the Creative Commons Attribution 4.0 License.
the Creative Commons Attribution 4.0 License.
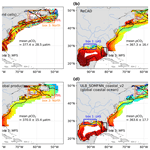
A machine-learning reconstruction of sea surface pCO2 in the North American Atlantic Coastal Ocean Margin from 1993 to 2021
Alizée Roobaert
Luping Song
Xiao-Hai Yan
Wei-Jun Cai
Insufficient spatiotemporal coverage of observations of the surface partial pressure of CO2 (pCO2) has hindered precise carbon cycle studies in coastal oceans and justifies the development of spatially and temporally continuous pCO2 data products. Earlier pCO2 products have difficulties in capturing the heterogeneity of regional variations and decadal trends of pCO2 in the North American Atlantic Coastal Ocean Margin (NAACOM). This study developed a regional reconstructed pCO2 product for the NAACOM (Reconstructed Coastal Acidification Database-pCO2, or ReCAD-NAACOM-pCO2) using a two-step approach combining random forest regression and linear regression. The product provides monthly pCO2 data at 0.25° spatial resolution from 1993 to 2021, enabling investigation of regional spatial differences, seasonal cycles, and decadal changes in pCO2. The observation-based reconstruction was trained using Surface Ocean CO2 Atlas (SOCAT) observations as observational values, with various satellite-derived and reanalysis environmental variables known to control sea surface pCO2 as model inputs. The product shows high accuracy during the model training, validation, and independent test phases, demonstrating robustness and a capability to accurately reconstruct pCO2 in regions or periods lacking direct observational data. Compared with all the observation samples from SOCAT, the pCO2 product yields a determination coefficient of 0.92, a root-mean-square error of 12.70 µatm, and an accumulative uncertainty of 23.25 µatm. The ReCAD-NAACOM-pCO2 product demonstrates its capability to resolve seasonal cycles, regional-scale variations, and decadal trends of pCO2 along the NAACOM. This new product provides reliable pCO2 data for more precise studies of coastal carbon dynamics in the NAACOM region. The dataset is publicly accessible at https://doi.org/10.5281/zenodo.14038561 (Wu et al., 2024a) and will be updated regularly.
- Article
(7617 KB) - Full-text XML
- BibTeX
- EndNote
Accurate and comprehensive datasets of the sea surface partial pressure of CO2 (pCO2) are necessary for quantifying coastal CO2 uptake and assessing the impact of climate change on coastal ocean ecosystems. On a global scale, the coastal ocean, covering 8.4 % (30.4 × 106 km2) of the global ocean surface area (Chen et al., 2013; Dai et al., 2022), plays a significant role in the global carbon budget, accounting for approximately 10.9 % of the global ocean CO2 uptake from the atmosphere (0.25 of 2.3 Pg C yr−1) on the global average (Dai et al., 2022; Friedlingstein et al., 2023). However, on regional scales, areal-based CO2 uptake in specific coastal regions are often much greater than those in open oceans despite their less distinguishable global means (Dai et al., 2022). This is because sea surface pCO2 is highly variable due to the influence of various physical and biogeochemical processes in coastal oceans, such as riverine input, upwelling, tidal mixing, and large-scale circulations (Laruelle et al., 2018; Roobaert et al., 2024b). Thus, accurately quantifying the CO2 uptake in specific coastal regions becomes particularly challenging when only using observations due to the incomplete coverage of pCO2 data in space and time.
This study focuses on the North American Atlantic Coastal Ocean Margin (NAACOM; Fig. 1). The entire region is defined as the area within 400 km of the coastline and is divided into six subregions following Fennel et al. (2019) based on their geographic locations: the Gulf of Mexico (GoMx), South Atlantic Bight (SAB), Mid-Atlantic Bight (MAB), Gulf of Maine (GoMe), Scotian Shelf (SS), and Gulf of St. Lawrence and Grand Banks (GStL&GB). The carbonate system in the NAACOM is influenced by large-scale circulations (Fig. 1), including the Gulf Stream and Labrador Current, as well as local processes like river discharge, export from marshes, and upwelling dynamics (Cai et al., 2020; Fennel et al., 2019; Wang et al., 2013). These complex physical and biogeochemical processes contribute to substantial spatial and temporal heterogeneity in sea surface pCO2 across the NAACOM (Cai et al., 2020). Elucidating the driving mechanisms of these spatiotemporal pCO2 variations necessitates extensive data coverage in time and space in this region. Over the past 2 decades, coastal field investigation efforts in this region have substantially increased through programs like the East Coast Ocean Acidification (ECOA) and Gulf of Mexico Ecosystems and Carbon Cruise (GOMECC) (Cai et al., 2020; Wang et al., 2013; Wanninkhof et al., 2015). Ongoing measurements from these cruises, combined with ongoing measurements from volunteer observing ships and buoys, are quality-controlled and compiled in the Surface Ocean CO2 Atlas (SOCAT) database (Bakker et al., 2016), substantially advancing our understanding of coastal inorganic carbon chemistry along the NAACOM (Cai et al., 2020).
Despite significant progress in observational efforts, the spatial and temporal coverage of pCO2 data remains limited in the NAACOM, with observations encompassing only 2.9 % of grid cells during the period 1993–2021 (Fig. 2). Observations are concentrated in the southern region, with fewer samples available during winter. This data scarcity introduces substantial uncertainty into air–sea CO2 exchange quantification and hinders comprehensive understanding of coastal inorganic carbon dynamics, particularly in areas north of Cape Cod where measurements are very sparse (Fig. 2). For example, reported air–sea CO2 fluxes for the GoMe exhibit a wide range spanning from −0.50 to +2.50 mol C m−2 yr−1, with conflicting reports characterizing it as a CO2 source (Fennel and Wilkin, 2009; Vandemark et al., 2011), CO2-neutral (Signorini et al., 2013), or a CO2 sink (Cahill et al., 2016; Rutherford et al., 2021), underscoring the need for improved pCO2 data coverage.
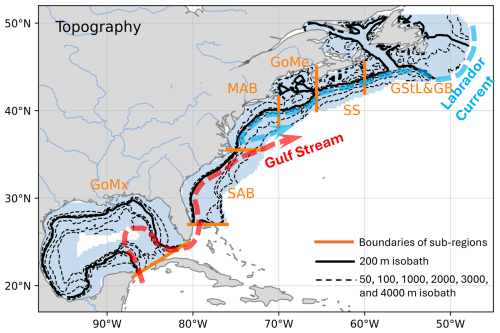
Figure 1Topography (m) and large-scale circulation along the North American Atlantic Coastal Ocean Margin (NAACOM). The study region, defined as coastal areas extending 400 km offshore, is indicated by blue shading. The thick black line is the 200 m isobath, which roughly marks the shelf break and typically defines the continental shelf boundary. The Gulf Stream (thick red dashed line with an arrow) flows northward along the eastern coast of the United States before veering eastward into the open Atlantic Ocean around Cape Hatteras. The Labrador Current (thick light-blue dashed line with an arrow) flows southward along the eastern coast of Canada before meeting the Gulf Stream. Following Fennel et al. (2019), the study region is divided into six subregions by straight orange lines: the Gulf of Mexico (GoMx), South Atlantic Bight (SAB), Mid-Atlantic Bight (MAB), Gulf of Maine (GoMe), Scotian Shelf (SS), and Gulf of St. Lawrence and Grand Banks (GStL&GB). Dashed contour lines indicate bathymetric depths of 50 and 100 m on the shelf (from the coastline to the 200 m isobath) and 1000, 2000, 3000, and 4000 m from the shelf break to the open ocean.
Recently, various pCO2 products, global or regional, with full coverage in time and space were developed as essential supplements to observations. These products usually employed diverse algorithms, environmental proxies from satellites and reanalysis products as model inputs, and SOCAT observations as constraints to reconstruct the pCO2 field with full temporal and spatial coverage. The development of those products has significantly advanced our understanding of inorganic carbon chemistry and the ocean carbon cycle. For example, seven global pCO2 products were used to evaluate the ocean CO2 uptake in the Global Carbon Budget 2023 edition (Friedlingstein et al., 2023). However, most of these products reconstruct pCO2 in the open ocean, with coastal regions often being extrapolated or excluded. Currently, only one pCO2 product has been developed specifically for the coastal ocean on a global scale (Laruelle et al., 2017; Roobaert et al., 2024a). This product was recently combined with an open-ocean product to create a global reconstruction of the ocean CO2 sink (Landschützer et al., 2020) and has since been utilized to narrow the variability in global reconstructions (Fay et al., 2021). However, global products primarily aim to ensure high accuracy of parameters on a global average; they may not guarantee equivalent accuracy for spatiotemporal variations on the regional scale. In comparison, regional pCO2 products have demonstrated superior capability in resolving detailed small-scale variations.
Within the NAACOM region, several area-specific pCO2 products have been reconstructed, focusing on specific regions such as the GoMx (e.g., Chen and Hu, 2019; Fu et al., 2020; Lohrenz and Cai, 2006) and the SAB and MAB (e.g., Wang et al., 2024; Xu et al., 2020). These regional and global pCO2 products are valuable for validating model estimations (Roobaert et al., 2022; Ross et al., 2023). However, existing products often have limitations in spatial coverage, temporal resolution, or trend analysis capabilities. For instance, Chen and Hu (2019) provided a high-resolution (4 km) pCO2 product for the GoMx, but this product faces challenges in capturing decadal changes in pCO2 (Wu et al., 2024b). Conversely, Xu et al. (2020) successfully captured decadal trends of pCO2 but only as area-averaged pCO2 time series for the SAB and MAB, lacking comprehensive spatial coverage. Signorini et al. (2013) reconstructed a product using multiple linear regression (MLR) covering the areas from the SAB to SS, but this only spans 8 years (2003–2010). Despite these valuable efforts, there remains a lack of comprehensive data products that adequately capture regional variations, seasonal cycles, and decadal changes in pCO2 simultaneously for the entire NAACOM.
This study aims to develop a regional pCO2 product specifically designed for the NAACOM, encompassing coastal regions extending 400 km offshore from the GoMx to the GB (Fig. 1). We integrated random forest and linear regression methods with hydrological parameters from satellite observations and reanalysis data to generate a monthly reconstructed pCO2 product at 0.25° spatial resolution spanning the period from 1993 to 2021. The pCO2 product, termed the Reconstructed Coastal Acidification Database or ReCAD-NAACOM-pCO2, is specifically designed to resolve the spatial variations, seasonal cycles, and decadal changes of pCO2 along the NAACOM.
The structure of this paper is as follows: Sect. 2 details the methodology used to reconstruct ReCAD-NAACOM-pCO2 and describes the datasets employed. Section 3 evaluates the accuracy of the reconstructed product, performance, and applicability in resolving seasonal cycles, regional variations, and decadal trends of pCO2. Sections 4 and 5 provide links to access the dataset and codes used to generate the dataset and figures presented in this study. The final section summarizes the conclusions. ReCAD-NAACOM-pCO2 demonstrates enhanced capability in resolving spatial variations and capturing the seasonal cycle and decadal trends of pCO2 compared to the global products across different subregions in the NAACOM. This product offers improved insights into coastal carbon dynamics in this complex region, addressing the need for a comprehensive pCO2 dataset in the NAACOM. Applications of this data product to examine the processes controlling the spatial variability, seasonal cycle, and decadal trends of pCO2 and air–sea CO2 flux will be published separately.
2.1 Observational data from SOCAT
The observational data for training the regression model were measurements of the seawater fugacity of CO2 (fCO2) extracted from the SOCAT database (2023 edition). fCO2 represents the pCO2 corrected for the nonideal behavior of the gas in seawater, and both are commonly used in oceanographic studies. SOCAT compiles quality-controlled fCO2 measurements from various platforms, including research vessels, commercial ships, and moorings (Bakker et al., 2016). This study used the monthly gridded SOCAT coastal product with a spatial resolution of 0.25° × 0.25° (but with data gaps). The gridded product incorporated measurements with quality flags A and B (uncertainty of 2 µatm) and C and D (uncertainty of 5 µatm) (Bakker et al., 2016). Over the period of 1993–2021, the SOCAT product encompassed 55 347 grid cells within our study area (Fig. 2), accounting for approximately 2.9 % of the total number of grid cells in the NAACOM. The observational data show a lower sampling density in the areas north of Cape Cod and the western and southern GoMx (blue box in Fig. 2). The temporal distribution of the samples exhibits a notable bias, with reduced collection during winter (Fig. 2d). Despite these spatial and temporal heterogeneities, the SOCAT observations provide coverage across all subregions and seasons of the NAACOM (Fig. 2). This comprehensive, albeit sparse, coverage facilitates the reconstruction of the fCO2 and pCO2 field through interpolation and regression techniques.
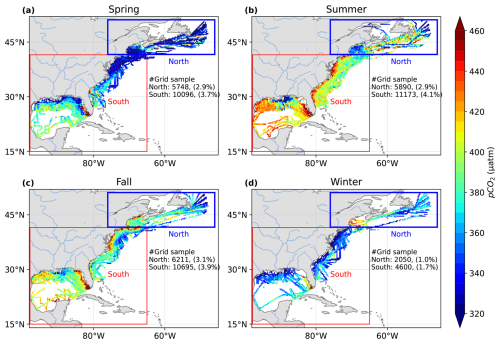
Figure 2Spatial distribution of sea surface pCO2 observations from the SOCAT database (2023 edition) in the NAACOM across the four seasons from 1993 to 2021. Grid samples with data were counted by season: (a) spring (March to May), (b) summer (June to August), (c) fall (September to November), and (d) winter (December to February). The study region is divided into northern (blue box) and southern (red box) areas at approximately 41.5° N (Cape Cod). The number and percentage of the grid samples are indicated for each region by season. The color scale represents pCO2 values (µatm). A higher sampling density is evident in the southern area. Winter shows the lowest overall sampling coverage. Note that the SOCAT database provides quality-controlled fCO2 measurements as the default parameters, which are subsequently converted into pCO2 using Eq. (2).
2.2 Model design
The procedures for developing and reconstructing the pCO2 product are illustrated in Fig. 3. Initially, the input variables and sea surface fCO2 data were matched to create a comprehensive dataset. To maintain consistency with the SOCAT database, which reports seawater CO2 concentrations as fCO2, we adopted fCO2 as the model training label and first-step output variable in our model. During the model development phase, fCO2 measurements served as training labels for the machine-learning algorithm. The matched dataset was then divided into two sets: X1, encompassing the periods 1993–2003 and 2006–2021, and X2, covering 2004–2005. Set X1 was randomly subdivided further, with 80 % allocated for model training and the remaining 20 % for the validation test. Set X2 served as an independent test set. The model training set (80 % of X1) was used to develop a two-step RFR–LR (random forest regression–linear regression) model. The RFR model is designed to capture complex, nonlinear relationships between the input variables and the target variable (i.e., fCO2), while the LR model is subsequently applied to mitigate potential systematic biases in RFR-derived fCO2 values arising from spatiotemporal heterogeneities in the SOCAT observational dataset (Fig. 2). RFR, an ensemble learning technique, combines multiple decision trees to produce more accurate and stable predictions (Breiman, 2001; Lu et al., 2019). Each decision tree in the RFR model is trained on a randomly selected subset of the input data, with the final prediction derived from the average output of all the trees. This approach mitigates overfitting and enhances the generalization performance of the model, making it particularly suitable for large datasets with complex, nonlinear variable relationships. The RFR model was trained using 10-fold cross-validation with optimized hyperparameters, including a minimum leaf size of 1, a bagging method for ensemble aggregation, and 300 learning cycles after tuning. After RFR model training, the LR model was applied to the RFR-estimated fCO2 (fCO2est) output to make sure that the RFR model was not systematically biased:
where fCO2obs is the observed fCO2 from SOCAT, a is the linear regression coefficient, b is the intercept, and ε is the residual that the linear model cannot resolve. This additional step was implemented to mitigate potential systematic bias in the RFR model that could arise from areas with a higher sampling density, thereby ensuring a more balanced representation across the entire study region. The comparison between the estimated pCO2 before and after LR calibration is presented in Appendix A. The calibration was applied to each grid cell individually. To increase the data pool for linear regression, samples within a 5 × 5 grid window in space (i.e., 1.25° × 1.25°) were aggregated for LR model development. As the available measurements could not cover every grid cell and were insufficient to produce continuous spatial maps of the calibration coefficients (i.e., a and b in Eq. 1), we employed a locally interpolated regression strategy similar to that of Carter et al. (2018). Mathematically, given the spatial and temporal continuity of fCO2est and fCO2obs, the coefficients a and b must also be continuous in space and time. Therefore, we linearly interpolated the coefficients a and b across the NAACOM. The interpolated coefficients were subsequently used to adjust the RFR-derived fCO2est.
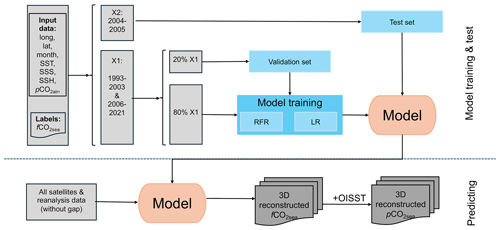
Figure 3A flowchart of the two-step machine-learning regression model for generating the reconstructed pCO2 product. The grey boxes represent the input and output datasets. The blue boxes illustrate the model training, validation testing, and independent test processes. The orange boxes represent the final trained model for predicting the reconstructed product. The two models in the orange boxes are identical. The training data, consisting of paired input variables (longitude, latitude, month, sea surface temperature (SST), sea surface salinity (SSS), sea surface height (SSH), atmospheric pCO2 (pCO2air), and the corresponding sea surface fCO2 (fCO2sea) labels), are divided into two sets: X1 (1993–2003 and 2006–2021) and X2 (2004–2005). X1 is randomly divided further into subsets for the model training set (80 %) and the validation set (20 %). The predictive model combines a random forest regression (RFR) algorithm and a linear regression (LR) algorithm. The trained and validated regression model is then applied to all satellite and reanalysis data (without gaps) to generate the 3D reconstructed fCO2sea product, which is then converted into pCO2sea with satellite SST data.
The validation set, comprising 20 % of X1 randomly sampled from 1993–2003 and 2006–2021, serves as a critical monitoring step for model evaluation. This subset plays two key roles: first, it tests hyperparameter tuning by providing independent performance metrics on unseen data, and second, it helps detect potential overfitting by monitoring the divergence between training and validation performance. While the validation set itself cannot prevent overfitting, it enables the detection of overfitting patterns when the performance of the model improves on training data but deteriorates on validation data. Through this continuous evaluation process, the validation set ensures more robust model development and helps achieve better generalization capabilities.
The independent test set (X2), covering the years 2004–2005, serves as a critical evaluation period specifically designed to assess the reliability of the model in predicting values for years that were completely excluded from both the training and validation phases. Because we intentionally withhold these 2 years from model development, this approach directly tests the capability of the model to generate reliable predictions and fill temporal data gaps for periods without observational data.
Finally, the trained model is applied to all the satellite and reanalysis data to generate the final gap-free reconstructed fCO2 data. As most products reported seawater CO2 concentrations as pCO2, we subsequently converted the reconstructed fCO2 values into pCO2 using the following equation (Takahashi et al., 2020), and our final product reports both fCO2 and pCO2:
2.3 Regression model input variables from satellite and reanalysis
The input variables for training the regression model are longitude (long), latitude (lat), month, sea surface temperature (SST), sea surface salinity (SSS), sea surface height (SSH), and atmospheric pCO2 (pCO2air). Longitude, latitude, and month serve as spatiotemporal predictors, enabling the algorithm to identify and capture regional and seasonal variability in fCO2 within the study area (Su et al., 2020; Yang et al., 2024). SST, SSS, and SSH are critical variables that characterize the physical and biogeochemical ocean settings, which play a crucial role in determining the spatial and temporal variability of fCO2. pCO2air represents the atmospheric forcing in the air–sea CO2 exchange. Including pCO2air is essential for accurately assessing the decadal pCO2 trend.
SST data were obtained from the National Oceanic and Atmospheric Administration (NOAA) Optimum Interpolation Sea Surface Temperature (OISST) v2.1 product (Huang et al., 2021). The OISST dataset is a global gridded SST analysis that blends observations from various sources, including satellites, ships, and buoys. The dataset employs an optimum interpolation technique to combine these observations and generate a daily SST field at a spatial resolution of 0.25° × 0.25°. For this study, the daily SST data were averaged to create a monthly product.
SSS data were obtained from the Simple Ocean Data Assimilation (SODA) v3.15.2 product (Carton et al., 2018). SODA is a comprehensive reanalysis dataset that integrates a global ocean model with observational data to estimate ocean state variables consistently. The SODA system assimilates observations from multiple sources, including floats, moorings, and ship-based measurements, thereby constraining the model output and enhancing the accuracy of the represented ocean physical properties, including SSS. The SODA v3.15.2 product offers monthly SSS data with a temporal resolution of 1 month and a spatial resolution of 0.5° × 0.5°, which were linearly interpolated to a 0.25° × 0.25° grid resolution to maintain consistency with other input variables and the gridded SOCAT fCO2 data. Note that such interpolation could potentially introduce additional errors. We doubled the SSS uncertainty in the region, assuming that this would encompass its true uncertainty (see Appendix B).
SSH data were extracted from the Global Ocean Gridded L4 Sea Surface Heights (CMEMS, 2021) created by the Copernicus Marine Environment Monitoring Service (CMEMS). Since 1993 (ongoing) this product has provided daily SSH data derived from altimeters with a spatial resolution of 0.25° × 0.25°. Daily SSH data were averaged to monthly means.
pCO2air data converted from the mole fraction of CO2 in dry air (xCO2air) were downloaded from the NOAA Marine Boundary Layer (MBL) reference product (Lan et al., 2023). The MBL reference product provides weekly zonal average xCO2air measurements from a global observation network. The xCO2air data were linearly interpolated to the same spatial and temporal resolution as the other input variables (0.25° × 0.25°, monthly). xCO2air was converted into pCO2air with the equation
where P is the total atmospheric pressure on the sea surface, which was downloaded from the fifth-generation reanalysis (ERA5) of the European Centre for Medium-Range Weather Forecasts (ECMWF) (Hersbach et al., 2019), and pw is the water vapor pressure, which was calculated using the formula of Weiss and Price (1980), SST from OISST, and SSS from SODA.
2.4 Evaluation of the models
The accuracy of the model outputs was assessed using several statistical metrics, including the coefficient of determination (R2), root-mean-square error (RMSE), mean absolute error (MAE), and mean bias error (MBE). These metrics were calculated for the training and validation set phases as well as for the independent validation set:
where i denotes the ith sample, yobs and are the observed pCO2 values from SOCAT and their average, yest represents the predicted pCO2 values from the final model, and N is the total number of matched samples.
2.5 Uncertainty of the reconstructed pCO2
The uncertainty of the estimated pCO2 in our product for each grid cell was accumulated from four sources of uncertainties: the direct pCO2 measurement uncertainty from SOCAT (uobs), gridding uncertainty (ugrid), mapping uncertainty (umap), and the uncertainty accumulated from the input variables (uinputs). The first three sources of uncertainty were calculated according to the approach used by earlier reconstructed pCO2 products (Landschützer et al., 2014; Roobaert et al., 2024a; Sharp et al., 2022). uobs is inherited from the SOCAT observations. The SOCAT database uses discrete samples with quality flags A and B (accuracy <2 µatm) and C and D (accuracy <5 µatm) to create the gridded file. Adopting a conservative approach, we used the maximum uobs of 5 µatm. ugrid was calculated as the standard deviation of the samples used to calculate the gridded fCO2 in each grid cell. umap is introduced by reconstructing the pCO2 using the RFR–LR model. It was evaluated as the RMSE between the reconstructed pCO2 and the observed pCO2 values following Roobaert et al. (2024a) and Sharp et al. (2022). Given that the derivation of uobs, ugrid, and umap is contingent upon SOCAT observations, these three uncertainties and the total uncertainty are reported on a subregional basis.
In addition to these three sources of uncertainty, this study incorporated cumulative uncertainties from input variables (uinputs), including SST, SSS, SSH, and pCO2air. These satellite-derived or reanalysis-based variables inherently possess uncertainties that propagate nonlinearly through the regression model, ultimately affecting the estimated pCO2 values (Wang et al., 2021, 2023). We employed a Monte Carlo simulation to calculate uinputs. For each input variable (SST, SSS, SSH, and pCO2air), we added white noise following a normal distribution , where is the uncertainty of the respective input variable xi. We then recalculated pCO2 using these noise-added inputs and determined the resulting changes in pCO2. This process was repeated 100 times for each input variable, and the resulting uncertainty in pCO2 from each variable was calculated as the standard deviation of the differences between the original reconstructed pCO2 and the pCO2 values after adding noise to each grid cell. The final uinputs was computed as the square root of the quadratic sum of these individual uncertainties from the four input variables. Detailed procedures for determining uinputs are described in Appendix B.
Assuming that these sources are independent, the uncertainty of the estimated gridded pCO2 in our product, , was calculated using the error propagation (Hughes and Hase, 2010; Taylor, 1997):
2.6 Comparison with the global reconstructed pCO2 product
The ReCAD-NAACOM-pCO2 product was evaluated through comparisons with seven reconstructed pCO2 products developed for the global ocean and used in the Global Carbon Budget 2023 edition (Friedlingstein et al., 2023) and one reconstructed pCO2 product specifically developed for the global coastal ocean (ULB_SOMFNN_coastal_v2; Roobaert et al., 2024a). These data products reconstructed pCO2sea data using different machine-learning algorithms. Detailed information on the products is summarized in Table 1.
Table 1References for the global pCO2 products used for comparison with ReCAD-NAACOM-pCO2 in this study. The abbreviations in the Methods column are RFRE for random-forest-based regression ensemble, SOM-FFN for self-organizing map–feed-forward network, MLR for multiple linear regression, FFNN for feed-forward neural network, XGB for the eXtreme Gradient Boosting algorithm, and GRaCER for geospatial random-cluster ensemble regression.
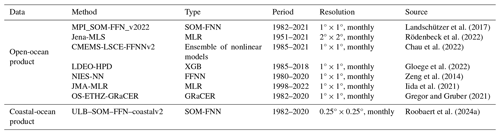
3.1 Evaluating the regression model performance
Our product employs a two-step RFR–LR algorithm to retrieve pCO2. The initial RFR step accurately captures most seasonal and decadal pCO2 variations across all six subregions (Appendix A). When comparing only at matching grid cells where SOCAT measurements are available, the differences (N=12) in the monthly mean climatology between the SOCAT- and RFR-derived pCO2 are less than 2 µatm on average, with standard deviations below 5 µatm across all the subregions (Fig. A1). However, the RFR-derived pCO2 shows lower accuracy in capturing long-term pCO2 changes in the GoMe and SAB. The subsequent LR calibration improves the performance significantly: R2 values increase from 0.69 to 0.81 in the GoMe and from 0.83 to 0.93 in the SAB, while the RMSE decreases from 12.43 to 10.51 µatm in the GoMe and from 10.83 to 8.12 µatm in the SAB (Fig. A2).
The ReCAD-NAACOM-pCO2 product demonstrated robust performance and high accuracy in capturing pCO2 variability across the NAACOM (Fig. 4). During the model training phase, the product achieved an R2 of 0.96, an RMSE of 9.1 µatm, an MAE of 5.92 µatm, and an MBE of 0.05 µatm (Fig. 4a). The model demonstrated comparable performance metrics during the validation phase (Fig. 4b). To further evaluate the generalizability and robustness of the model, we also conducted an independent test using data from 2004 to 2005 in which not all the data samples were included in the model training and validation sets. During this independent test phase, the pCO2 product maintained high accuracy, with R2=0.64, RMSE = 27.2 µatm, MAE = 18.86 µatm, and MBE = 0.07 µatm (Fig. 4c). Additionally, most independent validation samples were distributed around the 1:1 correspondence line, proving the ability of the models to predict pCO2 across unsampled spatial and temporal domains without overfitting. The model consistently demonstrated strong performance during the training, validation, and independent test phases across all the subregions (Table 2). Overall, compared with all the available samples in SOCAT, it achieved an R2 of 0.92, an RMSE of 12.70 µatm, an MAE of 7.55 µatm, and an MBE of 0.13 µatm for the entire NAACOM (Table 2), highlighting the generalizability of the ReCAD-NAACOM-pCO2 product and robustness in effectively capturing the variability in pCO2 and providing reliable predictions of pCO2 across the studied regions.
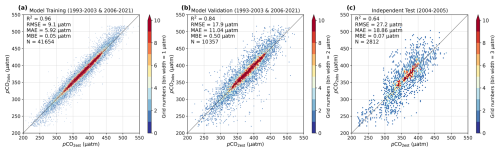
Figure 4Evaluation of the regression model for reconstructing the ReCAD-NAACOM-pCO2 product. The density scatterplots compare the product-estimated pCO2 (pCO2est) with the in situ SOCAT observations (pCO2obs) during the (a) model training phase (80 % of the samples during the periods 1993–2003 and 2006–2021), (b) validation phase (20 % of the samples during the periods 1993–2003 and 2006–2021), and (c) independent test phase (samples during the period 2004–2005). The statistical metrics include the coefficient of determination (R2), root-mean-square error (RMSE), mean absolute error (MAE), mean bias error (MBE), and number of samples (N). The color bar represents the number of data points in each bin.
Table 2Performance of the regression model during the model training, validation, and independent test phases across the different subregions. The metrics include the coefficient of determination (R2), root-mean-square error (RMSE), mean absolute error (MAE), and mean bias error (MBE). The subregions are the Gulf of Mexico (GoMx), South Atlantic Bight (SAB), Mid-Atlantic Bight (MAB), Gulf of Maine (GoMe), Scotian Shelf (SS), and Gulf of St. Lawrence and Grand Banks (GStL&GB).
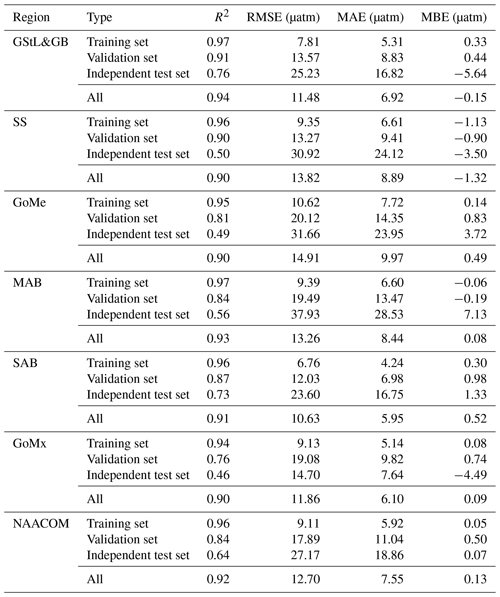
3.2 Spatial distribution of the product bias
The ReCAD-NAACOM-pCO2 product exhibited a negligible area-mean bias of +0.13 µatm with a standard deviation of 12.70 µatm when compared to all SOCAT observation grid cells across the entire NAACOM (Fig. 5 and Table 2). This small average difference suggests no consistent overestimation or underestimation by the regression model, indicating the reliability of the product in estimating the monthly and annual mean climatology of pCO2 across the entire NAACOM region.
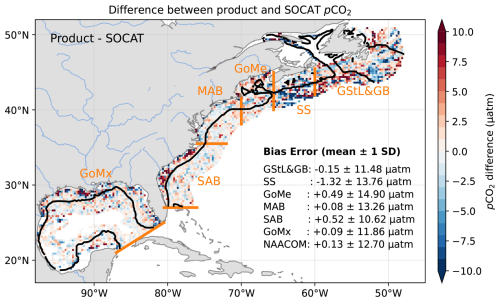
Figure 5Spatial distribution of the MBE between the ReCAD-NAACOM-pCO2 product and SOCAT observations across the NAACOM. The MBE is calculated for each grid cell as the average difference between product estimates and SOCAT observations. Positive values (red) indicate product overestimation, while negative values (blue) indicate underestimation relative to SOCAT. Regional MBE values with 1 standard deviation are shown for each subregion, corresponding to the values in the last column of Table 2. The overall bias error for the NAACOM is +0.13 ± 12.97 µatm. Following Fennel et al. (2019), the study region is divided into six subregions using straight orange lines: the GoMx, SAB, MAB, GoMe, SS, and GStL&GB. The thick black line is the 200 m isobath, which roughly marks the shelf break and typically defines the continental shelf boundary.
While the area-averaged difference is small, the differences are distributed heterogeneously in space. Larger differences (absolute difference >10 µatm) tend to occur in nearshore regions, particularly along the coastlines of the GoMx and SAB, as well as in northern areas such as the GoMe, SS, and GStL&GB (Fig. 5). These regional variations can be attributed to complex coastal processes such as terrestrial inputs, sparse observations in the northern areas (Lavoie et al., 2021; Rutherford et al., 2021; Salisbury and Jönsson, 2018), and less accurate satellite observations in the nearshore regions (Song et al., 2023). Conversely, smaller differences (absolute difference <2.5 µatm) are observed in the central parts of the GoMx, offshore regions of the SAB and MAB, and some nearshore regions of the SS and GB, which is likely due to more stable oceanic conditions in those regions. The regional MBEs for different machine-learning development phases (training, validation, and test sets) are detailed in Table 2. Despite these regional differences, the MBEs of both the validation set (−1.0 to 1.0 µatm) and the independent test set (−4.5 to 7.5 µatm) demonstrate minimal values across the subregions (Table 2), underscoring the effectiveness of the product in capturing the broader pCO2 patterns across the NAACOM.
3.3 Evaluating the capacity of the product to capture pCO2 seasonality
One of the primary objectives of this product is to capture the seasonal cycle of pCO2 across the NAACOM region. Figure 6 showcases the applicability of the product in capturing the pCO2 seasonal cycles across the southern and northern areas of the NAACOM (red and blue boxes in Fig. 2). The comparison of monthly climatologies between the gap-filled product and SOCAT observations reveals strong agreement in the southern region despite the coverage difference, with the product-estimated monthly means being only 3.05 ± 5.60 µatm higher than those of SOCAT (Fig. 6a) and suggesting that our product effectively captures the seasonal cycle where data are abundant.
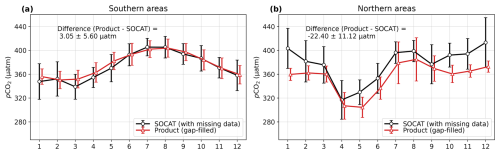
Figure 6Monthly mean climatology of pCO2 in the southern and northern areas of the NAACOM from 1993 to 2021. The subregions are (a) the southern areas with the red box in Fig. 2 and (b) the northern areas with the blue box in Fig. 2. Two data representations are shown: (1) SOCAT observations (black curves), which may be influenced by missing data, and (2) the complete gap-filled product output (red curves). The error bars denote 1 standard deviation of the monthly mean climatology of pCO2. The numbers indicate the mean difference (± 1 standard deviation) in the monthly climatological pCO2 calculated from the two sources, with positive values indicating higher product estimates compared to SOCAT observations. The x axis shows the months (1–12, where 1 represents January), and the y axis shows pCO2 (µatm).
In the northern region where SOCAT data are sparse, the gap-filling ability of the product is also demonstrated well. In the northern region, the area-averaged monthly pCO2 climatology calculated from the continuous reconstructed product is 22 ± 11.12 µatm lower than the SOCAT observations, which can be attributed to the limited observational coverage in this area. This area is characterized by sparse sampling, with the observational density approximately 50 % lower than in the southern region (Fig. 2) due to the smaller area and limited cruise coverage. For instance, the GStL region only has one summer cruise in the SOCAT database (Fig. 2b), and the SS and GoMe have particularly sparse winter observations (Fig. 2d). The higher latitudes typically exhibit larger seasonal amplitudes in pCO2, making the limited sampling from SOCAT particularly problematic for accurate characterization. Our gap-free product provides comprehensive spatial and temporal coverage, enabling more robust analysis of pCO2 patterns and variability in these historically undersampled regions.
Over the 29-year period, the product predicts smaller monthly standard deviations in the southern region (less than 40 µatm; error bars in Fig. 6a), suggesting higher model accuracy and less interannual variability in these areas. Conversely, larger monthly standard deviations are observed in the northern areas, suggesting potentially lower accuracy and remarkable interannual variability. However, the larger interannual variability in these areas may be an artifact of the limited observational data available for regression model training, resulting in greater uncertainty in the predictions. Despite differences in the mean monthly climatology, the similar seasonal pCO2 cycles calculated from SOCAT and the reconstructed product demonstrate the ability of the ReCAD-NAACOM-pCO2 product to represent seasonal pCO2 variability across diverse coastal environments. Nevertheless, there exist larger differences between the observations and reconstructed pCO2 in some months and regions (Fig. 6b), highlighting the importance of the gap-free product in an unbiased understanding of regional carbon cycles (Ren et al., 2024). Detailed sea surface pCO2 seasonal cycles and their controlling mechanisms across different subregions of the NAACOM will be presented in our subsequent work.
3.4 Evaluating the ability of the products to capture regional variation by comparing them to global products
The ReCAD-NAACOM-pCO2 product demonstrates the capability to resolve fine-scale regional spatial distributions of pCO2. Figure 7 illustrates the spatial distribution of the annual mean climatology of pCO2 across the NAACOM as observed by SOCAT and predicted by different global open- and coastal-ocean pCO2 products. Despite being affected by missing data, SOCAT observations (Fig. 7a) reveal significant regional variations in pCO2. In the Louisiana Shelf (LAS) estuary plume region (box 1 in Fig. 7), pCO2 values consistently remain below 340 µatm, while the West Florida Shelf (WFS; box 2 in Fig. 7) exhibits elevated values exceeding 400 µatm. These contrasting patterns have been reported in previous regional studies (Kealoha et al., 2020; Robbins et al., 2018; Wu et al., 2024b).
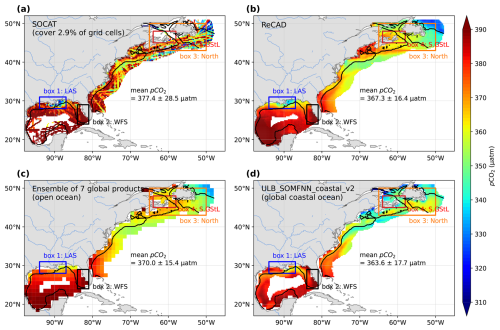
Figure 7Spatial distribution of the annual mean pCO2 climatology in the NAACOM from different sources. (a) SOCAT observations, (b) the ReCAD-NAACOM-pCO2 product, (c) the ensemble mean of the seven global open-ocean pCO2 products listed in Table 1, and (d) the coastal pCO2 product ULB_SOMFFN_coastal_v2 (Roobaert et al., 2024a). The black contour delineates the coastal-ocean margin. The three boxes represent subregions in the NAACOM: box 1 for the Louisiana Shelf (LAS), box 2 for the West Florida Shelf (WFS), box 3 for the entire northern region, and box 4 for the southern GStL (S.GStL). Mean pCO2 ± standard deviations of all the grid cells are provided for each dataset. The color scale represents pCO2 (µatm).
The ReCAD-NAACOM-pCO2 product demonstrates superior alignment with SOCAT observations in capturing those regional features that have been reported in previous observation-based studies (Fig. 7b), accurately representing the low pCO2 values in the LAS Mississippi River plume (box 1) and the elevated pCO2 levels in the WFS (box 2). In contrast, the global reconstructions of pCO2, represented by the ensemble of seven open-ocean pCO2 products (Fig. 7c), face challenges in resolving these regional pCO2 variations, as previously discussed in Wu et al. (2024b). The coastal pCO2 product from Roobaert et al. (2024a; ULB_SOMFFN_coastal_v2) also captures some small-scale structures like the low pCO2 in the LAS (Fig. 7d), but the ReCAD-NAACOM-pCO2 product exhibits values that are closer to the observations. In the northern region (box 3), the ReCAD-NAACOM-pCO2 product predicts higher pCO2 levels that are closer to observations in the nearshore region (Fig. 7b). This is not surprising, as ULB_SOMFNN_coastal_v2 is a global product known for its high accuracy on the global average.
In addition to these previously documented regional variations, our product reveals several notable features not previously captured by observations or other existing products. For instance, the GoMe displays intermediate pCO2 levels of around 380 µatm, which is distinctly higher than surrounding waters at comparable latitudes, a feature previously documented by a pCO2 product reconstructed using multiple linear regression (Signorini et al., 2013) and 5-year (2004–2009) mooring and cruise data (Vandemark et al., 2011). However, this contradicts two other studies based on numerical models (Cahill et al., 2016; Rutherford et al., 2021). In the southern GStL (S.GStL; box 4 in Fig. 7), pCO2 values are slightly higher compared to adjacent waters at similar latitudes, aligning with high nutrient concentrations typically observed in these river-influenced waters (Lavoie et al., 2021). These regional patterns could not be captured completely by the global products (Fig. 7c and d). The ability of the ReCAD-NAACOM-pCO2 product to resolve such regional features demonstrates its potential value for investigating coastal carbon dynamics and their responses to local and regional forcing factors in the NAACOM.
3.5 Evaluating the capacity of the product to detect decadal linear trends of pCO2
Using pCO2 products to accurately reconstruct pCO2 linear trends in coastal regions presents significant challenges due to the high spatial heterogeneity of coastal pCO2 dynamics. This heterogeneity often leads to sea surface pCO2 changes that deviate from atmospheric trends (Laruelle et al., 2018). Even when utilizing similar observational datasets, derived products may not consistently reflect the underlying trends. For instance, Wu et al. (2024b) examined the ability of various products to reflect pCO2 changes in the GoMx, a region where pCO2 trends exhibit significant spatial variability. Despite this heterogeneity, seven global open-ocean products (listed in Table 1) indicate trends similar to atmospheric pCO2 across the entire GoMx without regional differences. In contrast, the GoMx-specific regional product developed by Chen and Hu (2019) demonstrates no significant overall trend. The discrepancy in trend detection stems primarily from the design of the regression model and the selection of the input variables. These factors are critical in capturing the complex spatiotemporal variability of coastal pCO2 and its long-term evolution.
To assess the capability of the product in resolving decadal pCO2 trends, we conducted an analysis of the pCO2 evolution using three distinct regions within the NAACOM (three boxes in Fig. 7) as representative examples (Fig. 8). Decadal trends of deseasonalized time series were calculated following the protocol established by Sutton et al. (2022). The LAS (box 1 in Fig. 7) has been identified as an increasing CO2 sink characterized by a negative pCO2 rate increase from 2002 to 2021 (Wu et al., 2024b). Our product results for the extended period of 1993–2021 indicate that pCO2 increased at a rate of +0.44 ± 0.11 µatm yr−1 (Fig. 8a). This rate is significantly lower than the observed atmospheric pCO2 increase in this region during 2002–2021, which is approximately +1.8 µatm yr−1. These findings corroborate our previous conclusion that the LAS is an increasing CO2 sink, demonstrating the capability of our product in revealing long-term pCO2 trends in this dynamic river plume region, extending the analysis period by nearly a decade compared to previous studies. In contrast, the WFS (box 2 in Fig. 7) exhibits an accelerated pCO2 increase that is faster than the atmospheric pCO2 of around +2.0 µatm yr−1 (Fig. 8b), aligning with observations reported by Robbins et al. (2018), who found a transition from a CO2 sink to a source in this region during the 1990s.
Both ReCAD-NAACOM-pCO2 and SOCAT consistently report a pCO2 trend of around +2.3 to +2.5 µatm yr−1 in the northern region (box 3 in Fig. 7) over 1993–2021 (Fig. 8c), which is faster than the atmospheric pCO2 increase (around +2.0 µatm yr−1), suggesting that these areas are becoming a decreasing CO2 sink. However, limited observational data in this area necessitate cautious interpretation and warrant further validation in future research. Overall, the spatiotemporal heterogeneity in surface-ocean pCO2 trends across the NAACOM underscores the importance of long-term monitoring to elucidate the drivers of these trends, particularly in regions influenced by major current systems and in areas with limited observational data.
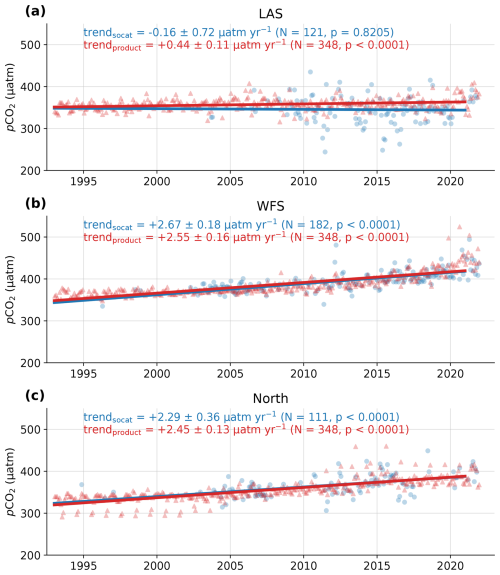
Figure 8Decadal linear trends of sea surface pCO2 in three regions of the NAACOM from 1993 to 2021. The blue and red dots are monthly average pCO2 values (deseasonalized) calculated from SOCAT observations and the reconstructed ReCAD-NAACOM-pCO2, respectively. The thick lines are linear fitted regression lines. The three regions are the boxes in Fig. 7: the (a) LAS (northern Gulf of Mexico shelf river plume region), (b) WFS, and (c) northern areas. Linear trends are calculated following the protocol established by Sutton et al. (2022). The numbers in parentheses are the number of months with data and p values.
3.6 Evaluating the uncertainty of the product
The uncertainty of the reconstructed pCO2 values in each grid cell was estimated by accumulating uncertainties from mapping (umap), gridding (ugrid), measurement (uobs), and input variables (uinputs; see Sect. 2.5 for further details on the calculation). To maintain a conservative estimate, we adopted the larger value of 5 µatm as uobs for all the data points. The gridded fCO2 values from SOCAT are reported as the averages of all samples collected within each grid cell. Accordingly, ugrid was quantified as the standard deviation of samples within each grid cell, calculated across six subregions. Following the previous literature (Roobaert et al., 2024a; Sharp et al., 2022), umap was calculated using the RMSE values of the model validation phase reported in Table 2. The uncertainty from the validation set (20 % of X1) was chosen for its sample size that was larger than the independent test set (X2) and for consistency with the 10-fold cross-validation results while avoiding potential underestimation from the training set. uinputs was calculated using a Monte Carlo simulation (Appendix B). These four sources of uncertainty were evaluated across different subregions of the NAACOM, as shown in Table 3. umap contributes the largest portion to the total number of uncertainties across all the sub-subregions, with a maximum value of up to 20.12 µatm in the GoMe. Overall, the ReCAD-NAACOM-pCO2 product demonstrates uncertainties ranging from 16 to 28 µatm across the six subregions and an average uncertainty of 23.25 µatm for the entire NAACOM.
Table 3Uncertainty estimates for the ReCAD-NAACOM-pCO2 product across the different subregions of the NAACOM. uobs, ugrid, umap, and uinputs represent the measurement uncertainty, gridding uncertainty, mapping uncertainty, and uncertainty accumulated from input variables, respectively (see Sect. 2.5 for further details). is the total combined uncertainty (µatm). The subregions are the GoMx, SAB, MAB, GoMe, SS, and GStL&GB.
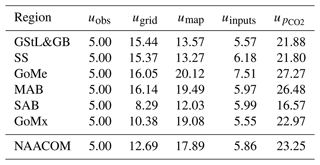
Our uncertainty estimation employs a conservative estimation using maximum values at the calculation step. This approach likely overestimates the true uncertainty. Despite this conservative method, our calculated uncertainty for the Atlantic margins is comparable to the 43.4 µatm reported by Sharp et al. (2022) for areas within 100 km of the North American Pacific margins, suggesting good performance of our product. It is important to note that our uncertainty calculation assumed independence among all the sources, which is a simplification. Recent research has highlighted that these uncertainties are often correlated (Ford et al., 2024). Future studies should consider these inter-variable correlations to refine uncertainty estimates. In addition, the uncertainties reported in this section and provided in the NetCDF file represent the propagated errors for individual pCO2 values in each grid cell. Methods to calculate uncertainties in regional averages of pCO2 or air–sea CO2 fluxes over specific spatial and temporal domains are detailed in Roobaert et al. (2024a) and Landschützer et al. (2014).
3.7 Challenges and limitations
Even though ReCAD-NAACOM-pCO2 resolves regional pCO2 variability with high accuracy in the NAACOM, this product still has room for improvement in the future. Potential areas of improvement include the 0.25° spatial resolution, which is inadequate for resolving submesoscale variability at the scale of 0.1–10 km (McWilliams, 1985). Furthermore, during the independent validation phase, the accuracy of the model-predicted values decreased in the GoMe (R2=0.49) and GoMx (R2=0.46) (Table 2), which may be due to the complex biological and physical conditions in the estuary plume regions in these two gulfs. In this study, we opted not to include chlorophyll-a (Chl-a) concentrations and wind speeds as input variables for model training and prediction. This decision was primarily due to the limited temporal coverage of satellite-derived Chl-a data, which only extends back to 1997 with the launch of the Sea-viewing Wide Field-of-view Sensor (SeaWiFS) satellite (O'Reilly et al., 1998). The inclusion of Chl-a would have restricted the temporal range of our model, potentially limiting its ability to capture long-term trends and variability in pCO2. Future versions of our model will aim to address this limitation. One potential approach is to develop a two-phase model: one phase for the period before 1997 without Chl-a data and another for the post-1997 period incorporating Chl-a information. Alternatively, we may explore methods to reconstruct historical Chl-a data or use proxy variables that correlate with biological productivity and are available for the entire study period.
In our previous work, we demonstrated that incorporating wind speeds and sea surface roughness data derived from synthetic aperture radar (SAR) could enhance model performance in predicting pCO2 at submesoscale resolutions (Wang et al., 2024). In this work, we evaluated the inclusion of wind speed as an input variable in our model. However, at the 0.25° resolution employed here, the addition of wind speed data did not significantly improve the model performance (it only increased the R2 by 0.1). Moreover, when using the same Monte Carlo simulation approach applied to other variables, incorporating wind speeds would introduce an additional 6 µatm uncertainty to pCO2 estimates, doubling the input-related uncertainties. Consequently, we excluded wind speeds from our regression model to reduce input-related uncertainties. Despite this omission, our product demonstrates robust capability in resolving regional variations, seasonal cycles, and decadal trends in pCO2, making it valuable for future studies.
The reconstructed fCO2 and pCO2 and the uncertainty in ReCAD (v1.1) are available as a NetCDF file at https://doi.org/10.5281/zenodo.14038561 (Wu et al., 2024a) and will be updated regularly.
The Python and MATLAB code used to process the data and create the figures included in this paper is provided at https://github.com/zelunwu/ReCAD (Wu, 2024).
The ReCAD-NAACOM-pCO2 product developed in this study represents a significant advancement in our ability to detect the spatial variations, seasonal cycles, and decadal changes of surface-ocean pCO2 dynamics in the NAACOM. By leveraging a two-step approach combining random forest and linear regression and a set of environmental predictors, we have created a high-resolution, long-term dataset (1993–2021 period) that captures the complex spatial and temporal variability of pCO2 across the region. On average, compared with all available samples from the SOCAT observations in our study region, the product has an R2 of 0.92, an RMSE of 12.70 µatm, an MAE of 7.55 µatm, and an MBE of 0.13 µatm for the entire NAACOM, with an average uncertainty of 23.25 µatm. The key findings from this study are the following:
-
The product demonstrates high accuracy and reliability, as evidenced by strong performance metrics during the training, validation, and independent test phases across the six subregions.
-
Distinct seasonal cycles are observed between the southern and northern subregions, with the product capturing nuanced features such as elevated pCO2 levels during fall and winter in the northern areas.
-
Comparison with global products highlights the superior ability of the ReCAD-NAACOM-pCO2 product to resolve small-scale coastal features and variability.
-
The pCO2 product successfully reconstructed decadal linear trends that were consistent with previous studies while also revealing a rapid increase in pCO2 in the northern region of the NAACOM.
While areas of future improvement exist, such as increasing spatial resolution and enhancing accuracy in estuary-plume-influenced regions, the ReCAD-NAACOM-pCO2 product provides a robust foundation for studying coastal carbon dynamics. This dataset will be valuable for investigating air–sea CO2 fluxes, assessing ocean acidification impacts, and understanding the role of coastal systems in the NAACOM.
Future research should validate the reconstructed trends, particularly in areas with limited observational data, and explore the mechanisms driving the spatiotemporal variability in pCO2 across the NAACOM region. Additionally, the methodologies developed here can contribute to a more comprehensive understanding of coastal-ocean carbon dynamics in the face of climate change and have the potential to be applied globally.
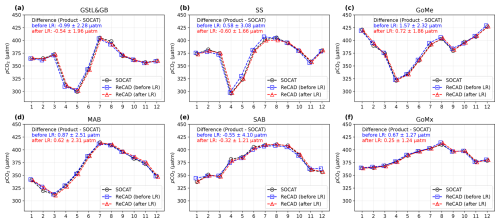
Figure A1Comparisons of the monthly pCO2 climatology with SOCAT observations across the six subregions: evaluations before and after LR calibration. Values indicate the mean difference (± 1 standard deviation; blue: before LR and red: after LR) between model-estimated pCO2 and SOCAT observations over the 12-month period, computed only at grid points and times where SOCAT measurements are available.
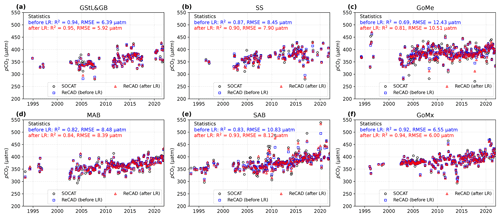
Figure A2Comparisons of deseasonalized monthly pCO2 anomalies with SOCAT observations across the six subregions: evaluations before and after LR calibration. Values indicate the R2 and RMSE between model-estimated pCO2 and SOCAT observations (blue: before LR; red: after LR), computed only at grid points and times where SOCAT measurements are available.
A crucial step in calculating uinputs is determining the uncertainties of the input variables. In our reconstructed model, there were four variables that needed to be evaluated: SST, SSS, SSH, and pCO2air. Our general principle was to adopt conservative estimates, using the largest reported uncertainty for each product when available.
SST errors are provided within the OISST product at the grid level. On the global average, OISST reports a mean bias and RMSE of −0.04 and 0.24 °C when compared with the observations on the global average (Huang et al., 2021). For our study region, we calculated the mean SST error across all the grid cells, yielding a value of 0.23 °C.
The SODA database assimilates observational data but does not directly provide SSS error estimates. Given this limitation in uncertainty reporting, we derived an estimate based on the RMSE between the model SSS and observations near our study region, as reported by Carton et al. (2018). Their analysis (their Fig. 8) indicates an RMSE exceeding 0.3 psu in the vicinity of our area of interest. In addition, interpolating the 0.5° SSS data to 0.25° resolution could potentially introduce more errors. To maintain a conservative approach in our uncertainty quantification, we doubled the uncertainty and adopted a value of 0.6 psu as the SSS uncertainty for our calculations.
SSH errors are directly provided in the dataset, which has a mean uncertainty of 1.8 cm in our study region.
pCO2air, calculated from xCO2air (MBL references), has a global mean uncertainty of 0.22 ppm.
To propagate these input uncertainties to the final pCO2 estimate, a Monte Carlo simulation approach was implemented:
-
For each input variable xi, random perturbations εi were generated following a normal distribution N(0,ui), where ui represents the uncertainty of the respective variable listed above.
-
Perturbed inputs (xi+εi) were used to calculate pCO2 with the established model.
-
The difference (Δi) between the reconstructed pCO2 before and after adding the perturbation was computed.
-
Steps 1, 2, and 3 were iterated 100 times for each input variable.
-
The uncertainty contribution from each variable was quantified as the standard deviation of the 100 Δi values in each grid cell.
The total uncertainty attributed to the input variables (uinputs) was then calculated as the square root of the quadratic sum of individual uncertainties:
The largest uncertainties propagated from these variables are sourced from SSS and SSH (Fig. B1a and c). Simulating salinity in coastal regions is still challenging due to complex land–ocean interaction. For the SSH, the greatest uncertainties were observed in the GoMe and GStL. Overall, uinputs is largest in the West Florida Shelf and nearshore waters around the GoMe, with a mean uinputs uncertainty of 5.9 ± 4.7 µatm for the entire NAACOM.
ZW: conceptualization, data curation, formal analysis, methodology, software, visualization, writing – original draft preparation, writing – review and editing. WL: funding acquisition, methodology, validation, writing – review and editing. AR: validation, writing – review and editing. LS: validation, writing – review and editing. XHY: project administration, supervision. WJC: conceptualization, project administration, supervision, validation, writing – review and editing.
The contact author has declared that none of the authors has any competing interests.
Publisher's note: Copernicus Publications remains neutral with regard to jurisdictional claims made in the text, published maps, institutional affiliations, or any other geographical representation in this paper. While Copernicus Publications makes every effort to include appropriate place names, the final responsibility lies with the authors.
The authors acknowledge the NOAA for providing the OISST data, the University of Maryland Ocean Climate Laboratory for the SODA dataset, and the European Union's CMEMS for the SSH data. We also express our gratitude to the scientific community for sharing their observational carbonate data in the SOCAT effort. SOCAT is an international effort endorsed by the International Ocean Carbon Coordination Project (IOCCP), the Surface Ocean Lower Atmosphere Study (SOLAS), and the Integrated Marine Biosphere Research (IMBeR) program to deliver a uniform quality-controlled surface-ocean CO2 database. The many researchers and funding agencies responsible for the collection of the data and the quality control are thanked for their contributions to SOCAT. We would also like to thank Fujian Satellite Date Development Company Ltd. and Fujian Hisea Digital Technology Company Ltd. for their cooperation in the pCO2 application. We would also like to thank the editor and two anonymous reviewers for their efforts in improving this work.
This work is part of Zelun Wu's PhD dissertation in the framework of the University of Delaware–Xiamen University Dual Degree Program in Oceanography.
This research has been supported by the Southern Marine Science and Engineering Guangdong Laboratory (Zhuhai) (grant no. SML2023SP238) for Wenfang Lu, the Industry-University Cooperation and Collaborative Education Projects (grant no. 202102245034), and the PhD fellowship from the State Key Laboratory of Marine Environmental Science at Xiamen University for Zelun Wu.
This paper was edited by Sabine Schmidt and reviewed by two anonymous referees.
Bakker, D. C. E., Pfeil, B., Landa, C. S., Metzl, N., O'Brien, K. M., Olsen, A., Smith, K., Cosca, C., Harasawa, S., Jones, S. D., Nakaoka, S., Nojiri, Y., Schuster, U., Steinhoff, T., Sweeney, C., Takahashi, T., Tilbrook, B., Wada, C., Wanninkhof, R., Alin, S. R., Balestrini, C. F., Barbero, L., Bates, N. R., Bianchi, A. A., Bonou, F., Boutin, J., Bozec, Y., Burger, E. F., Cai, W.-J., Castle, R. D., Chen, L., Chierici, M., Currie, K., Evans, W., Featherstone, C., Feely, R. A., Fransson, A., Goyet, C., Greenwood, N., Gregor, L., Hankin, S., Hardman-Mountford, N. J., Harlay, J., Hauck, J., Hoppema, M., Humphreys, M. P., Hunt, C. W., Huss, B., Ibánhez, J. S. P., Johannessen, T., Keeling, R., Kitidis, V., Körtzinger, A., Kozyr, A., Krasakopoulou, E., Kuwata, A., Landschützer, P., Lauvset, S. K., Lefèvre, N., Lo Monaco, C., Manke, A., Mathis, J. T., Merlivat, L., Millero, F. J., Monteiro, P. M. S., Munro, D. R., Murata, A., Newberger, T., Omar, A. M., Ono, T., Paterson, K., Pearce, D., Pierrot, D., Robbins, L. L., Saito, S., Salisbury, J., Schlitzer, R., Schneider, B., Schweitzer, R., Sieger, R., Skjelvan, I., Sullivan, K. F., Sutherland, S. C., Sutton, A. J., Tadokoro, K., Telszewski, M., Tuma, M., van Heuven, S. M. A. C., Vandemark, D., Ward, B., Watson, A. J., and Xu, S.: A multi-decade record of high-quality fCO2 data in version 3 of the Surface Ocean CO2 Atlas (SOCAT), Earth Syst. Sci. Data, 8, 383–413, https://doi.org/10.5194/essd-8-383-2016, 2016.
Breiman, L.: Random Forests, Machine Learning, 45, 5–32, https://doi.org/10.1023/A:1010933404324, 2001.
Cahill, B., Wilkin, J., Fennel, K., Vandemark, D., and Friedrichs, M. A. M.: Interannual and seasonal variabilities in air-sea CO2 fluxes along the U.S. eastern continental shelf and their sensitivity to increasing air temperatures and variable winds: U.S. East Coast Shelf Air-Sea CO2 Fluxes, J. Geophys. Res.-Biogeo., 121, 295–311, https://doi.org/10.1002/2015JG002939, 2016.
Cai, W.-J., Xu, Y.-Y., Feely, R. A., Wanninkhof, R., Jönsson, B., Alin, S. R., Barbero, L., Cross, J. N., Azetsu-Scott, K., Fassbender, A. J., Carter, B. R., Jiang, L.-Q., Pepin, P., Chen, B., Hussain, N., Reimer, J. J., Xue, L., Salisbury, J. E., Hernández-Ayón, J. M., Langdon, C., Li, Q., Sutton, A. J., Chen, C.-T. A., and Gledhill, D. K.: Controls on surface water carbonate chemistry along North American ocean margins, Nat. Commun., 11, 2691, https://doi.org/10.1038/s41467-020-16530-z, 2020.
Carter, B. R., Feely, R. A., Williams, N. L., Dickson, A. G., Fong, M. B., and Takeshita, Y.: Updated methods for global locally interpolated estimation of alkalinity, pH, and nitrate: LIR: Global alkalinity, pH, and nitrate estimates, Limnol. Oceanogr. Methods, 16, 119–131, https://doi.org/10.1002/lom3.10232, 2018.
Carton, J. A., Chepurin, G. A., and Chen, L.: SODA3: A New Ocean Climate Reanalysis, J. Climate, 31, 6967–6983, https://doi.org/10.1175/JCLI-D-18-0149.1, 2018.
Chau, T. T. T., Gehlen, M., and Chevallier, F.: A seamless ensemble-based reconstruction of surface ocean pCO2 and air–sea CO2 fluxes over the global coastal and open oceans, Biogeosciences, 19, 1087–1109, https://doi.org/10.5194/bg-19-1087-2022, 2022.
Chen, C.-T. A., Huang, T.-H., Chen, Y.-C., Bai, Y., He, X., and Kang, Y.: Air–sea exchanges of CO2 in the world's coastal seas, Biogeosciences, 10, 6509–6544, https://doi.org/10.5194/bg-10-6509-2013, 2013.
Chen, S. and Hu, C.: Environmental controls of surface water pCO2 in different coastal environments: Observations from marine buoys, Cont. Shelf Res., 183, 73–86, https://doi.org/10.1016/j.csr.2019.06.007, 2019.
E.U. Copernicus Marine Service Information (CMEMS): Global Ocean Gridded L4 Sea Surface Heights and Derived Variables Reprocessed (1993–ongoing), [data set], https://doi.org/10.48670/moi-00148, 2021.
Dai, M., Su, J., Zhao, Y., Hofmann, E. E., Cao, Z., Cai, W.-J., Gan, J., Lacroix, F., Laruelle, G. G., Meng, F., Müller, J. D., Regnier, P. A. G., Wang, G., and Wang, Z.: Carbon Fluxes in the Coastal Ocean: Synthesis, Boundary Processes and Future Trends, Annu. Rev. Earth Planet. Sci., 50, 593–626, https://doi.org/10.1146/annurev-earth-032320-090746, 2022.
Fay, A. R., Gregor, L., Landschützer, P., McKinley, G. A., Gruber, N., Gehlen, M., Iida, Y., Laruelle, G. G., Rödenbeck, C., Roobaert, A., and Zeng, J.: SeaFlux: harmonization of air–sea CO2 fluxes from surface pCO2 data products using a standardized approach, Earth Syst. Sci. Data, 13, 4693–4710, https://doi.org/10.5194/essd-13-4693-2021, 2021.
Fennel, K. and Wilkin, J.: Quantifying biological carbon export for the northwest North Atlantic continental shelves, Geophys. Res. Lett., 36, L18605, https://doi.org/10.1029/2009GL039818, 2009.
Fennel, K., Alin, S., Barbero, L., Evans, W., Bourgeois, T., Cooley, S., Dunne, J., Feely, R. A., Hernandez-Ayon, J. M., Hu, X., Lohrenz, S., Muller-Karger, F., Najjar, R., Robbins, L., Shadwick, E., Siedlecki, S., Steiner, N., Sutton, A., Turk, D., Vlahos, P., and Wang, Z. A.: Carbon cycling in the North American coastal ocean: a synthesis, Biogeosciences, 16, 1281–1304, https://doi.org/10.5194/bg-16-1281-2019, 2019.
Ford, D. J., Blannin, J., Watts, J., Watson, A. J., Landschützer, P., Jersild, A., and Shutler, J. D.: A Comprehensive Analysis of Air-Sea CO2 Flux Uncertainties Constructed From Surface Ocean Data Products, Global Biogeochem. Cy., 38, e2024GB008188, https://doi.org/10.1029/2024GB008188, 2024.
Friedlingstein, P., O'Sullivan, M., Jones, M. W., Andrew, R. M., Bakker, D. C. E., Hauck, J., Landschützer, P., Le Quéré, C., Luijkx, I. T., Peters, G. P., Peters, W., Pongratz, J., Schwingshackl, C., Sitch, S., Canadell, J. G., Ciais, P., Jackson, R. B., Alin, S. R., Anthoni, P., Barbero, L., Bates, N. R., Becker, M., Bellouin, N., Decharme, B., Bopp, L., Brasika, I. B. M., Cadule, P., Chamberlain, M. A., Chandra, N., Chau, T.-T.-T., Chevallier, F., Chini, L. P., Cronin, M., Dou, X., Enyo, K., Evans, W., Falk, S., Feely, R. A., Feng, L., Ford, D. J., Gasser, T., Ghattas, J., Gkritzalis, T., Grassi, G., Gregor, L., Gruber, N., Gürses, Ö., Harris, I., Hefner, M., Heinke, J., Houghton, R. A., Hurtt, G. C., Iida, Y., Ilyina, T., Jacobson, A. R., Jain, A., Jarníková, T., Jersild, A., Jiang, F., Jin, Z., Joos, F., Kato, E., Keeling, R. F., Kennedy, D., Klein Goldewijk, K., Knauer, J., Korsbakken, J. I., Körtzinger, A., Lan, X., Lefèvre, N., Li, H., Liu, J., Liu, Z., Ma, L., Marland, G., Mayot, N., McGuire, P. C., McKinley, G. A., Meyer, G., Morgan, E. J., Munro, D. R., Nakaoka, S.-I., Niwa, Y., O'Brien, K. M., Olsen, A., Omar, A. M., Ono, T., Paulsen, M., Pierrot, D., Pocock, K., Poulter, B., Powis, C. M., Rehder, G., Resplandy, L., Robertson, E., Rödenbeck, C., Rosan, T. M., Schwinger, J., Séférian, R., Smallman, T. L., Smith, S. M., Sospedra-Alfonso, R., Sun, Q., Sutton, A. J., Sweeney, C., Takao, S., Tans, P. P., Tian, H., Tilbrook, B., Tsujino, H., Tubiello, F., van der Werf, G. R., van Ooijen, E., Wanninkhof, R., Watanabe, M., Wimart-Rousseau, C., Yang, D., Yang, X., Yuan, W., Yue, X., Zaehle, S., Zeng, J., and Zheng, B.: Global Carbon Budget 2023, Earth Syst. Sci. Data, 15, 5301–5369, https://doi.org/10.5194/essd-15-5301-2023, 2023.
Fu, Z., Hu, L., Chen, Z., Zhang, F., Shi, Z., Hu, B., Du, Z., and Liu, R.: Estimating spatial and temporal variation in ocean surface pCO2 in the Gulf of Mexico using remote sensing and machine learning techniques, Sci. Total Environ., 745, 140965, https://doi.org/10.1016/j.scitotenv.2020.140965, 2020.
Gloege, L., Yan, M., Zheng, T., and McKinley, G. A.: Improved Quantification of Ocean Carbon Uptake by Using Machine Learning to Merge Global Models and pCO2 Data, J. Adv. Model. Earth Sy., 14, e2021MS002620, https://doi.org/10.1029/2021MS002620, 2022.
Gregor, L. and Gruber, N.: OceanSODA-ETHZ: a global gridded data set of the surface ocean carbonate system for seasonal to decadal studies of ocean acidification, Earth Syst. Sci. Data, 13, 777–808, https://doi.org/10.5194/essd-13-777-2021, 2021.
Hersbach, H., Bell, B., Berrisford, P., Biavati, G., Horányi, A., Muñoz Sabater, J., Nicolas, J., Peubey, C., Radu, R., Rozum, I., and others: ERA5 monthly averaged data on single levels from 1979 to present, Copernicus Climate Change Service (C3S) Climate Data Store (CDS), 10, 252–266, https://doi.org/10.24381/cds.f17050d7, 2019.
Huang, B., Liu, C., Banzon, V., Freeman, E., Graham, G., Hankins, B., Smith, T., and Zhang, H.-M.: Improvements of the Daily Optimum Interpolation Sea Surface Temperature (DOISST) Version 2.1, J. Climate, 34, 2923–2939, https://doi.org/10.1175/JCLI-D-20-0166.1, 2021.
Hughes, I. and Hase, T. P. A.: Measurements and their uncertainties: a practical guide to modern error analysis, Oxford University Press, New York, 136 pp., ISBN 978-0-19-956632-7, 978-0-19-956633-4, 2010.
Iida, Y., Takatani, Y., Kojima, A., and Ishii, M.: Global trends of ocean CO2 sink and ocean acidification: an observation-based reconstruction of surface ocean inorganic carbon variables, J. Oceanogr., 77, 323–358, https://doi.org/10.1007/s10872-020-00571-5, 2021.
Kealoha, A. K., Shamberger, K. E. F., DiMarco, S. F., Thyng, K. M., Hetland, R. D., Manzello, D. P., Slowey, N. C., and Enochs, I. C.: Surface Water CO2 variability in the Gulf of Mexico (1996–2017), Sci. Rep., 10, 12279, https://doi.org/10.1038/s41598-020-68924-0, 2020.
Lan, X., Tans, P., Thoning, K., and NOAA Global Monitoring Laboratory: NOAA Greenhouse Gas Marine Boundary Layer Reference – CO2, NOAA GML [data set], https://doi.org/10.15138/DVNP-F961, 2023.
Landschützer, P., Gruber, N., Bakker, D. C. E., and Schuster, U.: Recent variability of the global ocean carbon sink, Global Biogeochem. Cy., 28, 927–949, https://doi.org/10.1002/2014GB004853, 2014.
Landschützer, P., Gruber, N., and Bakker, D. C. E.: An observation-based global monthly gridded sea surface pCO2 product from 1982 onward and its monthly climatology (NCEI Accession 0160558), NOAA National Centers for Environmental Information [data set], https://doi.org/10.7289/V5Z899N6, 2017.
Landschützer, P., Laruelle, G. G., Roobaert, A., and Regnier, P.: A uniform pCO2 climatology combining open and coastal oceans, Earth Syst. Sci. Data, 12, 2537–2553, https://doi.org/10.5194/essd-12-2537-2020, 2020.
Laruelle, G. G., Landschützer, P., Gruber, N., Tison, J.-L., Delille, B., and Regnier, P.: Global high-resolution monthly pCO2 climatology for the coastal ocean derived from neural network interpolation, Biogeosciences, 14, 4545–4561, https://doi.org/10.5194/bg-14-4545-2017, 2017.
Laruelle, G. G., Cai, W.-J., Hu, X., Gruber, N., Mackenzie, F. T., and Regnier, P.: Continental shelves as a variable but increasing global sink for atmospheric carbon dioxide, Nat. Commun., 9, 454, https://doi.org/10.1038/s41467-017-02738-z, 2018.
Lavoie, D., Lambert, N., Starr, M., Chassé, J., Riche, O., Le Clainche, Y., Azetsu-Scott, K., Béjaoui, B., Christian, J. R., and Gilbert, D.: The Gulf of St. Lawrence Biogeochemical Model: A Modelling Tool for Fisheries and Ocean Management, Front. Mar. Sci., 8, 732269, https://doi.org/10.3389/fmars.2021.732269, 2021.
Lohrenz, S. E. and Cai, W.-J.: Satellite ocean color assessment of air-sea fluxes of CO2 in a river-dominated coastal margin: CO2 FLUXES in a River-Dominated Margin, Geophys. Res. Lett., 33, L01601, https://doi.org/10.1029/2005GL023942, 2006.
Lu, W., Su, H., Yang, X., and Yan, X.-H.: Subsurface temperature estimation from remote sensing data using a clustering-neural network method, Remote Sens. Environ., 229, 213–222, https://doi.org/10.1016/j.rse.2019.04.009, 2019.
McWilliams, J. C.: Submesoscale, coherent vortices in the ocean, Rev. Geophys., 23, 165–182, https://doi.org/10.1029/RG023i002p00165, 1985.
O'Reilly, J. E., Maritorena, S., Mitchell, B. G., Siegel, D. A., Carder, K. L., Garver, S. A., Kahru, M., and McClain, C.: Ocean color chlorophyll algorithms for SeaWiFS, J. Geophys. Res., 103, 24937–24953, https://doi.org/10.1029/98JC02160, 1998.
Ren, H., Lu, W., Xiao, W., Zhu, Q., Xiao, C., and Lai, Z.: Intraseasonal response of marine planktonic ecosystem to summertime Madden-Julian Oscillation in the South China Sea: A model study, Prog. Oceanogr., 224, 103251, https://doi.org/10.1016/j.pocean.2024.103251, 2024.
Robbins, L. L., Daly, K. L., Barbero, L., Wanninkhof, R., He, R., Zong, H., Lisle, J. T., Cai, W. -J., and Smith, C. G.: Spatial and Temporal Variability of pCO2, Carbon Fluxes, and Saturation State on the West Florida Shelf, J. Geophys. Res.-Oceans, 123, 6174–6188, https://doi.org/10.1029/2018JC014195, 2018.
Rödenbeck, C., DeVries, T., Hauck, J., Le Quéré, C., and Keeling, R. F.: Data-based estimates of interannual sea–air CO2 flux variations 1957–2020 and their relation to environmental drivers, Biogeosciences, 19, 2627–2652, https://doi.org/10.5194/bg-19-2627-2022, 2022.
Roobaert, A., Resplandy, L., Laruelle, G. G., Liao, E., and Regnier, P.: A framework to evaluate and elucidate the driving mechanisms of coastal sea surface pCO2 seasonality using an ocean general circulation model (MOM6-COBALT), Ocean Sci., 18, 67–88, https://doi.org/10.5194/os-18-67-2022, 2022.
Roobaert, A., Regnier, P., Landschützer, P., and Laruelle, G. G.: A novel sea surface pCO2-product for the global coastal ocean resolving trends over 1982–2020, Earth Syst. Sci. Data, 16, 421–441, https://doi.org/10.5194/essd-16-421-2024, 2024a.
Roobaert, A., Resplandy, L., Laruelle, G. G., Liao, E., and Regnier, P.: Unraveling the Physical and Biological Controls of the Global Coastal CO2 Sink, Global Biogeochem. Cy., 38, e2023GB007799, https://doi.org/10.1029/2023GB007799, 2024b.
Ross, A. C., Stock, C. A., Adcroft, A., Curchitser, E., Hallberg, R., Harrison, M. J., Hedstrom, K., Zadeh, N., Alexander, M., Chen, W., Drenkard, E. J., du Pontavice, H., Dussin, R., Gomez, F., John, J. G., Kang, D., Lavoie, D., Resplandy, L., Roobaert, A., Saba, V., Shin, S.-I., Siedlecki, S., and Simkins, J.: A high-resolution physical–biogeochemical model for marine resource applications in the northwest Atlantic (MOM6-COBALT-NWA12 v1.0), Geosci. Model Dev., 16, 6943–6985, https://doi.org/10.5194/gmd-16-6943-2023, 2023.
Rutherford, K., Fennel, K., Atamanchuk, D., Wallace, D., and Thomas, H.: A modelling study of temporal and spatial pCO2 variability on the biologically active and temperature-dominated Scotian Shelf, Biogeosciences, 18, 6271–6286, https://doi.org/10.5194/bg-18-6271-2021, 2021.
Salisbury, J. E. and Jönsson, B. F.: Rapid warming and salinity changes in the Gulf of Maine alter surface ocean carbonate parameters and hide ocean acidification, Biogeochemistry, 141, 401–418, https://doi.org/10.1007/s10533-018-0505-3, 2018.
Sharp, J. D., Fassbender, A. J., Carter, B. R., Lavin, P. D., and Sutton, A. J.: A monthly surface pCO2 product for the California Current Large Marine Ecosystem, Earth Syst. Sci. Data, 14, 2081–2108, https://doi.org/10.5194/essd-14-2081-2022, 2022.
Signorini, S. R., Mannino, A., Najjar, R. G., Friedrichs, M. A. M., Cai, W.-J., Salisbury, J., Wang, Z. A., Thomas, H., and Shadwick, E.: Surface ocean pCO2 seasonality and sea-air CO2 flux estimates for the North American east coast, J. Geophys. Res.-Oceans, 118, 5439–5460, https://doi.org/10.1002/jgrc.20369, 2013.
Song, L., Lee, Z., Shang, S., Huang, B., Wu, J., Wu, Z., Lu, W., and Liu, X.: On the spatial and temporal variations of primary production in the South China Sea, IEEE T. Geosci. Remote, 61, 4201514, https://doi.org/10.1109/TGRS.2023.3241209, 2023.
Su, H., Zhang, H., Geng, X., Qin, T., Lu, W., and Yan, X.-H.: OPEN: A New Estimation of Global Ocean Heat Content for Upper 2000 Meters from Remote Sensing Data, Remote Sensing, 12, 2294, https://doi.org/10.3390/rs12142294, 2020.
Sutton, A. J., Battisti, R., Carter, B., Evans, W., Newton, J., Alin, S., Bates, N. R., Cai, W.-J., Currie, K., Feely, R. A., Sabine, C., Tanhua, T., Tilbrook, B., and Wanninkhof, R.: Advancing best practices for assessing trends of ocean acidification time series, Front. Mar. Sci., 9, 1045667, https://doi.org/10.3389/fmars.2022.1045667, 2022.
Takahashi, T., Sutherland, S. C., and Kozyr, A.: Global Ocean Surface Water Partial Pressure of CO2 Database: Measurements Performed During 1957–2019 (LDEO Database Version 2019) (NCEI Accession 0160492). Version 9.9, NOAA National Centers for Environmental Information [data set], https://doi.org/10.3334/CDIAC/OTG.NDP088(V2015), 2020.
Taylor, J. R.: An introduction to error analysis: the study of uncertainties in physical measurements, 2nd edn., University Science Books, Sausalito, Calif, 327 pp., ISBN 978-0-935702-42-2, 978-0-935702-75-0, 1997.
Vandemark, D., Salisbury, J. E., Hunt, C. W., Shellito, S. M., Irish, J. D., McGillis, W. R., Sabine, C. L., and Maenner, S. M.: Temporal and spatial dynamics of CO2 air-sea flux in the Gulf of Maine, J. Geophys. Res., 116, C01012, https://doi.org/10.1029/2010JC006408, 2011.
Wang, T., Yu, P., Wu, Z., Lu, W., Liu, X., Li, Q. P., and Huang, B.: Revisiting the Intraseasonal Variability of Chlorophyll-a in the Adjacent Luzon Strait With a New Gap-Filled Remote Sensing Data Set, IEEE T. Geosci. Remote, 60, 4201311, https://doi.org/10.1109/TGRS.2021.3067646, 2021.
Wang, Y., Wu, Z., Lu, W., Yu, S., Li, S., Meng, L., Geng, X., and Yan, X.-H.: Remote sensing estimations of the seawater partial pressure of CO2 using sea surface roughness derived from Synthetic Aperture Radar, IEEE T. Geosci. Remote, 62, 4204913, https://doi.org/10.1109/TGRS.2024.3379984, 2024.
Wang, Z., Wang, G., Guo, X., Bai, Y., Xu, Y., and Dai, M.: Spatial reconstruction of long-term (2003–2020) sea surface pCO2 in the South China Sea using a machine-learning-based regression method aided by empirical orthogonal function analysis, Earth Syst. Sci. Data, 15, 1711–1731, https://doi.org/10.5194/essd-15-1711-2023, 2023.
Wang, Z. A., Bienvenu, D. J., Mann, P. J., Hoering, K. A., Poulsen, J. R., Spencer, R. G. M., and Holmes, R. M.: Inorganic carbon speciation and fluxes in the Congo River: The Congo River Inorganic Carbon System, Geophys. Res. Lett., 40, 511–516, https://doi.org/10.1002/grl.50160, 2013.
Wanninkhof, R., Barbero, L., Byrne, R., Cai, W.-J., Huang, W.-J., Zhang, J.-Z., Baringer, M., and Langdon, C.: Ocean acidification along the Gulf Coast and East Coast of the USA, Cont. Shelf Res., 98, 54–71, https://doi.org/10.1016/j.csr.2015.02.008, 2015.
Weiss, R. F. and Price, B. A.: Nitrous oxide solubility in water and seawater, Mar. Chem., 8, 347–359, https://doi.org/10.1016/0304-4203(80)90024-9, 1980.
Wu, Z.: ReCAD, GitHub [code], https://github.com/zelunwu/ReCAD, last access: 1 December 2024.
Wu, Z., Lu, W., Roobaert, A., Song, L., Yan, X.-H., and Cai, W.-J.: A Reconstructed Coastal Acidification Database (ReCAD) pCO2 data product for the North American Atlantic Coastal Ocean Margins (1.1), Zenodo [data set], https://doi.org/10.5281/zenodo.14038561, 2024a.
Wu, Z., Wang, H., Liao, E., Hu, C., Edwing, K., Yan, X.-H., and Cai, W.-J.: Air-sea CO2 flux in the Gulf of Mexico from observations and multiple machine-learning data products, Prog. Oceanogr., 223, 103244, https://doi.org/10.1016/j.pocean.2024.103244, 2024b.
Xu, Y., Cai, W., Wanninkhof, R., Salisbury, J., Reimer, J., and Chen, B.: Long-Term Changes of Carbonate Chemistry Variables Along the North American East Coast, J. Geophys. Res.-Oceans, 125, e2019JC015982, https://doi.org/10.1029/2019JC015982, 2020.
Yang, G. G., Wang, Q., Feng, J., He, L., Li, R., Lu, W., Liao, E., and Lai, Z.: Can three-dimensional nitrate structure be reconstructed from surface information with artificial intelligence? – A proof-of-concept study, Sci. Total Environ., 924, 171365, https://doi.org/10.1016/j.scitotenv.2024.171365, 2024.
Zeng, J., Nojiri, Y., Landschützer, P., Telszewski, M., and Nakaoka, S.: A Global Surface Ocean fCO2 Climatology Based on a Feed-Forward Neural Network, J. Atmos. Ocean. Tech., 31, 1838–1849, https://doi.org/10.1175/JTECH-D-13-00137.1, 2014.
- Abstract
- Introduction
- Data and methods
- Results and discussion
- Data availability
- Code availability
- Conclusions
- Appendix A: Before and after LR calibration
- Appendix B: Monte Carlo simulation in calculating uinputs
- Author contributions
- Competing interests
- Disclaimer
- Acknowledgements
- Financial support
- Review statement
- References
- Abstract
- Introduction
- Data and methods
- Results and discussion
- Data availability
- Code availability
- Conclusions
- Appendix A: Before and after LR calibration
- Appendix B: Monte Carlo simulation in calculating uinputs
- Author contributions
- Competing interests
- Disclaimer
- Acknowledgements
- Financial support
- Review statement
- References