E., Morel, X., Rocher, M., and Tzanos, D.: The Global Land Carbon Cycle Simulated With ISBA-CTRIP: Improvements Over the Last Decade, J. Adv. Model. Earth Sy., 12, e2019MS001886, https://doi.org/10.1029/2019MS001886, 2020.
Denman, K. L., Brasseur, G., Chidthaisong, A., Ciais, P., Cox, P. M., Dickinson, R. E., Hauglustaine, D., Heinze, C., Holland, E., Jacob, D., Lohmann, U., Ramachandran, S., Leite da Silva Dias, P., Wofsy, S. C., and Zhang, X.: Couplings Between Changes in the Climate System and Biogeochemistry, in: Climate Change 2007: The Physical Science Basis. Contribution of Working Group I to the Fourth Assessment Report of the Intergovernmental Panel on Climate Change, edited by: Solomon, S., Qin, D., Manning, M., Marquis, M., Averyt, K., Tignor, M. M. B., Miller, H. L., and Chen, Z. L., Cambridge University Press, Cambridge, UK and New York, USA, 499–587, ISBN 9780521705967, 2007.
Denvil-Sommer, A., Gehlen, M., and Vrac, M.: Observation system simulation experiments in the Atlantic Ocean for enhanced surface ocean pCO2 reconstructions, Ocean Sci., 17, 1011–1030, https://doi.org/10.5194/os-17-1011-2021, 2021.
DeVries, T.: The oceanic anthropogenic CO2 sink: Storage, air-sea fluxes, and transports over the industrial era, Global Biogeochem. Cy., 28, 631–647, https://doi.org/10.1002/2013GB004739, 2014.
DeVries, T., Holzer, M., and Primeau, F.: Recent increase in oceanic carbon uptake driven by weaker upper-ocean overturning, Nature, 542, 215–218, https://doi.org/10.1038/nature21068, 2017.
DeVries, T., Quéré, C. L., Andrews, O., Berthet, S., Hauck, J., Ilyina, T., Landschützer, P., Lenton, A., Lima, I. D., Nowicki, M., Schwinger, J., and Séférian, R.: Decadal trends in the ocean carbon sink, P. Natl. Acad. Sci. USA, 116, 11646–11651, https://doi.org/10.1073/pnas.1900371116, 2019.
DeVries, T., Yamamoto, K., Wanninkhof, R., Gruber, N., Hauck, J., Müller, J. D., Bopp, L., Carroll, D., Carter, B., Chau, T.-T.-T., Doney, S. C., Gehlen, M., Gloege, L., Gregor, L., Henson, S., Kim, J. H., Iida, Y., Ilyina, T., Landschützer, P., Le Quéré, C., Munro, D., Nissen, C., Patara, L., Pérez, F. F., Resplandy, L., Rodgers, K. B., Schwinger, J., Séférian, R., Sicardi, V., Terhaar, J., Triñanes, J., Tsujino, H., Watson, A., Yasunaka, S., and Zeng, J.: Magnitude, trends, and variability of the global ocean carbon sink from 1985–2018, Glob. Biogeochem. Cycles, 37, e2023GB007780, https://doi.org/10.1029/2023GB007780, 2023.
Di Giuseppe, F., Rémy, S., Pappenberger, F., and Wetterhall, F.: Using the Fire Weather Index (FWI) to improve the estimation of fire emissions from fire radiative power (FRP) observations, Atmos. Chem. Phys., 18, 5359–5370, https://doi.org/10.5194/acp-18-5359-2018, 2018.
Doney, S. C., Lima, I., Feely, R. A., Glover, D. M., Lindsay, K., Mahowald, N., Moore, J. K., and Wanninkhof, R.: Mechanisms governing interannual variability in upper-ocean inorganic carbon system and air–sea CO2 fluxes: Physical climate and atmospheric dust, Deep-Sea Res. Pt. II, 56, 640–655, https://doi.org/10.1016/j.dsr2.2008.12.006, 2009.
Dong, Y., Bakker, D. C. E., Bell, T. G., Huang, B., Landschützer, P., Liss, P. S., and Yang, M.: Update on the Temperature Corrections of Global Air-Sea CO2 Flux Estimates, Glob. Biogeochem. Cycles, 36, e2022GB007360, https://doi.org/10.1029/2022GB007360, 2022.
Edson, J. B., Jampana, V., Weller, R. A., Bigorre, S. P., Plueddemann, A. J., Fairall, C. W., Miller, S. D., Mahrt, L., Vickers, D., and Hersbach, H.: On the Exchange of Momentum over the Open Ocean, J. Phys. Oceanogr., 43, 1589–1610, https://doi.org/10.1175/JPO-D-12-0173.1, 2013.
EIA: Short-Term Energy Outlook: September 2023, U.S. Energy Information Administration, http://www.eia.gov/forecasts/steo/outlook.cfm (last access: 9 November 2023), 2023.
Erb, K.-H., Kastner, T., Luyssaert, S., Houghton, R. A., Kuemmerle, T., Olofsson, P., and Haberl, H.: Bias in the attribution of forest carbon sinks, Nat. Clim. Change, 3, 854–856, https://doi.org/10.1038/nclimate2004, 2013.
Erb, K.-H., Kastner, T., Plutzar, C., Bais, A. L. S., Carvalhais, N., Fetzel, T., Gingrich, S., Haberl, H., Lauk, C., Niedertscheider, M., Pongratz, J., Thurner, M., and Luyssaert, S.: Unexpectedly large impact of forest management and grazing on global vegetation biomass, Nature, 553, 73–76, https://doi.org/10.1038/nature25138, 2018.
Eskander, S. M. S. U. and Fankhauser, S.: Reduction in greenhouse gas emissions from national climate legislation, Nat. Clim. Chang., 10, 750–756, https://doi.org/10.1038/s41558-020-0831-z, 2020.
Etheridge, D. M., Steele, L. P., Langenfelds, R. L., Francey, R. J., Barnola, J.-M., and Morgan, V. I.: Natural and anthropogenic changes in atmospheric CO2 over the last 1000 years from air in Antarctic ice and firn, J. Geophys. Res., 101, 4115–4128, https://doi.org/10.1029/95JD03410, 1996.
Eyring, V., Bony, S., Meehl, G. A., Senior, C. A., Stevens, B., Stouffer, R. J., and Taylor, K. E.: Overview of the Coupled Model Intercomparison Project Phase 6 (CMIP6) experimental design and organization, Geosci. Model Dev., 9, 1937–1958, https://doi.org/10.5194/gmd-9-1937-2016, 2016.
FAO: Impact of the Ukraine-Russia conflict on global food security and related matters under the mandate of the Food and Agriculture Organization of the United Nations (FAO), Hundred and Seventieth Session of the Council, https://www.fao.org/3/nj164en/nj164en.pdf (last access: 9 November 2023), 2023.
Fay, A. R., Gregor, L., Landschützer, P., McKinley, G. A., Gruber, N., Gehlen, M., Iida, Y., Laruelle, G. G., Rödenbeck, C., Roobaert, A., and Zeng, J.: SeaFlux: harmonization of air–sea CO2 fluxes from surface pCO2 data products using a standardized approach, Earth Syst. Sci. Data, 13, 4693–4710, https://doi.org/10.5194/essd-13-4693-2021, 2021.
Feng, L., Palmer, P. I., Bösch, H., and Dance, S.: Estimating surface CO2 fluxes from space-borne CO2 dry air mole fraction observations using an ensemble Kalman Filter, Atmos. Chem. Phys., 9, 2619–2633, https://doi.org/10.5194/acp-9-2619-2009, 2009.
Feng, L., Palmer, P. I., Parker, R. J., Deutscher, N. M., Feist, D. G., Kivi, R., Morino, I., and Sussmann, R.: Estimates of European uptake of CO2 inferred from GOSAT XCO2 retrievals: sensitivity to measurement bias inside and outside Europe, Atmos. Chem. Phys., 16, 1289–1302, https://doi.org/10.5194/acp-16-1289-2016, 2016.
Flanagan, D.: 2017 Minerals Yearbook: Copper [Advance Release], Tech. rep., U.S. Geological Survey, https://pubs.usgs.gov/myb/vol1/2017/myb1-2017-copper.pdf (last access: 9 November 2023), 2021.
Forster, P. M., Smith, C. J., Walsh, T., Lamb, W. F., Lamboll, R., Hauser, M., Ribes, A., Rosen, D., Gillett, N., Palmer, M. D., Rogelj, J., von Schuckmann, K., Seneviratne, S. I., Trewin, B., Zhang, X., Allen, M., Andrew, R., Birt, A., Borger, A., Boyer, T., Broersma, J. A., Cheng, L., Dentener, F., Friedlingstein, P., Gutiérrez, J. M., Gütschow, J., Hall, B., Ishii, M., Jenkins, S., Lan, X., Lee, J.-Y., Morice, C., Kadow, C., Kennedy, J., Killick, R., Minx, J. C., Naik, V., Peters, G. P., Pirani, A., Pongratz, J., Schleussner, C.-F., Szopa, S., Thorne, P., Rohde, R., Rojas Corradi, M., Schumacher, D., Vose, R., Zickfeld, K., Masson-Delmotte, V., and Zhai, P.: Indicators of Global Climate Change 2022: annual update of large-scale indicators of the state of the climate system and human influence, Earth Syst. Sci. Data, 15, 2295–2327, https://doi.org/10.5194/essd-15-2295-2023, 2023.
Friedlingstein, P., Houghton, R. A., Marland, G., Hackler, J., Boden, T. A., Conway, T. J., Canadell, J. G., Raupach, M. R., Ciais, P., and Le Quéré, C.: Update on CO2 emissions, Nat. Geosci., 3, 811–812, https://doi.org/10.1038/ngeo1022, 2010.
Friedlingstein, P., Andrew, R. M., Rogelj, J., Peters, G. P., Canadell, J. G., Knutti, R., Luderer, G., Raupach, M. R., Schaeffer, M., van Vuuren, D. P., and Le Quéré, C.: Persistent growth of CO2 emissions and implications for reaching climate targets, Nat. Geosci., 7, 709–715, https://doi.org/10.1038/ngeo2248, 2014.
Friedlingstein, P., Jones, M. W., O'Sullivan, M., Andrew, R. M., Hauck, J., Peters, G. P., Peters, W., Pongratz, J., Sitch, S., Le Quéré, C., Bakker, D. C. E., Canadell, J. G., Ciais, P., Jackson, R. B., Anthoni, P., Barbero, L., Bastos, A., Bastrikov, V., Becker, M., Bopp, L., Buitenhuis, E., Chandra, N., Chevallier, F., Chini, L. P., Currie, K. I., Feely, R. A., Gehlen, M., Gilfillan, D., Gkritzalis, T., Goll, D. S., Gruber, N., Gutekunst, S., Harris, I., Haverd, V., Houghton, R. A., Hurtt, G., Ilyina, T., Jain, A. K., Joetzjer, E., Kaplan, J. O., Kato, E., Klein Goldewijk, K., Korsbakken, J. I., Landschützer, P., Lauvset, S. K., Lefèvre, N., Lenton, A., Lienert, S., Lombardozzi, D., Marland, G., McGuire, P. C., Melton, J. R., Metzl, N., Munro, D. R., Nabel, J. E. M. S., Nakaoka, S.-I., Neill, C., Omar, A. M., Ono, T., Peregon, A., Pierrot, D., Poulter, B., Rehder, G., Resplandy, L., Robertson, E., Rödenbeck, C., Séférian, R., Schwinger, J., Smith, N., Tans, P. P., Tian, H., Tilbrook, B., Tubiello, F. N., van der Werf, G. R., Wiltshire, A. J., and Zaehle, S.: Global Carbon Budget 2019, Earth Syst. Sci. Data, 11, 1783–1838, https://doi.org/10.5194/essd-11-1783-2019, 2019.
Friedlingstein, P., O'Sullivan, M., Jones, M. W., Andrew, R. M., Hauck, J., Olsen, A., Peters, G. P., Peters, W., Pongratz, J., Sitch, S., Le Quéré, C., Canadell, J. G., Ciais, P., Jackson, R. B., Alin, S., Aragão, L. E. O. C., Arneth, A., Arora, V., Bates, N. R., Becker, M., Benoit-Cattin, A., Bittig, H. C., Bopp, L., Bultan, S., Chandra, N., Chevallier, F., Chini, L. P., Evans, W., Florentie, L., Forster, P. M., Gasser, T., Gehlen, M., Gilfillan, D., Gkritzalis, T., Gregor, L., Gruber, N., Harris, I., Hartung, K., Haverd, V., Houghton, R. A., Ilyina, T., Jain, A. K., Joetzjer, E., Kadono, K., Kato, E., Kitidis, V., Korsbakken, J. I., Landschützer, P., Lefèvre, N., Lenton, A., Lienert, S., Liu, Z., Lombardozzi, D., Marland, G., Metzl, N., Munro, D. R., Nabel, J. E. M. S., Nakaoka, S.-I., Niwa, Y., O'Brien, K., Ono, T., Palmer, P. I., Pierrot, D., Poulter, B., Resplandy, L., Robertson, E., Rödenbeck, C., Schwinger, J., Séférian, R., Skjelvan, I., Smith, A. J. P., Sutton, A. J., Tanhua, T., Tans, P. P., Tian, H., Tilbrook, B., van der Werf, G., Vuichard, N., Walker, A. P., Wanninkhof, R., Watson, A. J., Willis, D., Wiltshire, A. J., Yuan, W., Yue, X., and Zaehle, S.: Global Carbon Budget 2020, Earth Syst. Sci. Data, 12, 3269–3340, https://doi.org/10.5194/essd-12-3269-2020, 2020.
Friedlingstein, P., Jones, M. W., O'Sullivan, M., Andrew, R. M., Bakker, D. C. E., Hauck, J., Le Quéré, C., Peters, G. P., Peters, W., Pongratz, J., Sitch, S., Canadell, J. G., Ciais, P., Jackson, R. B., Alin, S. R., Anthoni, P., Bates, N. R., Becker, M., Bellouin, N., Bopp, L., Chau, T. T. T., Chevallier, F., Chini, L. P., Cronin, M., Currie, K. I., Decharme, B., Djeutchouang, L. M., Dou, X., Evans, W., Feely, R. A., Feng, L., Gasser, T., Gilfillan, D., Gkritzalis, T., Grassi, G., Gregor, L., Gruber, N., Gürses, Ö., Harris, I., Houghton, R. A., Hurtt, G. C., Iida, Y., Ilyina, T., Luijkx, I. T., Jain, A., Jones, S. D., Kato, E., Kennedy, D., Klein Goldewijk, K., Knauer, J., Korsbakken, J. I., Körtzinger, A., Landschützer, P., Lauvset, S. K., Lefèvre, N., Lienert, S., Liu, J., Marland, G., McGuire, P. C., Melton, J. R., Munro, D. R., Nabel, J. E. M. S., Nakaoka, S.-I., Niwa, Y., Ono, T., Pierrot, D., Poulter, B., Rehder, G., Resplandy, L., Robertson, E., Rödenbeck, C., Rosan, T. M., Schwinger, J., Schwingshackl, C., Séférian, R., Sutton, A. J., Sweeney, C., Tanhua, T., Tans, P. P., Tian, H., Tilbrook, B., Tubiello, F., van der Werf, G. R., Vuichard, N., Wada, C., Wanninkhof, R., Watson, A. J., Willis, D., Wiltshire, A. J., Yuan, W., Yue, C., Yue, X., Zaehle, S., and Zeng, J.: Global Carbon Budget 2021, Earth Syst. Sci. Data, 14, 1917–2005, https://doi.org/10.5194/essd-14-1917-2022, 2022a.
Friedlingstein, P., O'Sullivan, M., Jones, M. W., Andrew, R. M., Gregor, L., Hauck, J., Le Quéré, C., Luijkx, I. T., Olsen, A., Peters, G. P., Peters, W., Pongratz, J., Schwingshackl, C., Sitch, S., Canadell, J. G., Ciais, P., Jackson, R. B., Alin, S. R., Alkama, R., Arneth, A., Arora, V. K., Bates, N. R., Becker, M., Bellouin, N., Bittig, H. C., Bopp, L., Chevallier, F., Chini, L. P., Cronin, M., Evans, W., Falk, S., Feely, R. A., Gasser, T., Gehlen, M., Gkritzalis, T., Gloege, L., Grassi, G., Gruber, N., Gürses, Ö., Harris, I., Hefner, M., Houghton, R. A., Hurtt, G. C., Iida, Y., Ilyina, T., Jain, A. K., Jersild, A., Kadono, K., Kato, E., Kennedy, D., Klein Goldewijk, K., Knauer, J., Korsbakken, J. I., Landschützer, P., Lefèvre, N., Lindsay, K., Liu, J., Liu, Z., Marland, G., Mayot, N., McGrath, M. J., Metzl, N., Monacci, N. M., Munro, D. R., Nakaoka, S.-I., Niwa, Y., O'Brien, K., Ono, T., Palmer, P. I., Pan, N., Pierrot, D., Pocock, K., Poulter, B., Resplandy, L., Robertson, E., Rödenbeck, C., Rodriguez, C., Rosan, T. M., Schwinger, J., Séférian, R., Shutler, J. D., Skjelvan, I., Steinhoff, T., Sun, Q., Sutton, A. J., Sweeney, C., Takao, S., Tanhua, T., Tans, P. P., Tian, X., Tian, H., Tilbrook, B., Tsujino, H., Tubiello, F., van der Werf, G. R., Walker, A. P., Wanninkhof, R., Whitehead, C., Willstrand Wranne, A., Wright, R., Yuan, W., Yue, C., Yue, X., Zaehle, S., Zeng, J., and Zheng, B.: Global Carbon Budget 2022, Earth Syst. Sci. Data, 14, 4811–4900, https://doi.org/10.5194/essd-14-4811-2022, 2022b.
Friedlingstein, P., O'Sullivan, M., Jones, M. W., Andrew, R. M., Bakker, D. E. C., Hauck, J., Landschützer, P., Le Quéré, C., Luijkx, I. T., Peters, G. P., Peters, W., Pongratz, J., Schwingshackl, C, Sitch, S., Canadell, J. G., Ciais, P., Jackson, R. B., Alin, S. R., Anthoni, P., Barbero, L., Bates, N. R., Becker, M., Bellouin, N., Decharme, B., Bopp, L., Brasika, I. B. M., Cadule, P., Chamberlain, M. A., Chandra, N., Chau, T.-T.-T., Chevallier, F., Chini, L. P., Cronin, M., Dou, X., Enyo, K., Evans, W., Falk, S., Feely, R. A., Feng, L., Ford, D. J., Gasser, T., Ghattas, J., Gkritzalis, T., Grassi, G., Gregor, L., Gruber, N., Gürses, Ö., Harris, I., Hefner, M., Heinke, J. Houghton, R. A., Hurtt, G. C., Iida, Y., Ilyina, T., Jacobson, A. R., Jain, A., Jarníková, T., Jersild, A., Jiang, F., Jin, Z., Joos, F., Kato, E., Keeling, R. F., Kennedy, K., Goldewijk, K. K., Knauer, J., Korsbakken, J. I., Körtzinger, A., Lan, X., Lefèvre, N., Li, H., Liu, J., Liu, Z., Ma, L., Marland, G., Mayot, N., McGuire, P. C., McKinley, G. A., Meyer, G., Morgan, E. J., Munro, D. R., Nakaoka, S.-I., Niwa, Y., O’Brien, K. M., Olsen, A., Omar, A. M., Ono, T., Paulsen, M., Pierrot, D., Pocock, K., Poulter, B., Powis, C. M., Rehder, G., Resplandy, L., Robertson, E., Rödenbeck, C., Rosan, T. M., Schwinger, J., Séférian, R., Smallman, T. L., Smith, S. M., Sospedra-Alfonso, R., Sun, Q., Sutton, A. J., Sweeney, C., Takao, S., Tans, P. P., Tian, H., Tilbrook, B., Tsujino, H., Tubiello, F., van der Werf, G. R., van Ooijen, E., Wanninkhof, R., Watanabe, M., Wimart-Rousseau, C., Yang, D., Yang, X., Yuan, W., Yue, X., Zaehle, S., Zeng, K., and Zheng, B.: Supplemental data of the Global Carbon Budget 2023, ICOS-ERIC Carbon Portal [data set], https://doi.org/10.18160/GCP-2023, 2023.
Ganzenmüller, R., Bultan, S., Winkler, K., Fuchs, R., Zabel, F., and Pongratz, J.: Land-use change emissions based on high-resolution activity data substantially lower than previously estimated, Environ. Res. Lett., 17, 064050, https://doi.org/10.1088/1748-9326/ac70d8, 2022.
Gasser, T., Crepin, L., Quilcaille, Y., Houghton, R. A., Ciais, P., and Obersteiner, M.: Historical CO2 emissions from land use and land cover change and their uncertainty, Biogeosciences, 17, 4075–4101, https://doi.org/10.5194/bg-17-4075-2020, 2020.
Gaubert, B., Stephens, B. B., Basu, S., Chevallier, F., Deng, F., Kort, E. A., Patra, P. K., Peters, W., Rödenbeck, C., Saeki, T., Schimel, D., Van der Laan-Luijkx, I., Wofsy, S., and Yin, Y.: Global atmospheric CO2 inverse models converging on neutral tropical land exchange, but disagreeing on fossil fuel and atmospheric growth rate, Biogeosciences, 16, 117–134, https://doi.org/10.5194/bg-16-117-2019, 2019.
GCP: The Global Carbon Budget 2007, http://www.globalcarbonproject.org/carbonbudget/archive.htm (last access: 9 November 2023), 2007.
Giglio, L., Schroeder, W., and Justice, C. O.: The collection 6 MODIS active fire detection algorithm and fire products, Remote Sens. Environ., 178, 31–41, https://doi.org/10.1016/j.rse.2016.02.054, 2016.
Giglio, L., Boschetti, L., Roy, D. P., Humber, M. L., and Justice, C. O.: The Collection 6 MODIS burned area mapping algorithm and product, Remote Sens. Environ., 217, 72–85, https://doi.org/10.1016/j.rse.2018.08.005, 2018.
Gilfillan, D. and Marland, G.: CDIAC-FF: global and national CO2 emissions from fossil fuel combustion and cement manufacture: 1751–2017, Earth Syst. Sci. Data, 13, 1667–1680, https://doi.org/10.5194/essd-13-1667-2021, 2021.
Global Carbon Project: Global Carbon Atlas, Global Carbon Project [data set], https://globalcarbonatlas.org/ (last access: 9 November 2023), 2023.
Gloege, L., McKinley, G. A., Landschützer, P., Fay, A. R., Frölicher, T. L., Fyfe, J. C., Ilyina, T., Jones, S., Lovenduski, N. S., Rodgers, K. B., Schlunegger, S., and Takano, Y.: Quantifying Errors in Observationally Based Estimates of Ocean Carbon Sink Variability, Global Biogeochem. Cy., 35, e2020GB006788, https://doi.org/10.1029/2020GB006788, 2021.
Gloege, L., Yan, M., Zheng, T., and McKinley, G. A.: Improved Quantification of Ocean Carbon Uptake by Using Machine Learning to Merge Global Models and pCO2 Data, J. Adv. Model. Earth Sy., 14, e2021MS002620, https://doi.org/10.1029/2021MS002620, 2022.
Goris, N., Tjiputra, J. F., Olsen, A., Schwinger, J., Lauvset, S. K., and Jeansson, E.: Constraining Projection-Based Estimates of the Future North Atlantic Carbon Uptake, J. Climate, 31, 3959–3978, https://doi.org/10.1175/JCLI-D-17-0564.1, 2018.
Grassi, G., House, J., Kurz, W. A., Cescatti, A., Houghton, R. A., Peters, G. P., Sanz, M. J., Viñas, R. A., Alkama, R., Arneth, A., Bondeau, A., Dentener, F., Fader, M., Federici, S., Friedlingstein, P., Jain, A. K., Kato, E., Koven, C. D., Lee, D., Nabel, J. E. M. S., Nassikas, A. A., Perugini, L., Rossi, S., Sitch, S., Viovy, N., Wiltshire, A., and Zaehle, S.: Reconciling global-model estimates and country reporting of anthropogenic forest CO2 sinks, Nat. Clim. Change, 8, 914–920, https://doi.org/10.1038/s41558-018-0283-x, 2018.
Grassi, G., Stehfest, E., Rogelj, J., van Vuuren, D., Cescatti, A., House, J., Nabuurs, G.-J., Rossi, S., Alkama, R., Viñas, R. A., Calvin, K., Ceccherini, G., Federici, S., Fujimori, S., Gusti, M., Hasegawa, T., Havlik, P., Humpenöder, F., Korosuo, A., Perugini, L., Tubiello, F. N., and Popp, A.: Critical adjustment of land mitigation pathways for assessing countries' climate progress, Nat. Clim. Chang., 11, 425–434, https://doi.org/10.1038/s41558-021-01033-6, 2021.
Grassi, G., Schwingshackl, C., Gasser, T., Houghton, R. A., Sitch, S., Canadell, J. G., Cescatti, A., Ciais, P., Federici, S., Friedlingstein, P., Kurz, W. A., Sanz Sanchez, M. J., Abad Viñas, R., Alkama, R., Bultan, S., Ceccherini, G., Falk, S., Kato, E., Kennedy, D., Knauer, J., Korosuo, A., Melo, J., McGrath, M. J., Nabel, J. E. M. S., Poulter, B., Romanovskaya, A. A., Rossi, S., Tian, H., Walker, A. P., Yuan, W., Yue, X., and Pongratz, J.: Harmonising the land-use flux estimates of global models and national inventories for 2000–2020, Earth Syst. Sci. Data, 15, 1093–1114, https://doi.org/10.5194/essd-15-1093-2023, 2023.
Gregor, L. and Gruber, N.: OceanSODA-ETHZ: a global gridded data set of the surface ocean carbonate system for seasonal to decadal studies of ocean acidification, Earth Syst. Sci. Data, 13, 777–808, https://doi.org/10.5194/essd-13-777-2021, 2021.
Gruber, N., Gloor, M., Mikaloff Fletcher, S. E., Doney, S. C., Dutkiewicz, S., Follows, M. J., Gerber, M., Jacobson, A. R., Joos, F., Lindsay, K., Menemenlis, D., Mouchet, A., Müller, S. A., Sarmiento, J. L., and Takahashi, T.: Oceanic sources, sinks, and transport of atmospheric CO2, Global Biogeochem. Cy., 23, GB1005, https://doi.org/10.1029/2008GB003349, 2009.
Gruber, N., Clement, D., Carter, B. R., Feely, R. A., van Heuven, S., Hoppema, M., Ishii, M., Key, R. M., Kozyr, A., Lauvset, S. K., Lo Monaco, C., Mathis, J. T., Murata, A., Olsen, A., Perez, F. F., Sabine, C. L., Tanhua, T., and Wanninkhof, R.: The oceanic sink for anthropogenic CO2 from 1994 to 2007, Science, 363, 1193–1199, https://doi.org/10.1126/science.aau5153, 2019.
Gruber, N., Bakker, D. C. E., DeVries, T., Gregor, L., Hauck, J., Landschützer, P., McKinley, G. A., and Müller, J. D.: Trends and variability in the ocean carbon sink, Nat. Rev. Earth Environ., 4, 119–134, https://doi.org/10.1038/s43017-022-00381-x, 2023.
Guan, D., Liu, Z., Geng, Y., Lindner, S., and Hubacek, K.: The gigatonne gap in China's carbon dioxide inventories, Nat. Clim. Change, 2, 672–675, https://doi.org/10.1038/nclimate1560, 2012.
Gulev, S. K., Thorne, P. W., Ahn, J., Dentener, F. J., Domingues, C. M., Gerland, S., Gong, D. S., Kaufman, S., Nnamchi, H. C., Quaas, J., Rivera, J. A., Sathyendranath, S., Smith, S. L., Trewin, B., von Shuckmann, K., and Vose, R. S.: Changing State of the Climate System. In: Climate Change 2021: The Physical Science Basis. Contribution of Working Group I to the Sixth Assessment Report of the Intergovernmental Panel on Climate Change [Masson-Delmotte, V., Zhai, P., Pirani, A., Connors, S. L., Péan, C., Berger, S., Caud, N., Chen, Y., Goldfarb, L., Gomis, M. I., Huang, M., Leitzell, K., Lonnoy, E., Matthews, J. B. R., Maycock, T.K., Waterfield, T., Yelekçi, O., Yu, R., and Zhou, B. (Eds.)]. Cambridge University Press, Cambridge, United Kingdom and New York, NY, USA, 287–422, https://doi.org/10.1017/9781009157896.004, 2021.
Guo, R., Wang, J., Bing, L., Tong, D., Ciais, P., Davis, S. J., Andrew, R. M., Xi, F., and Liu, Z.: Global CO2 uptake by cement from 1930 to 2019, Earth Syst. Sci. Data, 13, 1791–1805, https://doi.org/10.5194/essd-13-1791-2021, 2021.
Gürses, Ö., Oziel, L., Karakuş, O., Sidorenko, D., Völker, C., Ye, Y., Zeising, M., Butzin, M., and Hauck, J.: Ocean biogeochemistry in the coupled ocean–sea ice–biogeochemistry model FESOM2.1–REcoM3, Geosci. Model Dev., 16, 4883–4936, https://doi.org/10.5194/gmd-16-4883-2023, 2023.
Gütschow, J. and Pflüger, M.: The PRIMAP-hist national historical emissions time series v2.4.2 (1750–2021), Zenodo [data set], https://doi.org/10.5281/zenodo.7727475, 2023.
Gütschow, J., Jeffery, M. L., Gieseke, R., Gebel, R., Stevens, D., Krapp, M., and Rocha, M.: The PRIMAP-hist national historical emissions time series, Earth Syst. Sci. Data, 8, 571–603, https://doi.org/10.5194/essd-8-571-2016, 2016.
Hall, B. D., Crotwell, A. M., Kitzis, D. R., Mefford, T., Miller, B. R., Schibig, M. F., and Tans, P. P.: Revision of the World Meteorological Organization Global Atmosphere Watch (WMO/GAW) CO2 calibration scale, Atmos. Meas. Tech., 14, 3015–3032, https://doi.org/10.5194/amt-14-3015-2021, 2021.
Hansis, E., Davis, S. J., and Pongratz, J.: Relevance of methodological choices for accounting of land use change carbon fluxes, Global Biogeochem. Cycles, 29, 1230–1246, https://doi.org/10.1002/2014GB004997, 2015.
Hart, D.: Cheyenne supercomputer, NCAR CISL, https://doi.org/10.5065/D6RX99HX, 2021.
Hauck, J., Zeising, M., Le Quéré, C., Gruber, N., Bakker, D. C. E., Bopp, L., Chau, T. T. T., Gürses, Ö., Ilyina, T., Landschützer, P., Lenton, A., Resplandy, L., Rödenbeck, C., Schwinger, J., and Séférian, R.: Consistency and Challenges in the Ocean Carbon Sink Estimate for the Global Carbon Budget, Front. Mar. Sci., 7, 571720, https://doi.org/10.3389/fmars.2020.571720, 2020.
Hauck, J., Nissen, C., Landschützer, P., Rödenbeck, C., Bushinsky, S., and Olsen, A.: Sparse observations induce large biases in estimates of the global ocean CO2 sink: an ocean model subsampling experiment, Philos. Trans. R. Soc. Math. Phys. Eng. Sci., 381, 20220063, https://doi.org/10.1098/rsta.2022.0063, 2023.
Haverd, V., Smith, B., Nieradzik, L., Briggs, P. R., Woodgate, W., Trudinger, C. M., Canadell, J. G., and Cuntz, M.: A new version of the CABLE land surface model (Subversion revision r4601) incorporating land use and land cover change, woody vegetation demography, and a novel optimisation-based approach to plant coordination of photosynthesis, Geosci. Model Dev., 11, 2995–3026, https://doi.org/10.5194/gmd-11-2995-2018, 2018.
Heinke, J., Rolinski, S., and Müller, C.: Modelling the role of livestock grazing in C and N cycling in grasslands with LPJmL5.0-grazing, Geosci. Model Dev., 16, 2455–2475, https://doi.org/10.5194/gmd-16-2455-2023, 2023.
Hickler, T., Smith, B., Prentice, I. C., Mjöfors, K., Miller, P., Arneth, A., and Sykes, M. T.: CO2 fertilization in temperate FACE experiments not representative of boreal and tropical forests, Glob. Change Biol., 14, 1531–1542, https://doi.org/10.1111/j.1365-2486.2008.01598.x, 2008.
Hoesly, R. M., Smith, S. J., Feng, L., Klimont, Z., Janssens-Maenhout, G., Pitkanen, T., Seibert, J. J., Vu, L., Andres, R. J., Bolt, R. M., Bond, T. C., Dawidowski, L., Kholod, N., Kurokawa, J.-I., Li, M., Liu, L., Lu, Z., Moura, M. C. P., O'Rourke, P. R., and Zhang, Q.: Historical (1750–2014) anthropogenic emissions of reactive gases and aerosols from the Community Emissions Data System (CEDS), Geosci. Model Dev., 11, 369–408, https://doi.org/10.5194/gmd-11-369-2018, 2018.
Hong, C., Burney, J. A., Pongratz, J., Nabel, J. E. M. S., Mueller, N. D., Jackson, R. B., and Davis, S. J.: Global and regional drivers of land-use emissions in 1961–2017, Nature, 589, 554–561, https://doi.org/10.1038/s41586-020-03138-y, 2021.
Holding, T., Ashton, I. G., Shutler, J. D., Land, P. E., Nightingale, P. D., Rees, A. P., Brown, I., Piolle, J.-F., Kock, A., Bange, H. W., Woolf, D. K., Goddijn-Murphy, L., Pereira, R., Paul, F., Girard-Ardhuin, F., Chapron, B., Rehder, G., Ardhuin, F., and Donlon, C. J.: The FluxEngine air–sea gas flux toolbox: simplified interface and extensions for in situ analyses and multiple sparingly soluble gases, Ocean Sci., 15, 1707–1728, https://doi.org/10.5194/os-15-1707-2019, 2019.
Houghton, R. A. and Castanho, A.: Annual emissions of carbon from land use, land-use change, and forestry from 1850 to 2020, Earth Syst. Sci. Data, 15, 2025–2054, https://doi.org/10.5194/essd-15-2025-2023, 2023.
Houghton, R. A., House, J. I., Pongratz, J., van der Werf, G. R., DeFries, R. S., Hansen, M. C., Le Quéré, C., and Ramankutty, N.: Carbon emissions from land use and land-cover change, Biogeosciences, 9, 5125–5142, https://doi.org/10.5194/bg-9-5125-2012, 2012.
Huang, B., Thorne, P. W., Banzon, V. F., Boyer, T., Chep- 65 urin, G., Lawrimore, J. H., Menne, M. J., Smith, T. M., Vose, R. S., and Zhang, H.-M.: NOAA Extended Reconstructed Sea Surface Temperature (ERSST), Version 5, NOAA [data set], https://doi.org/10.7289/V5T72FNM, 2017.
Hubau, W., Lewis, S. L., Phillips, O. L., Affum-Baffoe, K., Beeckman, H., Cuní-Sanchez, A., Daniels, A. K., Ewango, C. E. N., Fauset, S., Mukinzi, J. M., Sheil, D., Sonké, B., Sullivan, M. J. P., Sunderland, T. C. H., Taedoumg, H., Thomas, S. C., White, L. J. T., Abernethy, K. A., Adu-Bredu, S., Amani, C. A., Baker, T. R., Banin, L. F., Baya, F., Begne, S. K., Bennett, A. C., Benedet, F., Bitariho, R., Bocko, Y. E., Boeckx, P., Boundja, P., Brienen, R. J. W., Brncic, T., Chezeaux, E., Chuyong, G. B., Clark, C. J., Collins, M., Comiskey, J. A., Coomes, D. A., Dargie, G. C., de Haulleville, T., Kamdem, M. N. D., Doucet, J.-L., Esquivel-Muelbert, A., Feldpausch, T. R., Fofanah, A., Foli, E. G., Gilpin, M., Gloor, E., Gonmadje, C., Gourlet-Fleury, S., Hall, J. S., Hamilton, A. C., Harris, D. J., Hart, T. B., Hockemba, M. B. N., Hladik, A., Ifo, S. A., Jeffery, K. J., Jucker, T., Yakusu, E. K., Kearsley, E., Kenfack, D., Koch, A., Leal, M. E., Levesley, A., Lindsell, J. A., Lisingo, J., Lopez-Gonzalez, G., Lovett, J. C., Makana, J.-R., Malhi, Y., Marshall, A. R., Martin, J., Martin, E. H., Mbayu, F. M., Medjibe, V. P., Mihindou, V., Mitchard, E. T. A., Moore, S., Munishi, P. K. T., Bengone, N. N., Ojo, L., Ondo, F. E., Peh, K. S.-H., Pickavance, G. C., Poulsen, A. D., Poulsen, J. R., Qie, L., Reitsma, J., Rovero, F., Swaine, M. D., Talbot, J., Taplin, J., Taylor, D. M., Thomas, D. W., Toirambe, B., Mukendi, J. T., Tuagben, D., Umunay, P. M., van der Heijden, G. M. F., Verbeeck, H., Vleminckx, J., Willcock, S., Wöll, H., Woods, J. T., and Zemagho, L.: Asynchronous carbon sink saturation in African and Amazonian tropical forests, Nature, 579, 80–87, https://doi.org/10.1038/s41586-020-2035-0, 2020.
Humphrey, V., Zscheischler, J., Ciais, P., Gudmundsson, L., Sitch, S., and Seneviratne, S. I.: Sensitivity of atmospheric CO2 growth rate to observed changes in terrestrial water storage, Nature, 560, 628–631, https://doi.org/10.1038/s41586-018-0424-4, 2018.
Humphrey, V., Berg, A., Ciais, P., Gentine, P., Jung, M., Reichstein, M., Seneviratne, S. I., and Frankenberg, C.: Soil moisture–atmosphere feedback dominates land carbon uptake variability, Nature, 592, 65–69, https://doi.org/10.1038/s41586-021-03325-5, 2021.
Huntzinger, D. N., Michalak, A. M., Schwalm, C., Ciais, P., King, A. W., Fang, Y., Schaefer, K., Wei, Y., Cook, R. B., Fisher, J. B., Hayes, D., Huang, M., Ito, A., Jain, A. K., Lei, H., Lu, C., Maignan, F., Mao, J., Parazoo, N., Peng, S., Poulter, B., Ricciuto, D., Shi, X., Tian, H., Wang, W., Zeng, N., and Zhao, F.: Uncertainty in the response of terrestrial carbon sink to environmental drivers undermines carbon-climate feedback predictions, Sci. Rep.-UK, 7, 4765, https://doi.org/10.1038/s41598-017-03818-2, 2017.
Iida, Y., Takatani, Y., Kojima, A., and Ishii, M.: Global trends of ocean CO2 sink and ocean acidification: an observation-based reconstruction of surface ocean inorganic carbon variables, J. Oceanogr., 77, 323–358, https://doi.org/10.1007/s10872-020-00571-5, 2021.
Ilyina, T., Li, H., Spring, A., Müller, W. A., Bopp, L., Chikamoto, M. O., Danabasoglu, G., Dobrynin, M., Dunne, J., Fransner, F., Friedlingstein, P., Lee, W., Lovenduski, N. S., Merryfield, W. j., Mignot, J., Park, J. y., Séférian, R., Sospedra-Alfonso, R., Watanabe, M., and Yeager, S.: Predictable Variations of the Carbon Sinks and Atmospheric CO2 Growth in a Multi-Model Framework, Geophys. Res. Lett., 48, e2020GL090695, https://doi.org/10.1029/2020GL090695, 2021.
IMF: International Monetary Fund: World Economic Outlook, http://www.imf.org (last access: 9 November 2023), 2023.
IPCC: Annex II: Glossary, Möller, V., Matthews, J. B. R., van Diemen, R., Méndez, C., Semenov, S., Fuglestvedt, J. S., and Reisinger, A., in: Climate Change 2022: Impacts, Adaptation, and Vulnerability. Contribution of Working Group II to the Sixth Assessment Report of the Intergovernmental Panel on Climate Change, edited by: Pörtner, H.-O., Roberts, D. C., Tignor, M., Poloczanska, E. S., Mintenbeck, K., Alegría, A., Craig, M., Langsdorf, S., Löschke, S., Möller, V., Okem, A., and Rama, B., in: Climate Change 2022 – Impacts, Adaptation and Vulnerability: Working Group II Contribution to the Sixth Assessment Report of the Intergovernmental Panel on Climate Change, edited by: Möller, V, Matthews, J. B. R., van Diemen, R., Méndez, C., Semenov, S., Fuglestvedt, J. S., and Reisinger, A., Cambridge University Press, Cambridge, UK and New York, NY, 2897–2930, https://doi.org/10.1017/9781009325844.029, 2023.
Ito, A. and Inatomi, M.: Use of a process-based model for assessing the methane budgets of global terrestrial ecosystems and evaluation of uncertainty, Biogeosciences, 9, 759–773, https://doi.org/10.5194/bg-9-759-2012, 2012.
Jackson, R. B., Canadell, J. G., Le Quéré, C., Andrew, R. M., Korsbakken, J. I., Peters, G. P., and Nakicenovic, N.: Reaching peak emissions, Nat. Clim. Change, 6, 7–10, https://doi.org/10.1038/nclimate2892, 2016.
Jackson, R. B., Le Quéré, C., Andrew, R. M., Canadell, J. G., Korsbakken, J. I., Liu, Z., Peters, G. P., and Zheng, B.: Global energy growth is outpacing decarbonization, Environ. Res. Lett., 13, 120401, https://doi.org/10.1088/1748-9326/aaf303, 2018.
Jackson, R. B., Friedlingstein, P., Andrew, R. M., Canadell, J. G., Le Quéré, C., and Peters, G. P.: Persistent fossil fuel growth threatens the Paris Agreement and planetary health, Environ. Res. Lett., 14, 121001, https://doi.org/10.1088/1748-9326/ab57b3, 2019.
Jackson, R. B., Friedlingstein, P., Quéré, C. L., Abernethy, S., Andrew, R. M., Canadell, J. G., Ciais, P., Davis, S. J., Deng, Z., Liu, Z., Korsbakken, J. I., and Peters, G. P.: Global fossil carbon emissions rebound near pre-COVID-19 levels, Environ. Res. Lett., 17, 031001, https://doi.org/10.1088/1748-9326/ac55b6, 2022.
Jacobson, A. R., Schuldt, K. N., Tans, P., Arlyn Andrews, Miller, J. B., Oda, T., Mund, J., Weir, B., Ott, L., Aalto, T., Abshire, J. B., Aikin, K., Aoki, S., Apadula, F., Arnold, S., Baier, B., Bartyzel, J., Beyersdorf, A., Biermann, T., Biraud, S. C., Boenisch, H., Brailsford, G., Brand, W. A., Chen, G., Huilin Chen, Lukasz Chmura, Clark, S., Colomb, A., Commane, R., Conil, S., Couret, C., Cox, A., Cristofanelli, P., Cuevas, E., Curcoll, R., Daube, B., Davis, K. J., De Wekker, S., Coletta, J. D., Delmotte, M., DiGangi, E., DiGangi, J. P., Di Sarra, A. G., Dlugokencky, E., Elkins, J. W., Emmenegger, L., Shuangxi Fang, Fischer, M. L., Forster, G., Frumau, A., Galkowski, M., Gatti, L. V., Gehrlein, T., Gerbig, C., Francois Gheusi, Gloor, E., Gomez-Trueba, V., Goto, D., Griffis, T., Hammer, S., Hanson, C., Haszpra, L., Hatakka, J., Heimann, M., Heliasz, M., Hensen, A., Hermansen, O., Hintsa, E., Holst, J., Ivakhov, V., Jaffe, D. A., Jordan, A., Joubert, W., Karion, A., Kawa, S. R., Kazan, V., Keeling, R. F., Keronen, P., Kneuer, T., Kolari, P., Kateřina Komínková, Kort, E., Kozlova, E., Krummel, P., Kubistin, D., Labuschagne, C., Lam, D. H. Y., Lan, X., Langenfelds, R. L., Laurent, O., Laurila, T., Lauvaux, T., Lavric, J., Law, B. E., Lee, J., Lee, O. S. M., Lehner, I., Lehtinen, K., Leppert, R., Leskinen, A., Leuenberger, M., Levin, I., Levula, J., Lin, J., Lindauer, M., Loh, Z., Lopez, M., Luijkx, I. T., Lunder, C. R., Machida, T., Mammarella, I., Manca, G., Manning, A., Manning, A., Marek, M. V., Martin, M. Y., Matsueda, H., McKain, K., Meijer, H., Meinhardt, F., Merchant, L., Mihalopoulos, N., Miles, N. L., Miller, C. E., Mitchell, L., Mölder, M., Montzka, S., Moore, F., Moossen, H., Morgan, E., Morgui, J.-A., Morimoto, S., Müller-Williams, J., Munger, J. W., Munro, D., Myhre, C. L., Nakaoka, S.-I., Necki, J., Newman, S., Nichol, S., Niwa, Y., Obersteiner, F., O'Doherty, S., Paplawsky, B., Peischl, J., Peltola, O., Piacentino, S., Pichon, J.-M., Pickers, P., Piper, S., Pitt, J., Plass-Dülmer, C., Platt, S. M., Prinzivalli, S., Ramonet, M., Ramos, R., Reyes-Sanchez, E., Richardson, S. J., Riris, H., Rivas, P. P., Ryerson, T., Saito, K., Sargent, M., Sasakawa, M., Scheeren, B., Schuck, T., Schumacher, M., Seifert, T., Sha, M. K., Shepson, P., Shook, M., Sloop, C. D., Smith, P., Stanley, K., Steinbacher, M., Stephens, B., Sweeney, C., Thoning, K.,Timas, H., Torn, M., Tørseth, K., Trisolino, P., Turnbull, J., van den Bulk, P., van Dinther, D., Vermeulen, A., Viner, B., Vitkova, G., Walker, S., Watson, A., Wofsy, S. C., Worsey, J., Worthy, D., Young, D., Zaehle, S., Zahn, A., and Zimnoch, M.: CarbonTracker CT2022, https://doi.org/10.25925/Z1GJ-3254, 2023a.
Jacobson, A. R., Schuldt, K. N., Tans, P., Arlyn Andrews, Miller, J. B., Oda, T., Mund, J., Weir, B., Ott, L., Aalto, T., Abshire, J. B., Aikin, K., Aoki, S., Apadula, F., Arnold, S., Baier, B., Bartyzel, J., Beyersdorf, A., Biermann, T., Biraud, S. C., Boenisch, H., Brailsford, G., Brand, W. A., Chen, G., Huilin Chen, Lukasz Chmura, Clark, S., Colomb, A., Commane, R., Conil, S., Couret, C., Cox, A., Cristofanelli, P., Cuevas, E., Curcoll, R., Daube, B., Davis, K. J., De Wekker, S., Coletta, J. D., Delmotte, M., DiGangi, E., DiGangi, J. P., Di Sarra, A. G., Dlugokencky, E., Elkins, J. W., Emmenegger, L., Shuangxi Fang, Fischer, M. L., Forster, G., Frumau, A., Galkowski, M., Gatti, L. V., Gehrlein, T., Gerbig, C., Francois Gheusi, Gloor, E., Gomez-Trueba, V., Goto, D., Griffis, T., Hammer, S., Hanson, C., Haszpra, L., Hatakka, J., Heimann, M., Heliasz, M., Hensen, A., Hermansen, O., Hintsa, E., Holst, J., Ivakhov, V., Jaffe, D. A., Jordan, A., Joubert, W., Karion, A., Kawa, S. R., Kazan, V., Keeling, R. F., Keronen, P., Kneuer, T., Kolari, P., Kateřina Komínková, Kort, E., Kozlova, E., Krummel, P., Kubistin, D., Labuschagne, C., Lam, D. H. Y., Lan, X., Langenfelds, R. L., Laurent, O., Laurila, T., Lauvaux, T., Lavric, J., Law, B. E., Lee, J., Lee, O. S. M., Lehner, I., Lehtinen, K., Leppert, R.,Leskinen, A., Leuenberger, M., Levin, I., Levula, J., Lin, J., Lindauer, M., Loh, Z., Lopez, M., Luijkx, I. T., Lunder, C. R., Machida, T., Mammarella, I., Manca, G., Manning, A., Manning, A., Marek, M. V., Martin, M. Y., Matsueda, H., McKain, K., Meijer, H., Meinhardt, F., Merchant, L., Mihalopoulos, N., Miles, N. L., Miller, C. E., Mitchell, L., Mölder, M., Montzka, S., Moore, F., Moossen, H., Morgan, E., Morgui, J.-A., Morimoto, S., Müller-Williams, J., Munger, J. W., Munro, D., Myhre, C. L., Nakaoka, S.-I., Necki, J., Newman, S., Nichol, S., Niwa, Y., Obersteiner, F., O'Doherty, S., Paplawsky, B., Peischl, J., Peltola, O., Piacentino, S., Pichon, J.-M., Pickers, P., Piper, S., Pitt, J., Plass-Dülmer, C., Platt, S. M., Prinzivalli, S., Ramonet, M., Ramos, R., Reyes-Sanchez, E., Richardson, S. J., Riris, H., Rivas, P. P., Ryerson, T., Saito, K., Sargent, M., Sasakawa, M., Scheeren, B., Schuck, T., Schumacher, M., Seifert, T., M. Sha, K., Shepson, P., Shook, M., Sloop, C. D., Smith, P., Stanley, K., Steinbacher, M., Stephens, B., Sweeney, C., Thoning, K.,Timas, H., Torn, M., Tørseth, K., Trisolino, P., Turnbull, J., van den Bulk, P., van Dinther, D., Vermeulen, A., Viner, B., Vitkova, G., Walker, S., Watson, A., Wofsy, S. C., Worsey, J., Worthy, D., Young, D., Zaehle, S., Zahn, A., and Zimnoch, M.: CarbonTracker CT-NRT.v2023-3, https://doi.org/10.25925/7TAF-J322, 2023b.
Jain, A. K., Meiyappan, P., Song, Y., and House, J. I.: CO2 emissions from land-use change affected more by nitrogen cycle, than by the choice of land-cover data, Glob. Change Biol., 19, 2893–2906, https://doi.org/10.1111/gcb.12207, 2013.
Janssens-Maenhout, G., Crippa, M., Guizzardi, D., Muntean, M., Schaaf, E., Dentener, F., Bergamaschi, P., Pagliari, V., Olivier, J. G. J., Peters, J. A. H. W., van Aardenne, J. A., Monni, S., Doering, U., Petrescu, A. M. R., Solazzo, E., and Oreggioni, G. D.: EDGAR v4.3.2 Global Atlas of the three major greenhouse gas emissions for the period 1970–2012, Earth Syst. Sci. Data, 11, 959–1002, https://doi.org/10.5194/essd-11-959-2019, 2019.
Jean-Michel, L., Eric, G., Romain, B.-B., Gilles, G., Angélique, M., Marie, D., Clément, B., Mathieu, H., Olivier, L. G., Charly, R., Tony, C., Charles-Emmanuel, T., Florent, G., Giovanni, R., Mounir, B., Yann, D., and Pierre-Yves, L. T.: The Copernicus Global 1/12∘ Oceanic and Sea Ice GLORYS12 Reanalysis, Front. Earth Sci., 9, 698876, https://doi.org/10.3389/feart.2021.698876, 2021.
Jiang, F., Wang, H., Chen, J. M., Ju, W., Tian, X., Feng, S., Li, G., Chen, Z., Zhang, S., Lu, X., Liu, J., Wang, H., Wang, J., He, W., and Wu, M.: Regional CO2 fluxes from 2010 to 2015 inferred from GOSAT XCO2 retrievals using a new version of the Global Carbon Assimilation System, Atmos. Chem. Phys., 21, 1963–1985, https://doi.org/10.5194/acp-21-1963-2021, 2021.
Jiang, F., Ju, W., He, W., Wu, M., Wang, H., Wang, J., Jia, M., Feng, S., Zhang, L., and Chen, J. M.: A 10-year global monthly averaged terrestrial net ecosystem exchange dataset inferred from the ACOS GOSAT v9 XCO2 retrievals (GCAS2021), Earth Syst. Sci. Data, 14, 3013–3037, https://doi.org/10.5194/essd-14-3013-2022, 2022.
Jin, Z., Wang, T., Zhang, H., Wang, Y., Ding, J., and Tian, X.: Constraint of satellite CO2 retrieval on the global carbon cycle from a Chinese atmospheric inversion system, Sci. China Earth Sci., 66, 609–618, https://doi.org/10.1007/s11430-022-1036-7, 2023.
Joos, F. and Spahni, R.: Rates of change in natural and anthropogenic radiative forcing over the past 20,000 years, P. Natl. Acad. Sci. USA, 105, 1425–1430, https://doi.org/10.1073/pnas.0707386105, 2008.
Jones, C. D., Hickman, J. E., Rumbold, S. T., Walton, J., Lamboll, R. D., Skeie, R. B., Fiedler, S., Forster, P. M., Rogelj, J., Abe, M., Botzet, M., Calvin, K., Cassou, C., Cole, J. N. S., Davini, P., Deushi, M., Dix, M., Fyfe, J. C., Gillett, N. P., Ilyina, T., Kawamiya, M., Kelley, M., Kharin, S., Koshiro, T., Li, H., Mackallah, C., Müller, W. A., Nabat, P., van Noije, T., Nolan, P., Ohgaito, R., Olivié, D., Oshima, N., Parodi, J., Reerink, T. J., Ren, L., Romanou, A., Séférian, R., Tang, Y., Timmreck, C., Tjiputra, J., Tourigny, E., Tsigaridis, K., Wang, H., Wu, M., Wyser, K., Yang, S., Yang, Y., and Ziehn, T.: The Climate Response to Emissions Reductions Due to COVID-19: Initial Results From CovidMIP, Geophys. Res. Lett., 48, e2020GL091883, https://doi.org/10.1029/2020GL091883, 2021.
Jones, M. W., Andrew, R. M., Peters, G. P., Janssens-Maenhout, G., De-Gol, A. J., Ciais, P., Patra, P. K., Chevallier, F., and Le Quéré, C.: Gridded fossil CO2 emissions and related O2 combustion consistent with national inventories 1959–2018, Sci Data, 8, 2, https://doi.org/10.1038/s41597-020-00779-6, 2021.
Jones, M. W., Abatzoglou, J. T., Veraverbeke, S., Andela, N., Lasslop, G., Forkel, M., Smith, A. J. P., Burton, C., Betts, R. A., van der Werf, G. R., Sitch, S., Canadell, J. G., Santín, C., Kolden, C., Doerr, S. H., and Le Quéré, C.: Global and Regional Trends and Drivers of Fire Under Climate Change, Rev. Geophys., 60, e2020RG000726, https://doi.org/10.1029/2020RG000726, 2022.
Jones, M. W., Andrew, R. M., Peters, G. P., Janssens-Maenhout, G., De-Gol, A. J., Dou, X., Liu, Z., Pickers, P., Ciais, P., Patra, P. K., Chevallier, F., and Le Quéré, C.: Gridded fossil CO2 emissions and related O2 combustion consistent with national inventories 1959–2022, Zenodo [data set], https://doi.org/10.5281/zenodo.8386803, 2023.
Jung, M., Reichstein, M., Schwalm, C. R., Huntingford, C., Sitch, S., Ahlström, A., Arneth, A., Camps-Valls, G., Ciais, P., Friedlingstein, P., Gans, F., Ichii, K., Jain, A. K., Kato, E., Papale, D., Poulter, B., Raduly, B., Rödenbeck, C., Tramontana, G., Viovy, N., Wang, Y.-P., Weber, U., Zaehle, S., and Zeng, N.: Compensatory water effects link yearly global land CO2 sink changes to temperature, Nature, 541, 516–520, https://doi.org/10.1038/nature20780, 2017.
Kaiser, J. W., Heil, A., Andreae, M. O., Benedetti, A., Chubarova, N., Jones, L., Morcrette, J.-J., Razinger, M., Schultz, M. G., Suttie, M., and van der Werf, G. R.: Biomass burning emissions estimated with a global fire assimilation system based on observed fire radiative power, Biogeosciences, 9, 527–554, https://doi.org/10.5194/bg-9-527-2012, 2012.
Kato, E., Kinoshita, T., Ito, A., Kawamiya, M., and Yamagata, Y.: Evaluation of spatially explicit emission scenario of land-use change and biomass burning using a process-based biogeochemical model, J. Land Use Sci., 8, 104–122, https://doi.org/10.1080/1747423X.2011.628705, 2013.
Kawasaki, T., Hasumi, H., and Tanaka, Y.: Role of tide-induced vertical mixing in the deep Pacific Ocean circulation, J. Oceanogr., 77, 173–184, https://doi.org/10.1007/s10872-020-00584-0, 2021.
Keeley, J. E. and Pausas, J. G.: Distinguishing disturbance from perturbations in fire-prone ecosystems, Int. J. Wildland Fire, 28, 282–287, https://doi.org/10.1071/WF18203, 2019.
Keeling, C. D., Bacastow, R. B., Bainbridge, A. E., Ekdahl, C. A., Guenther, P. R., Waterman, L. S., and Chin, J. F. S.: Atmospheric carbon dioxide variations at Mauna Loa Observatory, Hawaii, Tellus A., 28, 538–551, https://doi.org/10.1111/j.2153-3490.1976.tb00701.x, 1976.
Keeling, R. F. and Manning, A. C.: 5.15 – Studies of Recent Changes in Atmospheric O2 Content, in: Treatise on Geochemistry (Second Edition), edited by: Holland, H. D. and Turekian, K. K., Elsevier, Oxford, 385–404, https://doi.org/10.1016/B978-0-08-095975-7.00420-4, 2014.
Keeling, R. F., Manning, A. C., Paplawsky, W. J., and Cox, A. C.: On the long-term stability of reference gases for atmospheric and CO2 measurements, Tellus B, 59, 3–14, https://doi.org/10.1111/j.1600-0889.2006.00196.x, 2007.
Keppler, L. and Landschützer, P.: Regional Wind Variability Modulates the Southern Ocean Carbon Sink, Sci. Rep.-UK, 9, 7384, https://doi.org/10.1038/s41598-019-43826-y, 2019.
Khatiwala, S., Primeau, F., and Hall, T.: Reconstruction of the history of anthropogenic CO2 concentrations in the ocean, Nature, 462, 346–349, https://doi.org/10.1038/nature08526, 2009.
Khatiwala, S., Tanhua, T., Mikaloff Fletcher, S., Gerber, M., Doney, S. C., Graven, H. D., Gruber, N., McKinley, G. A., Murata, A., Ríos, A. F., and Sabine, C. L.: Global ocean storage of anthropogenic carbon, Biogeosciences, 10, 2169–2191, https://doi.org/10.5194/bg-10-2169-2013, 2013.
Kong, Y., Zheng, B., Zhang, Q., and He, K.: Global and regional carbon budget for 2015–2020 inferred from OCO-2 based on an ensemble Kalman filter coupled with GEOS-Chem, Atmos. Chem. Phys., 22, 10769–10788, https://doi.org/10.5194/acp-22-10769-2022, 2022.
Korsbakken, J. I., Peters, G. P., and Andrew, R. M.: Uncertainties around reductions in China's coal use and CO2 emissions, Nat. Clim. Change, 6, 687–690, https://doi.org/10.1038/nclimate2963, 2016.
Krinner, G., Viovy, N., de Noblet-Ducoudré, N., Ogée, J., Polcher, J., Friedlingstein, P., Ciais, P., Sitch, S., and Prentice, I. C.: A dynamic global vegetation model for studies of the coupled atmosphere-biosphere system: DVGM for coupled climate studies, Global Biogeochem. Cycles, 19, GB1015, https://doi.org/10.1029/2003GB002199, 2005.
Lacroix, F., Ilyina, T., and Hartmann, J.: Oceanic CO2 outgassing and biological production hotspots induced by pre-industrial river loads of nutrients and carbon in a global modeling approach, Biogeosciences, 17, 55–88, https://doi.org/10.5194/bg-17-55-2020, 2020.
Lacroix, F., Ilyina, T., Mathis, M., Laruelle, G. G., and Regnier, P.: Historical increases in land-derived nutrient inputs may alleviate effects of a changing physical climate on the oceanic carbon cycle, Glob. Change Biol., 27, 5491–5513, https://doi.org/10.1111/gcb.15822, 2021.
Lamboll, R. D., Nicholls, Z. R. J., Smith, C. J., Kikstra, J. S., Byers, E., and Rogelj, J.: Assessing the size and uncertainty of remaining carbon budgets, Nat. Clim. Change, https://doi.org/10.1038/s41558-023-01848-5, 2023.
Lan, X., Tans, P., and Thoning, K. W.: Trends in globally-averaged CO2 determined from NOAA Global Monitoring Laboratory measurements, Version 2023-09. National Oceanic and Atmospheric Administration, Global Monitoring Laboratory (NOAA/GML), https://gml.noaa.gov/ccgg/trends/global.html (last access: 9 November 2023), 2023.
Landschützer, P., Gruber, N., Haumann, F. A., Rödenbeck, C., Bakker, D. C. E., van Heuven, S., Hoppema, M., Metzl, N., Sweeney, C., Takahashi, T., Tilbrook, B., and Wanninkhof, R.: The reinvigoration of the Southern Ocean carbon sink, Science, 349, 1221–1224, https://doi.org/10.1126/science.aab2620, 2015.
Landschützer, P., Gruber, N., and Bakker, D. C. E.: Decadal variations and trends of the global ocean carbon sink: decadal air-sea CO2 flux variability, Global Biogeochem. Cycles, 30, 1396–1417, https://doi.org/10.1002/2015GB005359, 2016.
Law, R. M., Ziehn, T., Matear, R. J., Lenton, A., Chamberlain, M. A., Stevens, L. E., Wang, Y.-P., Srbinovsky, J., Bi, D., Yan, H., and Vohralik, P. F.: The carbon cycle in the Australian Community Climate and Earth System Simulator (ACCESS-ESM1) – Part 1: Model description and pre-industrial simulation, Geosci. Model Dev., 10, 2567–2590, https://doi.org/10.5194/gmd-10-2567-2017, 2017.
Lawrence, D. M., Fisher, R. A., Koven, C. D., Oleson, K. W., Swenson, S. C., Bonan, G., Collier, N., Ghimire, B., van Kampenhout, L., Kennedy, D., Kluzek, E., Lawrence, P. J., Li, F., Li, H., Lombardozzi, D., Riley, W. J., Sacks, W. J., Shi, M., Vertenstein, M., Wieder, W. R., Xu, C., Ali, A. A., Badger, A. M., Bisht, G., van den Broeke, M., Brunke, M. A., Burns, S. P., Buzan, J., Clark, M., Craig, A., Dahlin, K., Drewniak, B., Fisher, J. B., Flanner, M., Fox, A. M., Gentine, P., Hoffman, F., Keppel-Aleks, G., Knox, R., Kumar, S., Lenaerts, J., Leung, L. R., Lipscomb, W. H., Lu, Y., Pandey, A., Pelletier, J. D., Perket, J., Randerson, J. T., Ricciuto, D. M., Sanderson, B. M., Slater, A., Subin, Z. M., Tang, J., Thomas, R. Q., Val Martin, M., and Zeng, X.: The Community Land Model Version 5: Description of New Features, Benchmarking, and Impact of Forcing Uncertainty, J. Adv. Model Earth, Sy., 11, 4245–4287, https://doi.org/10.1029/2018MS001583, 2019.
Le Quéré, C., Rödenbeck, C., Buitenhuis, E. T., Conway, T. J., Langenfelds, R., Gomez, A., Labuschagne, C., Ramonet, M., Nakazawa, T., Metzl, N., Gillett, N., and Heimann, M.: Saturation of the Southern Ocean CO2 Sink Due to Recent Climate Change, Science, 316, 1735–1738, https://doi.org/10.1126/science.1136188, 2007.
Le Quéré, C., Raupach, M. R., Canadell, J. G., Marland, G., Bopp, L., Ciais, P., Conway, T. J., Doney, S. C., Feely, R. A., Foster, P., Friedlingstein, P., Gurney, K., Houghton, R. A., House, J. I., Huntingford, C., Levy, P. E., Lomas, M. R., Majkut, J., Metzl, N., Ometto, J. P., Peters, G. P., Prentice, I. C., Randerson, J. T., Running, S. W., Sarmiento, J. L., Schuster, U., Sitch, S., Takahashi, T., Viovy, N., van der Werf, G. R., and Woodward, F. I.: Trends in the sources and sinks of carbon dioxide, Nat. Geosci., 2, 831–836, https://doi.org/10.1038/ngeo689, 2009.
Le Quéré, C., Takahashi, T., Buitenhuis, E. T., Rödenbeck, C., and Sutherland, S. C.: Impact of climate change and variability on the global oceanic sink of CO2, Global Biogeochem. Cy., 24, GB4007, https://doi.org/10.1029/2009GB003599, 2010.
Le Quéré, C., Andres, R. J., Boden, T., Conway, T., Houghton, R. A., House, J. I., Marland, G., Peters, G. P., van der Werf, G. R., Ahlström, A., Andrew, R. M., Bopp, L., Canadell, J. G., Ciais, P., Doney, S. C., Enright, C., Friedlingstein, P., Huntingford, C., Jain, A. K., Jourdain, C., Kato, E., Keeling, R. F., Klein Goldewijk, K., Levis, S., Levy, P., Lomas, M., Poulter, B., Raupach, M. R., Schwinger, J., Sitch, S., Stocker, B. D., Viovy, N., Zaehle, S., and Zeng, N.: The global carbon budget 1959–2011, Earth Syst. Sci. Data, 5, 165–185, https://doi.org/10.5194/essd-5-165-2013, 2013.
Le Quéré, C., Peters, G. P., Andres, R. J., Andrew, R. M., Boden, T. A., Ciais, P., Friedlingstein, P., Houghton, R. A., Marland, G., Moriarty, R., Sitch, S., Tans, P., Arneth, A., Arvanitis, A., Bakker, D. C. E., Bopp, L., Canadell, J. G., Chini, L. P., Doney, S. C., Harper, A., Harris, I., House, J. I., Jain, A. K., Jones, S. D., Kato, E., Keeling, R. F., Klein Goldewijk, K., Körtzinger, A., Koven, C., Lefèvre, N., Maignan, F., Omar, A., Ono, T., Park, G.-H., Pfeil, B., Poulter, B., Raupach, M. R., Regnier, P., Rödenbeck, C., Saito, S., Schwinger, J., Segschneider, J., Stocker, B. D., Takahashi, T., Tilbrook, B., van Heuven, S., Viovy, N., Wanninkhof, R., Wiltshire, A., and Zaehle, S.: Global carbon budget 2013, Earth Syst. Sci. Data, 6, 235–263, https://doi.org/10.5194/essd-6-235-2014, 2014.
Le Quéré, C., Moriarty, R., Andrew, R. M., Peters, G. P., Ciais, P., Friedlingstein, P., Jones, S. D., Sitch, S., Tans, P., Arneth, A., Boden, T. A., Bopp, L., Bozec, Y., Canadell, J. G., Chini, L. P., Chevallier, F., Cosca, C. E., Harris, I., Hoppema, M., Houghton, R. A., House, J. I., Jain, A. K., Johannessen, T., Kato, E., Keeling, R. F., Kitidis, V., Klein Goldewijk, K., Koven, C., Landa, C. S., Landschützer, P., Lenton, A., Lima, I. D., Marland, G., Mathis, J. T., Metzl, N., Nojiri, Y., Olsen, A., Ono, T., Peng, S., Peters, W., Pfeil, B., Poulter, B., Raupach, M. R., Regnier, P., Rödenbeck, C., Saito, S., Salisbury, J. E., Schuster, U., Schwinger, J., Séférian, R., Segschneider, J., Steinhoff, T., Stocker, B. D., Sutton, A. J., Takahashi, T., Tilbrook, B., van der Werf, G. R., Viovy, N., Wang, Y.-P., Wanninkhof, R., Wiltshire, A., and Zeng, N.: Global carbon budget 2014, Earth Syst. Sci. Data, 7, 47–85, https://doi.org/10.5194/essd-7-47-2015, 2015a.
Le Quéré, C., Moriarty, R., Andrew, R. M., Canadell, J. G., Sitch, S., Korsbakken, J. I., Friedlingstein, P., Peters, G. P., Andres, R. J., Boden, T. A., Houghton, R. A., House, J. I., Keeling, R. F., Tans, P., Arneth, A., Bakker, D. C. E., Barbero, L., Bopp, L., Chang, J., Chevallier, F., Chini, L. P., Ciais, P., Fader, M., Feely, R. A., Gkritzalis, T., Harris, I., Hauck, J., Ilyina, T., Jain, A. K., Kato, E., Kitidis, V., Klein Goldewijk, K., Koven, C., Landschützer, P., Lauvset, S. K., Lefèvre, N., Lenton, A., Lima, I. D., Metzl, N., Millero, F., Munro, D. R., Murata, A., Nabel, J. E. M. S., Nakaoka, S., Nojiri, Y., O'Brien, K., Olsen, A., Ono, T., Pérez, F. F., Pfeil, B., Pierrot, D., Poulter, B., Rehder, G., Rödenbeck, C., Saito, S., Schuster, U., Schwinger, J., Séférian, R., Steinhoff, T., Stocker, B. D., Sutton, A. J., Takahashi, T., Tilbrook, B., van der Laan-Luijkx, I. T., van der Werf, G. R., van Heuven, S., Vandemark, D., Viovy, N., Wiltshire, A., Zaehle, S., and Zeng, N.: Global Carbon Budget 2015, Earth Syst. Sci. Data, 7, 349–396, https://doi.org/10.5194/essd-7-349-2015, 2015b.
Le Quéré, C., Andrew, R. M., Canadell, J. G., Sitch, S., Korsbakken, J. I., Peters, G. P., Manning, A. C., Boden, T. A., Tans, P. P., Houghton, R. A., Keeling, R. F., Alin, S., Andrews, O. D., Anthoni, P., Barbero, L., Bopp, L., Chevallier, F., Chini, L. P., Ciais, P., Currie, K., Delire, C., Doney, S. C., Friedlingstein, P., Gkritzalis, T., Harris, I., Hauck, J., Haverd, V., Hoppema, M., Klein Goldewijk, K., Jain, A. K., Kato, E., Körtzinger, A., Landschützer, P., Lefèvre, N., Lenton, A., Lienert, S., Lombardozzi, D., Melton, J. R., Metzl, N., Millero, F., Monteiro, P. M. S., Munro, D. R., Nabel, J. E. M. S., Nakaoka, S., O'Brien, K., Olsen, A., Omar, A. M., Ono, T., Pierrot, D., Poulter, B., Rödenbeck, C., Salisbury, J., Schuster, U., Schwinger, J., Séférian, R., Skjelvan, I., Stocker, B. D., Sutton, A. J., Takahashi, T., Tian, H., Tilbrook, B., van der Laan-Luijkx, I. T., van der Werf, G. R., Viovy, N., Walker, A. P., Wiltshire, A. J., and Zaehle, S.: Global Carbon Budget 2016, Earth Syst. Sci. Data, 8, 605–649, https://doi.org/10.5194/essd-8-605-2016, 2016.
Le Quéré, C., Andrew, R. M., Friedlingstein, P., Sitch, S., Pongratz, J., Manning, A. C., Korsbakken, J. I., Peters, G. P., Canadell, J. G., Jackson, R. B., Boden, T. A., Tans, P. P., Andrews, O. D., Arora, V. K., Bakker, D. C. E., Barbero, L., Becker, M., Betts, R. A., Bopp, L., Chevallier, F., Chini, L. P., Ciais, P., Cosca, C. E., Cross, J., Currie, K., Gasser, T., Harris, I., Hauck, J., Haverd, V., Houghton, R. A., Hunt, C. W., Hurtt, G., Ilyina, T., Jain, A. K., Kato, E., Kautz, M., Keeling, R. F., Klein Goldewijk, K., Körtzinger, A., Landschützer, P., Lefèvre, N., Lenton, A., Lienert, S., Lima, I., Lombardozzi, D., Metzl, N., Millero, F., Monteiro, P. M. S., Munro, D. R., Nabel, J. E. M. S., Nakaoka, S., Nojiri, Y., Padin, X. A., Peregon, A., Pfeil, B., Pierrot, D., Poulter, B., Rehder, G., Reimer, J., Rödenbeck, C., Schwinger, J., Séférian, R., Skjelvan, I., Stocker, B. D., Tian, H., Tilbrook, B., Tubiello, F. N., van der Laan-Luijkx, I. T., van der Werf, G. R., van Heuven, S., Viovy, N., Vuichard, N., Walker, A. P., Watson, A. J., Wiltshire, A. J., Zaehle, S., and Zhu, D.: Global Carbon Budget 2017, Earth Syst. Sci. Data, 10, 405–448, https://doi.org/10.5194/essd-10-405-2018, 2018a.
Le Quéré, C., Andrew, R. M., Friedlingstein, P., Sitch, S., Hauck, J., Pongratz, J., Pickers, P. A., Korsbakken, J. I., Peters, G. P., Canadell, J. G., Arneth, A., Arora, V. K., Barbero, L., Bastos, A., Bopp, L., Chevallier, F., Chini, L. P., Ciais, P., Doney, S. C., Gkritzalis, T., Goll, D. S., Harris, I., Haverd, V., Hoffman, F. M., Hoppema, M., Houghton, R. A., Hurtt, G., Ilyina, T., Jain, A. K., Johannessen, T., Jones, C. D., Kato, E., Keeling, R. F., Goldewijk, K. K., Landschützer, P., Lefèvre, N., Lienert, S., Liu, Z., Lombardozzi, D., Metzl, N., Munro, D. R., Nabel, J. E. M. S., Nakaoka, S., Neill, C., Olsen, A., Ono, T., Patra, P., Peregon, A., Peters, W., Peylin, P., Pfeil, B., Pierrot, D., Poulter, B., Rehder, G., Resplandy, L., Robertson, E., Rocher, M., Rödenbeck, C., Schuster, U., Schwinger, J., Séférian, R., Skjelvan, I., Steinhoff, T., Sutton, A., Tans, P. P., Tian, H., Tilbrook, B., Tubiello, F. N., van der Laan-Luijkx, I. T., van der Werf, G. R., Viovy, N., Walker, A. P., Wiltshire, A. J., Wright, R., Zaehle, S., and Zheng, B.: Global Carbon Budget 2018, Earth Syst. Sci. Data, 10, 2141–2194, https://doi.org/10.5194/essd-10-2141-2018, 2018b.
Le Quéré, C., Korsbakken, J. I., Wilson, C., Tosun, J., Andrew, R., Andres, R. J., Canadell, J. G., Jordan, A., Peters, G. P., and van Vuuren, D. P.: Drivers of declining CO2 emissions in 18 developed economies, Nat. Clim. Chang., 9, 213–217, https://doi.org/10.1038/s41558-019-0419-7, 2019.
Le Quéré, C., Peters, G. P., Friedlingstein, P., Andrew, R. M., Canadell, J. G., Davis, S. J., Jackson, R. B., and Jones, M. W.: Fossil CO2 emissions in the post-COVID-19 era, Nat. Clim. Chang., 11, 197–199, https://doi.org/10.1038/s41558-021-01001-0, 2021.
Levitus, S., Antonov, J. I., Boyer, T. P., Baranova, O. K., Garcia, H. E., Locarnini, R. A., Mishonov, A. V., Reagan, J. R., Seidov, D., Yarosh, E. S., and Zweng, M. M.: World ocean heat content and thermosteric sea level change (0–2000 m), 1955–2010, Geophys. Res. Lett., 39, https://doi.org/10.1029/2012GL051106, 2012.
Li, H., Ilyina, T., Müller, W. A., and Sienz, F.: Decadal predictions of the North Atlantic CO2 uptake, Nat. Commun., 7, 11076, https://doi.org/10.1038/ncomms11076, 2016.
Li, H., Ilyina, T., Müller, W. A., and Landschützer, P.: Predicting the variable ocean carbon sink, Sci. Adv., 5, eaav6471, https://doi.org/10.1126/sciadv.aav6471, 2019.
Li, H., Ilyina, T., Loughran, T., Spring, A., and Pongratz, J.: Reconstructions and predictions of the global carbon budget with an emission-driven Earth system model, Earth Syst. Dynam., 14, 101–119, https://doi.org/10.5194/esd-14-101-2023, 2023.
Li, W., Ciais, P., Peng, S., Yue, C., Wang, Y., Thurner, M., Saatchi, S. S., Arneth, A., Avitabile, V., Carvalhais, N., Harper, A. B., Kato, E., Koven, C., Liu, Y. Y., Nabel, J. E. M. S., Pan, Y., Pongratz, J., Poulter, B., Pugh, T. A. M., Santoro, M., Sitch, S., Stocker, B. D., Viovy, N., Wiltshire, A., Yousefpour, R., and Zaehle, S.: Land-use and land-cover change carbon emissions between 1901 and 2012 constrained by biomass observations, Biogeosciences, 14, 5053–5067, https://doi.org/10.5194/bg-14-5053-2017, 2017.
Liao, E., Resplandy, L., Liu, J., and Bowman, K. W.: Amplification of the Ocean Carbon Sink During El Niños: Role of Poleward Ekman Transport and Influence on Atmospheric CO2, Global Biogeochem. Cy., 34, e2020GB006574, https://doi.org/10.1029/2020GB006574, 2020.
Lienert, S. and Joos, F.: A Bayesian ensemble data assimilation to constrain model parameters and land-use carbon emissions, Biogeosciences, 15, 2909–2930, https://doi.org/10.5194/bg-15-2909-2018, 2018.
Liu, J., Baskaran, L., Bowman, K., Schimel, D., Bloom, A. A., Parazoo, N. C., Oda, T., Carroll, D., Menemenlis, D., Joiner, J., Commane, R., Daube, B., Gatti, L. V., McKain, K., Miller, J., Stephens, B. B., Sweeney, C., and Wofsy, S.: Carbon Monitoring System Flux Net Biosphere Exchange 2020 (CMS-Flux NBE 2020), Earth Syst. Sci. Data, 13, 299–330, https://doi.org/10.5194/essd-13-299-2021, 2021.
Liu, Z., Guan, D., Wei, W., Davis, S. J., Ciais, P., Bai, J., Peng, S., Zhang, Q., Hubacek, K., Marland, G., Andres, R. J., Crawford-Brown, D., Lin, J., Zhao, H., Hong, C., Boden, T. A., Feng, K., Peters, G. P., Xi, F., Liu, J., Li, Y., Zhao, Y., Zeng, N., and He, K.: Reduced carbon emission estimates from fossil fuel combustion and cement production in China, Nature, 524, 335–338, https://doi.org/10.1038/nature14677, 2015.
Liu, Z., Zeng, N., Liu, Y., Kalnay, E., Asrar, G., Wu, B., Cai, Q., Liu, D., and Han, P.: Improving the joint estimation of CO2 and surface carbon fluxes using a constrained ensemble Kalman filter in COLA (v1.0), Geosci. Model Dev., 15, 5511–5528, https://doi.org/10.5194/gmd-15-5511-2022, 2022.
Lo Monaco, C. and Metzl, N.: OISO-32 cruise, RV Marion Dufresne, https://doi.org/10.17600/18001858, 2022.
Lovenduski, N. S., Bonan, G. B., Yeager, S. G., Lindsay, K., and Lombardozzi, D. L.: High predictability of terrestrial carbon fluxes from an initialized decadal prediction system, Environ. Res. Lett., 14, 124074, https://doi.org/10.1088/1748-9326/ab5c55, 2019a.
Lovenduski, N. S., Yeager, S. G., Lindsay, K., and Long, M. C.: Predicting near-term variability in ocean carbon uptake, Earth Syst. Dynam., 10, 45–57, https://doi.org/10.5194/esd-10-45-2019, 2019b.
Lutz, F., Herzfeld, T., Heinke, J., Rolinski, S., Schaphoff, S., von Bloh, W., Stoorvogel, J. J., and Müller, C.: Simulating the effect of tillage practices with the global ecosystem model LPJmL (version 5.0-tillage), Geosci. Model Dev., 12, 2419–2440, https://doi.org/10.5194/gmd-12-2419-2019, 2019.
Ma, L., Hurtt, G., Ott, L., Sahajpal, R., Fisk, J., Lamb, R., Tang, H., Flanagan, S., Chini, L., Chatterjee, A., and Sullivan, J.: Global evaluation of the Ecosystem Demography model (ED v3.0), Geosci. Model Dev., 15, 1971–1994, https://doi.org/10.5194/gmd-15-1971-2022, 2022.
Magi, B. I., Rabin, S., Shevliakova, E., and Pacala, S.: Separating agricultural and non-agricultural fire seasonality at regional scales, Biogeosciences, 9, 3003–3012, https://doi.org/10.5194/bg-9-3003-2012, 2012.
Masarie, K. A. and Tans, P. P.: Extension and integration of atmospheric carbon dioxide data into a globally consistent measurement record, J. Geophys. Res., 100, 11593, https://doi.org/10.1029/95JD00859, 1995.
Mather, A. S.: The transition from deforestation to reforestation in Europe, in: Agricultural technologies and tropical deforestation, edited by: Angelsen, A. and Kaimowitz, D., CABI in association with centre for international Forestry Research, 35–52, 2001.
Mauritsen, T., Bader, J., Becker, T., Behrens, J., Bittner, M., Brokopf, R., Brovkin, V., Claussen, M., Crueger, T., Esch, M., Fast, I., Fiedler, S., Fläschner, D., Gayler, V., Giorgetta, M., Goll, D. S., Haak, H., Hagemann, S., Hedemann, C., Hohenegger, C., Ilyina, T., Jahns, T., Jimenéz-de-la-Cuesta, D., Jungclaus, J., Kleinen, T., Kloster, S., Kracher, D., Kinne, S., Kleberg, D., Lasslop, G., Kornblueh, L., Marotzke, J., Matei, D., Meraner, K., Mikolajewicz, U., Modali, K., Möbis, B., Müller, W. A., Nabel, J. E. M. S., Nam, C. C. W., Notz, D., Nyawira, S.-S., Paulsen, H., Peters, K., Pincus, R., Pohlmann, H., Pongratz, J., Popp, M., Raddatz, T. J., Rast, S., Redler, R., Reick, C. H., Rohrschneider, T., Schemann, V., Schmidt, H., Schnur, R., Schulzweida, U., Six, K. D., Stein, L., Stemmler, I., Stevens, B., von Storch, J.-S., Tian, F., Voigt, A., Vrese, P., Wieners, K.-H., Wilkenskjeld, S., Winkler, A., and Roeckner, E.: Developments in the MPI-M Earth System Model version 1.2 (MPI-ESM1.2) and Its Response to Increasing CO2, J. Adv. Model Earth Sy., 11, 998–1038, https://doi.org/10.1029/2018MS001400, 2019.
McGrath, M. J., Luyssaert, S., Meyfroidt, P., Kaplan, J. O., Bürgi, M., Chen, Y., Erb, K., Gimmi, U., McInerney, D., Naudts, K., Otto, J., Pasztor, F., Ryder, J., Schelhaas, M.-J., and Valade, A.: Reconstructing European forest management from 1600 to 2010, Biogeosciences, 12, 4291–4316, https://doi.org/10.5194/bg-12-4291-2015, 2015.
McKinley, G. A., Fay, A. R., Lovenduski, N. S., and Pilcher, D. J.: Natural Variability and Anthropogenic Trends in the Ocean Carbon Sink, Annu. Rev. Mar. Sci., 9, 125–150, https://doi.org/10.1146/annurev-marine-010816-060529, 2017.
McKinley, G. A., Fay, A. R., Eddebbar, Y. A., Gloege, L., and Lovenduski, N. S.: External Forcing Explains Recent Decadal Variability of the Ocean Carbon Sink, AGU Advances, 1, e2019AV000149, https://doi.org/10.1029/2019AV000149, 2020.
Meiyappan, P., Jain, A. K., and House, J. I.: Increased influence of nitrogen limitation on CO2 emissions from future land use and land use change, Global Biogeochem. Cycles, 29, 1524–1548, https://doi.org/10.1002/2015GB005086, 2015.
Melton, J. R., Arora, V. K., Wisernig-Cojoc, E., Seiler, C., Fortier, M., Chan, E., and Teckentrup, L.: CLASSIC v1.0: the open-source community successor to the Canadian Land Surface Scheme (CLASS) and the Canadian Terrestrial Ecosystem Model (CTEM) – Part 1: Model framework and site-level performance, Geosci. Model Dev., 13, 2825–2850, https://doi.org/10.5194/gmd-13-2825-2020, 2020.
Mercado, L. M., Bellouin, N., Sitch, S., Boucher, O., Huntingford, C., Wild, M., and Cox, P. M.: Impact of changes in diffuse radiation on the global land carbon sink, Nature, 458, 1014–1017, https://doi.org/10.1038/nature07949, 2009.
Merchant, C. J., Embury, O., Bulgin, C. E., Block, T., Corlett, G. K., Fiedler, E., Good, S. A., Mittaz, J., Rayner, N. A., Berry, D., Eastwood, S., Taylor, M., Tsushima, Y., Waterfall, A., Wilson, R., and Donlon, C.: Satellite-based time-series of sea-surface temperature since 1981 for climate applications, Sci. Data, 6, 223, https://doi.org/10.1038/s41597-019-0236-x, 2019.
Moorcroft, P. R., Hurtt, G. C., and Pacala, S. W.: A Method for Scaling Vegetation Dynamics: The Ecosystem Demography Model (ed), Ecol. Monogr., 71, 557–586, https://doi.org/10.1890/0012-9615(2001)071[0557:AMFSVD]2.0.CO;2, 2001.
Müller, J. D., Gruber, N., Carter, B., Feely, R., Ishii, M., Lange, N., Lauvset, S. K., Murata, A., Olsen, A., Pérez, F. F., Sabine, C., Tanhua, T., Wanninkhof, R., and Zhu, D.: Decadal Trends in the Oceanic Storage of Anthropogenic Carbon From 1994 to 2014, AGU Adv., 4, e2023AV000875, https://doi.org/10.1029/2023AV000875, 2023.
Nakano, H., Tsujino, H., Hirabara, M., Yasuda, T., Motoi, T., Ishii, M., and Yamanaka, G.: Uptake mechanism of anthropogenic CO2 in the Kuroshio Extension region in an ocean general circulation model, J. Oceanogr., 67, 765–783, https://doi.org/10.1007/s10872-011-0075-7, 2011.
NCEP: National Centers for Environmental Prediction. ONI Index. Cold & Warm Episodes by Season, https://origin.cpc.ncep.noaa.gov/products/analysis_monitoring/ensostuff/ONI_v5.php (last access: 9 November 2023), 2023.
NOAA/GML: Trends in Atmospheric Carbon Dioxide, NOAA Global Monitoring Laboratory, https://gml.noaa.gov/ccgg/trends/gl_gr.html (last access: 9 November 2023), 2019.
Niu, G.-Y., Yang, Z.-L., Mitchell, K. E., Chen, F., Ek, M. B., Barlage, M., Kumar, A., Manning, K., Niyogi, D., Rosero, E., Tewari, M., and Xia, Y.: The community Noah land surface model with multiparameterization options (Noah-MP): 1. Model description and evaluation with local-scale measurements, J. Geophys. Res.-Atmos., 116, D12109, https://doi.org/10.1029/2010JD015139, 2011.
Niwa, Y., Langenfelds, R., Krummel, P., Loh, Z., Worthy, D., Hatakka, J., Aalto, T., Ramonet, M., Delmotte, M., Schmidt, M., Gheusi, F., Mihalopoulos, N., Morgui, J. A., Andrews, A., Dlugokencky, E., Lee, J., Sweeney, C., Thoning, K., Tans, P., De Wekker, S., Fischer, M. L., Jaffe, D., McKain, K., Viner, B., Miller, J. B., Karion, A., Miller, C., Sloop, C. D., Saito, K., Aoki, S., Morimoto, S., Goto, D., Steinbacher, M., Myhre, C. L., Hermanssen, O., Stephens, B., Keeling, R., Afshar, S., Paplawsky, B., Cox, A., Walker, S., Schuldt, K., Mukai, H., Machida, T., Sasakawa, M., Nomura, S., Ito, A., Iida, Y., and Jones, M. W.: Long-term global CO2 fluxes estimated by NICAM-based Inverse Simulation for Monitoring CO2 (NISMON-CO2) (ver.2022.1), National Institute for Environmental Studies Japan [data set], https://doi.org/10.17595/20201127.001, 2020.
Niwa, Y., Ishijima, K., Ito, A., and Iida, Y.: Toward a long-term atmospheric CO2 inversion for elucidating natural carbon fluxes: technical notes of NISMON-CO2 v2021.1, Prog. Earth Planet Sci., 9, 42, https://doi.org/10.1186/s40645-022-00502-6, 2022.
Obermeier, W. A., Nabel, J. E. M. S., Loughran, T., Hartung, K., Bastos, A., Havermann, F., Anthoni, P., Arneth, A., Goll, D. S., Lienert, S., Lombardozzi, D., Luyssaert, S., McGuire, P. C., Melton, J. R., Poulter, B., Sitch, S., Sullivan, M. O., Tian, H., Walker, A. P., Wiltshire, A. J., Zaehle, S., and Pongratz, J.: Modelled land use and land cover change emissions – a spatio-temporal comparison of different approaches, Earth Syst. Dynam., 12, 635–670, https://doi.org/10.5194/esd-12-635-2021, 2021.
O'Rourke, P. R., Smith, S. J., Mott, A., Ahsan, H., McDuffie, E. E., Crippa, M., Klimont, Z., McDonald, B., Wang, S., Nicholson, M. B., Feng, L., and Hoesly, R. M.: CEDS v_2021_04_21 Release Emission Data, Zenodo [data set], https://doi.org/10.5281/zenodo.4741285, 2021.
O'Sullivan, M., Spracklen, D. V., Batterman, S. A., Arnold, S. R., Gloor, M., and Buermann, W.: Have Synergies Between Nitrogen Deposition and Atmospheric CO2 Driven the Recent Enhancement of the Terrestrial Carbon Sink?, Glob. Biogeochem. Cycles, 33, 163–180, https://doi.org/10.1029/2018GB005922, 2019.
O'Sullivan, M., Zhang, Y., Bellouin, N., Harris, I., Mercado, L. M., Sitch, S., Ciais, P., and Friedlingstein, P.: Aerosol–light interactions reduce the carbon budget imbalance, Environ. Res. Lett., 16, 124072, https://doi.org/10.1088/1748-9326/ac3b77, 2021.
O'Sullivan, M., Friedlingstein, P., Sitch, S., Anthoni, P., Arneth, A., Arora, V. K., Bastrikov, V., Delire, C., Goll, D. S., Jain, A., Kato, E., Kennedy, D., Knauer, J., Lienert, S., Lombardozzi, D., McGuire, P. C., Melton, J. R., Nabel, J. E. M. S., Pongratz, J., Poulter, B., Séférian, R., Tian, H., Vuichard, N., Walker, A. P., Yuan, W., Yue, X., and Zaehle, S.: Process-oriented analysis of dominant sources of uncertainty in the land carbon sink, Nat. Commun., 13, 4781, https://doi.org/10.1038/s41467-022-32416-8, 2022.
Our World in Data: CO2 Emissions, Our World in Data [data set], https://ourworldindata.org/co2-emissions (last access: 9 November 2023), 2023.
Palmer, P. I., Feng, L., Baker, D., Chevallier, F., Bösch, H., and Somkuti, P.: Net carbon emissions from African biosphere dominate pan-tropical atmospheric CO2 signal, Nat. Commun., 10, 3344, https://doi.org/10.1038/s41467-019-11097-w, 2019.
Pan, Y., Birdsey, R. A., Fang, J., Houghton, R., Kauppi, P. E., Kurz, W. A., Phillips, O. L., Shvidenko, A., Lewis, S. L., Canadell, J. G., Ciais, P., Jackson, R. B., Pacala, S. W., McGuire, A. D., Piao, S., Rautiainen, A., Sitch, S., and Hayes, D.: A Large and Persistent Carbon Sink in the World's Forests, Science, 333, 988–993, https://doi.org/10.1126/science.1201609, 2011.
Pendrill, F., Persson, U. M., Godar, J., Kastner, T., Moran, D., Schmidt, S., and Wood, R.: Agricultural and forestry trade drives large share of tropical deforestation emissions, Global Environ. Change, 56, 1–10, https://doi.org/10.1016/j.gloenvcha.2019.03.002, 2019.
Peters, G. P., Minx, J. C., Weber, C. L., and Edenhofer, O.: Growth in emission transfers via international trade from 1990 to 2008, P. Natl. Acad. Sci. USA, 108, 8903–8908, https://doi.org/10.1073/pnas.1006388108, 2011.
Peters, G. P., Marland, G., Le Quéré, C., Boden, T., Canadell, J. G., and Raupach, M. R.: Rapid growth in CO2 emissions after the 2008–2009 global financial crisis, Nat. Clim. Change, 2, 2–4, https://doi.org/10.1038/nclimate1332, 2012.
Peters, G. P., Andrew, R. M., Boden, T., Canadell, J. G., Ciais, P., Le Quéré, C., Marland, G., Raupach, M. R., and Wilson, C.: The challenge to keep global warming below 2 ∘C, Nat. Clim. Change, 3, 4–6, https://doi.org/10.1038/nclimate1783, 2013.
Peters, G. P., Le Quéré, C., Andrew, R. M., Canadell, J. G., Friedlingstein, P., Ilyina, T., Jackson, R. B., Joos, F., Korsbakken, J. I., McKinley, G. A., Sitch, S., and Tans, P.: Towards real-time verification of CO2 emissions, Nat. Clim. Change, 7, 848–850, https://doi.org/10.1038/s41558-017-0013-9, 2017.
Peters, G. P., Andrew, R. M., Canadell, J. G., Friedlingstein, P., Jackson, R. B., Korsbakken, J. I., Le Quéré, C., and Peregon, A.: Carbon dioxide emissions continue to grow amidst slowly emerging climate policies, Nat. Clim. Chang., 10, 3–6, https://doi.org/10.1038/s41558-019-0659-6, 2020.
Peters, W., Miller, J. B., Whitaker, J., Denning, A. S., Hirsch, A., Krol, M. C., Zupanski, D., Bruhwiler, L., and Tans, P. P.: An ensemble data assimilation system to estimate CO2 surface fluxes from atmospheric trace gas observations, J. Geophys. Res.-Atmos., 110, D24304, https://doi.org/10.1029/2005JD006157, 2005.
Petrescu, A. M. R., Peters, G. P., Janssens-Maenhout, G., Ciais, P., Tubiello, F. N., Grassi, G., Nabuurs, G.-J., Leip, A., Carmona-Garcia, G., Winiwarter, W., Höglund-Isaksson, L., Günther, D., Solazzo, E., Kiesow, A., Bastos, A., Pongratz, J., Nabel, J. E. M. S., Conchedda, G., Pilli, R., Andrew, R. M., Schelhaas, M.-J., and Dolman, A. J.: European anthropogenic AFOLU greenhouse gas emissions: a review and benchmark data, Earth Syst. Sci. Data, 12, 961–1001, https://doi.org/10.5194/essd-12-961-2020, 2020.
Pfeil, B., Olsen, A., Bakker, D. C. E., Hankin, S., Koyuk, H., Kozyr, A., Malczyk, J., Manke, A., Metzl, N., Sabine, C. L., Akl, J., Alin, S. R., Bates, N., Bellerby, R. G. J., Borges, A., Boutin, J., Brown, P. J., Cai, W.-J., Chavez, F. P., Chen, A., Cosca, C., Fassbender, A. J., Feely, R. A., González-Dávila, M., Goyet, C., Hales, B., Hardman-Mountford, N., Heinze, C., Hood, M., Hoppema, M., Hunt, C. W., Hydes, D., Ishii, M., Johannessen, T., Jones, S. D., Key, R. M., Körtzinger, A., Landschützer, P., Lauvset, S. K., Lefèvre, N., Lenton, A., Lourantou, A., Merlivat, L., Midorikawa, T., Mintrop, L., Miyazaki, C., Murata, A., Nakadate, A., Nakano, Y., Nakaoka, S., Nojiri, Y., Omar, A. M., Padin, X. A., Park, G.-H., Paterson, K., Perez, F. F., Pierrot, D., Poisson, A., Ríos, A. F., Santana-Casiano, J. M., Salisbury, J., Sarma, V. V. S. S., Schlitzer, R., Schneider, B., Schuster, U., Sieger, R., Skjelvan, I., Steinhoff, T., Suzuki, T., Takahashi, T., Tedesco, K., Telszewski, M., Thomas, H., Tilbrook, B., Tjiputra, J., Vandemark, D., Veness, T., Wanninkhof, R., Watson, A. J., Weiss, R., Wong, C. S., and Yoshikawa-Inoue, H.: A uniform, quality controlled Surface Ocean CO2 Atlas (SOCAT), Earth Syst. Sci. Data, 5, 125–143, https://doi.org/10.5194/essd-5-125-2013, 2013.
Piao, S., Ciais, P., Friedlingstein, P., de Noblet-Ducoudré, N., Cadule, P., Viovy, N., and Wang, T.: Spatiotemporal patterns of terrestrial carbon cycle during the 20th century, Global Biogeochem. Cy., 23, GB4026, https://doi.org/10.1029/2008GB003339, 2009.
Piao, S., Huang, M., Liu, Z., Wang, X., Ciais, P., Canadell, J. G., Wang, K., Bastos, A., Friedlingstein, P., Houghton, R. A., Le Quéré, C., Liu, Y., Myneni, R. B., Peng, S., Pongratz, J., Sitch, S., Yan, T., Wang, Y., Zhu, Z., Wu, D., and Wang, T.: Lower land-use emissions responsible for increased net land carbon sink during the slow warming period, Nat. Geosci., 11, 739–743, https://doi.org/10.1038/s41561-018-0204-7, 2018.
Pongratz, J., Reick, C. H., Houghton, R. A., and House, J. I.: Terminology as a key uncertainty in net land use and land cover change carbon flux estimates, Earth Syst. Dynam., 5, 177–195, https://doi.org/10.5194/esd-5-177-2014, 2014.
Poulter, B., Frank, D. C., Hodson, E. L., and Zimmermann, N. E.: Impacts of land cover and climate data selection on understanding terrestrial carbon dynamics and the CO2 airborne fraction, Biogeosciences, 8, 2027–2036, https://doi.org/10.5194/bg-8-2027-2011, 2011.
Poulter, B., Bastos, A., Canadell, J., Ciais, P., Gruber, N., Hauck, J., Jackson, R., Ishii, M., Müller, J., Patra, P., and Tian, H.: Inventorying Earth's Land and Ocean Greenhouse Gases, Eos, 103, https://doi.org/10.1029/2022EO179084, 2022.
Powis, C. M., Smith, S. M., Minx, J. C., and Gasser, T.: Quantifying global carbon dioxide removal deployment, Environ. Res. Lett., 18, 024022, https://doi.org/10.1088/1748-9326/acb450, 2023.
Prentice, I. C., Farquhar, G. D., Fasham, M. J. R., Goulden, M. L., Heimann, M., Jaramillo, V. J., Kheshgi, H. S., Le Quéré, C., Scholes, R. J., and Wallace, D. W. R.: The Carbon Cycle and Atmospheric Carbon Dioxide, in Climate Change 2001: The Scientific Basis. Contribution of Working Group I to the Third Assessment Report of the Intergovernmental Panel on Climate Change, edited by: Houghton, J. T., Ding, Y., Griggs, D. J., Noguer, M., van der Linden, P. J., Dai, X., Maskell, K., and Johnson, C. A., Cambridge University Press, Cambridge, United Kingdom and New York, NY, USA, 183–237, ISBN 978-0521014953, 2001.
Price, J. T. and Warren, R.: Literature Review of the Potential of “Blue Carbon” Activities to Reduce Emissions, https://avoid-net-uk.cc.ic.ac.uk/wp-content/uploads/delightful-downloads/2016/03/Literature-review-of-the-potential-of-blue-carbon-activities-to-reduce-emissions-AVOID2-WPE2.pdf (last access: 9 November 2023), 2016.
Qin, Y., Xiao, X., Wigneron, J.-P., Ciais, P., Brandt, M., Fan, L., Li, X., Crowell, S., Wu, X., Doughty, R., Zhang, Y., Liu, F., Sitch, S., and Moore, B.: Carbon loss from forest degradation exceeds that from deforestation in the Brazilian Amazon, Nat. Clim. Chang., 11, 442–448, https://doi.org/10.1038/s41558-021-01026-5, 2021.
Randerson, J. T., Chen, Y., van der Werf, G. R., Rogers, B. M., and Morton, D. C.: Global burned area and biomass burning emissions from small fires: BURNED AREA FROM SMALL FIRES, J. Geophys. Res.-Biogeo., 117, G04012, https://doi.org/10.1029/2012JG002128, 2012.
Raupach, M. R., Marland, G., Ciais, P., Le Quere, C., Canadell, J. G., Klepper, G., and Field, C. B.: Global and regional drivers of accelerating CO2 emissions, P. Natl. Acad. Sci. USA, 104, 10288–10293, https://doi.org/10.1073/pnas.0700609104, 2007.
Regnier, P., Resplandy, L., Najjar, R. G., and Ciais, P.: The land-to-ocean loops of the global carbon cycle, Nature, 603, 401–410, https://doi.org/10.1038/s41586-021-04339-9, 2022.
Reick, C. H., Gayler, V., Goll, D., Hagemann, S., Heidkamp, M., Nabel, J. E. M. S., Raddatz, T., Roeckner, E., Schnur, R., and Wilkenskjeld, S.: JSBACH 3 – The land component of the MPI Earth System Model: documentation of version 3.2, Max Plank Society [model documentation], https://doi.org/10.17617/2.3279802, 2021.
Remaud, M., Chevallier, F., Cozic, A., Lin, X., and Bousquet, P.: On the impact of recent developments of the LMDz atmospheric general circulation model on the simulation of CO2 transport, Geosci. Model Dev., 11, 4489–4513, https://doi.org/10.5194/gmd-11-4489-2018, 2018.
Resplandy, L., Keeling, R. F., Rödenbeck, C., Stephens, B. B., Khatiwala, S., Rodgers, K. B., Long, M. C., Bopp, L., and Tans, P. P.: Revision of global carbon fluxes based on a reassessment of oceanic and riverine carbon transport, Nat. Geosci., 11, 504–509, https://doi.org/10.1038/s41561-018-0151-3, 2018.
Rödenbeck, C., Houweling, S., Gloor, M., and Heimann, M.: CO2 flux history 1982–2001 inferred from atmospheric data using a global inversion of atmospheric transport, Atmos. Chem. Phys., 3, 1919–1964, https://doi.org/10.5194/acp-3-1919-2003, 2003.
Rödenbeck, C., Bakker, D. C. E., Metzl, N., Olsen, A., Sabine, C., Cassar, N., Reum, F., Keeling, R. F., and Heimann, M.: Interannual sea–air CO2 flux variability from an observation-driven ocean mixed-layer scheme, Biogeosciences, 11, 4599–4613, https://doi.org/10.5194/bg-11-4599-2014, 2014.
Rödenbeck, C., Zaehle, S., Keeling, R., and Heimann, M.: History of El Niño impacts on the global carbon cycle 1957–2017: a quantification from atmospheric CO2 data, Philos. T. Roy. Soc. B, 373, 20170303, https://doi.org/10.1098/rstb.2017.0303, 2018.
Rödenbeck, C., DeVries, T., Hauck, J., Le Quéré, C., and Keeling, R. F.: Data-based estimates of interannual sea–air CO2 flux variations 1957–2020 and their relation to environmental drivers, Biogeosciences, 19, 2627–2652, https://doi.org/10.5194/bg-19-2627-2022, 2022.
Rosan, T. M., Klein Goldewijk, K., Ganzenmüller, R., O'Sullivan, M., Pongratz, J., Mercado, L. M., Aragao, L. E. O. C., Heinrich, V., Randow, C. V., Wiltshire, A., Tubiello, F. N., Bastos, A., Friedlingstein, P., and Sitch, S.: A multi-data assessment of land use and land cover emissions from Brazil during 2000–2019, Environ. Res. Lett., 16, 074004, https://doi.org/10.1088/1748-9326/ac08c3, 2021.
Sakamoto, K., Nakano, H., Urakawa, S., Toyoda, T., Kawakami, Y., Tsujino, H., and Yamanaka, G.: Reference manual for the Meteorological Research Institute Community Ocean Model version 5 (MRI.COMv5), Technical Reports of the Meteorological Research Institute, No.87, https://doi.org/10.11483/mritechrepo.87, 2023.
Sarma, V. V. S. S., Sridevi, B., Metzl, N., Patra, P. K., Lachkar, Z., Chakraborty, K., Goyet, C., Levy, M., Mehari, M., and Chandra, N.: Air-Sea Fluxes of CO2 in the Indian Ocean Between 1985 and 2018: A Synthesis Based on Observation-Based Surface CO2, Hindcast and Atmospheric Inversion Models, Glob. Biogeochem. Cycles, 37, e2023GB007694, https://doi.org/10.1029/2023GB007694, 2023.
Schaphoff, S., von Bloh, W., Rammig, A., Thonicke, K., Biemans, H., Forkel, M., Gerten, D., Heinke, J., Jägermeyr, J., Knauer, J., Langerwisch, F., Lucht, W., Müller, C., Rolinski, S., and Waha, K.: LPJmL4 – a dynamic global vegetation model with managed land – Part 1: Model description, Geosci. Model Dev., 11, 1343–1375, https://doi.org/10.5194/gmd-11-1343-2018, 2018.
Schimel, D., Alves, D., Enting, I. G., Heimann, M., Joos, F., Raynaud, D., Wigley, T., Prater, M., Derwent, R., Ehhalt, D., Fraser, P., Sanhueza, E., Zhou, X., Jonas, P., Charlson, R., Rodhe, H., Sadasivan, S., Shine, K. P., Fouquart, Y., Ramaswamy, V., Solomon, S., Srinivasan, J., Albritton, D., Derwent, R., Isaksen, I., Lal, M., and Wuebbles, D.: Radiative Forcing of Climate Change, in: Climate Change 1995: The Science of Climate Change, Contribution of Working Group I to the Second Assessment Report of the Intergovernmental Panel on Climate Change, edited by: Houghton, J. T., Meira Rilho, L. G., Callander, B. A., Harris, N., Kattenberg, A., and Maskell, K., Cambridge University Press, Cambridge, United Kingdom and New York, NY, USA, ISBN 978-0521559621, 1995.
Schimel, D., Stephens, B. B., and Fisher, J. B.: Effect of increasing CO2 on the terrestrial carbon cycle, P. Natl. Acad. Sci. USA, 112, 436–441, https://doi.org/10.1073/pnas.1407302112, 2015.
Schuh, A. E., Jacobson, A. R., Basu, S., Weir, B., Baker, D., Bowman, K., Chevallier, F., Crowell, S., Davis, K. J., Deng, F., Denning, S., Feng, L., Jones, D., Liu, J., and Palmer, P. I.: Quantifying the Impact of Atmospheric Transport Uncertainty on CO2 Surface Flux Estimates, Global Biogeochem. Cycles, 33, 484–500, https://doi.org/10.1029/2018GB006086, 2019.
Schwinger, J., Goris, N., Tjiputra, J. F., Kriest, I., Bentsen, M., Bethke, I., Ilicak, M., Assmann, K. M., and Heinze, C.: Evaluation of NorESM-OC (versions 1 and 1.2), the ocean carbon-cycle stand-alone configuration of the Norwegian Earth System Model (NorESM1), Geosci. Model Dev., 9, 2589–2622, https://doi.org/10.5194/gmd-9-2589-2016, 2016.
Schwingshackl, C., Obermeier, W. A., Bultan, S., Grassi, G., Canadell, J. G., Friedlingstein, P., Gasser, T., Houghton, R. A., Kurz, W. A., Sitch, S., and Pongratz, J.: Differences in land-based mitigation estimates reconciled by separating natural and land-use CO2 fluxes at the country level, One Earth, 5, 1367–1376, https://doi.org/10.1016/j.oneear.2022.11.009, 2022.
Séférian, R., Nabat, P., Michou, M., Saint-Martin, D., Voldoire, A., Colin, J., Decharme, B., Delire, C., Berthet, S., Chevallier, M., Sénési, S., Franchisteguy, L., Vial, J., Mallet, M., Joetzjer, E., Geoffroy, O., Guérémy, J.-F., Moine, M.-P., Msadek, R., Ribes, A., Rocher, M., Roehrig, R., Salas-y-Mélia, D., Sanchez, E., Terray, L., Valcke, S., Waldman, R., Aumont, O., Bopp, L., Deshayes, J., Éthé, C., and Madec, G.: Evaluation of CNRM Earth System Model, CNRM-ESM2-1: Role of Earth System Processes in Present-Day and Future Climate, J. Adv. Model. Earth Sy., 11, 4182–4227, https://doi.org/10.1029/2019MS001791, 2019.
Seiler, C., Melton, J. R., Arora, V. K., Sitch, S., Friedlingstein, P., Anthoni, P., Goll, D., Jain, A. K., Joetzjer, E., Lienert, S., Lombardozzi, D., Luyssaert, S., Nabel, J. E. M. S., Tian, H., Vuichard, N., Walker, A. P., Yuan, W., and Zaehle, S.: Are Terrestrial Biosphere Models Fit for Simulating the Global Land Carbon Sink?, J. Adv. Model. Earth Sy., 14, e2021MS002946, https://doi.org/10.1029/2021MS002946, 2022.
Sellar, A. A., Jones, C. G., Mulcahy, J. P., Tang, Y., Yool, A., Wiltshire, A., O'Connor, F. M., Stringer, M., Hill, R., Palmieri, J., Woodward, S., Mora, L., Kuhlbrodt, T., Rumbold, S. T., Kelley, D. I., Ellis, R., Johnson, C. E., Walton, J., Abraham, N. L., Andrews, M. B., Andrews, T., Archibald, A. T., Berthou, S., Burke, E., Blockley, E., Carslaw, K., Dalvi, M., Edwards, J., Folberth, G. A., Gedney, N., Griffiths, P. T., Harper, A. B., Hendry, M. A., Hewitt, A. J., Johnson, B., Jones, A., Jones, C. D., Keeble, J., Liddicoat, S., Morgenstern, O., Parker, R. J., Predoi, V., Robertson, E., Siahaan, A., Smith, R. S., Swaminathan, R., Woodhouse, M. T., Zeng, G., and Zerroukat, M.: UKESM1: Description and Evaluation of the U.K. Earth System Model, J. Adv. Model. Earth Sy., 11, 4513–4558, https://doi.org/10.1029/2019MS001739, 2019.
Shu, S., Jain, A. K., Koven, C. D., and Mishra, U.: Estimation of Permafrost SOC Stock and Turnover Time Using a Land Surface Model With Vertical Heterogeneity of Permafrost Soils, Global Biogeochem. Cy., 34, e2020GB006585, https://doi.org/10.1029/2020GB006585, 2020.
Shutler, J. D., Land, P. E., Piolle, J.-F., Woolf, D. K., Goddijn-Murphy, L., Paul, F., Girard-Ardhuin, F., Chapron, B., and Donlon, C. J.: FluxEngine: A Flexible Processing System for Calculating Atmosphere–Ocean Carbon Dioxide Gas Fluxes and Climatologies, J. Atmos. Ocean. Tech., 33, 741–756, https://doi.org/10.1175/JTECH-D-14-00204.1, 2016.
Sitch, S., Huntingford, C., Gedney, N., Levy, P. E., Lomas, M., Piao, S. L., Betts, R., Ciais, P., Cox, P., Friedlingstein, P., Jones, C. D., Prentice, I. C., and Woodward, F. I.: Evaluation of the terrestrial carbon cycle, future plant geography and climate-carbon cycle feedbacks using five Dynamic Global Vegetation Models (DGVMs): Uncertainty In Land Carbon Cycle Feedbacks, Glob. Change Biol., 14, 2015–2039, https://doi.org/10.1111/j.1365-2486.2008.01626.x, 2008.
Smallman, T. L., Milodowski, D. T., Neto, E. S., Koren, G., Ometto, J., and Williams, M.: Parameter uncertainty dominates C-cycle forecast errors over most of Brazil for the 21st century, Earth Syst. Dynam., 12, 1191–1237, https://doi.org/10.5194/esd-12-1191-2021, 2021.
Smith, B., Wårlind, D., Arneth, A., Hickler, T., Leadley, P., Siltberg, J., and Zaehle, S.: Implications of incorporating N cycling and N limitations on primary production in an individual-based dynamic vegetation model, Biogeosciences, 11, 2027–2054, https://doi.org/10.5194/bg-11-2027-2014, 2014.
Smith, S., Geden, O., Nemet, G., Gidden, M., Lamb, W., Powis, C., Bellamy, R., Callaghan, M., Cowie, A., Cox, E. and Fuss, S., 2023. The State of Carbon Dioxide Removal – 1st Edition, https://doi.org/10.17605/OSF.IO/W3B4Z, 2023.
Sospedra-Alfonso, R., Merryfield, W. J., Boer, G. J., Kharin, V. V., Lee, W.-S., Seiler, C., and Christian, J. R.: Decadal climate predictions with the Canadian Earth System Model version 5 (CanESM5), Geosci. Model Dev., 14, 6863–6891, https://doi.org/10.5194/gmd-14-6863-2021, 2021.
Stephens, B. B., Keeling, R. F., Heimann, M., Six, K. D., Murnane, R., and Caldeira, K.: Testing global ocean carbon cycle models using measurements of atmospheric O2 and CO2 concentration, Glob. Biogeochem. Cycles, 12, 213–230, https://doi.org/10.1029/97GB03500, 1998.
Stephens, B. B., Gurney, K. R., Tans, P. P., Sweeney, C., Peters, W., Bruhwiler, L., Ciais, P., Ramonet, M., Bousquet, P., Nakazawa, T., Aoki, S., Machida, T., Inoue, G., Vinnichenko, N., Lloyd, J., Jordan, A., Heimann, M., Shibistova, O., Langenfelds, R. L., Steele, L. P., Francey, R. J., and Denning, A. S.: Weak Northern and Strong Tropical Land Carbon Uptake from Vertical Profiles of Atmospheric CO2, Science, 316, 1732–1735, https://doi.org/10.1126/science.1137004, 2007.
Stocker, T., Qin, D., and Platner, G.-K.: Climate Change 2013: The Physical Science Basis. Contribution of Working Group I to the Fifth Assessment Report of the Intergovernmental Panel on Climate Change, Intergovernmental Panel on Climate Change, Cambridge University Press, Cambridge, ISBN 9789291691388, 2013.
Swart, N. C., Cole, J. N. S., Kharin, V. V., Lazare, M., Scinocca, J. F., Gillett, N. P., Anstey, J., Arora, V., Christian, J. R., Hanna, S., Jiao, Y., Lee, W. G., Majaess, F., Saenko, O. A., Seiler, C., Seinen, C., Shao, A., Sigmond, M., Solheim, L., von Salzen, K., Yang, D., and Winter, B.: The Canadian Earth System Model version 5 (CanESM5.0.3), Geosci. Model Dev., 12, 4823–4873, https://doi.org/10.5194/gmd-12-4823-2019, 2019.
Takahashi, T., Sutherland, S. C., Wanninkhof, R., Sweeney, C., Feely, R. A., Chipman, D. W., Hales, B., Friederich, G., Chavez, F., Sabine, C., Watson, A., Bakker, D. C. E., Schuster, U., Metzl, N., Yoshikawa-Inoue, H., Ishii, M., Midorikawa, T., Nojiri, Y., Körtzinger, A., Steinhoff, T., Hoppema, M., Olafsson, J., Arnarson, T. S., Tilbrook, B., Johannessen, T., Olsen, A., Bellerby, R., Wong, C. S., Delille, B., Bates, N. R., and de Baar, H. J. W.: Climatological mean and decadal change in surface ocean pCO2, and net sea–air CO2 flux over the global oceans, Deep-Sea Res. Pt. II, 56, 554–577, https://doi.org/10.1016/j.dsr2.2008.12.009, 2009.
Terhaar, J., Frölicher, T. L., and Joos, F.: Southern Ocean anthropogenic carbon sink constrained by sea surface salinity, Sci. Adv., 7, eabd5964, https://doi.org/10.1126/sciadv.abd5964, 2021.
Terhaar, J., Frölicher, T. L., and Joos, F.: Observation-constrained estimates of the global ocean carbon sink from Earth system models, Biogeosciences, 19, 4431–4457, https://doi.org/10.5194/bg-19-4431-2022, 2022.
Tian, H., Xu, X., Lu, C., Liu, M., Ren, W., Chen, G., Melillo, J., and Liu, J.: Net exchanges of CO2, CH4, and N2O between China's terrestrial ecosystems and the atmosphere and their contributions to global climate warming, J. Geophys. Res.-Biogeo,, 116, G02011, https://doi.org/10.1029/2010JG001393, 2011.
Tian, H., Chen, G., Lu, C., Xu, X., Hayes, D. J., Ren, W., Pan, S., Huntzinger, D. N., and Wofsy, S. C.: North American terrestrial CO2 uptake largely offset by CH4 and N2O emissions: toward a full accounting of the greenhouse gas budget, Clim, Change, 129, 413–426, https://doi.org/10.1007/s10584-014-1072-9, 2015.
Tubiello, F. N., Conchedda, G., Wanner, N., Federici, S., Rossi, S., and Grassi, G.: Carbon emissions and removals from forests: new estimates, 1990–2020, Earth Syst. Sci. Data, 13, 1681–1691, https://doi.org/10.5194/essd-13-1681-2021, 2021.
Tuck, C.: 2022 Mineral Commodity Summary: Iron Ore, Tech. rep., U.S. Geological Survey, https://pubs.usgs.gov/periodicals/mcs2022/mcs2022-iron-ore.pdf (last access: 9 November 2023), 2022.
UNFCCC: Synthesis report for the technical assessment component of the first global stocktake, https://unfccc.int/documents/461466 (last access: 9 November 2023), 2022.
Urakawa, L. S., Tsujino, H., Nakano, H., Sakamoto, K., Yamanaka, G., and Toyoda, T.: The sensitivity of a depth-coordinate model to diapycnal mixing induced by practical implementations of the isopycnal tracer diffusion scheme, Ocean Model., 154, 101693, https://doi.org/10.1016/j.ocemod.2020.101693, 2020.
Vale, M. M., Berenguer, E., Argollo de Menezes, M., Viveiros de Castro, E. B., Pugliese de Siqueira, L., and Portela, R. de C. Q.: The COVID-19 pandemic as an opportunity to weaken environmental protection in Brazil, Biol. Conserv., 255, 108994, https://doi.org/10.1016/j.biocon.2021.108994, 2021.
van der Laan-Luijkx, I. T., van der Velde, I. R., van der Veen, E., Tsuruta, A., Stanislawska, K., Babenhauserheide, A., Zhang, H. F., Liu, Y., He, W., Chen, H., Masarie, K. A., Krol, M. C., and Peters, W.: The CarbonTracker Data Assimilation Shell (CTDAS) v1.0: implementation and global carbon balance 2001–2015, Geosci. Model Dev., 10, 2785–2800, https://doi.org/10.5194/gmd-10-2785-2017, 2017.
van der Velde, I. R., van der Werf, G. R., Houweling, S., Maasakkers, J. D., Borsdorff, T., Landgraf, J., Tol, P., van Kempen, T. A., van Hees, R., Hoogeveen, R., Veefkind, J. P., and Aben, I.: Vast CO2 release from Australian fires in 2019–2020 constrained by satellite, Nature, 597, 366–369, https://doi.org/10.1038/s41586-021-03712-y, 2021.
van der Werf, G. R., Randerson, J. T., Giglio, L., Collatz, G. J., Mu, M., Kasibhatla, P. S., Morton, D. C., DeFries, R. S., Jin, Y., and van Leeuwen, T. T.: Global fire emissions and the contribution of deforestation, savanna, forest, agricultural, and peat fires (1997–2009), Atmos. Chem. Phys., 10, 11707–11735, https://doi.org/10.5194/acp-10-11707-2010, 2010.
van der Werf, G. R., Randerson, J. T., Giglio, L., van Leeuwen, T. T., Chen, Y., Rogers, B. M., Mu, M., van Marle, M. J. E., Morton, D. C., Collatz, G. J., Yokelson, R. J., and Kasibhatla, P. S.: Global fire emissions estimates during 1997–2016, Earth Syst. Sci. Data, 9, 697–720, https://doi.org/10.5194/essd-9-697-2017, 2017.
van der Woude, A. M., Peters, W., Joetzjer, E., Lafont, S., Koren, G., Ciais, P., Ramonet, M., Xu, Y., Bastos, A., Botía, S., Sitch, S., de Kok, R., Kneuer, T., Kubistin, D., Jacotot, A., Loubet, B., Herig-Coimbra, P.-H., Loustau, D., and Luijkx, I. T.: Temperature extremes of 2022 reduced carbon uptake by forests in Europe, Nat. Commun., 14, 6218, https://doi.org/10.1038/s41467-023-41851-0, 2023.
van Wees, D., van der Werf, G. R., Randerson, J. T., Andela, N., Chen, Y., and Morton, D. C.: The role of fire in global forest loss dynamics, Glob. Change Biol., 27, 2377–2391, https://doi.org/10.1111/gcb.15591, 2021.
Vaittinada Ayar, P., Bopp, L., Christian, J. R., Ilyina, T., Krasting, J. P., Séférian, R., Tsujino, H., Watanabe, M., Yool, A., and Tjiputra, J.: Contrasting projections of the ENSO-driven CO2 flux variability in the equatorial Pacific under high-warming scenario, Earth Syst. Dynam., 13, 1097–1118, https://doi.org/10.5194/esd-13-1097-2022, 2022.
von Bloh, W., Schaphoff, S., Müller, C., Rolinski, S., Waha, K., and Zaehle, S.: Implementing the nitrogen cycle into the dynamic global vegetation, hydrology, and crop growth model LPJmL (version 5.0), Geosci. Model Dev., 11, 2789–2812, https://doi.org/10.5194/gmd-11-2789-2018, 2018.
Vuichard, N., Messina, P., Luyssaert, S., Guenet, B., Zaehle, S., Ghattas, J., Bastrikov, V., and Peylin, P.: Accounting for carbon and nitrogen interactions in the global terrestrial ecosystem model ORCHIDEE (trunk version, rev 4999): multi-scale evaluation of gross primary production, Geosci. Model Dev., 12, 4751–4779, https://doi.org/10.5194/gmd-12-4751-2019, 2019.
Walker, A. P., Quaife, T., Bodegom, P. M., De Kauwe, M. G., Keenan, T. F., Joiner, J., Lomas, M. R., MacBean, N., Xu, C., Yang, X., and Woodward, F. I.: The impact of alternative trait-scaling hypotheses for the maximum photosynthetic carboxylation rate (Vcmax) on global gross primary production, New Phytol., 215, 1370–1386, https://doi.org/10.1111/nph.14623, 2017.
Walker, A. P., De Kauwe, M. G., Bastos, A., Belmecheri, S., Georgiou, K., Keeling, R. F., McMahon, S. M., Medlyn, B. E., Moore, D. J. P., Norby, R. J., Zaehle, S., Anderson-Teixeira, K. J., Battipaglia, G., Brienen, R. J. W., Cabugao, K. G., Cailleret, M., Campbell, E., Canadell, J. G., Ciais, P., Craig, M. E., Ellsworth, D. S., Farquhar, G. D., Fatichi, S., Fisher, J. B., Frank, D. C., Graven, H., Gu, L., Haverd, V., Heilman, K., Heimann, M., Hungate, B. A., Iversen, C. M., Joos, F., Jiang, M., Keenan, T. F., Knauer, J., Körner, C., Leshyk, V. O., Leuzinger, S., Liu, Y., MacBean, N., Malhi, Y., McVicar, T. R., Penuelas, J., Pongratz, J., Powell, A. S., Riutta, T., Sabot, M. E. B., Schleucher, J., Sitch, S., Smith, W. K., Sulman, B., Taylor, B., Terrer, C., Torn, M. S., Treseder, K. K., Trugman, A. T., Trumbore, S. E., van Mantgem, P. J., Voelker, S. L., Whelan, M. E., and Zuidema, P. A.: Integrating the evidence for a terrestrial carbon sink caused by increasing atmospheric CO2, New Phytol., 229, 2413–2445, https://doi.org/10.1111/nph.16866, 2021.
Watanabe, M., Tatebe, H., Koyama, H., Hajima, T., Watanabe, M., and Kawamiya, M.: Importance of El Niño reproducibility for reconstructing historical CO2 flux variations in the equatorial Pacific, Ocean Sci., 16, 1431–1442, https://doi.org/10.5194/os-16-1431-2020, 2020.
Watson, A. J., Schuster, U., Shutler, J. D., Holding, T., Ashton, I. G. C., Landschützer, P., Woolf, D. K., and Goddijn-Murphy, L.: Revised estimates of ocean-atmosphere CO2 flux are consistent with ocean carbon inventory, Nat. Commun., 11, 4422, https://doi.org/10.1038/s41467-020-18203-3, 2020.
Watson, R. T., Rohde, H., Oeschger, H., and Siegenthaler, U.: Greenhouse Gases and Aerosols, in: Climate Change: The IPCC Scientific Assessment. Intergovernmental Panel on Climate Change (IPCC), edited by: Houghton, J. T., Jenkins, G. J., and Ephraums, J. J., Cambridge University Press, Cambridge, ISBN 978-0521403603, 1990.
Wenzel, S., Cox, P. M., Eyring, V., and Friedlingstein, P.: Projected land photosynthesis constrained by changes in the seasonal cycle of atmospheric CO2, Nature, 538, 499–501, https://doi.org/10.1038/nature19772, 2016.
Wilkenskjeld, S., Kloster, S., Pongratz, J., Raddatz, T., and Reick, C. H.: Comparing the influence of net and gross anthropogenic land-use and land-cover changes on the carbon cycle in the MPI-ESM, Biogeosciences, 11, 4817–4828, https://doi.org/10.5194/bg-11-4817-2014, 2014.
Wiltshire, A. J., Burke, E. J., Chadburn, S. E., Jones, C. D., Cox, P. M., Davies-Barnard, T., Friedlingstein, P., Harper, A. B., Liddicoat, S., Sitch, S., and Zaehle, S.: JULES-CN: a coupled terrestrial carbon–nitrogen scheme (JULES vn5.1), Geosci. Model Dev., 14, 2161–2186, https://doi.org/10.5194/gmd-14-2161-2021, 2021.
Winkler, K., Yang, H., Ganzenmüller, R., Fuchs, R., Ceccherini, G., Duveiller, G., Grassi, G., Pongratz, J., Bastos, A., Shvidenko, A., Araza, A., Herold, M., Wigneron, J.-P., and Ciais, P.: Changes in land use and management led to a decline in Eastern Europe's terrestrial carbon sink, Commun. Earth Environ., 4, 1–14, https://doi.org/10.1038/s43247-023-00893-4, 2023.
Woodward, F. I. and Lomas, M. R.: Vegetation dynamics – simulating responses to climatic change, Biol. Rev., 79, 643–670, https://doi.org/10.1017/S1464793103006419, 2004.
Wright, R. M., Le Quéré, C., Buitenhuis, E., Pitois, S., and Gibbons, M. J.: Role of jellyfish in the plankton ecosystem revealed using a global ocean biogeochemical model, Biogeosciences, 18, 1291–1320, https://doi.org/10.5194/bg-18-1291-2021, 2021.
Wunder, S., Kaimowitz, D., Jensen, S., and Feder, S.: Coronavirus, macroeconomy, and forests: What likely impacts?, For. Policy Econ., 131, 102536, https://doi.org/10.1016/j.forpol.2021.102536, 2021.
Xi, F., Davis, S. J., Ciais, P., Crawford-Brown, D., Guan, D., Pade, C., Shi, T., Syddall, M., Lv, J., Ji, L., Bing, L., Wang, J., Wei, W., Yang, K.-H., Lagerblad, B., Galan, I., Andrade, C., Zhang, Y., and Liu, Z.: Substantial global carbon uptake by cement carbonation, Nat. Geosci., 9, 880–883, https://doi.org/10.1038/ngeo2840, 2016.
Yang, D., Liu, Y., Feng, L., Wang, J., Yao, L., Cai, Z., Zhu, S., Lu, N., and Lyu, D.: The First Global Carbon Dioxide Flux Map Derived from TanSat Measurements, Adv. Atmos. Sci., 38, 1433–1443, https://doi.org/10.1007/s00376-021-1179-7, 2021.
Yang, X., Thornton, P., Ricciuto, D., Wang, Y., and Hoffman, F.: Global evaluation of terrestrial biogeochemistry in the Energy Exascale Earth System Model (E3SM) and the role of the phosphorus cycle in the historical terrestrial carbon balance, Biogeosciences, 20, 2813–2836, https://doi.org/10.5194/bg-20-2813-2023, 2023.
Yu, Z., Ciais, P., Piao, S., Houghton, R. A., Lu, C., Tian, H., Agathokleous, E., Kattel, G. R., Sitch, S., Goll, D., Yue, X., Walker, A., Friedlingstein, P., Jain, A. K., Liu, S., and Zhou, G.: Forest expansion dominates China's land carbon sink since 1980, Nat. Commun., 13, 5374, https://doi.org/10.1038/s41467-022-32961-2, 2022.
Yuan, W., Liu, D., Dong, W., Liu, S., Zhou, G., Yu, G., Zhao, T., Feng, J., Ma, Z., Chen, J., Chen, Y., Chen, S., Han, S., Huang, J., Li, L., Liu, H., Liu, S., Ma, M., Wang, Y., Xia, J., Xu, W., Zhang, Q., Zhao, X., and Zhao, L.: Multiyear precipitation reduction strongly decreases carbon uptake over northern China, J. Geophys. Res.-Biogeo., 119, 881–896, https://doi.org/10.1002/2014JG002608, 2014.
Yue, C., Ciais, P., Zhu, D., Wang, T., Peng, S. S., and Piao, S. L.: How have past fire disturbances contributed to the current carbon balance of boreal ecosystems?, Biogeosciences, 13, 675–690, https://doi.org/10.5194/bg-13-675-2016, 2016.
Yue, X. and Unger, N.: The Yale Interactive terrestrial Biosphere model version 1.0: description, evaluation and implementation into NASA GISS ModelE2, Geosci. Model Dev., 8, 2399–2417, https://doi.org/10.5194/gmd-8-2399-2015, 2015.
Zaehle, S. and Friend, A. D.: Carbon and nitrogen cycle dynamics in the O-CN land surface model: 1. Model description, site-scale evaluation, and sensitivity to parameter estimates: Site-scale evaluation of a C-N model, Global Biogeochem. Cycles, 24, GB1005, https://doi.org/10.1029/2009GB003521, 2010.
Zaehle, S., Ciais, P., Friend, A. D., and Prieur, V.: Carbon benefits of anthropogenic reactive nitrogen offset by nitrous oxide emissions, Nat. Geosci., 4, 601–605, https://doi.org/10.1038/ngeo1207, 2011.
Zaehle, S., Medlyn, B. E., De Kauwe, M. G., Walker, A. P., Dietze, M. C., Hickler, T., Luo, Y., Wang, Y.-P., El-Masri, B., Thornton, P., Jain, A., Wang, S., Warlind, D., Weng, E., Parton, W., Iversen, C. M., Gallet-Budynek, A., McCarthy, H., Finzi, A., Hanson, P. J., Prentice, I. C., Oren, R., and Norby, R. J.: Evaluation of 11 terrestrial carbon–nitrogen cycle models against observations from two temperate Free-Air CO2 Enrichment studies, New Phytol., 202, 803–822, https://doi.org/10.1111/nph.12697, 2014.
Zeng, J., Iida, Y., Matsunaga, T., and Shirai, T.: Surface ocean CO2 concentration and air-sea flux estimate by machine learning with modelled variable trends, Front. Mar. Sci., 9, 989233, https://doi.org/10.3389/fmars.2022.989233, 2022.
Zheng, B., Ciais, P., Chevallier, F., Chuvieco, E., Chen, Y., and Yang, H.: Increasing forest fire emissions despite the decline in global burned area, Sci. Adv., 7, eabh2646, https://doi.org/10.1126/sciadv.abh2646, 2021.
Zou, Y., Wang, Y., Ke, Z., Tian, H., Yang, J., and Liu, Y.: Development of a REgion-Specific Ecosystem Feedback Fire (RESFire) Model in the Community Earth System Model, J. Adv. Model. Earth Sy., 11, 417–445, https://doi.org/10.1029/2018MS001368, 2019.
Zscheischler, J., Mahecha, M. D., Avitabile, V., Calle, L., Carvalhais, N., Ciais, P., Gans, F., Gruber, N., Hartmann, J., Herold, M., Ichii, K., Jung, M., Landschützer, P., Laruelle, G. G., Lauerwald, R., Papale, D., Peylin, P., Poulter, B., Ray, D., Regnier, P., Rödenbeck, C., Roman-Cuesta, R. M., Schwalm, C., Tramontana, G., Tyukavina, A., Valentini, R., van der Werf, G., West, T. O., Wolf, J. E., and Reichstein, M.: Reviews and syntheses: An empirical spatiotemporal description of the global surface–atmosphere carbon fluxes: opportunities and data limitations, Biogeosciences, 14, 3685–3703, https://doi.org/10.5194/bg-14-3685-2017, 2017.
- Article
(12950 KB) - Full-text XML