the Creative Commons Attribution 4.0 License.
the Creative Commons Attribution 4.0 License.
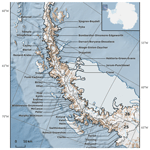
Calving front positions for 42 key glaciers of the Antarctic Peninsula Ice Sheet: a sub-seasonal record from 2013 to 2023 based on deep-learning application to Landsat multi-spectral imagery
Celia A. Baumhoer
Andreas Dietz
Mirko Scheinert
Martin Horwath
Calving front positions of marine-terminating glaciers are an essential parameter for understanding dynamic glacier changes and constraining ice modelling. In particular, for the Antarctic Peninsula, where the current ice mass loss is driven by dynamic glacier changes, accurate and comprehensive data products are of major importance. Current calving front data products are limited in coverage and temporal resolution because they rely on manual delineation, which is time-consuming and unfeasible for the increasing amount of satellite data. To simplify the mapping of calving fronts, we apply a deep-learning-based processing system designed to automatically delineate glacier fronts from multi-spectral Landsat imagery. The U-Net-based framework was initially trained on 869 Greenland glacier front positions. For this study, we extended the training data by 252 front positions of the Antarctic Peninsula. The data product presented here includes 4817 calving front locations of 42 key outlet glaciers from 2013 to 2023 and achieves a sub-seasonal temporal resolution. The mean difference between automated and manual extraction is estimated at 59.3±5.9 m. This dataset will help to better understand marine-terminating glacier dynamics on an intra-annual scale, study ice–ocean interactions in more detail and constrain glacier models. The data are publicly available at PANGAEA at https://doi.org/10.1594/PANGAEA.963725 (Loebel et al., 2024a).
- Article
(4812 KB) - Full-text XML
-
Supplement
(14200 KB) - BibTeX
- EndNote
From 1992 to 2020, the Antarctic Ice Sheet lost 2671±530 Gt of its ice, raising the global sea level by 7.4±1.5 mm (Otosaka et al., 2023). Mass loss is dominated by ice-dynamic processes, where a decrease in ice shelf thickness and extent reduces buttressing and thereby accelerates the ice flow discharge of grounded ice across the grounding line (Slater et al., 2020). At the Antarctic Peninsula (AP) in particular, increasing ice loss has been linked to ice shelf disintegration (Rott et al., 1996; Rignot et al., 2004; Rack and Rott, 2004; Cook and Vaughan, 2010). Thereby, atmospheric and oceanic influences cause ice shelf thinning and pre-condition collapse (Pritchard et al., 2012; Adusumilli et al., 2018). Although collapsing ice shelves do not directly contribute to sea level rise, they play an important role in stabilizing their tributary glaciers (Dupont and Alley, 2005). Once this support is lost, the dynamics of the tributary glaciers and their ice discharge increase, contributing directly to sea level rise (Rignot et al., 2004; Seehaus et al., 2018). This mechanism has been observed in a number of cases, most notably after the collapse of the Prince Gustav Ice Shelf (Glasser et al., 2011) and the Larsen-A and Larsen-B ice shelves (Hulbe et al., 2008; Rott et al., 2011, 2018). Beyond that, even marine-terminating glaciers that were not directly affected by ice shelf collapse, most of them flowing westward from the AP plateau, are experiencing changing dynamics as a result of a warming climate (Cook et al., 2016; Hogg et al., 2017; Wallis et al., 2023a; Davison et al., 2024). The negative ice mass change rates of the entire AP, Gt yr−1 between 1992 and 2020 and Gt yr−1 between 2017 and 2020, represent 14 % (between 1992 and 2020) and 18 % (between 2017 and 2020) of the mass loss rate of the Antarctic Ice Sheet (Otosaka et al., 2023). Monitoring of AP marine-terminating glaciers and ice shelves is of paramount importance for up-to-date diagnosis and reliable prediction of future changes.
One particularly important parameter of each glacier is the calving front position and its temporal variation. Firstly, calving front locations are the basis for mapping glacier area change. In this way, Cook et al. (2014) showed that the majority of the AP glaciers have decreased in area since the 1940s, with temporal trends indicating uniform retreat since the 1970s. Most significant area losses occurred in the north-eastern AP and are associated with ice shelf collapse. Area loss on the western coast shows a north–south gradient and has been linked to warming ocean water (Cook et al., 2016). Secondly, calving front locations are essential for studying and understanding ice–ocean interaction and the underlying processes. In this way, they help to understand the response of the AP to a warming climate. This applies to local studies of individual glaciers or glacier systems (Scambos et al., 2011; Seehaus et al., 2015, 2016) but also to regional studies (Friedl et al., 2018; Wallis et al., 2023a; Ochwat et al., 2024; Surawy-Stepney et al., 2024). Thirdly, calving front locations play an important role in constraining ice-dynamic models to improve simulations of future mass loss and sea level contributions (Alley et al., 2005; Barrand et al., 2013; Cornford et al., 2015). According to Pattyn and Morlighem (2020), calving is one of the key physical processes, where the lack of knowledge reduces the ability to accurately predict mass changes in the Antarctic Ice Sheet and define potential tipping points. Modelling studies for Jakobshavn Isbræ in Greenland identified calving as the dominant control on calving front migration, accounting for 90 % of the acceleration (Bondizo et al., 2017). Similar conclusions were also drawn by Vieli and Nick (2011), who emphasized the need for a robust representation of calving in ice sheet models.
Accurate glacier calving front data with both high temporal resolution and high spatial coverage are therefore critical. At present, however, these data products are not widely available for the AP. This is due to limitations of the manual and therefore time-consuming process of delineating these frontal positions from the increasing amount of satellite imagery available.
(Cook et al., 2021a)(GLIMS Consortium, 2005)Seehaus et al. (2015)Seehaus et al. (2016)(, )Gourmelon et al. (2022)Wallis et al. (2023b)Surawy-Stepney (2024)(Loebel et al., 2024a)Table 1Overview of publicly available glacier calving front datasets for the AP Ice Sheet. The number of fronts mapped by Cook et al. (2021a) is not documented. It is specified that more than 2000 aerial photographs and over 100 satellite images were used to compile the dataset. The datasets listed are those that include AP outlet glacier calving fronts. Data products focusing on ice shelves (e.g. Greene et al., 2022; Baumhoer et al., 2023; Andreasen et al., 2023) are not listed here.
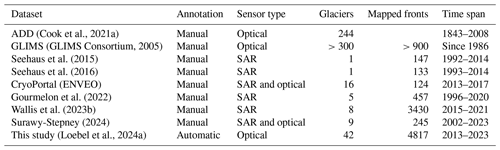
Table 1 gives an overview of the publicly available outlet glacier calving front datasets for the AP. The Antarctic Digital Database (ADD) (Cook et al., 2021b) and Global Land Ice Measurements from Space (GLIMS) (GLIMS Consortium, 2005; Raup et al., 2007) products have circum-Antarctic coverage but very limited temporal resolution. The calving front data of Seehaus et al. (2015), Seehaus et al. (2016), Wallis et al. (2023b) and Surawy-Stepney (2024) are by-products of glaciological studies. Calving fronts reported by Gourmelon et al. (2022) are part of a benchmark dataset developed to evaluate automated extraction from synthetic aperture radar (SAR) imagery. For the vast majority of the approximately 800 marine-terminating glaciers at the AP (Cook et al., 2014; Huber et al., 2017), current data products do not exploit the potential of available satellite observations. The availability of glacier calving front positions at the AP is limited, emphasizing the need for additional and more comprehensive data products. To generate these data products efficiently, we need to use automatic annotation methods.
In recent years, deep learning has emerged as the tool of choice to accomplish this task (Mohajerani et al., 2019; Baumhoer et al., 2019; Zhang et al., 2021; Heidler et al., 2021; Marochov et al., 2021; Periyasamy et al., 2022; Davari et al., 2022b, a; Heidler et al., 2022; Herrmann et al., 2023). This has already been demonstrated by Baumhoer et al. (2023), who applied neural networks to SAR imagery to generate a high-temporal-resolution dataset of Antarctic Ice Shelf frontal positions. In the AP, the IceLines dataset (Baumhoer et al., 2023) solely encompasses the Larsen Ice Shelf and excludes the outlet glaciers. Similar methods have been used to generate calving front data products for outlet glaciers in Greenland (Cheng et al., 2021; Zhang et al., 2023; Loebel et al., 2024d) and Svalbard (Li et al., 2024).
With this contribution, we provide a dense calving front data product for 42 key glaciers of the AP. We achieve this by applying a processing system initially developed for Greenland and incorporating new reference data. The locations of these glaciers are shown in Fig. 1. The period covered ranges from 2013 to 2023. The glaciers were chosen based on four criteria. We process all glaciers which (1) are part of the AP Ice Sheet, (2) are marine-terminating, (3) are listed in the Scientific Committee on Antarctic Research (SCAR) Composite Gazetteer of Antarctica (Cervellati et al., 2000) and (4) have a minimum calving front length of 5 km. The first three criteria define the scope of this dataset. Our product does not include glaciers on surrounding islands or ice shelve tributaries and the fourth criterion is related to processing limitations.
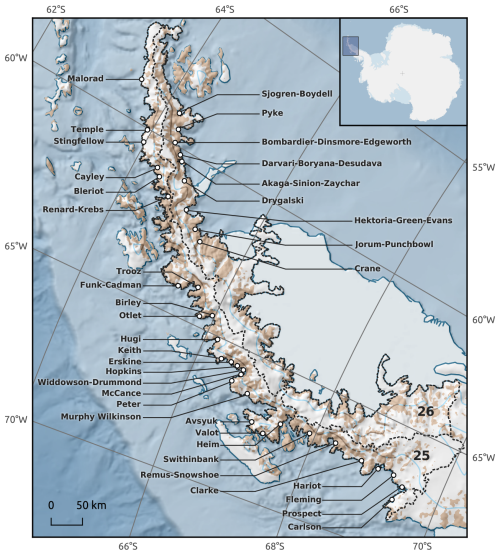
Figure 1Overview map of the Antarctic Peninsula and the 42 glaciers included in the presented data product. All the glaciers lie within drainage basins 25 and 26 mapped by Zwally et al. (2012).
The processing is based on the method previously described by Loebel et al. (2024d). Originally developed for marine-terminating outlet glaciers in Greenland, the method is built with a high degree of automatization. The main modification applied to the framework is the extension of the reference dataset to incorporate glaciers in the AP. Figure 2 gives a comprehensive overview of the processing system. The steps involved are described below, followed by an accuracy assessment of the results.
2.1 Calving front delineation using deep learning
Our processing is based on multi-spectral Landsat-8 and Landsat-9 Level-1 data. During pre-processing, nine available satellite bands, ranging from visible and infrared (VNIR) over short-wave infrared (SWIR) to thermal infrared (TIR), are cropped into 512 px × 512 px tiles with a unified ground sampling distance of 30 m centred at the corresponding calving front. With a width of about 15.3 km, these input tiles cover the calving fronts for most Greenland and AP outlet glaciers. To counteract image overexposure, we apply a cumulative count cut image enhancement, clipping the data between the 0.1st and 98th percentiles. Furthermore, all the bands are normalized between 0 and 1 by an 8-bit quantization. The ground truth reference was inferred by manual delineation for both training and testing our artificial neural network (ANN). To train the model, we apply 869 Greenland calving front positions and 252 calving fronts from 12 AP glaciers. Due to the similar morphologies of Greenland and AP outlet glaciers, these 869 Greenland calving front positions represent an ideal basis for a well-generalized ANN model. The 12 additional AP glaciers are Jorum, Punchbowl, Prospect, Hektoria–Green–Evans, Drygalski, Birley, Crane, Widdowson, Drummond, Fleming, Sjogren and Boydell. Expanding the training dataset is beneficial for accounting for the partly different glacier morphologies, such as the presence of free-floating glacier tongues. To avoid model overfitting, we make sure that the training data cover different calving and ice melange conditions as well as varying illumination and cloud conditions.
The applied ANN performs a land cover classification where an ocean class is semantically segmented from a glacier or land class. In particular, we use a modified U-Net (Ronneberger et al., 2015) with two additional contracting and expanding blocks. This modification results in a larger receptive field, which is helpful for calving front extraction (Heidler et al., 2021); 20 % of the input data are used for internal model validation and model selection. The training data are augmented eight times by rotation and mirroring. For model training, we used the Adam optimization algorithm (Kingma and Ba, 2014) on a binary cross-entropy loss function for 200 epochs and randomized batches of size 8. The model output is a floating point probability mask. Each image pixel is assigned a probability between 0 (water) and 1 (glacier and land). Since the terminus length of the Hektoria–Green–Evans glacier system exceeds the fixed window size, we infer five separate but partially overlapping predictions here. We then merge these five predictions by averaging the values where they overlap. During post-processing the prediction is vectorized using the Geospatial Data Abstraction Library (GDAL/OGR contributors, 2020) with a threshold of 0.5. The threshold of 0.5 is the boundary between the predicted water and glacier or land classes, i.e. the predicted coastline. The glacier front is then extracted by intersecting the predicted vectorized coastline with a static mask which is manually generated for each glacier. Masked predictions therefore only contain the calving front. This is important not only for producing a consistent data product but also for performing a correct accuracy assessment, as the land–ocean boundary is almost static, making it easier for the ANN to delineate.
For further analysis, the calving front location shape files are processed using the rectilinear box method (Moon and Joughin, 2008). We use this method not only to generate the time series of terminus area change but also to remove failed calving front extractions and separate outliers. In particular, calving fronts which do not split their corresponding glaciers box are discarded as failed extractions. In addition, we separate all entries that have an area difference of more than 20 % of the corresponding box width from the previous and following entries. Separated entries are checked manually and either (1) reinserted into the dataset if they were separated due to a true area change (e.g. due to calving of a large iceberg) or (2) discarded if the area change was due to a misclassification by the ANN.
For the generation of our data product, we downloaded 4991 Landsat-8 and Landsat-9 Level-1 scenes, all available data for the 42 glaciers until May 2023. These are then pre-processed into 30453 stacked (nine bands, 512 px × 512 px) raster subsets. Since consecutive Level-1 Landsat scenes of the same path overlap, we select a maximum of one entry per day by minimizing no-data pixels. The resulting 23 230 raster subsets are processed by the ANN. More than half of the extractions fail, mostly due to cloud cover, leaving 8688 calving fronts. After outlier separation and checking, our final data product contains 4817 calving front positions. The success rate, which we define here as the ratio of raster subsets going into ANN processing to final quality controlled data product entries, is 21 %. All the data product entries provided are full calving front extractions covering the entire calving front trajectory.
2.2 Accuracy assessment
The main accuracy assessment is done by comparing ANN-delineated calving front predictions to manual delineation for independent test imagery. The results of this comparison will validate our processing system and provide valuable metrics for comparing this method to existing studies. As an additional metric, we introduce the inter-model distance. Although the inter-model distance has limited reliability, it has the advantage that it can be determined for each ANN prediction without the need for manual delineation.
2.2.1 Comparison to manual delineation
The accuracy of the data product is estimated by comparing automated calving front extractions to manual delineations. Loebel et al. (2024d) already evaluated the processing system for accuracy and generalizability, with a particular emphasis on Greenland glaciers. Since we use additional training data for this analysis, we also apply a manually delineated test dataset, specifically for the AP. This test dataset contains 60 calving front locations over 20 glaciers. It includes eight glaciers which are not part of the training dataset. These eight test glaciers ensure the spatial transferability of our method. While the training data contain calving fronts from 2013 to 2021, the test dataset contains calving fronts for the separate period from 2022 to 2023. As ANN training is not deterministic, we train five separate models for our assessment. Our main error metric is the distance between the predicted delineation and the manual delineation. For this, we implement two different distance estimates. Firstly, we use an average minimum distance error, which we calculate by averaging the minimum distance every 30 m along the predicted front trajectory. This estimate is comparable to the ones used by Cheng et al. (2021), Loebel et al. (2022), Baumhoer et al. (2023) and Zhang et al. (2023). Secondly, we report the Hausdorff distance (Huttenlocher et al., 1993), which only considers the largest distance of all minimum distances along the two trajectories. The Hausdorff distance is therefore very sensitive to discrepancies, even along small sections of the glacier front between the ANN and the manually derived calving front.
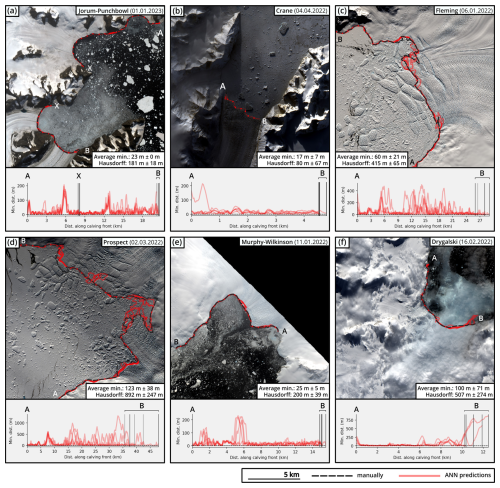
Figure 3Accuracy assessment results of six sample scenes from the test dataset. The dashed black lines show manually delineated calving fronts. The graphs at the bottom of each image show the minimum distance along the predicted trajectory (from A to B). The red lines show the five ANN predictions from five models. Overlap of the lines is indicated by the higher colour intensity. The average minimum distance and Hausdorff distances are given for each test image. Note that the endpoints B of the different models do not coincide due to the different lengths of the predicted fronts. For the locations of specific glaciers, see Fig. 1. Landsat imagery courtesy of the U.S. Geological Survey.
Figure 3 shows six test images for a diverse range of challenging conditions concerning ice melange, cloud cover, iceberg presence, low illumination and satellite scene borders. In addition, the minimum distance along the predicted trajectory (from A to B) is shown for each image. Our processing system reliably delineates calving fronts from a wide range of image conditions. These include a wide range of ocean, ice melange and illumination conditions as well as light cloud cover and images with calving fronts near the edge of a satellite scene. This is due to the large training dataset, which covers a wide variety of satellite images under these conditions. In addition, the integration of multi-spectral input data leads to more accurate predictions under these difficult conditions than using only single-band inputs (Loebel et al., 2022). Looking at the distance error along the predicted fronts, it is clear that the difference between manual delineation and ANNs is not uniform. This is also reflected in the Hausdorff and average minimum distance errors, which can vary greatly. Large minimum distance errors mostly occur due to inaccurate ANN predictions in difficult-to-delineate parts of the glacier front (e.g. at ∼11 km in Fig. 3f or at ∼15 km in Fig. 3c). However, it is important to note that, although we treat it as such here, our manual delineation is not a traditional ground truth, as it is also uncertain depending on the author and the satellite image. For difficult-to-delineate scenes (e.g. Prospect Glacier in Fig. 3d), it is not possible to attribute the error, as both the manual and ANN predictions are uncertain.
Table 2Results of the accuracy assessment presented as mean values with the corresponding standard deviations calculated over the five trained models. The average minimum distance and the Hausdorff distance estimates are provided as mean and median values over the test dataset. Also shown are the accuracy, precision, recall and F1 score. The binary classification metrics relate to the land or glacier class.

Table 2 gives an overview of the accuracy assessment over the entire test dataset. In addition to the average minimum distance and the Hausdorff distance estimates, we specify the binary classification metrics accuracy, precision, recall and F1 score. Whilst a high binary classification performance does not necessarily translate into an accurate prediction of the calving front trajectory, we report these values to facilitate the comparability of our results with other studies and datasets. Although completely different test datasets are involved, the 59.3±5.9 m mean average minimum distance calculated here aligns very well with the 61.2±7.5 m reported by Loebel et al. (2024d). When applied to the ESA-CCI (ENVEO, 2017) and CALFIN (Cheng et al., 2021) test datasets (as processed in Loebel et al., 2024d), which contain a further 100 and 110 additional test images from Greenland glaciers, we calculate mean average minimum distances of 79.1±5.3 and 78.7±3.8 m (see the extended accuracy assessment table in the Supplement). Furthermore, these results are in the broad range of other ANN-based calving front extraction methods using optical imagery, like Cheng et al. (2021) with 86.7±1.4 m and Zhang et al. (2023) with 79 m. The Hausdorff distance is commonly not reported in other automated glacier front delineation studies. However, Goliber et al. (2022) estimated the error in manual delineation by applying the median Hausdorff distance to duplicate delineations from different authors. Depending on the pairs of authors, the median errors range from 58.6 m to 7350 m with an overall median error of 107 m. This suggests that our method, which has a median Hausdorff error of 257±14.7 m, is in the range of possible manual delineation errors but has not yet reached human performance.
When assessing the accuracy only for the 23 test scenes of glaciers outside the training dataset, we calculate a mean delineation error of 51.9±6.7 m (median 37.3±5.3 m). Interestingly, the mean is lower and the median is higher than for an assessment over the 37 scenes from glaciers within the training dataset, where the mean (and median) delineation error is 65.3±7.7 m (median 33.8±1.5 m). This is likely because of training glaciers that have challenging-to-delineate calving conditions (like Prospect Glacier; see Fig. 3d). Figure 4 shows the distribution of the average minimum distance error accumulated over all the test scenes for the five trained models.
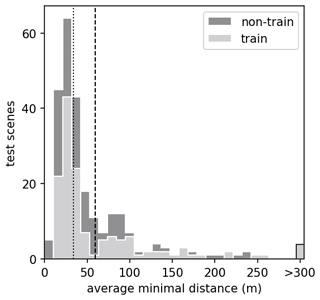
Figure 4Histogram of the average minimum distance between manual delineation and ANN prediction. The number of total test scenes corresponds to the test dataset multiplied by the five trained ANN models. The overall median is shown as a dotted line and the overall mean as a dashed line. The grey levels indicate whether the test scene is from a glacier that is part of the training dataset or not.
Based on these numbers, we confirm the high degree of ANN model generalization and hence the spatial transferability of our method.
2.2.2 Inter-model distance
As a secondary estimate of accuracy, we introduce the inter-model distance. We define the inter-model distance as the average minimum distance between two predicted calving front trajectories from two different ANN models that have the same architecture and use the same set of hyperparameters. Specifically for our case, we calculate a mean inter-model distance between the ANN model we use to generate our data product (randomly selected) and the four other trained models. The concept behind this metric is that the variability of different models predicting the same calving front is highly correlated with the accuracy of those predictions. The main motivation for introducing the mean inter-model distance is that it can be calculated for each calving front prediction without the need for manual delineation. Hence, the mean inter-model distance can be reported for each entry in our data product. However, limitations need to be considered when evaluating this metric. Although a low inter-model distance confirms that the ANN is confident in its prediction, this does not necessarily translate into an accurate calving front. Systematically incorrect predictions from all five ANN models (e.g. due to an iceberg being misclassified as a glacier) result in a low mean inter-model distance, when in fact the predicted front would be of low quality with a large distance to the manual delineation. That said, as incorrect predictions are mostly eliminated during quality control of the data product (see Sect. 2), the opposite is more likely to happen. Here, a high mean inter-model distance is calculated, although the predicted front is of good quality. This happens most often in satellite scenes with difficult image conditions where the ANN predictions have low confidence, causing some models to delineate the front correctly. An accurately delineated calving front prediction can therefore yield a high mean inter-model distance when at least one of the predictions of the other four models is of low quality. Overall, we believe that this mean inter-model distance is a useful estimate of accuracy, although it will likely overestimate the delineation error for some calving front predictions.
Figure 5 shows the distribution of the mean inter-model distance over all the calving front entries in our data product. Again, we distinguish whether the entry is from a glacier that is part of the training dataset or not, further validating the spatial transferability. Across our entire data product, we calculate a mean of 107.9 m and a median of 33.2 m. While the mean value is significantly higher than the mean average minimum distance of our test dataset, the median values are almost the same. It should also be noted that the mean inter-model distance varies considerably from glacier to glacier. Separate histograms for each of the 42 glaciers are included in the Supplement.
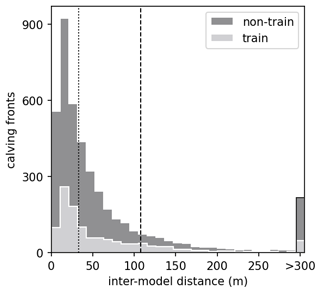
Figure 5Histogram of the mean inter-model distance over all calving front entries in our data product. The overall median is shown as a dotted line and the overall mean as a dashed line. The grey levels indicate whether the test scene is from a glacier that is part of the training dataset or not.
Table 3Temporal coverage of our ANN-generated time series. The numbers and the colour intensity indicate the number of processed calving front positions in the respective year.
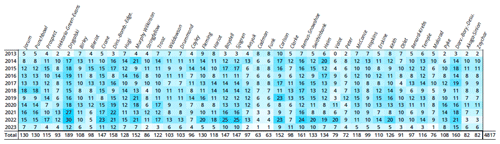
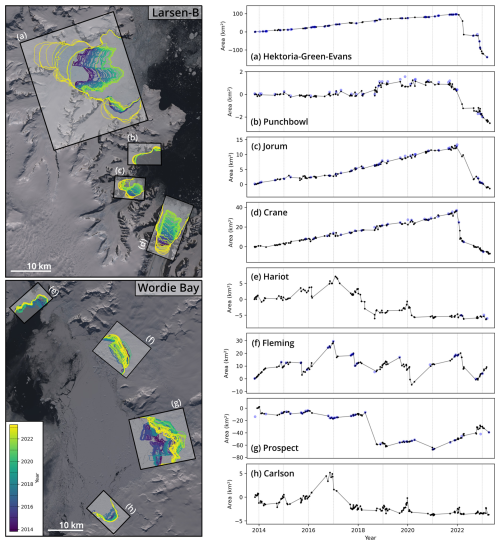
Figure 6Example time series of terminus area change generated by our processing system for (a–d) four AP glaciers in the Larsen-B embayment and (e–g) three glaciers at Wordie Bay. Colour-coded calving front locations are depicted on the maps on the left. The corresponding time series are shown on the right with the entries marked by black dots. The blue dots are additional validation marks that indicate the frontal positions of the manually delimited reference dataset. Landsat imagery courtesy of the U.S. Geological Survey.
The data product presented here has been created to provide glaciologists and glacier modellers with high-quality calving front positions of the AP Ice Sheet without the need for manual delineation. Figure 1 gives a spatial overview of the 42 processed glaciers. A tabular overview is given in Table 3. In total, the data record encompasses 4817 calving front positions over 42 marine-terminating glaciers. Since the data are derived from optical imagery, the time series have a 14-week gap during the polar night from May to mid-August. Outside the polar night, the dataset has one entry every 19.5 d on average. However, the sampling is irregular and primarily dependent on the satellite orbit and cloud cover. The time frame from 2013 to 2023 covers that of the IceLines dataset (Baumhoer et al., 2023), facilitating a combined analysis of circum-Antarctic calving front change.
Figure 6 gives eight example time series of the terminus area change within two regions of the AP. The terminus area change in glaciers in the Larsen-B embayment (Fig. 6a–d) is spatially correlated and shows a steady advance from 2013 until the end of 2021. At the beginning of 2022, our data show a simultaneous retreat of the four glaciers. Subsequently, the glacier tongues of the Hektoria–Green–Evans, Jorum and Crane glaciers collapsed. This simultaneous retreat is attributed to the disintegration of landfast sea ice inside the embayment in early 2022 and the resulting loss of buttressing (Ochwat et al., 2024). The glaciers in Wordie Bay (Fig. 6e–h) show more varied calving front dynamics. These range from stable calving front positions (Hariot Glacier and Carlson Glacier since mid-2020) over steady terminus advances superimposed by frequent calving events (Fleming Glacier) to large calving events (Prospect Glacier in 2018). The dynamic changes in this area are linked to the Wordie Ice Shelf and its disintegration between the 1960s and the late 1990s. This has led to increased ice flow and calving of the four main tributary glaciers, i.e. Hariot, Fleming, Carlson and Prospect (Friedl et al., 2018). Therefore, operational and temporally high-resolution monitoring of these glaciers is particularly important. An overview of the time series of all 42 glaciers of our data product is given in the Supplement.
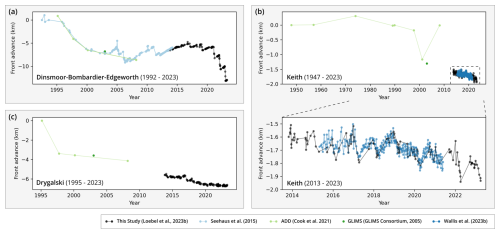
Figure 7Time series of glacier advance along the central flowline for (a) the Dinsmore–Bombardier–Edgeworth glacier system, (b) Keith Glacier and (c) Drygalski Glacier. The individual datasets are marked with different colours. Note that the axes have differing scales.
To put our data product into context with existing datasets, we compare the different time series. Figure 7 shows the time series of glacier advance for three examples. For each glacier, all available datasets were used (see Table 1). For the Dinsmoor–Bombardier–Edgeworth glacier system (Fig. 7a), there is generally very good agreement between the four datasets. Here, our data product provides a valuable continuation of the time series of Seehaus et al. (2015), which was delineated as part of a glaciological study (Seehaus et al., 2015) for this glacier. Similarly, for Keith Glacier (Fig. 7b), the dataset of Wallis et al. (2023a) has significant overlap with our time series. Although calving front change during this period is relatively small, the seasonal and sub-seasonal variations are captured by both datasets. Importantly, differences between these time series are in the range of both manual and ANN delineation accuracies. The time series of Drygalski Glacier (Fig. 7c) is representative of the majority of the glaciers in our data product. Here our time series is the first seasonal record, with the other available datasets being the GLIMS database (GLIMS Consortium, 2005) and the ADD (Cook et al., 2021b). These three examples emphasize that the quality of our automatically delineated calving fronts is comparable to that of existing manually extracted datasets. As a result, our data product is the first to combine seasonal temporal resolution with a large spatial coverage across the AP. Nevertheless, the importance of the GLIMS and ADD products must be emphasized, as these are still the only repositories that provide complete coverage and, for the ADD in particular, long-term observations going back to 1843.
The data product is stored in a georeferenced vector file format (GeoPackage and ESRI Shapefile), sorted by glacier and date within a file system structure. All the files are georeferenced using the Antarctic Polar Stereographic Projection (EPSG:3031). This allows for easy handling, e.g. by means of GIS software or geospatial data libraries. Calving front traces are stored separately for each glacier and each date as well as in a consolidated file. In addition to the full data product, annual entries are provided for each glacier. The annual entries also contain the full ANN coastline predictions, which are provided as both linestrings and polygonal masks. The attribute table of each file includes the glacier name, calving front date, type (glacier front or coastline), processing date, processing version, corresponding Landsat product identifier, mean inter-model distance and standard deviation of the inter-model distance. The file naming convention for each entry is [glacier name]_[YYYYMMDD]_[type].shp. An example entry would be prospect_20230408_glacier_front.shp.
The AP calving front location data record is publicly available from PANGAEA at https://doi.org/10.1594/PANGAEA.963725 (Loebel et al., 2024a). The calving front locations can be downloaded by clicking on the “View Dataset as HTML” button in the overview. All reference data applied in this study are publicly available. The Greenland reference data are available at https://doi.org/10.25532/OPARA-282 (Loebel et al., 2024e) and the AP reference data are available at https://doi.org/10.25532/OPARA-581 (Loebel et al., 2024c). We provide a containerized implementation (platform Docker) of the presented processing system. The software automatically extracts calving front positions from Landsat-8 or Landsat-9 Level-1 data archives for the glaciers used in this study or for user-defined coordinates. This enables analysis of glaciers that are outside our reference dataset or beyond the temporal framework of our study. The software is available at https://github.com/eloebel/glacier-front-extraction (last access: 24 July 2024) and https://doi.org/10.5281/zenodo.7755774 (Loebel, 2023a). Our implementation (software Python 3) of the rectilinear box method is available at https://github.com/eloebel/rectilinear-box-method (last access: 24 July 2024) and https://doi.org/10.5281/zenodo.7738605 (Loebel, 2023b). The processed time series of the terminus area change, provided in text file format, are available at https://doi.org/10.25532/OPARA-557 (Loebel et al., 2024b).
Accurate and temporally and spatially comprehensive calving front data are essential for understanding and modelling glacial evolution. This paper addresses this requirement and presents a new data record for glaciers at the AP. The data are generated by applying multi-spectral Landsat-8 and Landsat-9 imagery to a deep-learning-based processing system. We validated the processing system for accuracy, robustness and generalization capabilities using independent test data. The mean difference between automated and manual extraction is estimated at 59.3±5.9 m. The resulting data record contains 4817 calving front locations for 42 key outlet glaciers from 2013 to 2023. It achieves a sub-seasonal temporal resolution for all the processed glaciers, making it a valuable addition to the existing data records.
More broadly, this contribution shows that well-generalized ANN processing systems can be applied to various regions of interest, with only minor additions to reference data. With thousands of marine-terminating glaciers worldwide (RGI Consortium, 2017), this is particularly relevant for extracting calving fronts. In addition to applications for current satellite missions, there is also significant potential for improving historical data records by exploiting the vast amount of satellite imagery collected over the past decades. We expect that our presented data record will not only advance glaciological research for the AP but also contribute to future deep-learning-based calving front data products and data inter-comparison projects.
The supplement related to this article is available online at: https://doi.org/10.5194/essd-17-65-2025-supplement.
Contributions are according to CRediT. Conceptualization: EL, CAB, AD and MS. Data curation: EL. Formal analysis: EL. Funding acquisition: MH, MS and AD. Investigation: EL. Methodology: EL. Software: EL. Supervision: CAB, AD, MH and MS. Validation: EL. Visualization: EL. Writing (original draft): EL. Writing (review and editing): EL, CAB, MH and MS.
The contact author has declared that none of the authors has any competing interests.
Publisher's note: Copernicus Publications remains neutral with regard to jurisdictional claims made in the text, published maps, institutional affiliations, or any other geographical representation in this paper. While Copernicus Publications makes every effort to include appropriate place names, the final responsibility lies with the authors.
We thank the USGS for providing the Landsat-8 and Landsat-9 imagery. We are grateful to the TU Dresden Centre for Information Services and High-Performance Computing (ZIH) for providing their high-performance and storage infrastructure. We acknowledge the Norwegian Polar Institute's Quantarctica package.
This work was supported by the Helmholtz Association of German Research Centres as part of the Helmholtz Information and Data Science Incubator project “Artificial Intelligence for Cold Regions” (AI-CORE, grant no. ZT-I-0016) and by the German Federal Ministry of Education and Research (BMBF) project “Greenland Ice Sheet/Ocean Interaction” (GROCE2, grant no. 03F0778G).
This paper was edited by Charles Amory and reviewed by Benjamin Davison and two anonymous referees.
Adusumilli, S., Fricker, H. A., Siegfried, M. R., Padman, L., Paolo, F. S., and Ligtenberg, S. R.: Variable basal melt rates of Antarctic Peninsula ice shelves, 1994–2016, Geophys. Res. Lett., 45, 4086–4095, https://doi.org/10.1002/2017GL076652, 2018. a
Alley, R. B., Clark, P. U., Huybrechts, P., and Joughin, I.: Ice-sheet and sea-level changes, Science, 310, 456–460, https://doi.org/10.1126/science.1114613, 2005. a
Andreasen, J. R., Hogg, A. E., and Selley, H. L.: Change in Antarctic ice shelf area from 2009 to 2019, The Cryosphere, 17, 2059–2072, https://doi.org/10.5194/tc-17-2059-2023, 2023. a
Barrand, N. E., Hindmarsh, R. C., Arthern, R. J., Williams, C. R., Mouginot, J., Scheuchl, B., Rignot, E., Ligtenberg, S. R., Van Den Broeke, M. R., Edwards, T. L., Cook, A. J., and Simonsen, S. B.: Computing the volume response of the Antarctic Peninsula ice sheet to warming scenarios to 2200, J. Glaciol., 59, 397–409, https://doi.org/10.3189/2013JoG12J139, 2013. a
Baumhoer, C. A., Dietz, A. J., Kneisel, C., and Kuenzer, C.: Automated Extraction of Antarctic Glacier and Ice Shelf Fronts from Sentinel-1 Imagery Using Deep Learning, Remote Sens., 11, 2529, https://doi.org/10.3390/rs11212529, 2019. a
Baumhoer, C. A., Dietz, A. J., Heidler, K., and Kuenzer, C.: IceLines – A new data set of Antarctic ice shelf front positions, Sci. Data, 10, 138, https://doi.org/10.1038/s41597-023-02045-x, 2023. a, b, c, d, e
Bondizo, J. H., Morlighem, M., Seroussi, H., Kleiner, T., Rückamp, M., Mouginot, J., Moon, T., Larour, E. Y., and Humbert, A.: The mechanisms behind Jakobshavn Isbræ's acceleration and mass loss: A 3‐D thermomechanical model study, Geophys. Res. Lett., 44, 6252–6260, https://doi.org/10.1002/2017GL073309, 2017. a
Cervellati, R., Ramorino, C., Sievers, J., Thomson, J., and Clarke, D.: A composite gazetteer of Antarctica, Polar Record, 36, 278–285, https://doi.org/10.1017/S0032247400016739, 2000. a
Cheng, D., Hayes, W., Larour, E., Mohajerani, Y., Wood, M., Velicogna, I., and Rignot, E.: Calving Front Machine (CALFIN): glacial termini dataset and automated deep learning extraction method for Greenland, 1972–2019, The Cryosphere, 15, 1663–1675, https://doi.org/10.5194/tc-15-1663-2021, 2021. a, b, c, d
Cook, A., Vaughan, D., Luckman, A., and Murray, T.: A new Antarctic Peninsula glacier basin inventory and observed area changes since the 1940s, Antarct. Sci., 26, 614–624, https://doi.org/10.1017/S0954102014000200, 2014. a, b
Cook, A., Fox, A., and Thomson, J.: Coastal change data for the Antarctic Peninsula region, 1843 to 2008 (Version 1.0), NERC EDS UK Polar Data Centre [data set], https://doi.org/10.5285/07727663-9B94-4069-A486-67E4D82177D3, 2021a. a, b
Cook, A. J. and Vaughan, D. G.: Overview of areal changes of the ice shelves on the Antarctic Peninsula over the past 50 years, The Cryosphere, 4, 77–98, https://doi.org/10.5194/tc-4-77-2010, 2010. a
Cook, A. J., Holland, P., Meredith, M., Murray, T., Luckman, A., and Vaughan, D. G.: Ocean forcing of glacier retreat in the western Antarctic Peninsula, Science, 353, 283–286, https://doi.org/10.1126/science.aae0017, 2016. a, b
Cook, S. J., Christoffersen, P., Truffer, M., Chudley, T. R., and Abellán, A.: Calving of a Large Greenlandic Tidewater Glacier has Complex Links to Meltwater Plumes and Mélange, J. Geophys. Res.-Earth Surf., 126, e2020JF006051, https://doi.org/10.1029/2020JF006051, 2021b. a, b
Cornford, S. L., Martin, D. F., Payne, A. J., Ng, E. G., Le Brocq, A. M., Gladstone, R. M., Edwards, T. L., Shannon, S. R., Agosta, C., van den Broeke, M. R., Hellmer, H. H., Krinner, G., Ligtenberg, S. R. M., Timmermann, R., and Vaughan, D. G.: Century-scale simulations of the response of the West Antarctic Ice Sheet to a warming climate, The Cryosphere, 9, 1579–1600, https://doi.org/10.5194/tc-9-1579-2015, 2015. a
Davari, A., Baller, C., Seehaus, T., Braun, M., Maier, A., and Christlein, V.: Pixelwise Distance Regression for Glacier Calving Front Detection and Segmentation, IEEE T. Geosci. Remote, 60, 1–10, https://doi.org/10.1109/TGRS.2022.3158591, 2022a. a
Davari, A., Islam, S., Seehaus, T., Hartmann, A., Braun, M., Maier, A., and Christlein, V.: On Mathews Correlation Coefficient and Improved Distance Map Loss for Automatic Glacier Calving Front Segmentation in SAR Imagery, IEEE T. Geosci. Remote, 60, 1–12, https://doi.org/10.1109/TGRS.2021.3115883, 2022b. a
Davison, B. J., Hogg, A. E., Moffat, C., Meredith, M. P., and Wallis, B. J.: Widespread increase in discharge from west Antarctic Peninsula glaciers since 2018, The Cryosphere, 18, 3237–3251, https://doi.org/10.5194/tc-18-3237-2024, 2024. a
Dupont, T. and Alley, R.: Assessment of the importance of ice-shelf buttressing to ice-sheet flow, Geophys. Res. Lett., 32, L04503, https://doi.org/10.1029/2004GL022024, 2005. a
ENVEO: Ice Flow and Calving Front – Timeseries, ENVEO [data set], https://cryoportal.enveo.at/iv/calvingfront/, last access: 8 September 2023. a
ENVEO: Greenland Calving Front Dataset, 1990–2016, v3.0, ENVEO [data set], http://products.esa-icesheets-cci.org/products/downloadlist/CFL, (last access: 11 November 2021), 2017. a
Friedl, P., Seehaus, T. C., Wendt, A., Braun, M. H., and Höppner, K.: Recent dynamic changes on Fleming Glacier after the disintegration of Wordie Ice Shelf, Antarctic Peninsula, The Cryosphere, 12, 1347–1365, https://doi.org/10.5194/tc-12-1347-2018, 2018. a, b
GDAL/OGR contributors: GDAL/OGR Geospatial Data Abstraction software Library, Open Source Geospatial Foundation, https://gdal.org (last access: 8 January 2025), 2020. a
Glasser, N., Scambos, T., Bohlander, J., Truffer, M., Pettit, E., and Davies, B.: From ice-shelf tributary to tidewater glacier: continued rapid recession, acceleration and thinning of Röhss Glacier following the 1995 collapse of the Prince Gustav Ice Shelf, Antarctic Peninsula, J. Glaciol., 57, 397–406, https://doi.org/10.3189/002214311796905578, 2011. a
GLIMS Consortium: GLIMS Glacier Database (NSIDC-0272, Version 1), Boulder, Colorado USA, NASA National Snow and Ice Data Center Distributed Active Archive Center [data set], https://doi.org/10.7265/N5V98602, 2005. a, b, c
Goliber, S., Black, T., Catania, G., Lea, J. M., Olsen, H., Cheng, D., Bevan, S., Bjørk, A., Bunce, C., Brough, S., Carr, J. R., Cowton, T., Gardner, A., Fahrner, D., Hill, E., Joughin, I., Korsgaard, N. J., Luckman, A., Moon, T., Murray, T., Sole, A., Wood, M., and Zhang, E.: TermPicks: a century of Greenland glacier terminus data for use in scientific and machine learning applications, The Cryosphere, 16, 3215–3233, https://doi.org/10.5194/tc-16-3215-2022, 2022. a
Gourmelon, N., Seehaus, T., Braun, M., Maier, A., and Christlein, V.: Calving fronts and where to find them: a benchmark dataset and methodology for automatic glacier calving front extraction from synthetic aperture radar imagery, Earth Syst. Sci. Data, 14, 4287–4313, https://doi.org/10.5194/essd-14-4287-2022, 2022. a
Gourmelon, N., Seehaus, T., Braun, M. H., Maier, A., and Christlein, V.: CaFFe (CAlving Fronts and where to Find thEm: a benchmark dataset and methodology for automatic glacier calving front extraction from sar imagery), PANGAEA [data set], https://doi.org/10.1594/PANGAEA.940950, 2022. a
Greene, C. A., Gardner, A. S., Schlegel, N.-J., and Fraser, A. D.: Antarctic calving loss rivals ice-shelf thinning, Nature, 609, 948–953, https://doi.org/10.1038/s41586-022-05037-w, 2022. a
Heidler, K., Mou, L., Baumhoer, C., Dietz, A., and Zhu, X. X.: HED-UNet: Combined Segmentation and Edge Detection for Monitoring the Antarctic Coastline, IEEE T. Geosci. Remote, 60, 4300514, https://doi.org/10.1109/TGRS.2021.3064606, 2021. a, b
Heidler, K., Mou, L., Loebel, E., Scheinert, M., Lefèvre, S., and Zhu, X. X.: Deep Active Contour Models for Delineating Glacier Calving Fronts, in: IGARSS 2022–2022 IEEE International Geoscience and Remote Sensing Symposium, Kuala Lumpur, Malaysia, 2022, pp. 4490–4493, https://doi.org/10.1109/IGARSS46834.2022.9884819, 2022. a
Herrmann, O., Gourmelon, N., Seehaus, T., Maier, A., Fürst, J. J., Braun, M. H., and Christlein, V.: Out-of-the-box calving-front detection method using deep learning, The Cryosphere, 17, 4957–4977, https://doi.org/10.5194/tc-17-4957-2023, 2023. a
Hogg, A. E., Shepherd, A., Cornford, S. L., Briggs, K. H., Gourmelen, N., Graham, J. A., Joughin, I., Mouginot, J., Nagler, T., Payne, A. J., Rignot, E., and Wuite, J.: Increased ice flow in Western Palmer Land linked to ocean melting, Geophys. Res. Lett., 44, 4159–4167, 2017. a
Huber, J., Cook, A. J., Paul, F., and Zemp, M.: A complete glacier inventory of the Antarctic Peninsula based on Landsat 7 images from 2000 to 2002 and other preexisting data sets, Earth Syst. Sci. Data, 9, 115–131, https://doi.org/10.5194/essd-9-115-2017, 2017. a
Hulbe, C. L., Scambos, T. A., Youngberg, T., and Lamb, A. K.: Patterns of glacier response to disintegration of the Larsen B ice shelf, Antarctic Peninsula, Global Planet. Change, 63, 1–8, https://doi.org/10.1016/j.gloplacha.2008.04.001, 2008. a
Huttenlocher, D., Klanderman, G., and Rucklidge, W.: Comparing images using the Hausdorff distance, IEEE Transactions on Pattern Analysis and Machine Intelligence, 15, 850–863, https://doi.org/10.1109/34.232073, 1993. a
Kingma, D. P. and Ba, J.: Adam: A Method for Stochastic Optimization, arXiv [preprint], arXiv:1412.6980, 2014. a
Li, T., Heidler, K., Mou, L., Ignéczi, Á., Zhu, X. X., and Bamber, J. L.: A high-resolution calving front data product for marine-terminating glaciers in Svalbard, Earth Syst. Sci. Data, 16, 919–939, https://doi.org/10.5194/essd-16-919-2024, 2024. a
Loebel, E.: eloebel/glacier-front-extraction: Initial release v1.0.0, Zenodo [code], https://doi.org/10.5281/zenodo.7755774, 2023a. a
Loebel, E.: eloebel/rectilinear-box-method: Initial release v1.0.0, Zenodo [code], https://doi.org/10.5281/zenodo.7738605, 2023b. a
Loebel, E., Scheinert, M., Horwath, M., Heidler, K., Christmann, J., Phan, L. D., Humbert, A., and Zhu, X. X.: Extracting Glacier Calving Fronts by Deep Learning: The Benefit of Multispectral, Topographic, and Textural Input Features, IEEE T. Geosci. Remote, 60, 1–12, https://doi.org/10.1109/TGRS.2022.3208454, 2022. a, b
Loebel, E., Baumhoer, C. A., Dietz, A., Scheinert, M., and Horwath, M.: Glacier calving front locations for the Antarctic Peninsula Ice Sheet derived from remote sensing and deep learning from 2013 to 2023, PANGAEA [data set], https://doi.org/10.1594/PANGAEA.963725, 2024a. a, b, c
Loebel, E., Baumhoer, C. A., Dietz, A., Scheinert, M., and Horwath, M.: Terminus area change of 42 key glaciers of the Antarctic Peninsula from 2013 to 2023 derived from remote sensing and deep learning, TU Dresden OpARA [data set], https://doi.org/10.25532/OPARA-557, 2024b. a
Loebel, E., Baumhoer, C. A., Dietz, A., Scheinert, M., and Horwath, M.: Manually delineated glacier calving front locations of 20 marine-terminating glaciers of the Antarctic Peninsula from 2013 to 2023, TU Dresden OpARA [data set], https://doi.org/10.25532/OPARA-581, 2024c. a
Loebel, E., Scheinert, M., Horwath, M., Humbert, A., Sohn, J., Heidler, K., Liebezeit, C., and Zhu, X. X.: Calving front monitoring at a subseasonal resolution: a deep learning application for Greenland glaciers, The Cryosphere, 18, 3315–3332, https://doi.org/10.5194/tc-18-3315-2024, 2024d. a, b, c, d, e
Loebel, E., Scheinert, M., Horwath, M., Humbert, A., Sohn, J., Heidler, K., Liebezeit, C., and Zhu, X. X.: Manually delineated glacier calving front locations of 27 marine-terminating glaciers from 2013 to 2021, TU Dresden OpARA [data set], https://doi.org/10.25532/OPARA-282, 2024e. a
Marochov, M., Stokes, C. R., and Carbonneau, P. E.: Image classification of marine-terminating outlet glaciers in Greenland using deep learning methods, The Cryosphere, 15, 5041–5059, https://doi.org/10.5194/tc-15-5041-2021, 2021. a
Mohajerani, Y., Wood, M., Velicogna, I., and Rignot, E.: Detection of Glacier Calving Margins with Convolutional Neural Networks: A Case Study, Remote Sens., 11, 74, https://doi.org/10.3390/rs11010074, 2019. a
Moon, T. and Joughin, I.: Changes in ice front position on Greenland's outlet glaciers from 1992 to 2007, J. Geophys. Res.-Earth Surf., 113, F02022, https://doi.org/10.1029/2007JF000927, 2008. a
Ochwat, N. E., Scambos, T. A., Banwell, A. F., Anderson, R. S., Maclennan, M. L., Picard, G., Shates, J. A., Marinsek, S., Margonari, L., Truffer, M., and Pettit, E. C.: Triggers of the 2022 Larsen B multi-year landfast sea ice breakout and initial glacier response, The Cryosphere, 18, 1709–1731, https://doi.org/10.5194/tc-18-1709-2024, 2024. a, b
Otosaka, I. N., Shepherd, A., Ivins, E. R., Schlegel, N.-J., Amory, C., van den Broeke, M. R., Horwath, M., Joughin, I., King, M. D., Krinner, G., Nowicki, S., Payne, A. J., Rignot, E., Scambos, T., Simon, K. M., Smith, B. E., Sørensen, L. S., Velicogna, I., Whitehouse, P. L., A, G., Agosta, C., Ahlstrøm, A. P., Blazquez, A., Colgan, W., Engdahl, M. E., Fettweis, X., Forsberg, R., Gallée, H., Gardner, A., Gilbert, L., Gourmelen, N., Groh, A., Gunter, B. C., Harig, C., Helm, V., Khan, S. A., Kittel, C., Konrad, H., Langen, P. L., Lecavalier, B. S., Liang, C.-C., Loomis, B. D., McMillan, M., Melini, D., Mernild, S. H., Mottram, R., Mouginot, J., Nilsson, J., Noël, B., Pattle, M. E., Peltier, W. R., Pie, N., Roca, M., Sasgen, I., Save, H. V., Seo, K.-W., Scheuchl, B., Schrama, E. J. O., Schröder, L., Simonsen, S. B., Slater, T., Spada, G., Sutterley, T. C., Vishwakarma, B. D., van Wessem, J. M., Wiese, D., van der Wal, W., and Wouters, B.: Mass balance of the Greenland and Antarctic ice sheets from 1992 to 2020, Earth Syst. Sci. Data, 15, 1597–1616, https://doi.org/10.5194/essd-15-1597-2023, 2023. a, b
Pattyn, F. and Morlighem, M.: The uncertain future of the Antarctic Ice Sheet, Science, 367, 1331–1335, https://doi.org/10.1126/science.aaz5487, 2020. a
Periyasamy, M., Davari, A., Seehaus, T., Braun, M., Maier, A., and Christlein, V.: How to Get the Most Out of U-Net for Glacier Calving Front Segmentation, IEEE J. Sel. Top. Appl. Earth Obs., 15, 1712–1723, https://doi.org/10.1109/JSTARS.2022.3148033, 2022. a
Pritchard, H., Ligtenberg, S. R., Fricker, H. A., Vaughan, D. G., van den Broeke, M. R., and Padman, L.: Antarctic ice-sheet loss driven by basal melting of ice shelves, Nature, 484, 502–505, 2012. a
Rack, W. and Rott, H.: Pattern of retreat and disintegration of the Larsen B ice shelf, Antarctic Peninsula, Ann. Glaciol., 39, 505–510, https://doi.org/10.3189/172756404781814005, 2004. a
Raup, B., Racoviteanu, A., Khalsa, S. J. S., Helm, C., Armstrong, R., and Arnaud, Y.: The GLIMS geospatial glacier database: A new tool for studying glacier change, Global Planet. Change, 56, 101–110, https://doi.org/10.1016/j.gloplacha.2006.07.018, 2007. a
RGI Consortium: Randolph Glacier Inventory – A Dataset of Global Glacier Outlines, Version 6, Boulder, Colorado USA, National Snow and Ice Data Center [data set], https://doi.org/10.7265/4m1f-gd79, 2017. a
Rignot, E., Casassa, G., Gogineni, P., Krabill, W., Rivera, A., and Thomas, R.: Accelerated ice discharge from the Antarctic Peninsula following the collapse of Larsen B ice shelf, Geophys. Res. Lett., 31, L18401, https://doi.org/10.1029/2004GL020697, 2004. a, b
Ronneberger, O., Fischer, P., and Brox, T.: U-Net: Convolutional Networks for Biomedical Image Segmentation, In: Navab N., Hornegger J., Wells W., Frangi A. (eds) Medical Image Computing and Computer-Assisted Intervention – MICCAI 2015, 9351, 234–241, https://doi.org/10.1007/978-3-319-24574-4_28, 2015. a
Rott, H., Skvarca, P., and Nagler, T.: Rapid collapse of northern Larsen ice shelf, Antarctica, Science, 271, 788–792, https://doi.org/10.1126/science.271.5250.788, 1996. a
Rott, H., Müller, F., Nagler, T., and Floricioiu, D.: The imbalance of glaciers after disintegration of Larsen-B ice shelf, Antarctic Peninsula, The Cryosphere, 5, 125–134, https://doi.org/10.5194/tc-5-125-2011, 2011. a
Rott, H., Abdel Jaber, W., Wuite, J., Scheiblauer, S., Floricioiu, D., van Wessem, J. M., Nagler, T., Miranda, N., and van den Broeke, M. R.: Changing pattern of ice flow and mass balance for glaciers discharging into the Larsen A and B embayments, Antarctic Peninsula, 2011 to 2016, The Cryosphere, 12, 1273–1291, https://doi.org/10.5194/tc-12-1273-2018, 2018. a
Scambos, T. A., Berthier, E., and Shuman, C. A.: The triggering of subglacial lake drainage during rapid glacier drawdown: Crane Glacier, Antarctic Peninsula, Ann. Glaciol., 52, 74–82, https://doi.org/10.3189/172756411799096204, 2011. a
Seehaus, T., Marinsek, S., Helm, V., Skvarca, P., and Braun, M.: Changes in ice dynamics, elevation and mass discharge of Dinsmoor–Bombardier–Edgeworth glacier system, Antarctic Peninsula, Earth Planet. Sc. Lett., 427, 125–135, https://doi.org/10.1016/j.epsl.2015.06.047, 2015. a, b
Seehaus, T., Marinsek, S., Helm, V., Skvarca, P., and Braun, M. H.: Surface velocity fields, digital elevation models, ice front positions and grounding line derived from remote sensing data at Dinsmoor-Bombardier-Edgeworth glacier system, Antarctic Peninsula (1992–2014), PANGAEA [data set], https://doi.org/10.1594/PANGAEA.859573, 2015. a, b, c
Seehaus, T., Marinsek, S., Skvarca, P., van Wessem, J. M., Tijm-Reijmer, C. H., Seco, J., and Braun, M. H.: Surface velocity fields, digital elevation models and ice front positions derived from multi-mission SAR remote sensing data at Sjögren Inlet glaciers, Antarctic Peninsula, PANGAEA [data set], https://doi.org/10.1594/PANGAEA.859255, 2016. a, b
Seehaus, T., Cook, A. J., Silva, A. B., and Braun, M.: Changes in glacier dynamics in the northern Antarctic Peninsula since 1985, The Cryosphere, 12, 577–594, https://doi.org/10.5194/tc-12-577-2018, 2018. a
Seehaus, T. C., Marinsek, S., Skvarca, P., Van Wessem, J. M., Reijmer, C. H., Seco, J. L., and Braun, M. H.: Dynamic response of sjögren inlet glaciers, antarctic peninsula, to ice shelf breakup derived from multi-mission remote sensing time series, Front. Earth Sci., 4, 66, https://doi.org/10.3389/feart.2016.00066, 2016. a
Slater, T., Hogg, A. E., and Mottram, R.: Ice-sheet losses track high-end sea-level rise projections, Nat. Clim. Change, 10, 879–881, https://doi.org/10.1038/s41558-020-0893-y, 2020. a
Surawy-Stepney, T.: Data used in the article “The effect of landfast sea ice buttressing on ice dynamic speedup in the Larsen-B Embayment, Antarctica”, Zenodo [data set], https://doi.org/10.5281/zenodo.10580710, 2024. a, b
Surawy-Stepney, T., Hogg, A. E., Cornford, S. L., Wallis, B. J., Davison, B. J., Selley, H. L., Slater, R. A. W., Lie, E. K., Jakob, L., Ridout, A., Gourmelen, N., Freer, B. I. D., Wilson, S. F., and Shepherd, A.: The effect of landfast sea ice buttressing on ice dynamic speedup in the Larsen B embayment, Antarctica, The Cryosphere, 18, 977–993, https://doi.org/10.5194/tc-18-977-2024, 2024. a
Vieli, A. and Nick, F. M.: Understanding and Modelling Rapid Dynamic Changes of Tidewater Outlet Glaciers: Issues and Implications, Surv. Geophys., 32, 437–458, https://doi.org/10.1007/s10712-011-9132-4, 2011. a
Wallis, B. J., Hogg, A. E., van Wessem, J. M., Davison, B. J., and van den Broeke, M. R.: Widespread seasonal speed-up of west Antarctic Peninsula glaciers from 2014 to 2021, Nat. Geosci., 16, 231–237, https://doi.org/10.1038/s41561-023-01131-4, 2023a. a, b, c
Wallis, B. J., Hogg, A. E., van Wessem, J. M., and van den Broeke, M.: Data for Widespread seasonal speed-up of west Antarctic Peninsula glaciers from 2014–2021, Zenodo [data set], https://doi.org/10.5281/zenodo.7521416, 2023b. a, b
Zhang, E., Liu, L., Huang, L., and Ng, K. S.: An automated, generalized, deep-learning-based method for delineating the calving fronts of Greenland glaciers from multi-sensor remote sensing imagery, Remote Sens. Environ., 254, 112265, https://doi.org/10.1016/j.rse.2020.112265, 2021. a
Zhang, E., Catania, G., and Trugman, D. T.: AutoTerm: an automated pipeline for glacier terminus extraction using machine learning and a “big data” repository of Greenland glacier termini, The Cryosphere, 17, 3485–3503, https://doi.org/10.5194/tc-17-3485-2023, 2023. a, b, c
Zwally, H. J., Giovinetto, M. B., Beckley, M. A., and Saba, J. L.: Antarctic and Greenland drainage systems, GSFC cryospheric sciences laboratory [data set], https://earth.gsfc.nasa.gov/cryo/data/polar-altimetry/antarctic-and-greenland-drainage-systems (last access: 8 January 2025), 2012. a