the Creative Commons Attribution 4.0 License.
the Creative Commons Attribution 4.0 License.
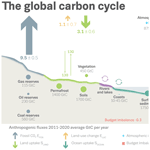
Global Carbon Budget 2021
Pierre Friedlingstein
Matthew W. Jones
Michael O'Sullivan
Robbie M. Andrew
Dorothee C. E. Bakker
Judith Hauck
Corinne Le Quéré
Glen P. Peters
Wouter Peters
Julia Pongratz
Stephen Sitch
Josep G. Canadell
Philippe Ciais
Rob B. Jackson
Simone R. Alin
Peter Anthoni
Nicholas R. Bates
Meike Becker
Nicolas Bellouin
Laurent Bopp
Thi Tuyet Trang Chau
Frédéric Chevallier
Louise P. Chini
Margot Cronin
Kim I. Currie
Bertrand Decharme
Laique M. Djeutchouang
Xinyu Dou
Wiley Evans
Richard A. Feely
Liang Feng
Thomas Gasser
Dennis Gilfillan
Thanos Gkritzalis
Giacomo Grassi
Luke Gregor
Nicolas Gruber
Özgür Gürses
Ian Harris
Richard A. Houghton
George C. Hurtt
Yosuke Iida
Tatiana Ilyina
Ingrid T. Luijkx
Atul Jain
Steve D. Jones
Etsushi Kato
Daniel Kennedy
Kees Klein Goldewijk
Jürgen Knauer
Jan Ivar Korsbakken
Arne Körtzinger
Peter Landschützer
Siv K. Lauvset
Nathalie Lefèvre
Sebastian Lienert
Junjie Liu
Gregg Marland
Patrick C. McGuire
Joe R. Melton
David R. Munro
Julia E. M. S. Nabel
Shin-Ichiro Nakaoka
Yosuke Niwa
Tsuneo Ono
Denis Pierrot
Benjamin Poulter
Gregor Rehder
Laure Resplandy
Eddy Robertson
Christian Rödenbeck
Thais M. Rosan
Jörg Schwinger
Clemens Schwingshackl
Roland Séférian
Adrienne J. Sutton
Colm Sweeney
Toste Tanhua
Pieter P. Tans
Hanqin Tian
Bronte Tilbrook
Francesco Tubiello
Guido R. van der Werf
Nicolas Vuichard
Chisato Wada
Rik Wanninkhof
Andrew J. Watson
David Willis
Andrew J. Wiltshire
Wenping Yuan
Sönke Zaehle
Jiye Zeng
Accurate assessment of anthropogenic carbon dioxide (CO2) emissions and their redistribution among the atmosphere, ocean, and terrestrial biosphere in a changing climate is critical to better understand the global carbon cycle, support the development of climate policies, and project future climate change. Here we describe and synthesize datasets and methodology to quantify the five major components of the global carbon budget and their uncertainties. Fossil CO2 emissions (EFOS) are based on energy statistics and cement production data, while emissions from land-use change (ELUC), mainly deforestation, are based on land use and land-use change data and bookkeeping models. Atmospheric CO2 concentration is measured directly, and its growth rate (GATM) is computed from the annual changes in concentration. The ocean CO2 sink (SOCEAN) is estimated with global ocean biogeochemistry models and observation-based data products. The terrestrial CO2 sink (SLAND) is estimated with dynamic global vegetation models. The resulting carbon budget imbalance (BIM), the difference between the estimated total emissions and the estimated changes in the atmosphere, ocean, and terrestrial biosphere, is a measure of imperfect data and understanding of the contemporary carbon cycle. All uncertainties are reported as ±1σ. For the first time, an approach is shown to reconcile the difference in our ELUC estimate with the one from national greenhouse gas inventories, supporting the assessment of collective countries' climate progress.
For the year 2020, EFOS declined by 5.4 % relative to 2019, with fossil emissions at 9.5 ± 0.5 GtC yr−1 (9.3 ± 0.5 GtC yr−1 when the cement carbonation sink is included), and ELUC was 0.9 ± 0.7 GtC yr−1, for a total anthropogenic CO2 emission of 10.2 ± 0.8 GtC yr−1 (37.4 ± 2.9 GtCO2). Also, for 2020, GATM was 5.0 ± 0.2 GtC yr−1 (2.4 ± 0.1 ppm yr−1), SOCEAN was 3.0 ± 0.4 GtC yr−1, and SLAND was 2.9 ± 1 GtC yr−1, with a BIM of −0.8 GtC yr−1. The global atmospheric CO2 concentration averaged over 2020 reached 412.45 ± 0.1 ppm. Preliminary data for 2021 suggest a rebound in EFOS relative to 2020 of +4.8 % (4.2 % to 5.4 %) globally.
Overall, the mean and trend in the components of the global carbon budget are consistently estimated over the period 1959–2020, but discrepancies of up to 1 GtC yr−1 persist for the representation of annual to semi-decadal variability in CO2 fluxes. Comparison of estimates from multiple approaches and observations shows (1) a persistent large uncertainty in the estimate of land-use changes emissions, (2) a low agreement between the different methods on the magnitude of the land CO2 flux in the northern extra-tropics, and (3) a discrepancy between the different methods on the strength of the ocean sink over the last decade. This living data update documents changes in the methods and datasets used in this new global carbon budget and the progress in understanding of the global carbon cycle compared with previous publications of this dataset (Friedlingstein et al., 2020, 2019; Le Quéré et al., 2018b, a, 2016, 2015b, a, 2014, 2013). The data presented in this work are available at https://doi.org/10.18160/gcp-2021 (Friedlingstein et al., 2021).
Global fossil CO2 emissions (excluding cement carbonation) in 2021 are returning towards their 2019 levels after decreasing 5.4 % in 2020. The 2020 decrease was 0.52 GtC yr−1 (1.9 GtCO2 yr−1), bringing 2020 emissions to 9.5 ± 0.5 GtC yr−1 (34.8 ± 1.8 GtCO2 yr−1), comparable to the emissions level of 2012. Preliminary estimates based on data available in March 2022 suggest fossil CO2 emissions rebounded 4.8 % in 2021 (4.2 % to 5.4 %), bringing emissions to 9.9 GtC yr−1 (36.4 GtCO2 yr−1), back to about the same level as in 2019 (10.0 ± 0.5 GtC yr−1, 36.7 ± 1.8 GtCO2 yr−1). Emissions from coal and gas in 2021 are expected to have rebounded above 2019 levels, while emissions from oil were still below their 2019 level. Emissions are expected to have been 5.7 % higher in 2021 than in 2019 in China, reaching 3.0 GtC (11.1 GtCO2), and also higher in India with a 3.2 % increase in 2021 relative to 2019, reaching 0.74 GtC (2.7 GtCO2). In contrast, projected 2021 emissions in the United States (1.4 GtC, 5.0 GtCO2), European Union (0.8 GtC, 2.8 GtCO2), and the rest of the world (4.0 GtC, 14.8 GtCO2, in aggregate) remained respectively 4.5 %, 5.3 %, and 4.0 % below their 2019 levels. These changes in 2021 emissions reflect the stringency of the COVID-19 confinement levels in 2020 and the pre-covid background trends in emissions in these countries.
Fossil CO2 emissions significantly decreased in 23 countries during the decade 2010–2019. Altogether, these 23 countries contribute to about 2.5 GtC yr−1 fossil fuel CO2 emissions over the last decade, only about one-quarter of world CO2 fossil emissions.
Global CO2 emissions from land use, land-use change, and forestry (LUC) converge based on revised data of land-use change and show a small decrease over the past two decades. Near-constant gross emissions estimated at 3.8 ± 0.6 GtC yr−1 in the 2011–2020 decade are only partly offset by growing carbon removals on managed land of 2.7 ± 0.4 GtC yr−1, resulting in the net emissions in managed land of 1.1 ± 0.7 GtC yr−1 (4.1 ± 2.6 GtCO2 yr−1). These net emissions decreased by 0.2 GtC in 2020 compared to 2019 levels, with large uncertainty. Preliminary estimates for emissions in 2021 suggest a 0.1 GtC decrease for 2021, giving net emissions of 0.8 GtC yr−1 (2.9 GtCO2 yr−1). The small decrease in net LUC emissions amidst large uncertainty prohibits robust conclusions concerning trend changes of total anthropogenic emissions. For the first time, we link the global carbon budget models' estimates to the official country reporting of national greenhouse gases inventories. While the global carbon budget distinguishes anthropogenic from natural drivers of land carbon fluxes, country reporting is area-based and attributes part of the natural terrestrial sink on managed land to the land-use sector. Accounting for this redistribution, the two approaches are shown to be consistent with each other. The remaining carbon budget for a 50 % likelihood to limit global warming to 1.5, 1.7, and 2 ∘C has respectively reduced to 120 GtC (420 GtCO2), 210 GtC (770 GtCO2) and 350 GtC (1270 GtCO2) from the beginning of 2022, equivalent to 11, 20, and 32 years, assuming 2021 emissions levels. Total anthropogenic emissions were 10.4 GtC yr−1 (38.0 GtCO2 yr−1) in 2020, with a preliminary estimate of 10.7 GtC yr−1 (39.3 GtCO2 yr−1) for 2021. The remaining carbon budget to keep global temperatures below these climate targets has shrunk by 21 GtC (77 GtCO2) since the release of the IPCC AR6 Working Group 1 assessment. Reaching zero CO2 emissions by 2050 entails cutting total anthropogenic CO2 emissions by about 0.4 GtC (1.4 GtCO2) each year on average, comparable to the decrease during 2020, highlighting the scale of the action needed.
The concentration of CO2 in the atmosphere is set to reach 414.7 ppm in 2021, 50 % above pre-industrial levels. The atmospheric CO2 growth was 5.1 ± 0.02 GtC yr−1 during the decade 2011–2020 (47 % of total CO2 emissions) with a preliminary 2021 growth rate estimate of around 5 GtC yr−1.
The ocean CO2 sink resumed a more rapid growth in the past decade after low or no growth during the 1991–2002 period. However, the growth of the ocean CO2 sink in the past decade has an uncertainty of a factor of 3, with estimates based on data products and estimates based on models showing an ocean sink increase of 0.9 and 0.3 GtC yr−1 since 2010, respectively. The discrepancy in the trend originates from all latitudes but is largest in the Southern Ocean. The ocean CO2 sink was 2.8 ± 0.4 GtC yr−1 during the decade 2011–2020 (26 % of total CO2 emissions), with a preliminary 2021 estimate of around 2.9 GtC yr−1.
The land CO2 sink continued to increase during the 2011–2020 period primarily in response to increased atmospheric CO2, albeit with large interannual variability. The land CO2 sink was 3.1 ± 0.6 GtC yr−1 during the 2011–2020 decade (29 % of total CO2 emissions), 0.5 GtC yr−1 larger than during the previous decade (2000–2009), with a preliminary 2021 estimate of around 3.3 GtC yr−1. Year-to-year variability in the land sink is about 1 GtC yr−1, making small annual changes in anthropogenic emissions hard to detect in global atmospheric CO2 concentration.
The concentration of carbon dioxide (CO2) in the atmosphere has increased from approximately 277 parts per million (ppm) in 1750 (Joos and Spahni, 2008), the beginning of the industrial era, to 412.4 ± 0.1 ppm in 2020 (Dlugokencky and Tans, 2022; Fig. 1). The atmospheric CO2 increase above pre-industrial levels was, initially, primarily caused by the release of carbon to the atmosphere from deforestation and other land-use change activities (Canadell et al., 2022). While emissions from fossil fuels started before the Industrial Era, they became the dominant source of anthropogenic emissions to the atmosphere from around 1950 and their relative share has continued to increase until the present. Anthropogenic emissions occur on top of an active natural carbon cycle that circulates carbon between the reservoirs of the atmosphere, ocean, and terrestrial biosphere on timescales from sub-daily to millennial, while exchanges with geologic reservoirs occur on longer timescales (Archer et al., 2009).
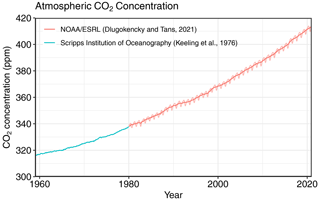
Figure 1Surface average atmospheric CO2 concentration (ppm). Since 1980, monthly data are from NOAA/ESRL (Dlugokencky and Tans, 2022) and are based on an average of direct atmospheric CO2 measurements from multiple stations in the marine boundary layer (Masarie and Tans, 1995). The 1958–1979 monthly data are from the Scripps Institution of Oceanography, based on an average of direct atmospheric CO2 measurements from the Mauna Loa and South Pole stations (Keeling et al., 1976). To account for the difference of mean CO2 and seasonality between the NOAA/ESRL and the Scripps station networks used here, the Scripps surface average (from two stations) was de-seasonalized and adjusted to match the NOAA/ESRL surface average (from multiple stations) by adding the mean difference of 0.667 ppm, calculated here from overlapping data during 1980–2012.
The global carbon budget (GCB) presented here refers to the mean, variations, and trends in the perturbation of CO2 in the environment, referenced to the beginning of the Industrial Era (defined here as 1750). This paper describes the components of the global carbon cycle over the historical period with a stronger focus on the recent period (since 1958, onset of atmospheric CO2 measurements), the last decade (2011–2020), the last year (2020), and the current year (2021). We quantify the input of CO2 to the atmosphere by emissions from human activities, the growth rate of atmospheric CO2 concentration, and the resulting changes in the storage of carbon in the land and ocean reservoirs in response to increasing atmospheric CO2 levels, climate change and variability, and other anthropogenic and natural changes (Fig. 2). An understanding of this perturbation budget over time and the underlying variability and trends of the natural carbon cycle is necessary to understand the response of natural sinks to changes in climate, CO2, and land-use change drivers, and to quantify emissions compatible with a given climate stabilization target.
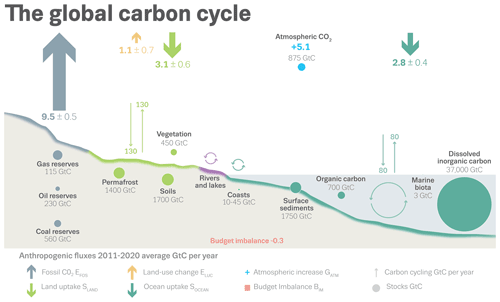
Figure 2Schematic representation of the overall perturbation of the global carbon cycle caused by anthropogenic activities, averaged globally for the decade 2011–2020. See legends for the corresponding arrows and units. The uncertainty in the atmospheric CO2 growth rate is very small (±0.02 GtC yr−1) and is neglected for the figure. The anthropogenic perturbation occurs on top of an active carbon cycle, with fluxes and stocks represented in the background and taken from Canadell et al. (2022) for all numbers, except for the carbon stocks in coasts which is from a literature review of coastal marine sediments (Price and Warren, 2016).
The components of the CO2 budget that are reported annually in this paper include separate and independent estimates for the CO2 emissions from (1) fossil fuel combustion and oxidation from all energy and industrial processes, also including cement production and carbonation (EFOS; GtC yr−1); (2) the emissions resulting from deliberate human activities on land, including those leading to land-use change (ELUC; GtC yr−1); and their partitioning among (3) the growth rate of atmospheric CO2 concentration (GATM; GtC yr−1), and the uptake of CO2 (the “CO2 sinks”) in (4) the ocean (SOCEAN; GtC yr−1) and (5) on land (SLAND; GtC yr−1). The CO2 sinks as defined here conceptually include the response of the land (including inland waters and estuaries) and ocean (including coasts and territorial seas) to elevated CO2 and changes in climate and other environmental conditions, although in practice not all processes are fully accounted for (see Sect. 2.7). Global emissions and their partitioning among the atmosphere, ocean, and land are in reality in balance. Due to the combination of imperfect spatial and/or temporal data coverage, errors in each estimate, and smaller terms not included in our budget estimate (discussed in Sect. 2.7), the independent estimates (1) to (5) above do not necessarily add up to zero. We therefore (a) additionally assess a set of global atmospheric inverse model results that by design close the global carbon balance (see Sect. 2.6), and (b) estimate a budget imbalance (BIM), which is a measure of the mismatch between the estimated emissions and the estimated changes in the atmosphere, land, and ocean, as follows:
GATM is usually reported in ppm yr−1, which we convert to units of carbon mass per year, GtC yr−1, using 1 ppm = 2.124 GtC (Ballantyne et al., 2012; Table 1). All quantities are presented in units of gigatonnes of carbon (GtC, 1015 gC), which is the same as petagrammes of carbon (PgC; Table 1). Units of gigatonnes of CO2 (or billion tonnes of CO2) used in policy are equal to 3.664 multiplied by the value in units of GtC.
Table 1Factors used to convert carbon in various units (by convention, Unit 1 = Unit 2× conversion).

a Measurements of atmospheric CO2 concentration have units of dry-air mole fraction; “ppm” is an abbreviation for micromole per mole of dry air. b The use of a factor of 2.124 assumes that all the atmosphere is well mixed within 1 year. In reality, only the troposphere is well mixed, and the growth rate of CO2 concentration in the less well-mixed stratosphere is not measured by sites from the NOAA network. Using a factor of 2.124 makes the approximation that the growth rate of CO2 concentration in the stratosphere is equal to that of the troposphere on a yearly basis.
We also include a quantification of EFOS by country, computed with both territorial and consumption-based accounting (see Sect. 2), and discuss missing terms from sources other than the combustion of fossil fuels (see Sect. 2.7).
The global CO2 budget has been assessed by the Intergovernmental Panel on Climate Change (IPCC) in all assessment reports (Prentice et al., 2001; Schimel et al., 1995; Watson et al., 1990; Denman et al., 2007; Ciais et al., 2013; Canadell et al., 2022), and by others (e.g. Ballantyne et al., 2012). The Global Carbon Project (GCP, https://www.globalcarbonproject.org, last access: 11 March 2022) has coordinated this cooperative community effort for the annual publication of global carbon budgets for the year 2005 (Raupach et al., 2007; including fossil emissions only), year 2006 (Canadell et al., 2007), year 2007 (GCP, 2007), year 2008 (Le Quéré et al., 2009), year 2009 (Friedlingstein et al., 2010), year 2010 (Peters et al., 2012b), year 2012 (Le Quéré et al., 2013; Peters et al., 2013), year 2013 (Le Quéré et al., 2014), year 2014 (Le Quéré et al., 2015a; Friedlingstein et al., 2014), year 2015 (Jackson et al., 2016; Le Quéré et al., 2015b), year 2016 (Le Quéré et al., 2016), year 2017 (Le Quéré et al., 2018a; Peters et al., 2017), year 2018 (Le Quéré et al., 2018b; Jackson et al., 2018), year 2019 (Friedlingstein et al., 2019; Jackson et al., 2019; Peters et al., 2020), and more recently the year 2020 (Friedlingstein et al., 2020; Le Quéré et al., 2021). Each of these papers updated previous estimates with the latest available information for the entire time series.
We adopt a range of ±1 standard deviation (σ) to report the uncertainties in our estimates, representing a likelihood of 68 % that the true value will be within the provided range if the errors have a Gaussian distribution, and no bias is assumed. This choice reflects the difficulty of characterizing the uncertainty in the CO2 fluxes between the atmosphere and the ocean and land reservoirs individually, particularly on an annual basis, as well as the difficulty of updating the CO2 emissions from land-use change. A likelihood of 68 % provides an indication of our current capability to quantify each term and its uncertainty given the available information. The uncertainties reported here combine statistical analysis of the underlying data, assessments of uncertainties in the generation of the datasets, and expert judgement of the likelihood of results lying outside this range. The limitations of current information are discussed in the paper and have been examined in detail elsewhere (Ballantyne et al., 2015; Zscheischler et al., 2017). We also use a qualitative assessment of confidence level to characterize the annual estimates from each term based on the type, amount, quality, and consistency of the evidence as defined by the IPCC (Stocker et al., 2013).
This paper provides a detailed description of the datasets and methodology used to compute the global carbon budget estimates for the industrial period, from 1750 to 2020, and in more detail for the period since 1959. It also provides decadal averages starting in 1960 including the most recent decade (2011–2020), results for the year 2020, and a projection for the year 2021. Finally, it provides cumulative emissions from fossil fuels and land-use change since the year 1750, the pre-industrial period; and since the year 1850, the reference year for historical simulations in IPCC AR6 (Eyring et al., 2016). This paper is updated every year using the format of “living data” to keep a record of budget versions and the changes in new data, revision of data, and changes in methodology that lead to changes in estimates of the carbon budget. Additional materials associated with the release of each new version will be posted at the Global Carbon Project (GCP) website (http://www.globalcarbonproject.org/carbonbudget, last access: 11 March 2022), with fossil fuel emissions also available through the Global Carbon Atlas (http://www.globalcarbonatlas.org, last access: 11 March 2022). With this approach, we aim to provide the highest transparency and traceability in the reporting of CO2, the key driver of climate change.
Multiple organizations and research groups around the world generated the original measurements and data used to complete the global carbon budget. The effort presented here is thus mainly one of synthesis, where results from individual groups are collated, analysed, and evaluated for consistency. We facilitate access to original data with the understanding that primary datasets will be referenced in future work (see Table 2 for how to cite the datasets). Descriptions of the measurements, models, and methodologies follow below, and detailed descriptions of each component are provided elsewhere.
This is the 16th version of the global carbon budget and the 10th revised version in the format of a living data update in Earth System Science Data. It builds on the latest published global carbon budget of Friedlingstein et al. (2020). The main changes are as follows: the inclusion of (1) data to year 2020 and a projection for the global carbon budget for year 2021, (2) a Kaya analysis to identify the driving factors behind the recent trends in fossil fuel emissions (changes in population, GDP per person, energy use per GDP, and CO2 emissions per unit energy), (3) an estimate of the ocean sink from models and data products combined, (4) an assessment of the relative contributions of increased atmospheric CO2 and climate change in driving the land and ocean sinks, and (5) an assessment of the current trends in anthropogenic emissions and implications for the remaining carbon budget for specific climate targets. The main methodological differences between recent annual carbon budgets (2016–2020) are summarized in Table 3 and previous changes since 2006 are provided in Table A7.
Table 3Main methodological changes in the global carbon budget since 2017. Methodological changes introduced in one year are kept for the following years unless noted. Empty cells mean there were no methodological changes introduced that year. Table A7 lists methodological changes from the first global carbon budget publication up to 2016.
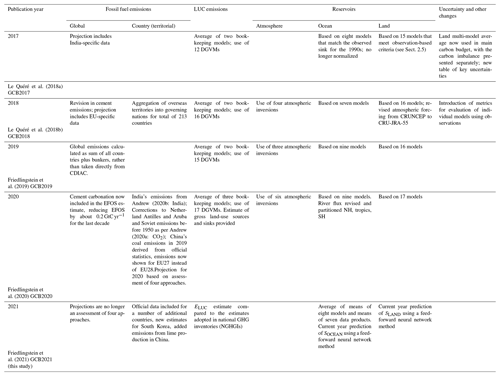
2.1 Fossil CO2 emissions (EFOS)
2.1.1 Historical period 1850–2020
The estimates of global and national fossil CO2 emissions (EFOS) include the oxidation of fossil fuels through both combustion (e.g. transport, heating) and chemical oxidation (e.g. carbon anode decomposition in aluminium refining) activities, and the decomposition of carbonates in industrial processes (e.g. the production of cement). We also include CO2 uptake from the cement carbonation process. Several emissions sources are not estimated or not fully covered: coverage of emissions from lime production are not global, and decomposition of carbonates in glass and ceramic production are included only for the “Annex 1” countries of the United Nations Framework Convention on Climate Change (UNFCCC) due to a lack of activity data. These omissions are considered to be minor. Short-cycle carbon emissions – for example from combustion of biomass – are not included here but are accounted for in the CO2 emissions from land use (see Sect. 2.2).
Our estimates of fossil CO2 emissions are derived using the standard approach of activity data and emission factors, relying on data collection by many other parties. Our goal is to produce the best estimate of this flux, and we therefore use a prioritization framework to combine data from different sources that have used different methods, while being careful to avoid double counting and undercounting of emissions sources. The CDIAC-FF emissions dataset, derived largely from UN energy data, forms the foundation, and we extend emissions to year Y-1 using energy growth rates reported by BP. We then proceed to replace estimates using data from what we consider to be superior sources, for example Annex 1 countries' official submissions to the UNFCCC. All data points are potentially subject to revision, not just the latest year. For full details see Andrew and Peters (2021).
Other estimates of global fossil CO2 emissions exist, and these are compared by Andrew (2020a). The most common reason for differences in estimates of global fossil CO2 emissions is a difference in which emissions sources are included in the datasets. Datasets such as those published by BP energy company, the US Energy Information Administration, and the International Energy Agency's “CO2 emissions from fuel combustion” are all generally limited to emissions from combustion of fossil fuels. In contrast, datasets such as PRIMAP-hist, CEDS, EDGAR, and GCP's dataset aim to include all sources of fossil CO2 emissions. See Andrew (2020a) for detailed comparisons and discussion.
Cement absorbs CO2 from the atmosphere over its lifetime, a process known as “cement carbonation”. We estimate this CO2 sink as the average of two studies in the literature (Cao et al., 2020; Guo et al., 2021). Both studies use the same model, developed by Xi et al. (2016), with different parameterizations and input data. Since carbonation is a function of both current and previous cement production, we extend these estimates by 1 year to 2020 by using the growth rate derived from the smoothed cement emissions (10-year smoothing) fitted to the carbonation data.
We use the Kaya identity for a simple decomposition of CO2 emissions into the key drivers (Raupach et al., 2007). While there are variations (Peters et al., 2017), we focus here on a decomposition of CO2 emissions into population, GDP per person, energy use per GDP, and CO2 emissions per energy use. Multiplying these individual components together returns the CO2 emissions. Using the decomposition, it is possible to attribute the change in CO2 emissions to the change in each of the drivers. This method gives a first-order understanding of what causes CO2 emissions to change each year.
2.1.2 2021 projection
We provide a projection of global CO2 emissions in 2021 by combining separate projections for China, the USA, the EU, India, and for all other countries combined. The methods are different for each of these. For China we combine monthly fossil fuel production data from the National Bureau of Statistics, import and export data from the Customs Administration, and monthly coal consumption estimates from SX Coal (2021), giving us partial data for the growth rates to date of natural gas, petroleum, and cement, and of the consumption itself for raw coal. We then use a regression model to project full-year emissions based on historical observations. For the USA our projection is taken directly from the Energy Information Administration's (EIA) Short-Term Energy Outlook (EIA, 2022), combined with the year-to-date growth rate of cement production. For the EU we use monthly energy data from Eurostat to derive estimates of monthly CO2 emissions through July, with coal emissions extended first through September using a statistical relationship with reported electricity generation from coal and other factors, then through December assuming normal seasonal patterns. EU emissions from natural gas – a strongly seasonal cycle – are extended through December using bias-adjusted Holt–Winters exponential smoothing (Chatfield, 1978). EU emissions from oil are derived using the EIA's projection of oil consumption for Europe. EU cement emissions are based on available year-to-date data from two of the largest producers, Germany and Poland. India's projected emissions are derived from estimates through August (September for coal) using the methods of Andrew (2020b) and extrapolated assuming normal seasonal patterns. Emissions for the rest of the world are derived using projected growth in economic production from the IMF (2022) combined with extrapolated changes in emissions intensity of economic production. More details on the EFOS methodology and its 2021 projection can be found in Appendix C1.
2.2 CO2 emissions from land use, land-use change, and forestry (ELUC)
The net CO2 flux from land use, land-use change, and forestry (ELUC, called land-use change emissions in the rest of the text) includes CO2 fluxes from deforestation, afforestation, logging and forest degradation (including harvest activity), shifting cultivation (cycle of cutting forest for agriculture, then abandoning), and regrowth of forests following wood harvest or abandonment of agriculture. Emissions from peat burning and drainage are added from external datasets.
Three bookkeeping approaches (updated estimates of BLUE (Hansis et al., 2015), OSCAR (Gasser et al., 2020), and H&N2017 (Houghton and Nassikas, 2017)) were used to quantify gross sources and sinks and the resulting net ELUC. Uncertainty estimates were derived from the dynamic global vegetation model (DGVM) ensemble for the time period prior to 1960, using for the recent decades an uncertainty range of ±0.7 GtC yr−1, which is a semi-quantitative measure for annual and decadal emissions and reflects our best value judgement that there is at least 68 % chance (±1σ) that the true land-use change emission lies within the given range, for the range of processes considered here. This uncertainty range had been increased from 0.5 GtC yr−1 after new bookkeeping models were included that indicated a larger spread than assumed before (Le Quéré et al., 2018). Projections for 2021 are based on fire activity from tropical deforestation and degradation as well as emissions from peat fires and drainage.
Our ELUC estimates follow the definition of global carbon cycle models of CO2 fluxes related to land use and land management and differ from IPCC definitions adopted in national GHG inventories (NGHGIs) for reporting under the UNFCCC, which additionally generally include, through adoption of the IPCC so-called managed land proxy approach, the terrestrial fluxes occurring on land defined by countries as managed. This partly includes fluxes due to environmental change (e.g. atmospheric CO2 increase), which are part of SLAND in our definition. This causes the global emission estimates to be smaller for NGHGIs than for the global carbon budget definition (Grassi et al., 2018). The same is the case for the Food Agriculture Organization (FAO) estimates of carbon fluxes on forest land, which include, compared to SLAND, both anthropogenic and natural sources on managed land (Tubiello et al., 2021). Using the approach outlined in Grassi et al. (2021), here we map as additional information the two definitions to each other, to provide a comparison of the anthropogenic carbon budget to the official country reporting to the climate convention. More details on the ELUC methodology can be found in Appendix C2.
2.3 Growth rate in atmospheric CO2 concentration (GATM)
2.3.1 Historical period
The rate of growth of the atmospheric CO2 concentration is provided for years 1959–2020 by the US National Oceanic and Atmospheric Administration Earth System Research Laboratory (NOAA/ESRL; Dlugokencky and Tans, 2022), which is updated from Ballantyne et al. (2012) and includes recent revisions to the calibration scale of atmospheric CO2 measurements (Hall et al., 2021). For the 1959–1979 period, the global growth rate is based on measurements of atmospheric CO2 concentration averaged from the Mauna Loa and South Pole stations, as observed by the CO2 Program at Scripps Institution of Oceanography (Keeling et al., 1976). For the 1980–2020 time period, the global growth rate is based on the average of multiple stations selected from the marine boundary layer sites with well-mixed background air (Ballantyne et al., 2012), after fitting each station with a smoothed curve as a function of time, and averaging by latitude band (Masarie and Tans, 1995). The annual growth rate is estimated by Dlugokencky and Tans (2022) from atmospheric CO2 concentration by taking the average of the most recent December–January months corrected for the average seasonal cycle and subtracting this same average 1 year earlier. The growth rate in units of ppm yr−1 is converted to units of GtC yr−1 by multiplying by a factor of 2.124 GtC ppm−1, assuming instantaneous mixing of CO2 throughout the atmosphere (Ballantyne et al., 2012).
Starting in 2020, NOAA/ESRL now provides estimates of atmospheric CO2 concentrations with respect to a new calibration scale, referred to as WMO-CO2-X2019, in line with the recommendation of the World Meteorological Organization (WMO) Global Atmosphere Watch (GAW) community (Hall et al., 2021). The WMO-CO2-X2019 scale improves upon the earlier WMO-CO2-X2007 scale by including a broader set of standards, which contain CO2 in a wider range of concentrations that span the range 250–800 ppm (versus 250–520 ppm for WMO-CO2-X2007). In addition, NOAA/ESRL made two minor corrections to the analytical procedure used to quantify CO2 concentrations, fixing an error in the second virial coefficient of CO2 and accounting for loss of a small amount of CO2 to materials in the manometer during the measurement process. The difference in concentrations measured using WMO-CO2-X2019 versus WMO-CO2-X2007 is 0.18 ppm at 400 ppm and the observational record of atmospheric CO2 concentrations have been revised accordingly. The revisions have been applied retrospectively in all cases where the calibrations were performed by NOAA/ESRL, thus affecting measurements made by members of the WMO-GAW programme and other regionally coordinated programmes (e.g. Integrated Carbon Observing System, ICOS). Changes to the CO2 concentrations measured across these networks propagate to the global mean CO2 concentrations. Comparing the estimates of GATM made by Dlugokencky and Tans (2020), used in the Global Carbon Budget 2020 (Friedlingstein et al., 2020), with updated estimates from Dlugokencky and Tans (2022), used here, we find that GATM reduced on average by −0.06 GtC yr−1 during 2010–2019 and by −0.01 GtC yr−1 during 1959–2019 due to the new calibration. These changes are well within the uncertainty ranges reported below. Hence the change in analytical procedures made by NOAA/ESRL has a negligible impact on the atmospheric growth rate GATM.
The uncertainty around the atmospheric growth rate is due to four main factors. First, the long-term reproducibility of reference gas standards (around 0.03 ppm for 1σ from the 1980s; Dlugokencky and Tans, 2022). Second, small unexplained systematic analytical errors that may have a duration of several months to 2 years come and go. They have been simulated by randomizing both the duration and the magnitude (determined from the existing evidence) in a Monte Carlo procedure. Third, the network composition of the marine boundary layer with some sites coming or going, gaps in the time series at each site, etc. (Dlugokencky and Tans, 2022). The latter uncertainty was estimated by NOAA/ESRL with a Monte Carlo method by constructing 100 “alternative” networks (Masarie and Tans, 1995; NOAA/ESRL, 2019). The second and third uncertainties, summed in quadrature, add up to 0.085 ppm on average (Dlugokencky and Tans, 2022). Fourth, the uncertainty associated with using the average CO2 concentration from a surface network to approximate the true atmospheric average CO2 concentration (mass-weighted, in three dimensions) as needed to assess the total atmospheric CO2 burden. In reality, CO2 variations measured at the stations will not exactly track changes in total atmospheric burden, with offsets in magnitude and phasing due to vertical and horizontal mixing. This effect must be very small on decadal and longer timescales, when the atmosphere can be considered well mixed. Preliminary estimates suggest this effect would increase the annual uncertainty, but a full analysis is not yet available. We therefore maintain an uncertainty around the annual growth rate based on the multiple stations' dataset ranges between 0.11 and 0.72 GtC yr−1, with a mean of 0.61 GtC yr−1 for 1959–1979 and 0.17 GtC yr−1 for 1980–2020, when a larger set of stations were available as provided by Dlugokencky and Tans (2022), but recognize further exploration of this uncertainty is required. At this time, we estimate the uncertainty of the decadal averaged growth rate after 1980 at 0.02 GtC yr−1 based on the calibration and the annual growth rate uncertainty but stretched over a 10-year interval. For years prior to 1980, we estimate the decadal averaged uncertainty to be 0.07 GtC yr−1 based on a factor proportional to the annual uncertainty prior and after 1980 ( GtC yr−1).
We assign a high confidence to the annual estimates of GATM because they are based on direct measurements from multiple and consistent instruments and stations distributed around the world (Ballantyne et al., 2012; Hall et al., 2021).
To estimate the total carbon accumulated in the atmosphere since 1750 or 1850, we use an atmospheric CO2 concentration of 277 ± 3 ppm or 286 ± 3 ppm, respectively, based on a cubic spline fit to ice core data (Joos and Spahni, 2008). For the construction of the cumulative budget shown in Fig. 3, we use the fitted estimates of CO2 concentration from Joos and Spahni (2008) to estimate the annual atmospheric growth rate using the conversion factors shown in Table 1. The uncertainty of ±3 ppm (converted to ±1σ) is taken directly from the IPCC's AR5 assessment (Ciais et al., 2013). Typical uncertainties in the growth rate in atmospheric CO2 concentration from ice core data are equivalent to ±0.1–0.15 GtC yr−1 as evaluated from the Law Dome data (Etheridge et al., 1996) for individual 20-year intervals over the period from 1850 to 1960 (Bruno and Joos, 1997).
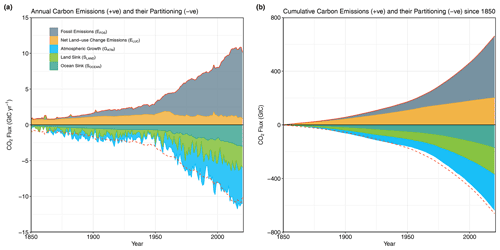
Figure 3Combined components of the global carbon budget illustrated in Fig. 2 as a function of time, for fossil CO2 emissions (EFOS, including a small sink from cement carbonation; grey) and emissions from land-use change (ELUC; brown), as well as their partitioning among the atmosphere (GATM; cyan), ocean (SOCEAN; blue), and land (SLAND; green). Panel (a) shows annual estimates of each flux and panel (b) the cumulative flux (the sum of all prior annual fluxes) since the year 1850. The partitioning is based on nearly independent estimates from observations (for GATM) and from process model ensembles constrained by data (for SOCEAN and SLAND) and does not exactly add up to the sum of the emissions, resulting in a budget imbalance (BIM) which is represented by the difference between the bottom red line (mirroring total emissions) and the sum of carbon fluxes in the ocean, land, and atmosphere reservoirs. All data are in GtC yr−1 (a) and GtC (b). The EFOS estimates are primarily from Gilfillan and Marland (2021), with uncertainty of about ±5 % (±1σ). The ELUC estimates are from three bookkeeping models (Table 4) with uncertainties of about ± 0.7 GtC yr−1. The GATM estimates prior to 1959 are from Joos and Spahni (2008) with uncertainties equivalent to about ±0.1–0.15 GtC yr−1 and from Dlugokencky and Tans (2022) since 1959 with uncertainties of about ±0.07 GtC yr−1 during 1959–1979 and ±0.02 GtC yr−1 since 1980. The SOCEAN estimate is the average from Khatiwala et al. (2013) and DeVries (2014) with uncertainty of about ±30 % prior to 1959, and the average of an ensemble of models and an ensemble of fCO2 data products (Table 4) with uncertainties of about ±0.4 GtC yr−1 since 1959. The SLAND estimate is the average of an ensemble of models (Table 4) with uncertainties of about ±1 GtC yr−1. See the text for more details of each component and their uncertainties.
Table 4References for the process models, fCO2-based ocean data products, and atmospheric inversions. All models and products are updated with new data to the end of year 2020, and the atmospheric forcing for the DGVMs has been updated as described in Sect. C2.2.
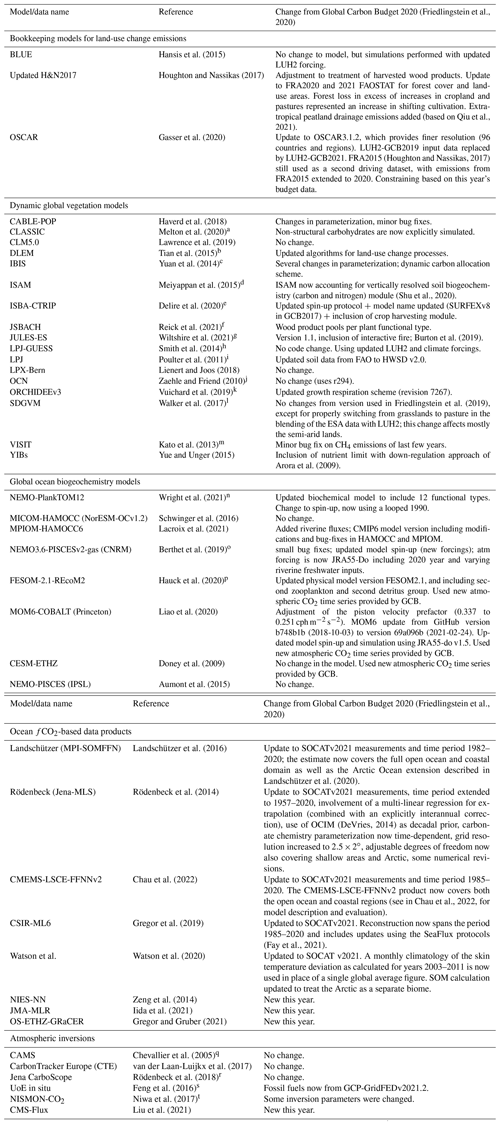
a See also Asaadi et al. (2018). b See also Tian et al. (2011). c The dynamic carbon allocation scheme was presented by Xia et al. (2015). d See also Jain et al. (2013). Soil biogeochemistry is updated based on Shu et al. (2020). e See also Decharme et al. (2019) and Seferian et al. (2019). f Mauritsen et al. (2019). g See also Sellar et al. (2019) and Burton et al. (2019). JULES-ES is the Earth System configuration of the Joint UK Land Environment Simulator as used in the UK Earth System Model (UKESM). h to account for the differences between the derivation of shortwave radiation from CRU cloudiness and DSWRF from CRUJRA, the photosynthesis scaling parameter αa was modified (−15 %) to yield similar results. i Compared to published version, decreased LPJ wood harvest efficiency so that 50 % of biomass was removed off-site compared to 85 % used in the 2012 budget. Residue management of managed grasslands increased so that 100 % of harvested grass enters the litter pool. j See also Zaehle et al. (2011). k See also Zaehle and Friend (2010) and Krinner et al. (2005). l See also Woodward and Lomas (2004). m See also Ito and Inatomi (2012). n See also Buitenhuis et al. (2013). o See also Séférian et al. (2019). p See also Schourup-Kristensen et al. (2014). q See also Remaud et al. (2018). r See also Rodenbeck et al. (2003). s See also Feng et al. (2009) and Palmer et al. (2019). t See also Niwa et al. (2020).
2.3.2 2021 projection
We provide an assessment of GATM for 2021 based on the monthly calculated global atmospheric CO2 concentration (GLO) through August (Dlugokencky and Tans, 2022), and bias-adjusted Holt–Winters exponential smoothing with additive seasonality (Chatfield, 1978) to project to January 2022. Additional analysis suggests that the first half of the year (the boreal winter–spring–summer transition) shows more interannual variability than the second half of the year (the boreal summer–autumn–winter transition), so that the exact projection method applied to the second half of the year has a relatively smaller impact on the projection of the full year. Uncertainty is estimated from past variability using the standard deviation of the last 5 years' monthly growth rates.
2.4 Ocean CO2 sink
The reported estimate of the global ocean anthropogenic CO2 sink SOCEAN is derived as the average of two estimates. The first estimate is derived as the mean over an ensemble of eight global ocean biogeochemistry models (GOBMs, Tables 4 and A2). The second estimate is obtained as the mean over an ensemble of seven observation-based data products (Tables 4 and A3). An eighth product (Watson et al., 2020) is shown but is not included in the ensemble average as it differs from the other products by adjusting the flux to a cool, salty ocean surface skin (see Appendix C3.1 for a discussion of the Watson product). The GOBMs simulate both the natural and anthropogenic CO2 cycles in the ocean. They constrain the anthropogenic air–sea CO2 flux (the dominant component of SOCEAN) by the transport of carbon into the ocean interior, which is also the controlling factor of present-day ocean carbon uptake in the real world. They cover the full globe and all seasons and were recently evaluated against surface ocean carbon observations, suggesting they are suitable to estimate the annual ocean carbon sink (Hauck et al., 2020). The data products are tightly linked to observations of fCO2 (fugacity of CO2, which equals pCO2 corrected for the non-ideal behaviour of the gas; Pfeil et al., 2013), which carry imprints of temporal and spatial variability but are also sensitive to uncertainties in gas-exchange parameterizations and data sparsity. Their asset is the assessment of interannual and spatial variability (Hauck et al., 2020). We further use two diagnostic ocean models to estimate SOCEAN over the industrial era (1781–1958).
The global fCO2-based flux estimates were adjusted to remove the pre-industrial ocean source of CO2 to the atmosphere of 0.61 GtC yr−1 from river input to the ocean (the average of 0.45 ± 0.18 GtC yr−1 by Jacobson et al., 2007, and 0.78 ± 0.41 GtC yr−1 by Resplandy et al., 2018), to satisfy our definition of SOCEAN (Hauck et al., 2020). The river flux adjustment was distributed over the latitudinal bands using the regional distribution of Aumont et al. (2001; north: 0.16 GtC yr−1, tropics: 0.15 GtC yr−1, south: 0.30 GtC yr−1), acknowledging that the boundaries of Aumont et al. (2001; namely 20∘ S and 20∘ N) are not consistent with the boundaries otherwise used in the GCB (30∘ S and 30∘ N). A recent modelling study (Lacroix et al., 2020) suggests that more of the riverine outgassing is located in the tropics than in the Southern Ocean, and hence this regional distribution is associated with a major uncertainty. Anthropogenic perturbations of river carbon and nutrient transport to the ocean are not considered (see Sect. 2.7).
We derive SOCEAN from GOBMs by using a simulation (sim A) with historical forcing of climate and atmospheric CO2, accounting for model biases and drift from a control simulation (sim B) with constant atmospheric CO2 and normal-year climate forcing. A third simulation (sim C) with historical atmospheric CO2 increase and normal-year climate forcing is used to attribute the ocean sink to CO2 (sim C minus sim B) and climate (sim A minus sim C) effects. Data products are adjusted to represent the full ocean area by a simple scaling approach when coverage is below 98 %. GOBMs and data products fall within the observational constraints over the 1990s (2.2 ± 0.7 GtC yr−1, Ciais et al., 2013) after applying adjustments.
We assign an uncertainty of ±0.4 GtC yr−1 to the ocean sink based on a combination of random (ensemble standard deviation) and systematic uncertainties (GOBMs bias in anthropogenic carbon accumulation, previously reported uncertainties in fCO2-based data products; see Sect. C3.3). We assess a medium confidence level to the annual ocean CO2 sink and its uncertainty because it is based on multiple lines of evidence, it is consistent with ocean interior carbon estimates (Gruber et al., 2019; see Sect. 3.5.5), and the results are consistent in that the interannual variability in the GOBMs and data-based estimates are all generally small compared to the variability in the growth rate of atmospheric CO2 concentration. We refrain from assigning a high confidence because of the systematic deviation between the GOBM and data product trends since around 2002. More details on the SOCEAN methodology can be found in Appendix C3.
The ocean CO2 sink forecast for the year 2021 is based on the annual historical and estimated 2021 atmospheric CO2 concentration (Dlugokencky and Tans, 2021), historical and estimated 2021 annual global fossil fuel emissions from this year's carbon budget, and the spring (March, April, May) oceanic Niño index (ONI) (NCEP, 2021). Using a non-linear regression approach, i.e. a feed-forward neural network, atmospheric CO2, the ONI, and the fossil fuel emissions are used as training data to best match the annual ocean CO2 sink (i.e. combined SOCEAN estimate from GOBMs and data products) from 1959 through 2020 from this year's carbon budget. Using this relationship, the 2021 SOCEAN can then be estimated from the projected 2021 input data using the non-linear relationship established during the network training. To avoid overfitting, the neural network was trained with a variable number of hidden neurons (varying between 2–5), and 20 % of the randomly selected training data were withheld for independent internal testing. Based on the best output performance (tested using the 20 % withheld input data), the best performing number of neurons was selected. In a second step, we trained the network 10 times using the best number of neurons identified in step 1 and different sets of randomly selected training data. The mean of the 10 training runs is considered our best forecast, whereas the standard deviation of the 10 ensembles provides a first-order estimate of the forecast uncertainty. This uncertainty is then combined with the SOCEAN uncertainty (0.4 GtC yr−1) to estimate the overall uncertainty of the 2021 prediction.
2.5 Terrestrial CO2 sink
The terrestrial land sink (SLAND) is thought to be due to the combined effects of fertilization by rising atmospheric CO2 and N inputs on plant growth, as well as the effects of climate change such as the lengthening of the growing season in northern temperate and boreal areas. SLAND does not include land sinks directly resulting from land use and land-use change (e.g. regrowth of vegetation) as these are part of the land-use flux (ELUC), although system boundaries make it difficult to exactly attribute CO2 fluxes on land between SLAND and ELUC (Erb et al., 2013).
SLAND is estimated from the multi-model mean of 17 DGVMs (Table A1). As described in Appendix C4, DGVM simulations include all climate variability and CO2 effects over land, with 12 DGVMs also including the effect of N inputs. The DGVM estimate of SLAND does not include the export of carbon to aquatic systems or its historical perturbation, which is discussed in Appendix D3. See Appendix C4 for DGVM evaluation and uncertainty assessment for SLAND, using the International Land Model Benchmarking system (ILAMB; Collier et al., 2018). More details on the SLAND methodology can be found in Appendix C4.
Like the ocean forecast, the land CO2 sink (SLAND) forecast is based on the annual historical and estimated 2021 atmospheric CO2 concentration (Dlugokencky and Tans 2021), historical and estimated 2021 annual global fossil fuel emissions from this year's carbon budget, and the summer (June, July, August) ONI (NCEP, 2021). All training data are again used to best match SLAND from 1959 through 2020 from this year's carbon budget using a feed-forward neural network. To avoid overfitting, the neural network was trained with a variable number of hidden neurons (varying between 2–15), larger than for SOCEAN prediction due to the stronger land carbon interannual variability. As done for SOCEAN, a pre-training step selects the optimal number of hidden neurons based on 20 % withheld input data, and in a second step, an ensemble of 10 forecasts is produced to provide the mean forecast plus uncertainty. This uncertainty is then combined with the SLAND uncertainty for 2020 (1.0 GtC yr−1) to estimate the overall uncertainty of the 2021 prediction.
2.6 The atmospheric perspective
The worldwide network of in situ atmospheric measurements and satellite-derived atmospheric CO2 column (xCO2) observations put a strong constraint on changes in the atmospheric abundance of CO2. This is true globally (hence our large confidence in GATM), but also regionally in regions with sufficient observational density found mostly in the extra-tropics. This allows atmospheric inversion methods to constrain the magnitude and location of the combined total surface CO2 fluxes from all sources, including fossil and land-use change emissions and land and ocean CO2 fluxes. The inversions assume EFOS to be well known, and they solve for the spatial and temporal distribution of land and ocean fluxes from the residual gradients of CO2 between stations that are not explained by fossil fuel emissions. By design, such systems close the carbon balance (BIM=0) and thus provide an additional perspective on the independent estimates of the ocean and land fluxes.
This year's release includes six inversion systems that are described in Table A4. Each system is rooted in Bayesian inversion principles but uses slightly different methodologies. These differences concern the selection of atmospheric CO2 data and the choice of a priori fluxes to refine with these data. They also differ in spatial and temporal resolution, assumed correlation structures, and mathematical approach of the models (see references in Table A4 for details). Importantly, the systems use a variety of transport models, which was demonstrated to be a driving factor behind differences in atmospheric inversion-based flux estimates, and specifically their distribution across latitudinal bands (Gaubert et al., 2019; Schuh et al., 2019). Multiple inversion systems (UoE, CTE, and CAMS) were previously tested with satellite xCO2 retrievals from GOSAT or OCO-2 measurements, but their results at the larger scales (as discussed in this work) did not deviate substantially from their in situ counterparts and are therefore not separately included. One inversion this year (CMS-Flux) used ACOS-GOSAT v9 retrievals between July 2009 and December 2014 and OCO-2 b10 retrievals between January to December 2015, in addition to the in situ observational CO2 mole fraction records.
The original products delivered by the inverse modellers were modified to facilitate the comparison to the other elements of the budget, specifically on three accounts: (1) global total fossil fuel emissions, (2) riverine CO2 transport, and (3) cement carbonation CO2 uptake. Details are given below. We note that with these adjustments the inverse results no longer represent the net atmosphere–surface exchange over land–ocean areas as sensed by atmospheric observations. Instead, for land, they become the net uptake of CO2 by vegetation and soils that is not exported by fluvial systems, similar to the DGVM estimates. For oceans, they become the net uptake of anthropogenic CO2, similar to the GOBMs estimates.
The inversion systems prescribe global fossil fuel emissions based on the GCP's Gridded Fossil Emissions Dataset version 2021.2 (GCP-GridFEDv2021.2; Jones et al., 2021b), which is an update to 2019 of the first version of GCP-GridFED presented by Jones et al. (2021a). GCP-GridFEDv2021.2 scales gridded estimates of CO2 emissions from EDGARv4.3.2 (Janssens-Maenhout et al., 2019) within national territories to match national emissions estimates provided by the GCB for the years 1959–2020, which were compiled following the methodology described in Sect. 2.1 with all datasets available on 14 August 2021 (Robbie Andrew, personal communication, 2021). Small differences between the systems due to for instance regridding to the transport model resolution are corrected for in the latitudinal partitioning we present, to ensure agreement with the estimate of EFOS in this budget. We also note that the ocean fluxes used as prior by five out of six inversions are part of the suite of the ocean process model or fCO2 data products listed in Sect. 2.4. Although these fluxes are further adjusted by the atmospheric inversions, it makes the inversion estimates of the ocean fluxes not completely independent of SOCEAN assessed here.
To facilitate comparisons to the independent SOCEAN and SLAND, we used the same corrections for transport and outgassing of carbon transported from land to ocean, as done for the observation-based estimates of SOCEAN (see Appendix C3). Furthermore, the inversions did not include a cement carbonation sink (see Sect. 2.1), and therefore this GCB component is implicitly part of their total land sink estimate. In the numbers presented in this budget, each year's global carbonation sink from cement was subtracted from each year's estimated land sink in each inversion, distributed proportionally to fossil fuel emissions per region (north, tropics, and south).
The atmospheric inversions are evaluated using vertical profiles of atmospheric CO2 concentrations (Fig. B4). More than 30 aircraft programmes over the globe, either regular programmes or repeated surveys over at least 9 months, have been used to assess model performance (with space–time observational coverage sparse in the SH and tropics, and denser in NH mid-latitudes; Table A6). The six models are compared to the independent aircraft CO2 measurements between 2 and 7 km above sea level between 2001 and 2020. Results are shown in Fig. B4 and discussed in Sect. 3.7.
With a relatively small ensemble (N=6) of systems that moreover share some a priori fluxes used with one another, or with the process-based models, it is difficult to justify using their mean and standard deviation as a metric for uncertainty across the ensemble. We therefore report their full range (min–max) without their mean. More details on the atmospheric inversions methodology can be found in Appendix C5.
2.7 Processes not included in the global carbon budget
The contribution of anthropogenic CO and CH4 to the global carbon budget is not fully accounted for in Eq. (1) and is described in Appendix D1. The contributions of other carbonates to CO2 emissions is described in Appendix D2. The contribution of anthropogenic changes in river fluxes is conceptually included in Eq. (1) in SOCEAN and in SLAND, but it is not represented in the process models used to quantify these fluxes. This effect is discussed in Appendix D3. Similarly, the loss of additional sink capacity from reduced forest cover is missing in the combination of approaches used here to estimate both land fluxes (ELUC and SLAND) and its potential effect is discussed and quantified in Appendix D4.
For each component of the global carbon budget, we present results for three different time periods: the full historical period, from 1850 to 2020; the six decades in which we have atmospheric concentration records from Mauna Loa (1960–2020), with a specific focus on last year (2020); and the projection for the current year (2021). Subsequently, we assess the combined constraints from the budget components (often referred to as a bottom-up budget) against the top-down constraints from inverse modelling of atmospheric observations. We do this for the global balance of the last decade, as well as for a regional breakdown of land and ocean sinks by broad latitude bands.
3.1 Fossil CO2 emissions
3.1.1 Historical period 1850–2020
Cumulative fossil CO2 emissions for 1850–2020 were 455 ± 25 GtC, including the cement carbonation sink (Fig. 3, Table 8).
In this period, 46 % of fossil CO2 emissions came from coal, 35 % from oil, 14 % from natural gas, 3 % from decomposition of carbonates, and 1 % from flaring.
In 1850, the UK accounted for 62 % of global fossil CO2 emissions. In 1891 the combined cumulative emissions of the current members of the European Union reached and subsequently surpassed the level of the UK. Since 1917 US cumulative emissions have been the largest. Over the entire period 1850–2020, US cumulative emissions amounted to 110 GtC (25 % of world total), the EU's to 80 GtC (18 %), and China's to 60 GtC (14 %).
There are three additional global datasets that include all sources of fossil CO2 emissions: CDIAC-FF (Gilfillan and Marland, 2021), CEDS version v_2021_04_21 (Hoesly et al., 2018; O'Rourke et al., 2021), and PRIMAP-hist version 2.3.1 (Gütschow et al., 2016, 2021), although these datasets are not independent. CDIAC-FF has the lowest cumulative emissions over 1750–2018 at 437 GtC, GCP has 443 GtC, CEDS 445 GtC, PRIMAP-hist TP 453 GtC, and PRIMAP-hist CR 455 GtC. CDIAC-FF excludes emissions from lime production, while neither CDIAC-FF nor GCP explicitly include emissions from international bunker fuels prior to 1950. CEDS has higher emissions from international shipping in recent years, while PRIMAP-hist has higher fugitive emissions than the other datasets. However, in general these four datasets are in relative agreement with total historical global emissions of fossil CO2.
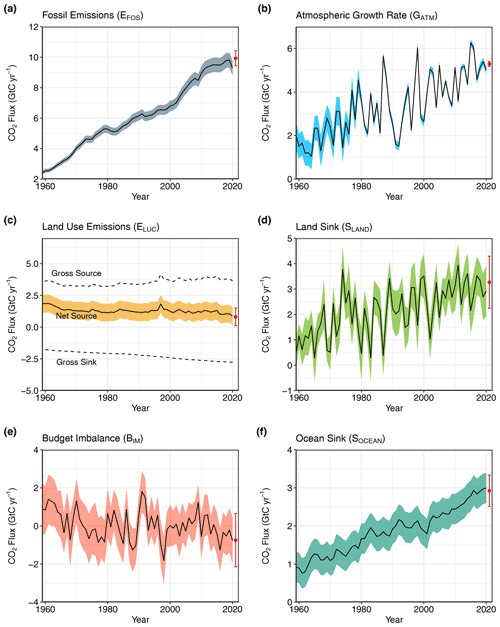
Figure 4Components of the global carbon budget and their uncertainties as a function of time, presented individually for (a) fossil CO2 emissions (EFOS), (b) growth rate in atmospheric CO2 concentration (GATM), (c) emissions from land-use change (ELUC), (d) the land CO2 sink (SLAND), (e) the ocean CO2 sink (SOCEAN), and (f) the budget imbalance that is not accounted for by the other terms. Positive values of SLAND and SOCEAN represent a flux from the atmosphere to land or the ocean. All data are in GtC yr−1 with the uncertainty bounds representing ±1 standard deviation in shaded colour. Data sources are as in Fig. 3. The red dots indicate our projections for the year 2021 and the red error bars the uncertainty in the projections (see methods).
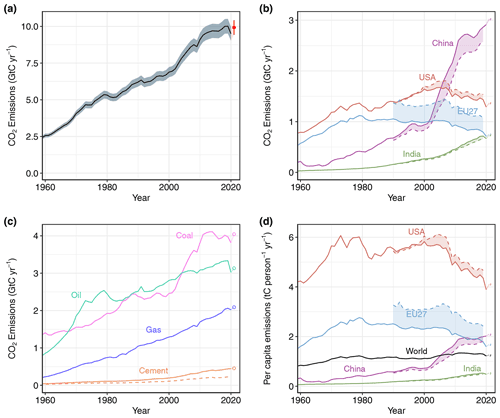
Figure 5Fossil CO2 emissions for (a) the globe, including an uncertainty of ±5 % (grey shading) and a projection through the year 2021 (red dot and uncertainty range); (b) territorial (solid lines) and consumption (dashed lines) emissions for the top three country emitters (USA, China, India) and for the European Union (EU27); (c) global emissions by fuel type, including coal, oil, gas, and cement, and cement minus cement carbonation (dashed); and (d) per capita emissions the world and for the large emitters as in panel (b). Territorial emissions are primarily from Gilfillan and Marland (2021) except national data for the USA and EU27 for 1990–2018, which are reported by the countries to the UNFCCC as detailed in the text; consumption-based emissions are updated from Peters et al. (2011b). See Sect. 2.1 and Appendix C1 for details of the calculations and data sources.
3.1.2 Recent period 1960–2020
Global fossil CO2 emissions, EFOS (including the cement carbonation sink), have increased every decade from an average of 3.0 ± 0.2 GtC yr−1 for the decade of the 1960s to an average of 9.5 ± 0.5 GtC yr−1 during 2011–2020 (Table 6, Figs. 2, 4 and 5). The growth rate in these emissions decreased between the 1960s and the 1990s, from 4.3 % yr−1 in the 1960s (1960–1969), 3.2 % yr−1 in the 1970s (1970–1979), and 1.6 % yr−1 in the 1980s (1980–1989), to 0.9 % yr−1 in the 1990s (1990–1999). After this period, the growth rate began increasing again in the 2000s at an average growth rate of 3.0 % yr−1, decreasing to 0.6 % yr−1 for the last decade (2011–2020). China's emissions increased by +1.0 % yr−1 on average over the last 10 years, dominating the global trend, followed by India's emissions increase by +3.9 % yr−1, while emissions decreased in EU27 by −1.9 % yr−1, and in the USA by −1.1 % yr−1. Figure 6 illustrates the spatial distribution of fossil fuel emissions for the 2011–2020 period.
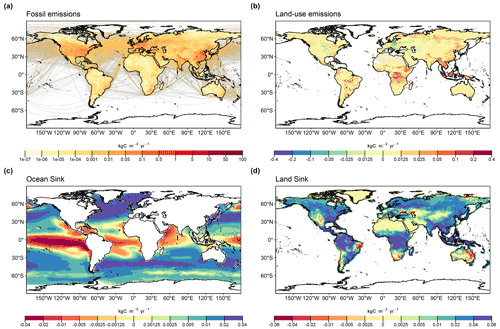
Figure 6The 2011–2020 decadal mean components of the global carbon budget, presented for (a) fossil CO2 emissions (EFOS), (b) land-use change emissions (ELUC), (c) the ocean CO2 sink (SOCEAN), and (d) the land CO2 sink (SLAND). Positive values for EFOS and ELUC represent a flux to the atmosphere, whereas positive values of SOCEAN and SLAND represent a flux from the atmosphere to the ocean or the land. In all panels, yellow/red (green/blue) colours represent a flux from (into) the land–ocean to (from) the atmosphere. All units are in kgC m−2 yr−1. Note the different scales in each panel. EFOS data shown are from GCP-GridFEDv2021.2. ELUC data shown are only from BLUE as the updated H&N2017 and OSCAR do not resolve gridded fluxes. SOCEAN data shown are the average of GOBMs and data product means, using GOBMs simulation A; no adjustment for bias and drift is applied to the gridded fields (see Sect. 2.4). SLAND data shown are the average of DGVMs for simulation S2 (see Sect. 2.5).
EFOS includes the uptake of CO2 by cement via carbonation which has increased with increasing stocks of cement products, from an average of 20 MtC yr−1 (0.02 GtC yr−1) in the 1960s to an average of 200 MtC yr−1 (0.2 GtC yr−1) during 2011–2020 (Fig. 5).
3.1.3 Final year 2020
Global fossil CO2 emissions were 5.4 % lower in 2020 than in 2019, because of the COVID-19 pandemic, with a decline of 0.5 GtC to reach 9.5 ± 0.5 GtC (9.3 ± 0.5 GtC when including the cement carbonation sink) in 2020 (Fig. 5), distributed among coal (40 %), oil (32 %), natural gas (21 %), cement (5 %), and others (2 %). Compared to the previous year, 2020 emissions from coal, oil, and gas declined by 4.4 %, 9.7 %, and 2.3 % respectively, while emissions from cement increased by 0.8 %. All growth rates presented are adjusted for the leap year, unless stated otherwise.
In 2020, the largest absolute contributions to global fossil CO2 emissions were from China (31 %), the USA (14 %), the EU27 (7 %), and India (7 %). These four regions account for 59 % of global CO2 emissions, while the rest of the world contributed 41 %, including international aviation and marine bunker fuels (2.9 % of the total). Growth rates for these countries from 2019 to 2020 were +1.4 % (China), −10.6 % (USA), −10.9 % (EU27), and −7.3 % (India), with −7.0 % for the rest of the world. The per capita fossil CO2 emissions in 2020 were 1.2 tC person−1 yr−1 for the globe, and were 3.9 (USA), 2.0 (China), 1.6 (EU27) and 0.5 (India) tC per person per year for the four highest emitting countries (Fig. 5).
The COVID-19-induced decline in emissions of −5.4 % in 2020 is close to the projected decline of −6.7 %, which was the median of four approaches, published in Friedlingstein et al. (2020) (Table 7). Of the four approaches, the “GCP” method was closest at −5.8 %. That method was based on national emissions projections for China, the USA, the EU27, and India using reported monthly activity data when available and projections of gross domestic product corrected for trends in fossil fuel intensity (IFOS) for the rest of the world. Of the regions, the projection for the EU27 was the least accurate, and the reasons for this are discussed by Andrew (2021).
Table 5Comparison of results from the bookkeeping method and budget residuals with results from the DGVMs and inverse estimates for different periods, the last decade, and the last year available. All values are in GtC yr−1. The DGVM uncertainties represent ±1σ of the decadal or annual (for 2020 only) estimates from the individual DGVMs: for the inverse models the range of available results is given. All values are rounded to the nearest 0.1 GtC and therefore columns do not necessarily add to zero.
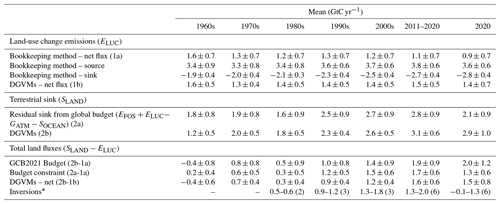
* Estimates are adjusted for the pre-industrial influence of river fluxes, for the cement carbonation sink, and adjusted to common EFOS (Sect. 2.6). The ranges given include varying numbers (in parentheses) of inversions in each decade (Table A4).
3.1.4 Year 2021 projection
Globally, we estimate that global fossil CO2 emissions will rebound 4.8 % in 2021 (4.2 % to 5.4 %) to 9.9 GtC (36.4 GtCO2), returning to near their 2019 emission levels of 10.0 GtC (36.7 GtCO2). Global increases in 2021 emissions per fuel types are +6.3 % (range 5.5 % to 7.0 %) for coal, +4.0 % (range 2.6 % to 5.4 %) for oil, +3.8 % (range 2.8 % to 4.8 %) for natural gas, and +3.2 % (range 1.7 % to 4.6 %) for cement.
For China, projected fossil emissions in 2021 are expected to increase by 4.3 % (range 3.0 % to 5.4 %) compared with 2020 emissions, bringing 2021 emissions for China to around 3.0 GtC yr−1 (11.1 GtCO2 yr−1). Chinese emissions appear to have risen in both 2020 and 2021 despite the economic disruptions of COVID-19. Increases in fuel-specific projections for China are +4.1 % for coal, +4.4 % for oil, +12.8 % natural gas, and a decrease of 0.1 % for cement.
For the USA, the Energy Information Administration (EIA) emissions projection for 2021 combined with cement clinker data from USGS gives an increase of 6.8 % (range 6.6 % to 7.0 %) compared to 2020, bringing USA 2021 emissions to around 1.4 GtC yr−1 (5.0 GtCO2 yr−1). This is based on separate projections for coal of +17.1 %, oil +9.0 %, natural gas −0.8 %, and cement +0.3 %.
For the European Union, our projection for 2021 is for an increase of 6.3 % (range 4.3 % to 8.3 %) over 2020, with 2021 emissions around 0.8 GtC yr−1 (2.8 GtCO2 yr−1). This is based on separate projections for coal of +14.6 %, oil +3.7 %, natural gas +4.6 %, and cement +0.3 %.
For India, our projection for 2021 is an increase of 11.2 % (range of 10.7 % to 11.7 %) over 2020, with 2021 emissions around 0.7 GtC yr−1 (2.7 GtCO2 yr−1). This is based on separate projections for coal of +13.9 %, oil +3.4 %, natural gas +4.8 %, and cement +21.6 %.
For the rest of the world, the expected growth rate for 2021 is 3.2 % (range 2.0 % to 4.3 %). This is computed using the GDP projection for the world (excluding China, the USA, the EU, and India) of 4.4 % made by the IMF (2022) and a decrease in IFOS of −1.7 % yr−1, which is the average over 2011–2020. The uncertainty range is based on the standard deviation of the interannual variability in IFOS during 2011–2020 of 0.6 % yr−1 and our estimates of uncertainty in the IMF's GDP forecast of 0.6 %. The methodology allows independent projections for coal, oil, natural gas, cement, and other components, which add to the total emissions in the rest of the world. The fuel-specific projected 2021 growth rates for the rest of the world are: +3.2 % (range 0.7 % to 5.8 %) for coal, +2.3 % (−0.3 % to +4.9 %) for oil, +4.1 % (2.6 % to 5.7 %) for natural gas, and +4.8 % (+2.7 % to +6.9 %) for cement.
Independently, the IEA has published two forecasts of global fossil energy CO2 emissions (i.e. a subset of fossil CO2 emissions), the first in April (4.8 %; IEA, 2021a) which was then revised in October to 4 % (IEA, 2021b). In March 2022 they also published a new, preliminary estimate of 6 % growth (IEA, 2021a). Carbon Monitor produces estimates of global emissions with low temporal lag, and their estimates suggest that emissions were 5.1 % higher than in 2020 (Carbon Monitor, 2022).
Table 6Decadal mean in the five components of the anthropogenic CO2 budget for different periods, and last year available. All values are in GtC yr−1, and uncertainties are reported as ±1σ. Fossil CO2 emissions include cement carbonation. The table also shows the budget imbalance (BIM), which provides a measure of the discrepancies among the nearly independent estimates and has an uncertainty exceeding ±1 GtC yr−1. A positive imbalance means the emissions are overestimated and/or the sinks are too small. All values are rounded to the nearest 0.1 GtC and therefore columns do not necessarily add to zero.
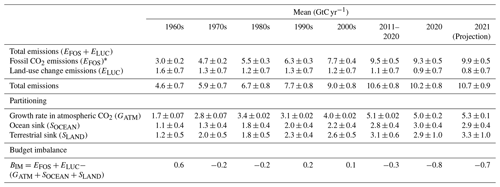
* Fossil emissions excluding the cement carbonation sink amount to 3.1 ± 0.2 GtC yr−1, 4.7 ± 0.2 GtC yr−1, 5.5 ± 0.3 GtC yr−1, 6.4 ± 0.3 GtC yr−1, 7.9 ± 0.4 GtC yr−1, and 9.7 ± 0.5 GtC yr−1 for the decades 1960s to 2010s respectively and to 9.5 ± 0.5 GtC yr−1 for 2020.
3.2 Emissions from land-use changes
3.2.1 Historical period 1850–2020
Cumulative CO2 emissions from land-use changes (ELUC) for 1850–2020 were 200 ± 65 GtC (Table 8; Figs. 3, 13). The cumulative emissions from ELUC are particularly uncertain, with large spread among individual estimates of 140 GtC (updated H&N2017), 270 GtC (BLUE), and 195 GtC (OSCAR) for the three bookkeeping models and a similar wide estimate of 190 ± 60 GtC for the DGVMs (all cumulative numbers are rounded to the nearest 5 GtC). These estimates are broadly consistent with indirect constraints from vegetation biomass observations, giving a cumulative source of 155 ± 50 GtC over the 1901–2012 period (Li et al., 2017). However, given the large spread, a best estimate is difficult to ascertain.
3.2.2 Recent period 1960–2020
In contrast to growing fossil emissions, CO2 emissions from land use, land-use change, and forestry have remained relatively constant, at around 1.3 ± 0.7 GtC yr−1 over the 1970–1999 period, and even show a slight decrease over the last 20 years, reaching 1.1 ± 0.7 GtC yr−1 for the 2011–2020 period (Table 6, Fig. 4), but with large spread across estimates (Table 5, Fig. 7). Emissions have been relatively constant in the DGVMs ensemble of models since the 1970s, with similar mean values until the 1990s as the bookkeeping mean and large model spread (Table 5, Fig. 7). The DGVMs average grows larger than the bookkeeping average in the recent decades and shows no sign of decreasing emissions, which is, however, expected as DGVM-based estimates include the loss of additional sink capacity, which grows with time, while the bookkeeping estimates do not (Appendix D4).
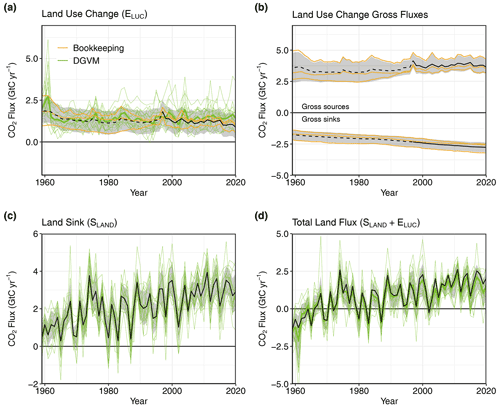
Figure 7CO2 exchanges between the atmosphere and the terrestrial biosphere as used in the global carbon budget (black with ±1σ uncertainty in grey shading in all panels). (a) CO2 emissions from land-use change (ELUC) with estimates from the three bookkeeping models (yellow lines) and DGVMs (green) shown individually, with DGVM ensemble means (dark green). The dashed line identifies the pre-satellite period before the inclusion of peatland burning. (b) CO2 gross sinks (positive, from regrowth after agricultural abandonment and wood harvesting) and gross sources (negative, from decaying material left dead on site, products after clearing of natural vegetation for agricultural purposes, wood harvesting, and for BLUE, degradation from primary to secondary land through usage of natural vegetation as rangeland, and also from emissions from peat drainage and peat burning) from the three bookkeeping models (yellow lines). The sum of the gross sinks and sources is ELUC shown in panel (a). (c) Land CO2 sink (SLAND) with individual DGVM estimates (green). (d) Total atmosphere–land CO2 fluxes (SLAND − ELUC), with individual DGVMs (green) and their multi-model mean (dark green).
ELUC is a net term of various gross fluxes, which comprise emissions and removals. Gross emissions are on average 2–4 times larger than the net ELUC emissions, and remained largely constant over the last 60 years, with a moderate increase from an average of 3.4 ± 0.9 GtC yr−1 for the decade of the 1960s to an average of 3.8 ± 0.6 GtC yr−1 during 2011–2020 (Fig. 7, Table 5), showing the relevance of land management such as harvesting or rotational agriculture. Increases in gross removals, from 1.9 ± 0.4 GtC yr−1 for the 1960s to 2.7 ± 0.4 GtC yr−1 for 2011–2020, were larger than the increase in gross emissions. Since the processes behind gross removals, foremost forest regrowth and soil recovery, are all slow, while gross emissions include a large instantaneous component, short-term changes in land-use dynamics, such as a temporary decrease in deforestation, influence gross emissions dynamics more than gross removal dynamics. It is these relative changes to each other that explain the decrease in net ELUC emissions over the last two decades and the last few years. Gross fluxes differ more across the three bookkeeping estimates than net fluxes, which is expected due to different process representation; in particular, treatment of shifting cultivation, which increases both gross emissions and removals, differs across models.
There is a decrease in net CO2 emissions from land-use change over the last decade (Fig. 7, Table 6), in contrast to earlier estimates of no clear trend across ELUC estimates (Friedlingstein et al., 2020; Hong et al., 2021). The trend in the last decade is now about −4 % yr−1, compared to the +1.8 % yr−1 reported by Friedlingstein et al. (2020). This decrease is principally attributable to changes in ELUC estimates from BLUE and OSCAR, which relate to changes in the underlying land-use forcing, LUH2 (Chini et al., 2021; Hurtt et al., 2020), based on HYDE3.3 (Klein Goldewijk et al., 2017a, b): HYDE3.3 now incorporates updated estimates of agricultural areas by the FAO and uses multi-annual land-cover maps from satellite remote sensing (ESA CCI Land Cover) to constrain contemporary land-cover patterns (see Appendix C2.2 for details). These changes lead to lower global ELUC estimates in the last two decades compared to earlier versions of the global carbon budget due most notably to lower emissions from cropland expansion, particularly in the tropical regions. Rosan et al. (2021) showed that for Brazil, the new HYDE3.3 version is closer to independent, regional estimates of land-use and land-cover change (MapBiomas, 2021) with respect to spatial patterns, but it shows less land-use and land-cover changes than these independent estimates, while HYDE3.2-based estimates had shown higher changes and lower emissions. The update in land-use forcing leads to a decrease in estimated emissions in Brazil across several models after the documented deforestation peak of 2003–2004 that preceded policies and monitoring systems decreasing deforestation rates (Rosan et al., 2021). However, estimated emissions based on the new land-use forcing do not reflect the rise in Brazilian deforestation in the last few years (Silva Junior et al., 2021), and associated increasing emissions from deforestation would have been missed here. The update in FAO agricultural areas in Brazil also implied that substantial interannual variability reported to earlier FAO assessment and captured by the HYDE3.2 version since 2000 was removed. Due to the asymmetry of (fast) decay (like clearing by fire) and (slower) regrowth, such reduced variability is expected to decrease annual emissions. Also, the approach by Houghton and Nassikas (2017) smooths land-use area changes before calculating carbon fluxes by a 5-year running mean, hence the three emission estimates are in better agreement than in previous GCB estimates. However, differences still exist, which highlight the need for accurate knowledge of land-use transitions and their spatial and temporal variability. A further caveat is that global land-use change data for model input does not capture forest degradation, which often occurs on small scales or without forest cover changes easily detectable from remote sensing and poses a growing threat to forest area and carbon stocks that may surpass deforestation effects (e.g. Matricardi et al., 2020; Qin et al., 2021).
Overall, therefore, we assign low confidence to the change towards a decreasing trend of land-use emissions over the last two decades as seen compared to the estimate of the global carbon budget 2020 (Friedlingstein et al., 2020). Our approach aims at using the most up-to-date data and methods, such as accounting for revisions of living databases of country-level agricultural statistics from FAO or including satellite remote-sensing information for spatial allocation. While we start from a well-documented methodology to provide gridded land-use data (Chini et al., 2021), not all changes in individual components are always documented, complicating the explanation of changes from one GCB to the next. The rising number of pan-tropical or global estimates of carbon stock changes based on satellite remote sensing of carbon densities and forest cover changes (Fan et al., 2019; Qin et al., 2021; Xu et al., 2022; Feng et al., 2022) may seem a promising path for independent evaluation of the land-use emissions term. However, comparison of satellite-derived fluxes to global model estimates is hampered for several reasons discussed by Pongratz et al. (2021). Most importantly, satellite-based estimates usually do not distinguish between anthropogenic drivers and natural forest cover losses (e.g. from drought or natural wildfires), which have also increased over time in some regions, including the tropics; ancillary information would be needed to attribute the observed signal of vegetation or carbon stock change to different drivers. Further, satellite-based estimates often only provide sub-component fluxes of ELUC, excluding soil or product pool changes. Since forest cover loss is better detectable from space than regrowth, satellite-based products often limit their estimates to emissions from forest loss, neglecting carbon uptake from regrowth of forests, as may occur following wood harvesting, abandonment, or natural disturbances; such products thus provide a subset of the gross emissions term (Fig. 7b) and cannot be compared to net emissions. Lastly, satellite-based fluxes typically quantify committed instead of actual emissions, i.e. legacy CO2 fluxes from potentially slow processes such as slash, soil carbon or product decay, or forest regrowth are not captured at the time they actually occur but are attributed to the time of the land-use change event (Pongratz et al., 2021). Using data on drivers of forest cover loss to isolate fluxes from agricultural expansion, and looking into gross emissions instead of the net land-use change flux, Feng et al. (2022) suggest a stronger increase in global gross emissions (though generally a smaller flux) than the bookkeeping models do (see gross fluxes in Fig. 7b). This is in line with Rosan et al. (2021) suggesting that the trend of net emissions in Brazil may be underestimated by the updated land-use data (though patterns have improved). Further studies are needed to robustly estimate the trend of global net land-use emissions. Progress is also needed on accurate quantifications of land-use dynamics, including less well observable management types such as shifting cultivation and wood harvesting, and their distinction from natural disturbances (Pongratz et al., 2021).
The highest land-use emissions occur in the tropical regions of all three continents, including the Arc of Deforestation in the Amazon basin (Fig. 6b). This is related to massive expansion of cropland, particularly in the last few decades in Latin America, Southeast Asia, and sub-Saharan Africa (Hong et al., 2021), to a substantial extent for export (Pendrill et al., 2019). Emission intensity is high in many tropical countries, particularly of Southeast Asia, due to high rates of land conversion in regions of carbon-dense and often still pristine, undegraded natural forests (Hong et al., 2021). Emissions are further increased by peat fires in equatorial Asia (GFED4s, van der Werf et al., 2017). Uptake due to land-use change occurs, particularly in Europe, partly related to expanding forest area as a consequence of the forest transition in the 19th and 20th century and subsequent regrowth of forest (Fig. 6b) (Mather, 2001; McGrath et al., 2015).
While the mentioned patterns are robust and supported by independent literature, we acknowledge that model spread is substantially larger on regional than on global level, as has been shown for bookkeeping models (Bastos et al., 2021) as well as DGVMs (Obermeier et al., 2021). A detailed analysis of country-level or regional uncertainties globally is beyond the scope of this study. Assessments for individual regions will be performed as part of REgional Carbon Cycle Assessment and Processes (RECCAP2; Ciais et al., 2022) or already exist for selected regions (e.g. for Europe Petrescu et al., 2020, for Brazil Rosan et al., 2021).
National GHG inventory data (NGHGI) under the LULUCF sector or data submitted by countries to FAOSTAT differ from the global models' definition of ELUC we adopt here in that in the NGHGI reporting, the natural fluxes (SLAND) are counted towards ELUC when they occur on managed land (Grassi et al., 2018). In order to compare our results to the NGHGI approach, we perform a re-mapping of our ELUC estimate by including the SLAND over managed forest from the DGVM simulations (following Grassi et al., 2021) to the bookkeeping ELUC estimate (see Appendix C2.3). For the 2011–2020 period, we estimate that 1.5 GtC yr−1 of SLAND occurred on managed forests and is then reallocated to ELUC here, as done in the NGHGI method. Doing so, our mean estimate of ELUC is reduced from a source of 1.1 GtC to a sink of −0.4 GtC, very similar to the NGHGI estimate of −0.6 GtC (Table A8).
Though estimates between GHGI, FAOSTAT, individual process-based models, and the mapped budget estimates still differ in value and need further analysis, the approach taken here provides a possibility to relate the global models' and NGHGI approach to each other routinely and thus link the anthropogenic carbon budget estimates of land CO2 fluxes directly to the Global Stocktake, as part of the UNFCCC Paris Agreement.
3.2.3 Final year 2020
The global CO2 emissions from land-use change are estimated as 0.9 ± 0.7 GtC in 2020, 0.2 GtC lower than 2019, which had featured particularly large peat and tropical deforestation and degradation fires. The surge in deforestation fires in the Amazon, causing about 30 % higher emissions from deforestation and degradation fires in 2019 over the previous decade, continued into 2020 (GFED4.1s, van der Werf et al., 2017). However, the unusually dry conditions for a non-El Niño year that occurred in Indonesia in 2019 and led to fire emissions from peat burning, deforestation, and degradation in equatorial Asia to be about twice as large as the average over the previous decade (GFED4.1s, van der Werf et al., 2017) ceased in 2020. However, confidence in the annual change remains low. While the mentioned fires are clearly attributable to land-use activity, foremost deforestation and peat burning, and may have been reinforced by dry weather conditions, as was the case in Indonesia in 2019, wildfires also occur naturally. In particular, the extreme fire events in recent years in Australia, Siberia, and California were unrelated to land-use change and are thus not attributed to ELUC, but to the natural land sink, and are discussed in Sect. 3.6.2.
Land-use change and related emissions may have been affected by the COVID-19 pandemic (e.g. Poulter et al., 2021). Although emissions from tropical deforestation and degradation fires have been decreasing from 2019 to 2020 on the global scale, they increased in Latin America (GFED4s; van der Werf et al., 2017). During the period of the pandemic, environmental protection policies and their implementation may have been weakened in Brazil (Vale et al., 2021). In other countries, too, monitoring capacities and legal enforcement of measures to reduce tropical deforestation have been reduced due to budget restrictions of environmental agencies or impairments to ground-based monitoring that prevents land grabs and tenure conflicts (Brancalion et al., 2020; Amador-Jiménez et al., 2020). Effects of the pandemic on trends in fire activity or forest cover changes are hard to separate from those of general political developments and environmental changes, and the long-term consequences of disruptions in agricultural and forestry economic activities (e.g. Gruère and Brooks, 2020; Golar et al., 2020; Beckman and Countryman, 2021) remain to be seen.
3.2.4 Year 2021 projection
With wet conditions in Indonesia and a below-average fire season in South America our preliminary estimate of ELUC for 2021 is substantially lower than the 2011–2020 average. By the end of September 2021 emissions from tropical deforestation and degradation fires were estimated to be 222 TgC, down from 347 TgC in 2019 and 288 in 2020 (315 TgC 1997–2020 average). Peat fire emissions in equatorial Asia were estimated to be 1 TgC, down from 117 TgC in 2019 and 2 TgC in 2020 (74 TgC 1997–2020 average) (GFED4.1s, van der Werf et al., 2017). Based on the fire emissions until the end of September, we expect ELUC emissions of around 0.8 GtC in 2021. Note that although our extrapolation is based on tropical deforestation and degradation fires, degradation attributable to selective logging, edge effects, or fragmentation will not be captured.
3.3 Total anthropogenic emissions
Cumulative anthropogenic CO2 emissions for 1850–2020 totalled 660 ± 65 GtC (2420 ± 240 GtCO2), of which almost 70 % (455 GtC) occurred since 1960 and more than 30 % (205 GtC) since 2000 (Tables 6 and 8). Total anthropogenic emissions more than doubled over the last 60 years, from 4.6 ± 0.7 GtC yr−1 for the decade of the 1960s to an average of 10.6 ± 0.8 GtC yr−1 during 2011–2020.
The total anthropogenic CO2 emissions from fossil plus land-use change amounted to 10.6 ± 0.8 GtC (38.9 ± 2.9 GtCO2) for the 2011–2020 decade, reaching 10.2 ± 0.8 GtC (37.2 ± 2.9 GtCO2) in 2020, while for 2021, we project global total anthropogenic CO2 emissions from fossil and land-use changes to be around 10.7 GtC (39.3 GtCO2).
During the historical period 1850–2020, 30 % of historical emissions were from land-use change and 70 % from fossil emissions. However, fossil emissions have grown significantly since 1960 while land-use changes have not, and consequently the contributions of land-use change to total anthropogenic emissions were smaller during recent periods (17 % during the period 1960–2020 and 10 % during 2011–2020).
Table 7Comparison of the projection with realized fossil CO2 emissions (EFOS). The “Actual” values are first the estimate available using actual data, and the “Projected” values refer to estimates made before the end of the year for each publication. Projections based on a different method from that described here during 2008–2014 are available in Le Quéré et al. (2016). All values are adjusted for leap years.
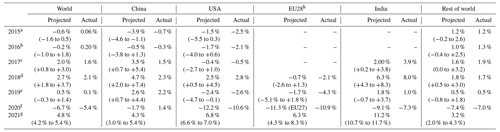
a Jackson et al. (2016) and Le Quéré et al. (2015a). b Le Quéré et al. (2016). c Le Quéré et al. (2018a). d Le Quéré et al. (2018b). e Friedlingstein et al. (2019), f Friedlingstein et al. (2020), g This study (median of four reported estimates, Sect. 3.1.4). h EU28 until 2019, EU27 from 2020.
Table 8Cumulative CO2 for different time periods in gigatonnes of carbon (GtC). All uncertainties are reported as ±1σ. Fossil CO2 emissions include cement carbonation. The budget imbalance (BIM) provides a measure of the discrepancies among the nearly independent estimates. All values are rounded to the nearest 5 GtC and therefore columns do not necessarily add to zero.
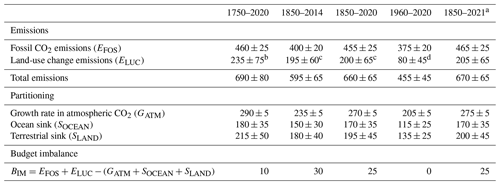
a Using projections for the year 2021. b Cumulative ELUC 1750–1849 of 30 GtC based on multi-model mean of Pongratz et al. (2009), Shevliakova et al. (2009), Zaehle et al. (2011), and Van Minnen et al. (2009). 1850–2020 from the mean of BLUE (Hansis et al., 2015), OSCAR (Gasser et al., 2020), and H&N2017 (Houghton and Nassikas, 2017). 1750–2020 uncertainty is estimated from standard deviation of DGVMs over 1870–2020 scaled by 1750–2020 emissions. c Cumulative ELUC based on BLUE, OSCAR, and H&N2017. Uncertainty is estimated from the standard deviation of DGVM estimates. d Cumulative ELUC based on BLUE, OSCAR, and H&N2017. Uncertainty is formed from the uncertainty in annual ELUC over 1959–2020, which is 0.7 GtC yr−1 multiplied by length of the time series.
3.4 Atmospheric CO2
3.4.1 Historical period 1850–2020
Atmospheric CO2 concentration was approximately 277 parts per million (ppm) in 1750 (Joos and Spahni, 2008), reaching 300 ppm in the 1910s, 350 ppm in the late 1980s, and 412.44 ± 0.1 ppm in 2020 (Dlugokencky and Tans, 2022; Fig. 1). The mass of carbon in the atmosphere increased by 48 % from 590 GtC in 1750 to 876 GtC in 2020. Current CO2 concentrations in the atmosphere are unprecedented in the last 2 million years, and the current rate of atmospheric CO2 increase is at least 10 times faster than at any other time during the last 800 000 years (Canadell et al., 2022).
3.4.2 Recent period 1960–2020
The growth rate in atmospheric CO2 level increased from 1.7 ± 0.07 GtC yr−1 in the 1960s to 5.1 ± 0.02 GtC yr−1 during 2011–2020 with important decadal variations (Table 6, Figs. 3 and 4).
During the last decade (2011–2020), the growth rate in atmospheric CO2 concentration continued to increase, albeit with large interannual variability (Fig. 4).
The airborne fraction (AF), defined as the ratio of atmospheric CO2 growth rate to total anthropogenic emissions,
provides a diagnostic of the relative strength of the land and ocean carbon sinks in removing part of the anthropogenic CO2 perturbation. The evolution of AF over the last 60 years shows no significant trend, remaining at around 45 %, albeit showing a large interannual variability driven by the year-to-year variability in GATM (Fig. 8). The observed stability of the airborne fraction over the 1960–2020 period indicates that the ocean and land CO2 sinks have been removing on average about 55 % of the anthropogenic emissions (see Sect. 3.5 and 3.6).
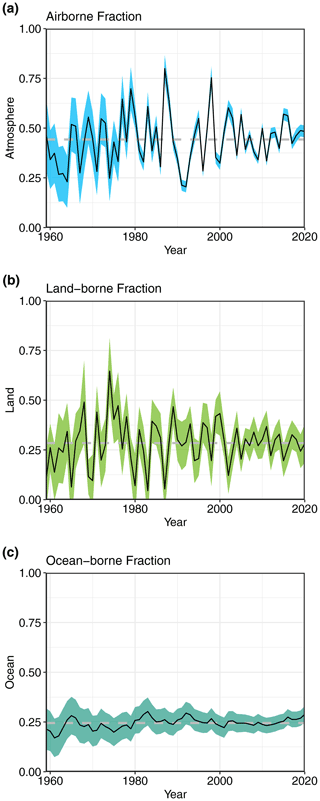
Figure 8The partitioning of total anthropogenic CO2 emissions (EFOS+ELUC) across (a) the atmosphere (airborne fraction), (b) land (land-borne fraction), and (c) ocean (ocean-borne fraction). Black lines represent the central estimate, and the coloured shading represents the uncertainty. The grey dashed lines represent the long-term average of the airborne (44 %), land-borne (28 %), and ocean-borne (24 %) fractions during 1959–2020.
3.4.3 Final year 2020
The growth rate in atmospheric CO2 concentration was 5.0 ± 0.2 GtC (2.37 ± 0.08 ppm) in 2020 (Fig. 4; Dlugokencky and Tans, 2022), very close to the 2011–2020 average. The 2020 decrease in EFOS and ELUC of about 0.7 GtC propagated to an atmospheric CO2 growth rate reduction of 0.38 GtC (0.18 ppm), given the significant interannual variability of the land carbon sink.
3.4.4 Year 2021 projection
The 2021 growth in atmospheric CO2 concentration (GATM) is projected to be about 5.3 GtC (2.49 ppm) based on GLO observations until the end of December 2021, bringing the atmospheric CO2 concentration to an expected level of 414.67 ppm averaged over the year, 50 % over the pre-industrial level.
3.5 Ocean sink
3.5.1 Historical period 1850–2020
Cumulated since 1850, the ocean sink adds up to 170 ± 35 GtC, with two-thirds of this amount being taken up by the global ocean since 1960. Over the historical period, the ocean sink increased in pace with the anthropogenic emissions exponential increase (Fig. 3b). Since 1850, the ocean has removed 26 % of total anthropogenic emissions.
3.5.2 Recent period 1960–2020
The ocean CO2 sink increased from 1.1 ± 0.4 GtC yr−1 in the 1960s to 2.8 ± 0.4 GtC yr−1 during 2011–2020 (Table 6), with interannual variations of the order of a few tenths of GtC yr−1 (Fig. 9). The ocean-borne fraction () has been remarkably constant, around 25 % on average (Fig. 8). Variations around this mean illustrate decadal variability of the ocean carbon sink. So far, there is no indication of a decrease in the ocean-borne fraction from 1960 to 2020. The increase in the ocean sink is primarily driven by the increased atmospheric CO2 concentration, with the strongest CO2-induced signal in the North Atlantic and the Southern Ocean (Fig. 10a). The effect of climate change is much weaker, reducing the ocean sink globally by 0.12 ± 0.07 GtC yr−1 or 5 % (2011–2020, range −0.8 % to −7.4 %), and does not show clear spatial patterns across the GOBMs ensemble (Fig. 10b). This is the combined effect of change and variability in all atmospheric forcing fields, previously attributed to wind and temperature changes in one model (Le Quéré et al., 2010).
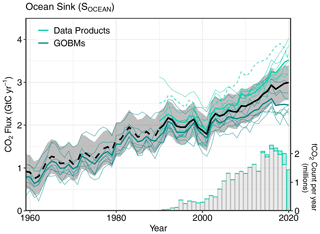
Figure 9Comparison of the anthropogenic atmosphere–ocean CO2 flux showing the budget values of SOCEAN (black; with the uncertainty in grey shading), individual ocean models (teal), and the ocean fCO2-based data products (cyan; with Watson et al. (2020) in dashed line as not used for ensemble mean). The fCO2-based data products were adjusted for the pre-industrial ocean source of CO2 from river input to the ocean, by subtracting a source of 0.61 GtC yr−1 to make them comparable to SOCEAN (see Sect. 2.4). Bar-plot in the lower right illustrates the number of fCO2 observations in the SOCAT v2021 database (Bakker et al., 2021). Grey bars indicate the number of data points in SOCAT v2020, and coloured bars the newly added observations in v2021.
The global net air–sea CO2 flux is a residual of large natural and anthropogenic CO2 fluxes into and out of the ocean with distinct regional and seasonal variations (Figs. 6 and B1). Natural fluxes dominate on regional scales but largely cancel out when integrated globally (Gruber et al., 2009). Mid-latitudes in all basins and the high-latitude North Atlantic dominate the ocean CO2 uptake where low temperatures and high wind speeds facilitate CO2 uptake at the surface (Takahashi et al., 2009). In these regions, mode, intermediate, and deep-water masses are formed that transport anthropogenic carbon into the ocean interior, thus allowing for continued CO2 uptake at the surface. Outgassing of natural CO2 occurs mostly in the tropics, especially in the equatorial upwelling region, and to a lesser extent in the North Pacific and polar Southern Ocean, mirroring a well-established understanding of regional patterns of air–sea CO2 exchange (e.g. Takahashi et al., 2009; Gruber et al., 2009). These patterns are also noticeable in the Surface Ocean CO2 Atlas (SOCAT) dataset, where an ocean fCO2 value above the atmospheric level indicates outgassing (Fig. B1). This map further illustrates the data sparsity in the Indian Ocean and the Southern Hemisphere in general.
Interannual variability of the ocean carbon sink is driven by climate variability with a first-order effect from a stronger ocean sink during large El Niño events (e.g. 1997–1998) (Fig. 9; Rödenbeck et al., 2014; Hauck et al., 2020). The GOBMs show the same patterns of decadal variability as the mean of the fCO2-based data products, with a stagnation of the ocean sink in the 1990s and a strengthening since the early 2000s (Fig. 9, Le Quéré et al., 2007; Landschützer et al., 2015, 2016; DeVries et al., 2017; Hauck et al., 2020; McKinley et al., 2020). Different explanations have been proposed for this decadal variability, ranging from the ocean's response to changes in atmospheric wind and pressure systems (e.g. Le Quéré et al., 2007; Keppler and Landschützer, 2019), including variations in upper ocean overturning circulation (DeVries et al., 2017), to the eruption of Mount Pinatubo and its effects on sea surface temperature and slowed atmospheric CO2 growth rate in the 1990s (McKinley et al., 2020). The main origin of the decadal variability is a matter of debate with a number of studies initially pointing to the Southern Ocean (see review in Canadell et al., 2022), but also contributions from the North Atlantic and North Pacific (Landschützer et al., 2016; DeVries et al., 2019) or a global signal (McKinley et al., 2020) were proposed.
Although all individual GOBMs and data products fall within the observational constraint, the ensemble means of GOBMs and data products adjusted for the riverine flux diverge over time with a mean offset increasing from 0.24 GtC yr−1 in the 1990s to 0.66 GtC yr−1 in the decade 2011–2020 and reaching 1.1 GtC yr−1 in 2020. The SOCEAN trend diverges with a factor-of-2 difference since 2002 (GOBMs: 0.3 ± 0.1 GtC yr−1 per decade, data products: 0.7 ± 0.2 GtC yr−1 per decade, best estimate: 0.5 GtC yr−1 per decade) and with a factor-of-3 since 2010 (GOBMs: 0.3 ± 0.1 GtC yr−1 per decade, data products: 0.9 ± 0.3 GtC yr−1 per decade, best estimate: 0.6 GtC yr−1 per decade). The GOBM estimate is lower than in the previous global carbon budget (Friedlingstein et al., 2020), because one high-sink model was not available. The effect of two models (CNRM, MOM6-COBALT) revising their estimates downwards was largely balanced by two models revising their estimate upwards (FESOM-REcoM, PlankTOM).
The discrepancy between the two types of estimates stems mostly from a larger Southern Ocean sink in the data products prior to 2001, and from a larger SOCEAN trend in the northern and southern extra-tropics since then (Fig. 12). Possible explanations for the discrepancy in the Southern Ocean could be missing winter observations and data sparsity in general (Bushinsky et al., 2019; Gloege et al., 2021), model biases (as indicated by the large model spread in the south, Fig. 12, and the larger model–data mismatch, Fig. B2), or uncertainties in the regional river flux adjustment (Hauck et al., 2020; Lacroix et al., 2020).
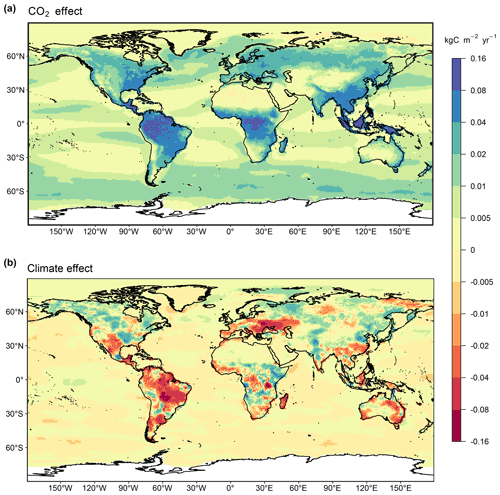
Figure 10Attribution of the atmosphere–ocean (SOCEAN) and atmosphere–land (SLAND) CO2 fluxes to (a) increasing atmospheric CO2 concentrations and (b) changes in climate, averaged over the previous decade 2011–2020. All data shown are from the processed-based GOBMs and DGVMs. The sum of ocean CO2 and climate effects will not equal the ocean sink shown in Fig. 6 which includes the fCO2-based data products. See Appendix Sects. C3.2 and C4.1 for attribution methodology. Units are in kgC m−2 yr−1 (note the non-linear colour scale).
During 2010–2016, the ocean CO2 sink appears to have intensified in line with the expected increase from atmospheric CO2 (McKinley et al., 2020). This effect is stronger in the fCO2-based data products (Fig. 9, GOBMs: +0.43 GtC yr−1, data products: +0.56 GtC yr−1). The reduction of −0.09 GtC yr−1 (range: −0.30 to +0.12 GtC yr−1) in the ocean CO2 sink in 2017 is consistent with the return to normal conditions after the El Niño in 2015–2016, which caused an enhanced sink in previous years. After 2017, the GOBMs ensemble mean suggests the ocean sink levels off at about 2.5 GtC yr−1, whereas the data products' estimate increases by 0.3 GtC yr−1 over the same period.
3.5.3 Final year 2020
The estimated ocean CO2 sink was 3.0 ± 0.4 GtC in 2020. This is the average of GOBMs and data products, and is a small increase of 0.02 GtC compared to 2019, in line with the competing effects from an expected sink strengthening from atmospheric CO2 growth and expected sink weakening from La Niña conditions. There is, however, a substantial difference between GOBMs and fCO2-based data products in their mean 2020 SOCEAN estimate (GOBMs: 2.5 GtC, data products: 3.5 GtC). While the GOBMs simulate a stagnation of the sink from 2019 to 2020 (−0.02 ± 0.11 GtC), the data products suggest an increase by 0.06 GtC, although not significant at the 1σ level (±0.13 GtC). Four models and four data products show an increase in SOCEAN (GOBMs up to +0.18 GtC, data product up to +0.21 GtC), while four models and three data products show no change or a decrease in SOCEAN (GOBMs down to −0.12 GtC, data products down to −0.13 GtC; Fig. 9). The data products have a larger uncertainty at the tails of the reconstructed time series (e.g. Watson et al., 2020). Specifically, the data products' estimate of the last year is regularly adjusted in the following release owing to the tail effect and an incrementally increasing data availability with 1–5 years lag (Fig. 9 bottom).
3.5.4 Year 2021 projection
Using a feed-forward neural network method (see Sect. 2.4) we project an ocean sink of 2.9 GtC for 2021. This is a reduction of the sink by 0.1 GtC relative to the 2020 value, which we attribute to La Niña conditions in January to May 2021 and projections of a re-emergence of La Niña later in the year.
3.5.5 Model evaluation
The evaluation of the ocean estimates (Fig. B2) shows an RMSE from annually detrended data of 1.3 to 2.8 µatm for the seven fCO2-based data products over the globe, relative to the fCO2 observations from the SOCAT v2021 dataset for the period 1990–2020. The GOBMs RMSEs are larger and range from 3.3 to 5.9 µatm. The RMSEs are generally larger at high latitudes compared to the tropics, for both the data products and the GOBMs. The data products have RMSEs of 1.3 to 3.6 µatm in the tropics, 1.3 to 2.7 µatm in the north, and 2.2 to 6.1 µatm in the south. Note that the data products are based on the SOCAT v2021 database; hence the latter are not an independent dataset for the evaluation of the data products. The GOBM RMSEs are more spread across regions, ranging from 2.7 to 4.3 µatm in the tropics, 2.9 to 6.9 µatm in the north, and 6.4 to 9.8 µatm in the south. The higher RMSEs occur in regions with stronger climate variability, such as the northern and southern high latitudes (poleward of the subtropical gyres). The upper ranges of the model RMSEs have decreased somewhat relative to Friedlingstein et al. (2020), owing to one model with upper-end RMSE not being represented this year, and the reduction of RMSE in one model (MPIOM-HAMOCC6), presumably related to the inclusion of riverine carbon fluxes.
The additional simulation C allows the steady-state anthropogenic carbon component (sim C – sim B) to be separated and the model flux and DIC inventory change to be compared directly to the interior ocean estimate of Gruber et al. (2019) without further assumptions. The GOBMs ensemble average of steady-state anthropogenic carbon inventory change 1994–2007 amounts to 2.1 GtC yr−1 and is significantly lower than the 2.6 ± 0.3 GtC yr−1 estimated by Gruber et al. (2019). Only the three models with the highest sink estimate fall within the range reported by Gruber et al. (2019). This suggests that most of the models underestimate anthropogenic carbon uptake by the ocean likely due to biases in ocean carbon transport and mixing from the surface mixed layer to the ocean interior.
The reported SOCEAN estimate from GOBMs and data products is 2.1 ± 0.4 GtC yr−1 over the period 1994 to 2007, which is in agreement with the ocean interior estimate of 2.2 ± 0.4 GtC yr−1 when accounting for the climate effect on the natural CO2 flux of −0.4 ± 0.24 GtC yr−1 (Gruber et al., 2019) to match the definition of SOCEAN used here (Hauck et al., 2020). This comparison depends critically on the estimate of the climate effect on the natural CO2 flux, which is smaller from the GOBMs (Sect. 3.5.2) than in Gruber et al. (2019).
3.6 Land sink
3.6.1 Historical period 1850–2020
Cumulated since 1850, the terrestrial CO2 sink amounts to 195 ± 45 GtC, 30 % of total anthropogenic emissions. Over the historical period, the sink increased in pace with the anthropogenic emissions exponential increase (Fig. 3b).
3.6.2 Recent period 1960–2020
The terrestrial CO2 sink increased from 1.2 ± 0.5 GtC yr−1 in the 1960s to 3.1 ± 0.6 GtC yr−1 during 2010–2019, with important interannual variations of up to 2 GtC yr−1 generally showing a decreased land sink during El Niño events (Fig. 7), responsible for the corresponding enhanced growth rate in atmospheric CO2 concentration. The larger land CO2 sink during 2010–2019 compared to the 1960s is reproduced by all the DGVMs in response to the combined atmospheric CO2 increase and the changes in climate, and consistent with constraints from the other budget terms (Table 5).
Over the period 1960 to present the increase in the global terrestrial CO2 sink is largely attributed to the CO2 fertilization effect in the models (Prentice et al., 2001; Piao et al., 2009), directly stimulating plant photosynthesis and increased plant water use in water-limited systems, with a small negative contribution of climate change (Fig. 10). There is a range of evidence to support a positive terrestrial carbon sink in response to increasing atmospheric CO2, albeit with uncertain magnitude (Walker et al., 2021). As expected from theory the greatest CO2 effect is simulated in the tropical forest regions, associated with warm temperatures and long growing seasons (Hickler et al., 2008) (Fig. 10a). However, evidence from tropical intact forest plots indicate an overall decline in the land sink across Amazonia (1985–2011), attributed to enhanced mortality offsetting productivity gains (Brienen et al., 2005; Hubau et al., 2020). During 2011–2020 the land sink is positive in all regions (Fig. 6) with the exception of central and eastern Brazil, southwest USA and northern Mexico, southeast Europe and central Asia, South Africa, and eastern Australia, where the negative effects of climate variability and change (i.e. reduced rainfall) counterbalance CO2 effects. This is clearly visible in Fig. 10 where the effects of CO2 (Fig. 10a) and climate (Fig. 10b) as simulated by the DGVMs are isolated. The negative effect of climate is the strongest in most of South America, Central America, southwest USA, and central Europe (Fig. 10b). Globally, climate change reduces the land sink by 0.45 ± 0.39 GtC yr−1 or 15 % (2011–2020).
In the past years several regions experienced record-setting fire events. While global burned area has declined over the past decades mostly due to declining fire activity in savannas (Andela et al., 2017), forest fire emissions are rising and have the potential to counter the negative fire trend in savannas (Zheng et al., 2021). Noteworthy events include the 2019–2020 Black Summer event in Australia (emissions of roughly 0.2 GtC; van der Velde et al., 2021) and Siberia in 2021, where emissions approached 0.4 GtC or 3 times the 1997–2020 average according to GFED4s. While other regions, including western USA and Mediterranean Europe, also experienced intense fire seasons in 2021 their emissions are substantially lower.
Despite these regional negative effects of climate change on SLAND, the efficiency of land to remove anthropogenic CO2 emissions has remained broadly constant over the last six decades, with a land-borne fraction () of ∼ 30 % (Fig. 8).
3.6.3 Final year 2020
The terrestrial CO2 sink from the DGVM ensemble was 2.9 ± 1.0 GtC in 2020, slightly below the decadal average of 3.1 GtC yr−1 (Fig. 4, Table 6). We note that the DGVM estimate for 2020 is significantly larger than the 2.1 ± 0.9 GtC yr−1 estimate from the residual sink from the global budget () (Table 5).
3.6.4 Year 2021 projection
Using a feed-forward neural network method (see Sect. 2.5) we project a land sink of 3.3 GtC for 2021. This is an increase in the land sink by 0.3 GtC relative to the 2020 value which we attribute to La Niña conditions in 2021.
3.6.5 Model evaluation
The evaluation of the DGVMs (Fig. B3) shows generally high skill scores across models for runoff, and to a lesser extent for vegetation biomass, GPP, and ecosystem respiration (Fig. B3a). Skill score was lowest for leaf area index and net ecosystem exchange, with the widest disparity among models for soil carbon. Further analysis of the results will be provided separately, focusing on the strengths and weaknesses in the DGVMs ensemble and its validity for use in the global carbon budget.
3.7 Partitioning the carbon sinks
3.7.1 Global sinks and spread of estimates
In the period 2011–2020, the bottom-up view of total global carbon sinks provided by the GCB () agrees closely with the top-down budget delivered by the atmospheric inversions. Figure 11 shows both total sink estimates of the last decade split by land and ocean, which match the difference between GATM and EFOS to within 0.06–0.17 GtC yr−1 for inverse models and to 0.3 GtC yr−1 for the GCB mean. The latter represents the BIM discussed in Sect. 3.8, which by design is minimal for the inverse models.
The distributions based on the individual models and data products reveal substantial spread but converge near the decadal means quoted in Tables 5 and 6. Sink estimates for SOCEAN and from inverse models are mostly non-Gaussian, while the ensemble of DGVMs appears more normally distributed justifying the use of a multi-model mean and standard deviation for their errors in the budget. Noteworthy is that the tails of the distributions provided by the land and ocean bottom-up estimates would not agree with the global constraint provided by the fossil fuel emissions and the observed atmospheric CO2 growth rate (EFOS−GATM). This illustrates the power of the atmospheric joint constraint from GATM and the global CO2 observation network it derives from.
3.7.2 Total atmosphere-to-land fluxes
The total atmosphere-to-land fluxes (SLAND−ELUC), calculated here as the difference between SLAND from the DGVMs and ELUC from the bookkeeping models, amounts to a 1.9 ± 0.9 GtC yr−1 sink during 2011–2020 (Table 5). Estimates of total atmosphere-to-land fluxes (SLAND−ELUC) from the DGVMs alone (1.6 ± 0.6 GtC yr−1) are consistent with this estimate and also with the global carbon budget constraint (, 1.7 ± 0.8 GtC yr−1 Table 5). Consistent with the bookkeeping model estimates, the DGVM-based ELUC is substantially lower than in Friedlingstein et al. (2020) due to the improved land-cover forcing (see Sect. 3.2.2), increasing their total atmosphere-to-land fluxes and hence the consistency with the budget constraint. For the last decade (2011–2020), the inversions estimate the net atmosphere-to-land uptake to lie within a range of 1.3 to 2.0 GtC yr−1, consistent with the GCB and DGVM estimates of SLAND−ELUC (Fig. 11, Fig. 2, top row).
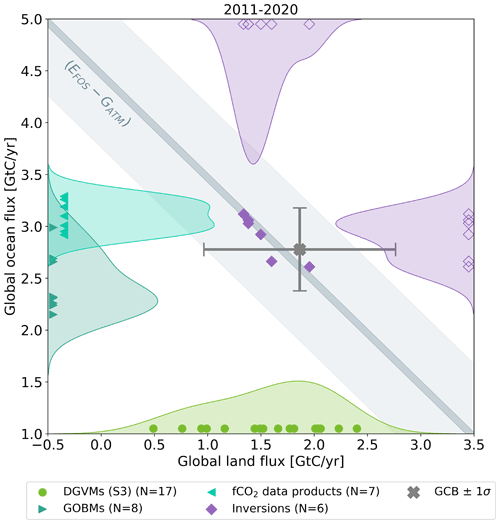
Figure 11The 2011–2020 decadal mean net atmosphere–ocean and atmosphere–land fluxes derived from the ocean models and fCO2 products (y axis, right- and left-pointing blue triangles respectively) and from the DGVMs (x axis, green symbols), and the same fluxes estimated from the six inversions (purple symbols on secondary x and y axis). The grey central point is the mean (±1σ) of SOCEAN and (SLAND − ELUC) as assessed in this budget. The shaded distributions show the density of the ensemble of individual estimates. The grey diagonal band represents the fossil fuel emissions minus the atmospheric growth rate from this budget (EFOS − GATM). Note that positive values are CO2 sinks.
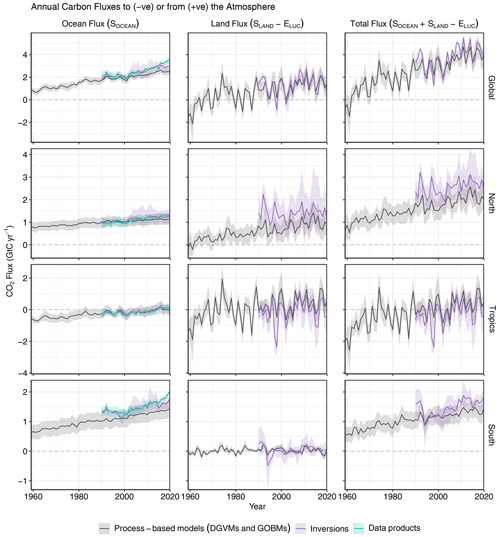
Figure 12CO2 fluxes between the atmosphere and the Earth's surface separated between land and oceans, globally and in three latitude bands. The ocean flux is SOCEAN and the land flux is the net atmosphere–land fluxes from the DGVMs. The latitude bands are (top row) global, (second row) north (> 30∘ N), (third row) tropics (30∘ S–30∘ N), and (bottom row) south (<30∘ S), and over ocean (left column), over land (middle column), and total (right column). Estimates are shown for process-based models (DGVMs for land, GOBMs for oceans), inversion models (land and ocean), and fCO2-based data products (ocean only). Positive values indicate a flux from the atmosphere to the land or the ocean. Mean estimates from the combination of the process models for the land and oceans are shown (black line) with ±1 standard deviation (1σ) of the model ensemble (grey shading). For the total uncertainty in the process-based estimate of the total sink, uncertainties are summed in quadrature. Mean estimates from the atmospheric inversions are shown (purple lines) with their full spread (purple shading). Mean estimates from the fCO2-based data products are shown for the ocean domain (light blue lines) with their ±1σ spread (light blue shading). The global SOCEAN (upper left) and the sum of SOCEAN in all three regions represents the anthropogenic atmosphere-to-ocean flux based on the assumption that the pre-industrial ocean sink was 0 GtC yr−1 when riverine fluxes are not considered. This assumption does not hold at the regional level, where pre-industrial fluxes can be significantly different from zero. Hence, the regional panels for SOCEAN represent a combination of natural and anthropogenic fluxes. Bias correction and area weighting were only applied to global SOCEAN; hence the sum of the regions is slightly different from the global estimate (< 0.06 GtC yr−1).
3.7.3 Total atmosphere-to-ocean fluxes
For the 2011–2020 period, the GOBMs (2.5 ± 0.6 GtC yr−1) produce a lower estimate for the ocean sink than the fCO2-based data products (3.1 ± 0.5 GtC yr−1), which shows up in Fig. 11 as a separate peak in the distribution from the GOBMs (triangle symbols pointing right) and from the fCO2-based products (triangle symbols pointing left). Atmospheric inversions (2.6 to 3.1 GtC yr−1) also suggest higher ocean uptake in the recent decade (Fig. 11, Fig. 12 top row). In interpreting these differences, we caution that the riverine transport of carbon taken up on land and outgassing from the ocean is a substantial (0.6 GtC yr−1) and uncertain term that separates the various methods. A recent estimate of decadal ocean uptake from observed O2 N2 ratios (Tohjima et al., 2019) also points towards a larger ocean sink, albeit with large uncertainty (2012–2016: 3.1 ± 1.5 GtC yr−1).
3.7.4 Regional breakdown and interannual variability
Figure 12 also shows the latitudinal partitioning of the total atmosphere-to-surface fluxes excluding fossil CO2 emissions () according to the multi-model average estimates from GOBMs and ocean fCO2-based products (SOCEAN) and DGVMs (SLAND−ELUC), and from atmospheric inversions (SOCEAN and SLAND−ELUC).
North
Despite being one of the most densely observed and studied regions of our globe, annual mean carbon sink estimates in the northern extra-tropics (north of 30∘ N) continue to differ by about 0.5 GtC yr−1. The atmospheric inversions suggest an atmosphere-to-surface sink () for 2011–2020 of 2.0 to 3.4 GtC yr−1, which is higher than the process models' estimate of 2.1 ± 0.5 GtC yr−1 (Fig. 12). The GOBMs (1.1 ± 0.2 GtC yr−1), fCO2-based data products (1.3 ± 0.1 GtC yr−1), and inversion models (0.9 to 1.5 GtC yr−1) produce consistent estimates of the ocean sink. Thus, the difference mainly arises from the total land flux (SLAND−ELUC) estimate, which is 1.0 ± 0.4 GtC yr−1 in the DGVMs compared to 0.7 to 2.4 GtC yr−1 in the atmospheric inversions (Fig. 12, second row).
Discrepancies in the northern land fluxes conforms with persistent issues surrounding the quantification of the drivers of the global net land CO2 flux (Arneth et al., 2017; Huntzinger et al., 2017) and the distribution of atmosphere-to-land fluxes between the tropics and high northern latitudes (Baccini et al., 2017; Schimel et al., 2015; Stephens et al., 2007; Ciais et al., 2019; Gaubert et al., 2019).
In the northern extra-tropics, the process models, inversions, and fCO2-based data products consistently suggest that most of the variability stems from the land (Fig. 12). Inversions generally estimate similar interannual variations (IAV) over land to DGVMs (0.28–0.47 vs. 0.20–0.73 GtC yr−1, averaged over 1990–2020), and they have higher IAV in ocean fluxes (0.03–0.19 GtC yr−1) relative to GOBMs (0.03–0.05 GtC yr−1, Fig. B2) and fCO2-based data products (0.03–0.09 GtC yr−1).
Tropics
In the tropics (30∘ S–30∘ N), both the atmospheric inversions and process models estimate a total carbon balance () that is close to neutral over the past decade. The GOBMs (0.0 ± 0.3 GtC yr−1), fCO2-based data products (0.03 ± 0.2 GtC yr−1), and inversion models (−0.2 to 0.2 GtC yr−1) all indicate an approximately neutral tropical ocean flux (see Fig. B1 for spatial patterns). DGVMs indicate a net land sink (SLAND−ELUC) of 0.6 ± 0.3 GtC yr−1, whereas the inversion models indicate a net land flux between −0.7 and 0.9 GtC yr−1, though with high uncertainty (Fig. 12, third row).
The tropical lands are the origin of most of the atmospheric CO2 interannual variability (Ahlström et al., 2015), consistently among the process models and inversions (Fig. 12). The interannual variability in the tropics is similar among the ocean data products (0.07–0.15 GtC yr−1) and the models (0.07–0.15 GtC yr−1, Fig. B2), which is the highest ocean sink variability of all regions. The DGVMs and inversions indicate that atmosphere-to-land CO2 fluxes are more variable than atmosphere-to-ocean CO2 fluxes in the tropics, with interannual variability of 0.4 to 1.2 and 0.6 to 1.1 GtC yr−1 respectively.
South
In the southern extra-tropics (south of 30∘ S), the atmospheric inversions suggest a total atmosphere-to-surface sink () for 2011–2020 of 1.6 to 1.9 GtC yr−1, slightly higher than the process models' estimate of 1.4 ± 0.3 GtC yr−1 (Fig. 12). An approximately neutral total land flux (SLAND−ELUC) for the southern extra-tropics is estimated by both the DGVMs (0.02 ± 0.05 GtC yr−1) and the inversion models (sink of −0.1 to 0.2 GtC yr−1). This means nearly all carbon uptake is due to oceanic sinks south of 30∘ S. The southern ocean flux in the fCO2-based data products (1.7 ± 0.1 GtC yr−1) and inversion estimates (1.4 to 1.8 GtC yr−1) is higher than in the GOBMs (1.4 ± 0.3 GtC yr−1) (Fig. 12, bottom row). This might be explained by the data products potentially underestimating the winter CO2 outgassing south of the Polar Front (Bushinsky et al., 2019), by model biases, or by the uncertainty in the regional distribution of the river flux adjustment (Aumont et al., 2001; Lacroix et al., 2020) applied to fCO2-based data products and inverse models to isolate the anthropogenic SOCEAN flux. CO2 fluxes from this region are more sparsely sampled by all methods, especially in wintertime (Fig. B1).
The interannual variability in the southern extra-tropics is low because of the dominance of ocean area with low variability compared to land areas. The split between land (SLAND−ELUC) and ocean (SOCEAN) shows a substantial contribution to variability in the south coming from the land, with no consistency between the DGVMs and the inversions or among inversions. This is expected due to the difficulty of separating exactly the land and oceanic fluxes when viewed from atmospheric observations alone. The SOCEAN interannual variability was found to be higher in the fCO2-based data products (0.09 to 0.14 GtC yr−1) compared to GOBMs (0.04 to 0.06 GtC yr−1) in 1990–2020 (Fig. B2). Model subsampling experiments recently illustrated that observation-based products may overestimate decadal variability in the Southern Ocean carbon sink by 30 % due to data sparsity, based on one data product with the highest decadal variability (Gloege et al., 2021).
Tropical vs. northern land uptake
A continuing conundrum is the partitioning of the global atmosphere–land flux between the Northern Hemisphere land and the tropical land (Stephens et al., 2017; Pan et al., 2011; Gaubert et al., 2019). It is of importance because each region has its own history of land-use change, climate drivers, and impact of increasing atmospheric CO2 and nitrogen deposition. Quantifying the magnitude of each sink is a prerequisite to understanding how each individual driver impacts the tropical and mid- to high-latitude carbon balance.
We define the north–south (N–S) difference as net atmosphere–land flux north of 30∘ N minus the net atmosphere–land flux south of 30∘ N. For the inversions, the N–S difference ranges from −0.1 to 2.9 GtC yr−1 across this year's inversion ensemble with an equal preference across models for either a small northern land sink and a tropical land sink (small N–S difference), a medium northern land sink and a neutral tropical land flux (medium N–S difference), or a large northern land sink and a tropical land source (large N–S difference).
In the ensemble of DGVMs the N–S difference is 0.5 ± 0.5 GtC yr−1, a much narrower range than the one from inversions. Only three DGVMs have a N–S difference larger than 1.0 GtC yr−1. The larger agreement across DGVMs than across inversions is to be expected as there is no correlation between northern and tropical land sinks in the DGVMs as opposed to the inversions where the sum of the two regions being well-constrained leads to an anti-correlation between these two regions. The much smaller spread in the N–S difference between the DGVMs could help to scrutinize the inverse models further. For example, a large northern land sink and a tropical land source in an inversion would suggest a large sensitivity to CO2 fertilization (the dominant factor driving the land sinks) for northern ecosystems, which would be not mirrored by tropical ecosystems. Such a combination could be hard to reconcile with the process understanding gained from the DGVMs ensembles and independent measurements (e.g. free-air CO2 enrichment experiments). Such investigations will be further pursued in the upcoming assessment from REgional Carbon Cycle Assessment and Processes (RECCAP2; Ciais et al., 2022).
3.8 Closing the global carbon cycle
3.8.1 Partitioning of cumulative emissions and sink fluxes
The global carbon budget over the historical period (1850–2020) is shown in Fig. 3.
Emissions during the period 1850–2020 amounted to 660 ± 65 GtC and were partitioned among the atmosphere (270 ± 5 GtC; 41 %), ocean (170 ± 35 GtC; 26 %), and the land (195 ± 45 GtC; 30 %). The cumulative land sink is almost equal to the cumulative land-use emissions (200 ± 65 GtC), making the global land nearly neutral over the whole 1850–2020 period.
The use of nearly independent estimates for the individual terms shows a cumulative budget imbalance of 25 GtC (4 %) during 1850–2020 (Fig. 3, Table 8), which, if correct, suggests that emissions are slightly too high by the same proportion (4 %) or that the combined land and ocean sinks are slightly underestimated (by about 7 %). The bulk of the imbalance could originate from the estimation of large ELUC between the mid-1920s and the mid-1960s which is unmatched by a growth in atmospheric CO2 concentration as recorded in ice cores (Fig. 3). However, the known loss of additional sink capacity of 30–40 GtC (over the 1850–2020 period) due to reduced forest cover has not been accounted for in our method and would further exacerbate the budget imbalance (Sect. 2.7.4).
For the more recent 1960–2020 period where direct atmospheric CO2 measurements are available, 375 ± 20 GtC (82 %) of the total emissions (EFOS+ELUC) were caused by fossil CO2 emissions, and 80 ± 45 GtC (18 %) by land-use change (Table 8). The total emissions were partitioned among the atmosphere (205 ± 5 GtC; 47 %), ocean (115 ± 25 GtC; 25 %), and the land (135 ± 25 GtC; 30 %), with a near-zero unattributed budget imbalance. All components except land-use change emissions have significantly grown since 1960, with important interannual variability in the growth rate in atmospheric CO2 concentration and in the land CO2 sink (Fig. 4), and some decadal variability in all terms (Table 6). Differences with previous budget releases are documented in Fig. B5.
The global carbon budget averaged over the last decade (2011–2020) is shown in Figs. 2 and 13b and Table 6. For this time period, 90 % of the total emissions (EFOS+ELUC) were from fossil CO2 emissions (EFOS), and 10 % from land-use change (ELUC). The total emissions were partitioned among the atmosphere (47 %), ocean (26 %), and land (29 %), with a near-zero unattributed budget imbalance (∼ 3 %). For single years, the budget imbalance can be larger (Fig. 4). For 2020, the combination of our sources and sinks estimates leads to a BIM of −0.8 GtC, suggesting an underestimation of the anthropogenic sources (potentially ELUC), and/or an overestimation of the combined land and ocean sinks
3.8.2 Carbon budget imbalance
The carbon budget imbalance (BIM; Eq. 1, Fig. 4) quantifies the mismatch between the estimated total emissions and the estimated changes in the atmosphere, land, and ocean reservoirs. The mean budget imbalance from 1960 to 2020 is very small (average of 0.03 GtC yr−1) and shows no trend over the full time series. The process models (GOBMs and DGVMs) and data products have been selected to match observational constraints in the 1990s, but no further constraints have been applied to their representation of trend and variability. Therefore, the near-zero mean and trend in the budget imbalance is seen as evidence of a coherent community understanding of the emissions and their partitioning on those timescales (Fig. 4). However, the budget imbalance shows substantial variability of the order of ±1 GtC yr−1, particularly over semi-decadal timescales, although most of the variability is within the uncertainty of the estimates. The positive carbon imbalance during the 1960s, and early 1990s, indicates that either the emissions were overestimated, or the sinks were underestimated during these periods. The reverse is true for the 1970s, 1980s, and for the 2011–2020 period (Fig. 4, Table 6).
We cannot attribute the cause of the variability in the budget imbalance with our analysis; we only note that the budget imbalance is unlikely to be explained by errors or biases in the emissions alone because of its large semi-decadal variability component, a variability that is untypical of emissions and has not changed in the past 60 years despite a near tripling in emissions (Fig. 4). Errors in SLAND and SOCEAN are more likely to be the main cause for the budget imbalance. For example, underestimation of SLAND by DGVMs has been reported following the eruption of Mount Pinatubo in 1991 possibly due to missing responses to changes in diffuse radiation (Mercado et al., 2009). Although in GCB2021 we have for the first time accounted for aerosol effects on solar radiation quantity and quality (diffuse vs. direct), most DGVMs only used the former as input (i.e. total solar radiation). Thus, the ensemble mean may not capture the full effects of volcanic eruptions, i.e. associated with high light scattering sulfate aerosols, on the land carbon sink (O'Sullivan et al., 2021). DGVMs are suspected to overestimate the land sink in response to the wet decade of the 1970s (Sitch et al., 2008). Quasi-decadal variability in the ocean sink has also been reported, with all methods agreeing on a smaller than expected ocean CO2 sink in the 1990s and a larger than expected sink in the 2000s (Fig. 9; Landschützer et al., 2016; DeVries et al., 2019; Hauck et al., 2020; McKinley et al., 2020). Errors in sink estimates could also be driven by errors in the climatic forcing data, particularly precipitation for SLAND and wind for SOCEAN.
The budget imbalance (BIM) was negative (−0.3 GtC yr−1) on average over 2011–2020, although the BIM uncertainty is large (1.1 GtC yr−1 over the decade). Also, the BIM shows substantial departure from zero on yearly timescales (Fig. 4), highlighting unresolved variability of the carbon cycle, likely in the land sink (SLAND), given its large year-to-year variability (Figs. 4e and 7).
Both the budget imbalance (BIM, Table 6) and the residual land sink from the global budget (, Table 5) include an error term due to the inconsistencies that arise from using ELUC from bookkeeping models, and SLAND from DGVMs, most notably the loss of additional sink capacity (see Sect. 2.7). Other differences include a better accounting of changing land-use practices and processes in bookkeeping models than in DGVMs, or the bookkeeping models' error of having present-day observed carbon densities fixed in the past. That the budget imbalance shows no clear trend towards larger values over time is an indication that these inconsistencies probably play a minor role compared to other errors in SLAND or SOCEAN.
Although the budget imbalance is near zero for the recent decades, it could be due to compensation of errors. We cannot exclude an overestimation of CO2 emissions, particularly from land-use change, given their large uncertainty, as has been suggested elsewhere (Piao et al., 2018), combined with an underestimate of the sinks. A larger SLAND would reconcile model results with inversion estimates for fluxes in the total land during the past decade (Fig. 12; Table 5). Likewise, a larger SOCEAN is also possible given the higher estimates from the data products (see Sect. 3.1.2, Figs. 9 and 12) and the recently suggested upward correction of the ocean carbon sink (Watson et al., 2020, Fig. 9). If SOCEAN were to be based on data products alone, with all data products including the Watson et al. (2020) adjustment, this would result in a 2011–2020 SOCEAN of nearly 4 GtC yr−1, outside of the range supported by the atmospheric inversions, with a negative BIM of more than 1 GtC yr−1 indicating that a closure of the budget could only be achieved with either anthropogenic emissions being larger and/or the net land sink being substantially smaller than estimated here. More integrated use of observations in the Global Carbon Budget, either on their own or for further constraining model results, should help resolve some of the budget imbalance (Peters et al., 2017).
Fossil CO2 emissions growth peaked at +3 % yr−1 during the 2000s, driven by the rapid growth in Chinese emissions. In the last decade, however, the growth rate for the preceding 10 years has slowly declined, reaching a low +0.4 % yr−1 from 2012–2021 (including the 2020 global decline and the expected 2021 emissions rebound). While this slowdown in global fossil CO2 emissions growth is welcome, it is far from what is needed to be consistent with the temperature goals of the Paris Agreement.
Since the 1990s, the average growth rate of fossil CO2 emissions has continuously declined across the group of developed countries of the Organisation for Economic Co-operation and Development (OECD), with emissions peaking in around 2005 and now declining at around 1 % yr−1 (Le Quéré et al., 2021). In the decade 2010–2019, territorial fossil CO2 emissions decreased significantly (at the 95 % confidence level) in 23 countries whose economies grew significantly (also at the 95 % confidence level): Barbados, Belgium, Croatia, Czech Republic, Denmark, Finland, France, Germany, Israel, Japan, Luxembourg, North Macedonia, Malta, Mexico, the Netherlands, Slovakia, Slovenia, Solomon Islands, Sweden, Switzerland, Tuvalu, United Kingdom, and USA (updated from Le Quéré et al., 2019). Altogether, these 23 countries contributed 2.5 GtC yr−1 over the last decade, about one-quarter of world CO2 fossil emissions. Consumption-based emissions are also falling significantly in 15 of these countries (Belgium, Croatia, Czech Republic, Denmark, Finland, France, Germany, Israel, Japan, Mexico, the Netherlands, Slovenia, Sweden, United Kingdom, and USA). Figure 14 shows that the emission declines in the USA and the EU27 are primarily driven by increased decarbonization (CO2 emissions per unit energy) in the last decade compared to the previous, with smaller contributions in the EU27 from slightly weaker economic growth and slightly larger declines in energy per GDP. These countries have stable or declining energy use and so decarbonization policies replace existing fossil fuel infrastructure (Le Quéré et al., 2019).
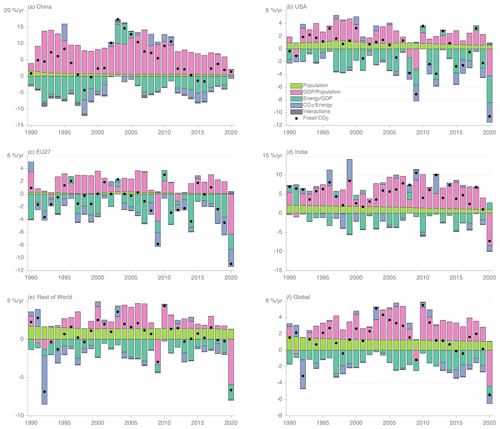
Figure 14Kaya decomposition of the main drivers of fossil CO2 emissions, considering population, GDP per person, energy per GDP, and CO2 emissions per energy use, for China (a), USA (b), EU27 (c), India (d), the rest of the world (e), and the world (f). Black dots are the annual fossil CO2 emissions growth rate, coloured bars are the contributions from the different drivers. A general trend is that population and GDP growth put upward pressure on emissions, while energy per GDP and more recently CO2 emissions per energy put downward pressure on emissions. The changes during 2020 led to a stark contrast to previous years, with different drivers in each region.
In contrast, fossil CO2 emissions continue to grow in non-OECD countries, although the growth rate has slowed from over 5 % yr−1 during the 2000s to around 2 % yr−1 in the last decade. A large part of this slowdown in non-OECD countries is due to China, which has seen emissions growth declining from nearly 10 % yr−1 in the 2000s to 2 % yr−1 in the last decade. Excluding China, non-OECD emissions grew at 3 % yr−1 in the 2000s compared to 2 % yr−1 in the last decade. Figure 14 shows that compared to the previous decade, China has had weaker economic growth in the last decade and a larger decarbonization rate, with more rapid declines in energy per GDP which are now back to levels during the 1990s. India and the rest of the world have strong economic growth that is not compensated by decarbonization or declines in energy per GDP, implying fossil CO2 emissions continue to grow. Despite the high deployment of renewables in some countries (e.g. India), fossil energy sources continue to grow to meet growing energy demand (Le Quéré et al., 2019).
Globally, fossil CO2 emissions growth is slowing, and this is primarily due to the emergence of climate policy and emission declines in OECD countries (Eskander and Fankhauser, 2020). At the aggregated global level, decarbonization shows a strong and growing signal in the last decade, with smaller contributions from lower economic growth and declines in energy per GDP. Despite the slowing growth in global fossil CO2 emissions, emissions are still growing, far from the reductions needed to meet the ambitious climate goals of the UNFCCC Paris agreement.
We update the remaining carbon budget assessed by the IPCC AR6 (Canadell et al., 2022), accounting for the 2020 and estimated 2021 emissions from fossil fuel combustion (EFOS) and land-use changes (ELUC). From January 2022, the remaining carbon (50 % likelihood) for limiting global warming to 1.5, 1.7, and 2 ∘C is estimated to amount to 120, 210, and 350 GtC (420, 770, 1270 GtCO2). These numbers include an uncertainty based on model spread (as in IPCC AR6), which is reflected through the percent likelihood of exceeding the given temperature threshold. These remaining amounts correspond respectively to about 11, 20, and 32 years from the beginning of 2022, at the 2021 level of total CO2 emissions. Reaching net-zero CO2 emissions by 2050 entails cutting total anthropogenic CO2 emissions by about 0.4 GtC (1.4 GtCO2) each year on average, comparable to the decrease during 2020.
Each year when the global carbon budget is published, each flux component is updated for all previous years to consider corrections that are the result of further scrutiny and verification of the underlying data in the primary input datasets. Annual estimates may be updated with improvements in data quality and timeliness (e.g. to eliminate the need for extrapolation of forcing data such as land use). Of all terms in the global budget, only the fossil CO2 emissions and the growth rate in atmospheric CO2 concentration are based primarily on empirical inputs supporting annual estimates in this carbon budget. The carbon budget imbalance, yet an imperfect measure, provides a strong indication of the limitations in observations in understanding and representing processes in models, and/or in the integration of the carbon budget components.
The persistent unexplained variability in the carbon budget imbalance limits our ability to verify reported emissions (Peters et al., 2017) and suggests we do not yet have a complete understanding of the underlying carbon cycle dynamics on annual to decadal timescales. Resolving most of this unexplained variability should be possible through different and complementary approaches. First, as intended with our annual updates, the imbalance as an error term is reduced by improvements of individual components of the global carbon budget that follow from improving the underlying data and statistics and by improving the models through the resolution of some of the key uncertainties detailed in Table 9. Second, additional clues to the origin and processes responsible for the variability in the budget imbalance could be obtained through a closer scrutiny of carbon variability in light of other Earth system data (e.g. heat balance, water balance), and the use of a wider range of biogeochemical observations to better understand the land–ocean partitioning of the carbon imbalance (e.g. oxygen, carbon isotopes). Finally, additional information could also be obtained through higher resolution and process knowledge at the regional level, and through the introduction of inferred fluxes such as those based on satellite CO2 retrievals. The limit of the resolution of the carbon budget imbalance is yet unclear, but most certainly not yet reached given the possibilities for improvements that lie ahead.
Table 9Major known sources of uncertainties in each component of the Global Carbon Budget, defined as input data or processes that have a demonstrated effect of at least ±0.3 GtC yr−1.
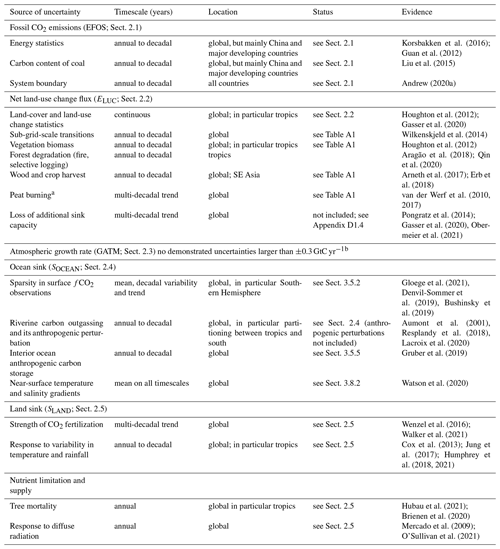
a As result of interactions between land use and climate. b The uncertainties in GATM have been estimated as ±0.2 GtC yr−1, although the conversion of the growth rate into a global annual flux assuming instantaneous mixing throughout the atmosphere introduces additional errors that have not yet been quantified.
Estimates of global fossil CO2 emissions from different datasets are in relatively good agreement when the different system boundaries of these datasets are considered (Andrew, 2020a). But while estimates of EFOS are derived from reported activity data requiring much fewer complex transformations than some other components of the budget, uncertainties remain, and one reason for the apparently low variation between datasets is precisely the reliance on the same underlying reported energy data. The budget excludes some sources of fossil CO2 emissions, which available evidence suggests are relatively small (< 1 %). We have added emissions from lime production in China and the US, but these are still absent in most other non-Annex I countries, and before 1990 in other Annex I countries. Further changes to EFOS this year are documented by Andrew and Peters (2021).
Estimates of ELUC suffer from a range of intertwined issues, including the poor quality of historical land-cover and land-use change maps, the rudimentary representation of management processes in most models, and the confusion in methodologies and boundary conditions used across methods (e.g. Arneth et al., 2017; Pongratz et al., 2014; see also Sect. 2.7.4 on the loss of sink capacity; Bastos et al., 2021). Uncertainties in current and historical carbon stocks in soils and vegetation also add uncertainty in the ELUC estimates. Unless a major effort to resolve these issues is made, little progress is expected in the resolution of ELUC. This is particularly concerning given the growing importance of ELUC for climate mitigation strategies, and the large issues in the quantification of the cumulative emissions over the historical period that arise from large uncertainties in ELUC.
By adding the DGVM estimates of CO2 fluxes due to environmental change from countries' managed forest areas (part of SLAND in this budget) to the budget ELUC estimate, we successfully reconciled the large gap between our ELUC estimate and the land-use flux from NGHGIs using the approach described in Grassi et al. (2021). This latter estimate has been used in the recent UNFCCC's Synthesis Report on Nationally Determined Contribution (UNFCCC, 2021b) to enable the total national emission estimates to be comparable with those of the IPCC. However, while Grassi et al. (2021) used only one DGVM, here 17 DGVMs are used, thus providing a more robust value to be used as potential adjustment in the policy context, e.g. to help assessing the collective countries' progress towards the goal of the Paris Agreement and avoiding double-accounting for the sink in managed forests. In the absence of this adjustment, collective progress would hence appear better than it is (Grassi et al., 2021).
The comparison of GOBMs, data products, and inversions highlights substantial discrepancy in the Southern Ocean (Fig. 12, Hauck et al., 2020). The long-standing sparse data coverage of fCO2 observations in the Southern compared to the Northern Hemisphere (e.g. Takahashi et al., 2009) continues to exist (Bakker et al., 2016, 2021, Fig. B1) and to lead to substantially higher uncertainty in the SOCEAN estimate for the Southern Hemisphere (Watson et al., 2020; Gloege et al., 2021). This discrepancy, which also hampers model improvement, points to the need for increased high-quality fCO2 observations, especially in the Southern Ocean. At the same time, model uncertainty is illustrated by the large spread of individual GOBM estimates (indicated by shading in Fig. 12) and highlights the need for model improvement. Further uncertainty stems from the regional distribution of the river flux adjustment term being based on one model study yielding the largest riverine outgassing flux south of 20∘ S (Aumont et al., 2001), with a recent study questioning this distribution (Lacroix et al., 2020). The diverging trends in SOCEAN from different methods is a matter of concern, which is unresolved. The assessment of the net land–atmosphere exchange from DGVMs and atmospheric inversions also shows substantial discrepancy, particularly for the estimate of the total land flux over the northern extra-tropics. This discrepancy highlights the difficulty of quantifying complex processes (CO2 fertilization, nitrogen deposition and fertilizers, climate change and variability, land management, etc.) that collectively determine the net land CO2 flux. Resolving the differences in the Northern Hemisphere land sink will require the consideration and inclusion of larger volumes of observations.
We provide metrics for the evaluation of the ocean and land models and the atmospheric inversions (Figs. B2 to B4). These metrics expand the use of observations in the global carbon budget, helping (1) to support improvements in the ocean and land carbon models that produce the sink estimates, and (2) to constrain the representation of key underlying processes in the models and to allocate the regional partitioning of the CO2 fluxes. However, GOBM skills have changed little since the introduction of the ocean model evaluation. An additional simulation this year allows for direct comparison with interior ocean anthropogenic carbon estimates and suggests that the models underestimate anthropogenic carbon uptake and storage. This is an initial step towards the introduction of a broader range of observations that we hope will support continued improvements in the annual estimates of the global carbon budget.
We assessed before that a sustained decrease of −1 % in global emissions could be detected at the 66 % likelihood level after a decade only (Peters et al., 2017). Similarly, a change in behaviour of the land and/or ocean carbon sink would take as long to detect, and much longer if it emerges more slowly. To continue reducing the carbon imbalance on annual to decadal timescales, regionalizing the carbon budget and integrating multiple variables are powerful ways to shorten the detection limit and ensure the research community can rapidly identify issues of concern in the evolution of the global carbon cycle under the current rapid and unprecedented changing environmental conditions.
The estimation of global CO2 emissions and sinks is a major effort by the carbon cycle research community that requires a careful compilation and synthesis of measurements, statistical estimates, and model results. The delivery of an annual carbon budget serves two purposes. First, there is a large demand for up-to-date information on the state of the anthropogenic perturbation of the climate system and its underpinning causes. A broad stakeholder community relies on the datasets associated with the annual carbon budget including scientists, policy makers, businesses, journalists, and non-governmental organizations engaged in adapting to and mitigating human-driven climate change. Second, over the last decades we have seen unprecedented changes in the human and biophysical environments (e.g. changes in the growth of fossil fuel emissions, impact of COVID-19 pandemic, Earth's warming, and strength of the carbon sinks), which call for frequent assessments of the state of the planet, a better quantification of the causes of changes in the contemporary global carbon cycle, and an improved capacity to anticipate its evolution in the future. Building this scientific understanding to meet the extraordinary climate mitigation challenge requires frequent, robust, transparent, and traceable datasets and methods that can be scrutinized and replicated. This paper via “living data” helps to keep track of new budget updates.
The data presented here are made available in the belief that their wide dissemination will lead to greater understanding and new scientific insights into how the carbon cycle works, how humans are altering it, and how we can mitigate the resulting human-driven climate change. Full contact details and information on how to cite the data shown here are given at the top of each page in the accompanying database and summarized in Table 2.
The accompanying database includes two Excel files organized in the following spreadsheets:
The file Global_Carbon_Budget_2021v1.0.xlsx includes the following:
-
summary;
-
the global carbon budget (1959–2020);
-
the historical global carbon budget (1750–2020);
-
global CO2 emissions from fossil fuels and cement production by fuel type, and the per capita emissions (1959–2020);
-
CO2 emissions from land-use change from the individual methods and models (1959–2020);
-
ocean CO2 sink from the individual ocean models and fCO2-based products (1959–2020);
-
terrestrial CO2 sink from the DGVMs (1959–2020).
The file National_Carbon_Emissions_2021v1.0.xlsx includes the following:
-
summary;
-
territorial country CO2 emissions from fossil CO2 emissions (1959–2020);
-
consumption country CO2 emissions from fossil CO2 emissions and emissions transfer from the international trade of goods and services (1990–2019) using CDIAC/UNFCCC data as reference;
-
emissions transfers (Consumption minus territorial emissions; 1990–2019);
-
country definitions;
-
details of disaggregated countries;
-
details of aggregated countries.
Both spreadsheets are published by the Integrated Carbon Observation System (ICOS) Carbon Portal and are available at https://doi.org/10.18160/gcp-2021 (Friedlingstein et al., 2021). National emissions data are also available from the Global Carbon Atlas (http://www.globalcarbonatlas.org/, last access: 11 March 2022) and from Our World in Data (https://ourworldindata.org/CO2-emissions, last access: 11 March 2022).
Table A1Comparison of the processes included in the bookkeeping method and DGVMs in their estimates of ELUC and SLAND. See Table 4 for model references. All models include deforestation and forest regrowth after abandonment of agriculture (or from afforestation activities on agricultural land). Processes relevant for ELUC are only described for the DGVMs used with land-cover change in this study. n/a – not applicable
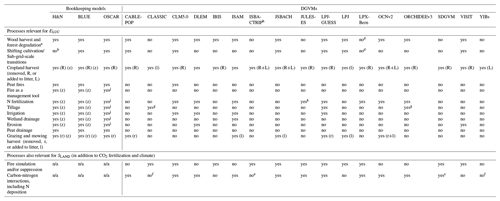
Table A2Comparison of the processes and model set-up for the global ocean biogeochemistry models for their estimates of SOCEAN. See Table 4 for model references. NA – not available
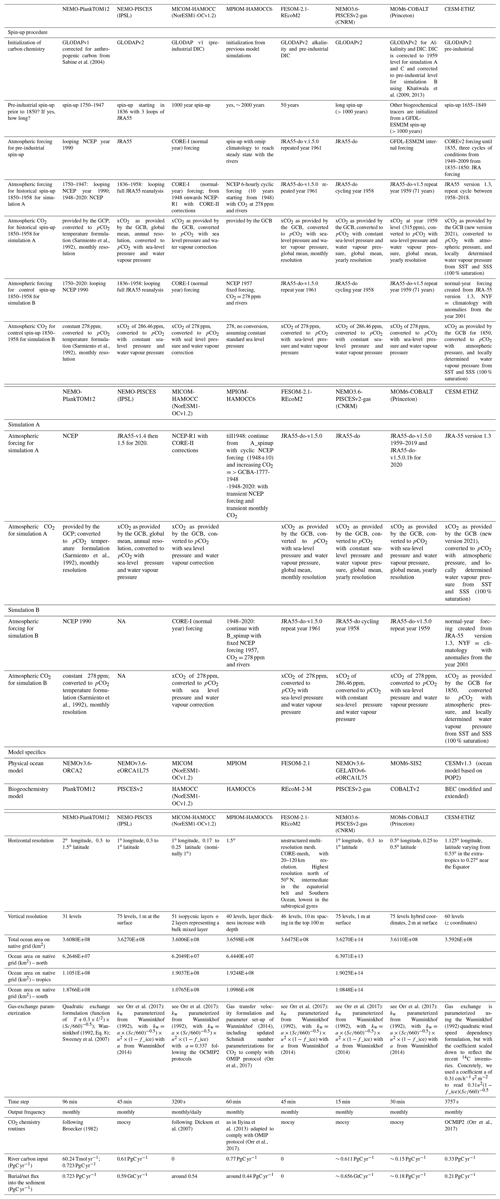
Table A3Description of ocean data products used for assessment of SOCEAN. See Table 4 for references.
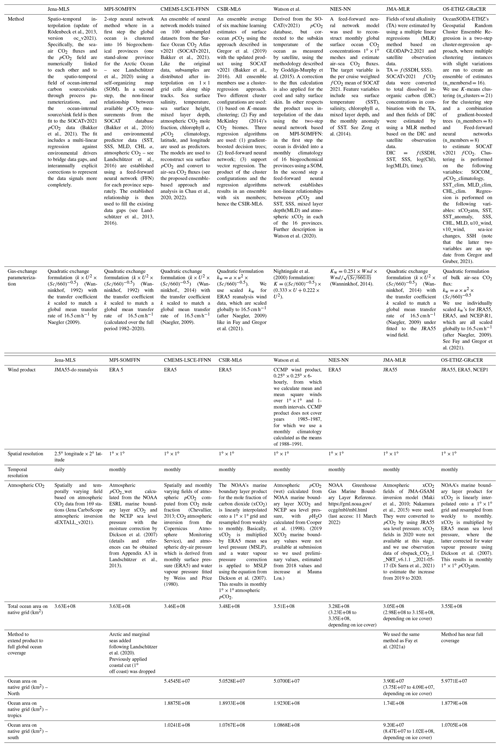
Table A4Comparison of the inversion set-up and input fields for the atmospheric inversions. Atmospheric inversions include the full CO2 fluxes, including the anthropogenic and pre-industrial fluxes. Hence they need to be adjusted for the pre-industrial flux of CO2 from the land to the ocean that is part of the natural carbon cycle before they can be compared with SOCEAN and SLAND from process models. See Table 4 for references.
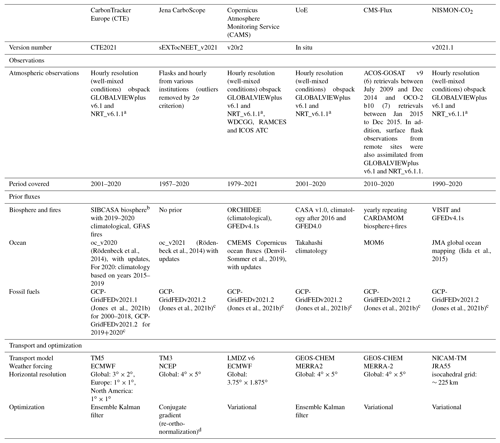
a Cox et al. (2021); Di Sarra et al. (2021). b van der Velde et al. (2014). c GCP-GridFEDv2021.2 (Jones et al., 2021b) is an update through the year 2020 of the GCP-GridFED dataset presented by Jones et al. (2021a). d Ocean prior not optimized.
Table A5Attribution of fCO2 measurements for the year 2020 included in SOCATv2021 (Bakker et al., 2016, 2021) to inform ocean fCO2-based data products.
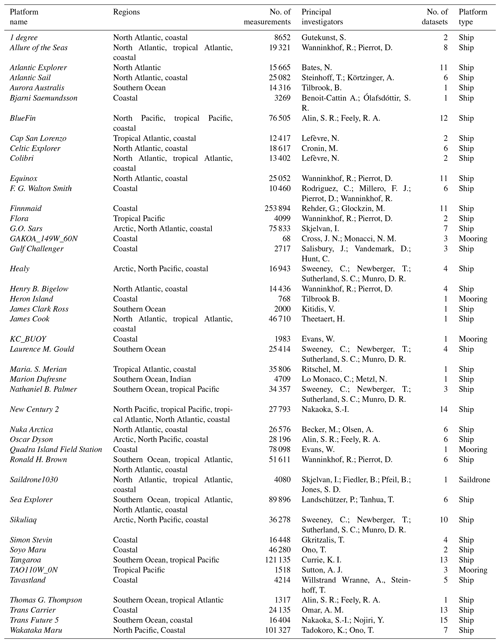
Table A6Aircraft measurement programmes archived by Cooperative Global Atmospheric Data Integration Project (CGADIP; Cox et al., 2021) that contribute to the evaluation of the atmospheric inversions (Fig. B4).
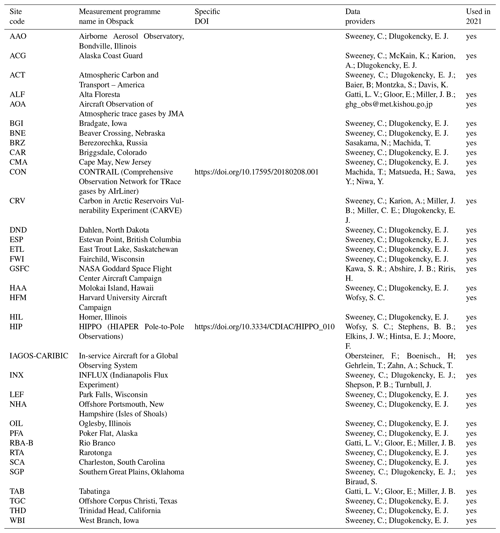
Table A7Main methodological changes in the global carbon budget since first publication. Methodological changes introduced in one year are kept for the following years unless noted. Empty cells mean there were no methodological changes introduced that year.
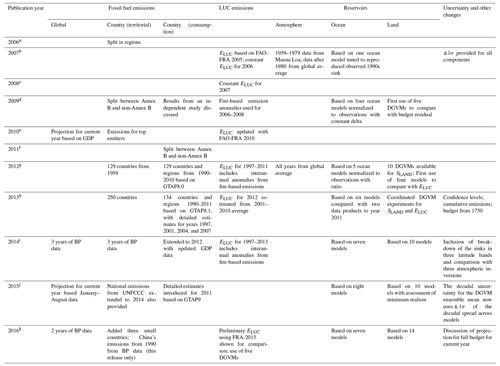
a Raupach et al. (2007). b Canadell et al. (2007). c GCP (2007). d Le Quéré et al. (2009). e Friedlingstein et al. (2010). f Peters et al. (2012b). g Le Quéré et al. (2013), Peters et al. (2013). h Le Quéré et al. (2014). i Le Quéré et al. (2015a). j Le Quéré et al. (2015b) k Le Quéré et al. (2016)
Table A8Mapping of global carbon cycle models' land flux definitions to the definition of the LULUCF net flux used in national reporting to UNFCCC. Non-intact lands are used here as proxy for “managed lands” in the country reporting.
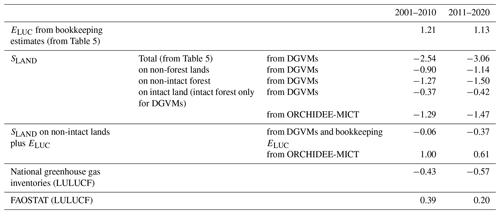
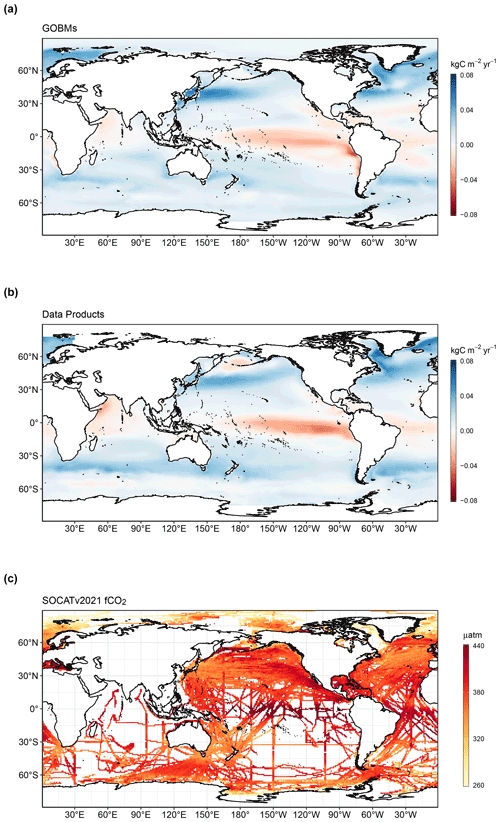
Figure B1Ensemble mean air–sea CO2 flux from (a) global ocean biogeochemistry models and (b) fCO2-based data products, averaged over 2011–2020 period (kgC m−2 yr−1). Positive numbers indicate a flux into the ocean. (c) Gridded SOCAT v2021 fCO2 measurements, averaged over the 2011–2020 period (µatm). In (a) model simulation A is shown. The data products represent the contemporary flux, i.e. including outgassing of riverine carbon, which is estimated to amount to 0.615 GtC yr−1.
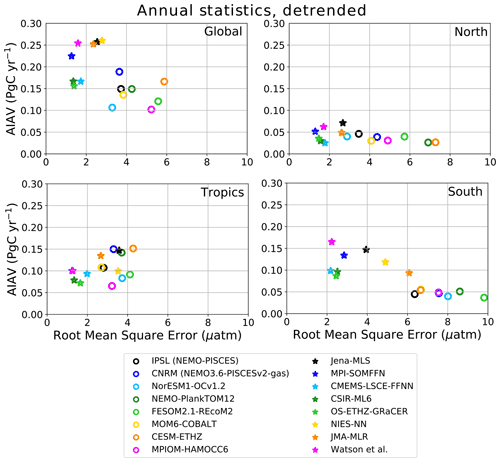
Figure B2Evaluation of the GOBMs and data products using the root mean squared error (RMSE) for the period 1990 to 2020, between the individual surface ocean fCO2 mapping schemes and the SOCAT v2021 database. The y axis shows the amplitude of the interannual variability (A-IAV, taken as the standard deviation of a detrended time series calculated as a 12-month running mean over the monthly flux time series, Rödenbeck et al., 2015). Results are presented for the globe, north (> 30∘ N), tropics (30∘ S–30∘ N), and south (< 30∘ S) for the GOBMs (see legend circles) and for the fCO2-based data products (star symbols). The fCO2-based data products use the SOCAT database and therefore are not independent from the data (see Sect. 2.4.1).
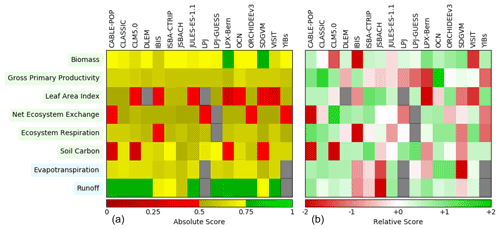
Figure B3Evaluation of the DGVMs using the International Land Model Benchmarking system (ILAMB; Collier et al., 2018) (a) absolute skill scores and (b) skill scores relative to other models. The benchmarking is done with observations for vegetation biomass (Saatchi et al., 2011; and GlobalCarbon unpublished data; Avitabile et al., 2016), GPP (Jung et al., 2010; Lasslop et al., 2010), leaf area index (De Kauwe et al., 2011; Myneni et al., 1997), net ecosystem exchange (Jung et al., 2010; Lasslop et al., 2010), ecosystem respiration (Jung et al., 2010; Lasslop et al., 2010), soil carbon (Hugelius et al., 2013; Todd-Brown et al., 2013), evapotranspiration (De Kauwe et al., 2011), and runoff (Dai and Trenberth, 2002). For each model–observation comparison a series of error metrics are calculated, scores are then calculated as an exponential function of each error metric, and finally for each variable the multiple scores from different metrics and observational datasets are combined to give the overall variable scores shown in (a). Overall variable scores increase from 0 to 1 with improvements in model performance. The set of error metrics vary with dataset and can include metrics based on the period mean, bias, root mean squared error, spatial distribution, interannual variability, and seasonal cycle. The relative skill score shown in (b) is a Z score, which indicates in units of standard deviation the model scores relative to the multi-model mean score for a given variable. Grey boxes represent missing model data.
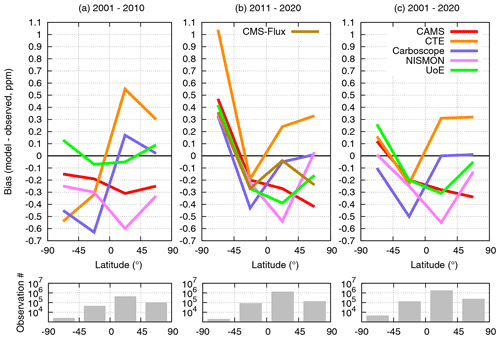
Figure B4Evaluation of the atmospheric inversion products. The mean of the model minus observations is shown for four latitude bands in three periods: (a) 2001–2010, (b) 2011–2020, (c) 2001–2020. The six models are compared to independent CO2 measurements made onboard aircraft over many places of the world between 2 and 7 km above sea level. Aircraft measurements archived in the Cooperative Global Atmospheric Data Integration Project (CGADIP; Cox et al., 2021) from sites, campaigns, or programmes that cover at least 9 months between 2001 and 2020 and that have not been assimilated have been used to compute the biases of the differences in four 45∘ latitude bins. Land and ocean data are used without distinction, and observation density varies strongly with latitude and time as seen in the lower panels.
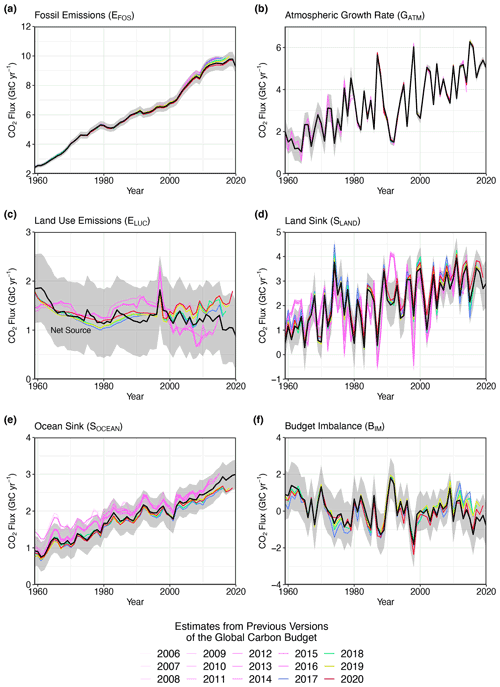
Figure B5Comparison of the estimates of each component of the global carbon budget in this study (black line) with the estimates released annually by the GCP since 2006. Grey shading shows the uncertainty bounds representing ±1 standard deviation of the current global carbon budget, based on the uncertainty assessments described in Appendix C. CO2 emissions from (a) fossil CO2 emissions (EFOS) and (b) land-use change (ELUC), as well as their partitioning among (c) the atmosphere (GATM), (d) the land (SLAND), and (e) the ocean (SOCEAN). See legend for the corresponding years, and Tables 3 and A7 for references. The budget year corresponds to the year when the budget was first released. All values are in GtC yr−1.
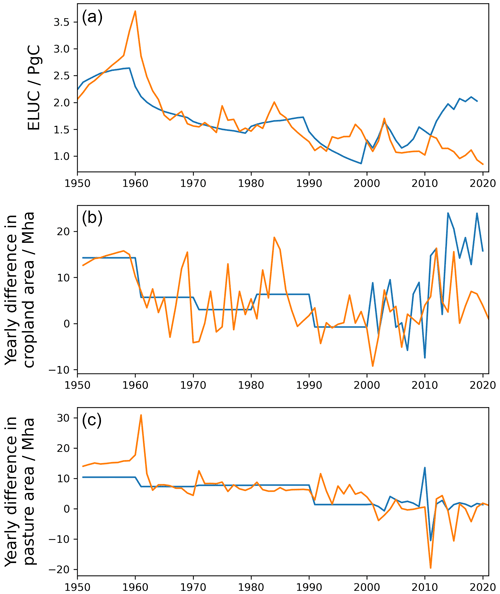
Figure B6Changes in the HYDE/LUH2 land-use forcing from last year's global carbon budget (Friedlingstein et al., 2020, in blue) to this year (orange). Shown are year-to-year changes in cropland area (b) and pasture area (c). To illustrate the relevance of the update in the land-use forcing to the recent trends in ELUC, (a) shows the land-use emission estimate from the bookkeeping model BLUE (original model output, i.e. excluding peat fire and drainage emissions).
C1 Methodology fossil fuel CO2 emissions (EFOS)
C1.1 Cement carbonation
From the moment it is created, cement begins to absorb CO2 from the atmosphere, a process known as “cement carbonation”. We estimate this CO2 sink, as the average of two studies in the literature (Cao et al., 2020; Guo et al., 2021). Both studies use the same model, developed by Xi et al. (2016), with different parameterizations and input data, with the estimate of Guo and colleagues being a revision of Xi et al. (2016). The trends of the two studies are very similar. Modelling cement carbonation requires estimation of a large number of parameters, including the different types of cement material in different countries, the lifetime of the structures before demolition, of cement waste after demolition, and the volumetric properties of structures, among others (Xi et al., 2016). Lifetime is an important parameter because demolition results in the exposure of new surfaces to the carbonation process. The main reasons for differences between the two studies appear to be the assumed lifetimes of cement structures and the geographic resolution, but the uncertainty bounds of the two studies overlap. In the present budget, we include the cement carbonation carbon sink in the fossil CO2 emission component (EFOS).
C1.2 Emissions embodied in goods and services
CDIAC, UNFCCC, and BP national emission statistics “include greenhouse gas emissions and removals taking place within national territory and offshore areas over which the country has jurisdiction” (Rypdal et al., 2006) and are called territorial emission inventories. Consumption-based emission inventories allocate emissions to products that are consumed within a country and are conceptually calculated as the territorial emissions minus the “embodied” territorial emissions to produce exported products plus the emissions in other countries to produce imported products (consumption = territorial – exports + imports). Consumption-based emission attribution results (e.g. Davis and Caldeira, 2010) provide additional information to territorial-based emissions that can be used to understand emission drivers (Hertwich and Peters, 2009) and quantify emission transfers by the trade of products between countries (Peters et al., 2011b). The consumption-based emissions have the same global total but reflect the trade-driven movement of emissions across the Earth's surface in response to human activities. We estimate consumption-based emissions from 1990–2018 by enumerating the global supply chain using a global model of the economic relationships between economic sectors within and between every country (Andrew and Peters, 2013; Peters et al., 2011a). Our analysis is based on the economic and trade data from the Global Trade and Analysis Project (GTAP; Narayanan et al., 2015), and we make detailed estimates for the years 1997 (GTAP version 5), 2001 (GTAP6), 2004, 2007, and 2011 (GTAP9.2), covering 57 sectors and 141 countries and regions. The detailed results are then extended into an annual time series from 1990 to the latest year of the gross domestic product (GDP) data (2018 in this budget), using GDP data by expenditure in the current exchange rate of US dollars (USD; from the UN National Accounts Main Aggregrates Database; UN, 2021) and time series of trade data from GTAP (based on the methodology in Peters et al., 2011a). We estimate the sector-level CO2 emissions using the GTAP data and methodology, include the flaring and cement emissions from CDIAC, and then scale the national totals (excluding bunker fuels) to match the emission estimates from the carbon budget. We do not provide a separate uncertainty estimate for the consumption-based emissions, but based on model comparisons and sensitivity analysis, they are unlikely to be significantly different than for the territorial emission estimates (Peters et al., 2012a).
C1.3 Uncertainty assessment for EFOS
We estimate the uncertainty of the global fossil CO2 emissions at ±5 % (scaled down from the published ±10 % at ±2σ to the use of ±1σ bounds reported here; Andres et al., 2012). This is consistent with a more detailed analysis of uncertainty of ±8.4 % at ±2σ (Andres et al., 2014) and at the high-end of the range of ±5 %–10 % at ±2σ reported by Ballantyne et al. (2015). This includes an assessment of uncertainties in the amounts of fuel consumed, the carbon and heat contents of fuels, and the combustion efficiency. While we consider a fixed uncertainty of ±5 % for all years, the uncertainty as a percentage of emissions is growing with time because of the larger share of global emissions from emerging economies and developing countries (Marland et al., 2009). Generally, emissions from mature economies with good statistical processes have an uncertainty of only a few per cent (Marland, 2008), while emissions from strongly developing economies such as China have uncertainties of around ±10 % (for ±1σ; Gregg et al., 2008; Andres et al., 2014). Uncertainties of emissions are likely to be mainly systematic errors related to underlying biases of energy statistics and to the accounting method used by each country.
C1.4 Growth rate in emissions
We report the annual growth rate in emissions for adjacent years (in percent per year) by calculating the difference between the two years and then normalizing to the emissions in the first year: (EFOS(t0+1) − EFOS(t0))(t0) ×100 %. We apply a leap-year adjustment where relevant to ensure valid interpretations of annual growth rates. This affects the growth rate by about 0.3 % yr−1 () and causes calculated growth rates to go up approximately 0.3 % if the first year is a leap year and down 0.3 % if the second year is a leap year. The relative growth rate of EFOS over time periods of greater than 1 year can be rewritten using its logarithm equivalent as follows:
Here we calculate relative growth rates in emissions for multi-year periods (e.g. a decade) by fitting a linear trend to ln(EFOS) in Eq. (2), reported in percent per year.
C1.5 Emissions projection for EFOS
To gain insight into emission trends for 2021, we provide an assessment of global fossil CO2 emissions, EFOS, by combining individual assessments of emissions for China, USA, the EU, and India (the four countries and regions with the largest emissions), and the rest of the world. We provide full year estimates for two datasets: IEA (2021b) and our own analysis. This approach differs from last year where we used four independent estimates including our own, because of the unique circumstances related to the COVID-19 pandemic. This year's analysis is more in line with earlier budgets.
Previous editions of the Global Carbon Budget (GCB) have estimated year-to-date (YTD) emissions, and performed projections, using sub-annual energy consumption data from a variety of sources depending on the country or region. The YTD estimates have then been projected to the full year using specific methods for each country or region. The methods described in detail below.
China
We use the growth in total fossil CO2 emissions in 2021 reported by the National Bureau of Statistics (NBS) in their 2022 Statistical Communique (NBS, 2022). This report includes growth rates of energy consumption for coal, oil, and natural gas as well as the growth in cement production, which are used to determine the changes in emissions from these four categories.
USA
We use emissions estimated by the U.S. Energy Information Administration (EIA) in their Short-Term Energy Outlook (STEO) for emissions from fossil fuels to get both a YTD and a full-year projection (EIA, 2022). The STEO also includes a near-term forecast based on an energy forecasting model which is updated monthly (last update with preliminary data through September 2021) and takes into account expected temperatures, household expenditures by fuel type, energy markets, policies, and other effects. We combine this with our estimate of emissions from cement production using the monthly U.S. cement clinker production data from USGS for January–June 2021, assuming changes in cement production over the first part of the year apply throughout the year.
India
We use monthly emissions estimates for India updated from Andrew (2020b) through August 2021. These estimates are derived from many official monthly energy and other activity data sources to produce direct estimates of national CO2 emissions, without the use of proxies. Emissions from coal are then extended to September using a regression relationship based on power generated from coal, coal dispatches by Coal India Ltd., the composite PMI, time, and days per month. For the last 3–4 months of the year, each series is extrapolated assuming typical trends.
EU
We use a refinement to the methods presented by Andrew (2021), deriving emissions from monthly energy data reported by Eurostat. Some data gaps are filled using data from the Joint Organisations Data Initiative (JODI, 2022). Sub-annual cement production data are limited, but data for Germany and Poland, the two largest producers, suggest a small decline. For fossil fuels this provides estimates through July. We extend coal emissions through September using a regression model built from generation of power from hard coal, power from brown coal, total power generation, and the number of working days in Germany and Poland, the two biggest coal consumers in the EU. These are then extended through the end of the year assuming typical trends. We extend oil emissions by building a regression model between our monthly CO2 estimates and oil consumption reported by the EIA for Europe in its Short-Term Energy Outlook (October edition), and then using this model with EIA's monthly forecasts. For natural gas, the strong seasonal signal allows the use of the bias-adjusted Holt–Winters exponential smoothing method (Chatfield, 1978).
Rest of the world
We use the close relationship between the growth in GDP and the growth in emissions (Raupach et al., 2007) to project emissions for the current year. This is based on a simplified Kaya identity, whereby EFOS (GtC yr−1) is decomposed by the product of GDP (USD yr−1) and the fossil fuel carbon intensity of the economy (IFOS; GtC USD−1) as follows:
Taking a time derivative of Eq. (3) and rearranging gives the following:
where the left-hand term is the relative growth rate of EFOS, and the right-hand terms are the relative growth rates of GDP and IFOS, respectively, which can simply be added linearly to give the overall growth rate.
The IFOS is based on GDP in constant PPP (purchasing power parity) from the International Energy Agency (IEA) up to 2017 (IEA/OECD, 2019) and extended using the International Monetary Fund (IMF) growth rates through 2020 (IMF, 2022). Interannual variability in IFOS is the largest source of uncertainty in the GDP-based emissions projections. We thus use the standard deviation of the annual IFOS for the period 2009–2019 as a measure of uncertainty, reflecting a ±1σ as in the rest of the carbon budget.
World
The global total is the sum of each of the countries and regions.
C2 Methodology CO2 emissions from land use, land-use change, and forestry (ELUC)
The net CO2 flux from land use, land-use change, and forestry (ELUC, called land-use change emissions in the rest of the text) includes CO2 fluxes from deforestation, afforestation, logging and forest degradation (including harvest activity), shifting cultivation (cycle of cutting forest for agriculture, then abandoning), and regrowth of forests following wood harvest or abandonment of agriculture. Emissions from peat burning and drainage are added from external datasets (see Sect. C2.1 below). Only some land-management activities are included in our land-use change emissions estimates (Table A1). Some of these activities lead to emissions of CO2 to the atmosphere, while others lead to CO2 sinks. ELUC is the net sum of emissions and removals due to all anthropogenic activities considered. Our annual estimate for 1960–2020 is provided as the average of results from three bookkeeping approaches (Sect. C2.1 below): an estimate using the Bookkeeping of Land Use Emissions model (Hansis et al., 2015; hereafter BLUE) and one using the compact Earth system model OSCAR (Gasser et al., 2020), both BLUE and OSCAR being updated here to new land-use forcing covering the time period until 2020, and an updated version of the estimate published by Houghton and Nassikas (2017) (hereafter updated H&N2017). All three datasets are then extrapolated to provide a projection for 2021 (Sect. C2.5 below). In addition, we use results from dynamic global vegetation models (DGVMs; see Sect. 2.5 and Table 4) to help quantify the uncertainty in ELUC (Sect. C2.4), and thus better characterize our understanding. Note that in this budget, we use the scientific ELUC definition, which counts fluxes due to environmental changes on managed land towards SLAND, as opposed to the national greenhouse gas inventories under the UNFCCC, which include them in ELUC and thus often report smaller land-use emissions (Grassi et al., 2018; Petrescu et al., 2020). However, we provide a methodology of mapping of the two approaches to each other further below (Sect. C2.3).
C2.1 Bookkeeping models
Land-use change CO2 emissions and uptake fluxes are calculated by three bookkeeping models. These are based on the original bookkeeping approach of Houghton (2003) that keeps track of the carbon stored in vegetation and soils before and after a land-use change (transitions between various natural vegetation types, croplands, and pastures). Literature-based response curves describe decay of vegetation and soil carbon, including transfer to product pools of different lifetimes, as well as carbon uptake due to regrowth. In addition, the bookkeeping models represent long-term degradation of primary forest as lowered standing vegetation and soil carbon stocks in secondary forests and include forest management practices such as wood harvests.
BLUE and the updated H&N2017 exclude land ecosystems' transient response to changes in climate, atmospheric CO2, and other environmental factors and base the carbon densities on contemporary data from literature and inventory data. Since carbon densities thus remain fixed over time, the additional sink capacity that ecosystems provide in response to CO2 fertilization and some other environmental changes is not captured by these models (Pongratz et al., 2014). On the contrary, OSCAR includes this transient response, and it follows a theoretical framework (Gasser and Ciais, 2013) that allows the separation of bookkeeping land-use emissions and the loss of additional sink capacity. Only the former is included here, while the latter is discussed in Appendix D4. The bookkeeping models differ in (1) computational units (spatially explicit treatment of land-use change for BLUE, regional- or mostly country-level for the updated H&N2017 and OSCAR), (2) processes represented (see Table A1), and (3) carbon densities assigned to vegetation and soil of each vegetation type (literature-based for the updated H&N2017 and BLUE, calibrated to DGVMs for OSCAR). A notable difference between models exists with respect to the treatment of shifting cultivation. The update of H&N2017 changed the approach over the earlier H&N2017 version: H&N2017 had assumed the “excess loss” of tropical forests (i.e. when FRA indicated a forest loss larger than the increase in agricultural areas from FAO) resulted from converting forests to croplands at the same time older croplands were abandoned. Those abandoned croplands began to recover to forests after 15 years. The updated H&N2017 now assumes that forest loss in excess of increases in cropland and pastures represented an increase in shifting cultivation. When the excess loss of forests was negative, it was assumed that shifting cultivation was returned to forest. Historical areas in shifting cultivation were extrapolated taking into account country-based estimates of areas lying fallow in 1980 (FAO/UNEP, 1981) and expert opinion (from Heinimann et al., 2017). In contrast, the BLUE and OSCAR models include sub-grid-scale transitions between all vegetation types. Furthermore, the updated H&N2017 assume conversion of natural grasslands to pasture, while BLUE and OSCAR allocate pasture proportionally on all natural vegetation that exists in a grid cell. This is one reason for generally higher emissions in BLUE and OSCAR. Bookkeeping models do not directly capture carbon emissions from peat fires, which can create large emissions and interannual variability due to synergies of land-use and climate variability in Southeast Asia, particularly during El-Niño events, nor emissions from the organic layers of drained peat soils. To correct for this, the updated H&N2017 includes carbon emissions from burning and draining of peatlands in Indonesia, Malaysia, and Papua New Guinea (based on the Global Fire Emission Database, GFED4s; van der Werf et al., 2017, for fire and Hooijer et al., 2010, for drainage). Further, estimates of carbon losses from peatlands in extra-tropical regions are added from Qiu et al. (2021). We add GFED4s peat fire emissions to BLUE and OSCAR output as well as the global FAO peat drainage emissions 1990–2018 from croplands and grasslands (Conchedda and Tubiello, 2020), keeping post-2018 emissions constant. We linearly increase tropical drainage emissions from 0 in 1980, consistent with H&N2017's assumption, and keep emissions from the often old drained areas of the extra-tropics constant pre-1990. This adds 9.0 GtC for FAO compared to 5.6 GtC for Hooijer et al. (2010). Peat fires add another 2.0 GtC over the same period.
The three bookkeeping estimates used in this study differ with respect to the land-use change data used to drive the models. The updated H&N2017 base their estimates directly on the Forest Resource Assessment of the FAO which provides statistics on forest-area change and management at intervals of 5 years currently updated until 2020 (FAO, 2020). The data are based on country reporting to FAO and may include remote-sensing information in more recent assessments. Changes in land use other than forests are based on annual, national changes in cropland and pasture areas reported by FAO (FAOSTAT, 2021). On the other hand, BLUE uses the harmonized land-use change data LUH2-GCB2021 covering the entire 850–2020 period (an update to the previously released LUH2 v2h dataset; Hurtt et al., 2017, 2020), which was also used as input to the DGVMs (Sect. C2.2). It describes land-use change, also based on the FAO data as described in Sect. C2.2 as well as the HYDE3.3 dataset (Klein Goldewijk et al., 2017a, b), but provided at a quarter-degree spatial resolution, considering sub-grid-scale transitions between primary forest, secondary forest, primary non-forest, secondary non-forest, cropland, pasture, rangeland, and urban land (Hurtt et al., 2020; Chini et al., 2021). LUH2-GCB2021 provides a distinction between rangelands and pasture, based on inputs from HYDE. To constrain the models' interpretation on whether rangeland implies the original natural vegetation to be transformed to grassland or not (e.g. browsing on shrubland), a forest mask was provided with LUH2-GCB2021; forest is assumed to be transformed to grasslands, while other natural vegetation remains (in case of secondary vegetation) or is degraded from primary to secondary vegetation (Ma et al., 2020). This is implemented in BLUE. OSCAR was run with both LUH2-GCB2021 and FAO/FRA (as used by Houghton and Nassikas, 2017), where emissions from the latter were extended beyond 2015 with constant 2011–2015 average values. The best-guess OSCAR estimate used in our study is a combination of results for LUH2-GCB2021 and FAO/FRA land-use data and a large number of perturbed parameter simulations weighted against an observational constraint. All three bookkeeping estimates were extended from 2020 to provide a projection for 2021 by adding the annual change in emissions from tropical deforestation and degradation and peat burning and drainage to the respective model's estimate for 2020 (van der Werf et al., 2017; Conchedda and Tubiello, 2020).
For ELUC from 1850 onwards we average the estimates from BLUE, the updated H&N2017 and OSCAR. For the cumulative numbers starting 1750 an average of four earlier publications is added (30 ± 20 PgC 1750–1850, rounded to nearest 5; Le Quéré et al., 2016).
We provide estimates of the gross land-use change fluxes from which the reported net land-use change flux, ELUC, is derived as a sum. Gross fluxes are derived internally by the three bookkeeping models: gross emissions stem from decaying material left dead on site and from products after clearing of natural vegetation for agricultural purposes, wood harvesting, emissions from peat drainage and peat burning, and, for BLUE, additionally from degradation from primary to secondary land through usage of natural vegetation as rangeland. Gross removals stem from regrowth after agricultural abandonment and wood harvesting. Gross fluxes for the updated H&N2017 2016–2020 and for the 2021 projection of all three models were based on a regression of gross sources (including peat emissions) to net emissions for recent years.
Due to an artefact in the HYDE3.3 dataset expressed as an abrupt shift in the pattern of pastures and rangelands in 1960, the year 1960 exhibits much larger gross transitions between natural vegetation and pastures and rangelands than prior and subsequent years. Although these gross transitions cancel out in terms of net area changes causing large abrupt transitions, an unrealistic peak in emissions occurs around 1960 in BLUE and OSCAR. To correct for this, we replace the estimates for 1959–1961 by the average of 1958 and 1962 in both BLUE and OSCAR. Abrupt transitions will immediately influence gross emissions, which have a larger instantaneous component. Processes with longer timescales, such as slow legacy emissions and regrowth, are inseparable from the carbon dynamics due to subsequent land-use change events. We therefore do not adjust gross removals, but only gross emissions to match the corrected net flux. Since DGVM estimates are only used for an uncertainty range of ELUC, which is independent of land-use changes, no correction is applied to the DGVM data.
C2.2 Dynamic global vegetation models (DGVMs)
Land-use change CO2 emissions have also been estimated using an ensemble of 17 DGVM simulations. The DGVMs account for deforestation and regrowth, the most important components of ELUC, but they do not represent all processes resulting directly from human activities on land (Table A1). All DGVMs represent processes of vegetation growth and mortality, as well as decomposition of dead organic matter associated with natural cycles, and include the vegetation and soil carbon response to increasing atmospheric CO2 concentration and to climate variability and change. Most models explicitly simulate the coupling of carbon and nitrogen cycles and account for atmospheric N deposition and N fertilizers (Table A1). The DGVMs are independent from the other budget terms except for their use of atmospheric CO2 concentration to calculate the fertilization effect of CO2 on plant photosynthesis.
DGVMs that do not simulate sub-grid-scale transitions (i.e. net land-use emissions; see Table A1) used the HYDE land-use change dataset (Klein Goldewijk et al., 2017a, b), which provides annual (1700–2019), half-degree, fractional data on cropland and pasture. The data are based on the available annual FAO statistics of change in agricultural land area available until 2015. The new HYDE3.3 cropland and grazing land dataset which now in addition to having FAO country-level statistics is constrained spatially based on multi-year satellite land-cover maps from ESA CCI LC. Data from HYDE3.3 are based on a FAO which includes yearly data from 1961 up to and including the year 2017. After the year 2017 HYDE extrapolates the cropland, pasture, and urban data linearly based on the trend over the previous 5 years, to generate data until the year 2020. HYDE also uses satellite imagery from ESA-CCI from 1992–2018 for more detailed yearly allocation of cropland and grazing land, with the ESA area data scaled to match the FAO annual totals at country level. The 2018 map is also used for the 2019–2020 period. The original 300 m resolution data from ESA were aggregated to a 5 arcmin resolution according to the classification scheme as described in Klein Goldewijk et al. (2017a). DGVMs that simulate sub-grid-scale transitions (i.e. gross land-use emissions; see Table A1) also use the LUH2-GCB2021 dataset, an update of the more comprehensive harmonized land-use dataset (Hurtt et al., 2020), that further includes fractional data on primary and secondary forest vegetation, as well as all underlying transitions between land-use states (850–2020; Hurtt et al., 2011, 2017, 2020; Chini et al., 2021; Table A1). This new dataset is of quarter-degree fractional areas of land-use states and all transitions between those states, including a new wood harvest reconstruction and new representation of shifting cultivation, crop rotations, and management information including irrigation and fertilizer application. The land-use states include five different crop types in addition to the pasture–rangeland split discussed before. Wood harvest patterns are constrained with Landsat-based tree cover loss data (Hansen et al., 2013). Updates of LUH2-GCB2021 over last year's version (LUH2-GCB2020) are using the most recent HYDE/FAO release (covering the time period up to 2021 included). We also use the most recent FAO wood harvest data for all years from 1961 to 2019. After the year 2019 we extrapolated the wood harvest data until the year 2020. The HYDE3.3 population data are also used to extend the wood harvest time series back in time. Other wood harvest inputs (for years prior to 1961) remain the same in LUH2. With the switch from HYDE3.2 to HYDE3.3 changes in the land-use forcing compared to the version used in the GCB2020 (Friedlingstein et al., 2020) are pronounced. They are thus compared in Fig. 6b and their relevance for land-use emissions discussed in Sect. 3.4.2. DGVMs implement land-use change differently (e.g. an increased cropland fraction in a grid cell can either be at the expense of grassland or shrubs, or forest, the latter resulting in deforestation; land-cover fractions of the non-agricultural land differ between models). Similarly, model-specific assumptions are applied to convert deforested biomass or deforested area, and other forest product pools into carbon, and different choices are made regarding the allocation of rangelands as natural vegetation or pastures.
The difference between two DGVM simulations (see Sect. C4.1 below), one forced with historical changes in land-use and a second with time-invariant pre-industrial land cover and pre-industrial wood harvest rates, allows quantification of the dynamic evolution of vegetation biomass and soil carbon pools in response to land-use change in each model (ELUC). Using the difference between these two DGVM simulations to diagnose ELUC means the DGVMs account for the loss of additional sink capacity (around 0.4 ± 0.3 GtC yr−1; see Sect. 2.7.4, Appendix D4), while the bookkeeping models do not.
As a criterion for inclusion in this carbon budget, we only retain models that simulate a positive ELUC during the 1990s, as assessed in the IPCC AR4 (Denman et al., 2007) and AR5 (Ciais et al., 2013). All DGVMs met this criterion, although one model was not included in the ELUC estimate from DGVMs as it exhibited a spurious response to the transient land-cover change forcing after its initial spin-up.
C2.3 Mapping of national GHG inventory data to ELUC
An approach was implemented to reconcile the large gap between ELUC from bookkeeping models and land use, land-use change, and forestry (LULUCF) from national GHG inventories (NGHGIs) (see Table A8). This gap is due to different approaches to calculating “anthropogenic” CO2 fluxes related to land-use change and land management (Grassi et al., 2018). In particular, the land sinks due to environmental change on managed lands are treated as non-anthropogenic in the global carbon budget, while they are generally considered as anthropogenic in NGHGIs (“indirect anthropogenic fluxes”; Eggleston et al., 2006). Building on previous studies (Grassi et al., 2021), the approach implemented here adds the DGVM estimates of CO2 fluxes due to environmental change from countries' managed forest area (part of the SLAND) to the original ELUC flux. This sum is expected to be conceptually more comparable to LULUCF than simply ELUC.
ELUC data are taken from bookkeeping models, in line with the global carbon budget approach. To determine SLAND on managed forest, the following steps were taken: Spatially gridded data of “natural” forest NBP (net biome productivity) (SLAND, i.e. due to environmental change and excluding land-use change fluxes) were obtained with S2 runs from DGVMs up to 2019 from the TRENDY v9 dataset. Results were first masked with the Hansen forest map (Hansen et al., 2013), with a 20 % tree cover and following the FAO definition of forest (isolated pixels with maximum connectivity less than 0.5 ha are excluded), and then further masked with the “intact” forest map for the year 2013, i.e. forest areas characterized by no remotely detected signs of human activity (Potapov et al., 2017). This way, we obtained the SLAND in “intact” and “non-intact” forest area, which previous studies (Grassi et al., 2021) indicated to be a good proxy, respectively, for “unmanaged” and “managed” forest area in the NGHGI. Note that only four models (CABLE-POP, CLASSIC, YIBs and ORCHIDEE-CNP) had forest NBP at grid cell level. Two models (OCN and ISBA-CTRIP) provided forest NEP and simulated disturbances at pixel level that were used as basis, in addition to forest cover fraction, to estimate forest NBP. For the other DGVMs, when a grid cell had forest, all the NBP was allocated to forest.
LULUCF data from NGHGIs are from Grassi et al. (2021) until 2017, updated until 2019 for UNFCCC Annex I countries. For non-Annex I countries, the years 2018 and 2019 were assumed to be equal to the average 2013–2017. These data include all CO2 fluxes from land considered managed, which in principle encompasses all land uses (forest land, cropland, grassland, wetlands, settlements, and other land), changes among them, and emissions from organic soils and from fires. In practice, although almost all Annex I countries report all land uses, many non-Annex I countries report only on deforestation and forest land, and few countries report on other land uses. In most cases, NGHGIs include most of the natural response to recent environmental change, because they use direct observations (e.g. national forest inventories) that do not allow the separation of direct and indirect anthropogenic effects (Eggleston et al., 2006).
To provide additional, largely independent assessments of fluxes on unmanaged vs. managed lands, we include a DGVM that allows diagnosis of fluxes from unmanaged vs. managed lands by tracking vegetation cohorts of different ages separately. This model, ORCHIDEE-MICT (Yue et al., 2018), was run using the same LUH2 forcing as the DGVMs used in this budget (Sect. 2.5) and the bookkeeping models BLUE and OSCAR (Sect. 2.2). Old-aged forest was classified as primary forest after a certain threshold of carbon density was reached again, and the model-internal distinction between primary and secondary forest used as proxies for unmanaged vs. managed forests; agricultural lands are added to the latter to arrive at total managed land.
Table A8 shows the resulting mapping of global carbon cycle models' land flux definitions to that of the NGHGI (discussed in Sect. 3.2.2). ORCHIDEE-MICT estimates for SLAND on intact forests are expected to be higher than those based on DGVMs in combination with the NGHGI managed and unmanaged forest data because the unmanaged forest area, with about 27 mio km2, is estimated to be substantially larger by ORCHIDEE-MICT than that, with less than 10 mio km2, by the NGHGI, while managed forest area is estimated to be smaller (22 compared to 32 mio km2). Related to this, SLAND on non-intact lands plus ELUC is a larger source estimated by ORCHIDEE-MICT compared to the NGHGI. We also show as comparison FAOSTAT emissions totals (FAO, 2021), which include emissions from net forest conversion and fluxes on forest land (Tubiello et al., 2021) as well as CO2 emissions from peat drainage and peat fires.
C2.4 Uncertainty assessment for ELUC
Differences between the bookkeeping models and DGVMs originate from three main sources: the different methodologies, which among others lead to inclusion of the loss of additional sink capacity in DGVMs (see Appendix D1.4), the underlying land-use/land-cover dataset, and the different processes represented (Table A1). We examine the results from the DGVMs and of the bookkeeping method and use the resulting variations as a way to characterize the uncertainty in ELUC.
Despite these differences, the ELUC estimate from the DGVMs multi-model mean is consistent with the average of the emissions from the bookkeeping models (Table 5). However, there are large differences among individual DGVMs (standard deviation at around 0.5 GtC yr−1; Table 5), between the bookkeeping estimates (average difference 1850–2020 BLUE-updated H&N2017 of 0.8 GtC yr−1, BLUE-OSCAR of 0.4 GtC yr−1, OSCAR-updated H&N2017 of 0.3 GtC yr−1), and between the updated estimate of H&N2017 and its previous model version (Houghton et al., 2012). A factorial analysis of differences between BLUE and H&N2017 attributed them particularly to differences in carbon densities between natural and managed vegetation or primary and secondary vegetation (Bastos et al., 2021). Earlier studies additionally showed the relevance of the different land-use forcing as applied (in updated versions) also in the current study (Gasser et al., 2020).
The uncertainty in ELUC of ±0.7 GtC yr−1 reflects our best value judgement that there is at least 68 % chance (±1σ) that the true land-use change emission lies within the given range, for the range of processes considered here. Prior to the year 1959, the uncertainty in ELUC was taken from the standard deviation of the DGVMs. We assign low confidence to the annual estimates of ELUC because of the inconsistencies among estimates and of the difficulties of quantifying some of the processes in DGVMs.
C2.5 Emissions projections for ELUC
We project the 2021 land-use emissions for BLUE, the updated H&N2017, and OSCAR, starting from their estimates for 2020 assuming unaltered peat drainage, which has low interannual variability, and the highly variable emissions from peat fires, tropical deforestation, and degradation as estimated using active fire data (MCD14ML; Giglio et al., 2016). The latter scale almost linearly with GFED over large areas (van der Werf et al., 2017) and thus allow for tracking fire emissions in deforestation and tropical peat zones in near-real time.
C3 Methodology ocean CO2 sink (SOCEAN)
C3.1 Observation-based estimates
We primarily use the observational constraints assessed by IPCC of a mean ocean CO2 sink of 2.2 ± 0.7 GtC yr−1 for the 1990s (90 % confidence interval; Ciais et al., 2013) to verify that the GOBMs provide a realistic assessment of SOCEAN. This is based on indirect observations with seven different methodologies and their uncertainties, using the methods that are deemed most reliable for the assessment of this quantity (Denman et al., 2007; Ciais et al., 2013). The observation-based estimates use the ocean–land CO2 sink partitioning from observed atmospheric CO2 and O2 N2 concentration trends (Manning and Keeling, 2006; Keeling and Manning, 2014), an oceanic inversion method constrained by ocean biogeochemistry data (Mikaloff Fletcher et al., 2006), and a method based on penetration timescale for chlorofluorocarbons (McNeil et al., 2003). The IPCC estimate of 2.2 GtC yr−1 for the 1990s is consistent with a range of methods (Wanninkhof et al., 2013). We refrain from using the IPCC estimates for the 2000s (2.3 ± 0.7 GtC yr−1), and the period 2002–2011 (2.4 ± 0.7 GtC yr−1, Ciais et al., 2013) as these are based on trends derived mainly from models and one data product (Ciais et al., 2013). Additional constraints summarized in AR6 (Canadell et al., 2022) are the interior ocean anthropogenic carbon change (Gruber et al., 2019) and ocean sink estimate from atmospheric CO2 and O2 N2 (Tohjima et al., 2019) which are used for model evaluation and discussion, respectively.
We also use eight estimates of the ocean CO2 sink and its variability based on surface ocean fCO2 maps obtained by the interpolation of surface ocean fCO2 measurements from 1990 onwards due to severe restriction in data availability prior to 1990 (Fig. 9). These estimates differ in many respects: they use different maps of surface fCO2, different atmospheric CO2 concentrations, wind products and different gas-exchange formulations as specified in Table A3. We refer to them as fCO2-based flux estimates. The measurements underlying the surface fCO2 maps are from the Surface Ocean CO2 Atlas version 2021 (SOCATv2021; Bakker et al., 2021), which is an update of version 3 (Bakker et al., 2016) and contains quality-controlled data through 2020 (see data attribution Table A5). Each of the estimates uses a different method to then map the SOCAT v2021 data to the global ocean. The methods include a data-driven diagnostic method (Rödenbeck et al., 2013; referred to here as Jena-MLS), three neural network models (Landschützer et al., 2014; referred to as MPI-SOMFFN; Chau et al., 2022; Copernicus Marine Environment Monitoring Service, referred to here as CMEMS-LSCE-FFNN; and Zeng et al., 2014; referred to as NIES-FNN), two cluster regression approaches (Gregor et al., 2019; referred to here as CSIR-ML6; and Gregor and Gruber, 2021, referred to as OS-ETHZ-GRaCER), and a multi-linear regression method (Iida et al., 2021; referred to as JMA-MLR). The ensemble mean of the fCO2-based flux estimates is calculated from these seven mapping methods. Further, we show the flux estimate of Watson et al. (2020), who also use the MPI-SOMFFN method to map the adjusted fCO2 data to the globe, but resulting in a substantially larger ocean sink estimate, owing to a number of adjustments they applied to the surface ocean fCO2 data and the gas-exchange parameterization. Concretely, these authors adjusted the SOCAT fCO2 downward to account for differences in temperature between the depth of the ship intake and the relevant depth right near the surface and included a further adjustment to account for the cool surface skin temperature effect. The Watson et al. flux estimate hence differs from the others by their choice of adjusting the flux to a cool, salty ocean surface skin. Watson et al. (2020) showed that this temperature adjustment leads to an upward correction of the ocean carbon sink, up to 0.9 GtC yr−1, that, if correct, should be applied to all fCO2-based flux estimates. So far, this adjustment is based on a single line of evidence and hence associated with low confidence until further evidence is available. The Watson et al. flux estimate presented here is therefore not included in the ensemble mean of the fCO2-based flux estimates. This choice will be re-evaluated in upcoming budgets based on further lines of evidence.
The CO2 flux from each fCO2-based product is either already at or above 98 % areal coverage (Jena-MLS, OS-ETHZ-GRaCER), filled by the data provider (using Fay et al., 2021, method for JMA-MLR; and Landschützer et al., 2020, methodology for MPI-SOMFFN) or scaled for the remaining products by the ratio of the total ocean area covered by the respective product to the total ocean area (361.9×106 km2) from ETOPO1 (Amante and Eakins, 2009; Eakins and Sharman, 2010). In products where the covered area varies with time (e.g. CMEMS-LSCE-FFNN) we use the maximum area coverage. The lowest coverage is 93 % (NIES-NN), resulting in a maximum adjustment factor of 1.08 (Table A3, Hauck et al., 2020).
We further use results from two diagnostic ocean models, Khatiwala et al. (2013) and DeVries (2014), to estimate the anthropogenic carbon accumulated in the ocean prior to 1959. The two approaches assume constant ocean circulation and biological fluxes, with SOCEAN estimated as a response in the change in atmospheric CO2 concentration calibrated to observations. The uncertainty in cumulative uptake of ±20 GtC (converted to ± 1σ) is taken directly from the IPCC's review of the literature (Rhein et al., 2013), or about ±30 % for the annual values (Khatiwala et al., 2009).
C3.2 Global ocean biogeochemistry models (GOBMs)
The ocean CO2 sink for 1959–2019 is estimated using eight GOBMs (Table A2). The GOBMs represent the physical, chemical, and biological processes that influence the surface ocean concentration of CO2 and thus the air–sea CO2 flux. The GOBMs are forced by meteorological reanalysis and atmospheric CO2 concentration data available for the entire time period. They mostly differ in the source of the atmospheric forcing data (meteorological reanalysis), spin-up strategies, and in their horizontal and vertical resolutions (Table A2). All GOBMs except one (CESM-ETHZ) do not include the effects of anthropogenic changes in nutrient supply (Duce et al., 2008). They also do not include the perturbation associated with changes in riverine organic carbon (see Sect. 2.7.3).
Three sets of simulations were performed with each of the GOBMs. Simulation A applied historical changes in climate and atmospheric CO2 concentration. Simulation B is a control simulation with constant atmospheric forcing (normal-year or repeated-year forcing) and constant pre-industrial atmospheric CO2 concentration. Simulation C is forced with historical changes in atmospheric CO2 concentration, but repeated year or normal-year atmospheric climate forcing. To derive SOCEAN from the model simulations, we subtracted the annual time series of the control simulation B from the annual time series of simulation A. Assuming that drift and bias are the same in simulations A and B, we thereby correct for any model drift. Further, this difference also removes the natural steady-state flux (assumed to be 0 GtC yr−1 globally without rivers) which is often a major source of biases. Simulation B of IPSL had to be treated differently as it was forced with constant atmospheric CO2 but observed historical changes in climate. For IPSL, we fitted a linear trend to the simulation B and subtracted this linear trend from simulation A. This approach assures that the interannual variability is not removed from IPSL simulation A.
The absolute correction for bias and drift per model in the 1990s varied between < 0.01 and 0.26 GtC yr−1, with six models having positive biases, and one model having essentially no bias (NorESM). The remaining model (MPI) uses riverine input and therefore simulates outgassing in simulation B, i.e. a seemingly negative bias. By subtracting simulation B, the ocean carbon sink of the MPI model also follows the definition of SOCEAN. This correction reduces the model mean ocean carbon sink by 0.03 GtC yr−1 in the 1990s. The ocean models cover 99 % to 101 % of the total ocean area, so that area scaling is not necessary.
C3.3 GOBM evaluation and uncertainty assessment for SOCEAN
The ocean CO2 sink for all GOBMs and the ensemble mean falls within 90 % confidence of the observed range, or 1.5 to 2.9 GtC yr−1 for the 1990s (Ciais et al., 2013) after applying adjustments. An exception is the MPI model, which simulates a low ocean carbon sink of 1.38 GtC yr−1 for the 1990s in simulation A owing to the inclusion of riverine carbon flux. After adjusting to the GCB's definition of SOCEAN by subtracting simulation B, the MPI model falls into the observed range with an estimated sink of 1.69 GtC yr−1.
The GOBMs and data products have been further evaluated using the fugacity of sea surface CO2 (fCO2) from the SOCAT v2021 database (Bakker et al., 2016, 2021). We focused this evaluation on the root mean squared error (RMSE) between observed and modelled fCO2 and on a measure of the amplitude of the interannual variability of the flux (modified after Rödenbeck et al., 2015). The RMSE is calculated from detrended annually and regionally averaged time series calculated from GOBMs and data product fCO2 subsampled to open ocean (water depth > 400 m) SOCAT sampling points to measure the misfit between large-scale signals (Hauck et al., 2020) The amplitude of the SOCEAN interannual variability (A-IAV) is calculated as the temporal standard deviation of the detrended CO2 flux time series (Rödenbeck et al., 2015; Hauck et al., 2020). These metrics are chosen because RMSE is the most direct measure of data–model mismatch and the A-IAV is a direct measure of the variability of SOCEAN on interannual timescales. We apply these metrics globally and by latitude bands. Results are shown in Fig. B2 and discussed in Sect. 3.5.5.
We quantify the 1σ uncertainty around the mean ocean sink of anthropogenic CO2 by assessing random and systematic uncertainties for the GOBMs and data products. The random uncertainties are taken from the ensemble standard deviation (0.3 GtC yr−1 for GOBMs, 0.3 GtC yr−1 for data products). We derive the GOBMs' systematic uncertainty by the deviation of the DIC inventory change 1994–2007 from the Gruber et al. (2019) estimate (0.5 GtC yr−1) and suggest these are related to physical transport (mixing, advection) into the ocean interior. For the data products, we consider systematic uncertainties stemming from uncertainty in fCO2 observations (0.2 GtC yr−1, Takahashi et al., 2009; Wanninkhof et al., 2013), gas-transfer velocity (0.2 GtC yr−1, Ho et al., 2011; Wanninkhof et al., 2013; Roobaert et al., 2018), wind product (0.1 GtC yr−1, Fay et al., 2021), river flux adjustment (0.2 GtC yr−1, Jacobson et al., 2007; Resplandy et al., 2018), and fCO2 mapping (0.2 GtC yr−1, Landschützer et al., 2014). Combining these uncertainties as their squared sums, we assign an uncertainty of ±0.6 GtC yr−1 to the GOBMs ensemble mean and an uncertainty of ±0.5 GtC yr−1 to the data-product ensemble mean. These uncertainties are propagated as σ( GtC yr−1 and result in an ±0.4 GtC yr−1 uncertainty around the best estimate of SOCEAN.
We examine the consistency between the variability of the model-based and the fCO2-based data products to assess confidence in SOCEAN. The interannual variability of the ocean fluxes (quantified as A-IAV, the standard deviation after detrending, Fig. B2) of the seven fCO2-based data products plus the Watson et al. (2020) product for 1990–2020 ranges from 0.16 to 0.26 GtC yr−1 with the lower estimates by the three ensemble methods (CSIR-ML6, CMEMS-LSCE-FFNN, OS-ETHZ-GRaCER). The inter-annual variability in the GOBMs ranges between 0.10 and 0.19 GtC yr−1; hence there is overlap with the lower A-IAV estimates of three data products.
Individual estimates (both GOBMs and data products) generally produce a higher ocean CO2 sink during strong El Niño events. There is emerging agreement between GOBMs and data products on the patterns of decadal variability of SOCEAN with a global stagnation in the 1990s and an extra-tropical strengthening in the 2000s (McKinley et al., 2020; Hauck et al., 2020). The central estimates of the annual flux from the GOBMs and the fCO2-based data products have a correlation r of 0.94 (1990–2020). The agreement between the models and the data products reflects some consistency in their representation of underlying variability since there is little overlap in their methodology or use of observations.
C4 Methodology land CO2 sink (SLAND)
C4.1 DGVM simulations
The DGVM runs were forced by either the merged monthly Climate Research Unit (CRU) and 6-hourly Japanese 55-year Reanalysis (JRA-55) dataset or by the monthly CRU dataset, both providing observation-based temperature, precipitation, and incoming surface radiation on a 0.5∘ × 0.5∘ grid and updated to 2020 (Harris et al., 2014, 2020). The combination of CRU monthly data with 6-hourly forcing from JRA-55 (Kobayashi et al., 2015) is performed with methodology used in previous years (Viovy, 2016) adapted to the specifics of the JRA-55 data.
New to this budget is the revision of incoming short-wave radiation fields to take into account aerosol impacts and the division of total radiation into direct and diffuse components as summarized below.
The diffuse fraction dataset offers 6-hourly distributions of the diffuse fraction of surface shortwave fluxes over the period 1901–2020. Radiative transfer calculations are based on monthly-averaged distributions of tropospheric and stratospheric aerosol optical depth and 6-hourly distributions of cloud fraction. Methods follow those described in the Methods section of Mercado et al. (2009), but with updated input datasets.
The time series of speciated tropospheric aerosol optical depth is taken from the historical and RCP8.5 simulations by the HadGEM2-ES climate model (Bellouin et al., 2011). To correct for biases in HadGEM2-ES, tropospheric aerosol optical depths are scaled over the whole period to match the global and monthly averages obtained over the period 2003–2020 by the CAMS Reanalysis of atmospheric composition (Inness et al., 2019), which assimilates satellite retrievals of aerosol optical depth.
The time series of stratospheric aerosol optical depth is taken from the Sato et al. (1993) climatology, which has been updated to 2012. Years 2013–2020 are assumed to be background years and so replicate the background year 2010. That assumption is supported by the Global Space-based Stratospheric Aerosol Climatology time series (1979–2016; Thomason et al., 2018). The time series of cloud fraction is obtained by scaling the 6-hourly distributions simulated in the Japanese Reanalysis (Kobayashi et al., 2015) to match the monthly-averaged cloud cover in the CRU TS v4.03 dataset (Harris et al., 2021). Surface radiative fluxes account for aerosol–radiation interactions from both tropospheric and stratospheric aerosols, and for aerosol–cloud interactions from tropospheric aerosols, except mineral dust. Tropospheric aerosols are also assumed to exert interactions with clouds.
The radiative effects of those aerosol–cloud interactions are assumed to scale with the radiative effects of aerosol–radiation interactions of tropospheric aerosols, using regional scaling factors derived from HadGEM2-ES. Diffuse fraction is assumed to be 1 in cloudy sky conditions. Atmospheric constituents other than aerosols and clouds are set to a constant standard mid-latitude summer atmosphere, but their variations do not affect the diffuse fraction of surface shortwave fluxes.
In summary, the DGVM forcing data include time-dependent gridded climate forcing, global atmospheric CO2 (Dlugokencky and Tans, 2022), gridded land-cover changes (see Appendix C2.2), and gridded nitrogen deposition and fertilizers (see Table A1 for specific models details).
Four simulations were performed with each of the DGVMs. Simulation 0 (S0) is a control simulation which uses fixed pre-industrial (year 1700) atmospheric CO2 concentrations, cycles early 20th century (1901–1920) climate, and applies a time-invariant pre-industrial land-cover distribution and pre-industrial wood harvest rates. Simulation 1 (S1) differs from S0 by applying historical changes in atmospheric CO2 concentration and N inputs. Simulation 2 (S2) applies historical changes in atmospheric CO2 concentration, N inputs, and climate, while applying time-invariant pre-industrial land-cover distribution and pre-industrial wood harvest rates. Simulation 3 (S3) applies historical changes in atmospheric CO2 concentration, N inputs, climate, and land-cover distribution and wood harvest rates.
S2 is used to estimate the land sink component of the global carbon budget (SLAND). S3 is used to estimate the total land flux but is not used in the global carbon budget. We further separate SLAND into contributions from CO2 (= S1 − S0) and climate (= S2 − S1 − S0).
C4.2 DGVM evaluation and uncertainty assessment for SLAND
We apply three criteria for minimum DGVM realism by including only those DGVMs with (1) steady state after spin-up; (2) global net land flux (SLAND − ELUC) that is an atmosphere-to-land carbon flux over the 1990s ranging between −0.3 and 2.3 GtC yr−1, within 90 % confidence of constraints by global atmospheric and oceanic observations (Keeling and Manning, 2014; Wanninkhof et al., 2013); and (3) global ELUC that is a carbon source to the atmosphere over the 1990s, as already mentioned in Sect. C2.2. All 17 DGVMs meet these three criteria.
In addition, the DGVM results are also evaluated using the International Land Model Benchmarking system (ILAMB; Collier et al., 2018). This evaluation is provided here to document, encourage, and support model improvements through time. ILAMB variables cover key processes that are relevant for the quantification of SLAND and resulting aggregated outcomes. The selected variables are vegetation biomass, gross primary productivity, leaf area index, net ecosystem exchange, ecosystem respiration, evapotranspiration, soil carbon, and runoff (see Fig. B3 for the results and for the list of observed databases). Results are shown in Fig. B3 and discussed in Sect. 3.6.5.
For the uncertainty for SLAND, we use the standard deviation of the annual CO2 sink across the DGVMs, averaging to about ±0.6 GtC yr−1 for the period 1959 to 2019. We attach a medium confidence level to the annual land CO2 sink and its uncertainty because the estimates from the residual budget and averaged DGVMs match well within their respective uncertainties (Table 5).
C5 Methodology atmospheric inversions
Six atmospheric inversions (details of each in Table A4) were used to infer the spatio-temporal distribution of the CO2 flux exchanged between the atmosphere and the land or oceans. These inversions are based on Bayesian inversion principles with prior information on fluxes and their uncertainties. They use very similar sets of surface measurements of CO2 time series (or subsets thereof) from various flask and in situ networks. One inversion system also used satellite xCO2 retrievals from GOSAT and OCO-2.
Each inversion system uses different methodologies and input data but is rooted in Bayesian inversion principles. These differences mainly concern the selection of atmospheric CO2 data and prior fluxes, as well as the spatial resolution, assumed correlation structures, and mathematical approach of the models. Each system uses a different transport model, which was demonstrated to be a driving factor behind differences in atmospheric inversion-based flux estimates, and specifically their distribution across latitudinal bands (Gaubert et al., 2019; Schuh et al., 2019).
The inversion systems prescribe same global fossil fuel emissions for EFOS, specifically the GCP's Gridded Fossil Emissions Dataset version 2021 (GCP-GridFEDv2021.2; Jones et al., 2021b), which is an update through 2020 of the first version of GCP-GridFED presented by Jones et al. (2021a). GCP-GridFEDv2021.2 scales gridded estimates of CO2 emissions from EDGARv4.3.2 (Janssens-Maenhout et al., 2019) within national territories to match national emissions estimates provided by the GCP for the years 1959–2020, which were compiled following the methodology described in Appendix C1 based on all information available on 31 July 2021 (Robbie Andrew, personal communication, 2021). Typically, the GCP-GridFED adopts the seasonal variation in emissions (the monthly distribution of annual emissions) from EDGAR and applies small corrections based on heating or cooling degree days to account for the effects of inter-annual climate variability on the seasonality emissions (Jones et al., 2021a). However, strategies taken to deal with the COVID-19 pandemic during 2020 mean that the seasonality of emissions diverged substantially in 2020 from a typical year. To account for this change, GCP-GridFEDv2021.2 adopts the national seasonality in emissions from Carbon Monitor (Liu et al., 2020a, b) during the years 2019–2020 (Jones et al., 2021b).
The consistent use of GCP-GridFEDv2021.2 for EFOS ensures a close alignment with the estimate of EFOS used in this budget assessment, enhancing the comparability of the inversion-based estimate with the flux estimates deriving from DGVMs, GOBMs, and fCO2-based methods. To account for small differences in regridding, and the use of a slightly earlier file version (GCP-GridFEDv2021.1) for 2000–2018 in CarbonTracker Europe, small fossil fuel corrections were applied to all inverse models to make the estimated uptake of atmospheric CO2 fully consistent. Finally, we note that GCP-GridFEDv2021.2 includes emissions from cement production, but it does not include the cement carbonation CO2 sink (Xi et al., 2016; Cao et al., 2020; Guo et al., 2021) that is applied to the GCB estimate of EFOS in Table 6.
The land and ocean CO2 fluxes from atmospheric inversions contain anthropogenic perturbation and natural pre-industrial CO2 fluxes. On annual timescales, natural pre-industrial fluxes are primarily land CO2 sinks and ocean CO2 sources corresponding to carbon taken up on land, transported by rivers from land to ocean, and outgassed by the ocean. These pre-industrial land CO2 sinks are thus compensated over the globe by ocean CO2 sources corresponding to the outgassing of riverine carbon inputs to the ocean, using the exact same numbers and distribution as described for the oceans in Sect. 2.4. To facilitate the comparison, we adjusted the inverse estimates of the land and ocean fluxes per latitude band with these numbers to produce historical perturbation CO2 fluxes from inversions. Finally, for the presentation of the comparison in Fig. 11 we modified the fossil-fuel-corrected and riverine-adjusted land sinks from the inversions further, by removing a 0.2 GtC yr−1 CO2 sink that is ascribed to cement carbonation in the GCB, rather than to terrestrial ecosystems. The latter is not applied in the inversion products released through GCB or the original data portals of these products.
All participating atmospheric inversions are checked for consistency with the annual global growth rate, as both are derived from the global surface network of atmospheric CO2 observations. In this exercise, we use the conversion factor of 2.086 GtC ppm−1 to convert the inverted carbon fluxes to mole fractions, as suggested by Prather (2012). This number is specifically suited for the comparison to surface observations that do not respond uniformly, nor immediately, to each year's summed sources and sinks. This factor is therefore slightly smaller than the GCB conversion factor in Table 1 (2.142 GtC ppm−1, Ballantyne et al., 2012). Overall, the inversions agree with the growth rate with biases between 0.03–0.08 ppm (0.06–0.17 GtC yr−1) on the decadal average.
The atmospheric inversions are also evaluated using vertical profiles of atmospheric CO2 concentrations (Fig. B4). More than 30 aircraft programmes over the globe, either regular programmes or repeated surveys over at least 9 months, have been used in order to draw a robust picture of the model performance (with space–time data coverage irregular and denser in the 0–45∘ N latitude band; Table A6). The six models are compared to the independent aircraft CO2 measurements between 2 and 7 km above sea level between 2001 and 2020. Results are shown in Fig. B4, where the inversions generally match the atmospheric mole fractions to within 0.6 ppm at all latitudes, except for CarbonTracker Europe in 2010–2020 over the more sparsely sampled Southern Hemisphere.
D1 Contribution of anthropogenic CO and CH4 to the global carbon budget
Equation (1) includes only partly the net input of CO2 to the atmosphere from the chemical oxidation of reactive carbon-containing gases from sources other than the combustion of fossil fuels, such as (1) cement process emissions, since these do not come from combustion of fossil fuels; (2) the oxidation of fossil fuels; and (3) the assumption of immediate oxidation of vented methane in oil production. However, it omits any other anthropogenic carbon-containing gases that are eventually oxidized in the atmosphere, such as anthropogenic emissions of CO and CH4. An attempt is made in this section to estimate their magnitude and identify the sources of uncertainty. Anthropogenic CO emissions are from incomplete fossil fuel and biofuel burning and deforestation fires. The main anthropogenic emissions of fossil CH4 that matter for the global (anthropogenic) carbon budget are the fugitive emissions of coal, oil, and gas sectors (see below). These emissions of CO and CH4 contribute a net addition of fossil carbon to the atmosphere.
In our estimate of EFOS we assumed (Sect. 2.1.1) that all the fuel burned is emitted as CO2, thus CO anthropogenic emissions associated with incomplete fossil fuel combustion and its atmospheric oxidation into CO2 within a few months are already counted implicitly in EFOS and should not be counted twice (same for ELUC and anthropogenic CO emissions by deforestation fires). Anthropogenic emissions of fossil CH4 are, however, not included in EFOS, because these fugitive emissions are not included in the fuel inventories. Yet they contribute to the annual CO2 growth rate after CH4 gets oxidized into CO2. Emissions of fossil CH4 represent 30 % of total anthropogenic CH4 emissions (Saunois et al., 2020; their top-down estimate is used because it is consistent with the observed CH4 growth rate), that is 0.083 GtC yr−1 for the decade 2008–2017. Assuming steady state, an amount equal to this fossil CH4 emission is all converted to CO2 by OH oxidation, thus explaining 0.083 GtC yr−1 of the global CO2 growth rate with an uncertainty range of 0.061 to 0.098 GtC yr−1 taken from the min–max of top-down estimates in Saunois et al. (2020). If this min–max range is assumed to be 2σ because Saunois et al. (2020) did not account for the internal uncertainty of their min and max top-down estimates, it translates into a 1σ uncertainty of 0.019 GtC yr−1.
Other anthropogenic changes in the sources of CO and CH4 from wildfires, vegetation biomass, wetlands, ruminants, or permafrost changes are similarly assumed to have a small effect on the CO2 growth rate. The CH4 and CO emissions and sinks are published and analysed separately in the Global Methane Budget and Global Carbon Monoxide Budget publications, which follow a similar approach to that presented here (Saunois et al., 2020; Zheng et al., 2019).
D2 Contribution of other carbonates to CO2 emissions
Although we do account for cement carbonation (a carbon sink), the contribution of emissions of fossil carbonates (carbon sources) other than cement production is not systematically included in estimates of EFOS, except at the national level where they are accounted for in the UNFCCC national inventories. The missing processes include CO2 emissions associated with the calcination of lime and limestone outside cement production. Carbonates are also used in various industries, including in iron and steel manufacture and in agriculture. They are found naturally in some coals. CO2 emissions from fossil carbonates other than cement are estimated to amount to about 1 % of EFOS (Crippa et al., 2019), though some of these carbonate emissions are included in our estimates (e.g. via UNFCCC inventories).
D3 Anthropogenic carbon fluxes in the land-to-ocean aquatic continuum
The approach used to determine the global carbon budget refers to the mean, variations, and trends in the perturbation of CO2 in the atmosphere, referenced to the pre-industrial era. Carbon is continuously displaced from the land to the ocean through the land–ocean aquatic continuum (LOAC) comprising freshwaters, estuaries, and coastal areas (Bauer et al., 2013; Regnier et al., 2013). A substantial fraction of this lateral carbon flux is entirely “natural” and is thus a steady-state component of the pre-industrial carbon cycle. We account for this pre-industrial flux where appropriate in our study (see Appendix C3). However, changes in environmental conditions and land-use change have caused an increase in the lateral transport of carbon into the LOAC – a perturbation that is relevant for the global carbon budget presented here.
The results of the analysis of Regnier et al. (2013) can be summarized in two points of relevance for the anthropogenic CO2 budget. First, the anthropogenic perturbation of the LOAC has increased the organic carbon export from terrestrial ecosystems to the hydrosphere by as much as 1.0 ±0.5 GtC yr−1 since pre-industrial times, mainly owing to enhanced carbon export from soils. Second, this exported anthropogenic carbon is partly respired through the LOAC, partly sequestered in sediments along the LOAC, and to a lesser extent transferred to the open ocean where it may accumulate or be outgassed. The increase in storage of land-derived organic carbon in the LOAC carbon reservoirs (burial) and in the open ocean combined is estimated by Regnier et al. (2013) at 0.65 ± 0.35 GtC yr−1. The inclusion of LOAC-related anthropogenic CO2 fluxes should affect estimates of SLAND and SOCEAN in Eq. (1) but does not affect the other terms. Representation of the anthropogenic perturbation of LOAC CO2 fluxes is, however, not included in the GOBMs and DGVMs used in our global carbon budget analysis presented here.
D4 Loss of additional land sink capacity
Historical land-cover change was dominated by transitions from vegetation types that can provide a large carbon sink per area unit (typically, forests) to others less efficient in removing CO2 from the atmosphere (typically, croplands). The resultant decrease in land sink, called the “loss of additional sink capacity”, can be calculated as the difference between the actual land sink under changing land cover and the counterfactual land sink under pre-industrial land cover. This term is not accounted for in our global carbon budget estimate. Here, we provide a quantitative estimate of this term to be used in the discussion. Seven of the DGVMs used in Friedlingstein et al. (2019) performed additional simulations with and without land-use change under cycled pre-industrial environmental conditions. The resulting loss of additional sink capacity amounts to 0.9 ± 0.3 GtC yr−1 on average over 2009–2018 and 42 ± 16 GtC accumulated between 1850 and 2018 (Obermeier et al., 2021). OSCAR, emulating the behaviour of 11 DGVMs, finds values of the loss of additional sink capacity of 0.7 ± 0.6 GtC yr−1 and 31 ± 23 GtC for the same time period (Gasser et al., 2020). Since the DGVM-based ELUC estimates are only used to quantify the uncertainty around the bookkeeping models' ELUC, we do not add the loss of additional sink capacity to the bookkeeping estimate.
PF, MWJ, MOS, CLQ, RMA, DCEB, JH, GPP, WP, JP, and SS designed the study, conducted the analysis, and wrote the paper with input from JGC, PC, and RBJ. RMA, GPP, and JIK produced the fossil fuel emissions and their uncertainties and analysed the emissions data. DG and GM provided fossil fuel emission data. JP, TG, CS, and RAH provided the bookkeeping land-use change emissions. JH, LB, OG, NG, TI, LR, JS, RS, and DW provided an update of the global ocean biogeochemical models. SRA, TTTC, LD, LG, YI, PL, CR, AJW, and JZ provided an update of the ocean fCO2 data products, with synthesis by JH. MB, NRB, KIC, MC, WE, RAF, SRA, TG, AK, NL, SKL, DRM, ClS, CoS, SN, CW, TO, DP, GR, AJS, BT, TT, CW, and RW provided ocean fCO2 measurements for the year 2020, with synthesis by DCEB and SDJ. PA, BD, AKJ, DK, EK, JK, SL, PCM, JRM, JEMSN, BP, HT, NV, AJW, WY, XY, and SZ provided an update of the dynamic global vegetation models, with synthesis by SS. WP, FC, LF, ITL, JL, YN, and CR provided an updated atmospheric inversion, developed the protocol, and produced the evaluation, with synthesis by WP. RMA provided predictions of the 2021 emissions and atmospheric CO2 growth rate. PL provided the predictions of the 2021 ocean and land sinks. LPC, GCH, KKG, TMS, and GRvdW provided forcing data for land-use change. GG, FT, and CY provided data for the land-use change NGHGI mapping. PPT provided key atmospheric CO2 data. MWJ produced the historical record of atmospheric CO2 concentration and growth rate, including the atmospheric CO2 forcing. MOS and NB produced the aerosol diffuse radiative forcing for the DGVMs. IH provided the climate forcing data for the DGVMs. ER provided the evaluation of the DGVMs. MWJ provided the emissions prior for use in the inversion models. XD provided seasonal emissions data for years 2019–2020 for the emission prior. MWJ and MOS developed a new data management pipeline which automates many aspects of the data collation, analysis, plotting, and synthesis. PF, MWJ, and MOS revised all figures, tables, text, and/or numbers to ensure the update was clear from the 2020 edition and in line with the Global Carbon Atlas (http://globalcarbonatlas.org, last access: 11 March 2022).
At least one of the (co-)authors is a member of the editorial board of Earth System Science Data. The peer-review process was guided by an independent editor, and the authors have also no other competing interests to declare.
Publisher's note: Copernicus Publications remains neutral with regard to jurisdictional claims in published maps and institutional affiliations.
We thank all people and institutions who provided the data used in this global carbon budget 2021 and the Global Carbon Project members for their input throughout the development of this publication. We thank Nigel Hawtin for producing Figs. 2 and 13. We thank Omar Jamil and Freddy Wordingham for technical support. We thank Ed Dlugokencky for providing atmospheric CO2 measurements. We thank Vivek Arora, Ian G. C. Ashton, Erik Buitenhuis, Fatemeh Cheginig, Christian Ethé, Marion Gehlen, Lonneke Goddijn-Murphy, Thomas Holding, Fabrice Lacroix, Enhui Liao, Pedro M. S. Monteiro, Naiquing Pan, Tristan Quaife, Shijie Shu, Jamie D. Shutler, Jade Skye, Anthony Walker, and David K. Woolf for their involvement in the development, use, and analysis of the models and data products used here. We thank Markus Ritschel, Carmen Rodriguez, Claire Lo Monaco, Nicolas Metzl, Vassilis Kitidis, Sören Gutekunst, Anne Willstrand Wranne, Tobias Steinhoff, Jessica N. Cross, Natalie M. Monacci, Alice Benoit-Cattin, Sólveig R. Ólafsdóttir, Joe Salisbury, Doug Vandemark, and Christopher W. Hunt, who contributed to the provision of surface ocean CO2 observations for the year 2020 (see Table A5). We also thank Benjamin Pfeil, Rocío Castaño-Primo, Camilla Landa, and Maren Karlsen of the Ocean Thematic Centre of the EU Integrated Carbon Observation System (ICOS) Research Infrastructure; Kevin O'Brien and Eugene Burger of NOAA's Pacific Marine Environmental Laboratory; and Alex Kozyr of NOAA's National Centers for Environmental Information, for their contribution to surface ocean CO2 data and metadata management. We thank the scientists, institutions, and funding agencies responsible for the collection and quality control of the data in SOCAT as well as the International Ocean Carbon Coordination Project (IOCCP), the Surface Ocean Lower Atmosphere Study (SOLAS), and the Integrated Marine Biosphere Research (IMBeR) programme for their support. We thank data providers ObsPack GLOBALVIEWplus v6.1 and NRT v6.1.1 for atmospheric CO2 observations. We thank the individuals and institutions that provided the databases used for the models evaluations used here. We thank Fortunat Joos, Samar Khatiwala, and Timothy DeVries for providing historical data. Nicolas Vuichard thanks the whole ORCHIDEE group. Yosuke Niwa thanks CSIRO, EC, EMPA, FMI, IPEN, JMA, LSCE, NCAR, NIES, NILU, NIWA, NOAA, SIO, and TU/NIPR for providing data for NISMON-CO2. We thank Kevin Bowman (NASA JPL) for contribution to the CMS-Flux results. Junjie Liu thanks the Jet Propulsion Laboratory, California Institute of Technology. This is PMEL contribution 5317. Steve D. Jones thanks the data management team at the Bjerknes Climate Data Centre. Wiley Evans thanks the Tula Foundation for funding support. Australian ocean CO2 data were sourced from Australia's Integrated Marine Observing System (IMOS); IMOS is enabled by the National Collaborative Research Infrastructure Strategy (NCRIS). Margot Cronin thanks Anthony English, Clynt Gregory, and Gordon Furey (P&O Maritime Services) as well as Tobias Steinhoff for their support. Nathalie Lefèvre thanks the crew of the Cap San Lorenzo and the US IMAGO of IRD Brest for technical support. Gregor Rehder is grateful for the skilful technical support of Michael Glockzin and Bernd Sadkowiak. Matthew W. Jones thanks Anthony J. De-Gol for his technical and conceptual assistance with the development of GCP-GridFED. We thank Ana Bastos and Joana Melo for helpful comments on land-use emission estimates. FAOSTAT is funded by FAO member states through their contributions to the FAO Regular Programme, and data contributions by national experts are greatly acknowledged. The views expressed in this paper are the authors' only and do not necessarily reflect those of FAO. Finally, we thank all funders who have supported the individual and joint contributions to this work (see Table A9), as well as the reviewers of this paper and previous versions, and the many researchers who have provided feedback.
For a list of all funders that have supported this research, please refer to Table A9.
This paper was edited by David Carlson and reviewed by Hélène Peiro and one anonymous referee.
Ahlström, A., Raupach, M. R., Schurgers, G., Smith, B., Arneth, A., Jung, M., Reichstein, M., Canadell, J. G., Friedlingstein, P., Jain, A. K., Kato, E., Poulter, B., Sitch, S., Stocker, B. D., Viovy, N., Wang, Y. P., Wiltshire, A., Zaehle, S., and Zeng, N.: The dominant role of semi-arid ecosystems in the trend and variability of the land CO2 sink, Science, 348, 895–899, https://doi.org/10.1126/science.aaa1668, 2015.
Amador-Jiménez, M., Millner, N., Palmer, C., Pennington, R. T., and Sileci, L.: The Unintended Impact of Colombia's Covid-19 Lockdown on Forest Fires, Environ. Resource Econ., 76, 1081–1105, https://doi.org/10.1007/s10640-020-00501-5, 2020.
Amante, C. and Eakins, B. W.: ETOPO1 Global Relief Model converted to PanMap layer format, PANGAEA, https://doi.org/10.1594/PANGAEA.769615, 2009.
Andela, N., Morton, D. C., Giglio, L., Chen, Y., van der Werf, G. R., Kasibhatla, P. S., DeFries, R. S., Collatz, G. J., Hantson, S., Kloster, S., Bachelet, D., Forrest, M., Lasslop, G., Li, F., Mangeon, S., Melton, J. R., Yue, C., and Randerson, J. T.: A human-driven decline in global burned area, Science, 356, 1356–1362, https://doi.org/10.1126/science.aal4108, 2017.
Andres, R. J., Boden, T. A., Bréon, F.-M., Ciais, P., Davis, S., Erickson, D., Gregg, J. S., Jacobson, A., Marland, G., Miller, J., Oda, T., Olivier, J. G. J., Raupach, M. R., Rayner, P., and Treanton, K.: A synthesis of carbon dioxide emissions from fossil-fuel combustion, Biogeosciences, 9, 1845–1871, https://doi.org/10.5194/bg-9-1845-2012, 2012.
Andres, R. J., Boden, T. A., and Higdon, D.: A new evaluation of the uncertainty associated with CDIAC estimates of fossil fuel carbon dioxide emission, Tellus B, 66, 23616, https://doi.org/10.3402/tellusb.v66.23616, 2014.
Andrew, R. M.: A comparison of estimates of global carbon dioxide emissions from fossil carbon sources, Earth Syst. Sci. Data, 12, 1437–1465, https://doi.org/10.5194/essd-12-1437-2020, 2020a.
Andrew, R. M.: Timely estimates of India's annual and monthly fossil CO2 emissions, Earth Syst. Sci. Data, 12, 2411–2421, https://doi.org/10.5194/essd-12-2411-2020, 2020b.
Andrew, R. M.: Towards near real-time, monthly fossil CO2 emissions estimates for the European Union with current-year projections, Atmos. Pollut. Res., 12, 101229, https://doi.org/10.1016/j.apr.2021.101229, 2021.
Andrew, R. M. and Peters, G. P.: A multi-region input–output table based on the global trade analysis project database (GTAP-MRIO), Economic Systems Research, 25, 99–121, https://doi.org/10.1080/09535314.2012.761953, 2013.
Andrew, R. M. and Peters, G. P.: The Global Carbon Project's fossil CO2 emissions dataset (2021v34), Zenodo [data set], https://doi.org/10.5281/ZENODO.5569235, 2021.
Aragão, L. E. O. C., Anderson, L. O., Fonseca, M. G., Rosan, T. M., Vedovato, L. B., Wagner, F. H., Silva, C. V. J., Silva Junior, C. H. L., Arai, E., Aguiar, A. P., Barlow, J., Berenguer, E., Deeter, M. N., Domingues, L. G., Gatti, L., Gloor, M., Malhi, Y., Marengo, J. A., Miller, J. B., Phillips, O. L., and Saatchi, S.: 21st Century drought-related fires counteract the decline of Amazon deforestation carbon emissions, Nat. Commun., 9, 536, https://doi.org/10.1038/s41467-017-02771-y, 2018.
Archer, D., Eby, M., Brovkin, V., Ridgwell, A., Cao, L., Mikolajewicz, U., Caldeira, K., Matsumoto, K., Munhoven, G., Montenegro, A., and Tokos, K.: Atmospheric Lifetime of Fossil Fuel Carbon Dioxide, Annu. Rev. Earth Pl. Sci., 37, 117–134, https://doi.org/10.1146/annurev.earth.031208.100206, 2009.
Arneth, A., Sitch, S., Pongratz, J., Stocker, B. D., Ciais, P., Poulter, B., Bayer, A. D., Bondeau, A., Calle, L., Chini, L. P., Gasser, T., Fader, M., Friedlingstein, P., Kato, E., Li, W., Lindeskog, M., Nabel, J. E. M. S., Pugh, T. A. M., Robertson, E., Viovy, N., Yue, C., and Zaehle, S.: Historical carbon dioxide emissions caused by land-use changes are possibly larger than assumed, Nat. Geosci., 10, 79–84, https://doi.org/10.1038/ngeo2882, 2017.
Arora, V. K., Boer, G. J., Christian, J. R., Curry, C. L., Denman, K. L., Zahariev, K., Flato, G. M., Scinocca, J. F., Merryfield, W. J., and Lee, W. G.: The Effect of Terrestrial Photosynthesis Down Regulation on the Twentieth-Century Carbon Budget Simulated with the CCCma Earth System Model, J. Climate, 22, 6066–6088, https://doi.org/10.1175/2009JCLI3037.1, 2009.
Asaadi, A., Arora, V. K., Melton, J. R., and Bartlett, P.: An improved parameterization of leaf area index (LAI) seasonality in the Canadian Land Surface Scheme (CLASS) and Canadian Terrestrial Ecosystem Model (CTEM) modelling framework, Biogeosciences, 15, 6885–6907, https://doi.org/10.5194/bg-15-6885-2018, 2018.
Aumont, O., Orr, J. C., Monfray, P., Ludwig, W., Amiotte-Suchet, P., and Probst, J.-L.: Riverine-driven interhemispheric transport of carbon, Global Biogeochem. Cy., 15, 393–405, https://doi.org/10.1029/1999GB001238, 2001.
Aumont, O., Ethé, C., Tagliabue, A., Bopp, L., and Gehlen, M.: PISCES-v2: an ocean biogeochemical model for carbon and ecosystem studies, Geosci. Model Dev., 8, 2465–2513, https://doi.org/10.5194/gmd-8-2465-2015, 2015.
Avitabile, V., Herold, M., Heuvelink, G. B. M., Lewis, S. L., Phillips, O. L., Asner, G. P., Armston, J., Ashton, P. S., Banin, L., Bayol, N., Berry, N. J., Boeckx, P., de Jong, B. H. J., DeVries, B., Girardin, C. A. J., Kearsley, E., Lindsell, J. A., Lopez-Gonzalez, G., Lucas, R., Malhi, Y., Morel, A., Mitchard, E. T. A., Nagy, L., Qie, L., Quinones, M. J., Ryan, C. M., Ferry, S. J. W., Sunderland, T., Laurin, G. V., Gatti, R. C., Valentini, R., Verbeeck, H., Wijaya, A., and Willcock, S.: An integrated pan-tropical biomass map using multiple reference datasets, Glob. Change Biol., 22, 1406–1420, https://doi.org/10.1111/gcb.13139, 2016.
Baccini, A., Walker, W., Carvalho, L., Farina, M., Sulla-Menashe, D., and Houghton, R. A.: Tropical forests are a net carbon source based on aboveground measurements of gain and loss, Science, 358, 230–234, https://doi.org/10.1126/science.aam5962, 2017.
Bakker, D. C. E., Pfeil, B., Landa, C. S., Metzl, N., O'Brien, K. M., Olsen, A., Smith, K., Cosca, C., Harasawa, S., Jones, S. D., Nakaoka, S., Nojiri, Y., Schuster, U., Steinhoff, T., Sweeney, C., Takahashi, T., Tilbrook, B., Wada, C., Wanninkhof, R., Alin, S. R., Balestrini, C. F., Barbero, L., Bates, N. R., Bianchi, A. A., Bonou, F., Boutin, J., Bozec, Y., Burger, E. F., Cai, W.-J., Castle, R. D., Chen, L., Chierici, M., Currie, K., Evans, W., Featherstone, C., Feely, R. A., Fransson, A., Goyet, C., Greenwood, N., Gregor, L., Hankin, S., Hardman-Mountford, N. J., Harlay, J., Hauck, J., Hoppema, M., Humphreys, M. P., Hunt, C. W., Huss, B., Ibánhez, J. S. P., Johannessen, T., Keeling, R., Kitidis, V., Körtzinger, A., Kozyr, A., Krasakopoulou, E., Kuwata, A., Landschützer, P., Lauvset, S. K., Lefèvre, N., Lo Monaco, C., Manke, A., Mathis, J. T., Merlivat, L., Millero, F. J., Monteiro, P. M. S., Munro, D. R., Murata, A., Newberger, T., Omar, A. M., Ono, T., Paterson, K., Pearce, D., Pierrot, D., Robbins, L. L., Saito, S., Salisbury, J., Schlitzer, R., Schneider, B., Schweitzer, R., Sieger, R., Skjelvan, I., Sullivan, K. F., Sutherland, S. C., Sutton, A. J., Tadokoro, K., Telszewski, M., Tuma, M., van Heuven, S. M. A. C., Vandemark, D., Ward, B., Watson, A. J., and Xu, S.: A multi-decade record of high-quality fCO2 data in version 3 of the Surface Ocean CO2 Atlas (SOCAT), Earth Syst. Sci. Data, 8, 383–413, https://doi.org/10.5194/essd-8-383-2016, 2016.
Bakker, D. C. E., Alin, S. R., Castaño-Primo, R., Cronin, M., Gkritzalis, T., Kozyr, A., Lauvset, S. K., Metzl, N., Munro, D. R., Nakaoka, S.-I., O'Brien, K. M., Olsen, A., Omar, A. M., Pfeil, B., Pierrot, D., Rodriguez, C., Steinhoff, T., Sutton, A. J., Tilbrook, B., Wanninkhof, R., Willstrand Wranne, A., Ahmed, M., Andersson, A., Apelthun, L. B., Bates, N., Battisti, R., Beaumont, L., Becker, M., Benoit-Cattin, A., Berghoff, C. F., Boutin, J., Burger, E. F., Burgers, T. M., Cantoni, C., Cattrijsse, A., Chierici, M., Cross, J. N., Coppola, L., Cosca, C. E., Currie, K. I., De Carlo, E. H., Else, B., Enright, M. P., Ericson, Y., Evans, W., Feely, R. A., Fiedler, B., Fransson, A., García-Ibáñez, M. I., Gehrung, M., Glockzin, M., González Dávila, M., Gutekunst, S., Hermes, R., Humphreys, M. P., Hunt, C. W., Ibánhez, J. S. P., Jones, S. D., Kitidis, V., Körtzinger, A., Kosugi, N., Landa, C. S., Landschützer, P., Lefèvre, N., Lo Monaco, C., Luchetta, A., Lutz, V. A., Macovei, V. A., Manke, A. B., Merlivat, L., Millero, F. J., Monacci, N. M., Negri, R. M., Newberger, T., Newton, J., Nickford, S. E., Nojiri, Y., Ohman, M., Ólafsdóttir, S. R., Sweeney, C., Ono, T., Palter, J. B., Papakyriakou, T., Peterson, W. T., Plueddemann, A. J., Qi, D., Rehder, G., Ritschel, M., Rutgersson, A., Sabine, C. L., Salisbury, J. E., Santana-Casiano, J. M., Schlitzer, R., Send, U., Skjelvan, I., Smith, K., Sparnocchia, S., Sullivan, K. F., Sutherland, S. C., Szuts, Z. B., Tadokoro, K., Tanhua, T., Telszewski, M., Theetaert, H., Vandemark, D., Voynova, Y. G., Wada, C., Weller, R. A., and Woosley, R. J.: Surface Ocean CO2 Atlas Database Version 2021 (SOCATv2021) (NCEI Accession 0235360), NOAA National Centers for Environmental Information [data set], https://doi.org/10.25921/YG69-JD96, 2021.
Ballantyne, A. P., Alden, C. B., Miller, J. B., Tans, P. P., and White, J. W. C.: Increase in observed net carbon dioxide uptake by land and oceans during the past 50 years, Nature, 488, 70–72, https://doi.org/10.1038/nature11299, 2012.
Ballantyne, A. P., Andres, R., Houghton, R., Stocker, B. D., Wanninkhof, R., Anderegg, W., Cooper, L. A., DeGrandpre, M., Tans, P. P., Miller, J. B., Alden, C., and White, J. W. C.: Audit of the global carbon budget: estimate errors and their impact on uptake uncertainty, Biogeosciences, 12, 2565–2584, https://doi.org/10.5194/bg-12-2565-2015, 2015.
Bastos, A., O'Sullivan, M., Ciais, P., Makowski, D., Sitch, S., Friedlingstein, P., Chevallier, F., Rödenbeck, C., Pongratz, J., Luijkx, I. T., Patra, P. K., Peylin, P., Canadell, J. G., Lauerwald, R., Li, W., Smith, N. E., Peters, W., Goll, D. S., Jain, A. K., Kato, E., Lienert, S., Lombardozzi, D. L., Haverd, V., Nabel, J. E. M. S., Poulter, B., Tian, H., Walker, A. P., and Zaehle, S.: Sources of Uncertainty in Regional and Global Terrestrial CO2 Exchange Estimates, Global Biogeochem. Cy., 34, e2019GB006393, https://doi.org/10.1029/2019GB006393, 2020.
Bastos, A., Hartung, K., Nützel, T. B., Nabel, J. E. M. S., Houghton, R. A., and Pongratz, J.: Comparison of uncertainties in land-use change fluxes from bookkeeping model parameterisation, Earth Syst. Dynam., 12, 745–762, https://doi.org/10.5194/esd-12-745-2021, 2021.
Bauer, J. E., Cai, W.-J., Raymond, P. A., Bianchi, T. S., Hopkinson, C. S., and Regnier, P. A. G.: The changing carbon cycle of the coastal ocean, Nature, 504, 61–70, https://doi.org/10.1038/nature12857, 2013.
Beckman, J. and Countryman, A. M.: The Importance of Agriculture in the Economy: Impacts from COVID-19, Am. J. Agr. Econ., 103, 1595–1611, https://doi.org/10.1111/ajae.12212, 2021.
Bellouin, N., Rae, J., Jones, A., Johnson, C., Haywood, J., and Boucher, O.: Aerosol forcing in the Climate Model Intercomparison Project (CMIP5) simulations by HadGEM2-ES and the role of ammonium nitrate, J. Geophys. Res-Atmos., 116, D20206, https://doi.org/10.1029/2011JD016074, 2011.
Berthet, S., Séférian, R., Bricaud, C., Chevallier, M., Voldoire, A., and Ethé, C.: Evaluation of an Online Grid-Coarsening Algorithm in a Global Eddy-Admitting Ocean Biogeochemical Model, J. Adv. Model. Earth Sy., 11, 1759–1783, https://doi.org/10.1029/2019MS001644, 2019.
Brancalion, P. H. S., Broadbent, E. N., de-Miguel, S., Cardil, A., Rosa, M. R., Almeida, C. T., Almeida, D. R. A., Chakravarty, S., Zhou, M., Gamarra, J. G. P., Liang, J., Crouzeilles, R., Hérault, B., Aragão, L. E. O. C., Silva, C. A., and Almeyda-Zambrano, A. M.: Emerging threats linking tropical deforestation and the COVID-19 pandemic, Perspect. Ecol. Cons., 18, 243–246, https://doi.org/10.1016/j.pecon.2020.09.006, 2020.
Brienen, R. J. W., Phillips, O. L., Feldpausch, T. R., Gloor, E., Baker, T. R., Lloyd, J., Lopez-Gonzalez, G., Monteagudo-Mendoza, A., Malhi, Y., Lewis, S. L., Vásquez Martinez, R., Alexiades, M., Álvarez Dávila, E., Alvarez-Loayza, P., Andrade, A., Aragão, L. E. O. C., Araujo-Murakami, A., Arets, E. J. M. M., Arroyo, L., Aymard C., G. A., Bánki, O. S., Baraloto, C., Barroso, J., Bonal, D., Boot, R. G. A., Camargo, J. L. C., Castilho, C. V., Chama, V., Chao, K. J., Chave, J., Comiskey, J. A., Cornejo Valverde, F., da Costa, L., de Oliveira, E. A., Di Fiore, A., Erwin, T. L., Fauset, S., Forsthofer, M., Galbraith, D. R., Grahame, E. S., Groot, N., Hérault, B., Higuchi, N., Honorio Coronado, E. N., Keeling, H., Killeen, T. J., Laurance, W. F., Laurance, S., Licona, J., Magnussen, W. E., Marimon, B. S., Marimon-Junior, B. H., Mendoza, C., Neill, D. A., Nogueira, E. M., Núñez, P., Pallqui Camacho, N. C., Parada, A., Pardo-Molina, G., Peacock, J., Peña-Claros, M., Pickavance, G. C., Pitman, N. C. A., Poorter, L., Prieto, A., Quesada, C. A., Ramírez, F., Ramírez-Angulo, H., Restrepo, Z., Roopsind, A., Rudas, A., Salomão, R. P., Schwarz, M., Silva, N., Silva-Espejo, J. E., Silveira, M., Stropp, J., Talbot, J., ter Steege, H., Teran-Aguilar, J., Terborgh, J., Thomas-Caesar, R., Toledo, M., Torello-Raventos, M., Umetsu, R. K., van der Heijden, G. M. F., van der Hout, P., Guimarães Vieira, I. C., Vieira, S. A., Vilanova, E., Vos, V. A., and Zagt, R. J.: Long-term decline of the Amazon carbon sink, Nature, 519, 344–348, https://doi.org/10.1038/nature14283, 2015.
Broecker, W. S.: Ocean chemistry during glacial time, Geochim. Cosmochim. Ac., 46, 1689–1705, https://doi.org/10.1016/0016-7037(82)90110-7, 1982.
Bruno, M. and Joos, F.: Terrestrial carbon storage during the past 200 years: A Monte Carlo Analysis of CO2 data from ice core and atmospheric measurements, Global Biogeochem. Cy., 11, 111–124, https://doi.org/10.1029/96GB03611, 1997.
Buitenhuis, E. T., Hashioka, T., and Quéré, C. L.: Combined constraints on global ocean primary production using observations and models: Ocean Primary Production, Global Biogeochem. Cy., 27, 847–858, https://doi.org/10.1002/gbc.20074, 2013.
Burton, C., Betts, R., Cardoso, M., Feldpausch, T. R., Harper, A., Jones, C. D., Kelley, D. I., Robertson, E., and Wiltshire, A.: Representation of fire, land-use change and vegetation dynamics in the Joint UK Land Environment Simulator vn4.9 (JULES), Geosci. Model Dev., 12, 179–193, https://doi.org/10.5194/gmd-12-179-2019, 2019.
Bushinsky, S. M., Landschützer, P., Rödenbeck, C., Gray, A. R., Baker, D., Mazloff, M. R., Resplandy, L., Johnson, K. S., and Sarmiento, J. L.: Reassessing Southern Ocean Air-Sea CO2 Flux Estimates With the Addition of Biogeochemical Float Observations, Global Biogeochem. Cy., 33, 1370–1388, https://doi.org/10.1029/2019GB006176, 2019.
Canadell, J. G., Le Quere, C., Raupach, M. R., Field, C. B., Buitenhuis, E. T., Ciais, P., Conway, T. J., Gillett, N. P., Houghton, R. A., and Marland, G.: Contributions to accelerating atmospheric CO2 growth from economic activity, carbon intensity, and efficiency of natural sinks, P. Natl. Acad. Sci. USA, 104, 18866–18870, https://doi.org/10.1073/pnas.0702737104, 2007.
Canadell, J. G., Monteiro, P. M. S., Costa, M. H., Cotrim da Cunha, L., Cox, P. M., Eliseev, A. V., Henson, S., Ishii, M., Jaccard, S., Koven, C., Lohila, A., Patra, P. K., Piao, S., Rogelj, J., Syampungani, S., Zaehle, S., and Zickfeld, K.: Global Carbon and other Biogeochemical Cycles and Feedbacks, in: Climate Change 2021: The Physical Science Basis, Contribution of Working Group I to the Sixth Assessment Report of the Intergovernmental Panel on Climate Change, edited by: Masson-Delmotte, V., Zhai, P., Pirani, A., Connors, S. L., Péan, C., Berger, S., Caud, N., Chen, Y., Goldfarb, L., Gomis, M. I., Huang, M., Leitzell, K., Lonnoy, E., Matthews, J. B. R., Maycock, T. K., Waterfield, T., Yelekçi, O., Yu, R., and Zhou, B., Cambridge University Press, in press, 2022.
Cao, Z., Myers, R. J., Lupton, R. C., Duan, H., Sacchi, R., Zhou, N., Reed Miller, T., Cullen, J. M., Ge, Q., and Liu, G.: The sponge effect and carbon emission mitigation potentials of the global cement cycle, Nat. Commun., 11, 3777, https://doi.org/10.1038/s41467-020-17583-w, 2020.
Carbon Monitor: CO2 Emissions Variation, https://carbonmonitor.org/, last access: 11 March 2022.
Chatfield, C.: The Holt-Winters Forecasting Procedure, J. Roy. Stat. Soc. Ser. C, 27, 264–279, https://doi.org/10.2307/2347162, 1978.
Chau, T. T. T., Gehlen, M., and Chevallier, F.: Quality Information Doceument for Global Ocean Surface Carbon Product MULTIOBS_GLO_BIO_CARBON_SURFACE_REP_015_008, https://hal.archives-ouvertes.fr/hal-02957656/document (last access: 11 March 2022), Laboratoire des Sciences du Climat et de l’Environnement, 2020.
Chau, T. T. T., Gehlen, M., and Chevallier, F.: A seamless ensemble-based reconstruction of surface ocean pCO2 and air–sea CO2 fluxes over the global coastal and open oceans, Biogeosciences, 19, 1087–1109, https://doi.org/10.5194/bg-19-1087-2022, 2022.
Chevallier, F.: On the parallelization of atmospheric inversions of CO2 surface fluxes within a variational framework, Geosci. Model Dev., 6, 783–790, https://doi.org/10.5194/gmd-6-783-2013, 2013.
Chevallier, F., Fisher, M., Peylin, P., Serrar, S., Bousquet, P., Bréon, F.-M., Chédin, A., and Ciais, P.: Inferring CO2 sources and sinks from satellite observations: Method and application to TOVS data, J. Geophys. Res., 110, D24309, https://doi.org/10.1029/2005JD006390, 2005.
Chini, L., Hurtt, G., Sahajpal, R., Frolking, S., Klein Goldewijk, K., Sitch, S., Ganzenmüller, R., Ma, L., Ott, L., Pongratz, J., and Poulter, B.: Land-use harmonization datasets for annual global carbon budgets, Earth Syst. Sci. Data, 13, 4175–4189, https://doi.org/10.5194/essd-13-4175-2021, 2021.
Ciais, P., Sabine, C., Bala, G., Bopp, L., Brovkin, V., Canadell, J. G., Chhabra, A., DeFries, R., Galloway, J., Heimann, M., Jones, C., Le Quéré, C., Myneni, R., Piao, S., Thornton, P., Willem, J., Friedlingstein, P., and Munhoven, G.: Carbon and Other Biogeochemical Cycles, in: Climate Change 2013: The Physical Science Basis, Contribution of Working Group I to the Fifth Assessment Report of the Intergovernmental Panel on Climate Change, edited by: Intergovernmental Panel on Climate Change, Cambridge University Press, Cambridge, UK, 465–570, 2013.
Ciais, P., Tan, J., Wang, X., Roedenbeck, C., Chevallier, F., Piao, S.-L., Moriarty, R., Broquet, G., Le Quéré, C., Canadell, J. G., Peng, S., Poulter, B., Liu, Z., and Tans, P.: Five decades of northern land carbon uptake revealed by the interhemispheric CO2 gradient, Nature, 568, 221–225, https://doi.org/10.1038/s41586-019-1078-6, 2019.
Ciais, P., Bastos, A., Chevallier, F., Lauerwald, R., Poulter, B., Canadell, J. G., Hugelius, G., Jackson, R. B., Jain, A., Jones, M., Kondo, M., Luijkx, I. T., Patra, P. K., Peters, W., Pongratz, J., Petrescu, A. M. R., Piao, S., Qiu, C., Von Randow, C., Regnier, P., Saunois, M., Scholes, R., Shvidenko, A., Tian, H., Yang, H., Wang, X., and Zheng, B.: Definitions and methods to estimate regional land carbon fluxes for the second phase of the REgional Carbon Cycle Assessment and Processes Project (RECCAP-2), Geosci. Model Dev., 15, 1289–1316, https://doi.org/10.5194/gmd-15-1289-2022, 2022.
Collier, N., Hoffman, F. M., Lawrence, D. M., Keppel-Aleks, G., Koven, C. D., Riley, W. J., Mu, M., and Randerson, J. T.: The International Land Model Benchmarking (ILAMB) System: Design, Theory, and Implementation, J. Adv. Model. Earth Sy., 10, 2731–2754, https://doi.org/10.1029/2018MS001354, 2018.
Conchedda, G. and Tubiello, F. N.: Drainage of organic soils and GHG emissions: validation with country data, Earth Syst. Sci. Data, 12, 3113–3137, https://doi.org/10.5194/essd-12-3113-2020, 2020.
Cooper, D. J., Watson, A. J., and Ling, R. D.: Variation of pCO2 along a North Atlantic shipping route (U.K. to the Caribbean): A year of automated observations, Mar. Chem., 60, 147–164, https://doi.org/10.1016/S0304-4203(97)00082-0, 1998.
Cox, A., Di Sarra, A. G., Vermeulen, A., Manning, A., Beyersdorf, A., Zahn, A., Manning, A., Watson, A., Karion, A., Hensen, A., Arlyn A., Frumau, A., Colomb, A., Scheeren, B., Law, B., Baier, B., Munger, B., Paplawsky, B., Viner, B., Stephens, B., Daube, B., Labuschagne, C., Myhre, C. L., Hanson, C., Miller, C. E., Plass-Duelmer, C., Gerbig, C., Sloop, C. D., Sweeney, C., Kubistin, D., Goto, D., Jaffe, D., Say, D., Van Dinther, D., Bowling, D., Lam, D. H. Y., Munro, D., Dickon Y., Worthy, D., Dlugokencky, E., Kozlova, E., Gloor, E., Cuevas, E., Reyes-Sanchez, E., Hintsa, E., Kort, E., Morgan, E., Obersteiner, F., Apadula, F., Gheusi, F., Meinhardt, F., Moore, F., Vitkova, G., Chen, G., Bentz, G., Manca, G., Brailsford, G., Forster, G., Boenisch, H., Riris, H., Meijer, H., Timas, H., Matsueda, H., Huilin C., Levin, I., Lehner, I., Mammarella, I., Bartyzel, J., Abshire, J. B., Elkins, J. W., Levula, J., Necki, J., Pichon, J. M., Peischl, J., Müller- Williams, J., Turnbull, J., Miller, J. B., Lee, J., Lin, J., Morgui, J.-A., DiGangi, J. P., Lavric, J., Hatakka, J., Coletta, J. D., Worsey, J., Holst, J., Kominkova, K., McKain, K., Saito, K., Aikin, K., Davis, K., Thoning, K., Tørseth, K., Haszpra, L., Mitchell, L., Gatti, L.V., Emmenegger, L., Chmura, M., Merchant, L., Sha, M. K., Delmotte, M.,Fischer, Marc L., Schumacher, M., Torn, M., Leuenberger, M., Heimann, M., Steinbacher, M., De Mazière, M., Sargent, M., Lindauer, M., Mölder, M., Martin, M. Y., Shook, M., Galkowski, M., Heliasz, M., Marek, M. V., Ramonet, M., Miroslaw Z., Lopez, M., Sasakawa, M., Mihalopoulos, N., Miles, N., Lee, O. S.M., Laurent, O., Peltola, O., Hermanssen, O., Trisolino, P., Cristofanelli, P., Kolari, P., Krummel, P., Shepson, P., Smith, P., Rivas, P. P., Bakwin, P., Bergamaschi, P., Keronen, P., Tans, P., Van Den Bulk, P., Keeling, R., Ramos, R., Langenfelds, R., Leppert, R., Curcoll, R., Commane, R., Newman, S., Piacentino, S., Hammer, S., Richardson, S., Biraud, S. C., Conil, S., Clark, S., Morimoto, S., Shuangxi F., Aoki, S., O'Doherty, S., Sites Climadat, Zaehle, S., De Wekker, S., Kawa, S. R., Montzka, S., Walker, S., Piper, S., Wofsy, S., Nichol, S., Schuck, T., Lauvaux, T., Ryerson, T., Seifert, T., Griffis, T., Biermann, T., Gehrlein, T., Machida, T., Laurila, T., Aalto, T., Gomez-Trueba, V., Kazan, V., Ivakhov, V., Joubert, W., Niwa, Y., and Loh, Z.: Multi-laboratory compilation of atmospheric carbon dioxide data for the period 1957–2019; obspack_CO2_1_GLOBALVIEWplus_v6.1_2021-03-01, NOAA Global Monitoring Laboratory [data set], https://doi.org/10.25925/20201204, 2021.
Cox, P. M., Pearson, D., Booth, B. B., Friedlingstein, P., Huntingford, C., Jones, C. D., and Luke, C. M.: Sensitivity of tropical carbon to climate change constrained by carbon dioxide variability, Nature, 494, 341–344, https://doi.org/10.1038/nature11882, 2013.
Crippa, M., Janssens-Maenhout, G., Guizzardi, D., Van Dingenen, R., and Dentener, F.: Contribution and uncertainty of sectorial and regional emissions to regional and global PM2.5 health impacts, Atmos. Chem. Phys., 19, 5165–5186, https://doi.org/10.5194/acp-19-5165-2019, 2019.
Dai, A. and Trenberth, K. E.: Estimates of Freshwater Discharge from Continents: Latitudinal and Seasonal Variations, J. Hydrometeorol., 3, 660–687, https://doi.org/10.1175/1525-7541(2002)003<0660:EOFDFC>2.0.CO;2, 2002.
Davis, S. J. and Caldeira, K.: Consumption-based accounting of CO2 emissions, P. Natl. Acad. Sci. USA, 107, 5687–5692, https://doi.org/10.1073/pnas.0906974107, 2010.
Decharme, B., Delire, C., Minvielle, M., Colin, J., Vergnes, J.-P., Alias, A., Saint-Martin, D., Séférian, R., Sénési, S., and Voldoire, A.: Recent Changes in the ISBA-CTRIP Land Surface System for Use in the CNRM-CM6 Climate Model and in Global Off-Line Hydrological Applications, J. Adv. Model Earth Sy., 11, 1207–1252, https://doi.org/10.1029/2018MS001545, 2019.
De Kauwe, M. G., Disney, M. I., Quaife, T., Lewis, P., and Williams, M.: An assessment of the MODIS collection 5 leaf area index product for a region of mixed coniferous forest, Remote Sens. Environ., 115, 767–780, https://doi.org/10.1016/j.rse.2010.11.004, 2011.
Delire, C., Séférian, R., Decharme, B., Alkama, R., Calvet, J.-C., Carrer, D., Gibelin, A.-L., Joetzjer, E., Morel, X., Rocher, M., and Tzanos, D.: The Global Land Carbon Cycle Simulated With ISBA-CTRIP: Improvements Over the Last Decade, J. Adv. Model Earth Sy., 12, e2019MS001886, https://doi.org/10.1029/2019MS001886, 2020.
Denman, K. L., Brasseur, G., Chidthaisong, A., Ciais, P., Cox, P. M., Dickinson, R. E., Hauglustaine, D., Heinze, C., Holland, E., Jacob, D., Lohmann, U., Ramachandran, S., Leite da Silva Dias, P., Wofsy, S. C., and Zhang, X.: Couplings Between Changes in the Climate System and Biogeochemistry, in: Climate Change 2007: The Physical Science Basis. Contribution of Working Group I to the Fourth Assessment Report of the Intergovernmental Panel on Climate Change, edited by: Solomon, S., Qin, D., Manning, M., Marquis, M., Averyt, K., Tignor, M. M. B., Miller, H. L., and Chen, Z. L., Cambridge University Press, Cambridge, UK and New York, USA, 499–587, 2007.
Denvil-Sommer, A., Gehlen, M., Vrac, M., and Mejia, C.: LSCE-FFNN-v1: a two-step neural network model for the reconstruction of surface ocean pCO2 over the global ocean, Geosci. Model Dev., 12, 2091–2105, https://doi.org/10.5194/gmd-12-2091-2019, 2019.
DeVries, T.: The oceanic anthropogenic CO2 sink: Storage, air-sea fluxes, and transports over the industrial era, Global Biogeochem. Cy., 28, 631–647, https://doi.org/10.1002/2013GB004739, 2014.
DeVries, T., Holzer, M., and Primeau, F.: Recent increase in oceanic carbon uptake driven by weaker upper-ocean overturning, Nature, 542, 215–218, https://doi.org/10.1038/nature21068, 2017.
DeVries, T., Quéré, C. L., Andrews, O., Berthet, S., Hauck, J., Ilyina, T., Landschützer, P., Lenton, A., Lima, I. D., Nowicki, M., Schwinger, J., and Séférian, R.: Decadal trends in the ocean carbon sink, P. Natl. Acad. Sci. USA, 116, 11646–11651, https://doi.org/10.1073/pnas.1900371116, 2019.
Dickson, A. G., Sabine, C. L., and Christian, J. R.: Guide to best practices for ocean CO2 measurement, Sidney, British Columbia, North Pacific Marine Science Organization, 191 pp., PICES Special Publication 3, IOCCP Report 8, https://doi.org/10.25607/OBP-1342, 2007.
Di Sarra, A. G., Karion, A., Arlyn Andrews, Colomb, A., Scheeren, B., Viner, B., Myhre, C. L., Miller, C. E., Plass-Duelmer, C., Plass-Duelmer, C., Sloop, C. D., Sweeney, C., Kubistin, D., Jaffe, D., Dlugokencky, E., Vitkova, G., Manca, G., Huilin Chen, Lehner, I., Mammarella, I., Pichon, J. M., Müller-Williams, J., Miller, J. B., Lee, J., Hatakka, J., Holst, J., Kominkova, K., McKain, K., Thoning, K., Tørseth, K., Emmenegger, L., Sha, M. K., Delmotte, M., Fischer, M. L., Schumacher, M., Leuenberger, M., Steinbacher, M., De Mazière, M., Lindauer, M., Mölder, M., Heliasz, M., Marek, M. V., Ramonet, M., Lopez, M., Laurent, O., Hermanssen, O., Trisolino, P., Cristofanelli, P., Smith, P., Bakwin, P., Bergamaschi, P., Keronen, P., Tans, P., Piacentino, S., Biraud, S. C., Conil, S., De Wekker, S., Biermann, T., Laurila, T., Aalto, T., and Kazan, V.: Multi-laboratory compilation of atmospheric carbon dioxide data for the years 2020–2021; obspack_CO2_1_NRT_v6.1.1_2021-05-17, NOAA Global Monitoring Laboratory [data set], https://doi.org/10.25925/20210517, 2021.
Dlugokencky, E. and Tans, P.: Trends in atmospheric carbon dioxide, National Oceanic and Atmospheric Administration, Earth System Research Laboratory (NOAA/ESRL), http://www.esrl.noaa.gov/gmd/ccgg/trends/global.html, last access: 16 November 2020.
Dlugokencky, E. and Tans, P.: Trends in atmospheric carbon dioxide, National Oceanic and Atmospheric Administration, Earth System Research Laboratory (NOAA/ESRL), http://www.esrl.noaa.gov/gmd/ccgg/trends/global.html, last access: 11 March 2022.
Doney, S. C., Lima, I., Feely, R. A., Glover, D. M., Lindsay, K., Mahowald, N., Moore, J. K., and Wanninkhof, R.: Mechanisms governing interannual variability in upper-ocean inorganic carbon system and air–sea CO2 fluxes: Physical climate and atmospheric dust, Deep-Sea Res. Pt. II, 56, 640–655, https://doi.org/10.1016/j.dsr2.2008.12.006, 2009.
Duce, R. A., LaRoche, J., Altieri, K., Arrigo, K. R., Baker, A. R., Capone, D. G., Cornell, S., Dentener, F., Galloway, J., Ganeshram, R. S., Geider, R. J., Jickells, T., Kuypers, M. M., Langlois, R., Liss, P. S., Liu, S. M., Middelburg, J. J., Moore, C. M., Nickovic, S., Oschlies, A., Pedersen, T., Prospero, J., Schlitzer, R., Seitzinger, S., Sorensen, L. L., Uematsu, M., Ulloa, O., Voss, M., Ward, B., and Zamora, L.: Impacts of Atmospheric Anthropogenic Nitrogen on the Open Ocean, Science, 320, 893–897, https://doi.org/10.1126/science.1150369, 2008.
Eakins, B. W. and Sharman, G. F.: National Geophysical Data Center: Volumes of the World's Oceans from ETOPO1, U.S. Department of Commerce, https://www.ngdc.noaa.gov/mgg/global/etopo1_ocean_volumes.html (last access: 11 March 2022), 2010.
Eggleston, H. S., Buendia, L., Miwa, K., Ngara, T., and Tanabe, K.: Volume 4: Agriculture, forestry and land use. in: 2006 IPCC guidelines for national greenhouse gas inventories, https://www.ipcc-nggip.iges.or.jp/public/2006gl/vol4.html (last access: 11 March 2022), 2006.
EIA: U.S. Energy Information Administration: Short-Term Energy Outlook, http://www.eia.gov/forecasts/steo/outlook, last access: 11 March 2022.
Erb, K.-H., Kastner, T., Luyssaert, S., Houghton, R. A., Kuemmerle, T., Olofsson, P., and Haberl, H.: Bias in the attribution of forest carbon sinks, Nat. Clim. Change, 3, 854–856, https://doi.org/10.1038/nclimate2004, 2013.
Erb, K.-H., Kastner, T., Plutzar, C., Bais, A. L. S., Carvalhais, N., Fetzel, T., Gingrich, S., Haberl, H., Lauk, C., Niedertscheider, M., Pongratz, J., Thurner, M., and Luyssaert, S.: Unexpectedly large impact of forest management and grazing on global vegetation biomass, Nature, 553, 73–76, https://doi.org/10.1038/nature25138, 2018.
Eskander, S. M. S. U. and Fankhauser, S.: Reduction in greenhouse gas emissions from national climate legislation, Nat. Clim. Change, 10, 750–756, https://doi.org/10.1038/s41558-020-0831-z, 2020.
Etheridge, D. M., Steele, L. P., Langenfelds, R. L., Francey, R. J., Barnola, J.-M., and Morgan, V. I.: Natural and anthropogenic changes in atmospheric CO2 over the last 1000 years from air in Antarctic ice and firn, J. Geophys. Res., 101, 4115–4128, https://doi.org/10.1029/95JD03410, 1996.
Eyring, V., Bony, S., Meehl, G. A., Senior, C. A., Stevens, B., Stouffer, R. J., and Taylor, K. E.: Overview of the Coupled Model Intercomparison Project Phase 6 (CMIP6) experimental design and organization, Geosci. Model Dev., 9, 1937–1958, https://doi.org/10.5194/gmd-9-1937-2016, 2016.
Fan, L., Wigneron, J.-P., Ciais, P., Chave, J., Brandt, M., Fensholt, R., Saatchi, S. S., Bastos, A., Al-Yaari, A., Hufkens, K., Qin, Y., Xiao, X., Chen, C., Myneni, R. B., Fernandez-Moran, R., Mialon, A., Rodriguez-Fernandez, N. J., Kerr, Y., Tian, F., and Peñuelas, J.: Satellite-observed pantropical carbon dynamics, Nat. Plants, 5, 944–951, https://doi.org/10.1038/s41477-019-0478-9, 2019.
FAO: Global Forest Resources Assessment 2020: Main report, FAO, Rome, Italy, 184 pp., https://doi.org/10.4060/ca9825en, 2020.
FAO: FAOSTAT Statistical Database, domains Climate Change, http://www.fao.org/faostat/en/#data/GT (last access: 11 March 2022), 2021.
FAOSTAT: FAOSTAT: Food and Agriculture Organization Statistics Division, http://faostat.fao.org/ (last access: 11 March 2022), 2021.
FAO/UNEP: Food and Agriculture Organisation/United Nations Environment Programme: The state of food and agriculture 1981, https://www.fao.org/3/ap661e/ap661e.pdf (last access: 11 March 2022), 1981.
Fay, A. R. and McKinley, G. A.: Global open-ocean biomes: mean and temporal variability, Earth Syst. Sci. Data, 6, 273–284, https://doi.org/10.5194/essd-6-273-2014, 2014.
Fay, A. R., Gregor, L., Landschützer, P., McKinley, G. A., Gruber, N., Gehlen, M., Iida, Y., Laruelle, G. G., Rödenbeck, C., Roobaert, A., and Zeng, J.: SeaFlux: harmonization of air–sea CO2 fluxes from surface pCO2 data products using a standardized approach, Earth Syst. Sci. Data, 13, 4693–4710, https://doi.org/10.5194/essd-13-4693-2021, 2021.
Feng, L., Palmer, P. I., Bösch, H., and Dance, S.: Estimating surface CO2 fluxes from space-borne CO2 dry air mole fraction observations using an ensemble Kalman Filter, Atmospheric Chem. Phys., 9, 2619–2633, https://doi.org/10.5194/acp-9-2619-2009, 2009.
Feng, L., Palmer, P. I., Parker, R. J., Deutscher, N. M., Feist, D. G., Kivi, R., Morino, I., and Sussmann, R.: Estimates of European uptake of CO2 inferred from GOSAT XCO2 retrievals: sensitivity to measurement bias inside and outside Europe, Atmos. Chem. Phys., 16, 1289–1302, https://doi.org/10.5194/acp-16-1289-2016, 2016.
Feng, Y., Zeng, Z., Searchinger, T. D., Ziegler, A. D., Wu, J., Wang, D., He, X., Elsen, P. R., Ciais, P., Xu, R., Guo, Z., Peng, L., Tao, Y., Spracklen, D. V., Holden, J., Liu, X., Zheng, Y., Xu, P., Chen, J., Jiang, X., Song, X.-P., Lakshmi, V., Wood, E. F., and Zheng, C.: Doubling of annual forest carbon loss over the tropics during the early twenty-first century, Nat. Sustain., https://doi.org/10.1038/s41893-022-00854-3, 2022.
Friedlingstein, P., Houghton, R. A., Marland, G., Hackler, J., Boden, T. A., Conway, T. J., Canadell, J. G., Raupach, M. R., Ciais, P., and Le Quéré, C.: Update on CO2 emissions, Nat. Geosci., 3, 811–812, https://doi.org/10.1038/ngeo1022, 2010.
Friedlingstein, P., Andrew, R. M., Rogelj, J., Peters, G. P., Canadell, J. G., Knutti, R., Luderer, G., Raupach, M. R., Schaeffer, M., van Vuuren, D. P., and Le Quéré, C.: Persistent growth of CO2 emissions and implications for reaching climate targets, Nat. Geosci., 7, 709–715, https://doi.org/10.1038/ngeo2248, 2014.
Friedlingstein, P., Jones, M. W., O'Sullivan, M., Andrew, R. M., Hauck, J., Peters, G. P., Peters, W., Pongratz, J., Sitch, S., Le Quéré, C., Bakker, D. C. E., Canadell, J. G., Ciais, P., Jackson, R. B., Anthoni, P., Barbero, L., Bastos, A., Bastrikov, V., Becker, M., Bopp, L., Buitenhuis, E., Chandra, N., Chevallier, F., Chini, L. P., Currie, K. I., Feely, R. A., Gehlen, M., Gilfillan, D., Gkritzalis, T., Goll, D. S., Gruber, N., Gutekunst, S., Harris, I., Haverd, V., Houghton, R. A., Hurtt, G., Ilyina, T., Jain, A. K., Joetzjer, E., Kaplan, J. O., Kato, E., Klein Goldewijk, K., Korsbakken, J. I., Landschützer, P., Lauvset, S. K., Lefèvre, N., Lenton, A., Lienert, S., Lombardozzi, D., Marland, G., McGuire, P. C., Melton, J. R., Metzl, N., Munro, D. R., Nabel, J. E. M. S., Nakaoka, S.-I., Neill, C., Omar, A. M., Ono, T., Peregon, A., Pierrot, D., Poulter, B., Rehder, G., Resplandy, L., Robertson, E., Rödenbeck, C., Séférian, R., Schwinger, J., Smith, N., Tans, P. P., Tian, H., Tilbrook, B., Tubiello, F. N., van der Werf, G. R., Wiltshire, A. J., and Zaehle, S.: Global Carbon Budget 2019, Earth Syst. Sci. Data, 11, 1783–1838, https://doi.org/10.5194/essd-11-1783-2019, 2019.
Friedlingstein, P., O'Sullivan, M., Jones, M. W., Andrew, R. M., Hauck, J., Olsen, A., Peters, G. P., Peters, W., Pongratz, J., Sitch, S., Le Quéré, C., Canadell, J. G., Ciais, P., Jackson, R. B., Alin, S., Aragão, L. E. O. C., Arneth, A., Arora, V., Bates, N. R., Becker, M., Benoit-Cattin, A., Bittig, H. C., Bopp, L., Bultan, S., Chandra, N., Chevallier, F., Chini, L. P., Evans, W., Florentie, L., Forster, P. M., Gasser, T., Gehlen, M., Gilfillan, D., Gkritzalis, T., Gregor, L., Gruber, N., Harris, I., Hartung, K., Haverd, V., Houghton, R. A., Ilyina, T., Jain, A. K., Joetzjer, E., Kadono, K., Kato, E., Kitidis, V., Korsbakken, J. I., Landschützer, P., Lefèvre, N., Lenton, A., Lienert, S., Liu, Z., Lombardozzi, D., Marland, G., Metzl, N., Munro, D. R., Nabel, J. E. M. S., Nakaoka, S.-I., Niwa, Y., O'Brien, K., Ono, T., Palmer, P. I., Pierrot, D., Poulter, B., Resplandy, L., Robertson, E., Rödenbeck, C., Schwinger, J., Séférian, R., Skjelvan, I., Smith, A. J. P., Sutton, A. J., Tanhua, T., Tans, P. P., Tian, H., Tilbrook, B., van der Werf, G., Vuichard, N., Walker, A. P., Wanninkhof, R., Watson, A. J., Willis, D., Wiltshire, A. J., Yuan, W., Yue, X., and Zaehle, S.: Global Carbon Budget 2020, Earth Syst. Sci. Data, 12, 3269–3340, https://doi.org/10.5194/essd-12-3269-2020, 2020.
Friedlingstein, P., Jones, M. W., O'Sullivan, M., Andrew, R. M., Bakker, D. C. E., Hauck, J., Le Quéré, C., Peters, G. P., Peters, W., Pongratz, J., Sitch, S., Canadell, J. G., Ciais, P., Jackson, R. B., Anthoni, P., Bates, N. R., Becker, M., Bopp, L., Tuyet, T., Chau, T., Chevallier, F., Chini, L. P., Cronin, M., Currie, K. I., Decharme, B., Djeutchouang, L. M., Dou, X., Evans, W., Feely, R. A., Feng, L., Gasser, T., Gilfillan, D., Gkritzalis, T., Grassi, G., Gregor, L., Gruber, N., Gürses, Ö., Harris, I., Houghton, R. A., Hurtt, G. C., Iida, Y., Ilyina, T., Luijkx, I. T., Jain, A. K., Jones, S. D., Kato, E., Kennedy, D., Klein Goldewijk, K., Knauer, J., Korsbakken, J. I., Körtzinger, A., Landschützer, P., Lauvset, S. K., Lefèvre, N., Lienert, S., Liu, J., Marland, G., McGuire, P. C., Melton, J. R., Munro, D. R., Nabel, J. E. M. S., Nakaoka, S.-I., Niwa, Y., Ono, T., Pierrot, D., Poulter, B., Rehder, G., Resplandy, L., Robertson, E., Rödenbeck, C., Schwinger, J., Schwingshackl, C., Séférian, R., Sutton, A. J., Tanhua, T., Tans, P., Tian, H., Tilbrook, B., Tubiello, F., van der Werf, G. R., Vuichard, N., Wanninkhof, R., Watson, A. J., Willis, D., Wiltshire, A. J., Wenping Yuan, Yue, C., Yue, X., Zaehle, S., and Zeng, J.: Supplemental data of the Global Carbon Budget 2021, ICOS-ERIC Carbon Portal [data set], https://doi.org/10.18160/gcp-2021, 2021.
Gasser, T. and Ciais, P.: A theoretical framework for the net land-to-atmosphere CO2 flux and its implications in the definition of “emissions from land-use change”, Earth Syst. Dynam., 4, 171–186, https://doi.org/10.5194/esd-4-171-2013, 2013.
Gasser, T., Crepin, L., Quilcaille, Y., Houghton, R. A., Ciais, P., and Obersteiner, M.: Historical CO2 emissions from land use and land cover change and their uncertainty, Biogeosciences, 17, 4075–4101, https://doi.org/10.5194/bg-17-4075-2020, 2020.
Gaubert, B., Stephens, B. B., Basu, S., Chevallier, F., Deng, F., Kort, E. A., Patra, P. K., Peters, W., Rödenbeck, C., Saeki, T., Schimel, D., Van der Laan-Luijkx, I., Wofsy, S., and Yin, Y.: Global atmospheric CO2 inverse models converging on neutral tropical land exchange, but disagreeing on fossil fuel and atmospheric growth rate, Biogeosciences, 16, 117–134, https://doi.org/10.5194/bg-16-117-2019, 2019.
Giglio, L., Schroeder, W., and Justice, C. O.: The collection 6 MODIS active fire detection algorithm and fire products, Remote Sens. Environ., 178, 31–41, https://doi.org/10.1016/j.rse.2016.02.054, 2016.
Gilfillan, D. and Marland, G.: CDIAC-FF: global and national CO2 emissions from fossil fuel combustion and cement manufacture: 1751–2017, Earth Syst. Sci. Data, 13, 1667–1680, https://doi.org/10.5194/essd-13-1667-2021, 2021.
Gloege, L., McKinley, G. A., Landschützer, P., Fay, A. R., Frölicher, T. L., Fyfe, J. C., Ilyina, T., Jones, S., Lovenduski, N. S., Rodgers, K. B., Schlunegger, S., and Takano, Y.: Quantifying Errors in Observationally Based Estimates of Ocean Carbon Sink Variability, Global Biogeochem. Cy., 35, e2020GB006788, https://doi.org/10.1029/2020GB006788, 2021.
Goddijn-Murphy, L. M., Woolf, D. K., Land, P. E., Shutler, J. D., and Donlon, C.: The OceanFlux Greenhouse Gases methodology for deriving a sea surface climatology of CO2 fugacity in support of air–sea gas flux studies, Ocean Sci., 11, 519–541, https://doi.org/10.5194/os-11-519-2015, 2015.
Golar, G., Malik, A., Muis, H., Herman, A., Nurudin, N., and Lukman, L.: The social-economic impact of COVID-19 pandemic: implications for potential forest degradation, Heliyon, 6, e05354, https://doi.org/10.1016/j.heliyon.2020.e05354, 2020.
Grassi, G., House, J., Kurz, W. A., Cescatti, A., Houghton, R. A., Peters, G. P., Sanz, M. J., Viñas, R. A., Alkama, R., Arneth, A., Bondeau, A., Dentener, F., Fader, M., Federici, S., Friedlingstein, P., Jain, A. K., Kato, E., Koven, C. D., Lee, D., Nabel, J. E. M. S., Nassikas, A. A., Perugini, L., Rossi, S., Sitch, S., Viovy, N., Wiltshire, A., and Zaehle, S.: Reconciling global-model estimates and country reporting of anthropogenic forest CO2 sinks, Nat. Clim. Change, 8, 914–920, https://doi.org/10.1038/s41558-018-0283-x, 2018.
Grassi, G., Stehfest, E., Rogelj, J., van Vuuren, D., Cescatti, A., House, J., Nabuurs, G.-J., Rossi, S., Alkama, R., Viñas, R. A., Calvin, K., Ceccherini, G., Federici, S., Fujimori, S., Gusti, M., Hasegawa, T., Havlik, P., Humpenöder, F., Korosuo, A., Perugini, L., Tubiello, F. N., and Popp, A.: Critical adjustment of land mitigation pathways for assessing countries' climate progress, Nat. Clim. Chang., 11, 425–434, https://doi.org/10.1038/s41558-021-01033-6, 2021.
Gregg, J. S., Andres, R. J., and Marland, G.: China: Emissions pattern of the world leader in CO2 emissions from fossil fuel consumption and cement production, Geophys. Res. Lett., 35, L08806, https://doi.org/10.1029/2007GL032887, 2008.
Gregor, L. and Gruber, N.: OceanSODA-ETHZ: a global gridded data set of the surface ocean carbonate system for seasonal to decadal studies of ocean acidification, Earth Syst. Sci. Data, 13, 777–808, https://doi.org/10.5194/essd-13-777-2021, 2021.
Gregor, L., Lebehot, A. D., Kok, S., and Scheel Monteiro, P. M.: A comparative assessment of the uncertainties of global surface ocean CO2 estimates using a machine-learning ensemble (CSIR-ML6 version 2019a) – have we hit the wall?, Geosci. Model Dev., 12, 5113–5136, https://doi.org/10.5194/gmd-12-5113-2019, 2019.
Gruber, N., Gloor, M., Mikaloff Fletcher, S. E., Doney, S. C., Dutkiewicz, S., Follows, M. J., Gerber, M., Jacobson, A. R., Joos, F., Lindsay, K., Menemenlis, D., Mouchet, A., Müller, S. A., Sarmiento, J. L., and Takahashi, T.: Oceanic sources, sinks, and transport of atmospheric CO2, Global Biogeochem. Cy., 23, https://doi.org/10.1029/2008GB003349, 2009.
Gruber, N., Clement, D., Carter, B. R., Feely, R. A., van Heuven, S., Hoppema, M., Ishii, M., Key, R. M., Kozyr, A., Lauvset, S. K., Lo Monaco, C., Mathis, J. T., Murata, A., Olsen, A., Perez, F. F., Sabine, C. L., Tanhua, T., and Wanninkhof, R.: The oceanic sink for anthropogenic CO2 from 1994 to 2007, 363, 1193–1199, https://doi.org/10.1126/science.aau5153, 2019.
Gruère, G. and Brooks, J.: Viewpoint: Characterising early agricultural and food policy responses to the outbreak of COVID-19, Food Policy, 100, 102017, https://doi.org/10.1016/j.foodpol.2020.102017, 2021.
Guan, D., Liu, Z., Geng, Y., Lindner, S., and Hubacek, K.: The gigatonne gap in China's carbon dioxide inventories, Nat. Clim. Change, 2, 672–675, https://doi.org/10.1038/nclimate1560, 2012.
Guo, R., Wang, J., Bing, L., Tong, D., Ciais, P., Davis, S. J., Andrew, R. M., Xi, F., and Liu, Z.: Global CO2 uptake by cement from 1930 to 2019, Earth Syst. Sci. Data, 13, 1791–1805, https://doi.org/10.5194/essd-13-1791-2021, 2021.
Gütschow, J., Jeffery, M. L., Gieseke, R., Gebel, R., Stevens, D., Krapp, M., and Rocha, M.: The PRIMAP-hist national historical emissions time series, Earth Syst. Sci. Data, 8, 571–603, https://doi.org/10.5194/essd-8-571-2016, 2016.
Gütschow, J., Günther, A., and Pflüger, M.: The PRIMAP-hist national historical emissions time series (1750–2019) v2.3.1, Zenodo, https://doi.org/10.5281/zenodo.5494497, 2021.
Hall, B. D., Crotwell, A. M., Kitzis, D. R., Mefford, T., Miller, B. R., Schibig, M. F., and Tans, P. P.: Revision of the World Meteorological Organization Global Atmosphere Watch (WMO/GAW) CO2 calibration scale, 14, 3015–3032, https://doi.org/10.5194/amt-14-3015-2021, 2021.
Hansen, M. C., Potapov, P. V., Moore, R., Hancher, M., Turubanova, S. A., Tyukavina, A., Thau, D., Stehman, S. V., Goetz, S. J., Loveland, T. R., Kommareddy, A., Egorov, A., Chini, L., Justice, C. O., and Townshend, J. R. G.: High-Resolution Global Maps of 21st-Century Forest Cover Change, Science, 342, 850–853, https://doi.org/10.1126/science.1244693, 2013.
Hansis, E., Davis, S. J., and Pongratz, J.: Relevance of methodological choices for accounting of land use change carbon fluxes, Global Biogeochem. Cy., 29, 1230–1246, https://doi.org/10.1002/2014GB004997, 2015.
Harris, I., Jones, P. D., Osborn, T. J., and Lister, D. H.: Updated high-resolution grids of monthly climatic observations – the CRU TS3.10 Dataset, Int. J. Climatol., 34, 623–642, https://doi.org/10.1002/joc.3711, 2014.
Harris, I., Osborn, T. J., Jones, P., and Lister, D.: Version 4 of the CRU TS monthly high-resolution gridded multivariate climate dataset, Sci. Data, 7, 109, https://doi.org/10.1038/s41597-020-0453-3, 2020.
Hauck, J., Zeising, M., Le Quéré, C., Gruber, N., Bakker, D. C. E., Bopp, L., Chau, T. T. T., Gürses, Ö., Ilyina, T., Landschützer, P., Lenton, A., Resplandy, L., Rödenbeck, C., Schwinger, J., and Séférian, R.: Consistency and Challenges in the Ocean Carbon Sink Estimate for the Global Carbon Budget, Front. Mar. Sci., 7, 571720, https://doi.org/10.3389/fmars.2020.571720, 2020.
Haverd, V., Smith, B., Nieradzik, L., Briggs, P. R., Woodgate, W., Trudinger, C. M., Canadell, J. G., and Cuntz, M.: A new version of the CABLE land surface model (Subversion revision r4601) incorporating land use and land cover change, woody vegetation demography, and a novel optimisation-based approach to plant coordination of photosynthesis, Geosci. Model Dev., 11, 2995–3026, https://doi.org/10.5194/gmd-11-2995-2018, 2018.
Heinimann, A., Mertz, O., Frolking, S., Christensen, A. E., Hurni, K., Sedano, F., Chini, L. P., Sahajpal, R., Hansen, M., and Hurtt, G.: A global view of shifting cultivation: Recent, current, and future extent, PLOS ONE, 12, e0184479, https://doi.org/10.1371/journal.pone.0184479, 2017.
Hertwich, E. G. and Peters, G. P.: Carbon Footprint of Nations: A Global, Trade-Linked Analysis, Environ. Sci. Technol., 43, 6414–6420, https://doi.org/10.1021/es803496a, 2009.
Hickler, T., Smith, B., Prentice, I. C., Mjöfors, K., Miller, P., Arneth, A., and Sykes, M. T.: CO2 fertilization in temperate FACE experiments not representative of boreal and tropical forests, 14, Glob. Change Biol., 1531–1542, https://doi.org/10.1111/j.1365-2486.2008.01598.x, 2008.
Ho, D. T., Wanninkhof, R., Schlosser, P., Ullman, D. S., Hebert, D., and Sullivan, K. F.: Toward a universal relationship between wind speed and gas exchange: Gas transfer velocities measured with 3He/SF6 during the Southern Ocean Gas Exchange Experiment, J. Geophys. Res.-Oceans, 116, C00F04, https://doi.org/10.1029/2010JC006854, 2011.
Hoesly, R. M., Smith, S. J., Feng, L., Klimont, Z., Janssens-Maenhout, G., Pitkanen, T., Seibert, J. J., Vu, L., Andres, R. J., Bolt, R. M., Bond, T. C., Dawidowski, L., Kholod, N., Kurokawa, J.-I., Li, M., Liu, L., Lu, Z., Moura, M. C. P., O'Rourke, P. R., and Zhang, Q.: Historical (1750–2014) anthropogenic emissions of reactive gases and aerosols from the Community Emissions Data System (CEDS), Geosci. Model Dev., 11, 369–408, https://doi.org/10.5194/gmd-11-369-2018, 2018.
Hong, C., Burney, J. A., Pongratz, J., Nabel, J. E. M. S., Mueller, N. D., Jackson, R. B., and Davis, S. J.: Global and regional drivers of land-use emissions in 1961–2017, Nature, 589, 554–561, https://doi.org/10.1038/s41586-020-03138-y, 2021.
Hooijer, A., Page, S., Canadell, J. G., Silvius, M., Kwadijk, J., Wösten, H., and Jauhiainen, J.: Current and future CO2 emissions from drained peatlands in Southeast Asia, Biogeosciences, 7, 1505–1514, https://doi.org/10.5194/bg-7-1505-2010, 2010.
Houghton, R. A.: Why are estimates of the terrestrial carbon balance so different?, Glob. Change Biol., 9, 500–509, https://doi.org/10.1046/j.1365-2486.2003.00620.x, 2003.
Houghton, R. A. and Nassikas, A. A.: Global and regional fluxes of carbon from land use and land cover change 1850-2015: Carbon Emissions From Land Use, Global Biogeochem. Cy., 31, 456–472, https://doi.org/10.1002/2016GB005546, 2017.
Houghton, R. A., House, J. I., Pongratz, J., van der Werf, G. R., DeFries, R. S., Hansen, M. C., Le Quéré, C., and Ramankutty, N.: Carbon emissions from land use and land-cover change, Biogeosciences, 9, 5125–5142, https://doi.org/10.5194/bg-9-5125-2012, 2012.
Hubau, W., Lewis, S. L., Phillips, O. L., Affum-Baffoe, K., Beeckman, H., Cuní-Sanchez, A., Daniels, A. K., Ewango, C. E. N., Fauset, S., Mukinzi, J. M., Sheil, D., Sonké, B., Sullivan, M. J. P., Sunderland, T. C. H., Taedoumg, H., Thomas, S. C., White, L. J. T., Abernethy, K. A., Adu-Bredu, S., Amani, C. A., Baker, T. R., Banin, L. F., Baya, F., Begne, S. K., Bennett, A. C., Benedet, F., Bitariho, R., Bocko, Y. E., Boeckx, P., Boundja, P., Brienen, R. J. W., Brncic, T., Chezeaux, E., Chuyong, G. B., Clark, C. J., Collins, M., Comiskey, J. A., Coomes, D. A., Dargie, G. C., de Haulleville, T., Kamdem, M. N. D., Doucet, J.-L., Esquivel- Muelbert, A., Feldpausch, T. R., Fofanah, A., Foli, E. G., Gilpin, M., Gloor, E., Gonmadje, C., Gourlet-Fleury, S., Hall, J. S., Hamilton, A. C., Harris, D. J., Hart, T. B., Hockemba, M. B. N., Hladik, A., Ifo, S. A., Jeffery, K. J., Jucker, T., Yakusu, E. K., Kearsley, E., Kenfack, D., Koch, A., Leal, M. E., Levesley, A., Lindsell, J. A., Lisingo, J., Lopez-Gonzalez, G., Lovett, J. C., Makana, J.-R., Malhi, Y., Marshall, A. R., Martin, J., Mar- tin, E. H., Mbayu, F. M., Medjibe, V. P., Mihindou, V., Mitchard, E. T. A., Moore, S., Munishi, P. K. T., Bengone, N. N., Ojo, L., Ondo, F. E., Peh, K. S.-H., Pickavance, G. C., Poulsen, A. D., Poulsen, J. R., Qie, L., Reitsma, J., Rovero, F., Swaine, M. D., Talbot, J., Taplin, J., Taylor, D. M., Thomas, D. W., Toirambe, B., Mukendi, J. T., Tuagben, D., Umunay, P. M., van der Heijden, G. M. F., Verbeeck, H., Vleminckx, J., Willcock, S., Wöll, H., Woods, J. T., and Zemagho, L.: Asynchronous carbon sink saturation in African and Amazonian tropical forests, Nature, 579, 80–87, https://doi.org/10.1038/s41586-020-2035-0, 2020.
Hugelius, G., Bockheim, J. G., Camill, P., Elberling, B., Grosse, G., Harden, J. W., Johnson, K., Jorgenson, T., Koven, C. D., Kuhry, P., Michaelson, G., Mishra, U., Palmtag, J., Ping, C.-L., O'Donnell, J., Schirrmeister, L., Schuur, E. A. G., Sheng, Y., Smith, L. C., Strauss, J., and Yu, Z.: A new data set for estimating organic carbon storage to 3 m depth in soils of the northern circumpolar permafrost region, Earth Syst. Sci. Data, 5, 393–402, https://doi.org/10.5194/essd-5-393-2013, 2013.
Humphrey, V., Zscheischler, J., Ciais, P., Gudmundsson, L., Sitch, S., and Seneviratne, S. I.: Sensitivity of atmospheric CO2 growth rate to observed changes in terrestrial water storage, Nature, 560, 628–631, https://doi.org/10.1038/s41586-018-0424-4, 2018.
Humphrey, V., Berg, A., Ciais, P., Gentine, P., Jung, M., Reichstein, M., Seneviratne, S. I., and Frankenberg, C.: Soil moisture–atmosphere feedback dominates land carbon uptake variability, Nature, 592, 65–69, https://doi.org/10.1038/s41586-021-03325-5, 2021.
Huntzinger, D. N., Michalak, A. M., Schwalm, C., Ciais, P., King, A. W., Fang, Y., Schaefer, K., Wei, Y., Cook, R. B., Fisher, J. B., Hayes, D., Huang, M., Ito, A., Jain, A. K., Lei, H., Lu, C., Maignan, F., Mao, J., Parazoo, N., Peng, S., Poulter, B., Ricciuto, D., Shi, X., Tian, H., Wang, W., Zeng, N., and Zhao, F.: Uncertainty in the response of terrestrial carbon sink to environmental drivers undermines carbon-climate feedback predictions, Sci. Rep., 7, 4765, https://doi.org/10.1038/s41598-017-03818-2, 2017.
Hurtt, G., Chini, L., Sahajpal, R., Frolking, S., Bodirsky, B. L., Calvin, K., Doelman, J., Fisk, J., Fujimori, S., Klein Goldewijk, K., Hasegawa, T., Havlik, P., Heinimann, A., Humpenöder, F., Jungclaus, J., Kaplan, J., Krisztin, T., Lawrence, D., Lawrence, P., Mertz, O., Pongratz, J., Popp, A., Riahi, K., Shevliakova, E., Stehfest, E., Thornton, P., van Vuuren, D., and Zhang, X.: input4MIPs.CMIP6.CMIP.UofMD.UofMD-landState-2-1-h, World Climate Research Programme [data set], https://doi.org/10.22033/ESGF/input4MIPs.1127, 2017.
Hurtt, G. C., Chini, L. P., Frolking, S., Betts, R. A., Feddema, J., Fischer, G., Fisk, J. P., Hibbard, K., Houghton, R. A., Janetos, A., Jones, C. D., Kindermann, G., Kinoshita, T., Klein Goldewijk, K., Riahi, K., Shevliakova, E., Smith, S., Stehfest, E., Thomson, A., Thornton, P., van Vuuren, D. P., and Wang, Y. P.: Harmonization of land-use scenarios for the period 1500–2100: 600 years of global gridded annual land-use transitions, wood harvest, and resulting secondary lands, Climatic Change, 109, 117–161, https://doi.org/10.1007/s10584-011-0153-2, 2011.
Hurtt, G. C., Chini, L., Sahajpal, R., Frolking, S., Bodirsky, B. L., Calvin, K., Doelman, J. C., Fisk, J., Fujimori, S., Klein Goldewijk, K., Hasegawa, T., Havlik, P., Heinimann, A., Humpenöder, F., Jungclaus, J., Kaplan, J. O., Kennedy, J., Krisztin, T., Lawrence, D., Lawrence, P., Ma, L., Mertz, O., Pongratz, J., Popp, A., Poulter, B., Riahi, K., Shevliakova, E., Stehfest, E., Thornton, P., Tubiello, F. N., van Vuuren, D. P., and Zhang, X.: Harmonization of global land use change and management for the period 850–2100 (LUH2) for CMIP6, Geosci. Model Dev., 13, 5425–5464, https://doi.org/10.5194/gmd-13-5425-2020, 2020.
IEA: International Energy Agency: Global Energy Review, https://www.iea.org/reports/global-energy-review-2021 (last access: 11 March 2022), 2021a.
IEA: International Energy Agency: World Energy Outlook, https://www.iea.org/reports/world-energy-outlook-2021 (last access: 11 March 2022), 2021b.
IEA/OECD: International Energy Agency/Organisation for Economic Cooperation and Development: CO2 emissions from fuel combustion, https://webstore.iea.org/CO2-emissions-from-fuel-combustion-2019-highlights (last access: 11 March 2022), 2019.
Iida, Y., Kojima, A., Takatani, Y., Nakano, T., Sugimoto, H., Midorikawa, T., and Ishii, M.: Trends in pCO2 and sea–air CO2 flux over the global open oceans for the last two decades, J. Oceanogr., 71, 637–661, https://doi.org/10.1007/s10872-015-0306-4, 2015.
Iida, Y., Takatani, Y., Kojima, A., and Ishii, M.: Global trends of ocean CO2 sink and ocean acidification: an observation-based reconstruction of surface ocean inorganic carbon variables, J. Oceanogr., 77, 323–358, https://doi.org/10.1007/s10872-020-00571-5, 2021.
Ilyina, T., Six, K. D., Segschneider, J., Maier-Reimer, E., Li, H., and Núñez-Riboni, I.: Global ocean biogeochemistry model HAMOCC: Model architecture and performance as component of the MPI-Earth system model in different CMIP5 experimental realizations: The Model Hamocc within Mpi-Esm in Cmip5, J. Adv. Model. Earth Sy., 5, 287–315, https://doi.org/10.1029/2012MS000178, 2013.
IMF: International Monetary Fund: World Economic Outlook, http://www.imf.org, last access: 11 March 2022.
Inness, A., Ades, M., Agustí-Panareda, A., Barré, J., Benedictow, A., Blechschmidt, A.-M., Dominguez, J. J., Engelen, R., Eskes, H., Flemming, J., Huijnen, V., Jones, L., Kipling, Z., Massart, S., Parrington, M., Peuch, V.-H., Razinger, M., Remy, S., Schulz, M., and Suttie, M.: The CAMS reanalysis of atmospheric composition, Atmos. Chem. Phys., 19, 3515–3556, https://doi.org/10.5194/acp-19-3515-2019, 2019.
Ito, A. and Inatomi, M.: Use of a process-based model for assessing the methane budgets of global terrestrial ecosystems and evaluation of uncertainty, Biogeosciences, 9, 759–773, https://doi.org/10.5194/bg-9-759-2012, 2012.
Jackson, R. B., Canadell, J. G., Le Quéré, C., Andrew, R. M., Korsbakken, J. I., Peters, G. P., and Nakicenovic, N.: Reaching peak emissions, Nat. Clim. Change, 6, 7–10, https://doi.org/10.1038/nclimate2892, 2016.
Jackson, R. B., Le Quéré, C., Andrew, R. M., Canadell, J. G., Korsbakken, J. I., Liu, Z., Peters, G. P., and Zheng, B.: Global energy growth is outpacing decarbonization, Environ. Res. Lett., 13, 120401, https://doi.org/10.1088/1748-9326/aaf303, 2018.
Jackson, R. B., Friedlingstein, P., Andrew, R. M., Canadell, J. G., Le Quéré, C., and Peters, G. P.: Persistent fossil fuel growth threatens the Paris Agreement and planetary health, Environ. Res. Lett., 14, 121001, https://doi.org/10.1088/1748-9326/ab57b3, 2019.
Jacobson, A. R., Mikaloff Fletcher, S. E., Gruber, N., Sarmiento, J. L., and Gloor, M.: A joint atmosphere-ocean inversion for surface fluxes of carbon dioxide: 1. Methods and global-scale fluxes: JOINT INVERSION-METHODS AND GLOBAL FLUXES, Global Biogeochem. Cy., 21, GB1019, https://doi.org/10.1029/2005GB002556, 2007.
Jain, A. K., Meiyappan, P., Song, Y., and House, J. I.: CO2 emissions from land-use change affected more by nitrogen cycle, than by the choice of land-cover data, Glob. Change Biol., 19, 2893–2906, https://doi.org/10.1111/gcb.12207, 2013.
Janssens-Maenhout, G., Crippa, M., Guizzardi, D., Muntean, M., Schaaf, E., Dentener, F., Bergamaschi, P., Pagliari, V., Olivier, J. G. J., Peters, J. A. H. W., van Aardenne, J. A., Monni, S., Doering, U., Petrescu, A. M. R., Solazzo, E., and Oreggioni, G. D.: EDGAR v4.3.2 Global Atlas of the three major greenhouse gas emissions for the period 1970–2012, Earth Syst. Sci. Data, 11, 959–1002, https://doi.org/10.5194/essd-11-959-2019, 2019.
JODI: Joint Organisations Data Initiative, https://www.jodidata.org, last access: 11 March 2022.
Jones, M. W., Andrew, R. M., Peters, G. P., Janssens-Maenhout, G., De-Gol, A. J., Ciais, P., Patra, P. K., Chevallier, F., and Le Quéré, C.: Gridded fossil CO2 emissions and related O2 combustion consistent with national inventories 1959–2018, Sci. Data, 8, 2, https://doi.org/10.1038/s41597-020-00779-6, 2021a.
Jones, M. W., Andrew, R. M., Peters, G. P., Janssens-Maenhout, G., De-Gol, A. J., Dou, X., Liu, Z., Ciais, P., Patra, P. K., Chevallier, F., and Le Quéré, C.: Gridded fossil CO2 emissions and related O2 combustion consistent with national inventories 1959–2020, Zenodo [data set], https://doi.org/10.5281/zenodo.5565199, 2021b.
Joos, F. and Spahni, R.: Rates of change in natural and anthropogenic radiative forcing over the past 20,000 years, P. Natl. Acad. Sci. USA, 105, 1425–1430, https://doi.org/10.1073/pnas.0707386105, 2008.
Jung, M., Reichstein, M., Ciais, P., Seneviratne, S. I., Sheffield, J., Goulden, M. L., Bonan, G., Cescatti, A., Chen, J., de Jeu, R., Dolman, A. J., Eugster, W., Gerten, D., Gianelle, D., Gobron, N., Heinke, J., Kimball, J., Law, B. E., Montagnani, L., Mu, Q., Mueller, B., Oleson, K., Papale, D., Richardson, A. D., Roupsard, O., Running, S., Tomelleri, E., Viovy, N., Weber, U., Williams, C., Wood, E., Zaehle, S., and Zhang, K.: Recent decline in the global land evapotranspiration trend due to limited moisture supply, Nature, 467, 951–954, https://doi.org/10.1038/nature09396, 2010.
Jung, M., Reichstein, M., Schwalm, C. R., Huntingford, C., Sitch, S., Ahlström, A., Arneth, A., Camps-Valls, G., Ciais, P., Friedlingstein, P., Gans, F., Ichii, K., Jain, A. K., Kato, E., Papale, D., Poulter, B., Raduly, B., Rödenbeck, C., Tramontana, G., Viovy, N., Wang, Y.-P., Weber, U., Zaehle, S., and Zeng, N.: Compensatory water effects link yearly global land CO2 sink changes to temperature, Nature, 541, 516–520, https://doi.org/10.1038/nature20780, 2017.
Kato, E., Kinoshita, T., Ito, A., Kawamiya, M., and Yamagata, Y.: Evaluation of spatially explicit emission scenario of land-use change and biomass burning using a process-based biogeochemical model, J. Land Use., 8, 104–122, https://doi.org/10.1080/1747423X.2011.628705, 2013.
Keeling, C. D., Bacastow, R. B., Bainbridge, A. E., Ekdahl, C. A., Guenther, P. R., Waterman, L. S., and Chin, J. F. S.: Atmospheric carbon dioxide variations at Mauna Loa Observatory, Hawaii, Tellus A., 28, 538–551, https://doi.org/10.1111/j.2153-3490.1976.tb00701.x, 1976.
Keeling, R. F. and Manning, A. C.: 5.15 – Studies of Recent Changes in Atmospheric O2 Content, in: Treatise on Geochemistry, 2nd Edn., edited by: Holland, H. D. and Turekian, K. K., Elsevier, Oxford, 385–404, https://doi.org/10.1016/B978-0-08-095975-7.00420-4, 2014.
Keppler, L. and Landschützer, P.: Regional Wind Variability Modulates the Southern Ocean Carbon Sink, Sci. Rep, 9, 7384, https://doi.org/10.1038/s41598-019-43826-y, 2019.
Khatiwala, S., Primeau, F., and Hall, T.: Reconstruction of the history of anthropogenic CO2 concentrations in the ocean, Nature, 462, 346–349, https://doi.org/10.1038/nature08526, 2009.
Khatiwala, S., Tanhua, T., Mikaloff Fletcher, S., Gerber, M., Doney, S. C., Graven, H. D., Gruber, N., McKinley, G. A., Murata, A., Ríos, A. F., and Sabine, C. L.: Global ocean storage of anthropogenic carbon, Biogeosciences, 10, 2169–2191, https://doi.org/10.5194/bg-10-2169-2013, 2013.
Klein Goldewijk, K., Beusen, A., Doelman, J., and Stehfest, E.: Anthropogenic land use estimates for the Holocene – HYDE 3.2, Earth Syst. Sci. Data, 9, 927–953, https://doi.org/10.5194/essd-9-927-2017, 2017a.
Klein Goldewijk, K., Dekker, S. C., and van Zanden, J. L.: Per-capita estimations of long-term historical land use and the consequences for global change research, J. Land Use Sci., 1747423X.2017.1354938, https://doi.org/10.1080/1747423X.2017.1354938, 2017b.
Kobayashi, S., Ota, Y., Harada, Y., Ebita, A., Moriya, M., Onoda, H., Onogi, K., Kamahori, H., Kobayashi, C., Endo, H., Miyaoka, K., and Takahashi, K.: The JRA-55 Reanalysis: General Specifications and Basic Characteristics, J. Meteorol. Soc. Jpn., 93, 5–48, https://doi.org/10.2151/jmsj.2015-001, 2015.
Korsbakken, J. I., Peters, G. P., and Andrew, R. M.: Uncertainties around reductions in China's coal use and CO2 emissions, Nat. Clim. Change, 6, 687–690, https://doi.org/10.1038/nclimate2963, 2016.
Krinner, G., Viovy, N., de Noblet-Ducoudré, N., Ogée, J., Polcher, J., Friedlingstein, P., Ciais, P., Sitch, S., and Prentice, I. C.: A dynamic global vegetation model for studies of the coupled atmosphere-biosphere system: DVGM for coupled climate studies, Global Biogeochem. Cy., 19, GB1015, https://doi.org/10.1029/2003GB002199, 2005.
Lacroix, F., Ilyina, T., and Hartmann, J.: Oceanic CO2 outgassing and biological production hotspots induced by pre-industrial river loads of nutrients and carbon in a global modeling approach, Biogeosciences, 17, 55–88, https://doi.org/10.5194/bg-17-55-2020, 2020.
Lacroix, F., Ilyina, T., Mathis, M., Laruelle, G. G., and Regnier, P.: Historical increases in land-derived nutrient inputs may alleviate effects of a changing physical climate on the oceanic carbon cycle, Glob. Change Biol., 27, 5491–5513, https://doi.org/10.1111/gcb.15822, 2021.
Landschützer, P., Gruber, N., Bakker, D. C. E., Schuster, U., Nakaoka, S., Payne, M. R., Sasse, T. P., and Zeng, J.: A neural network-based estimate of the seasonal to inter-annual variability of the Atlantic Ocean carbon sink, Biogeosciences, 10, 7793–7815, https://doi.org/10.5194/bg-10-7793-2013, 2013.
Landschützer, P., Gruber, N., Bakker, D. C. E., and Schuster, U.: Recent variability of the global ocean carbon sink, Global Biogeochem. Cy., 28, 927–949, https://doi.org/10.1002/2014GB004853, 2014.
Landschützer, P., Gruber, N., Haumann, F. A., Rödenbeck, C., Bakker, D. C. E., van Heuven, S., Hoppema, M., Metzl, N., Sweeney, C., Takahashi, T., Tilbrook, B., and Wanninkhof, R.: The reinvigoration of the Southern Ocean carbon sink, Science, 349, 1221–1224, https://doi.org/10.1126/science.aab2620, 2015.
Landschützer, P., Gruber, N., and Bakker, D. C. E.: Decadal variations and trends of the global ocean carbon sink: decadal air-sea CO2 flux variability, Global Biogeochem. Cy., 30, 1396–1417, https://doi.org/10.1002/2015GB005359, 2016.
Landschützer, P., Laruelle, G. G., Roobaert, A., and Regnier, P.: A uniform pCO2 climatology combining open and coastal oceans, Earth Syst. Sci. Data, 12, 2537–2553, https://doi.org/10.5194/essd-12-2537-2020, 2020.
Lasslop, G., Reichstein, M., Papale, D., Richardson, A. D., Arneth, A., Barr, A., Stoy, P., and Wohlfahrt, G.: Separation of net ecosystem exchange into assimilation and respiration using a light response curve approach: critical issues and global evaluation: Separation of NEE into GPP and RECO, Glob. Change Biol., 16, 187–208, https://doi.org/10.1111/j.1365-2486.2009.02041.x, 2010.
Lawrence, D. M., Fisher, R. A., Koven, C. D., Oleson, K. W., Swenson, S. C., Bonan, G., Collier, N., Ghimire, B., van Kampenhout, L., Kennedy, D., Kluzek, E., Lawrence, P. J., Li, F., Li, H., Lombardozzi, D., Riley, W. J., Sacks, W. J., Shi, M., Vertenstein, M., Wieder, W. R., Xu, C., Ali, A. A., Badger, A. M., Bisht, G., van den Broeke, M., Brunke, M. A., Burns, S. P., Buzan, J., Clark, M., Craig, A., Dahlin, K., Drewniak, B., Fisher, J. B., Flanner, M., Fox, A. M., Gentine, P., Hoffman, F., Keppel-Aleks, G., Knox, R., Kumar, S., Lenaerts, J., Leung, L. R., Lipscomb, W. H., Lu, Y., Pandey, A., Pelletier, J. D., Perket, J., Randerson, J. T., Ricciuto, D. M., Sanderson, B. M., Slater, A., Subin, Z. M., Tang, J., Thomas, R. Q., Val Martin, M., and Zeng, X.: The Community Land Model Version 5: Description of New Features, Benchmarking, and Impact of Forcing Uncertainty, J. Adv. Model. Earth Sy., 11, 4245–4287, https://doi.org/10.1029/2018MS001583, 2019.
Le Quéré, C., Rödenbeck, C., Buitenhuis, E. T., Conway, T. J., Langenfelds, R., Gomez, A., Labuschagne, C., Ramonet, M., Nakazawa, T., Metzl, N., Gillett, N., and Heimann, M.: Saturation of the Southern Ocean CO2 Sink Due to Recent Climate Change, Science, 316, 1735–1738, https://doi.org/10.1126/science.1136188, 2007.
Le Quéré, C., Raupach, M. R., Canadell, J. G., Marland, G., Bopp, L., Ciais, P., Conway, T. J., Doney, S. C., Feely, R. A., Foster, P., Friedlingstein, P., Gurney, K., Houghton, R. A., House, J. I., Huntingford, C., Levy, P. E., Lomas, M. R., Majkut, J., Metzl, N., Ometto, J. P., Peters, G. P., Prentice, I. C., Randerson, J. T., Running, S. W., Sarmiento, J. L., Schuster, U., Sitch, S., Takahashi, T., Viovy, N., van der Werf, G. R., and Woodward, F. I.: Trends in the sources and sinks of carbon dioxide, Nat. Geosci., 2, 831–836, https://doi.org/10.1038/ngeo689, 2009.
Le Quéré, C., Andres, R. J., Boden, T., Conway, T., Houghton, R. A., House, J. I., Marland, G., Peters, G. P., van der Werf, G. R., Ahlström, A., Andrew, R. M., Bopp, L., Canadell, J. G., Ciais, P., Doney, S. C., Enright, C., Friedlingstein, P., Huntingford, C., Jain, A. K., Jourdain, C., Kato, E., Keeling, R. F., Klein Goldewijk, K., Levis, S., Levy, P., Lomas, M., Poulter, B., Raupach, M. R., Schwinger, J., Sitch, S., Stocker, B. D., Viovy, N., Zaehle, S., and Zeng, N.: The global carbon budget 1959–2011, Earth Syst. Sci. Data, 5, 165–185, https://doi.org/10.5194/essd-5-165-2013, 2013.
Le Quéré, C., Peters, G. P., Andres, R. J., Andrew, R. M., Boden, T. A., Ciais, P., Friedlingstein, P., Houghton, R. A., Marland, G., Moriarty, R., Sitch, S., Tans, P., Arneth, A., Arvanitis, A., Bakker, D. C. E., Bopp, L., Canadell, J. G., Chini, L. P., Doney, S. C., Harper, A., Harris, I., House, J. I., Jain, A. K., Jones, S. D., Kato, E., Keeling, R. F., Klein Goldewijk, K., Körtzinger, A., Koven, C., Lefèvre, N., Maignan, F., Omar, A., Ono, T., Park, G.-H., Pfeil, B., Poulter, B., Raupach, M. R., Regnier, P., Rödenbeck, C., Saito, S., Schwinger, J., Segschneider, J., Stocker, B. D., Takahashi, T., Tilbrook, B., van Heuven, S., Viovy, N., Wanninkhof, R., Wiltshire, A., and Zaehle, S.: Global carbon budget 2013, Earth Syst. Sci. Data, 6, 235–263, https://doi.org/10.5194/essd-6-235-2014, 2014.
Le Quéré, C., Moriarty, R., Andrew, R. M., Peters, G. P., Ciais, P., Friedlingstein, P., Jones, S. D., Sitch, S., Tans, P., Arneth, A., Boden, T. A., Bopp, L., Bozec, Y., Canadell, J. G., Chini, L. P., Chevallier, F., Cosca, C. E., Harris, I., Hoppema, M., Houghton, R. A., House, J. I., Jain, A. K., Johannessen, T., Kato, E., Keeling, R. F., Kitidis, V., Klein Goldewijk, K., Koven, C., Landa, C. S., Landschützer, P., Lenton, A., Lima, I. D., Marland, G., Mathis, J. T., Metzl, N., Nojiri, Y., Olsen, A., Ono, T., Peng, S., Peters, W., Pfeil, B., Poulter, B., Raupach, M. R., Regnier, P., Rödenbeck, C., Saito, S., Salisbury, J. E., Schuster, U., Schwinger, J., Séférian, R., Segschneider, J., Steinhoff, T., Stocker, B. D., Sutton, A. J., Takahashi, T., Tilbrook, B., van der Werf, G. R., Viovy, N., Wang, Y.-P., Wanninkhof, R., Wiltshire, A., and Zeng, N.: Global carbon budget 2014, Earth Syst. Sci. Data, 7, 47–85, https://doi.org/10.5194/essd-7-47-2015, 2015a.
Le Quéré, C., Moriarty, R., Andrew, R. M., Canadell, J. G., Sitch, S., Korsbakken, J. I., Friedlingstein, P., Peters, G. P., Andres, R. J., Boden, T. A., Houghton, R. A., House, J. I., Keeling, R. F., Tans, P., Arneth, A., Bakker, D. C. E., Barbero, L., Bopp, L., Chang, J., Chevallier, F., Chini, L. P., Ciais, P., Fader, M., Feely, R. A., Gkritzalis, T., Harris, I., Hauck, J., Ilyina, T., Jain, A. K., Kato, E., Kitidis, V., Klein Goldewijk, K., Koven, C., Landschützer, P., Lauvset, S. K., Lefèvre, N., Lenton, A., Lima, I. D., Metzl, N., Millero, F., Munro, D. R., Murata, A., Nabel, J. E. M. S., Nakaoka, S., Nojiri, Y., O'Brien, K., Olsen, A., Ono, T., Pérez, F. F., Pfeil, B., Pierrot, D., Poulter, B., Rehder, G., Rödenbeck, C., Saito, S., Schuster, U., Schwinger, J., Séférian, R., Steinhoff, T., Stocker, B. D., Sutton, A. J., Takahashi, T., Tilbrook, B., van der Laan-Luijkx, I. T., van der Werf, G. R., van Heuven, S., Vandemark, D., Viovy, N., Wiltshire, A., Zaehle, S., and Zeng, N.: Global Carbon Budget 2015, Earth Syst. Sci. Data, 7, 349–396, https://doi.org/10.5194/essd-7-349-2015, 2015b.
Le Quéré, C., Andrew, R. M., Canadell, J. G., Sitch, S., Korsbakken, J. I., Peters, G. P., Manning, A. C., Boden, T. A., Tans, P. P., Houghton, R. A., Keeling, R. F., Alin, S., Andrews, O. D., Anthoni, P., Barbero, L., Bopp, L., Chevallier, F., Chini, L. P., Ciais, P., Currie, K., Delire, C., Doney, S. C., Friedlingstein, P., Gkritzalis, T., Harris, I., Hauck, J., Haverd, V., Hoppema, M., Klein Goldewijk, K., Jain, A. K., Kato, E., Körtzinger, A., Landschützer, P., Lefèvre, N., Lenton, A., Lienert, S., Lombardozzi, D., Melton, J. R., Metzl, N., Millero, F., Monteiro, P. M. S., Munro, D. R., Nabel, J. E. M. S., Nakaoka, S., O'Brien, K., Olsen, A., Omar, A. M., Ono, T., Pierrot, D., Poulter, B., Rödenbeck, C., Salisbury, J., Schuster, U., Schwinger, J., Séférian, R., Skjelvan, I., Stocker, B. D., Sutton, A. J., Takahashi, T., Tian, H., Tilbrook, B., van der Laan-Luijkx, I. T., van der Werf, G. R., Viovy, N., Walker, A. P., Wiltshire, A. J., and Zaehle, S.: Global Carbon Budget 2016, Earth Syst. Sci. Data, 8, 605–649, https://doi.org/10.5194/essd-8-605-2016, 2016.
Le Quéré, C., Andrew, R. M., Friedlingstein, P., Sitch, S., Pongratz, J., Manning, A. C., Korsbakken, J. I., Peters, G. P., Canadell, J. G., Jackson, R. B., Boden, T. A., Tans, P. P., Andrews, O. D., Arora, V. K., Bakker, D. C. E., Barbero, L., Becker, M., Betts, R. A., Bopp, L., Chevallier, F., Chini, L. P., Ciais, P., Cosca, C. E., Cross, J., Currie, K., Gasser, T., Harris, I., Hauck, J., Haverd, V., Houghton, R. A., Hunt, C. W., Hurtt, G., Ilyina, T., Jain, A. K., Kato, E., Kautz, M., Keeling, R. F., Klein Goldewijk, K., Körtzinger, A., Landschützer, P., Lefèvre, N., Lenton, A., Lienert, S., Lima, I., Lombardozzi, D., Metzl, N., Millero, F., Monteiro, P. M. S., Munro, D. R., Nabel, J. E. M. S., Nakaoka, S., Nojiri, Y., Padin, X. A., Peregon, A., Pfeil, B., Pierrot, D., Poulter, B., Rehder, G., Reimer, J., Rödenbeck, C., Schwinger, J., Séférian, R., Skjelvan, I., Stocker, B. D., Tian, H., Tilbrook, B., Tubiello, F. N., van der Laan-Luijkx, I. T., van der Werf, G. R., van Heuven, S., Viovy, N., Vuichard, N., Walker, A. P., Watson, A. J., Wiltshire, A. J., Zaehle, S., and Zhu, D.: Global Carbon Budget 2017, Earth Syst. Sci. Data, 10, 405–448, https://doi.org/10.5194/essd-10-405-2018, 2018a.
Le Quéré, C., Andrew, R. M., Friedlingstein, P., Sitch, S., Hauck, J., Pongratz, J., Pickers, P. A., Korsbakken, J. I., Peters, G. P., Canadell, J. G., Arneth, A., Arora, V. K., Barbero, L., Bastos, A., Bopp, L., Chevallier, F., Chini, L. P., Ciais, P., Doney, S. C., Gkritzalis, T., Goll, D. S., Harris, I., Haverd, V., Hoffman, F. M., Hoppema, M., Houghton, R. A., Hurtt, G., Ilyina, T., Jain, A. K., Johannessen, T., Jones, C. D., Kato, E., Keeling, R. F., Goldewijk, K. K., Landschützer, P., Lefèvre, N., Lienert, S., Liu, Z., Lombardozzi, D., Metzl, N., Munro, D. R., Nabel, J. E. M. S., Nakaoka, S., Neill, C., Olsen, A., Ono, T., Patra, P., Peregon, A., Peters, W., Peylin, P., Pfeil, B., Pierrot, D., Poulter, B., Rehder, G., Resplandy, L., Robertson, E., Rocher, M., Rödenbeck, C., Schuster, U., Schwinger, J., Séférian, R., Skjelvan, I., Steinhoff, T., Sutton, A., Tans, P. P., Tian, H., Tilbrook, B., Tubiello, F. N., van der Laan-Luijkx, I. T., van der Werf, G. R., Viovy, N., Walker, A. P., Wiltshire, A. J., Wright, R., Zaehle, S., and Zheng, B.: Global Carbon Budget 2018, Earth Syst. Sci. Data, 10, 2141–2194, https://doi.org/10.5194/essd-10-2141-2018, 2018b.
Le Quéré, C., Korsbakken, J. I., Wilson, C., Tosun, J., Andrew, R., Andres, R. J., Canadell, J. G., Jordan, A., Peters, G. P., and van Vuuren, D. P.: Drivers of declining CO2 emissions in 18 developed economies, Nat. Clim. Change, 9, 213–217, https://doi.org/10.1038/s41558-019-0419-7, 2019.
Le Quéré, C., Peters, G. P., Friedlingstein, P., Andrew, R. M., Canadell, J. G., Davis, S. J., Jackson, R. B., and Jones, M. W.: Fossil CO2 emissions in the post-COVID-19 era, Nat. Clim. Change, 11, 197–199, https://doi.org/10.1038/s41558-021-01001-0, 2021.
Li, W., Ciais, P., Peng, S., Yue, C., Wang, Y., Thurner, M., Saatchi, S. S., Arneth, A., Avitabile, V., Carvalhais, N., Harper, A. B., Kato, E., Koven, C., Liu, Y. Y., Nabel, J. E. M. S., Pan, Y., Pongratz, J., Poulter, B., Pugh, T. A. M., Santoro, M., Sitch, S., Stocker, B. D., Viovy, N., Wiltshire, A., Yousefpour, R., and Zaehle, S.: Land-use and land-cover change carbon emissions between 1901 and 2012 constrained by biomass observations, Biogeosciences, 14, 5053–5067, https://doi.org/10.5194/bg-14-5053-2017, 2017.
Liao, E., Resplandy, L., Liu, J., and Bowman, K. W.: Amplification of the Ocean Carbon Sink During El Niños: Role of Poleward Ekman Transport and Influence on Atmospheric CO2, Global Biogeochem. Cy., 34, e2020GB006574, https://doi.org/10.1029/2020GB006574, 2020.
Lienert, S. and Joos, F.: A Bayesian ensemble data assimilation to constrain model parameters and land-use carbon emissions, Biogeosciences, 15, 2909–2930, https://doi.org/10.5194/bg-15-2909-2018, 2018.
Liu, J., Baskaran, L., Bowman, K., Schimel, D., Bloom, A. A., Parazoo, N. C., Oda, T., Carroll, D., Menemenlis, D., Joiner, J., Commane, R., Daube, B., Gatti, L. V., McKain, K., Miller, J., Stephens, B. B., Sweeney, C., and Wofsy, S.: Carbon Monitoring System Flux Net Biosphere Exchange 2020 (CMS-Flux NBE 2020), Earth Syst. Sci. Data, 13, 299–330, https://doi.org/10.5194/essd-13-299-2021, 2021.
Liu, Z., Guan, D., Wei, W., Davis, S. J., Ciais, P., Bai, J., Peng, S., Zhang, Q., Hubacek, K., Marland, G., Andres, R. J., Crawford-Brown, D., Lin, J., Zhao, H., Hong, C., Boden, T. A., Feng, K., Peters, G. P., Xi, F., Liu, J., Li, Y., Zhao, Y., Zeng, N., and He, K.: Reduced carbon emission estimates from fossil fuel combustion and cement production in China, Nature, 524, 335–338, https://doi.org/10.1038/nature14677, 2015.
Liu, Z., Ciais, P., Deng, Z., Davis, S. J., Zheng, B., Wang, Y., Cui, D., Zhu, B., Dou, X., Ke, P., Sun, T., Guo, R., Zhong, H., Boucher, O., Bréon, F.-M., Lu, C., Guo, R., Xue, J., Boucher, E., Tanaka, K., and Chevallier, F.: Carbon Monitor, a near-real-time daily dataset of global CO2 emission from fossil fuel and cement production, Sci. Data, 7, 392, https://doi.org/10.1038/s41597-020-00708-7, 2020a.
Liu, Z., Ciais, P., Deng, Z., Lei, R., Davis, S. J., Feng, S., Zheng, B., Cui, D., Dou, X., Zhu, B., Guo, R., Ke, P., Sun, T., Lu, C., He, P., Wang, Y., Yue, X., Wang, Y., Lei, Y., Zhou, H., Cai, Z., Wu, Y., Guo, R., Han, T., Xue, J., Boucher, O., Boucher, E., Chevallier, F., Tanaka, K., Wei, Y., Zhong, H., Kang, C., Zhang, N., Chen, B., Xi, F., Liu, M., Bréon, F.-M., Lu, Y., Zhang, Q., Guan, D., Gong, P., Kammen, D. M., He, K., and Schellnhuber, H. J.: Near-real-time monitoring of global CO2 emissions reveals the effects of the COVID-19 pandemic, Nat. Commun., 11, 5172, https://doi.org/10.1038/s41467-020-18922-7, 2020b.
Ma, L., Hurtt, G. C., Chini, L. P., Sahajpal, R., Pongratz, J., Frolking, S., Stehfest, E., Klein Goldewijk, K., O'Leary, D., and Doelman, J. C.: Global rules for translating land-use change (LUH2) to land-cover change for CMIP6 using GLM2, Geosci. Model Dev., 13, 3203–3220, https://doi.org/10.5194/gmd-13-3203-2020, 2020.
Manning, A. and Keeling, R. F.: Global oceanic and land biotic carbon sinks from the https://doi.org/10.1111/j.1600-0889.2006.00175.x, 2006.
Maki, T., Ikegami, M., Fujita, T., Hirahara, T., Yamada, K., Mori, K., Takeuchi, A., Tsutsumi, Y., Suda, K., and Conway, T. J.: New technique to analyse global distributions of CO2 concentrations and fluxes from non-processed observational data, Tellus B., 62, 797–809, https://doi.org/10.1111/j.1600-0889.2010.00488.x, 2010.
MapBiomas: MapBiomas Collection 5, https://plataforma.brasil.mapbiomas.org/ (last access: 11 March 2022), 2021.
Marland, G.: Uncertainties in Accounting for CO2 From Fossil Fuels, 12, 136–139, https://doi.org/10.1111/j.1530-9290.2008.00014.x, 2008.
Marland, G., Hamal, K., and Jonas, M.: How Uncertain Are Estimates of CO2 Emissions?, J. Indust. Ecol., 13, 4–7, https://doi.org/10.1111/j.1530-9290.2009.00108.x, 2009.
Masarie, K. A. and Tans, P. P.: Extension and integration of atmospheric carbon dioxide data into a globally consistent measurement record, J. Geophys. Res., 100, 11593, https://doi.org/10.1029/95JD00859, 1995.
Mather, A. S.: The transition from deforestation to reforestation in Europe, in: Agricultural technologies and tropical deforestation, edited by: Angelsen, A. and Kaimowitz, D., CABI in association with centre for international Forestry Research, 35–52, 2001.
Matricardi, E. A. T., Skole, D. L., Costa, O. B., Pedlowski, M. A., Samek, J. H., and Miguel, E. P.: Long-term forest degradation surpasses deforestation in the Brazilian Amazon, Science, 369, 1378–1382, https://doi.org/10.1126/science.abb3021, 2020.
Mauritsen, T., Bader, J., Becker, T., Behrens, J., Bittner, M., Brokopf, R., Brovkin, V., Claussen, M., Crueger, T., Esch, M., Fast, I., Fiedler, S., Fläschner, D., Gayler, V., Giorgetta, M., Goll, D. S., Haak, H., Hagemann, S., Hedemann, C., Hohenegger, C., Ilyina, T., Jahns, T., Jimenéz-de-la-Cuesta, D., Jungclaus, J., Kleinen, T., Kloster, S., Kracher, D., Kinne, S., Kleberg, D., Lasslop, G., Kornblueh, L., Marotzke, J., Matei, D., Meraner, K., Mikolajewicz, U., Modali, K., Möbis, B., Müller, W. A., Nabel, J. E. M. S., Nam, C. C. W., Notz, D., Nyawira, S.-S., Paulsen, H., Peters, K., Pincus, R., Pohlmann, H., Pongratz, J., Popp, M., Raddatz, T. J., Rast, S., Redler, R., Reick, C. H., Rohrschneider, T., Schemann, V., Schmidt, H., Schnur, R., Schulzweida, U., Six, K. D., Stein, L., Stemmler, I., Stevens, B., von Storch, J.-S., Tian, F., Voigt, A., Vrese, P., Wieners, K.-H., Wilkenskjeld, S., Winkler, A., and Roeckner, E.: Developments in the MPI-M Earth System Model version 1.2 (MPI-ESM1.2) and Its Response to Increasing CO2, J. Adv. Model Earth Sy., 11, 998–1038, https://doi.org/10.1029/2018MS001400, 2019.
McGrath, M. J., Luyssaert, S., Meyfroidt, P., Kaplan, J. O., Bürgi, M., Chen, Y., Erb, K., Gimmi, U., McInerney, D., Naudts, K., Otto, J., Pasztor, F., Ryder, J., Schelhaas, M.-J., and Valade, A.: Reconstructing European forest management from 1600 to 2010, Biogeosciences, 12, 4291–4316, https://doi.org/10.5194/bg-12-4291-2015, 2015.
McKinley, G. A., Fay, A. R., Eddebbar, Y. A., Gloege, L., and Lovenduski, N. S.: External Forcing Explains Recent Decadal Variability of the Ocean Carbon Sink, AGU Adv., 1, e2019AV000149, https://doi.org/10.1029/2019AV000149, 2020.
McNeil, B. I.: Anthropogenic CO2 Uptake by the Ocean Based on the Global Chlorofluorocarbon Data Set, Science, 299, 235–239, https://doi.org/10.1126/science.1077429, 2003.
Meiyappan, P., Jain, A. K., and House, J. I.: Increased influence of nitrogen limitation on CO2 emissions from future land use and land use change, Global Biogeochem. Cy., 29, 1524–1548, https://doi.org/10.1002/2015GB005086, 2015.
Melton, J. R., Arora, V. K., Wisernig-Cojoc, E., Seiler, C., Fortier, M., Chan, E., and Teckentrup, L.: CLASSIC v1.0: the open-source community successor to the Canadian Land Surface Scheme (CLASS) and the Canadian Terrestrial Ecosystem Model (CTEM) – Part 1: Model framework and site-level performance, Geosci. Model Dev., 13, 2825–2850, https://doi.org/10.5194/gmd-13-2825-2020, 2020.
Mercado, L. M., Bellouin, N., Sitch, S., Boucher, O., Huntingford, C., Wild, M., and Cox, P. M.: Impact of changes in diffuse radiation on the global land carbon sink, Nature, 458, 1014–1017, https://doi.org/10.1038/nature07949, 2009.
Mikaloff Fletcher, S. E., Gruber, N., Jacobson, A. R., Doney, S. C., Dutkiewicz, S., Gerber, M., Follows, M., Joos, F., Lindsay, K., Menemenlis, D., Mouchet, A., Müller, S. A., and Sarmiento, J. L.: Inverse estimates of anthropogenic CO2 uptake, transport, and storage by the ocean: air-sea exchange of anthropogenic carbon, Global Biogeochem. Cy., 20, https://doi.org/10.1029/2005GB002530, 2006.
Myneni, R. B., Ramakrishna, R., Nemani, R., and Running, S. W.: Estimation of global leaf area index and absorbed par using radiative transfer models, IEEE T. Geosci. Remote, 35, 1380–1393, https://doi.org/10.1109/36.649788, 1997.
Naegler, T.: Reconciliation of excess 14C-constrained global CO2 piston velocity estimates, Tellus B., 61, 372–384, https://doi.org/10.1111/j.1600-0889.2008.00408.x, 2009.
Nakamura, T., Yamazaki, K., Iwamoto, K., Honda, M., Miyoshi, Y., Ogawa, Y., and Ukita, J.: A negative phase shift of the winter AO/NAO due to the recent Arctic sea-ice reduction in late autumn, J. Geophys. Res.-Atmos., 120, 3209–3227, https://doi.org/10.1002/2014JD022848, 2015.
Narayanan, B., Aguiar, A., and McDougall, R.: Global Trade, Assistance, and Production: The GTAP 9 Data Base, Cent. Glob. Trade Anal. Purdue Univ., https:// www.gtap.agecon.purdue.edu/databases/v9/default.asp (last access: 11 March 2022), 2015.
NBS: National Bureau of Statistics of China: Statistical Communiqué of the People's Republic of China on the 2021 National Economic and Social Development, http://www.stats.gov.cn/english/PressRelease/202202/t20220227_1827963.html, last access: 11 March 2022.
NCEP: National Centers for Environmental Prediction, ONI Index, Cold & Warm Episodes by Season, https://origin.cpc.ncep.noaa.gov/products/analysis_monitoring/ensostuff/ONI_v5.php (last access: 11 March 2022), 2021.
Nightingale, P. D., Liss, P. S., and Schlosser, P.: Measurements of air-sea gas transfer during an open ocean algal bloom, Geophys. Res. Lett., 27, 2117–2120, https://doi.org/10.1029/2000GL011541, 2000.
Niwa, Y., Fujii, Y., Sawa, Y., Iida, Y., Ito, A., Satoh, M., Imasu, R., Tsuboi, K., Matsueda, H., and Saigusa, N.: A 4D-Var inversion system based on the icosahedral grid model (NICAM-TM 4D-Var v1.0) – Part 2: Optimization scheme and identical twin experiment of atmospheric CO2 inversion, Geosci. Model Dev., 10, 2201–2219, https://doi.org/10.5194/gmd-10-2201-2017, 2017.
Niwa, Y., Langenfelds, R., Krummel, P., Loh, Z., Worthy, D., Hatakka, J., Aalto, T., Ramonet, M., Delmotte, M., Schmidt, M., Gheusi, F., Mihalopoulos, N., Morgui, J. A., Andrews, A., Dlugokencky, E., Lee, J., Sweeney, C., Thoning, K., Tans, P., De Wekker, S., Fischer, M. L., Jaffe, D., McKain, K., Viner, B., Miller, J. B., Karion, A., Miller, C., Sloop, C. D., Saito, K., Aoki, S., Morimoto, S., Goto, D., Steinbacher, M., Myhre, C., Catherine Lund, Hermanssen, O., Stephens, B., Keeling, R., Afshar, S., Paplawsky, B., Cox, A., Walker, S., Schuldt, K., Mukai, H., Machida, T., Sasakawa, M., Nomura, S., Ito, A., Iida, Y., and Jones, M. W.: Long-term global CO2 fluxes estimated by NICAM-based Inverse Simulation for Monitoring CO2 (NISMON-CO2) (ver.2020.1), National Institute for Environmental Studies, Japan [data set], https://doi.org/10.17595/20201127.001, 2020.
NOAA/ESRL: NOAA Greenhouse Gas Marine Boundary Layer Reference, https://www.esrl.noaa.gov/gmd/ccgg/mbl/mbl.html (last access: 11 March 2022), 2019.
Obermeier, W. A., Nabel, J. E. M. S., Loughran, T., Hartung, K., Bastos, A., Havermann, F., Anthoni, P., Arneth, A., Goll, D. S., Lienert, S., Lombardozzi, D., Luyssaert, S., McGuire, P. C., Melton, J. R., Poulter, B., Sitch, S., Sullivan, M. O., Tian, H., Walker, A. P., Wiltshire, A. J., Zaehle, S., and Pongratz, J.: Modelled land use and land cover change emissions – a spatio-temporal comparison of different approaches, Earth Syst. Dynam., 12, 635–670, https://doi.org/10.5194/esd-12-635-2021, 2021.
O'Rourke, P. R., Smith, S. J., Mott, A., Ahsan, H., McDuffie, E. E., Crippa, M., Klimont, Z., McDonald, B., Wang, S., Nicholson, M. B., Feng, L., and Hoesly, R. M.: CEDS v_2021_04_21 Release Emission Data, Zenodo [data set], https://doi.org/10.5281/zenodo.4741285, 2021.
Orr, J. C., Najjar, R. G., Aumont, O., Bopp, L., Bullister, J. L., Danabasoglu, G., Doney, S. C., Dunne, J. P., Dutay, J.-C., Graven, H., Griffies, S. M., John, J. G., Joos, F., Levin, I., Lindsay, K., Matear, R. J., McKinley, G. A., Mouchet, A., Oschlies, A., Romanou, A., Schlitzer, R., Tagliabue, A., Tanhua, T., and Yool, A.: Biogeochemical protocols and diagnostics for the CMIP6 Ocean Model Intercomparison Project (OMIP), Geosci. Model Dev., 10, 2169–2199, https://doi.org/10.5194/gmd-10-2169-2017, 2017.
O'Sullivan, M., Zhang, Y., Bellouin, N., Harris, I., Mercado, L. M., Sitch, S., Ciais, P., and Friedlingstein, P.: Aerosol–light interactions reduce the carbon budget imbalance, Environ. Res. Lett., 16, 124072, https://doi.org/10.1088/1748-9326/ac3b77, 2021.
Palmer, P. I., Feng, L., Baker, D., Chevallier, F., Bösch, H., and Somkuti, P.: Net carbon emissions from African biosphere dominate pan-tropical atmospheric CO2 signal, Nat. Commun., 10, 3344, https://doi.org/10.1038/s41467-019-11097-w, 2019.
Pan, Y., Birdsey, R. A., Fang, J., Houghton, R., Kauppi, P. E., Kurz, W. A., Phillips, O. L., Shvidenko, A., Lewis, S. L., Canadell, J. G., Ciais, P., Jackson, R. B., Pacala, S. W., McGuire, A. D., Piao, S., Rautiainen, A., Sitch, S., and Hayes, D.: A Large and Persistent Carbon Sink in the World's Forests, Science, 333, 988–993, https://doi.org/10.1126/science.1201609, 2011.
Pendrill, F., Persson, U. M., Godar, J., Kastner, T., Moran, D., Schmidt, S., and Wood, R.: Agricultural and forestry trade drives large share of tropical deforestation emissions, Global Environ. Chang., 56, 1–10, https://doi.org/10.1016/j.gloenvcha.2019.03.002, 2019.
Peters, G. P., Andrew, R., and Lennox, J.: Constructing an environmentally-extended multi-regional input–output table using the GTAP database, Economic Syst. Res., 23, 131–152, https://doi.org/10.1080/09535314.2011.563234, 2011a.
Peters, G. P., Minx, J. C., Weber, C. L., and Edenhofer, O.: Growth in emission transfers via international trade from 1990 to 2008, P. Natl. Acad. Sci. USA, 108, 8903–8908, https://doi.org/10.1073/pnas.1006388108, 2011b.
Peters, G. P., Davis, S. J., and Andrew, R.: A synthesis of carbon in international trade, Biogeosciences, 9, 3247–3276, https://doi.org/10.5194/bg-9-3247-2012, 2012a.
Peters, G. P., Marland, G., Le Quéré, C., Boden, T., Canadell, J. G., and Raupach, M. R.: Rapid growth in CO2 emissions after the 2008–2009 global financial crisis, Nat. Clim. Change, 2, 2–4, https://doi.org/10.1038/nclimate1332, 2012b.
Peters, G. P., Andrew, R. M., Boden, T., Canadell, J. G., Ciais, P., Le Quéré, C., Marland, G., Raupach, M. R., and Wilson, C.: The challenge to keep global warming below 2 ∘C, Nat. Clim Change, 3, 4–6, https://doi.org/10.1038/nclimate1783, 2013.
Peters, G. P., Le Quéré, C., Andrew, R. M., Canadell, J. G., Friedlingstein, P., Ilyina, T., Jackson, R. B., Joos, F., Korsbakken, J. I., McKinley, G. A., Sitch, S., and Tans, P.: Towards real-time verification of CO2 emissions, Nat. Clim. Change, 7, 848–850, https://doi.org/10.1038/s41558-017-0013-9, 2017.
Peters, G. P., Andrew, R. M., Canadell, J. G., Friedlingstein, P., Jackson, R. B., Korsbakken, J. I., Le Quéré, C., and Peregon, A.: Carbon dioxide emissions continue to grow amidst slowly emerging climate policies, Nat. Clim. Change, 10, 3–6, https://doi.org/10.1038/s41558-019-0659-6, 2020.
Petrescu, A. M. R., Peters, G. P., Janssens-Maenhout, G., Ciais, P., Tubiello, F. N., Grassi, G., Nabuurs, G.-J., Leip, A., Carmona-Garcia, G., Winiwarter, W., Höglund-Isaksson, L., Günther, D., Solazzo, E., Kiesow, A., Bastos, A., Pongratz, J., Nabel, J. E. M. S., Conchedda, G., Pilli, R., Andrew, R. M., Schelhaas, M.-J., and Dolman, A. J.: European anthropogenic AFOLU greenhouse gas emissions: a review and benchmark data, Earth Syst. Sci. Data, 12, 961–1001, https://doi.org/10.5194/essd-12-961-2020, 2020.
Pfeil, B., Olsen, A., Bakker, D. C. E., Hankin, S., Koyuk, H., Kozyr, A., Malczyk, J., Manke, A., Metzl, N., Sabine, C. L., Akl, J., Alin, S. R., Bates, N., Bellerby, R. G. J., Borges, A., Boutin, J., Brown, P. J., Cai, W.-J., Chavez, F. P., Chen, A., Cosca, C., Fassbender, A. J., Feely, R. A., González-Dávila, M., Goyet, C., Hales, B., Hardman-Mountford, N., Heinze, C., Hood, M., Hoppema, M., Hunt, C. W., Hydes, D., Ishii, M., Johannessen, T., Jones, S. D., Key, R. M., Körtzinger, A., Landschützer, P., Lauvset, S. K., Lefèvre, N., Lenton, A., Lourantou, A., Merlivat, L., Midorikawa, T., Mintrop, L., Miyazaki, C., Murata, A., Nakadate, A., Nakano, Y., Nakaoka, S., Nojiri, Y., Omar, A. M., Padin, X. A., Park, G.-H., Paterson, K., Perez, F. F., Pierrot, D., Poisson, A., Ríos, A. F., Santana-Casiano, J. M., Salisbury, J., Sarma, V. V. S. S., Schlitzer, R., Schneider, B., Schuster, U., Sieger, R., Skjelvan, I., Steinhoff, T., Suzuki, T., Takahashi, T., Tedesco, K., Telszewski, M., Thomas, H., Tilbrook, B., Tjiputra, J., Vandemark, D., Veness, T., Wanninkhof, R., Watson, A. J., Weiss, R., Wong, C. S., and Yoshikawa-Inoue, H.: A uniform, quality controlled Surface Ocean CO2 Atlas (SOCAT), Earth Syst. Sci. Data, 5, 125–143, https://doi.org/10.5194/essd-5-125-2013, 2013.
Piao, S., Ciais, P., Friedlingstein, P., de Noblet-Ducoudré, N., Cadule, P., Viovy, N., and Wang, T.: Spatiotemporal patterns of terrestrial carbon cycle during the 20th century, Global Biogeochem. Cy., 23, GB4026, https://doi.org/10.1029/2008GB003339, 2009.
Piao, S., Huang, M., Liu, Z., Wang, X., Ciais, P., Canadell, J. G., Wang, K., Bastos, A., Friedlingstein, P., Houghton, R. A., Le Quéré, C., Liu, Y., Myneni, R. B., Peng, S., Pongratz, J., Sitch, S., Yan, T., Wang, Y., Zhu, Z., Wu, D., and Wang, T.: Lower land-use emissions responsible for increased net land carbon sink during the slow warming period, Nat. Geosci., 11, 739–743, https://doi.org/10.1038/s41561-018-0204-7, 2018.
Pongratz, J., Reick, C. H., Houghton, R. A., and House, J. I.: Terminology as a key uncertainty in net land use and land cover change carbon flux estimates, Earth Syst. Dynam., 5, 177–195, https://doi.org/10.5194/esd-5-177-2014, 2014.
Pongratz, J., Schwingshackl, C., Bultan, S., Obermeier, W., Havermann, F., and Guo, S.: Land Use Effects on Climate: Current State, Recent Progress, and Emerging Topics, Curr. Clim. Change Rep., 7, 99–120, https://doi.org/10.1007/s40641-021-00178-y, 2021.
Potapov, P., Hansen, M. C., Laestadius, L., Turubanova, S., Yaroshenko, A., Thies, C., Smith, W., Zhuravleva, I., Komarova, A., Minnemeyer, S., and Esipova, E.: The last frontiers of wilderness: Tracking loss of intact forest landscapes from 2000 to 2013, Sci. Adv., 3, e1600821, https://doi.org/10.1126/sciadv.1600821, 2017.
Poulter, B., Frank, D. C., Hodson, E. L., and Zimmermann, N. E.: Impacts of land cover and climate data selection on understanding terrestrial carbon dynamics and the CO2 airborne fraction, Biogeosciences, 8, 2027–2036, https://doi.org/10.5194/bg-8-2027-2011, 2011.
Poulter, B., Freeborn, P. H., Jolly, W. M., and Varner, J. M.: COVID-19 lockdowns drive decline in active fires in southeastern United States, P. Natl. Acad. Sci. USA, 118, e2105666118, https://doi.org/10.1073/pnas.2105666118, 2021.
Prather, M.: Interactive comment on “Carbon dioxide and climate impulse response functions for the computation of greenhouse gas metrics: a multi-model analysis” by F. Joos et al.,Atmos. Chem. Phys. Discuss., 12, C11515–C11515, 2012.
Prentice, I. C., Farquhar, G. D., Fasham, M. J. R., Goulden, M. L., Heimann, M., Jaramillo, V. J., Kheshgi, H. S., Le Quéré, C., Scholes, R. J., and Wallace, D. W. R.: The Carbon Cycle and Atmospheric Carbon Dioxide, in: Climate Change 2001: The Scientific Basis. Contribution of Working Group I to the Third Assessment Report of the Intergovernmental Panel on Climate Change, edited by: Houghton, J. T., Ding, Y., Griggs, D. J., Noguer, M., van der Linden, P. J., Dai, X., Maskell, K., and Johnson, C. A., Cambridge University Press, Cambridge, United Kingdom and New York, NY, USA, 183–237, 2001.
Price, J. T. and Warren, R.: Literature Review of the Potential of “Blue Carbon” Activities to Reduce Emissions, https://avoid-net-uk.cc.ic.ac.uk/wp-content/uploads/delightful-downloads/2016/03/Literature-review-of-the-potential-of-blue-carbon-activities-to-reduce-emissions-AVOID2-WPE2.pdf (last access: 11 March 2022), 2016.
Qin, Y., Xiao, X., Wigneron, J.-P., Ciais, P., Brandt, M., Fan, L., Li, X., Crowell, S., Wu, X., Doughty, R., Zhang, Y., Liu, F., Sitch, S., and Moore, B.: Carbon loss from forest degradation exceeds that from deforestation in the Brazilian Amazon, Nat. Clim. Change, 11, 442–448, https://doi.org/10.1038/s41558-021-01026-5, 2021.
Qiu, C., Ciais, P., Zhu, D., Guenet, B., Peng, S., Petrescu, A. M. R., Lauerwald, R., Makowski, D., Gallego-Sala, A. V., Charman, D. J., and Brewer, S. C.: Large historical carbon emissions from cultivated northern peatlands, Sci. Adv., 7, eabf1332, https://doi.org/10.1126/sciadv.abf1332, 2021.
Raupach, M. R., Marland, G., Ciais, P., Le Quere, C., Canadell, J. G., Klepper, G., and Field, C. B.: Global and regional drivers of accelerating CO2 emissions, P. Natl. Acad. Sci. USA, 104, 10288–10293, https://doi.org/10.1073/pnas.0700609104, 2007.
Regnier, P., Friedlingstein, P., Ciais, P., Mackenzie, F. T., Gruber, N., Janssens, I. A., Laruelle, G. G., Lauerwald, R., Luyssaert, S., Andersson, A. J., Arndt, S., Arnosti, C., Borges, A. V., Dale, A. W., Gallego-Sala, A., Goddéris, Y., Goossens, N., Hartmann, J., Heinze, C., Ilyina, T., Joos, F., LaRowe, D. E., Leifeld, J., Meysman, F. J. R., Munhoven, G., Raymond, P. A., Spahni, R., Suntharalingam, P., and Thullner, M.: Anthropogenic perturbation of the carbon fluxes from land to ocean, Nat. Geosci., 6, 597–607, https://doi.org/10.1038/ngeo1830, 2013.
Reick, C. H., Gayler, V., Goll, D., Hagemann, S., Heidkamp, M., Nabel, J. E. M. S., Raddatz, T., Roeckner, E., Schnur, R., and Wilkenskjeld, S.: JSBACH 3 – The land component of the MPI Earth System Model: documentation of version 3.2, https://doi.org/10.17617/2.3279802, 2021.
Remaud, M., Chevallier, F., Cozic, A., Lin, X., and Bousquet, P.: On the impact of recent developments of the LMDz atmospheric general circulation model on the simulation of CO2 transport, Geosci. Model Dev., 11, 4489–4513, https://doi.org/10.5194/gmd-11-4489-2018, 2018.
Resplandy, L., Keeling, R. F., Rödenbeck, C., Stephens, B. B., Khatiwala, S., Rodgers, K. B., Long, M. C., Bopp, L., and Tans, P. P.: Revision of global carbon fluxes based on a reassessment of oceanic and riverine carbon transport, Nat. Geosci., 11, 504–509, https://doi.org/10.1038/s41561-018-0151-3, 2018.
Rhein, M., Rintoul, S. R., Aoki, S., Campos, E., Chambers, D., Feely, R. A., Gulev, S., Johnson, G. C., Josey, S. A., Kostianoy, A., Mauritzen, C., Roemmich, D., and Talley, L. D.: Observations: Ocean, edited by: Stocker, T. F., Qin, D., Plattner, G.-K., Tignor, M., Allen, S. K., Boschung, J., Nauels, A., Xia, Y., Bex, V., and Midgley, P. M., Cambridge University Press, 255–316, 2013.
Rödenbeck, C., Houweling, S., Gloor, M., and Heimann, M.: CO2 flux history 1982–2001 inferred from atmospheric data using a global inversion of atmospheric transport, Atmos. Chem. Phys., 3, 1919–1964, https://doi.org/10.5194/acp-3-1919-2003, 2003.
Rödenbeck, C., Keeling, R. F., Bakker, D. C. E., Metzl, N., Olsen, A., Sabine, C., and Heimann, M.: Global surface-ocean pCO2 and sea–air CO2 flux variability from an observation-driven ocean mixed-layer scheme, Ocean Sci., 9, 193–216, https://doi.org/10.5194/os-9-193-2013, 2013.
Rödenbeck, C., Bakker, D. C. E., Metzl, N., Olsen, A., Sabine, C., Cassar, N., Reum, F., Keeling, R. F., and Heimann, M.: Interannual sea–air CO2 flux variability from an observation-driven ocean mixed-layer scheme, Biogeosciences, 11, 4599–4613, https://doi.org/10.5194/bg-11-4599-2014, 2014.
Rödenbeck, C., Bakker, D. C. E., Gruber, N., Iida, Y., Jacobson, A. R., Jones, S., Landschützer, P., Metzl, N., Nakaoka, S., Olsen, A., Park, G.-H., Peylin, P., Rodgers, K. B., Sasse, T. P., Schuster, U., Shutler, J. D., Valsala, V., Wanninkhof, R., and Zeng, J.: Data-based estimates of the ocean carbon sink variability – first results of the Surface Ocean pCO2 Mapping intercomparison (SOCOM), Biogeosciences, 12, 7251–7278, https://doi.org/10.5194/bg-12-7251-2015, 2015.
Rödenbeck, C., Zaehle, S., Keeling, R., and Heimann, M.: History of El Niño impacts on the global carbon cycle 1957–2017: a quantification from atmospheric CO2 data, Philos. T. R. Soc. B., 373, 20170303, https://doi.org/10.1098/rstb.2017.0303, 2018.
Roobaert, A., Laruelle, G. G., Landschützer, P., and Regnier, P.: Uncertainty in the global oceanic CO2 uptake induced by wind forcing: quantification and spatial analysis, Biogeosciences, 15, 1701–1720, https://doi.org/10.5194/bg-15-1701-2018, 2018.
Rosan, T. M., Klein Goldewijk, K., Ganzenmüller, R., O'Sullivan, M., Pongratz, J., Mercado, L. M., Aragao, L. E. O. C., Heinrich, V., Randow, C. V., Wiltshire, A., Tubiello, F. N., Bastos, A., Friedlingstein, P., and Sitch, S.: A multi-data assessment of land use and land cover emissions from Brazil during 2000–2019, Environ. Res. Lett., 16, 074004, https://doi.org/10.1088/1748-9326/ac08c3, 2021.
Rypdal, K., Paciornik, N., Eggleston, S., Goodwin, J., Irving, W., Penman, J., and Woodfield, M.: Volume 1: Introduction to the 2006 Guidelines in: 2006 IPCC guidelines for national greenhouse gas inventories, https://www.ipccnggip.iges.or.jp/public/2006gl/vol1.html (last access: 11 March 2022), 2006.
Saatchi, S. S., Harris, N. L., Brown, S., Lefsky, M., Mitchard, E. T. A., Salas, W., Zutta, B. R., Buermann, W., Lewis, S. L., Hagen, S., Petrova, S., White, L., Silman, M., and Morel, A.: Benchmark map of forest carbon stocks in tropical regions across three continents, P. Natl. Acad. Sci. USA, 108, 9899–9904, https://doi.org/10.1073/pnas.1019576108, 2011.
Sabine, C. L., Feely, R. A., Gruber, N., Key, R. M., Lee, K., Bullister, J. L., Wanninkhof, R., Wong, C. S., Wallace, D. W. R., Tilbrook, B., Millero, F. J., Peng, T.-H., Kozyr, A., Ono, T., and Rios, A. F.: The Oceanic Sink for Anthropogenic CO2, Science, 305, 367–371, https://doi.org/10.1126/science.1097403, 2004.
Sarmiento, J. L., Orr, J. C., and Siegenthaler, U.: A perturbation simulation of CO2 uptake in an ocean general circulation model, 97, 3621–3645, https://doi.org/10.1029/91JC02849, 1992.
Sato, M., Hansen, J. E., McCormick, M. P., and Pollack, J. B.: Stratospheric aerosol optical depths, 1850–1990, J. Geophys. Res.-Atmos., 98, 22987–22994, https://doi.org/10.1029/93JD02553, 1993.
Saunois, M., Stavert, A. R., Poulter, B., Bousquet, P., Canadell, J. G., Jackson, R. B., Raymond, P. A., Dlugokencky, E. J., Houweling, S., Patra, P. K., Ciais, P., Arora, V. K., Bastviken, D., Bergamaschi, P., Blake, D. R., Brailsford, G., Bruhwiler, L., Carlson, K. M., Carrol, M., Castaldi, S., Chandra, N., Crevoisier, C., Crill, P. M., Covey, K., Curry, C. L., Etiope, G., Frankenberg, C., Gedney, N., Hegglin, M. I., Höglund-Isaksson, L., Hugelius, G., Ishizawa, M., Ito, A., Janssens-Maenhout, G., Jensen, K. M., Joos, F., Kleinen, T., Krummel, P. B., Langenfelds, R. L., Laruelle, G. G., Liu, L., Machida, T., Maksyutov, S., McDonald, K. C., McNorton, J., Miller, P. A., Melton, J. R., Morino, I., Müller, J., Murguia-Flores, F., Naik, V., Niwa, Y., Noce, S., O'Doherty, S., Parker, R. J., Peng, C., Peng, S., Peters, G. P., Prigent, C., Prinn, R., Ramonet, M., Regnier, P., Riley, W. J., Rosentreter, J. A., Segers, A., Simpson, I. J., Shi, H., Smith, S. J., Steele, L. P., Thornton, B. F., Tian, H., Tohjima, Y., Tubiello, F. N., Tsuruta, A., Viovy, N., Voulgarakis, A., Weber, T. S., van Weele, M., van der Werf, G. R., Weiss, R. F., Worthy, D., Wunch, D., Yin, Y., Yoshida, Y., Zhang, W., Zhang, Z., Zhao, Y., Zheng, B., Zhu, Q., Zhu, Q., and Zhuang, Q.: The Global Methane Budget 2000–2017, Earth Syst. Sci. Data, 12, 1561–1623, https://doi.org/10.5194/essd-12-1561-2020, 2020.
Schimel, D., Alves, D., Enting, I. G., Heimann, M., Joos, F., Raynaud, D., Wigley, T., Prater, M., Derwent, R., Ehhalt, D., Fraser, P., Sanhueza, E., Zhou, X., Jonas, P., Charlson, R., Rodhe, H., Sadasivan, S., Shine, K. P., Fouquart, Y., Ramaswamy, V., Solomon, S., Srinivasan, J., Albritton, D., Derwent, R., Isaksen, I., Lal, M., and Wuebbles, D.: Radiative Forcing of Climate Change, in: Climate Change 1995 The Science of Climate Change, Contribution of Working Group I to the Second Assessment Report of the Intergovernmental Panel on Climate Change, edited by: Houghton, J. T., Meira Rilho, L. G., Callander, B. A., Harris, N., Kattenberg, A., and Maskell, K., Cambridge University Press, Cambridge, United Kingdom and New York, NY, USA, 1995.
Schimel, D., Stephens, B. B., and Fisher, J. B.: Effect of increasing CO2 on the terrestrial carbon cycle, P. Natl. Acad. Sci. USA, 112, 436–441, https://doi.org/10.1073/pnas.1407302112, 2015.
Schourup-Kristensen, V., Sidorenko, D., Wolf-Gladrow, D. A., and Völker, C.: A skill assessment of the biogeochemical model REcoM2 coupled to the Finite Element Sea Ice–Ocean Model (FESOM 1.3), Geosci. Model Dev., 7, 2769–2802, https://doi.org/10.5194/gmd-7-2769-2014, 2014.
Schuh, A. E., Jacobson, A. R., Basu, S., Weir, B., Baker, D., Bowman, K., Chevallier, F., Crowell, S., Davis, K. J., Deng, F., Denning, S., Feng, L., Jones, D., Liu, J., and Palmer, P. I.: Quantifying the Impact of Atmospheric Transport Uncertainty on CO2 Surface Flux Estimates, Global Biogeochem. Cy., 33, 484–500, https://doi.org/10.1029/2018GB006086, 2019.
Schwinger, J., Goris, N., Tjiputra, J. F., Kriest, I., Bentsen, M., Bethke, I., Ilicak, M., Assmann, K. M., and Heinze, C.: Evaluation of NorESM-OC (versions 1 and 1.2), the ocean carbon-cycle stand-alone configuration of the Norwegian Earth System Model (NorESM1), Geosci. Model Dev., 9, 2589–2622, https://doi.org/10.5194/gmd-9-2589-2016, 2016.
Séférian, R., Nabat, P., Michou, M., Saint-Martin, D., Voldoire, A., Colin, J., Decharme, B., Delire, C., Berthet, S., Chevallier, M., Sénési, S., Franchisteguy, L., Vial, J., Mallet, M., Joetzjer, E., Geoffroy, O., Guérémy, J.-F., Moine, M.-P., Msadek, R., Ribes, A., Rocher, M., Roehrig, R., Salas-y-Mélia, D., Sanchez, E., Terray, L., Valcke, S., Waldman, R., Aumont, O., Bopp, L., Deshayes, J., Éthé, C., and Madec, G.: Evaluation of CNRM Earth System Model, CNRM-ESM2-1: Role of Earth System Processes in Present-Day and Future Climate, J. Adv. Model. Earth Sy., 11, 4182–4227, https://doi.org/10.1029/2019MS001791, 2019.
Sellar, A. A., Jones, C. G., Mulcahy, J. P., Tang, Y., Yool, A., Wiltshire, A., O'Connor, F. M., Stringer, M., Hill, R., Palmieri, J., Woodward, S., Mora, L., Kuhlbrodt, T., Rumbold, S. T., Kelley, D. I., Ellis, R., Johnson, C. E., Walton, J., Abraham, N. L., Andrews, M. B., Andrews, T., Archibald, A. T., Berthou, S., Burke, E., Blockley, E., Carslaw, K., Dalvi, M., Edwards, J., Folberth, G. A., Gedney, N., Griffiths, P. T., Harper, A. B., Hendry, M. A., Hewitt, A. J., Johnson, B., Jones, A., Jones, C. D., Keeble, J., Liddicoat, S., Morgenstern, O., Parker, R. J., Predoi, V., Robertson, E., Siahaan, A., Smith, R. S., Swaminathan, R., Woodhouse, M. T., Zeng, G., and Zerroukat, M.: UKESM1: Description and Evaluation of the U.K. Earth System Model, J. Adv. Model. Earth Sy., 11, 4513–4558, https://doi.org/10.1029/2019MS001739, 2019.
Shu, S., Jain, A. K., Koven, C. D., and Mishra, U.: Estimation of Permafrost SOC Stock and Turnover Time Using a Land Surface Model with Vertical Heterogeneity of Permafrost Soils, Global Biogeochem. Cy., 34, e2020GB006585, https://doi.org/10.1029/2020GB006585, 2020.
Silva Junior, C. H. L., Pessôa, A. C. M., Carvalho, N. S., Reis, J. B. C., Anderson, L. O., and Aragão, L. E. O. C.: The Brazilian Amazon deforestation rate in 2020 is the greatest of the decade, Nat. Ecol. Evol., 5, 144–145, https://doi.org/10.1038/s41559-020-01368-x, 2021.
Sitch, S., Huntingford, C., Gedney, N., Levy, P. E., Lomas, M., Piao, S. L., Betts, R., Ciais, P., Cox, P., Friedlingstein, P., Jones, C. D., Prentice, I. C., and Woodward, F. I.: Evaluation of the terrestrial carbon cycle, future plant geography and climate-carbon cycle feedbacks using five Dynamic Global Vegetation Models (DGVMs): Uncertainty In Land Carbon Cycle Feedbacks, Glob. Change Biol., 14, 2015–2039, https://doi.org/10.1111/j.1365-2486.2008.01626.x, 2008.
Smith, B., Wårlind, D., Arneth, A., Hickler, T., Leadley, P., Siltberg, J., and Zaehle, S.: Implications of incorporating N cycling and N limitations on primary production in an individual-based dynamic vegetation model, Biogeosciences, 11, 2027–2054, https://doi.org/10.5194/bg-11-2027-2014, 2014.
Stephens, B. B., Gurney, K. R., Tans, P. P., Sweeney, C., Peters, W., Bruhwiler, L., Ciais, P., Ramonet, M., Bousquet, P., Nakazawa, T., Aoki, S., Machida, T., Inoue, G., Vinnichenko, N., Lloyd, J., Jordan, A., Heimann, M., Shibistova, O., Langenfelds, R. L., Steele, L. P., Francey, R. J., and Denning, A. S.: Weak Northern and Strong Tropical Land Carbon Uptake from Vertical Profiles of Atmospheric CO2, Science, 316, 1732–1735, https://doi.org/10.1126/science.1137004, 2007.
Stocker, T., Qin, D., and Platner, G.-K.: Climate Change 2013: The Physical Science Basis. Contribution of Working Group I to the Fifth Assessment Report of the Intergovernmental Panel on Climate Change, edited by: Intergovernmental Panel on Climate Change, Cambridge University Press, Cambridge, 2013.
Sweeney, C., Gloor, E., Jacobson, A. R., Key, R. M., McKinley, G., Sarmiento, J. L., and Wanninkhof, R.: Constraining global air-sea gas exchange for CO2 with recent bomb 14C measurements, Global Biogeochem. Cy., 21, GB2015, https://doi.org/10.1029/2006GB002784, 2007.
SX Coal: Monthly coal consumption estimates, http://www.sxcoal.com/ (last access: 11 March 2022), 2021.
Takahashi, T., Sutherland, S. C., Wanninkhof, R., Sweeney, C., Feely, R. A., Chipman, D. W., Hales, B., Friederich, G., Chavez, F., Sabine, C., Watson, A., Bakker, D. C. E., Schuster, U., Metzl, N., Yoshikawa-Inoue, H., Ishii, M., Midorikawa, T., Nojiri, Y., Körtzinger, A., Steinhoff, T., Hoppema, M., Olafsson, J., Arnarson, T. S., Tilbrook, B., Johannessen, T., Olsen, A., Bellerby, R., Wong, C. S., Delille, B., Bates, N. R., and de Baar, H. J. W.: Climatological mean and decadal change in surface ocean pCO2, and net sea–air CO2 flux over the global oceans, Deep-Sea Res. Pt II, 56, 554–577, https://doi.org/10.1016/j.dsr2.2008.12.009, 2009.
The Global Carbon Budget 2007 (GCP): http://www.globalcarbonproject.org/carbonbudget/archive.htm (last access: 8 October 2021), 2007.
Thomason, L. W., Ernest, N., Millán, L., Rieger, L., Bourassa, A., Vernier, J.-P., Manney, G., Luo, B., Arfeuille, F., and Peter, T.: A global space-based stratospheric aerosol climatology: 1979–2016, Earth Syst. Sci. Data, 10, 469–492, https://doi.org/10.5194/essd-10-469-2018, 2018.
Tian, H., Xu, X., Lu, C., Liu, M., Ren, W., Chen, G., Melillo, J., and Liu, J.: Net exchanges of CO2, CH4, and N2O between China's terrestrial ecosystems and the atmosphere and their contributions to global climate warming, J. Geophys. Res., 116, G02011, https://doi.org/10.1029/2010JG001393, 2011.
Tian, H., Chen, G., Lu, C., Xu, X., Hayes, D. J., Ren, W., Pan, S., Huntzinger, D. N., and Wofsy, S. C.: North American terrestrial CO2 uptake largely offset by CH4 and N2O emissions: toward a full accounting of the greenhouse gas budget, Climatic Change, 129, 413–426, https://doi.org/10.1007/s10584-014-1072-9, 2015.
Todd-Brown, K. E. O., Randerson, J. T., Post, W. M., Hoffman, F. M., Tarnocai, C., Schuur, E. A. G., and Allison, S. D.: Causes of variation in soil carbon simulations from CMIP5 Earth system models and comparison with observations, Biogeosciences, 10, 1717–1736, https://doi.org/10.5194/bg-10-1717-2013, 2013.
Tohjima, Y., Mukai, H., Machida, T., Hoshina, Y., and Nakaoka, S.-I.: Global carbon budgets estimated from atmospheric O2/N2 and CO2 observations in the western Pacific region over a 15-year period, Atmos. Chem. Phys., 19, 9269–9285, https://doi.org/10.5194/acp-19-9269-2019, 2019.
Tubiello, F. N., Conchedda, G., Wanner, N., Federici, S., Rossi, S., and Grassi, G.: Carbon emissions and removals from forests: new estimates, 1990–2020, Earth Syst. Sci. Data, 13, 1681–1691, https://doi.org/10.5194/essd-13-1681-2021, 2021.
UN: United Nations Statistics Division: National Accounts Main Aggregates Database, http://unstats.un.org/unsd/snaama/Introduction.asp (last access: 11 March 2022), 2021.
UNFCCC: National Inventory Submissions, https://unfccc.int/ghg-inventories-annex-i-parties/2021 (last access: 11 March 2022), 2021a.
UNFCCC: Nationally determined contributions under the Paris Agreement, Synthesis report by the secretariat, https://unfccc.int/documents/306848 (last access: 11 March 2022), 2021b.
Vale, M. M., Berenguer, E., Argollo de Menezes, M., Viveiros de Castro, E. B., Pugliese de Siqueira, L., and Portela, R. de C. Q.: The COVID-19 pandemic as an opportunity to weaken environmental protection in Brazil, Biol. Conserv., 255, 108994, https://doi.org/10.1016/j.biocon.2021.108994, 2021.
van der Laan-Luijkx, I. T., van der Velde, I. R., van der Veen, E., Tsuruta, A., Stanislawska, K., Babenhauserheide, A., Zhang, H. F., Liu, Y., He, W., Chen, H., Masarie, K. A., Krol, M. C., and Peters, W.: The CarbonTracker Data Assimilation Shell (CTDAS) v1.0: implementation and global carbon balance 2001–2015, Geosci. Model Dev., 10, 2785–2800, https://doi.org/10.5194/gmd-10-2785-2017, 2017.
van der Velde, I. R., Miller, J. B., Schaefer, K., van der Werf, G. R., Krol, M. C., and Peters, W.: Terrestrial cycling of 13CO2 by photosynthesis, respiration, and biomass burning in SiBCASA, Biogeosciences, 11, 6553–6571, https://doi.org/10.5194/bg-11-6553-2014, 2014.
van der Velde, I. R., van der Werf, G. R., Houweling, S., Maasakkers, J. D., Borsdorff, T., Landgraf, J., Tol, P., van Kempen, T. A., van Hees, R., Hoogeveen, R., Veefkind, J. P., and Aben, I.: Vast CO2 release from Australian fires in 2019–2020 constrained by satellite, Nature, 597, 366–369, https://doi.org/10.1038/s41586-021-03712-y, 2021.
van der Werf, G. R., Randerson, J. T., Giglio, L., Collatz, G. J., Mu, M., Kasibhatla, P. S., Morton, D. C., DeFries, R. S., Jin, Y., and van Leeuwen, T. T.: Global fire emissions and the contribution of deforestation, savanna, forest, agricultural, and peat fires (1997–2009), Atmos. Chem. Phys., 10, 11707–11735, https://doi.org/10.5194/acp-10-11707-2010, 2010.
van der Werf, G. R., Randerson, J. T., Giglio, L., van Leeuwen, T. T., Chen, Y., Rogers, B. M., Mu, M., van Marle, M. J. E., Morton, D. C., Collatz, G. J., Yokelson, R. J., and Kasibhatla, P. S.: Global fire emissions estimates during 1997–2016, Earth Syst. Sci. Data, 9, 697–720, https://doi.org/10.5194/essd-9-697-2017, 2017.
Viovy, N.: CRUNCEP data set, ftp://nacp.ornl.gov/synthesis/2009/frescati/temp/land_use_change/original/readme.htm (last access: 11 March 2022), 2016.
Vuichard, N., Messina, P., Luyssaert, S., Guenet, B., Zaehle, S., Ghattas, J., Bastrikov, V., and Peylin, P.: Accounting for carbon and nitrogen interactions in the global terrestrial ecosystem model ORCHIDEE (trunk version, rev 4999): multi-scale evaluation of gross primary production, Geosci. Model Dev., 12, 4751–4779, https://doi.org/10.5194/gmd-12-4751-2019, 2019.
Walker, A. P., Quaife, T., Bodegom, P. M., De Kauwe, M. G., Keenan, T. F., Joiner, J., Lomas, M. R., MacBean, N., Xu, C., Yang, X., and Woodward, F. I.: The impact of alternative trait-scaling hypotheses for the maximum photosynthetic carboxylation rate (Vcmax) on global gross primary production, New Phytol., 215, 1370–1386, https://doi.org/10.1111/nph.14623, 2017.
Walker, A. P., De Kauwe, M. G., Bastos, A., Belmecheri, S., Georgiou, K., Keeling, R. F., McMahon, S. M., Medlyn, B. E., Moore, D. J. P., Norby, R. J., Zaehle, S., Anderson-Teixeira, K. J., Battipaglia, G., Brienen, R. J. W., Cabugao, K. G., Cailleret, M., Campbell, E., Canadell, J. G., Ciais, P., Craig, M. E., Ellsworth, D. S., Farquhar, G. D., Fatichi, S., Fisher, J. B., Frank, D. C., Graven, H., Gu, L., Haverd, V., Heilman, K., Heimann, M., Hungate, B. A., Iversen, C. M., Joos, F., Jiang, M., Keenan, T. F., Knauer, J., Körner, C., Leshyk, V. O., Leuzinger, S., Liu, Y., MacBean, N., Malhi, Y., McVicar, T. R., Penuelas, J., Pongratz, J., Powell, A. S., Riutta, T., Sabot, M. E. B., Schleucher, J., Sitch, S., Smith, W. K., Sulman, B., Taylor, B., Terrer, C., Torn, M. S., Treseder, K. K., Trugman, A. T., Trumbore, S. E., van Mantgem, P. J., Voelker, S. L., Whelan, M. E., and Zuidema, P. A.: Integrating the evidence for a terrestrial carbon sink caused by increasing atmospheric CO2, New Phytol., 229, 2413–2445, https://doi.org/10.1111/nph.16866, 2021.
Wanninkhof, R.: Relationship between wind speed and gas exchange over the ocean, J. Geophys. Res.-Oceans, 97, 7373–7382, https://doi.org/10.1029/92JC00188, 1992.
Wanninkhof, R.: Relationship between wind speed and gas exchange over the ocean revisited, Limnol. Oceanograph. 12, 351–362, https://doi.org/10.4319/lom.2014.12.351, 2014.
Wanninkhof, R., Park, G.-H., Takahashi, T., Sweeney, C., Feely, R., Nojiri, Y., Gruber, N., Doney, S. C., McKinley, G. A., Lenton, A., Le Quéré, C., Heinze, C., Schwinger, J., Graven, H., and Khatiwala, S.: Global ocean carbon uptake: magnitude, variability and trends, Biogeosciences, 10, 1983–2000, https://doi.org/10.5194/bg-10-1983-2013, 2013.
Watson, A. J., Schuster, U., Shutler, J. D., Holding, T., Ashton, I. G. C., Landschützer, P., Woolf, D. K., and Goddijn-Murphy, L.: Revised estimates of ocean-atmosphere CO2 flux are consistent with ocean carbon inventory, Nat. Commun., 11, 4422, https://doi.org/10.1038/s41467-020-18203-3, 2020.
Watson, R. T., Rohde, H., Oeschger, H., and Siegenthaler, U.: Greenhouse Gases and Aerosols, in: Climate Change: The IPCC Scientific Assessment. Intergovernmental Panel on Climate Change (IPCC), edited by: Houghton, J. T., Jenkins, G. J., and Ephraums, J. J., Cambridge University Press, Cambridge, 140,https://archive.ipcc.ch/ipccreports/far/wg_I/ipcc_far_wg_I_chapter_01.pdf (last access: 11 March 2022), 1990.
Weiss, R. F. and Price, B. A.: Nitrous oxide solubility in water and seawater, Mar. Chem., 8, 347–359, https://doi.org/10.1016/0304-4203(80)90024-9, 1980.
Wenzel, S., Cox, P. M., Eyring, V., and Friedlingstein, P.: Projected land photosynthesis constrained by changes in the seasonal cycle of atmospheric CO2, Nature, 538, 499–501, https://doi.org/10.1038/nature19772, 2016.
Wilkenskjeld, S., Kloster, S., Pongratz, J., Raddatz, T., and Reick, C. H.: Comparing the influence of net and gross anthropogenic land-use and land-cover changes on the carbon cycle in the MPI-ESM, Biogeosciences, 11, 4817–4828, https://doi.org/10.5194/bg-11-4817-2014, 2014.
Wiltshire, A. J., Burke, E. J., Chadburn, S. E., Jones, C. D., Cox, P. M., Davies-Barnard, T., Friedlingstein, P., Harper, A. B., Liddicoat, S., Sitch, S., and Zaehle, S.: JULES-CN: a coupled terrestrial carbon–nitrogen scheme (JULES vn5.1), Geosci. Model Dev., 14, 2161–2186, https://doi.org/10.5194/gmd-14-2161-2021, 2021.
Woodward, F. I. and Lomas, M. R.: Vegetation dynamics – simulating responses to climatic change, Biol. Rev., 79, 643–670, https://doi.org/10.1017/S1464793103006419, 2004.
Wright, R. M., Le Quéré, C., Buitenhuis, E., Pitois, S., and Gibbons, M. J.: Role of jellyfish in the plankton ecosystem revealed using a global ocean biogeochemical model, Biogeosciences, 18, 1291–1320, https://doi.org/10.5194/bg-18-1291-2021, 2021..
Xi, F., Davis, S. J., Ciais, P., Crawford-Brown, D., Guan, D., Pade, C., Shi, T., Syddall, M., Lv, J., Ji, L., Bing, L., Wang, J., Wei, W., Yang, K.-H., Lagerblad, B., Galan, I., Andrade, C., Zhang, Y., and Liu, Z.: Substantial global carbon uptake by cement carbonation, Nat. Geosci., 9, 880–883, https://doi.org/10.1038/ngeo2840, 2016.
Xia, J., Chen, Y., Liang, S., Liu, D., and Yuan, W.: Global simulations of carbon allocation coefficients for deciduous vegetation types, Tellus B., 67, 28016, https://doi.org/10.3402/tellusb.v67.28016, 2015.
Xu, R., Li, Y., Teuling, A. J., Zhao, L., Spracklen, D. V., Garcia-Carreras, L., Meier, R., Chen, L., Zheng, Y., Lin, H., and Fu, B.: Contrasting impacts of forests on cloud cover based on satellite observations, Nat. Commun., 13, 670, https://doi.org/10.1038/s41467-022-28161-7, 2022.
Yuan, W., Liu, D., Dong, W., Liu, S., Zhou, G., Yu, G., Zhao, T., Feng, J., Ma, Z., Chen, J., Chen, Y., Chen, S., Han, S., Huang, J., Li, L., Liu, H., Liu, S., Ma, M., Wang, Y., Xia, J., Xu, W., Zhang, Q., Zhao, X., and Zhao, L.: Multiyear precipitation reduction strongly decreases carbon uptake over northern China, J. Geophys. Res.-Biogeo., 119, 881–896, https://doi.org/10.1002/2014JG002608, 2014.
Yue, C., Ciais, P., Luyssaert, S., Li, W., McGrath, M. J., Chang, J., and Peng, S.: Representing anthropogenic gross land use change, wood harvest, and forest age dynamics in a global vegetation model ORCHIDEE-MICT v8.4.2, Geosci. Model Dev., 11, 409–428, https://doi.org/10.5194/gmd-11-409-2018, 2018.
Yue, X. and Unger, N.: The Yale Interactive terrestrial Biosphere model version 1.0: description, evaluation and implementation into NASA GISS ModelE2, Geosci. Model Dev., 8, 2399–2417, https://doi.org/10.5194/gmd-8-2399-2015, 2015.
Zaehle, S. and Friend, A. D.: Carbon and nitrogen cycle dynamics in the O-CN land surface model: 1. Model description, site-scale evaluation, and sensitivity to parameter estimates: Site-scale evaluation of a C-N model, Global Biogeochem. Cy., 24, GB1005, https://doi.org/10.1029/2009GB003521, 2010.
Zaehle, S., Ciais, P., Friend, A. D., and Prieur, V.: Carbon benefits of anthropogenic reactive nitrogen offset by nitrous oxide emissions, Nat. Geosci., 4, 601–605, https://doi.org/10.1038/ngeo1207, 2011.
Zeng, J., Nojiri, Y., Landschützer, P., Telszewski, M., and Nakaoka, S.: A Global Surface Ocean fCO2 Climatology Based on a Feed-Forward Neural Network, J. Atmos. Ocean. Tech., 31, 1838–1849, https://doi.org/10.1175/JTECH-D-13-00137.1, 2014.
Zheng, B., Chevallier, F., Yin, Y., Ciais, P., Fortems-Cheiney, A., Deeter, M. N., Parker, R. J., Wang, Y., Worden, H. M., and Zhao, Y.: Global atmospheric carbon monoxide budget 2000–2017 inferred from multi-species atmospheric inversions, Earth Syst. Sci. Data, 11, 1411–1436, https://doi.org/10.5194/essd-11-1411-2019, 2019.
Zheng, B., Ciais, P., Chevallier, F., Chuvieco, E., Chen, Y., and Yang, H.: Increasing forest fire emissions despite the decline in global burned area, Sci. Adv., 7, eabh2646, https://doi.org/10.1126/sciadv.abh2646, 2021.
Zscheischler, J., Mahecha, M. D., Avitabile, V., Calle, L., Carvalhais, N., Ciais, P., Gans, F., Gruber, N., Hartmann, J., Herold, M., Ichii, K., Jung, M., Landschützer, P., Laruelle, G. G., Lauerwald, R., Papale, D., Peylin, P., Poulter, B., Ray, D., Regnier, P., Rödenbeck, C., Roman-Cuesta, R. M., Schwalm, C., Tramontana, G., Tyukavina, A., Valentini, R., van der Werf, G., West, T. O., Wolf, J. E., and Reichstein, M.: Reviews and syntheses: An empirical spatiotemporal description of the global surface–atmosphere carbon fluxes: opportunities and data limitations, Biogeosciences, 14, 3685–3703, https://doi.org/10.5194/bg-14-3685-2017, 2017.
- Abstract
- Executive summary
- Introduction
- Methods
- Results
- Tracking progress towards mitigation targets
- Discussion
- Conclusions
- Data availability
- Appendix A: Supplementary tables
- Appendix B: Supplementary figures
- Appendix C: Extended methodology
- Appendix D: Processes not included in the global carbon budget
- Author contributions
- Competing interests
- Disclaimer
- Acknowledgements
- Financial support
- Review statement
- References
- Article
(16098 KB) - Full-text XML
- Abstract
- Executive summary
- Introduction
- Methods
- Results
- Tracking progress towards mitigation targets
- Discussion
- Conclusions
- Data availability
- Appendix A: Supplementary tables
- Appendix B: Supplementary figures
- Appendix C: Extended methodology
- Appendix D: Processes not included in the global carbon budget
- Author contributions
- Competing interests
- Disclaimer
- Acknowledgements
- Financial support
- Review statement
- References