the Creative Commons Attribution 4.0 License.
the Creative Commons Attribution 4.0 License.
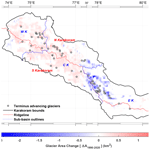
Interdecadal glacier inventories in the Karakoram since the 1990s
Fuming Xie
Yongpeng Gao
Tobias Bolch
Andreas Kääb
Shimei Duan
Wenfei Miao
Jianfang Kang
Yaonan Zhang
Xiran Pan
Caixia Qin
Kunpeng Wu
Miaomiao Qi
Xianhe Zhang
Ying Yi
Fengze Han
Xiaojun Yao
Xin Wang
Zongli Jiang
Donghui Shangguan
Yong Zhang
Richard Grünwald
Muhammad Adnan
Jyoti Karki
Muhammad Saifullah
Multi-temporal glacier inventories provide key information about the glaciers, their characteristics, and changes and are inevitable for glacier modelling and investigating geodetic mass changes. However, to date, no consistent multi-temporal glacier inventory for the whole of the Karakoram exists, negatively affecting the monitoring of spatio-temporal variations in glaciers' geometric parameters and their related applications. We used a semi-automatic method combining automatic segmentation and manual correction and produced a multi-temporal Karakoram glacier inventory (KGI) compiled from Landsat TM/ETM+/OLI (Thematic Mapper, Enhanced Thematic Mapper Plus, and Operational Land Imager) images for the 1990s, 2000s, 2010s, and 2020s. Our assessments using independent multiple digitisation of 37 glaciers show that the KGI is sufficiently accurate, with an overall uncertainty of ±3.68 %. We also performed uncertainty evaluation for the contiguous glacier polygons using a buffer of half a pixel, which resulted in an average mapping uncertainty of ±5.21 %. We calculated more than 20 attributes for each glacier, including coordinates, area, supraglacial debris area, date information, and topographic parameters derived from the ASTER GDEM (Advanced Spaceborne Thermal Emission and Reflection Radiometer global digital elevation model). According to KGI-2020s, approximately 10 500 alpine glaciers (>0.01 km2 each) cover an area of 22 510±828 km2 of which 10.18±0.38 % (2290±84 km2) is covered by supraglacial debris. Over the past 3 decades, the glaciers experienced a loss of clean ice and/or snow area but a gain in supraglacial debris. Supraglacial debris cover has increased by 17.63±1.44 % (343.30±27.95 km2), while non-debris-covered glaciers decreased by 1.56±0.24 % (319.85±49.92 km2). The total glacier area was relatively stable and showed only a slight insignificant increase of 23.45±28.85 km2 (0.10±0.13 %). The glacier area has declined by 3.27±0.24 % in the eastern Karakoram, while the glacier area slightly increased in central (0.65±0.10 %) and western Karakoram (1.26±0.11 %). Supraglacial debris has increased over the whole of Karakoram, especially in areas above 4200 m a.s.l. (above sea level), showing an upward shift. The glacier area changes were characterised by strong spatial heterogeneity, influenced by surging and advancing glaciers. However, due to global warming, the glaciers are on average retreating. This is in particular true for small and debris-free glaciers. The multi-temporal KGI data are available at the National Cryosphere Desert Data Center of China: https://doi.org/10.12072/ncdc.glacier.db2386.2022 (F. Xie et al., 2022).
- Article
(12752 KB) - Full-text XML
-
Supplement
(3098 KB) - BibTeX
- EndNote
The Karakoram mountains are centred on the western Tibetan Plateau (see Fig. 1a and b) and host more than 20 000 km2 of glaciers, making this region one of the most glacierised areas outside of the polar regions. As the main source of the rivers' natural flow, meltwater from the Karakoram glaciers has a significant influence on the socio-economic development in the upper Indus River basin and the Tarim River basin areas to the north of the mountains (Winiger et al., 2005; Liu et al., 2006, 2020; Immerzeel et al., 2020; Nie et al., 2021). Due to global warming in recent decades, changes in glacier-related resources and hazards present threats to the safety of critical infrastructure and the sustainable livelihoods of local communities living within the basin (Immerzeel et al., 2020; Bazai et al., 2021; Gao et al., 2021). In the past few decades, remote-sensing-based mass balance, glacier flow, and surge or advance characteristics indicate that the glaciers in Karakoram are exhibiting anomalous behaviour (Hewitt, 2005; Gardelle et al., 2012; Kääb et al., 2012; Bolch et al., 2017; Dehecq et al., 2018; Farinotti et al., 2020; Wu et al., 2020, 2021).
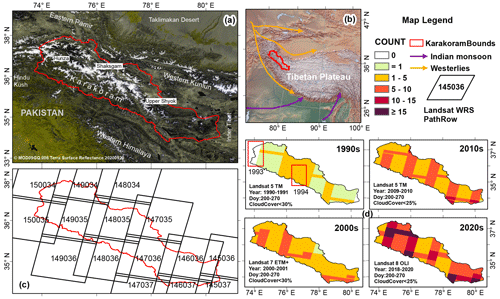
Figure 1Overview of the study region (Karakoram mountains). (a) Karakoram mountains physiography. (b) General climatology trends in Central Asia. The orange and blue arrow lines represent the westerly and monsoon directions, respectively (Yao et al., 2012). The Karakoram boundary is a reasonable revised boundary with reference to Bhambri et al. (2017) and can be accessed freely via https://github.com/1923xfmingynu/Subdivision-Of-High-mountain-Asia (last access: 14 February 2023). (c) Worldwide Reference System (WRS) path and row values of Landsat images used in this study. The blue area represents the glaciated area. (d) Number of Landsat observations (COUNT) in each pixel; the details in the lower-left corner show the filtered image collection information: Landsat sensor name (TM/ETM+/OLI) and image acquisition time range, including year range, day of year (DOY), and cloud cover threshold. Due to the poor quality of satellite imagery in some areas (red rectangle) in the 1990s, two scenes from 1993 and 1994 are used in the image composite.
Glacier inventories provide fundamental baseline information about the glaciers and are needed for many applications (e.g. glacier mass balance, glacier modelling). Generally, a glacier inventory contains an identifier (ID), name (if available), location (i.e. coordinates), size, and other relevant information concerning each glacier (Paul et al., 2009; Paul, 2017). Since the 1970s, thanks to the development of remote sensing and geographic information system (GIS) technology, a series of international glacier inventories such as the World Glacier Inventory (WGI) and the Randolph Glacier Inventory (RGI), as well as glacier inventories submitted to GLIMS (Global Land Ice Measurements from Space), has been developed through the efforts of glaciologists from different countries (Müller and Scherler, 1980; Shih et al., 1980; Paul et al., 2002; Pfeffer et al., 2014). To date, several glacier inventories exist in the Karakoram area, some of which include information about supraglacial debris cover. These inventories include the Glacier Area Mapping for Discharge from the Asian Mountains (GAMDAM) glacier inventory (GGI15) (Nuimura et al., 2015) and its updated version (GGI18) (Sakai, 2019), the Second Chinese Glacier Inventory (SCGI) (Liu et al., 2015; Guo et al., 2015), the International Centre for Integrated Mountain Development (ICIMOD) glacier inventory (Bajracharya and Shrestha, 2011), and the Glaciers_cci (CCI) glacier inventory (Mölg et al., 2018). However, due to differences and deficiencies in the remotely sensed imagery, variable data years, and glacier outline extraction methods used in previous glacier inventories, their results have poor comparability, thus failing to meet the requirements of glacier change analysis. Moreover, the presented areas of glacier coverage differ substantially in parts for the different available inventories (Bolch, 2019; Bolch et al., 2019; Bhambri et al., 2022).
The two versions of the GAMDAM inventory (GGI15 and GGI18) were generated by manual delineation with the exclusion of glacierised areas steeper than 40∘. These inventories have a difference in area of 9430 km2. The differences can be mainly attributed to the omission of glacier areas above the bergschrund (Sakai, 2019). Moreover, the first one used Landsat images acquired around the year 2000, and the second one also used scenes spanning 1990–2010 in case the scenes used in the first version were unsuitable (Sakai, 2019). Whereas the ICIMOD inventory was compiled from Landsat TM/ETM+ (Thematic Mapper and Enhanced Thematic Mapper Plus) images acquired between 2005 and 2009, the SCGI inventory only includes part of the Karakoram mountains and uses the Landsat TM/ETM+ satellite images between 2007 and 2011. The CCI inventory differs somewhat because the clean ice/snow parts are identified automatically using the image ratio technique and are based upon the Landsat images from 1998 to 2002. The debris-covered areas were manually mapped with the support of coherence images generated using ALOS-1 PALSAR-1 (Advanced Land Observing Satellite-1 Phased Array type L-band Synthetic Aperture Radar) scenes from 2007 and 2009 (Mölg et al., 2018). In the RGI 6.0 (RGI Consortium, 2017), the glaciers for the Karakoram region were extracted from the CCI inventory. A further approach to constraining changes in Karakoram glaciers was presented by Rankl et al. (2014), who focused only on surge-type glaciers and large glaciers with lengths > 3 km and areas > 0.15 km2. Few studies present glacier area changes for smaller parts of the Karakoram (e.g. Minora et al., 2016, for the Central Karakoram National Park for 2001–2010 or Bhambri et al., 2013, for the Shyok Valley for 1973 to 2011). Both found overall no significant net are changes but large variability due to the presence of surging glaciers. However, consistent multi-temporal homogeneous glacier inventories covering the whole Karakoram mountains do not exist, thereby negatively affecting the monitoring of spatio-temporal variations in the glaciers' geometric parameters and hampering explanation of their anomalous behaviour discussed by both international scientists and policymakers.
In most parts of the Karakoram, debris-covered glaciers are dominating (∼10 % of glacier area covered by debris). Thus, if this debris cover and its evolution are neglected, hydrological models may underestimate the longevity of glacier-sourced water resources and eventually overestimate the effects of glacier melt on sea-level rise (Herreid and Pellicciotti, 2020; Zhang and Liu, 2021). Many studies have reported that the supraglacial debris cover is expanding in different parts of the world (Mölg et al., 2019; Tielidze et al., 2020; Xie et al., 2020b). Herreid et al. (2017) report no net change in the debris-covered area in central Karakoram from 1977 to 2014. However, variations in the debris cover across the whole Karakoram region remain unknown. Mapping glaciers with debris cover is still challenging (Paul et al., 2004; Pfeffer et al., 2014; Farinotti et al., 2020), with various data and approaches having been proposed to address this issue, including using SAR (synthetic aperture radar) coherence maps, optical bands, thermal infrared features, terrain parameters, machine learning, and other methods (Bhambri et al., 2011; Mölg et al., 2018; Alifu et al., 2020; Xie et al., 2020a).
The present study aims to present precise and homogeneous multi-temporal glacier inventories for the whole Karakoram. We developed a high-performance cloud-removal and image-compositing algorithm to produce high-quality images for the glacierised regions of Karakoram for the extraction of glacier outlines. These will provide important baseline data for glacier research and related applications.
2.1 Datasets
This subsection lists all datasets covering the Karakoram that are used to produce and assist in the analysis of the multi-temporal glacier inventory, including optical images from different satellite sensors, digital elevation models (DEMs), four previous glacier inventories, three supraglacial debris extents, two surge-type glacier inventories, two modelled ice thickness datasets, hydrological basins, and river networks. Table 1 summarises their key characteristics, presenting their sources, date, and access link.
At least 12 Landsat images are required to cover the glacierised area of the Karakoram (Fig. 1c). We selected level 1 terrain-corrected products of the Landsat TM/ETM+/OLI (Operational Land Imager) scene (L1TP) images with 30 m spatial resolution representing the years 1990–2020, including 65 TM, 39 ETM+, and 82 OLI images (Table S1 in the Supplement). The satellite images were identified and processed using Google Earth Engine (GEE). An Asia North Albers Equal Area Conic projection coordinate system with the central meridian 76∘ and the standard parallels 33 and 38∘ was used for all spatial data in this study. Firstly, the available images were initially filtered from the Landsat image collection by selecting the melting season (days of year: 200–270), region of interest (Karakoram boundary), and cloud cover (<25 %–30 %). The filter criteria depend on the data quality and observations recorded in different periods as shown in the lower-left corner of Fig. 1d. We set the image interval to 2 years to ensure representativeness and reliability of the composite image as much as possible, a value smaller than the interval of 3 years deemed accepted in previous report (Paul et al., 2013). For the period of the 1990s, suitable images within 1 or 2 years were not sufficient to cover the whole Karakoram area; thus, two images from 1993 and 1994 were also selected for areas with no observations or unsuitable cloud conditions (see red box in Fig. 1d). This resulted in a more than 3-year interval in these areas, thus maybe increasing the inter-annual variation error in the glacier outlines. Then, we applied GEE's own cloud-removal algorithm (ee.Algorithms.Landsat.simpleCloudScore) (Gorelick et al., 2017) to all the images and composited an image needed for glacier inventory. In the image compositing, if more than 10 observations were reached at a given point, only the observations from the first 10 cloudiness images were accepted to generate an image composite in order to reduce the data source complexity of the composite image.
To assess the uncertainty of our new glacier inventory data, we evaluated the glacier outlines derived from coarser-spatial-resolution satellite imagery using the glacier outlines identified from high-resolution images or mean value of multiple digitisations from lower-resolution data. In an ideal case the outlines should be digitised several times (multi-manual digitisation – MMD) to consider the variability in the analysts (“round-robin”) (Paul et al., 2013) (see Sect. 2.6). Therefore, several Sentinel–2 10 m scenes and a Planet 3m image are used.
Nuimura et al. (2015) used the ICESat GLA 14 (level-2 altimetry product) data to evaluate DEM accuracy data in glaciers of High Mountain Asia and found that the ASTER GDEM (Advanced Spaceborne Thermal Emission and Reflection Radiometer global digital elevation model) version 2 (v2) is more suitable in comparison to the Shuttle Radar Topography Mission (SRTM) DEM. Compared to ASTER GDEM v2, the updated v3 product has fewer void areas due to an increased amount of ASTER stereo image data and further developed processing that improves the vertical and horizontal accuracy of the data (Abrams, 2016). Therefore, topographic information (e.g. slope, aspect, and median elevation) for all glaciers and the generation of ridgelines (see Sect. 3.3) in this study was obtained from ASTER GDEM v3 data. All ASTER GDEM v3 tiles were downloaded from the Land Processes Distributed Active Archive Center and mosaicked in the local Python GADL environment.
In the preliminary extraction of glacier outlines (see Sect. 2.2), we relied on the previous glacier boundaries, and we qualitatively compared area differences between different glacier inventories and supraglacial debris extents, as well as illuminated the variations in surge-type glaciers. Therefore, four previous glacier inventories, i.e. GGI18, CCI, SCGI, and ICIMOD; three supraglacial debris extents (Mölg et al., 2018; Scherler et al., 2018; Herreid and Pellicciotti, 2020); and two surge-type glacier inventories (Bhambri et al., 2017; Guillet et al., 2022) were used in this study without any modifications.
Furthermore, in the results and discussion, we analysed glacier changes in different sub-basins and highlighted the value of multi-temporal glacier inventories in ice volume calculation. Hydrological basins provided by the HydroSHEDS project (Lehner and Grill, 2013) and two published simulated ice thickness datasets (Farinotti et al., 2019; Millan et al., 2022a) were also used.
2.2 Extraction of glacier outlines
Accurate glacier outline identification can be challenging; because glaciers exhibit a wide range of surface characteristics, they can be interpreted differently by the cartographer, surveyor, or remote sensing specialist (Paul, 2017; Paul et al., 2009). Moreover, poor-quality satellite imagery (i.e. scenes with clouds, large shadows, or seasonal snow cover), debris-covered glaciers, rock glaciers, and perennial snow-covered depressions (potential presence of some ice underneath) are all sources of uncertainty in glacier outline mapping that may negatively affect the accuracy of the resulting outlines. Therefore, we used a semi-automatic method (band ratio + manual correction) to compile glacier inventories. This approach is widely applied (Bolch et al., 2010a; Guo et al., 2015; Paul et al., 2017).
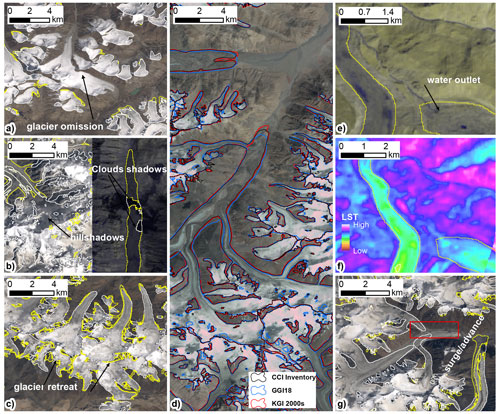
Figure 2Types of manual correction. (a) Glaciers missed in previous inventories. (b) Areas contaminated by hill shadows or cloud shadows. (c) Glaciers that have retreated and require updating. (d) Comparison of the revised glacier outlines with those from the CCI and GGI18 glacier inventories, with a base map of Landsat 7 ETM+ composited in the 2000s. Panels (e) and (f) are auxiliary methods for mapping debris-covered glaciers. The location of the water outlet is used to determine the position of the glacier tongue, and the land surface temperature images are used to distinguish between debris-covered glaciers and exposed bedrock. (g) Glacier terminus advance may be caused by glacier surges (red rectangle). Except for panel (d), the yellow and white lines represent the glaciers identified by the automatic threshold segmentation method as debris-covered and debris-free parts, respectively.
Firstly, we calculated the normalised difference snow index (NDSI) and initially considered areas with NDSI ≥ 0.4 as clean ice and/or snow (or debris-free areas) (Dozier, 1989; Xie et al., 2020b), while other areas were labelled as initial debris-covered ice, followed by manual correction (see next section). As previous studies have found, optimised thresholds for different periods can lead to the overestimation of glacier area (Xie et al., 2020b); additionally, the glacier area has insensitivity to different thresholds when using high-quality satellite images on gentler terrain (Guo et al., 2015). Accordingly, we adopted the empirical NDSI threshold uniformly across the whole Karakoram for mapping clean ice and/or snow. A similar threshold was also used for generating glacier inventories for large regions elsewhere (e.g. Ke et al., 2016). Second, since many pixels outside of the glacier extent are considered to be supraglacial debris in the initial debris-covered data, glacier outlines from previous glacier inventories were used as a mask to eliminate them, as suggested in similar studies (Bolch et al., 2010a; Scherler et al., 2018; Baumann et al., 2020). We combined two earlier glacier inventories (90 % CCI + 10 % GGI18, to fully cover Karakoram glaciers) as the mask layer for KGI-1990s (Karakoram glacier inventory), and the subsequent KGI-2000s, KGI-2010s, and KGI-2020s rely on the earlier revision of the glacier inventory (KGI-1990s, KGI-2000s, and KGI-2010s in that order). This approach is suitable for retreating glaciers but cuts off advancing glaciers. These were corrected by manual post-processing. Moreover, to remove the “salt-and-pepper” effect in the initial binary map, we applied a morphological filter with a 5×5 square kernel as described in Xie et al. (2020b). Then, the binary map was converted into vector format, retaining a “type” attribute describing whether each polygon is debris-covered ice or clean ice and/or snow, followed by the manual correction.
2.3 Manual correction
There are five typical error types in initial glacier outlines that require revision, including errors due to glacier omission (Fig. 2a), clouds or shadow (Fig. 2b), glacier commission (Fig. 2c), glacier advance or surge (Fig. 2g), and errors due to debris and ponds. To eliminate these errors, we have established detailed criteria and applied manual correction of multi-temporal glacier outlines using Landsat SWIR–NIR–RED (near-infrared, short-wave infrared, and red) or NIR–RED–GRE (near-infrared, red, and green) (false) colour composites of images (30 m resolution). These were combined with other optional baseline data, such as the 15 m resolution panchromatic Landsat band, the land surface temperature (LST) map derived from the Landsat thermal infrared band (Kraaijenbrink et al., 2017; Liao et al., 2020), contour lines derived from ASTER GDEM, and Google Earth images. Most of the debris-covered ice sections were remapped using the panchromatic band, LST, topography, and Google Earth images, while the ice tongues of debris-covered glaciers were identified by the location of their meltwater outlets (see Fig. 2e and f). The emergence of ice cliffs and ponds on glacier surfaces was also used in our present glacier inventory to identify the site of debris-covered glaciers and classify them as part of the debris-covered ice. Paul et al. (2013) observed that there are large uncertainties in manually identified glacier outlines due to the influence of seasonal snow cover, shadows, and complex underlying glacier surface types, such as supraglacial debris cover, rock glaciers, and stagnant ice, resulting in large deviations in the glacier extent even by different experienced interpreters. This issue is especially prevalent for glacier tongues with thick debris, which completely depend on visual interpretation to map the glacier, thus leading to further uncertainties. Therefore, to reduce the artificial interferences caused by subjectivity, all the correction work in this study was performed by the main author only.
2.4 Ice divides
Segmentation of complex glacier polygons, such as glaciers connected in their accumulation areas, can be achieved by using ridgelines derived from a DEM (Mölg et al., 2018). Thus, we adopted the method proposed by Bolch et al. (2010a), using the ASTER GDEM v3 dataset. First, we merged the four periods of glacier outlines into a new version with a 1 km buffer. This was then used to clip the sink-filled DEM. A flow direction grid was then calculated to generate a raw drainage basin using watershed analysis (Fig. 3). Then manual corrections were performed to delete small basins (<0.01 km2), based on a three-dimensional topographic map derived from the DEM datasets and a hill-shadow (see the base map of Fig. 3). The ridgelines were then extracted to split glacier complexes into single glaciers. After identifying the ice divides, we performed some batch processing on the revised glacier outlines, including filling voids (eliminating contained parts only) with an area of less than 4000 m2 (i.e. less than 5 pixels), deleting small supraglacial debris patches (<4000 m2), and eliminating glaciers smaller than 0.01 km2. This resulted in glacier area changes of only 1.63 to max 6.97 km2 in the different periods.
2.5 Attribute data
We included more than 20 attributes for each analysed glacier. In addition to the GLIMS ID linked from the RGI inventory, each glacier was given a unique KGI ID according to the GLIMS ID encoding style that is automatically calculated via Python programming using the centroid latitude and longitude of the glacier polygon. For terrain attributes, the minimum, median, and maximum elevation, plus median slope and aspect, were calculated from the ASTER GDEM v3. Since in particular large glaciers (>5 km2) may consist of different branches and tributary glaciers with different aspects or glaciers with large bends, the resulting representativeness of the average aspect may be poor; we thus also appended an attribute of a manually identified aspect according to the approximate orientation of each glacier. In addition, other information, such as longitude, latitude, glacier area, debris area, name, date, glacier surface type (clean ice or debris), terminus status (whether advancing), and the name of the operator were included. For the corresponding date of all inventoried glaciers, we not only provide the year range of the composited image dates but also calculate the probability that the edge pixels of the glaciers originate from a certain date. This is done on the premise that the inter-annual difference in pixels inside the glacier does not affect the accuracy of glacier extent. However, this assumption may affect the supraglacial debris cover. As shown in Fig. S1 in the Supplement, we generated a date image to track the source image for each pixel in the composited image; this indicated that the composite images had little inter-annual variation for most glaciers in the inventory, as the pixels in most composite scenes were derived from data from the same year.
2.6 Estimation of the uncertainty
Potential error sources of the glacier inventory include errors from satellite data quality (i.e. due to seasonal snow cover), DEMs, methods of glacier delineation, complex underlying glacier surface types, and quality of ridgelines (for single glaciers). In general, we applied two different uncertainty assessment methods. In the round-robin method, seven experienced participants repeatedly digitised 37 glaciers between 0.10 and 100 km2 in size, located in the Hunza Valley, western Karakoram, based on high-resolution images (Sentinel–2 and Planet) and lower-resolution images (Landsat 8), and the spatial locations are shown in Fig. S2. The standard deviation (SD) of the digitised glacier area (MMD results) values is used to evaluate the precision of the analysts' digitisation, and the difference between its mean area value (as the “truth” of glacier area) and the area calculated from the glacier inventory are used to determine which glacier extent has lower uncertainty, i.e. the KGI or the MMD outlines. Results of MMD are shown in Table S2. The digitisation on the Landsat OLI scene had an SD of 1.34 %–25.93 % (mean 2.29 %), with the largest value for the small glaciers and partly debris-covered glaciers (Fig. 4), while the SD of the Sentinel-2 scene was smaller with a mean of 1.28 % (from 0.48 % to 7.39 %). Apart from errors at debris-covered tongues or margins, interpretation differences in the accumulation area are considered a key error source, including differences in the interpretation of snow patches and glacier extents (Fig. 4). Additionally, scatter plots (Fig. S3) of the linear regression for both the MMD and KGI-2020s glacier area showed a strong positive correlation between them, with a correlation coefficient (r) value of 0.99 (p<0.001) and a root mean square error (RMSE) of 0.78 km2, thus confirming the high accuracy of the KGI data. The mean area difference in KGI outlines and the MMD mean values based on the Landsat image is −6.92 % but 5.58 % based on Sentinel–2 images. The mean area difference between the KGI outlines and the high-resolution image with only one digitisation is −6.39 % (Sentinel-2) and 2.56 % (Planet). The area difference for the different glaciers varied from −124.73 % to 35.24 % (−1.90 to 2.61 km2), influenced by glacier size, presence of tributary glaciers, and whether glaciers were debris-covered or debris-free. The resultant total mapping uncertainty is ±3.68 %, which meets the requirements of the Global Observing System for Climate (GCOS, 2016). The results presented above are consistent with previous accuracy assessments that reported the mean area differences between the automatically derived and manually digitised outlines in high-resolution images between 2 % and 5 % (Andreassen et al., 2008; Paul et al., 2013, 2017).
As a second measure of uncertainty, we applied the buffer method (Bolch et al., 2010a; Granshaw and Fountain, 2006) on the contiguous glacier polygons. Accordingly, a buffer of pixels (i.e. 15 m) for the KGI outlines (Fig. 5a) was generated, and the difference between the area of the buffer and the KGI was used as the uncertainty measure. The uncertainties for the four periods of KGI data are ±5.31 %, ±5.18 %, ±5.12 %, and ±5.21 %, with an average of ±5.21 %. In terms of the debris-covered areas, generally, a buffer of ±1 or 2 pixels (30 or 60 m) was suggested in previous research (Mölg et al., 2018; Paul et al., 2020). The uncertainty of the debris portion in this study was evaluated through the ratio of the glacier area to the debris-covered area multiplied by the uncertainty of a buffer of ±1 pixel (30 m) (Fig. 5b), resulting in uncertainties of ±27.89 %, ±29.39 %, ±28.20 %, and ±29.76 % for the four periods, with a mean value of ±28.81 %.
For the whole glacier, the mapping uncertainty based on the round-robin experiment is within the estimation range based on the buffer method, indicating that the round-robin value can be used as a reasonable estimation of the uncertainty. Hence, we used this value ( %) as the uncertainty value for all KGI data in this study. The area change uncertainty (σΔ) was estimated according to the standard error propagation as the root sum square of the uncertainty for outlines mapped from different periods, but it only considers the glacier parts which showed change in the 1990s and 2020s (ΔA1990s and ΔA2020s) (Bhambri et al., 2011; Zhang et al., 2018; Li et al., 2022), calculated as
3.1 Characteristics and status of the Karakoram glaciers
We mapped a total of 10 498 glaciers with an area of 22 510.73±828.39 km2 (Table 2). All glaciers are divided into nine areal classes of ≤0.05 to ≥100 km2. A total of 7941 (75.60 % of all glaciers) are smaller than 1 km2 but cover only a total area of 2098.45±77.22 km2 (9.30 % of the total). In total, 26 glaciers exceed 100 km2, which covers 7430.64±273.45 km2 or 32.50 % of the total glacier area in Karakoram. Siachen (1118.09±41.14 km2), Baltoro (849.44±31.26 km2), Biafo (579.25±21.32 km2), and Hispar (542.07±19.95 km2) glaciers are larger than 500 km2. A total of 20 % (2175) of the glaciers with an area within size classes 1–5 and 10–50 km2 cover a total area of 8519.63±313.52 km2. Glaciers with size classes 5–10 and 50–100 km2 are roughly equal in area, each class covering about 2200 km2. In total, 581 glaciers, ranging from 5 to 100 km2, cover 8736.78±321.51 km2, meaning that all these glaciers plus the 26 glaciers exceeding 100 km2 occupy 72 % of the total area of the Karakoram glaciers.
Table 2Number and area of glaciers according to different size classes or surface types during 1990–2020.
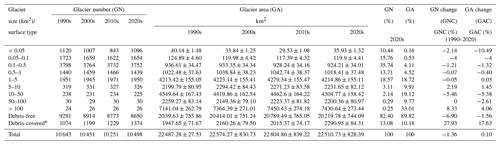
* Glacier number: number of debris-covered glaciers greater than 1 km2; glacier area: total area of all debris-covered ice.
We divided the Karakoram into northern and southern slopes (NK and SK) by ridgelines and into eastern, central, and western parts (EK, CK, and WK) using the Indus and Tarim sub-basin outlines (Fig. 6). A total of 55.30 % of the glaciers are concentrated in CK, covering an area of 12 547.12±461.73 km2, followed by the glaciers in the WK. Fewer than one-fifth, i.e. 3954.30±145.52 km2 or 17.60±0.89 %, of the total glaciers are located in the EK. A high proportion of debris cover is a typical feature of Karakoram glaciers, with a debris-covered percentage of 2.63±0.10 % (104.94±3.83 km2) in the eastern and 11.11±0.41 % (1382.68±50.88 km2) and 13.16±0.48 % (804.32±29.60 km2) in the central and western regions, respectively. Additionally, the glacier areas in NK and SK differ by about 20 %, 12 321.74±453.44 and 10 188.99±374.95 km2. However, the supraglacial debris cover in SK (1500.85±55.23 km2, 65.51±2.41 %) is around twice that recorded in NK (790.09±29.08 km2).
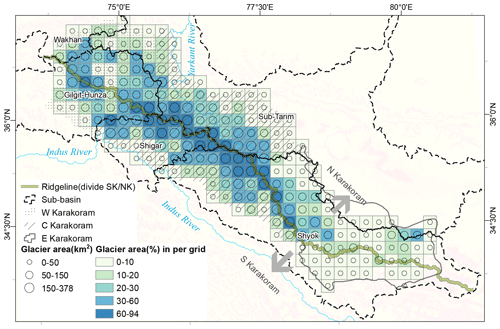
Figure 6Spatial distribution of Karakoram glaciers. For regional comparison, the Karakoram mountains were divided into western, central, and eastern Karakoram (WK, CK, and EK) according to the Indus and Tarim sub-basins, as well as into northern and southern Karakoram based on the main central ridgeline (green line). The major sub-basin divisions (Wakhan, Gilgit–Hunza, Shigar, sub-Tarim, and Shyok) in the Karakoram mountains are also shown. Hollow circles and coloured grids represent the glacier area and glacier proportion on the 20 km × 20 km grid.
Moreover, we divide the Karakoram mountains into five sub-basins: Gilgit–Hunza, Shigar, Shyok, Wakhan, and sub-Tarim (Fig. 6). Among these, the Shyok sub-basin housed the largest extent of glaciers (7918.54±291.40 km2), followed by the Tarim sub-basin (5574.06±205.13 km2) and then Gilgit–Hunza (4974.34±183.06 km2) and Shigar (3481.02±128.10 km2). The percentage of debris cover is greatest in the Shigar (16.64 %) and Gilgit–Hunza (14.72 %) basins, while the sub-Tarim basin has the lowest debris coverage (5.87 %).
3.2 Supraglacial debris cover of Karakoram glaciers
Supraglacial debris cover is widespread in the Karakoram. A total of 1848 debris-covered glaciers (17.63 % of all glaciers) with a total area of 2290.95±84.31 km2 of supraglacial debris area ( % of total glacier area) were mapped in KGI-2020s. The total area of debris-covered glaciers is more than 16 800 km2, of which 74.40 % of the glaciers (1374) are larger than 1 km2. The supraglacial debris area of glaciers smaller than 1 km2 is only 49.73±1.83 km2, accounting for 2.17±0.08 % of the total debris coverage. Hence, there is no substantial debris cover on these small glaciers. The debris-covered area of the 26 glaciers larger than 100 km2 dominates, representing almost half (47.5 %) of the total debris-covered area with an area of 1088.83±40.07 km2. This is followed by the 10–50 km2 size class glaciers, which cover a total area of 413.90±15.23 km2 (18.10±0.67 %). Also, despite the glacier number being on the same order of magnitude in size classes 5–10 and 50–100 km2, the latter's debris coverage (321.21±11.82 km2) is twice that of the former (170.76±6.28 km2), indicating that the larger the glacier is, the greater its likelihood and the severity of its surface debris cover will be.
From 1990 to 2020, the debris cover increased by 17.63±1.44 % (343.30±27.95 km2), compared to a 1.56±0.24 % decrease (319.85±49.92 km2) in the clean ice or snow part. All different size classes show debris cover increases on their surfaces (Table S3). Debris-covered areas on glaciers > 100 km2 and in size classes in 1–5 km2 present the most significant changes, increasing by 113.18±10.87 km2 (11.60±1.11 %) and 79.12±5.08 km2 (44.16±2.84 %), respectively. A similar trend was also found in glaciers smaller than 1 km2, in which the debris-covered area increased by 19.47±1.32 km2 (64.34±4.36 %) in total; however, this accounted for only 5.67 % of the total recorded debris increase. Except for glaciers smaller than 1 km2, the growth rates of debris coverage in size classes between 1–5 km2 ( %) and 5–10 km2 (32.14±2.12 %) are the most significant. Our results indicate that supraglacial debris cover is expanding on large debris-covered glaciers, while many small- and medium-sized debris-free glaciers are transforming into debris-covered glaciers. Over the study period, approximately 500 debris-free glaciers developed into debris-covered glaciers with a decadal growth rate of 12.20 % (i.e. about 166 transform into debris-covered glaciers every decade), more than 97 % of which are smaller than 10 km2. According to KGI-2020s, among the glaciers larger than 5 km2, there are 457 (14 379.96±529.18 km2) glaciers with supraglacial debris coverage greater than 0.1 km2. The area of these debris-covered glaciers increased by 98.06±13.43 km2 (0.69±0.09 %) from 1990 to 2020, while that of debris-free glaciers decreased by 12.72±2.64 km2 (0.68±0.14 %).
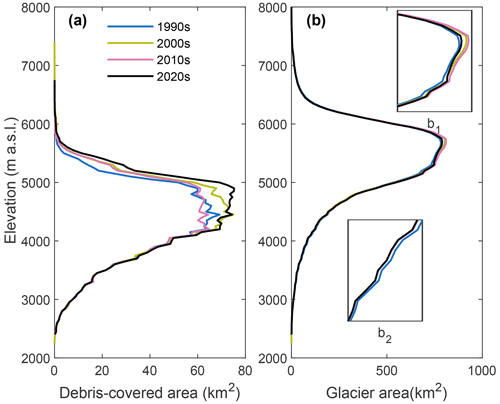
Figure 7Altitudinal profiles of the glacier surface area at 50 m intervals for debris-covered ice (a) and all glaciers (b), showing variations from 1990 to 2020. The subpanels (b1) and (b2) inserted in figure (b) correspond to zoomed-in sections of the altitude intervals of 5000–6000 and 3500–4000 m a.s.l.
3.3 Glacier elevation, slope, and aspect
The Karakoram glaciers have a wide range of elevations with a mean altitude of ∼5300 m a.s.l. (above sea level) and a median altitude of ∼5500 m a.s.l. (5200–6200 m a.s.l.). The hypsometry of the glacier area (Fig. 7a and b) indicates that the distribution of glaciers is concentrated at altitudes of 5300–5700 m a.s.l., whereas the debris-covered sections are primarily distributed at altitudes of 4000–5000 m a.s.l. and gradually disappear at altitudes above 6000 m a.s.l. The altitudinal profiles of the glacier surface area (Fig. 7b) indicate that glaciers lose area at low altitude, while near the altitude zone at 5000–6000 m a.s.l., it shows an increasing trend during 1990–2010, followed a decreasing trend since the 2010s. In terms of glacier orientation (Fig. 8a and b), most glaciers have a south, east, or southeast aspect (52.40 %), with only a few glaciers facing to the north. While there are equal numbers of east- and southeast-facing glaciers, the area of the southeast-facing glaciers is twice as large as that of the east-facing glaciers. Similarly, the area of the north-facing glaciers is not as small as that of the west-facing glaciers, which occupy the smallest area. The southeast- and north-facing glaciers in the Karakoram mountains are dominated by large glaciers. As depicted in Fig. 9a and b, the slope of all glaciers is mainly concentrated between 0 and 50∘, with almost no glaciers with slopes greater than 70∘, whereas supraglacial debris is widespread in areas with slopes less than 15∘. This may be due to broad, flat valley areas providing favourable terrain conditions for the accumulation and enrichment of supraglacial debris. Previous studies have used a slope value of less than 24∘ (Paul et al., 2004) or 25∘ (Xie et al., 2020b) to limit the extent of debris, even though there are steep slopes with debris, which may result from steep ice cliffs or crevasses. Overall, compared to debris-free glaciers, debris-covered glaciers have a broader altitude range (i.e. lower minimum elevation and higher maximum elevation) and lower slopes (Table S4).
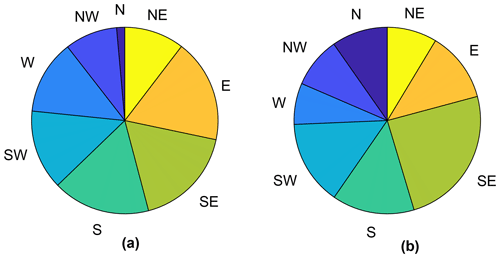
Figure 8The pie charts show the distributions of glaciers' number (a) and area (b) according to the aspect.
4.1 Comparison with previous glacier inventories
In order to determine major differences between previous glacier inventories and our glacier inventory in the Karakoram, we compared it with the SCGI (5114.68 km2, glacier area within the KGI range), ICIMOD (11 612.82 km2), CCI (20 444.40 km2), and GGI18 (20 036.55 km2) inventories. However, due to the different approaches, data sources, and methods among different glacier inventories, it cannot be compared without a high level of uncertainty, so this is only a qualitative comparison. The Karakoram boundary used by us is different from that in previous studies (Bolch et al., 2012, 2019; Bhambri et al., 2022), so the qualitative comparison is conducted only for areas covered by both inventories. Among these, the SCGI and ICIMOD inventories were compared with KGI-2010s. We then contrasted the GGI18 and CCI inventories with KGI-2000s to minimise the inter-annual variation between the compared data. For comparison the glacier areas were aggregated onto a 5 km × 5 km grid (Fig. 10). Our results indicate that there is a substantial difference between the ICIMOD inventory and KGI data. In some areas, the glacier area in a single grid cell is underestimated by 15.38 km2 (253.40 %), with a total RMSE of 3.78 km2. The GGI18 and SCGI inventories exhibited differences from the KGI data between 1.45–7.11 and 1.34–8.60 km2, with RMSE values of 1.41 and 1.53 km2, respectively. The difference between the CCI inventory and KGI-2000s was found to be the smallest, with RMSE and r values of 0.65 km2 and 0.99 (p<0.001), while the difference in glacier area per grid was between 2.42–3.32 km2. The clear underestimation of glacier area in the ICIMOD inventory may relate to the fact that clean ice and/or snow cover at high altitudes, steep headwalls, and areas affected by hill shadows or clouds were not considered (Bajracharya and Shrestha, 2011) (see also Fig. S4). Similar factors still affect the updated GAMDAM inventory (GGI18), resulting in glacier area values smaller than ours in most regions; in contrast, the difference between the CCI and KGI inventories was relatively small because a similar approach was adopted, with only differences in data source and interpreter (see Fig. 2d). In addition to the above factors, different interpreters' identification of debris-covered glaciers and inter-annual variation between comparative data are potential error sources in all glacier inventories. To sum up, such glacier inventories produced through different methodological standards, data sources, and interpreters contain many uncertainties from different sources, and therefore homogeneous multi-temporal glacier inventories are required to calculate glacier changes. Multi-temporal homogeneous glacier inventories are an important data source either for basic glacier outliers or as a validation set when computing glacier mass changes and associated run-off from projection models. Based on published ice thickness data, we calculated the ice volume in Karakoram is (Farinotti et al., 2019) or km3 (Millan et al., 2022b), which has a potential contribution to sea-level rise of 4.88±1.27 or 7.11±3.07 mm. Taking into account different glacier extents in different periods, these projections will produce a variable error of 0.36 %–0.49 %.
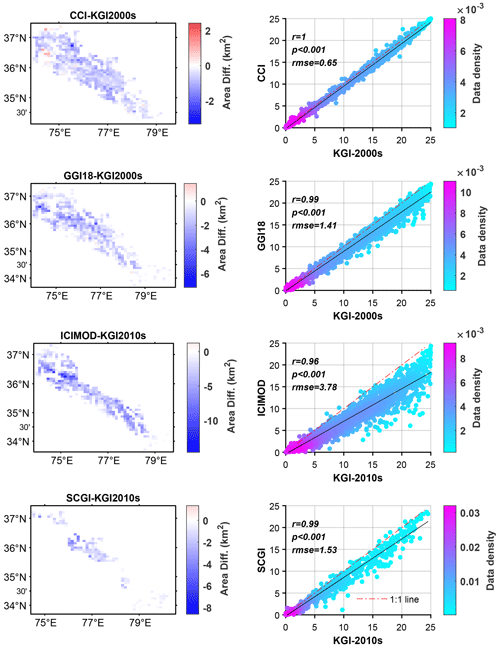
Figure 10Comparison between the KGI inventory and previous glacier inventories. All data are aggregated on a 5 km × 5 km grid. The map in the left column represents the area difference between previous glacier inventories and the KGI inventory from the same or closest period (SCGI and ICIMOD inventories are compared with KGI-2010s, while the updated GAMDAM (GGI18) and CCI inventories are compared with KGI-2000s). The right column illustrates, from top to bottom, the scattergrams of glacier area in each 5 km grid cell of the KGI inventory plotted against the GGI18, CCI, ICIMOD, and SCGI inventories in the Karakoram mountains.
We performed another comparison in regions where both previous data and our inventory mapped supraglacial debris. The results indicate that the debris-covered area in the CCI inventory is higher (18.47 km2) than that of KGI-2000s, while the total debris cover mapped by Scherler et al. (2018) is much lower than KGI-2020s (in total, ∼18 % less). The latter is a particularly large difference, which may be related to the inter-annual variation (5 years), lack of checks or manual correction, and overlooking the terminal changes caused by glacier surges or advances in the results extracted by Scherler et al. (2018). Moreover, the supraglacial debris cover on glaciers larger than 2 km2 mapped by Herreid and Pellicciotti (2020) using Landsat images from 1986 to 2016 (median 2013) was 2095.96 km2, while that of area for glaciers in four periods of KGI were 1859.24, 2045.21, 1931.95, and 2163.05 km2 (mean 1999.86 km2, ∼5 % less). Hence, compared to previous glacier inventory or supraglacial debris data, our KGI data have obvious advantages in aspects including Landsat image compositing, glacier initial outline mapping, and manual correction. Thus, the quality of KGI data is considered to be reliable and sufficiently accurate.
4.2 Spatial-temporal variations in the Karakoram glaciers
The total glacier area in the Karakoram was overall relatively stable from 1990 to 2020, with a slight, insignificant increase of 23.45±28.85 km2 (0.10±0.13 %) on average. However, the total glacier number decreased by 1.36 % in this period. Small glaciers (<1 km2) experienced a strong decrease in area, particularly those smaller than 0.05 km2. In contrast, large glaciers with areas exceeding 100 km2 showed an increase in area which compensated for the total area decrease for smaller glaciers to some extent. In terms of time series, the total glacier area showed an increasing trend from 1990 to 2010 and a decreasing trend after the 2010s. This may indicate a weakening of the abnormal behaviour of glaciers in the Karakoram owing to the continuous warming.
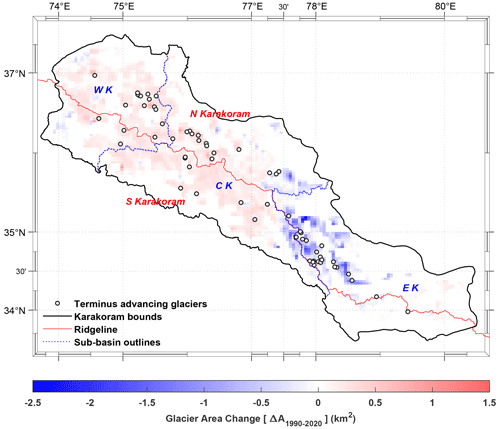
Figure 11Glacier change in the Karakoram during 1990–2020. Glacier area aggregated on a 5 km × 5 km grid. White dots represent the glaciers that advanced during the period 1990–2020. The Karakoram mountains were divided into western, central, and eastern Karakoram (WK, CK, and EK) according to the Indus and Tarim sub-basins, as well as into northern and southern Karakoram based on the main central ridgeline.
Our results indicate that the glacier area has declined by 3.27±0.24 % (133.50±9.84 km2) in EK, in contrast to a slightly increased area in CK (0.65±0.10 %, 80.89±12.61 km2) and WK (1.26±0.11 %, 76.06±6.49 km2) (Fig. 11, Table S5). This finding is also consistent with the negative mass balance for EK glaciers in the past decade and balanced budgets in other parts of the Karakoram (Vijay and Braun, 2018; Berthier and Brun, 2019). However, contrary to the area changes, the number of glaciers in CK and WK has decreased by 2.82 % and 2.24 %, respectively. Nevertheless, due to the influence of seasonal or annual variations in snow patches resulting in uncertainties in glacier number changes, especially for small glaciers, these changes might not be significant. Furthermore, our results show that glaciers on the northern slope of the Karakoram mountains are potentially undergoing a loss in area ( %), while the area of southern slope glaciers has potentially increased by 0.84±0.18 %. Hence, it is of particular importance to consider topographic conditions when explaining the spatial heterogeneity in glacier change, as also shown elsewhere (Xie and Liu, 2009; Garg et al., 2017). We suggest that this may be due to the wetter conditions, as well as the increased precipitation on the south side of the main divide, allowing glaciers in this area to continue to grow and store (Xie and Liu, 2009; Dimri, 2021).
The abundance of supraglacial debris may also partially explain the variability in glacier change observed in the Karakoram. The debris cover increased across the whole region, with EK experiencing the fastest growth (an increase of 105.05±5.16 % or 53.25±2.62 km2) during the past 3 decades. This trend was followed by CK (18.99±1.47 %, 220.65±17.09 km2) and WK (9.44±1.13 %, 69.40±8.27 km2). The debris cover in both NK and SK areas has increased by roughly the same order of magnitude (∼170 km2). On the southern slope of the Karakoram, 13.81±0.51 % of the glacierised areas are covered by debris, and the glacier area increased by 0.84±0.19 %, while on the northern slope, the glacier area shrank by 0.49±0.08 % under a relatively small debris coverage (5.60±0.21 %). These results show that there is a relationship between debris coverage and glacier area change, i.e. the area of glaciers with higher debris coverage is increasing or remaining stable and vice versa. From 1990 to 2020, the debris coverage has notably increased in areas above 4200 m a.s.l., indicating that supraglacial debris in the Karakoram was experiencing the same upward evolution. This was also observed in other mountain ranges such as the Greater Caucasus (Tielidze et al., 2020) and western Swiss Alps (Mölg et al., 2019) and is an inevitable evolution process of debris-covered glaciers under global warming (Herreid and Pellicciotti, 2020). Increased snow avalanche activity and rockfalls at high altitudes may have brought more debris to the glacier (Hewitt, 2005), thus leading to an increase in supraglacial debris, in addition to the effects of global warming, such as thinning of the glaciers, exposure of more englacial debris, and increased number of paraglacial slope failures (Zhang et al., 2011, 2015; Zhong et al., 2022).
By focusing on terminus changes, we observed that the advance exceeds the recession for most of the glaciers. Similar to Rankl et al. (2014), we identified 65 terminus-advancing glaciers with a total area of 1647.19±60.62 km2. In KGI-2020s, we found 63 single glacier units, since two of the glaciers had already become branches of other existing glaciers over the past 3 decades, all of which greatly contributed to the increase in glacier area by up to 25.33±0.93 km2. Figure 11 also shows a clear spatial coincidence between glacier regions with increased area and the distribution of the advancing glaciers, which presumably could also be confirmed by the amount of increased area and terminal change area of the advanced glaciers. In addition, our data show that most advancing glaciers (71.43 %) are surge-type glaciers, which represents abnormal glacier behaviour in the Karakoram compared to other regions in High Mountain Asia (Farinotti et al., 2020). The most recent inventory of surge-type glaciers (Guillet et al., 2022) has compiled 223 glaciers in the Karakoram, the number of which is consistent with the results of Bhambri et al. (2017), but the glaciers were not completely identical. In KGI-2020s, there are 607 glaciers in size classes greater than 5 km2, including 157 surge-type glaciers, which have expanded by 89.92±6.97 km2 (0.89±0.07 %) in total area and 136.03±14.73 km2 (10.61±1.15 %) in supraglacial debris coverage in the past 3 decades, while the 450 non-surge-type glaciers lost area of 4.58±6.61 km2 (0.08±0.11 %). Compared to normal glaciers, these anomalous glaciers (i.e. surging and advancing glaciers) are directly responsible for the area growth of the Karakoram glaciers.
Moreover, referring to the suggestions of Braithwaite and Raper (2009) and Sakai et al. (2015), we assume that median glacier elevation could act as a proxy for long-term equilibrium line altitude, which is correlated with the glacier mass balance budget and can be used to describe the state and fate of glaciers (Fujita and Nuimura, 2011). Among the five sub-basins in the Karakoram (see Fig. 6), as shown in Table S6, the median elevations of glaciers in the three basins with increasing glacier coverage decreased, while the altitudes increased in the basins with decreasing glacier area. Spatially, as pointed out by Bolch et al. (2012), the median elevations increase with the distance from the moisture source (Fig. S5). The glaciers in the northwest, exposed to the westerlies and heavily debris-covered, have a relatively low median elevation, while the glaciers north and northeast of the main ridge of the Karakoram have a clearly higher median elevation. On the whole, the median elevation of the Karakoram glaciers showed an increasing trend during 1990–2020, indicating that glacier melting likely is becoming more intense, the annual glacier run-off is moving towards a maximum (peak meltwater) (Huss and Hock, 2018; Nie et al., 2021). Especially in the melt-dominated Tarim and Indus basins, accelerated glacier melt is the main contributor to rising 21st century streamflow, which increases before peak water and then declines (Huss and Hock, 2018; Rounce et al., 2020; Nie et al., 2021).
4.3 Challenges in multi-temporal glacier inventories
Algorithms for identifying debris-covered glaciers have been developed, but the accuracy is too low if no manual corrections are applied. Like the method in the present study, semi-automated approaches are the most prevalent, which will always rely on existing glacier boundaries or time-consuming manual corrections (Paul et al., 2004; Bolch et al., 2007; Bhambri et al., 2011; Racoviteanu and Williams, 2012; Smith et al., 2015; Mölg et al., 2018). Although pixel-based machine learning has been used to map debris-covered ice (Alifu et al., 2020; Xie et al., 2020b; Z. Xie et al., 2022), isolated pixels, irregular outlines, or voids in the results have forced it to be revised to meet the needs of glacier inventories. In addition, available remotely sensed imagery in high quality (cloud-free, low or no seasonal snow, small shadows, geometrically calibrated) is required for precisely compiling glacier inventories. Even if satellite images from the end of the ablation season are used, some mapping errors may complicate the results for investigating glacier changes due to inter-annual variations in snow cover in the melting season or remaining seasonal snowfields (Yi et al., 2021). For example, during the 2010s when the overall glacier area was abnormal and the area of the clean ice and/or snow part was high, the identified debris cover was significantly lower as a result of the high snow cover in 2009 (see Fig. S6).
To obtain inventories of the time before higher-resolution multi-spectral earth-observation satellites were launched (e.g. Landsat 4, launched in 1982), topographic maps were often used to map glacier outlines. However, these have large uncertainties (Bhambri and Bolch, 2009; Bolch et al., 2010b; Guo et al., 2015; Paul, 2017). For the 1960s and 1970s, declassified Corona KH-4 (spatial resolution 8–2 m) and Hexagon KH-9 (12–8 m) data and Landsat Multispectral Scanner System (MSS; 60 m) images were used to compile inventories in some regions (Bolch et al., 2008, 2010b; Schmidt and Nüsser, 2012; Bhambri et al., 2013; Gardent et al., 2014; Pieczonka and Bolch, 2015; Weber et al., 2020; Bhattacharya et al., 2021). However, the available optical imagery used in current glacier inventories is often limited due to occlusion by frequent cloud layers during the melting season; this, in turn, increases the inter-annual variation in the inventory results and further prolongs the time interval between multi-temporal glacier inventories. Fortunately, this situation improved with the launch of Sentinel-2A and Sentinel-2B and Landsat 8 and 9, except in cloudy areas like the southeastern Tibetan Plateau. Although SAR coherence maps have been used in previous glacier inventories, to overcome these limitations in optical imagery, most focus on the identification of supraglacial debris (Frey et al., 2012; Robson et al., 2015; Ke et al., 2016; Mölg et al., 2018; Alifu et al., 2020), and only a few studies have been used to map glacier outlines (Atwood et al., 2014; Yang et al., 2016; Lippl et al., 2018). Therefore, in the future, greater availability of cloud computing platforms to develop SAR image processing algorithms and make extensive use of optical remote imagery will greatly help the development of multi-temporal glacier inventories. Moreover, if glacier extents in past periods can be reconstructed based on characteristic glacial landforms like lateral and terminal moraines (Shi and Liu, 2000) and using existing aerial imagery and satellite maps, the temporal resolution of multi-temporal glacier inventories can be extended, such as for glacier inventories from the Little Ice Age (e.g. Lucchesi et al., 2014; Meier et al., 2018).
The KGI datasets are available from the National Cryosphere Desert Data Center of China at https://doi.org/10.12072/ncdc.glacier.db2386.2022 (F. Xie et al., 2022) for users' assessments and applications. The data files contain shapefiles for the complete glaciers and the debris-covered section. Additionally, a description file is included.
Glacier inventories are the baseline information for many applications such as hydrological modelling in climate change studies. In this study, first we generated inventories which allowed us to systematically detect glacier change patterns in the Karakoram range over the past 3 decades by using Landsat scenes and ancillary data through a semi-automatic method based on the GEE cloud-based platform. Validation using the round-robin method showed an overall mapping uncertainty of ±3.68 % which is within the accuracy range required for glacier inventories by GCOS. We also compared the KGI with previous glacier inventories and evaluated it by applying uncertainties of buffers of pixels (15 m) and 1 pixel (30 m) to the glacier outlines. These assessments indicate that our results are reliable and sufficiently accurate. In the 2020s, there were approximately 10 500 glaciers in the Karakoram mountains, covering an area of 22 510.73±828.39 km2, of which 10.18±0.38 % (2290.95±84.31 km2) is covered by supraglacial debris. During the past 30 years, the glaciers experienced a loss of clean ice area and a gain in supraglacial debris. The total glacier area in the Karakoram remained relatively stable from 1990 to 2020, with a slight but insignificant increase of 23.45±28.85 km2 (0.10±0.13 %), while the supraglacial debris cover increased clearly by 17.63±1.44 % (343.30±27.95 km2), in contrast to a 1.56±0.24 % (319.85±49.92 km2) decrease in clean ice/snow. These glacier areal changes were characterised by strong spatial heterogeneity and dominated by surging and advancing glaciers. However, the glaciers are experiencing the negative effects of faster snow/ice melting caused by global warming, with small and clean glaciers being more sensitive. This represents an increasing threat of regional water scarcity and glacier-related hazards in the downstream basin. Most importantly, the KGI data in this study will provide basic glacier outlines for GLIMS and the forthcoming planned release of the next versions of the RGI, as well as for other glacier-related research applications.
The supplement related to this article is available online at: https://doi.org/10.5194/essd-15-847-2023-supplement.
SL designed the framework of the glacier inventory. FX programmed the glacier mapping algorithms, manually revised glacier outlines and ridgelines, and wrote the first manuscript. SL edited the first draft. TB reviewed and improved the manuscript. FX, YuZ, YG, SD, WM, FH, XR, and CQ digitised glaciers using Sentinel-2 imagery. JKan and YaZ assisted in publishing datasets. KW, XZ, and YY provided help in the production of figures. AK, XY, QL, XW, ZJ, DS, and YoZ discussed and improved the manuscript. RG, MA, JKar, and MS helped with English proofreading.
The contact author has declared that none of the authors has any competing interests.
Publisher's note: Copernicus Publications remains neutral with regard to jurisdictional claims in published maps and institutional affiliations.
We thank the Google Earth Engine developer for the freely available cloud-computing platform and USGS/NASA for Landsat imagery and the ASTER GDEM version 3 product. We acknowledge previous glacier inventory data from Glaciers_cci, GAMDAM glacier inventory, ICIMOD glacier inventory, and the Second Chinese Glacier Inventory for supporting this study results.
This research has been supported by the International Science and Technology Innovation Cooperation Program of the State Key Research and Development Program (grant no. 2021YFE0116800), the National Natural Science Foundation of China (grant no. 42171129), the Open Foundation from National Cryosphere Desert Data Center (grant no. 2021KF01), the Second Tibetan Plateau Scientific Expedition and Research programme (grant no. 2019QZKK0208), the Pakistan Science Foundation (grant no. PSF/CRP/Consrm-488), the Director Fund of the International Research Center of Big Data for Sustainable Development Goals (grant no. CBAS2022DF020), the Postgraduate Research and Innovation Foundation of Yunnan University (grant no. 2021Z018), and the Scientific Research Fund project of Yunnan Education Department (grant no. 2022Y059).
This paper was edited by Niccolò Dematteis and reviewed by Rakesh Bhambri and one anonymous referee.
Abrams, M.: Aster Global Dem Version 3, and New Aster Water Body Dataset, ISPRS - International Archives of the Photogrammetry, Remote Sens. Spat. Inform. Sci., XLI-B4, 107–110, https://doi.org/10.5194/isprsarchives-XLI-B4-107-2016, 2016.
Alifu, H., Vuillaume, J.-F., Johnson, B. A., and Hirabayashi, Y.: Machine-learning classification of debris-covered glaciers using a combination of Sentinel-1/-2 (SAR/optical), Landsat 8 (thermal) and digital elevation data, Geomorphology, 369, 107365, https://doi.org/10.1016/j.geomorph.2020.107365, 2020.
Andreassen, L. M., Paul, F., Kaab, A., and Hausberg, J. E.: Landsat-derived glacier inventory for Jotunheimen, Norway, and deduced glacier changes since the 1930s, The Cryosphere, 2, 131–145, https://doi.org/10.5194/tc-2-131-2008, 2008.
Atwood, D. K., Meyer, F., and Arendt, A.: Using L-band SAR coherence to delineate glacier extent, Can. J. Remote Sens., 36, S186–S195, https://doi.org/10.5589/m10-014, 2014.
Bajracharya, S. R. and Shrestha, B.: The Status of Glaciers in the Hindu Kush-Himalayan Region, International Centre for Integrated Mountain Development, Kathmandu, Nepal, https://doi.org/10.53055/ICIMOD.551, 2011.
Baumann, S., Anderson, B., Chinn, T., Mackintosh, A., Collier, C., Lorrey, A. M., Rack, W., Purdie, H., and Eaves, S.: Updated inventory of glacier ice in New Zealand based on 2016 satellite imagery, J. Glaciol., 67, 13–26, https://doi.org/10.1017/jog.2020.78, 2020.
Bazai, N. A., Cui, P., Carling, P. A., Wang, H., Hassan, J., Liu, D., Zhang, G., and Jin, W.: Increasing glacial lake outburst flood hazard in response to surge glaciers in the Karakoram, Earth-Sci. Rev., 212, 103432, https://doi.org/10.1016/j.earscirev.2020.103432, 2021.
Berthier, E. and Brun, F.: Karakoram geodetic glacier mass balances between 2008 and 2016: persistence of the anomaly and influence of a large rock avalanche on Siachen Glacier, J. Glaciol., 65, 494–507, https://doi.org/10.1017/jog.2019.32, 2019.
Bhambri, R. and Bolch, T.: Glacier mapping: a review with special reference to the Indian Himalayas, Prog. Phys. Geogr., 33, 672–704, https://doi.org/10.1177/0309133309348112, 2009.
Bhambri, R., Bolch, T., and Chaujar, R. K.: Mapping of debris-covered glaciers in the Garhwal Himalayas using ASTER DEMs and thermal data, Int. J. Remote Sens., 32, 8095–8119, https://doi.org/10.1080/01431161.2010.532821, 2011.
Bhambri, R., Bolch, T., Kawishwar, P., Dobhal, D. P., Srivastava, D., and Pratap, B.: Heterogeneity in glacier response in the upper Shyok valley, northeast Karakoram, The Cryosphere, 7, 1385–1398, https://doi.org/10.5194/tc-7-1385-2013, 2013.
Bhambri, R., Hewitt, K., Kawishwar, P., and Pratap, B.: Surge-type and surge-modified glaciers in the Karakoram, Sci. Rep., 7, 15391, https://doi.org/10.1038/s41598-017-15473-8, 2017.
Bhambri, R., Chand, P., Nüsser, M., Kawishwar, P., Kumar, A., Gupta, A. K., Verma, A., and Tiwari, S. K.: Reassessing the Karakoram Through Historical Archives, in: Environmental Change in South Asia: Essays in Honor of Mohammed Taher, edited by: Saikia, A. and Thapa, P., Springer International Publishing, Cham, 139–169, https://doi.org/10.1007/978-3-030-47660-1_8, 2022.
Bhattacharya, A., Bolch, T., Mukherjee, K., King, O., Menounos, B., Kapitsa, V., Neckel, N., Yang, W., and Yao, T.: High Mountain Asian glacier response to climate revealed by multi-temporal satellite observations since the 1960s, Nat. Commun., 12, 4133, https://doi.org/10.1038/s41467-021-24180-y, 2021.
Bolch, T.: Past and Future Glacier Changes in the Indus River Basin, in: Indus River Basin, edited by: Bolch, T., Elsevier Inc., 85–97, https://doi.org/10.1016/B978-0-12-812782-7.00004-7, 2019.
Bolch, T., Buchroithner, M. F., Kunert, A., and Kamp, U.: Automated delineation of debris-covered glaciers based on ASTER data, in: GeoInformation in Europe, edited by: Gomarasca, M. A., Bolch, T., Buchroithner, M. F., Kunert, A., and Kamp, U., Millpress, Netherlands, 403–410, ISBN 978-90-5966-061-8, 2007.
Bolch, T., Buchroithner, M., Pieczonka, T., and Kunert, A.: Planimetric and volumetric glacier changes in the Khumbu Himal, Nepal, since 1962 using Corona, Landsat TM and ASTER data, J. Glaciol., 54, 592–600, https://doi.org/10.3189/002214308786570782, 2008.
Bolch, T., Menounos, B., and Wheate, R.: Landsat-based inventory of glaciers in western Canada, 1985–2005, Remote Sens. Environ., 114, 127–137, https://doi.org/10.1016/j.rse.2009.08.015, 2010a.
Bolch, T., Yao, T., Kang, S., Buchroithner, M. F., Scherer, D., Maussion, F., Huintjes, E., and Schneider, C.: A glacier inventory for the western Nyainqentanglha Range and the Nam Co Basin, Tibet, and glacier changes 1976–2009, The Cryosphere, 4, 419–433, https://doi.org/10.5194/tc-4-419-2010, 2010b.
Bolch, T., Kulkarni, A., Kääb, A., Huggel, C., Paul, F., Cogley, J. G., Frey, H., Kargel, J. S., Fujita, K., Scheel, M., Bajracharya, S., and Stoffel, M.: The State and Fate of Himalayan Glaciers, Science, 336, 310–315, https://doi.org/10.1126/science.1215828, 2012.
Bolch, T., Pieczonka, T., Mukherjee, K., and Shea, J.: Brief communication: Glaciers in the Hunza catchment (Karakoram) have been nearly in balance since the 1970s, The Cryosphere, 11, 531–539, https://doi.org/10.5194/tc-11-531-2017, 2017.
Bolch, T., Shea, J. M., Liu, S., Azam, F. M., Gao, Y., Gruber, S., Immerzeel, W. W., Kulkarni, A., Li, H., Tahir, A. A., Zhang, G., and Zhang, Y.: Status and Change of the Cryosphere in the Extended Hindu Kush Himalaya Region, in: The Hindu Kush Himalaya Assessment, edited by: Bolch, T., Shea, J. M., Liu, S., Azam, F. M., Gao, Y., Gruber, S., Immerzeel, W. W., Kulkarni, A., Li, H., Tahir, A. A., Zhang, G., and Zhang, Y., Springer, 209–255, https://doi.org/10.1007/978-3-319-92288-1_7, 2019.
Braithwaite, R. J. and Raper, S. C. B.: Estimating equilibrium-line altitude (ELA) from glacier inventory data, Ann. Glaciol., 50, 127–132, https://doi.org/10.3189/172756410790595930, 2009.
Dehecq, A., Gourmelen, N., Gardner, A. S., Brun, F., Goldberg, D., Nienow, P. W., Berthier, E., Vincent, C., Wagnon, P., and Trouvé, E.: Twenty-first century glacier slowdown driven by mass loss in High Mountain Asia, Nat. Geosci., 12, 22–27, https://doi.org/10.1038/s41561-018-0271-9, 2018.
Dimri, A. P.: Decoding the Karakoram Anomaly, Sci. Total Environ., 788, 147864, https://doi.org/10.1016/j.scitotenv.2021.147864, 2021.
Dozier, J.: Spectral Signature of Alpine Snow Cover from the Landsat Thematic Mapper, Remote Sens. Environ., 28, 9–22, https://doi.org/10.1016/0034-4257(89)90101-6, 1989.
Farinotti, D., Huss, M., Fürst, J. J., Landmann, J., Machguth, H., Maussion, F., and Pandit, A.: A consensus estimate for the ice thickness distribution of all glaciers on Earth, Nat. Geosci., 12, 168–173, https://doi.org/10.1038/s41561-019-0300-3, 2019.
Farinotti, D., Immerzeel, W. W., and Dehecq, A.: Manifestations and mechanisms of the Karakoram glacier Anomaly, Nat. Geosci., 13, 8–16, https://doi.org/10.1038/s41561-019-0513-5, 2020.
Frey, H., Paul, F., and Strozzi, T.: Compilation of a glacier inventory for the western Himalayas from satellite data: methods, challenges, and results, Remote Sens. Environ., 124, 832–843, https://doi.org/10.1016/j.rse.2012.06.020, 2012.
Fujita, K. and Nuimura, T.: Spatially heterogeneous wastage of Himalayan glaciers, P. Natl. Acad. Sci. USA, 108, 14011–14014, https://doi.org/10.1073/pnas.1106242108, 2011.
Gao, Y., Liu, S., Qi, M., Xie, F., Wu, K., and Zhu, Y.: Glacier-Related Hazards Along the International Karakoram Highway: Status and Future Perspectives, Frontiers in Earth Science, 9, 611501, https://doi.org/10.3389/feart.2021.611501, 2021.
Gardelle, J., Berthier, E., and Arnaud, Y.: Slight mass gain of Karakoram glaciers in the early twenty-first century, Nat. Geosci., 5, 322–325, https://doi.org/10.1038/ngeo1450, 2012.
Gardent, M., Rabatel, A., Dedieu, J.-P., and Deline, P.: Multitemporal glacier inventory of the French Alps from the late 1960s to the late 2000s, Global Planet. Change, 120, 24–37, https://doi.org/10.1016/j.gloplacha.2014.05.004, 2014.
Garg, P. K., Shukla, A., and Jasrotia, A. S.: Influence of topography on glacier changes in the central Himalaya, India, Global Planet. Change, 155, 196–212, https://doi.org/10.1016/j.gloplacha.2017.07.007, 2017.
GCOS: The Global Observing System for Climate: Implementation Needs (GCOS-200), WMO, https://library.wmo.int/index.php?lvl=notice_display&id=19838 (last access: 14 February 2023), 2016.
Gorelick, N., Hancher, M., Dixon, M., Ilyushchenko, S., Thau, D., and Moore, R.: Google Earth Engine: Planetary-scale geospatial analysis for everyone, Remote Sens. Environ., 202, 18–27, https://doi.org/10.1016/j.rse.2017.06.031, 2017.
Granshaw, F. D. and Fountain, A. G.: Glacier change (1958–1998) in the North Cascades National Park Complex, Washington, USA, J. Glaciol., 52, 251–256, https://doi.org/10.3189/172756506781828782, 2006.
Guillet, G., King, O., Lv, M., Ghuffar, S., Benn, D., Quincey, D., and Bolch, T.: A regionally resolved inventory of High Mountain Asia surge-type glaciers, derived from a multi-factor remote sensing approach, The Cryosphere, 16, 603–623, https://doi.org/10.5194/tc-16-603-2022, 2022.
Guo, W., Liu, S., Xu, J., Wu, L., Shangguan, D., Yao, X., Wei, J., Bao, W., Yu, P., Liu, Q., and Jiang, Z.: The second Chinese glacier inventory: data, methods and results, J. Glaciol., 61, 357–372, https://doi.org/10.3189/2015JoG14J209, 2015.
Herreid, S. and Pellicciotti, F.: The state of rock debris covering Earth's glaciers, Nat. Geosci., 13, 621–627, https://doi.org/10.1038/s41561-020-0615-0, 2020.
Herreid, S., Pellicciotti, F., Ayala, A., Chesnokova, A., Kienholz, C., Shea, J., and Shrestha, A.: Satellite observations show no net change in the percentage of supraglacial debris-covered area in northern Pakistan from 1977 to 2014, J. Glaciol., 61, 524–536, https://doi.org/10.3189/2015JoG14J227, 2017.
Hewitt, K.: The Karakoram Anomaly? Glacier Expansion and the `Elevation Effect', Karakoram Himalaya, Mt. Res. Dev., 25, 332–340, https://doi.org/10.1659/0276-4741(2005)025[0332:Tkagea]2.0.Co;2, 2005.
Huss, M. and Hock, R.: Global-scale hydrological response to future glacier mass loss, Nat. Clim. Change, 8, 135–140, https://doi.org/10.1038/s41558-017-0049-x, 2018.
Immerzeel, W. W., Lutz, A. F., Andrade, M., Bahl, A., Biemans, H., Bolch, T., Hyde, S., Brumby, S., Davies, B. J., Elmore, A. C., Emmer, A., Feng, M., Fernandez, A., Haritashya, U., Kargel, J. S., Koppes, M., Kraaijenbrink, P. D. A., Kulkarni, A. V., Mayewski, P. A., Nepal, S., Pacheco, P., Painter, T. H., Pellicciotti, F., Rajaram, H., Rupper, S., Sinisalo, A., Shrestha, A. B., Viviroli, D., Wada, Y., Xiao, C., Yao, T., and Baillie, J. E. M.: Importance and vulnerability of the world's water towers, Nature, 577, 364–369, https://doi.org/10.1038/s41586-019-1822-y, 2020.
Kääb, A., Berthier, E., Nuth, C., Gardelle, J., and Arnaud, Y.: Contrasting patterns of early twenty-first-century glacier mass change in the Himalayas, Nature, 488, 495–498, https://doi.org/10.1038/nature11324, 2012.
Ke, L., Ding, X., Zhang, L. E. I., Hu, J. U. N., Shum, C. K., and Lu, Z.: Compiling a new glacier inventory for southeastern Qinghai–Tibet Plateau from Landsat and PALSAR data, J. Glaciol., 62, 579–592, https://doi.org/10.1017/jog.2016.58, 2016.
Kraaijenbrink, P. D. A., Bierkens, M. F. P., Lutz, A. F., and Immerzeel, W. W.: Impact of a global temperature rise of 1.5 degrees Celsius on Asia's glaciers, Nature, 549, 257–260, https://doi.org/10.1038/nature23878, 2017.
Lehner, B. and Grill, G.: Global river hydrography and network routing: baseline data and new approaches to study the world's large river systems, Hydrol. Process., 27, 2171–2186, https://doi.org/10.1002/hyp.9740, 2013.
Li, Z., Wang, N., Chen, A. a., Liang, Q., and Yang, D.: Slight change of glaciers in the Pamir over the period 2000–2017, Arct. Antarct. Alp. Res., 54, 13–24, https://doi.org/10.1080/15230430.2022.2028475, 2022.
Liao, H., Liu, Q., Zhong, Y., and Lu, X.: Landsat-Based Estimation of the Glacier Surface Temperature of Hailuogou Glacier, Southeastern Tibetan Plateau, Between 1990 and 2018, Remote Sens., 12, 2105, https://doi.org/10.3390/rs12132105, 2020.
Lippl, S., Vijay, S., and Braun, M.: Automatic delineation of debris-covered glaciers using InSAR coherence derived from X-, C- and L-band radar data: a case study of Yazgyl Glacier, J. Glaciol., 64, 811–821, https://doi.org/10.1017/jog.2018.70, 2018.
Liu, S., Ding, Y., Shangguan, D., Zhang, Y., Li, J., Han, H., Wang, J., and Xie, C.: Glacier retreat as a result of climate warming and increased precipitation in the Tarim river basin, northwest China, Ann. Glaciol., 43, 91–96, https://doi.org/10.3189/172756406781812168, 2006.
Liu, S., Yao, X., Shangguan, D., Guo, W., Wei, J., Xu, J., Bao, W., and Wu, L.: The contemporary glaciers in China based on the Second Chinese Glacier Inventory, Acta Geogr. Sin., 70, 3–16, https://doi.org/10.11821/dlxb201501001, 2015.
Liu, S., Wu, T., Wang, X., Wu, X., Yao, X., Liu, Q., Zhang, Y., Wei, J., and Zhu, X.: Changes in the global cryosphere and their impacts: A review and new perspective, Sci. Cold Arid Reg., 12, 343–354, https://doi.org/10.3724/SP.J.1226.2020.00343, 2020.
Lucchesi, S., Fioraso, G., Bertotto, S., and Chiarle, M.: Little Ice Age and contemporary glacier extent in the Western and South-Western Piedmont Alps (North-Western Italy), J. Maps, 10, 409–423, https://doi.org/10.1080/17445647.2014.880226, 2014.
Meier, W. J. H., Grießinger, J., Hochreuther, P., and Braun, M. H.: An Updated Multi-Temporal Glacier Inventory for the Patagonian Andes With Changes Between the Little Ice Age and 2016, Front. Earth Sci., 6, 62, https://doi.org/10.3389/feart.2018.00062, 2018.
Millan, R., Mouginot, J., Rabatel, A., and Morlighem, M.: Ice velocity and thickness of the world's glaciers, Nat. Geosci., 15, 124–129, https://doi.org/10.1038/s41561-021-00885-z, 2022a.
Millan, R., Mouginot, J., Rabatel, A., and Morlighem, M.: Ice velocity and thickness of the world's glaciers, Nat. Geosci., 15, 124–129, https://doi.org/10.1038/s41561-021-00885-z, 2022b.
Minora, U., Bocchiola, D., D'Agata, C., Maragno, D., Mayer, C., Lambrecht, A., Vuillermoz, E., Senese, A., Compostella, C., Smiraglia, C., and Diolaiuti, G. A.: Glacier area stability in the Central Karakoram National Park (Pakistan) in 2001–2010: The “Karakoram Anomaly” in the spotlight, Prog. Phys. Geogr., 40, 629–660, https://doi.org/10.1177/0309133316643926, 2016.
Mölg, N., Bolch, T., Rastner, P., Strozzi, T., and Paul, F.: A consistent glacier inventory for Karakoram and Pamir derived from Landsat data: distribution of debris cover and mapping challenges, Earth Syst. Sci. Data, 10, 1807–1827, https://doi.org/10.5194/essd-10-1807-2018, 2018.
Mölg, N., Bolch, T., Walter, A., and Vieli, A.: Unravelling the evolution of Zmuttgletscher and its debris cover since the end of the Little Ice Age, The Cryosphere, 13, 1889–1909, https://doi.org/10.5194/tc-13-1889-2019, 2019.
Müller, F. and Scherler, K.: Introduction to the world glacier inventory, IAHS Publication, http://iahs.info/redbooks/a126/iahs_126_0000Intro.pdf (last access: 8 August 2022), 1980.
Nie, Y., Pritchard, H. D., Liu, Q., Hennig, T., Wang, W., Wang, X., Liu, S., Nepal, S., Samyn, D., Hewitt, K., and Chen, X.: Glacial change and hydrological implications in the Himalaya and Karakoram, Nat. Rev. Earth Environ., 2, 91–106, https://doi.org/10.1038/s43017-020-00124-w, 2021.
Nuimura, T., Sakai, A., Taniguchi, K., Nagai, H., Lamsal, D., Tsutaki, S., Kozawa, A., Hoshina, Y., Takenaka, S., Omiya, S., Tsunematsu, K., Tshering, P., and Fujita, K.: The GAMDAM glacier inventory: a quality-controlled inventory of Asian glaciers, The Cryosphere, 9, 849–864, https://doi.org/10.5194/tc-9-849-2015, 2015.
Paul, F. (Ed.): Glacier Inventory, in: International Encyclopedia of Geography: People, the Earth, Environment and Technology, Wiley, 1–12, https://doi.org/10.1002/9781118786352.wbieg0877, 2017.
Paul, F., Kääb, A., Maisch, M., Hoelzle, M., and Haeberli, W.: The new remote-sensing-derived Swiss glacier inventory: I. Methods, Ann. Glaciol., 34, 355–361, https://doi.org/10.3189/172756402781817941, 2002.
Paul, F., Huggel, C., and Kääb, A.: Combining satellite multispectral image data and a digital elevation model for mapping debris-covered glaciers, Remote Sens. Environ., 89, 510–518, https://doi.org/10.1016/j.rse.2003.11.007, 2004.
Paul, F., Barry, R. G., Cogley, J. G., Frey, H., Haeberli, W., Ohmura, A., Ommanney, C. S. L., Raup, B., Rivera, A., and Zemp, M.: Recommendations for the compilation of glacier inventory data from digital sources, Ann. Glaciol., 50, 119–126, https://doi.org/10.3189/172756410790595778, 2009.
Paul, F., Barrand, N. E., Baumann, S., Berthier, E., Bolch, T., Casey, K., Frey, H., Joshi, S. P., Konovalov, V., Le Bris, R., Mölg, N., Nosenko, G., Nuth, C., Pope, A., Racoviteanu, A., Rastner, P., Raup, B., Scharrer, K., Steffen, S., and Winsvold, S.: On the accuracy of glacier outlines derived from remote-sensing data, Ann. Glaciol., 54, 171–182, https://doi.org/10.3189/2013AoG63A296, 2013.
Paul, F., Bolch, T., Briggs, K., Kääb, A., McMillan, M., McNabb, R., Nagler, T., Nuth, C., Rastner, P., Strozzi, T., and Wuite, J.: Error sources and guidelines for quality assessment of glacier area, elevation change, and velocity products derived from satellite data in the Glaciers_cci project, Remote Sens. Environ., 203, 256–275, https://doi.org/10.1016/j.rse.2017.08.038, 2017.
Paul, F., Rastner, P., Azzoni, R. S., Diolaiuti, G., Fugazza, D., Le Bris, R., Nemec, J., Rabatel, A., Ramusovic, M., Schwaizer, G., and Smiraglia, C.: Glacier shrinkage in the Alps continues unabated as revealed by a new glacier inventory from Sentinel-2, Earth Syst. Sci. Data, 12, 1805–1821, https://doi.org/10.5194/essd-12-1805-2020, 2020.
Pfeffer, W. T., Arendt, A. A., Bliss, A., Bolch, T., Cogley, J. G., Gardner, A. S., Hagen, J.-O., Hock, R., Kaser, G., Kienholz, C., Miles, E. S., Moholdt, G., Mölg, N., Paul, F., Radiæ, V., Rastner, P., Raup, B. H., Rich, J., and Sharp, M. J.: The Randolph Glacier Inventory: a globally complete inventory of glaciers, J. Glaciol., 60, 537–552, https://doi.org/10.3189/2014JoG13J176, 2014.
Pieczonka, T. and Bolch, T.: Region-wide glacier mass budgets and area changes for the Central Tien Shan between ∼1975 and 1999 using Hexagon KH-9 imagery, Global Planet. Change, 128, 1–13, https://doi.org/10.1016/j.gloplacha.2014.11.014, 2015.
Racoviteanu, A. and Williams, M. W.: Decision Tree and Texture Analysis for Mapping Debris-Covered Glaciers in the Kangchenjunga Area, Eastern Himalaya, Remote Sens. 4, 3078–3109, https://doi.org/10.3390/rs4103078, 2012.
Rankl, M., Kienholz, C., and Braun, M.: Glacier changes in the Karakoram region mapped by multimission satellite imagery, The Cryosphere, 8, 977–989, https://doi.org/10.5194/tc-8-977-2014, 2014.
RGI Consortium: Randolph Glacier Inventory – A Dataset of Global Glacier Outlines: Version 6.0: Technical Report, Global Land Ice Measurements from Space, Digital Media, Colorado, USA, https://doi.org/10.7265/N5-RGI-60, 2017.
Robson, B. A., Nuth, C., Dahl, S. O., Hölbling, D., Strozzi, T., and Nielsen, P. R.: Automated classification of debris-covered glaciers combining optical, SAR and topographic data in an object-based environment, Remote Sens. Environ., 170, 372–387, https://doi.org/10.1016/j.rse.2015.10.001, 2015.
Rounce, D. R., Hock, R., and Shean, D. E.: Glacier Mass Change in High Mountain Asia Through 2100 Using the Open-Source Python Glacier Evolution Model (PyGEM), Front. Earth Sci., 7, 331, https://doi.org/10.3389/feart.2019.00331, 2020.
Sakai, A.: Brief communication: Updated GAMDAM glacier inventory over high-mountain Asia, The Cryosphere, 13, 2043–2049, https://doi.org/10.5194/tc-13-2043-2019, 2019.
Sakai, A., Nuimura, T., Fujita, K., Takenaka, S., Nagai, H., and Lamsal, D.: Climate regime of Asian glaciers revealed by GAMDAM glacier inventory, The Cryosphere, 9, 865–880, https://doi.org/10.5194/tc-9-865-2015, 2015.
Scherler, D., Wulf, H., and Gorelick, N.: Global Assessment of Supraglacial Debris-Cover Extents, Geophys. Res. Lett., 45, 11798–11805, https://doi.org/10.1029/2018gl080158, 2018.
Schmidt, S. and Nüsser, M.: Changes of High Altitude Glaciers from 1969 to 2010 in the Trans-Himalayan Kang Yatze Massif, Ladakh, Northwest India, Arct. Antarct. Alp. Res., 44, 107–121, https://doi.org/10.1657/1938-4246-44.1.107, 2012.
Shi, Y. and Liu, S.: Estimation on the response of glaciers in China to the global warming in the 21st century, Chinese Sci. Bull., 45, 668–672, https://doi.org/10.1007/BF02886048, 2000.
Shih, Y. F., Hsieh, T. C., Cheng, P. H., and Li, C. C.: Distribution, features and variations of glaciers in China, World Glacier Inventory – Inventaire mondial des Glaciers, IAHS Publication, http://hydrologie.org/redbooks/a126/iahs_126_0111.pdf (last access: 9 August 2022), 1980.
Smith, T., Bookhagen, B., and Cannon, F.: Improving semi-automated glacier mapping with a multi-method approach: applications in central Asia, The Cryosphere, 9, 1747–1759, https://doi.org/10.5194/tc-9-1747-2015, 2015.
Tielidze, L. G., Bolch, T., Wheate, R. D., Kutuzov, S. S., Lavrentiev, I. I., and Zemp, M.: Supra-glacial debris cover changes in the Greater Caucasus from 1986 to 2014, The Cryosphere, 14, 585–598, https://doi.org/10.5194/tc-14-585-2020, 2020.
Vijay, S. and Braun, M.: Early 21st century spatially detailed elevation changes of Jammu and Kashmir glaciers (Karakoram–Himalaya), Global Planet. Change, 165, 137–146, https://doi.org/10.1016/j.gloplacha.2018.03.014, 2018.
Weber, P., Andreassen, L. M., Boston, C. M., Lovell, H., and Kvarteig, S.: An ∼1899 glacier inventory for Nordland, northern Norway, produced from historical maps, J. Glaciol., 66, 259–277, https://doi.org/10.1017/jog.2020.3, 2020.
Winiger, M., Gumpert, M., and Yamout, H.: Karakorum-Hindukush-western Himalaya: assessing high-altitude water resources, Hydrol. Process., 19, 2329–2338, https://doi.org/10.1002/hyp.5887, 2005.
Wu, K., Liu, S., Jiang, Z., Zhu, Y., Xie, F., Gao, Y., Yi, Y., Tahir, A. A., and Muhammad, S.: Surging Dynamics of Glaciers in the Hunza Valley under an Equilibrium Mass State since 1990, Remote Sens., 12, 2922, https://doi.org/10.3390/rs12182922, 2020.
Wu, K., Liu, S., Jiang, Z., Liu, Q., Zhu, Y., Yi, Y., Xie, F., Ahmad Tahir, A., and Saifullah, M.: Quantification of glacier mass budgets in the Karakoram region of Upper Indus Basin during the early twenty-first century, J. Hydrol., 603, 127095, https://doi.org/10.1016/j.jhydrol.2021.127095, 2021.
Xie, F., Liu, S., Gao, Y., Zhu, Y., Wu, K., Qi, M., Duan, S., and Tahir, A. M.: Derivation of Supraglacial Debris Cover by Machine Learning Algorithms on the Gee Platform: A Case Study of Glaciers in the Hunza Valley, ISPRS Ann. Photogram. Remote Sens. Spat. Inform. Sci., V-3-2020, 417–424, https://doi.org/10.5194/isprs-annals-V-3-2020-417-2020, 2020a.
Xie, F., Liu, S., Wu, K., Zhu, Y., Gao, Y., Qi, M., Duan, S., Saifullah, M., and Tahir, A. A.: Upward Expansion of Supra-Glacial Debris Cover in the Hunza Valley, Karakoram, During 1990–2019, Front. Earth Sci., 8, 308, https://doi.org/10.3389/feart.2020.00308, 2020b.
Xie, F., Liu, S., Duan, S., Miao, W., Pan, X., and Qin, C.: Interdecadal glacier inventories in the Karakoram since the 1990s, National Cryosphere Desert Data Center [data set], https://doi.org/10.12072/ncdc.glacier.db2386.2022, 2022.
Xie, Z. and Liu, C.: Introduction to glaciology, Shanghai Universal Science Press, ISBN 9787542744494, 2009.
Xie, Z., Haritashya, U. K., Asari, V. K., Bishop, M. P., Kargel, J. S., and Aspiras, T. H.: GlacierNet2: A hybrid Multi-Model learning architecture for alpine glacier mapping, Int. J. Appl. Earth Obs. Geoinf., 112, 102921, https://doi.org/10.1016/j.jag.2022.102921, 2022.
Yang, Y., Li, Z., Huang, L., Tian, B., and Chen, Q.: Extraction of glacier outlines and water-eroded stripes using high-resolution SAR imagery, Int. J. Remote Sens., 37, 1016–1034, https://doi.org/10.1080/01431161.2016.1145365, 2016.
Yao, T., Thompson, L., Yang, W., Yu, W., Gao, Y., Guo, X., Yang, X., Duan, K., Zhao, H., Xu, B., Pu, J., Lu, A., Xiang, Y., Kattel, D. B., and Joswiak, D.: Different glacier status with atmospheric circulations in Tibetan Plateau and surroundings, Nat. Clim. Change, 2, 663–667, https://doi.org/10.1038/nclimate1580, 2012.
Yi, Y., Liu, S., Zhu, Y., Wu, K., Xie, F., and Saifullah, M.: Spatiotemporal heterogeneity of snow cover in the central and western Karakoram Mountains based on a refined MODIS product during 2002–2018, Atmos. Res., 250, 105402, https://doi.org/10.1016/j.atmosres.2020.105402, 2021.
Zhang, Y. and Liu, S. (Eds.): Modeling of the Mass Balance of Glaciers with Debris Cover, in: Geo-intelligence for Sustainable Development, Springer, Singapore, 191–212, https://doi.org/10.1007/978-981-16-4768-0_12, 2021.
Zhang, Y., Fujita, K., Shiyin, L., Liu, Q., and Nuimura, T.: Distribution of debris thickness and its effect on ice melt at Hailuogou glacier, southeastern Tibetan Plateau, using in situ surveys and ASTER imagery, J. Glaciol., 57, 1147–1157, https://doi.org/10.3189/002214311798843331, 2011.
Zhang, Y., Hirabayashi, Y., Fujita, K., Liu, S., and Liu, Q.: Heterogeneity in supraglacial debris thickness and its role in glacier mass changes of the Mount Gongga, Sci. China Earth Sci., 59, 170–184, https://doi.org/10.1007/s11430-015-5118-2, 2015.
Zhang, Z., Liu, S., Zhang, Y., Wei, J., Jiang, Z., and Wu, K.: Glacier variations at Aru Co in western Tibet from 1971 to 2016 derived from remote-sensing data, J. Glaciol., 64, 397–406, https://doi.org/10.1017/jog.2018.34, 2018.
Zhong, Y., Liu, Q., Westoby, M., Nie, Y., Pellicciotti, F., Zhang, B., Cai, J., Liu, G., Liao, H., and Lu, X.: Intensified paraglacial slope failures due to accelerating downwasting of a temperate glacier in Mt. Gongga, southeastern Tibetan Plateau, Earth Surf. Dynam., 10, 23–42, https://doi.org/10.5194/esurf-10-23-2022, 2022.