the Creative Commons Attribution 4.0 License.
the Creative Commons Attribution 4.0 License.
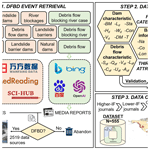
A worldwide event-based debris flow barrier dam dataset from 1800 to 2023
Haiguang Cheng
Shuang Liu
Xiaopeng Zhang
Hao Li
Qiyuan Zhang
Lan Ning
Manish Raj Gouli
Pu Li
Anna Yang
Peng Zhao
Junyu Liu
Debris flows, as a special kind of landslide, often block rivers to form barrier dams and trigger a series of disasters such as upstream aggradation and outburst floods. The understanding of debris flow barrier dams (DFBDs) is poor, mostly due to existing studies focusing on individual events and a lack of summarization of multiple DFBD events. The existing global or regional datasets of landslide barrier dams (LDs) contain only a few cases of DFBDs and ignore the differences between DFBDs and other landslide barrier dams (LDs), such as the dams of rock slides, debris avalanches, or earth slides. To fill this gap, we reviewed 2519 high-quality literature and media reports. Focusing on identified debris flow damming events, a rigorous data review and validation process was conducted using Google Earth. A systematic approach was employed to prioritize conflicting information from various data sources. Consequently, a global dataset was compiled, encompassing 555 historical DFBDs from 1800 to 2023.
This pioneering global dataset includes 6 categories and 38 attributes, detailing DFBDs. It captures basic information (location, the date of formation, etc.), dam characteristics (height, length, volume, etc.), lake characteristics (area, capacity, length), debris flow characteristics (velocity, discharge, volume, etc.), failure characteristics (peak discharge, loss of life, etc.), and climate characteristics (precipitation and temperature). Our dataset elucidates that DFBDs exhibit key features of instability, complete blockage, and overtopping failure. The number of such dams has notably increased, especially in China. A total of 15 % of channels showed recurrent debris flows, resulting in DFBDs that make up 35 % of all DFBDs. Further analysis suggests that the Ls (AHV) model should be used for priority use, followed by the DBI model, in the stability assessment of DFBDs. Compared to other barrier dam datasets, our dataset is more targeted places greater emphasis on the review of raw data, and stresses the unification of terminology and concepts (i.e., blockage modes and stability), ensuring the consistency and accuracy of the data. The dataset and results in this work may help to deepen the understanding of DFBD formation, distribution, and evolution. The DFBD dataset can be accessed through this link: https://doi.org/10.5281/zenodo.14766647 (Cheng et al., 2025).
- Article
(5464 KB) - Full-text XML
-
Supplement
(1353 KB) - BibTeX
- EndNote
Debris flows, composed of fine- and coarse-grained components, boulders, wood, and water, are a rapid two-phase flow with non-zero yield stress (Hungr et al., 2014). When debris flows carry large amounts of sediment and flow rapidly in a valley, they may accumulate in a narrow river channel and form a barrier dam, known as a debris flow barrier dam (DFBD) (Fan et al., 2020; Yin et al., 2016; Yu et al., 2022; Zhang et al., 2022a). The formation of such a barrier dam not only changes the original hydrogeological conditions but may also result in secondary disasters, such as floods, landslides, and even larger debris flows, posing a serious threat to human society and the natural environment (Cui et al., 2016; Gouli et al., 2025; Hu et al., 2011; Liu et al., 2019). For example, on 7 August 2010, triggered by heavy rainfall, a large-scale debris flow broke out in Luojiayu and Sanyanyu Gully in Zhouqu County, China. After the debris flow passed through Zhouqu City, it blocked the Bailong River and formed a submerged dam (Fig. 1a). The water level in the upper reaches rose sharply, which submerged half of Zhouqu City, resulting in 1364 casualties and 401 missing persons (Chong et al., 2021; Hu et al., 2010). The submerged dam had strong fluidity, which makes conventional emergency response measures ineffective and poses a significant challenge to rescue and disaster relief efforts. Under such critical circumstances, military forces were urgently deployed to conduct blasting operations for flood discharge (Fig. 1b).
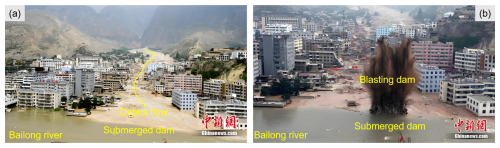
Figure 1Post-disaster images of the Zhouqu debris flow. (a) The debris flow rushed into the Bailong River, forming a submerged dam; (b) blasting operations on the debris flow barrier dam to accelerate discharge. The images were obtained from China News Service (https://www.chinanews.com.cn/, last access: 15 February 2025).
Compared with other types of landslide barrier dams (LDs), DFBDs possess unique characteristics, with differences that are primarily manifested in dam geometry, material composition, and internal structure. In terms of dam geometry, DFBDs have lower heights and gentler upstream and downstream slopes than other types of LDs (Cheng et al., 2007a, b; Dang et al., 2009). Regarding material composition, DFBD materials have a near-saturated water content, which is significantly higher than that of other types of LDs (Cheng et al., 2007a, b; Dang et al., 2009; Wang et al., 2017). Moreover, DFBDs have a higher clay content (Dang et al., 2009; Liu et al., 2014) and more rounded particles compared to other types of LDs (Dang et al., 2009). In terms of internal structure, DFBDs are more compact, with poorer grain sorting and lower permeability (Dang et al., 2009; Liu et al., 2014). The differences mentioned above make the stability and failure characteristics of DFBDs distinctly different from those of other LDs (Ruan et al., 2021).
Currently, studies on DFBDs mainly focus on single events (Hu et al., 2010, 2011) or on physical and numerical experiments conducted with single events as the prototypes, focusing on the research of river obstruction by debris flows (Chen et al., 2022; Dang et al., 2009; Ruan et al., 2021). In terms of properties and scale, debris flows that form barrier dams are typically large-scale and cohesive, with high density and uniformity, exhibiting considerable resistance to erosion (Chen et al., 2019; Ruan et al., 2021). In terms of topography, the rivers and valleys blocked by DFBDs are generally narrow, with steep terrain slopes (Song et al., 2023; Wang et al., 2017; Yu et al., 2022).
Isolated studies of individual DFBD events cannot reflect the overall distribution characteristics. However, statistical analysis of a large amount of historical data on barrier dam disasters can help to clarify this issue. Some scholars have conducted extensive studies on parameters such as the geometric characteristics, breaching, longevity, and stability of barrier dams by establishing datasets (Casagli et al., 2003; Dong et al., 2014; Fan et al., 2012a, 2017; Peng and Zhang, 2012a, b; Tacconi Stefanelli et al., 2015, 2016). However, there are relatively few cases of DFBDs in these datasets. The conclusions drawn from these barrier dam datasets may not be applicable to DFBDs. Therefore, there is an urgent need to establish a global comprehensive dataset specifically for DFBDs, laying a data foundation for in-depth research on such dams in the future, which is one of the goals of this study.
After the formation of a barrier dam, timely predictions of the stability of the dam and of the outburst peak discharge are key to formulating disaster reduction measures, and this is also a hot topic in barrier-dam-related research (Azimi et al., 2015; Casagli and Ermini, 1999; Korup, 2004). Based on statistical analysis methods, some scholars analyzed the influence of dam structure characteristics, dam material characteristics, hydrological characteristics, and other factors on the stability of dams and established some models for evaluating barrier dam stability (Dong et al., 2011; Ermini and Casagli, 2003). Other studies based on historical statistical cases summarized parameter models for peak discharge to achieve rapid prediction of the peak discharge of barrier dam breaches (Azimi et al., 2015; Hakimzadeh et al., 2014; Hooshyaripor et al., 2014; Xu and Zhang, 2009). For example, Xu and Zhang (2009) collected 182 dam break cases and used a multi-parameter nonlinear regression method for statistical regression analysis. They established the relationships between the breach dimensions, peak discharge, and parameters of the barrier dam and the failure mode. However, these studies did not strictly differentiate between types of barrier dams, focusing more on LDs. Considering the fact that DFBDs have unique characteristics compared to LDs (Cheng et al., 2007a, b; Dang et al., 2009; Ruan et al., 2021), the applicability of stability and peak-discharge models, originally designed for LDs, to DFBDs remains unclear. This constitutes the second key issue to be explored in this study.
This study established a dataset containing 555 DFBDs worldwide by reviewing 2519 literature and media reports. This dataset includes information on DFBDs, such as the formation time, location, geometric characteristics, longevity, peak discharge, failure characteristics, blockage modes, failure mechanisms, stability, and loss of life. A detailed analysis was conducted on the spatiotemporal distribution, blockage modes, failure mechanisms, longevity, and stability of DFBDs. The applicability of stability and peak-discharge models originally designed for LDs to DFBDs is discussed. Compared with other barrier dam datasets, our dataset focuses exclusively on DFBDs and stands out for its emphasis on the unity of terminology and concepts, as well as for the review and validation of raw data, to ensure data consistency and accuracy.
2.1 Data sources
In the process of building the dataset, we adopted a comprehensive and systematic approach to collect and analyze data. The data sources, amounting to 2519, mainly included peer-reviewed scientific literature, data released by government agencies, proceedings from professional conferences, and reports from authoritative news media. We placed special emphasis on selecting publications that have a good reputation and professionalism in the field to ensure the accuracy and authority of the data. To ensure breadth and diversity in the data, we have made every effort to consult academic journal literature from different countries and regions (China, Japan, Taiwan, the United States, Italy, etc.) to obtain records of DFBDs from various perspectives. Additionally, recognizing that media reports offer real-time and first-hand information on the formation and impact of DFBDs, we meticulously collected and reviewed coverage from mainstream media, including both government and non-government media.
Many barrier dam datasets have been established by compiling significant historical events. Although they have included a limited number of DFBDs, with the related information being incomprehensive, they have provided us with a wealth of clues that facilitate the collection of information on DFBDs. These datasets are one of the main data sources in this study (Table 1). We conducted a rigorous screening of barrier dams in the existing dataset and further supplemented and refined the information related to the screened DFBDs. Furthermore, the majority of the cases in our dataset were sourced from individual studies of DFBD events from various regions.
2.2 Dataset content
The statistical principles used for data collection are outlined here. We collected all DFBDs if they had clear records of the country and location in the literature and media reports. Therefore, it can be considered to be the case that the data collection process is free of subjective bias and that the dataset has statistical significance. The descriptions were classified and organized into six categories: basic information, debris flow characteristics, dam characteristics, lake characteristics, failure characteristics, and climate characteristics. These categories included 38 attributes to characterize DFBDs, as detailed in Table 2.
-
The basic information includes the name, country, longitude and latitude, date of formation, trigger, reference, and reliability.
-
The debris flow characteristics include the debris flow channel slope gradient, debris flow channel length, debris flow gully basin area, debris flow density, debris flow velocity, debris flow peak discharge, and debris flow volume.
-
The dam characteristics include the blockage modes, dam volume, dam height, dam length, dam width, dam material, longevity, stability, and controls.
-
The lake characteristics include lake length, lake area, and lake volume.
-
The failure characteristics include failure mechanism, breach depth, breach top width, breach bottom width, breaching time, peak discharge, average discharge, and loss of life.
-
The climate characteristics include precipitation and temperature.
Detailed explanations for each attribute are provided in Table 2, which can be referred to for further information.
Incorporating relevant information on debris flows and climate into this dataset can provide a more comprehensive record of detailed information on DFBD events. In addition, these categories provide convenience for potential users, enabling them to cross-validate and compare this dataset with other datasets, such as that outlined in “Two multi-temporal datasets to track debris flow after the 2008 Wenchuan earthquake” (Wang et al., 2022) and the ITAlian rainfall-induced LandslIdes CAtalogue (Peruccacci et al., 2023). This cross-application and mutual corroboration enhances the reliability of data and the universality of application.
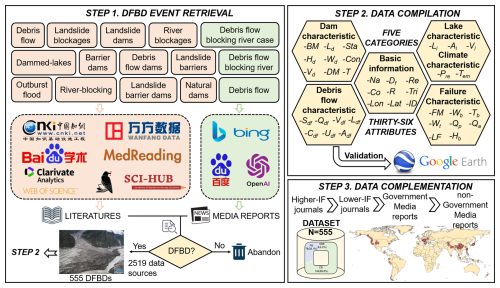
Figure 3Procedure for the compilation of the dataset. The logos of CNKI, Wanfang Data, Baidu Scholar, MedReading, Web of Science, Sci-Hub, Baidu, Microsoft Bing, OpenAI, and Google Earth displayed in the figure are trademarks and are copyrighted content of their respective companies. The use of these logos in this paper is solely for the purpose of illustrating research tools and data sources, falls under fair use principles, and is for non-commercial purposes. The photo used for step 1, sourced from https://www.news.cn (last access: 15 February 2025), depicted the scene of the Sedongpu Gully debris flow blocking the river on 17 October 2018. For step 3, the base map of the world was sourced from GADM (https://gadm.org, last access: 15 February 2025).
2.3 Data-processing procedure
We followed a meticulously designed data-processing procedure: first, we conducted a comprehensive search for DFBD events; second, we reviewed and validated key data; and, lastly, we carried out data complementation (Fig. 3). Throughout each step, we adhered to strict standards to ensure that all data included in the dataset underwent a thorough review to eliminate potential biases and errors.
In the first step, we were committed to extensively collecting DFBD events, carefully reviewing a wide range of academic literature and media reports in both Chinese and other languages. The collection of Chinese literature mainly relied on online service platforms such as CNKI, Wanfang Data, Baidu Scholar, and MedReading. For non-Chinese literature, our search encompassed international academic databases such as Google Scholar and Web of Science, complemented by other reputable academic resources. It is important to note that the term barrier dams can vary depending on the authors, regions, and research focuses, leading to the use of different terms in parallel. Common synonyms identified in the literature include landslide blockages, landslide dams, stream or river blockages, natural dams, landslide barriers, and dammed lakes. To identify DFBDs as comprehensively as possible, we used a range of keywords, including debris flow, landslide blockages, landslide dams, river blockages, natural dams, landslide barriers, dammed lakes, barrier dams, debris flow dams, landslide barrier dams, outburst floods, and river blocking for event search. We also combined these with regional and national terms, such as Chinese debris flow barrier dams, to improve the relevance of the search. To ensure search consistency and minimize errors, we used unified search keywords for both Chinese and non-Chinese literature. In addition, we inputted keywords such as debris flow blocking river, debris flow dam incident, and debris flow into search engines such as Baidu and Google, as well as ChatGPT, and combined regional information to retrieve relevant dam events. Among the identified barrier dam cases, those of DFBDs were selected and included in this dataset, and the term debris flow barrier dam (DFBD) was adopted. We repeated this step multiple times between 2022 and 2024 to ensure that any updated DFBD events are included in our dataset.
In the second step, we conducted a thorough review and validation of the information gathered from the literature and media reports. It is important to note that we applied different validation methods to process various attributes of DFBDs, as detailed below.
-
DFBD-ID. We organized the DFBDs by the first letter of their names.
-
Name. When compiling this attribute, we strictly followed the original names of DFBDs as documented in the data sources. These names are typically derived from the channels of the debris flows or the rivers that were obstructed. It is worth noting that a DFBD may have different names in different data sources. To avoid information redundancy and confusion, we have carefully verified the DFBDs using Google Earth based on geographical coordinates and the date of formation. We have identified and removed the DFBD events that were reported repeatedly in different data sources due to naming differences, ensuring that each event is uniquely named and recorded only once in our dataset.
-
Country. When country information was reported, it was verified through Google Earth. When errors were identified, we used the inquiry results from Google Earth. When data sources lacked clear country information, we determined the event's country using geographic coordinates or prominent landmarks with Google Earth. This process ensures the accuracy and reliability of the country information recorded in our dataset, thereby enhancing the overall quality of the data.
-
Longitude and latitude. When determining and verifying the longitude and latitude information, we followed the procedures outlined below.
- 1.
The following is for cases where the data sources provided latitude and longitude information:
- a.
When the data sources included latitude and longitude information and when corresponding imagery was available on Google Earth, we verified these coordinates through the platform. If discrepancies existed between the latitude and longitude provided by the data sources and the results provided by Google Earth, we prioritized the Google Earth data. This is because Google Earth offers continuously updated satellite imagery and geographic data, while manually recorded literature and news reports may contain inaccuracies or biases. The automated data collection and processing capabilities of Google Earth help mitigate the risk of such human errors.
- b.
For the events with a long history, we cannot locate imagery on Google Earth, and so we depended on the geographical coordinates reported in the data source.
- a.
- 2.
The following is for cases where the data sources did not provide latitude and longitude information:
- a.
If the corresponding remote sensing imagery was accessible, we located the landmarks described in the data sources on Google Earth. We then compared the imagery from before and after the formation date of the DFBD to determine its geographical coordinates.
- b.
If no corresponding remote sensing imagery was available, we did not record any geographical-coordinate information.
- a.
- 1.
-
Date of formation. We obtained the formation dates of DFBDs by referring to the literature or news reports and primarily used Google Earth for verification.
- 1.
The following is for cases where the formation dates were recorded in the literature or news reports:
- a.
When corresponding Google Earth imagery was available, we used Google Earth to verify the formation dates. If the formation dates provided in the literature or news reports were consistent with the Google Earth imagery, we considered this information to be reliable and included the formation dates in our dataset; if there was a discrepancy between the formation dates provided in the data sources and the information from Google Earth, we believed it was not feasible to accurately determine the formation dates and, therefore, did not record them.
- b.
However, for some events that date back a long time (for example, DFBDs formed between 1800 and 1900), Google Earth did not provide relevant imagery. In such cases, we relied on the available literature or news reports to determine the formation dates.
- a.
- 2.
If the date of formation was not recorded in the literature or news reports, our dataset would not include the formation date information.
- 1.
-
Trigger, debris flow channel slope gradient, debris flow channel length, debris flow gully basin area, debris flow density, debris flow velocity, debris flow peak discharge, debris flow volume, dam volume, dam height, dam length, dam width, dam material, controls, lake length, lake area, lake volume, failure mechanism, breach depth, breach bottom width, breach top width, breaching time, peak discharge, average discharge, loss of life, and reference. These attribute data were derived from reports from different data sources. If there was conflicting information among different data sources, we proceeded to the third step of data complementation.
-
Precipitation and temperature. Fick and Hijmans (2017) established a global historical climate dataset, which was updated in January 2020. Their dataset includes monthly average precipitation and temperature data from 1970 to 2000, with a spatial resolution of 30 s (approximately 1 km2). In our study, for DFBDs formed between 1970 and 2000, we extracted the corresponding precipitation and temperature data from the dataset by Fick and Hijmans (2017) and associated these data with the respective DFBD cases. For DFBDs formed outside the period of 1970 to 2000, we did not include the precipitation and temperature data.
-
Blockage mode. Costa and Schuster (1988) proposed six blockage modes: types I, II, III, IV, V, and VI. In this dataset, we reclassified the DFBD blockage modes based on event descriptions from the data sources. We classified type I as partial blockage; types II, III, IV, and V as complete blockage; and type VI as submerged-dam blockage (Fig. 2b). This classification method is helpful to more precisely record and understand the different blocking characteristics of DFBDs.
-
Longevity. We divided the DFBDs from different data sources into two types: (1) dams that have been clearly reported to have failed and (2) dams that have not been clearly reported to have failed. For the former, we included the longevity according to the report. For the second type, according to the latitude and longitude information, we used Google Earth to view the latest remote sensing images to confirm the current status of the dams. If a dam was no longer present, we compared historical remote sensing images across different time periods to determine its existence duration (i.e., longevity).
-
Stability. We evaluated the stability of DFBDs based on their real-time status. When the literature and media reports indicated that dams had failed, we classified them as unstable dams. On the contrary, if the data sources claimed that the dams still existed, we used Google Earth to further confirm their current status. Once Google Earth images showed that they still existed, we classified them as stable dams; if they were not found or were confirmed to no longer exist, they were classified as unstable dams.
-
Reference. We kept detailed records of data sources to ensure traceability and transparency of information. For academic literature, we detailed key information such as the publisher, date of publication, title, authors' names, and DOIs. For media reports, we also meticulously recorded the URL links of the reports so that users could directly access the original reports.
-
Reliability. We determined the credibility of DFBD events based on the number of data sources. When there was only a single literature or news report on a DFBD, we classified the reliability of this event as low. When a DFBD event was reported by two data sources, the reliability of this event was classified as medium. When an event was reported by three or more data sources, we considered the reliability of the event to be high.
The third step is data complementation. In the situations where there is conflicting information among different data sources, we adopted a hierarchy of information sources based on perceived reliability with regard to resolving the issue: priority was given to literature published in journals with higher-impact factors as these data have undergone peer review and are of high reliability and authoritativeness; next were publications in journals with lower-impact factors; and, finally, we referred to news reports published on official government websites, which are accurate and timely due to their official certification. In very few cases, when there were no data from the above sources, we referred to reports from non-government media. In our dataset, the number of cases obtained from non-government media is minimal, accounting for less than 1 % of the total. According to this priority rule, we incorporated the conflicting information into our dataset to ensure the accuracy and reliability of the data.
2.4 Data analysis tools
During the construction and analysis of this dataset, we employed a variety of tools to enhance work efficiency and to ensure data accuracy. First, we rigorously validated the data using Google Earth and preserved all intermediate files generated from remote sensing imagery. These files, stored in KMZ format, have been uploaded as supplementary materials to facilitate future reference and verification. Additionally, we utilized ArcMap 10.8 software to extract temperature and precipitation data and completed the relevant charting tasks. In the data-processing phase, we primarily used Excel for data organization and analysis and employed Origin software to create clear and accurate data charts that intuitively present our research findings.
3.1 Reliability
Based on an in-depth review of 2519 literature and news reports, we have recorded 555 DFBD events. To evaluate the reliability of these events, we have introduced the key attribute of reliability. According to our analysis, out of the 555 DFBD events, a total of 494 have high reliability, accounting for 89 % of the total. In addition, there are 48 events with medium reliability, making up 8.7 % of the total, and there are only 13 events with low reliability, accounting for only 2.3 % (Fig. 4).
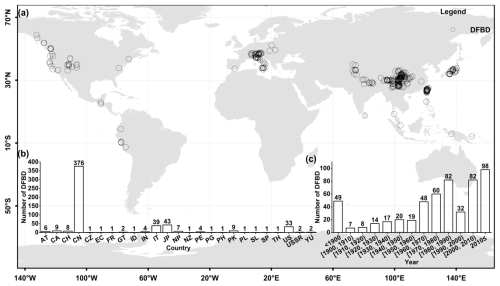
Figure 5Worldwide DFBD spatiotemporal distribution. (a) Spatial distribution, (b) the number of DFBDs in each country, and (c) temporal distribution. In Fig. 5a, the base map of the world was sourced from GADM (https://gadm.org, last access: 15 February 2025).
3.2 Spatiotemporal distribution of the DFBDs
The 555 DFBDs lie in different countries, including 39 dams in Italy, 43 dams in Japan, 376 dams in China, 33 dams in the United States, and a total of 64 dams in other countries (Fig. 5a and b).
Since the 1900s, the number of global DFBDs has shown an overall upward trend. Between the 1900s and 1960s, the number experienced fluctuations and increases, but the growth range was relatively small (Fig. 5c). During this period, society's awareness of and attention to such disasters were insufficient, resulting in limited records and reports. Between the 1960s and 1990s, the number of DFBDs showed a more significant increase. However, between the 1990s and 2000s, the number of reported DFBDs worldwide decreased significantly (approximately 1.5 times) compared to the previous decade. Since 2000, the number of DFBDs has risen significantly, peaking in the last decade. Global climate change may be one of the key factors leading to an increase in debris flows (Ma et al., 2024; Sharma et al., 2024; Yu et al., 2021). With the rise in global temperatures, extreme weather events such as heavy rainfall, droughts, and floods have become more frequent. These extreme weather conditions are highly likely to induce the formation of debris flows and the blocking of rivers to form dams.
The number of Chinese DFBDs in the dataset is significantly high, which can mainly be attributed to the following reasons. (1) The first is active geological activity. China is located at the junction of multiple tectonic plates, with complex geological structures and active neotectonic movements, leading to frequent earthquakes. Earthquakes cause rock fragmentation and mountain loosening, producing a large amount of loose soil and stone and providing a rich source of material for the formation of debris flows. For example, after the 2008 Wenchuan earthquake, a large number of debris flow dam events occurred in the earthquake-affected area and its surroundings (Fan et al., 2012a, b, 2017, 2019; Shi et al., 2015). (2) Next is diverse climatic conditions. China has a rich variety of climate types, with a prominent monsoon climate and concentrated rainfall, often in the form of heavy storms. In some mountainous areas, intense rainfall over a short period can rapidly increase surface runoff, carrying a large amount of silt, rocks, and other materials to form debris flows. Additionally, in high-altitude glacial regions, the melting of glaciers and snow due to rising temperatures in summer can also provide ample water sources for debris flows, promoting the formation of debris flow and DFBDs. (3) Finally, there is complex topography and geomorphology. China has a vast mountainous area with significant terrain undulations, crisscrossing valleys, and notable elevation differences. In particular, in the western and southwestern regions, such as at the edges of the Tibetan Plateau (Jiang et al., 2022; Zhou et al., 2024) and in the Hengduan Mountains (Zhou et al., 2022), the high mountains and deep valleys, with steep slopes and rapid streams, provide favorable topographical conditions for the formation of DFBDs (Fig. 6a). A large amount of loose solid material tends to accumulate in valleys, and, once triggered by an appropriate water source, it is easy to form debris flows that can dam rivers and create DFBDs. Although other countries like Japan frequently experience debris flows, there are few topographical conditions, such as deep valleys and high reliefs, that are conducive to the formation of debris flow dams; therefore, there are fewer DFBDs in Japan.
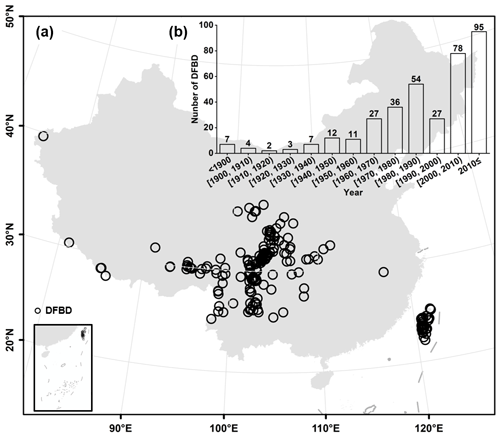
Figure 6Chinese DFBD spatiotemporal distribution. (a) Spatial distribution and (b) temporal distribution. In (a), the base map was sourced from GADM (https://gadm.org, last access: 15 February 2025). Publisher's remark: please note that the above figure contains disputed territories.
Since the early 1960s, China has vigorously promoted railway and highway construction, but mountain disasters such as debris flows have grown to be increasingly prominent. In response to these challenges, the Chinese Academy of Sciences established the Institute of Glaciology and Cryopedology in Lanzhou and the Institute of Mountain Hazards and Environment in Chengdu to conduct systematic research on debris flow disasters. The establishment of these institutes marked the beginning of Chinese scholars' focus on the phenomenon of debris flows blocking rivers. In particular, the 2008 Wenchuan earthquake triggered many debris flows, further exacerbating the formation of DFBDs. These events not only had a huge impact on the local area but also elevated research and prevention efforts to an unprecedented level. After the Wenchuan earthquake, Chinese scholars have intensified research and prevention efforts with regard to DFBDs, leading to a significant increase in reported DFBD cases (Fig. 6b).
3.3 DFBD blockage modes and failure mechanisms
The identification of DFBD blockage modes is helpful in predicting the river-blocking situation. It also enhances our understanding of the formation mechanisms of DFBDs and provides a scientific basis for their prevention and management (Chen et al., 2019; Dang et al., 2009; Yu et al., 2022).
This dataset recorded blockage modes for 278 DFBDs. Among these cases, 194 dams (69.8 %) exhibited complete blockage, 78 dams (28.1 %) exhibited partial blockage, and only 6 dams (2.2 %) exhibited submerged-dam blockage (Fig. 7b). Figure 7b demonstrates that complete blockage is the most prevalent river-blocking mode caused by debris flows. Notably, DFBDs with submerged-blockage modes are highly concealed and difficult to detect through direct observation. This suggests potential underreporting of such cases, indicating that the actual number of submerged dams may be underestimated.
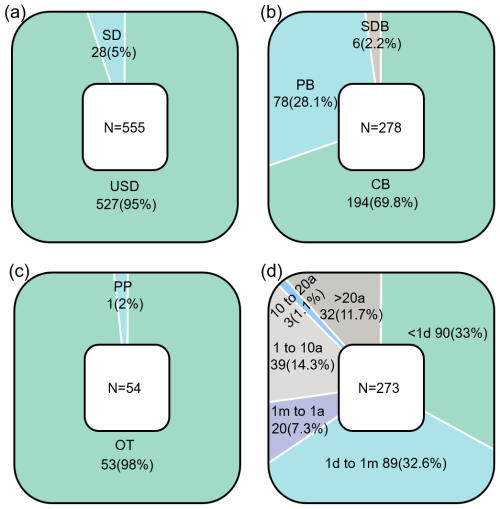
Figure 7The stability, blockage modes, failure mechanisms, and longevity of DFBDs. (a) Stability, (b) blockage modes, (c) failure mechanisms, and (d) longevity. Notation: in Fig. 7a, SD means stable dam, and USD means unstable dam; in Fig. 7b, CB means complete blockage, PB means partial blockage, and SDB means submerged-dam blockage; in Fig. 7c, OT means overtopping, and PP means piping.
We compiled the failure mechanisms of 54 DFBDs, among which the overtopping (OT) accounts for an overwhelming 98 % (Fig. 7c). There is only one case for piping (PP). The fluidity of debris flows inherently limits the height of DFBDs but increases their widths. This results in DFBDs with smaller upstream and downstream slopes (Ruan et al., 2021). Additionally, the DFBDs are almost completely saturated, and the soil of the dams has strong water retention. This is especially pronounced in barrier dams formed by viscous debris flows. Therefore, there are no cases of slope failure in our dataset. On the other hand, before the debris flow merges into the main river, the solid-phase–liquid-phase materials inside it have been fully mixed after long-distance transportation. The high content of fine particles results in no obvious connected pores or seepage channels inside the DFBD. Even if seepage occurs, the long seepage channel (due to the large dam width) makes it difficult to form a complete seepage channel in a short time. Therefore, the probability of PP in DFBDs is relatively low.
3.4 Stability and longevity
Current empirical classification schemes for barrier dam stability, developed by Ermini and Casagli (2003), Korup (2004), and Tacconi Stefanelli et al. (2016), can be traced back to the original definition by Casagli and Ermini (1999). This initial concept was limited to barrier dams that had either catastrophically failed or remained intact. In these studies, stability refers to the state of the dam and the dammed lake at the time of inspection without considering the length of time the dam has remained intact, i.e., its longevity. According to this definition, a barrier dam is considered to be stable if the dammed lake is still present or has been filled with sediments during the analysis. The latter scenario implies that the dam was capable of holding back the lake water (either by maintaining an inflow–outflow balance through seepage or spillway flow) and enabled continuous sediment deposition in the lake until it was silted up. Conversely, dams classified as unstable have experienced catastrophic breaching. Evidence of such includes deep gullies; an impoundment with little sediment; erosional signs in the remaining sediments, suggesting rapid water drawdown; and flood-deposited sediments downstream (Fan et al., 2020).
Out of 555 DFBDs, only 28 dams still exist, indicating that the stability of DFBDs is relatively poor (Fig. 7a). Moreover, all the existing dams exhibit complete-blockage modes, suggesting that DFBDs with partial- or submerged-blockage modes are unstable.
As illustrated in Fig. 7d, the DFBDs tend to fail within a brief period. A total of 33 % of the dams failed within 1 d, and 32.6 % of the dams failed within 1 d to a month. About 73 % of DFBDs have a longevity of less than a year. Due to the generally lower height of DFBDs (Ruan et al., 2021), the reservoir behind the dam is more likely to reach full capacity quickly. In addition, DFBDs exhibit high rheological activity and poor overall structural integrity (Iverson, 1997). The surface of the dam has a weaker ability to resist overflow erosion. Once the water reaches the top of the dam, it can quickly erode the DFBD materials. Consequently, DFBDs have relatively short longevity.
DFBDs are characterized by rapid outbursts and require close monitoring and attention. The formation of DFBDs and their subsequent rapid failure can trigger a series of secondary disasters, which often cause significant economic losses and casualties. According to our statistics, at least 5255 deaths are attributed to the 47 DFBD events recorded in this dataset. However, events with known casualties represent only 8.5 % of the total DFBD events. This suggests that the actual global death toll could be significantly higher than currently estimated, possibly exceeding our imagination.
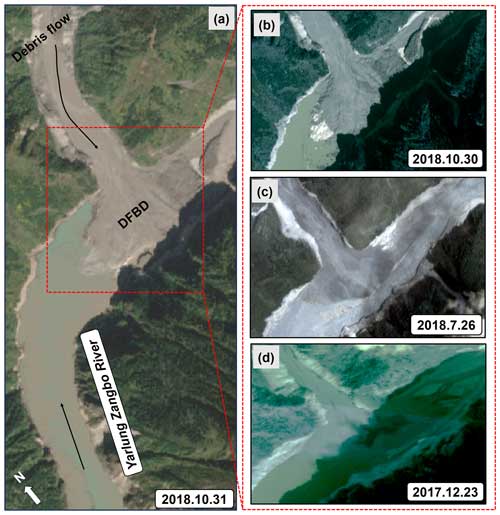
Figure 8Repeated DFBDs due to glacial debris flows generated in the Sedongpu catchment, upper Yarlung Zangbo, eastern Tibet. (a) Remote sensing image after the events on 17 and 29 October 2018 (31 October 2018), (b) remote sensing image after the event of 17 October 2018 (30 October 2018), (c) remote sensing image of 26 July 2018, and (d) remote sensing image after the event of 22 December 2017 (23 December 2017). The remote sensing image in (a) is from Sentinel-2 (https://dataspace.copernicus.eu/, last access: 6 April 2025), and those in (b–d) are sourced from PlanetScope (https://www.planet.com/, last access: 6 April 2025).
3.5 The phenomenon of repeated river blockage
Some debris flow gullies, due to the presence of a large amount of loose material within their basins, have repeatedly experienced debris flows triggered by factors such as rainfall, causing river blockages (Hu et al., 2019; Zhang et al., 2022b). In addition, after a debris flow event, a significant amount of material on the slopes along the gully remains in a loosely cemented state, which can easily be remobilized into the main channel by heavy rainfall, leading to multiple river blockages and dam formations (Wang et al., 2022). The repeated formation of DFBDs significantly increases their hazard potential. The hazards associated with DFBDs are mainly manifested in four ways: (1) upstream inundation caused by DFBDs (Hu et al., 2022; Rizzo et al., 2023; Wang et al., 2015); (2) downstream abnormal flood disasters caused by DFBD failure (Takayama et al., 2021; Veh et al., 2020; Yang et al., 2022); (3) sedimentation in downstream river channels caused by the outflow or failure of the DFBD, leading to riverbed aggradation and reduced flood conveyance capacity of the river channels (Cao et al., 2011; Jiang et al., 2021; Vázquez-Tarrío et al., 2024); and (4) the high risk of the residual dam material transforming into debris flows under heavy rainfall after the DFBD has released its impounded water (Chen et al., 2022).
It is a common phenomenon for debris flows to occur multiple times in the same channel and to form multiple DFBDs. For example, from 2017 to 2018, at least four debris flows occurred in the Sedongpu basin, upstream of the Yarlung Zangbo River in eastern Tibet, China, which repeatedly blocked the Yarlung Zangbo River (Tong et al., 2018; Zhang et al., 2022b) (Fig. 8). On 21 December 2017, a glacial debris flow erupted in the Sedongpu Valley, blocking the Yarlung Zangbo River (Fig. 8d). The DFBD was breached 3 d later. On 26 July 2018, the Yarlung Zangbo River experienced another temporary blockage. The resulting barrier lake was not large and posed no serious threat (Fig. 8c). However, on 17 October 2018, a disaster chain composed of an ice-rock avalanche and glacial debris flow formed a DFBD (Fig. 8a and b). This DFBD impounded a massive barrier lake with a volume of approximately 0.605 × 109 m3, and the maximum water depth in front of the dam was about 79.43 m (Jin, 2019). The DFBD was breached 3 d later, but there was still a narrow spillway, and the possibility of re-blockage remained. Therefore, a small-scale glacier debris flow on 29 October 2018 caused further blockage of the river, forming a DFBD with a volume of 0.326 × 109 m3 and a maximum depth of approximately 0.77 m (Fig. 8a and b). The two DFBDs in 2018 posed a serious threat to the upstream village of Gala.
According to the records in this dataset, 555 DFBDs predominantly occurred in 426 different gullies or river channels. It is particularly noteworthy that 63 gullies, exhibiting high activity, have experienced multiple debris flows and formed 192 DFBDs. This phenomenon reveals an important issue: for the channels that are in an active debris flow phase, timely engineering measures should be taken to prevent repeated river blockages and DFBD formation.
4.1 Applicability of LD stability models to DFBDs
Due to the limitations of investigation conditions and the risks associated with dam failure, it is difficult to obtain the geological, physical, and mechanical parameters of barrier dams. Nonetheless, the geometric characteristics of dams can be obtained in time through satellite optical images or UAV aerial photography. Therefore, it is of great practical significance to use geometric parameters to quickly evaluate and predict the stability of barrier dams (Table 3) as this can provide timely decision support for emergency response.
It should be pointed out that the stability models based on morphological parameters in Table 3 ignore the category of barrier dams and primarily focus on LD cases. However, Ruan et al. (2021) highlighted that DFBDs differ significantly from LDs. To date, no specific models or criteria have been developed to assess the stability of DFBDs. When LD stability models (Table 2) are applied to analyze DFBD stability, can they effectively evaluate it? Which model is more suitable for the stability analysis of DFBDs? This issue remains unaddressed, but further investigation is essential.
Since certain parameters are missing for some cases in the dataset, the number of samples used for each model varies. Additionally, studies and regions adopt inconsistent definitions and criteria for dam stability, leading to the development of diverse stability assessment models. LD stability models, due to inconsistencies in their parameters and definitions, introduce significant ambiguity in barrier dam stability assessments. This study determines dam stability based on real-time dam status. To ensure consistency in stability criteria, all models validated in Table 3 rely on real-time status indicators.
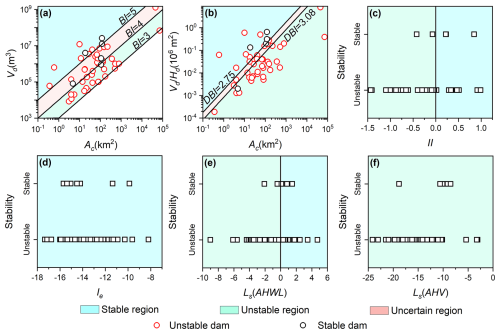
Figure 9Stability calculation results. (a) Results of BI model, (b) results of DBI model, (c) results of II model, (d) results of Ie model, (e) results of Ls (AHWL) model, and (f) results of Ls (AHV) model.
Following Zhong and Shan (2019), the performance of each evaluation method was compared and analyzed using three metrics: misjudgment rate (F), conservative accuracy rate (Rc), and absolute accuracy rate (R). The misjudgment rate F is defined as the probability that a DFBD is truly unstable but is misclassified as stable by the model. In practical applications, models with lower misjudgment rates should be prioritized. The absolute accuracy rate R refers to the probability of exact agreement between the dam's actual stability status and the model's prediction. The conservative accuracy rate Rc is the probability of the dam being truly stable but erroneously classified as unstable, combined with the absolute accuracy rate R. The calculation results are shown in Fig. 9 and Table 4.
According to Fig. 9 and Table 4, the absolute accuracy rates R of the BI and Ie models are relatively low (BI: 51.02 %; Ie: 19.15 %), suggesting that these models are unsuitable for assessing DFBD stability. The DBI, II, and Ls (AHWL) models exhibit high absolute accuracy rates R and conservative accuracy rates Rc, both exceeding 70 %. Among the three models, the DBI model demonstrates the lowest misjudgment rate (F = 16 %), indicating its superior applicability in relation to DFBDs compared to the others. The Ls (AHV) model achieves the highest absolute accuracy rate R, the highest conservative accuracy rate Rc, and the lowest misjudgment rate F. Based on these findings, the Ls (AHV) model is recommended as the primary choice for DFBD stability evaluation, with the DBI model being a secondary option. The BI, II, and Ie models are not recommended for use.
The stability of a dam inherently depends on its own characteristics (Ashraf et al., 2021; Costa and Schuster, 1988; Latrubesse et al., 2020), including its geotechnical properties (Fan et al., 2020; Pisaniello et al., 2015; Schuster, 2000). The empirical models are often parameter models derived from historical statistical cases, which have been limited in number and have often failed to cover all the types, all the geographical environments, and all the formation conditions of barrier dams. Barrier dams in different regions and with different causes have their own unique characteristics. For example, LDs and DFBDs differ significantly in terms of both material structure and formation mechanisms. Therefore, the predictive validity of the BI, II, and Ie models is significantly reduced in these contexts.
We propose that barrier dams should be meticulously categorized based on their formation mechanisms, and the existing dataset should be expanded through additional case studies. This aligns with the primary objective of establishing this dataset.
4.2 Applicability of LD peak-discharge models to DFBDs
As a critical parameter in dam breach analysis, peak discharge serves as a prerequisite for barrier dam risk assessment and downstream flood routing simulations, directly determining the severity of downstream disasters (Stuart-Smith et al., 2021; Zhong et al., 2021). Therefore, accurately and rapidly predicting the outburst–flow peak discharge following barrier dam formation is critically important (Bazai et al., 2021; Dubey and Goyal, 2020; Vilca et al., 2021).
Table 5Empirical models used for LD peak-discharge prediction.
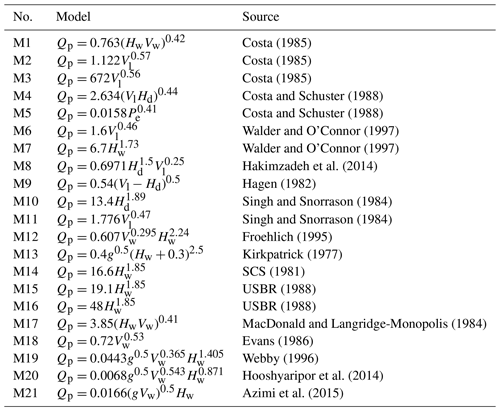
Note that Hw is the depth of the breach (m), Vw is the released water volume (m3), Vl is the volume of the barrier lake (m3), Hd is the dam height (m), Pe is the potential energy of the waterbody, and g is the acceleration of gravity (m s−2).
The peak discharge depends on the failure mechanism and characteristics of the dam itself (Costa and Schuster, 1988; Latrubesse et al., 2020), such as its geotechnical properties (Pisaniello et al., 2015; Schuster, 2000). However, it is difficult to obtain the complete parameters required for the calculation of the dam break dynamic models in a short time. The empirical models derived from historical statistical cases have been widely used. Table 5 lists the empirical models applied to predict the peak discharge of LD breaches worldwide. It should be noted that the models in Table 5 are based on specific barrier dam cases, predominantly LDs. These empirical models do not distinguish the type of barrier dam and have good applicability to LDs. No peak-discharge models currently exist for DFBDs.
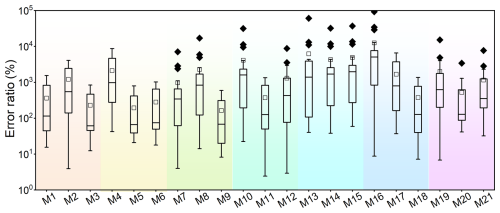
Figure 10Error ratio (ER) of peak discharge calculated from the different models in Table 5, where , Pv is the predicted value, and Av is the actual value.
Figure 10 shows that 21 LD peak-discharge models exhibit poor applicability to DFBDs, with calculated values consistently overestimating actual DFBD peak discharges. Consequently, existing empirical peak-discharge models should be applied cautiously for DFBD predictions.
The peak-discharge models in Table 5 were derived from the statistics of historical events. Their sample sizes were limited, and they ignored the failure mechanism and the geotechnical properties of dams while not strictly distinguishing between different types of barrier dams. As a result, their prediction accuracy is affected by the region and the type of dam; therefore, the models in Table 5 are not suitable for predicting the peak discharge of DFBDs (Fig. 10). Establishing a peak-discharge model suitable for DFBDs is a key issue to be solved in the future. This dataset can provide rich cases and basic data to help solve this problem.
4.3 Comparison with barrier dam datasets
Some studies have established datasets of barrier dams through the collation and reconstruction of historical events. These datasets contain a large number of barrier dams. For example, Tacconi Stefanelli et al. (2016) summarized 300 Italian barrier dams through field investigations, air photo interpretation, and estimation based on historical and bibliographic information. Schuster and Costa (1986) established the first dataset containing 187 barrier dams worldwide by reviewing the literature from various regions. Fan et al. (2020) compiled a comprehensive dataset encompassing 1887 dams by integrating various datasets.
Compared with other dam datasets, ours contains only 555 DFBDs, a relatively small number. However, other datasets primarily focus on LDs, with limited coverage of DFBDs. Our dataset is highly targeted, only focusing on DFBDs. Additionally, some datasets are obtained by summarizing other datasets, while our dataset places greater emphasis on the review and validation of raw data rather than being a simple summary of other datasets. The DFBDs in this dataset are mostly derived from case studies scattered across various regions (Dang et al., 2009; Wei et al., 2018; Yin et al., 2016), which requires us to extensively review original literature rather than merely relying on superficial dataset compilation, thus avoiding the errors that might arise from a simple dataset aggregation. For example, during the data collection process, we identified a common issue where some individual case study documents confused the concepts of dam length and width (for instance, Tian et al., 2023). After correcting these errors, we included the correct data for dam length and width in the dataset. Furthermore, this paper integrates data from different sources to provide a comprehensive perspective, precisely describing the characteristics of DFBDs with 38 attributes. This dataset is the first of its kind dedicated to DFBDs.
Costa and Schuster (1988) proposed one of the most widely accepted classifications of barrier dam blockage modes, which relates the dam's shape and size to the valley it blocks. However, some scholars have conducted a geomorphological classification of DFBD blockage modes based on hydrodynamics, dam size, and the width of the main valley, identifying three modes: submerged-dam blockage, partial blockage, and complete blockage (Fig. 2). This classification criterion is more in line with the characteristics of DFBDs (Chen et al., 2019; Dang et al., 2009; Yu et al., 2022; Zou et al., 2020). The classification criteria of Costa and Schuster (1988) may be more applicable to LDs. However, other datasets still use the classification criteria of Costa and Schuster (1988) to categorize DFBD blockage modes, leading to confusion with regard to terminology and inconsistency in the criteria of subsequent research. Therefore, this dataset has re-identified the blocking patterns of DFBDs.
The stability of a barrier dam is a dynamic process. Scholars have defined this stability from diverse perspectives: Korup (2004) defined stability based on dam longevity, proposing that a barrier dam is stable if its lake persists for over a decade. Liao et al. (2022) and Xu (2020) proposed that a dam without breaching within 1 year can be classified as stable. However, some scholars have defined the stability of a barrier dam from the perspective of the dam's real-time condition, considering it by means of an instantaneous definition. When specifically analyzing a barrier dam, if the barrier lake still exists or has been filled due to the accumulation of gravel and sediment, it can be considered to be stable (Casagli and Ermini, 1999; Ermini and Casagli, 2003; Tacconi Stefanelli et al., 2016). It is evident that there is considerable divergence in the understanding of the stability of barrier dams. This divergence not only leads to confusion with regard to the different stability criteria being applied to different dams within the same datasets; it also poses significant challenges to the research on the stability of barrier dams. Based on the real-time status being used to assess the stability of the dams, it is possible to differentiate between barrier dams that have failed and those that have not failed. Therefore, our dataset judges the stability based on the real-time status of the DFBDs, re-evaluates, and compiles the stability of all DFBDs.
4.4 Limitations in this work
While this dataset offers valuable data, it acknowledges certain limitations with regard to specific aspects. Firstly, the dataset contains some ancient events, and the authenticity of the historical records may be difficult to review fully, especially when it comes to details such as the geometric characteristics of DFBDs. Additionally, some attribute information regarding the DFBDs is still lacking in completeness, such as the data on the failure characteristics.
In addition, we acknowledge that our dataset does not encompass all DFBD events. The number, 555 dams, seems unreasonably low for a “worldwide” scale, which may be attributed to the following reasons: (1) in the process of data collection, it is inevitable that some literature or reports might be missing and that some unreported events may not be included. (2) It is obvious that only large-scale debris flows have the potential to dam rivers. However, the number of debris flow events is smaller than that of other types of landslides with the same magnitude. (3) Current research on barrier dams focuses more on LDs, with less attention being given to DFBDs (see Table 1), hence the limited availability of literature we could consult. (4) Due to their poor stability (Fig. 7a) and short-lived existence (Fig. 7d), many DFBDs quickly disappeared, and it is difficult to detect and record them timeously. (5) Finally, for the records of early debris flow disasters, people paid more attention to the influences on human lives and infrastructure while lacking a sufficient understanding of and attention to the blockage of river channels by debris flows. As a result, such events were often overlooked in historical records, leading to a seemingly smaller number when viewed from the perspective of historical data statistics.
In this dataset, the number of DFBDs in China is significantly higher than that in other countries and regions, which may be attributed to the fact that China's active geological activity, diverse climatic conditions, and complex topographic and geomorphologic conditions are more conducive to the formation of DFBDs (see Sect. 3.2 for details). However, we cannot rule out the possibility that the spatial distribution of DFBDs in this dataset may be biased. In our efforts to create a global DFBD dataset, we encountered challenges that are common in the data collection process, which may contribute to such biases. For instance, the recording and reporting of DFBD events can vary by region, influenced by local research focuses, data recording practices, and the availability of scientific resources. Furthermore, access to DFBD event data in some countries may be restricted due to data privacy policies, language barriers, or a lack of digitization. The diversity of languages in global literature and reports adds complexity to data collection, particularly when extracting information from non-English sources. Additionally, different countries and regions may employ varying standards and definitions for DFBD events, complicating data comparison and integration. Our team's geographical and resource acquisition advantages facilitate the collection of a greater number of Chinese DFBD cases.
The objective of this study is to amass and catalog DFBD events and their related information as comprehensively as possible, with the aim of establishing a global DFBD dataset which serves as a valuable repository of data and providing a multidimensional perspective for DFBD research. Currently, this dataset represents a preliminary attempt, and while it has its limitations, it is relatively comprehensive and well-documented. We have laid a foundational framework, and, in future work, we plan to refine this dataset from two perspectives. First, we plan to interpret the geometric characteristics of the DFBDs and lakes using remote sensing imagery. Second, we aim to uncover unreported DFBDs through field investigations. Additionally, we look forward to and welcome active participation from experts and contributors in various fields to jointly promote the continuous improvement and expansion of the dataset through interdisciplinary collaboration and the integration of multi-source data.
The data can be freely downloaded via Zenodo at https://doi.org/10.5281/zenodo.14766647 (Cheng et al., 2025).
In this study, we systematically reviewed 2519 high-quality literature sources and media reports, identifying 555 global DFBD events spanning 1800–2023. This effort resulted in the first comprehensive DFBD dataset, advancing the field significantly. Our dataset described the characteristics of DFBDs using 6 categories and 38 attributes, including basic information (latitude and longitude, etc.), debris flow characteristics (debris flow velocity, debris flow peak discharge, etc.), dam characteristics (dam height, dam volume, etc.), lake characteristics (lake area, lake volume, etc.), failure characteristics (peak discharge, loss of life, etc.), and climate characteristics (precipitation and temperature). We rigorously reviewed and verified this information via Google Earth and developed a conflict resolution method for multi-source data discrepancies. Considering the current lack of unified standards for distinguishing river-blocking modes and the confusion surrounding stability concepts, this dataset reassessed and reintegrated river-blocking modes and the stability of DFBDs. Finally, based on the data included in this dataset, the applicability of LD stability models and peak-discharge models to DFBDs was discussed. Key conclusions are as follows:
-
Since the 1960s, the number of DFBDs has increased rapidly, which may be related to global climate degradation. Moreover, compared with other countries and regions, China has a larger number of DFBDs.
-
The most common blockage mode for DFBDs is complete blockage (69.8 %), and the most common failure mechanism is overtopping (98 %). In addition, DFBDs tend to have relatively poor stability, with about 73 % of DFBDs failing within 1 year after formation.
-
Repeated river-blocking events are common: 15 % of rivers experienced multiple debris flows, forming 192 DFBDs (35 % of the total).
-
The Ls (AHV) model and the DBI model perform well in the stability assessment of DFBDs. However, the peak-discharge models of LDs are not suitable for DFBDs.
Compared with other barrier dam datasets, although this dataset does not have an obvious advantage in the number of cases, our dataset is the first of its kind dedicated to DFBDs. We place special emphasis on the unification of terminology and concepts, as well as on the review of raw data, to ensure data consistency and accuracy. We believe that this dataset can provide a rich set of foundational data for studies related to debris flow river blocking and enhance our understanding of DFBDs. Of course, there are still some limitations that require further improvement. We will continue to improve upon and update this dataset in future work.
The supplement related to this article is available online at https://doi.org/10.5194/essd-17-1573-2025-supplement.
Conceptualization: KH. Methodology: KH and HC. Validation: XZ, HL, and QZ. Formal analysis: KH, SL, and HC. Data curation: KH and HC. Writing (original draft preparation): HC. Writing (review and editing): KH, SL, HC, MRG, PL, LN, AY, LW, and JL. Visualization: HC and PZ. Supervision: KH. Funding acquisition: KH.
The contact author has declared that none of the authors has any competing interests.
Publisher's note: Copernicus Publications remains neutral with regard to jurisdictional claims made in the text, published maps, institutional affiliations, or any other geographical representation in this paper. While Copernicus Publications makes every effort to include appropriate place names, the final responsibility lies with the authors.
The authors would like to acknowledge the support from the Dongchuan Debris Flow Observation and Research Station (DDFORS) of the Chinese Academy of Sciences. We sincerely thank the handling topic editor, Yuanzhi Yao; the handling chief editor, Hanqin Tian; the reviewer, Thanh-Nhan-Duc Tran; and the two anonymous reviewers for their valuable time and insightful comments.
This work was jointly supported by the Second Tibetan Plateau Scientific Expedition and Research Program (grant no. 2019QZKK0902), the Key R&D Program of Xizang Autonomous Region (grant no. XZ202301ZY0039G), the Sichuan Science and Technology Program (grant no. 2024YFFK0108), the National Natural Science Foundation of China (grant no. 52409109), and the Science and Technology Research Program of the Institute of Mountain Hazards and Environment, Chinese Academy of Sciences (grant no. IMHE-ZDRW-01).
This paper was edited by Yuanzhi Yao and reviewed by Thanh-Nhan-Duc Tran and two anonymous referees.
Ashraf, A., Iqbal, M. B., Mustafa, N., Naz, R., and Ahmad, B.: Prevalent risk of glacial lake outburst flood hazard in the Hindu Kush–Karakoram–Himalaya region of Pakistan, Environ. Earth Sci., 80, 451, https://doi.org/10.1007/s12665-021-09740-1, 2021.
Azimi, R., Vatankhah, A. R., and Kouchakzadeh, S.: Predicting peak discharge from breached embankment dams, in: the 36th IAHR World Congress, 28 June–3 July 2015, Hague, Netherlands, https://www.researchgate.net/publication/279845430_PREDICTING_PEAK_DISCHARGE_FROM_BREACHED_EMBANKMENT_DAMS (last access: 6 April 2025), 2015.
Bazai, N. A., Cui, P., Carling, P. A., Wang, H., Hassan, J., Liu, D., Zhang, G., and Jin, W.: Increasing glacial lake outburst flood hazard in response to surge glaciers in the Karakoram, Earth-Sci. Rev., 212, 103432, https://doi.org/10.1016/j.earscirev.2020.103432, 2021.
Cao, Z., Yue, Z., and Pender, G.: Landslide dam failure and flood hydraulics. Part II: coupled mathematical modelling, Nat. Hazards, 59, 1021–1045, https://doi.org/10.1007/s11069-011-9815-7, 2011.
Casagli, N. and Ermini, L.: Geomorphic analysis of landslide dams in the Northern Apennine, Transactions of the Japanese Geomorphological Union, 20, 219–249, https://flore.unifi.it/handle/2158/206201 (last access: 6 April 2025), 1999.
Casagli, N., Ermini, L., and Rosati, G.: Determining grain size distribution of the material composing landslide dams in the Northern Apennines: sampling and processing methods, Eng. Geol., 69, 83–97, https://doi.org/10.1016/S0013-7952(02)00249-1, 2003.
Chai, H. J., Liu, H. C., and Zhang, Z. Y.: The catalog of Chinese landslide dam events, Journal of Geological Hazards and Environment Preservation, 6, 1–9, https://kns.cnki.net/kcms2/article/abstract?v=bYx4kmIyqoUE5l (last access: 6 April 2025), 1995 (in Chinese).
Chen, H., Ruan, H., Chen, J., Li, X., and Yu, Y.: Review of investigations on hazard chains triggered by river blocking debris flows and dam break floods, Front. Earth Sci., 10, 830044, https://doi.org/10.3389/feart.2022.830044, 2022.
Chen, K. T., Chen, X. Q., Niu, Z. P., and Guo, X. J.: Early identification of river blocking induced by tributary debris flow based on dimensionless volume index, Landslides, 16, 2335–2352, https://doi.org/10.1007/s10346-019-01221-8, 2019.
Cheng, H. G., Hu, K. H., Liu, S., Zhang, X. P., Li, H., Zhang, Q. Y., Ning, L., Manish, R. G., Li, P., Yang, A. N., Liu, J. Y., and Wei, L.: A worldwide event-based debris-flow barrier dam dataset from 1800 to the 2023, Zenodo [Data set], https://doi.org/10.5281/zenodo.14766647, 2025.
Cheng, Z. L., Dang, C., Liu, J. J., and Gong, Y. W.: Experiments of debris flow damming in Southeast Tibet, Earth Science Frontiers, 14, 181–185, https://doi.org/10.1016/S1872-5791(08)60010-X, 2007a.
Cheng, Z. L., Geng, X. Y., Dang, C., and Liu, J. J.: Modeling experiment of break of debris-flow dam, Wuhan University Journal of Natural Sciences, 12, 588–594, https://doi.org/10.1007/s11859-006-0302-z, 2007b.
Chong, Y., Chen, G., Meng, X., Yang, Y., Shi, W., Bian, S., Zhang, Y., and Yue, D.: Quantitative analysis of artificial dam failure effects on debris flows – A case study of the Zhouqu '8.8' debris flow in northwestern China, Sci. Total Environ., 792, 148439, https://doi.org/10.1016/j.scitotenv.2021.148439, 2021.
Costa, J. E.: Floods from dam failures, Open File Rep. 85-560, USGS, https://doi.org/10.3133/ofr85560, 1985.
Costa, J. E. and Schuster, R. L.: The formation and failure of natural dams, Geol. Soc. Am. Bull., 100, 1054–1068, https://doi.org/10.1130/0016-7606(1988)100<1054:TFAFON>2.3.CO;2, 1988.
Costa, J. E. and Schuster, R. L.: Documented historical landslide dams from around the world, Vancouver, WA, Open File Rep. 91-239, USGS, https://doi.org/10.3133/ofr91239, 1991.
Cui, P., Lei, Y., Hu, K., Zhou, G. G. D., Zhu, X., and Chen, H.: Amplification mechanism and hazard analysis for Zhouqu giant debris flow, International Journal of Erosion Control Engineering, 9, 71–79, https://doi.org/10.13101/ijece.9.71, 2016.
Dang, C., Cui, P., and Cheng, Z. L.: The formation and failure of debris flow-dams, background, key factors and model tests: case studies from China, Environ. Geol., 57, 1901–1910, https://doi.org/10.1007/s00254-008-1479-6, 2009.
Dong, J. J., Tung, Y. H., Chen, C. C., Liao, J. J., and Pan, Y. W.: Logistic regression model for predicting the failure probability of a landslide dam, Eng. Geol., 117, 52–61, https://doi.org/10.1016/j.enggeo.2010.10.004, 2011.
Dong, J. J., Lai, P. J., Chang, C. P., Yang, S. H., Yeh, K. C., Liao, J. J., and Pan, Y. W.: Deriving land-slide dam geometry from remote sensing images for the rapid assessment of critical parameters related to dam-breach hazards, Landslides, 11, 93–105, https://doi.org/10.1007/s10346-012-0375-z, 2014.
Dubey, S. and Goyal, M. K.: Glacial lake outburst flood hazard, downstream impact, and risk over the Indian Himalayas, Water Resour. Res., 56, e2019WR026533, https://doi.org/10.1029/2019WR026533, 2020.
Ermini, L. and Casagli, N.: Prediction of the behaviour of landslide dams using a geomorphological dimensionless index, Earth Surf. Proc. Land., 28, 31–47, https://doi.org/10.1002/esp.424, 2003.
Evans, S. G.: The maximum discharge of outburst floods caused by the breaching of man-made and natural dams, Can. Geotech. J., 23, 385–387, https://doi.org/10.1139/t86-053, 1986.
Fan, X. M., van Westen, C. J., Xu, Q., Gorum, T., and Dai, F.: Analysis of landslide dams induced by the 2008 Wenchuan earthquake, J. Asian Earth Sci., 57, 25–37, https://doi.org/10.1016/j.jseaes.2012.06.002, 2012a.
Fan, X., van Westen, C. J., Korup, O., Gorum, T., Xu, Q., Dai, F., Huang, R., and Wang, G.: Transient water and sediment storage of the decaying landslide dams induced by the 2008 Wenchuan earthquake, China, Geomorphology, 171–172, 58–68, https://doi.org/10.1016/j.geomorph.2012.05.003, 2012b.
Fan, X., Xu, Q., van Westen, C. J., Huang, R., and Tang, R.: Characteristics and classification of landslide dams associated with the 2008 Wenchuan earthquake, Geoenvironmental Disasters, 4, 12, https://doi.org/10.1186/s40677-017-0079-8, 2017.
Fan, X., Scaringi, G., Domènech, G., Yang, F., Guo, X., Dai, L., He, C., Xu, Q., and Huang, R.: Two multi-temporal datasets that track the enhanced landsliding after the 2008 Wenchuan earthquake, Earth Syst. Sci. Data, 11, 35–55, https://doi.org/10.5194/essd-11-35-2019, 2019.
Fan, X., Dufresne, A., Siva Subramanian, S., Strom, A., Hermanns, R., Tacconi Stefanelli, C., Hewitt, K., Yunus, A. P., Dunning, S., Capra, L., Geertsema, M., Miller, B., Casagli, N., Jansen, J. D., and Xu, Q.: The formation and impact of landslide dams – State of the art, Earth-Sci. Rev., 203, 103116, https://doi.org/10.1016/j.earscirev.2020.103116, 2020.
Fick, S. E. and Hijmans, R. J.: WorldClim 2: new 1-km spatial resolution climate surfaces for global land areas, Int. J. Climatol., 37, 4302–4315, https://doi.org/10.1002/joc.5086, 2017.
Froehlich, D. C.: Peak outflow from breached embankment dam, Journal of Water Resources Planning and Management, 121, 90–97, https://doi.org/10.1061/(ASCE)0733-9496(1995)121:1(90), 1995.
Gouli, M. R., Hu, K. H., Khadka, N., Liu, S., Shu, Y. F., Adhikari, M., and Talchabhadel, R.: Quantitative assessment of the GLOF risk along China–Nepal transboundary basins by integrating remote sensing, machine learning, and hydrodynamic model, Int. J. Disast. Risk Re., 118, 105231, https://doi.org/10.1016/j.ijdrr.2025.105231, 2025.
Hagen, V. K.: Re-evaluation of design and dam safety, in: 14th International Commission on Large Dams Congress, 3–7 May 1982, Rio de Janerio, 475–491, https://www.researchgate.net/publication/288007025_Re-evaluation_of_design_floods_and_dam_safety (last access: 6 April 2025), 1982.
Hakimzadeh, H., Nourani, V., and Amini, A. B.: Genetic programming simulation of dam breach hydrograph and peak outflow discharge, J. Hydrol. Eng., 19, 757–768, https://doi.org/10.1061/(ASCE)HE.1943-5584.0000849, 2014.
Hooshyaripor, F., Tahershamsi, A., and Golian, S.: Application of copula method and neural networks for predicting peak outflow from breached embankments, J. Hydro-Environ. Res., 8, 292–303, https://doi.org/10.1016/j.jher.2013.11.004, 2014.
Hu, K., Zhang, X., You, Y., Hu, X., Liu, W., and Li, Y.: Landslides and dammed lakes triggered by the 2017 Ms6.9 Milin earthquake in the Tsangpo gorge, Landslides, 16, 993–1001, https://doi.org/10.1007/s10346-019-01168-w, 2019.
Hu, K., Zhang, X., Gouli, M. R., Liu, S., and Nie, Y.: Retrospective analysis and hazard assessment of Gega glacial lake in the eastern Himalayan syntaxis, Natural Hazards Research, 2, 331–342, https://doi.org/10.1016/j.nhres.2022.11.003, 2022.
Hu, K. H., Ge, Y., Cui, P., Guo, X. J., and Yang, W.: Preliminary analysis of extra-large-scale debris flow disaster in Zhouqu County of Gansu Province, Mountain Research, 28, 628–634, https://doi.org/10.16089/j.cnki.1008-2786.2010.05.012, 2010.
Hu, K. H., Wei, F. Q., and Li, Y.: Real-time measurement and preliminary analysis of debris-flow impact force at Jiangjia Ravine, China, Earth Surf. Proc. Land., 36, 9, 1268–1278, https://doi.org/10.1002/esp.2155, 2011.
Hungr, O., Leroueil, S., and Picarelli, L.: The Varnes classification of landslide types, an update, Landslides, 11, 167–194, https://doi.org/10.1007/s10346-013-0436-y, 2014.
Iverson, R. M.: The physics of debris flows, Rev. Geophys., 35, 245–296, https://doi.org/10.1029/97RG00426, 1997.
Jiang, H., Zou, Q., Zhou, B., Hu, Z., Li, C., Yao, S., and Yao, H.: Susceptibility assessment of debris flows coupled with ecohydrological activation in the eastern Qinghai-Tibet Plateau, Remote Sens.-Basel, 14, 1444, https://doi.org/10.3390/rs14061444, 2022.
Jiang, X., Cheng, H., Gao, L., and Liu, W.: The formation and geometry characteristics of boulder bars due to outburst floods triggered by overtopped landslide dam failure, Earth Surf. Dynam., 9, 1263–1277, https://doi.org/10.5194/esurf-9-1263-2021, 2021.
Jin, X.: Review and reflections on emergency response countermeasures for barrier lakes in Jinsha river and Yarlung Zangbo river, Yangtze River, 50, 5–9, https://doi.org/10.16232/j.cnki.1001-4179.2019.03.002, 2019 (in Chinese).
Kirkpatrick, G. W.: Guidelines for evaluating spillway capacity, Water Power Dam Constr., 29, 29–33, http://pascal-francis.inist.fr/vibad/index.php?action=getRecordDetail&idt=PASCAL7780430034 (last access: 6 April 2025), 1977.
Korup, O.: Geomorphometric characteristics of New Zealand landslide dams, Eng. Geol., 73, 13–35, https://doi.org/10.1016/j.enggeo.2003.11.003, 2004.
Latrubesse, E. M., Park, E., Sieh, K., Dang, T. D., Lin, Y. N., and Yun, S.: Dam failure and a catastrophic flood in the Mekong basin (Bolaven Plateau), southern Laos, 2018, Geomorphology, 362, 107221, https://doi.org/10.1016/j.geomorph.2020.107221, 2020.
Liao, H. M., Yang, X. G., Lu, G. D., Tao, J., and Zhou, J. W.: A geotechnical index for landslide dam stability assessment, Geomat. Nat. Haz. Risk, 13, 854–876, https://doi.org/10.1080/19475705.2022.2048906, 2022.
Liu, J., You, Y., Chen, X., Liu, J., and Chen, X.: Characteristics and hazard prediction of large-scale debris flow of Xiaojia Gully in Yingxiu Town, Sichuan Province, China, Eng. Geol., 180, 55–67, https://doi.org/10.1016/j.enggeo.2014.03.017, 2014.
Liu, W., Carling, P. A., Hu, K., Wang, H., Zhou, Z., Zhou, L., Liu, D., Lai, Z., and Zhang, X.: Outburst floods in China: A review, Earth-Sci. Rev., 197, 102895, https://doi.org/10.1016/j.earscirev.2019.102895, 2019.
Ma, C., Chen, Y., Hu, K., Du, C., Dong, J., and Lyu, L. Q.: Climate warming triggered a glacial lake outburst flood and debris flow events in an Alpine Watershed, Western Himalayas, Tibet Plateau, B. Eng. Geol. Environ., 83, 201, https://doi.org/10.1007/s10064-024-03706-w, 2024.
MacDonald, T. C. and Langridge-Monopolis, J.: Breaching characteristics of dam failures, J. Hydraul. Eng., 110, 567–586, https://doi.org/10.1061/(ASCE)0733-9429(1984)110:5(567), 1984.
Peng, M. and Zhang, L. M.: Breaching parameters of landslide dams, Landslides, 9, 13–31, https://doi.org/10.1007/s10346-011-0271-y, 2012a.
Peng, M. and Zhang, L. M.: Analysis of human risks due to dam break floods-part 2: application to Tangjiashan landslide dam failure, Nat. Hazards, 64, 1899–1923, https://doi.org/10.1007/s11069-012-0336-9, 2012b.
Peruccacci, S., Gariano, S. L., Melillo, M., Solimano, M., Guzzetti, F., and Brunetti, M. T.: The ITAlian rainfall-induced LandslIdes CAtalogue, an extensive and accurate spatio-temporal catalogue of rainfall-induced landslides in Italy, Earth Syst. Sci. Data, 15, 2863–2877, https://doi.org/10.5194/essd-15-2863-2023, 2023.
Pisaniello, J. D., Dam, T. T., and Tingey-Holyoak, J. L.: International small dam safety assurance policy benchmarks to avoid dam failure flood disasters in developing countries, J. Hydrol., 531, 1141–1153, https://doi.org/10.1016/j.jhydrol.2015.09.077, 2015.
Rizzo, C., Maranzoni, A., and D'Oria, M.: Probabilistic mapping and sensitivity assessment of dam-break flood hazard, Hydrolog. Sci. J., 68, 700–718, https://doi.org/10.1080/02626667.2023.2174026, 2023.
Ruan, H., Chen, H., Li, Y., Chen, J. G., and Li, H., B.: Study on the downcutting rate of a debris flow dam based on grain-size distribution, Geomorphology, 391, 107891, https://doi.org/10.1016/j.geomorph.2021.107891, 2021.
Schuster, R. L.: Dams built on pre-existing landslides, in: GeoEng 2000 – Geotechnical and Geological Engineering: International Society for Rock Mechanics and Rock Engineering, 19–24 November 2000, Melbourne, Australia, 1537–1589, https://pubs.usgs.gov/publication/70206221 (last access: 7 April 2025), 2000.
Schuster, R. L. and Costa, J. E.: Landslide dams: processes, risk, and mitigation, Amer Society of Civil Engineers, New York, 164 pp., ISBN 0-87262-524-9, 1986.
Sharma, A., Sajjad, H., Roshani, and Rahaman, M. H.: A systematic review for assessing the impact of climate change on landslides: research gaps and directions for future research, Spatial Information Research, 32, 165–185, https://doi.org/10.1007/s41324-023-00551-z, 2024.
Shi, Z. M., Wang, Y. Q., Peng, M., Chen, J. F., and Yuan, J.: Characteristics of the landslide dams induced by the 2008 Wenchuan earthquake and dynamic behavior analysis using large-scale shaking table tests, Eng. Geol., 194, 25–37, https://doi.org/10.1016/j.enggeo.2014.10.009, 2015.
Singh, K. P. and Snorrason, A.: Sensitivity of outflow peaks and flood stages to the selection of dam breach parameters and simulation models, J. Hydrol., 68, 295–310, https://doi.org/10.1016/0022-1694(84)90217-8, 1984.
SCS – Soil Conservation Service: Simplified dam-breach routing procedure, U. S. Dept. of Agriculture, Washington, D.C., https://directives.nrcs.usda.gov/sites/default/files2/1720451567/TR-210-66, Simplified Dam-Breach Routing Procedure.pdf (last access: 6 April 2025), 1981.
Song, Z., Fan, G., Chen, Y., and Liu, D.: Identification method of river blocking by debris flow in the middle reaches of the Dadu River, Southwest of China, Water, 15, 4301, https://doi.org/10.3390/w15244301, 2023.
Stuart-Smith, R. F., Roe, G. H., Li, S. J., and Allen, M. R.: Increased outburst flood hazard from Lake Palcacocha due to human-induced glacier retreat, Nat. Geosci., 14, 85–90, https://doi.org/10.1038/s41561-021-00686-4, 2021.
Tacconi Stefanelli, C., Catani, F., and Casagli, N.: Geomorphological investigations on landslide dams, Geoenvironmental Disasters, 2, 21, https://doi.org/10.1186/s40677-015-0030-9, 2015.
Tacconi Stefanelli, C., Segoni, S., Casagli, N., and Catani, F.: Geomorphic indexing of landslide dams evolution, Eng. Geol., 208, 1–10, https://doi.org/10.1016/j.enggeo.2016.04.024, 2016.
Takayama, S., Miyata, S., Fujimoto, M., and Satofuka, Y.: Numerical simulation method for predicting a flood hydrograph due to progressive failure of a landslide dam, Landslides, 18, 3655–3670, https://doi.org/10.1007/s10346-021-01712-7, 2021.
USBR – The United States Bureau of Reclamation: Downstream hazard classification guidelines, ACER Technical Memorandum No. 11, U. S. Bureau of Reclamation, US Department of the Interior, Denver, https://damtoolbox.org/wiki/Downstream_Hazard_Classification_Guidelines_(ACER_TM_11) (last access: 7 February 2025), 1988.
Tian, Y., Jiang, L., and Guo, J.: Numerical simulation of glacier avalanche-river blocking-outburst in Sedongpu gully of the Yarlung Zangbo River, J. Geol., 47, 196–202, 2023 (in Chinese).
Tong, L., Tu, J., Pei, L., Guo, Z., Zheng, X., Fan, J., Zhong, C., Liu, C., Wang, S., He, P., and Chen, H.: Preliminary discussion of the frequently debris flow events in Sedongpu basin at Gyalaperi peak, Yarlung Zangbo river, Journal of Engineering Geology, 26, 1552-1561, https://doi.org/10.13544/j.cnki.jeg.2018-401, 2018 (in Chinese).
Tong, Y. X.: Quantitative analysis for stability of landslide dams, MS thesis, National Central University, Taiwan, https://ncu.primo.exlibrisgroup.com/discovery/search?vid=886UST_NCU:886UST_NCU (last access: 7 April 2025), 2008 (in Chinese).
Vázquez-Tarrío, D., Ruiz-Villanueva, V., Garrote, J., Benito, G., Calle, M., Lucía, A., and Díez-Herrero, A.: Effects of sediment transport on flood hazards: Lessons learned and remaining challenges, Geomorphology, 446, 108976, https://doi.org/10.1016/j.geomorph.2023.108976, 2024.
Veh, G., Korup, O., and Walz, A.: Hazard from Himalayan glacier lake outburst floods, P. Natl. Acad. Sci. USA, 117, 907–912, https://doi.org/10.1073/pnas.1914898117, 2020.
Vilca, O., Mergili, M., Emmer, A., Frey, H., and Huggel, C.: The 2020 glacial lake outburst flood process chain at Lake Salkantaycocha (Cordillera Vilcabamba, Peru), Landslides, 18, 2211–2223, https://doi.org/10.1007/s10346-021-01670-0, 2021.
Walder, J. and O'Connor, J.: Methods for predicting peak discharge of floods caused by failure of natural and constructed dams, Water Resour. Res., 33, 2337–2348, https://doi.org/10.1029/97WR01616, 1997.
Wang, L., Chang, M., Dou, X., Ma, G., and Yang, C.: Analysis of river blocking induced by a debris flow, Geofluids, 2017, 1268135, https://doi.org/10.1155/2017/1268135, 2017.
Wang, L., Chang, M., Le, J., and Zhang, N.: Two multi-temporal datasets to track debris flow after the 2008 Wenchuan earthquake, Scientific Data, 9, 525, https://doi.org/10.1038/s41597-022-01658-y, 2022.
Wang, S., Qin, D., and Xiao, C.: Moraine-dammed lake distribution and outburst flood risk in the Chinese Himalaya, J. Glaciol., 61, 115–126, https://doi.org/10.3189/2015JoG14J097, 2015.
Wang, Z., Hu, K., and Liu, S.: Classification and sediment estimation for debris flow-prone catchments in the Parlung Zangbo Basin on the southeastern Tibet, Geomorphology, 413, 108348, https://doi.org/10.1016/j.geomorph.2022.108348, 2022.
Webby, M. G.: Discussion of “Peak Outflow from Breached Embankment Dam” by David C. Froehlich, J. Water Res. Pl., 122, 316–317, https://doi.org/10.1061/(ASCE)0733-9496(1996)122:4(316), 1996.
Wei, R., Zeng, Q., Davies, T., and Yin, Q.: Geohazard cascade and mechanism of large debris flows in Tianmo gully, SE Tibetan Plateau and implications to hazard monitoring, Eng. Geol., 233, 172–182, https://doi.org/10.1016/j.enggeo.2017.12.013, 2018.
Wu, H., Shan, Z. G., Ni, W. D., and Wang, K. J.: Study on the application of rapid evaluation model of landslide dam stability, Journal of Engineering Geology, 29, 135–143, https://doi.org/10.13544/j.cnki.jeg.2021-0464, 2021 (in Chinese).
Xu, F.: A rapid evaluation model of the stability of landslide dam, Journal of Natural Disasters, 29, 54–63, https://doi.org/10.13577/j.jnd.2020.0206, 2020 (in Chinese).
Xu, Y. and Zhang, L. M.: Breaching Parameters for earth and rockfill dams, J. Geotech. Geoenviron. Eng., 135, 1957–1970, https://doi.org/10.1061/(ASCE)GT.1943-5606.0000162, 2009.
Yan, R.: Secondary disaster and environmental effect of landslides and collapsed dams in the upper reaches of Minjiang River, Master thesis, Sichuan University, https://kns.cnki.net/kcms2/article/abstract?v=bYx4kmIyqoVeZP (last access: 6 April 2025), 2006.
Yang, A., Wang, H., Liu, W., Hu, K., Liu, D., Wu, C., and Hu, X.: Two megafloods in the middle reach of Yarlung Tsangpo River since Last-glacial period: Evidence from giant bars, Global Planet. Change, 208, 103726, https://doi.org/10.1016/j.gloplacha.2021.103726, 2022.
Yin, Y., Cheng, Y., Liang, J., and Wang, W.: Heavy-rainfall-induced catastrophic rockslide-debris flow at Sanxicun, Dujiangyan, after the Wenchuan Ms 8.0 earthquake, Landslides, 13, 9–23, https://doi.org/10.1007/s10346-015-0554-9, 2016.
Yu, B., Yang, C., and Yu, M.: Experimental study on the critical condition of river blockage by a viscous debris flow, Catena, 213, 106198, https://doi.org/10.1016/j.catena.2022.106198, 2022.
Yu, G. A., Yao, W., Huang, H. Q., and Liu, Z.: Debris flows originating in the mountain cryosphere under a changing climate: A review, Prog. Phys. Geog., 45, 339–374, 2021.
Zhang, Q., Hu, K., Wei, L., and Liu, W.: Rapid changes in fluvial morphology in response to the high-energy Yigong outburst flood in 2000: Integrating channel dynamics and flood hydraulics, J. Hydrol., 612, 128199, https://doi.org/10.1016/j.jhydrol.2022.128199, 2022a.
Zhang, X. P., Hu, K. H., Liu, S., Nie, Y., and Han, Y.: Comprehensive interpretation of the Sedongpu glacier-related mass flows in the eastern Himalayan syntaxis, J. Mt. Sci., 19, 1672–6316, 2022b.
Zhong, Q. M. and Shan, Y. B.: Comparison of rapid evaluation methods for barrier dam's stability, Yangtze River, 50, 20–24+64, https://doi.org/10.16232/j.cnki.1001-4179.2019.04.004, 2019.
Zhong, Q. M., Wang, L., Chen, S., Chen, Z. Y., Shan, Y. B., Zhang, Q., Ren, Q., Mei, S. Y., Jiang, J. D., Hu, L., and Liu, J. X.: Breaches of embankment and landslide dams – State of the art review, Earth-Sci. Rev., 12, 103597, https://doi.org/10.1016/j.earscirev.2021.103597, 2021.
Zhou, Y., Yue, D., Liang, G., Li, S., Zhao, Y., Chao, Z., and Meng, X.: Risk assessment of debris flow in a mountain-basin area, Western China, Remote Sens.-Basel, 14, 2942, https://doi.org/10.3390/rs14122942, 2022.
Zhou, Y., Hu, X., Xi, C., Wen, H., Cao, X., Jin, T., Zhou, R., Zhang, Y., and Gong, X.: Glacial debris flow susceptibility mapping based on combined models in the Parlung Tsangpo Basin, China, J. Mt. Sci., 21, 1231–1245, https://doi.org/10.1007/s11629-023-8500-0, 2024.
Zou, Q., Cui, P., Jiang, H., Wang, J., Li, C., and Zhou, B.: Analysis of regional river blocking by debris flows in response to climate change, Sci. Total Environ., 741, 140262, https://doi.org/10.1016/j.scitotenv.2020.140262, 2020.