the Creative Commons Attribution 4.0 License.
the Creative Commons Attribution 4.0 License.
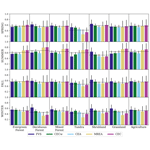
Observational partitioning of water and CO2 fluxes at National Ecological Observatory Network (NEON) sites: a 5-year dataset of soil and plant components for spatial and temporal analysis
Einara Zahn
Long-term time series of transpiration, evaporation, plant net photosynthesis, and soil respiration are essential for addressing numerous research questions related to ecosystem functioning. However, quantifying these fluxes is challenging due to the lack of reliable and direct measurement techniques, which has left gaps in the understanding of their temporal cycles and spatial variability. To help address this open challenge, we generated a dataset of these four components by implementing five (conventional and novel) approaches to partition total evapotranspiration (ET) and CO2 fluxes into plant and soil fluxes across 47 National Ecological Observatory Network (NEON) sites. The final dataset (https://doi.org/10.5281/zenodo.12191876; Zahn and Bou-Zeid, 2024) spans a 5-year period and covers various ecosystems, including forests, grasslands, and agricultural terrain. This is the first comprehensive dataset covering such a wide spatial and temporal distribution. Overall, we observed good agreement across most methods for ET components, increasing confidence in these estimates. Partitioning of CO2 components, on the other hand, was found to be less robust and more dependent on prior knowledge of water use efficiency. This highlights some limitations of these present methods that we discuss, emphasizing the broader challenge posed by the lack of an accurate reference method to validate against. Despite these limitations, this dataset has several potential applications, especially in addressing critical questions regarding the response of ecosystems to extreme weather events, which are expected to become more severe and frequent with climate change.
- Article
(3734 KB) - Full-text XML
-
Supplement
(424 KB) - BibTeX
- EndNote
Plant transpiration and photosynthesis are important components of the global water and CO2 cycles. At the ecosystem level, these quantities reflect the complex interactions within the soil–vegetation–atmosphere continuum. Given the complexity of these interactions and the challenges associated with measuring or modeling leaf–atmosphere exchanges, many open questions remain. For instance, the competing effects of rising CO2 and temperature on plant water use efficiency (Mengis et al., 2015; Kirschbaum and McMillan, 2018; Dusenge et al., 2019; Baslam et al., 2020; Wang et al., 2022) are still being debated, together with their response and resilience to more frequent and intense droughts and changes in soil moisture conditions (Maxwell and Condon, 2016; Lesk et al., 2021). In addition to the challenges of investigating such nonlinear processes, one of the main barriers to advancing research in this area has been the lack of long-term observations and/or reliable estimates of plant transpiration and CO2 assimilation over various types of vegetation and soils.
Eddy covariance (EC) is a reliable approach to continuously monitor evapotranspiration (ET) and net CO2 (Fc) fluxes across ecosystems in a standardized manner. However, separate measurement of the individual ecosystem components, i.e., plant transpiration (T) and soil evaporation (E), together with plant gross primary production (GPP) and ecosystem respiration (Reco), is still difficult. Traditional measurement techniques, such as sap flow and lysimeters, often lack the temporal and spatial resolutions necessary for capturing ET flux components comprehensively (Kool et al., 2014; Stoy et al., 2019). Other techniques, such as soil chambers (Kool et al., 2014), stable isotopes (Good et al., 2014), and carbonyl sulfide as a tracer for plant fluxes (Wohlfahrt et al., 2011), are not yet deployable at large spatial and temporal scales. Additionally, all of these methods carry their own uncertainties, can be logistically challenging to implement, and are often costly to scale up. Partitioning of CO2 fluxes into GPP and Reco can also be done based on nighttime CO2 flux extrapolation (Reichstein et al., 2005) and light-response curves (Lasslop et al., 2010); this method has been widely implemented and is part of FLUXNET products (Pastorello et al., 2020). Nonetheless, it cannot partition ET components. As a result, there is a scarcity of datasets representing the temporal and spatial variabilities of both ET and CO2 flux components across a broad range of ecosystems and weather conditions.
An alternative approach to obtain long-term time series of flux components is partitioning of evapotranspiration and net CO2 exchanges into their respective plant and soil components based strictly on the analysis of their turbulent perturbation signals. This approach is the focus of this paper, which applies five recently developed and tested partitioning methods to disentangle the contributions of transpiration, evaporation, net photosynthesis, and soil respiration to the total ecosystem fluxes measured across all the EC towers of the National Science Foundation's National Ecological Observatory Network (NEON). The methods employed in this study have been rigorously evaluated in previous studies that combined experimental data from EC sites and virtual EC data from large-eddy simulations. Although, as with all partitioning approaches, the validation is hindered by a lack of a universally accepted accurate standard method, previous research has highlighted conditions under which these turbulence methods agree and are more reliable, together with conditions under which their partitioning estimates may have larger errors. In the dataset we produce in this work, we consolidate the results from five partitioning methods and calculate their ensemble average, providing a measure of uncertainty for each flux period at each site.
With a total of 47 sites spanning diverse ecosystems in the United States, this dataset can be an invaluable resource for future investigation of the spatial variability and ecological and meteoclimatic drivers shaping flux dynamics across different landscapes. It has the potential to contribute to the advancement of our understanding of ecosystem processes and their responses to current and future climates, in turn better informing ecosystem management and climate change mitigation and adaptation practices.
NEON is a comprehensive initiative designed to collect long-term open-access environmental observations in the continental United States (Metzger et al., 2019). It operates a network of field sites strategically located to capture the diversity of ecosystems and environmental conditions present in the country. One of the main features of NEON is the standardized collection of data across sites, including biological, hydrological, and meteorological observations. As part of its effort to understand land–atmosphere exchanges of energy, water, and CO2, NEON has a total of 47 eddy-covariance towers spread across 24 states (see Table 1), where most sites are located in deciduous and evergreen forests (Fig. 1).
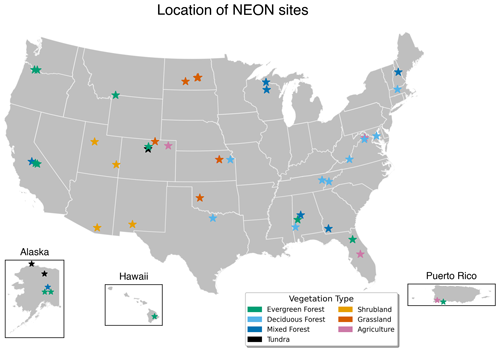
Figure 1Locations of the 47 eddy-covariance towers maintained by NEON. Note that Alaska, Hawaii, and Puerto Rico are not scaled proportionally to the continental US area.
Table 1Description of the 47 sites included in the present paper. In addition to the NEON site identification (ID) and state, the table lists the geographical coordinates, the ratio between the eddy-covariance measurement height and the mean canopy height (), and the predominant vegetation type around the tower following Fiorella et al. (2021). The last two columns represent the availability of ET fluxes during the daytime hours (when solar radiation > 10 W m2) in the 5 years of record and the availability of transpiration estimates, based on the partitioning approaches used here, in the same period. The range represents the availability according to the different methods (least available to most available). Availability is computed after gap-filling as described in Sect. 3.4.2.
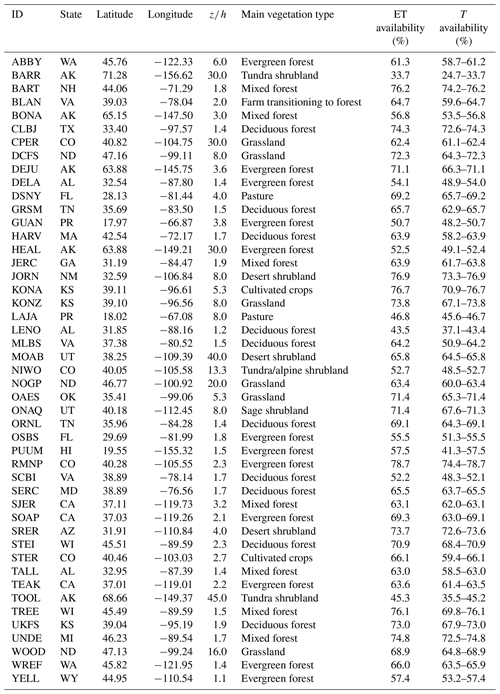
For the purpose of implementing the partitioning methods, we downloaded instantaneous measurements from the eddy-covariance towers from 2019 to 2023 for all 47 locations. All sites were equipped with the same gas analyzer (LICOR LI7200) and sonic anemometers (Campbell Scientific, CSAT-3 3-D) collecting data at a frequency of 20 Hz. These time series were processed and prepared to be used by the five partitioning methods, as described in the next section. In addition to raw data, we also downloaded the processed eddy-covariance data (product level 4) for the same period (NEON, 2024a, b), which include evapotranspiration and net ecosystem CO2 fluxes as processed by eddy4R (Metzger et al., 2017). Fluxes computed by eddy4R include all standard corrections, such as high-frequency spectral correction, some of which are only applied to the final turbulent fluxes and not the instantaneous time series. Thus, we use the total turbulent fluxes as quantified by NEON to rescale all the flux components that we computed (T, E, R, and P), ensuring that they are consistent with the corrected fluxes reported by the network.
As the last step of postprocessing, a gap-filling algorithm was implemented to maximize the availability of flux-partitioning estimates. Additional meteorological variables were downloaded using the R package neonUtilities (Lunch et al., 2024) and used as features to implement the Extreme Gradient Boosting (XGBoost) algorithm. The selected variables (inputs) included air temperature (NEON, 2024g, h), incoming solar radiation (NEON, 2024i, j), relative humidity (NEON, 2024e, f), photosynthetically active radiation (NEON, 2024c, d), and wind speed (NEON, 2024k, l). All quantities were measured at the top of the tower. The sensible heat flux and friction velocity, included in the bundled data products, were also used. Due to the presence of long gaps and/or noisy measurements, precipitation and soil moisture were not included as features.
Below, we describe the methods used to partition the data and the processing steps followed to generate the final dataset. A diagram summarizing all the preprocessing and postprocessing steps is shown in Fig. 2. We implemented five methods to partition evapotranspiration into soil evaporation and plant transpiration as well as net CO2 flux into soil respiration and net plant photosynthesis. It is important to note that these quantities differ from GPP and ecosystem respiration (see Wohlfahrt and Gu, 2015, for a detailed discussion of the different definitions of CO2 flux components). Since these methods are based on principles of turbulent transport, they do not provide the information needed to separate soil respiration from plant respiration. Instead, the framework effectively partitions below-canopy fluxes, which include respiration and evaporation from soil, roots, and possibly the lower parts of tree trunks, and above-canopy fluxes, which encompass plant transpiration and net photosynthesis. Thus, none of these approaches allows for the distinction between canopy and ground respiration. Another limitation of these models is that they cannot distinguish between transpiration and evaporation of intercepted water on the leaves. Nonetheless, since EC data are often lost through poor data quality during and immediately following rainfall periods, many such periods are likely removed from the time series already. With these definitions in mind, we refer to net photosynthesis simply as photosynthesis (P) and plant transpiration simply as transpiration (T) in the following sections, while evaporation (E) and respiration (R) are considered to originate solely from the ground or soil levels. We advise future users to carefully note the specific definitions of each component, as this is essential for making meaningful comparisons across different partitioning products.
3.1 Partitioning approaches
The partitioning methods implemented in this study are based on the idea of transport similarity between CO2 and water vapor. It assumes that turbulent structures, or “eddies”, simultaneously transport evaporation and respiration from the soil towards the sensor, where the respective concentrations of these “event samples” are measured. Similarly, turbulence also transports air parcels from the canopy, where net photosynthesis and plant transpiration take place. By separating the signature of soil and canopy events in the time series, these methods try to infer the respective magnitude of each flux component. Note that, while both evapotranspiration components are sources of water vapor (i.e., they are positive fluxes), CO2 fluxes are a combination of a source (soil respiration) and a sink (photosynthesis). This feature of the CO2 flux is what creates this framework, since it works as a tracer that identifies the origin of water vapor. However, another consequence of this feature is that, while the components E and T are bounded by E+T, the magnitudes of R>0 and P<0 are not constrained by . As a result, partitioning results for CO2 components tend to be more uncertain. Nonetheless, as will be explained in the next section, two of the partitioning methods implemented here require the water use efficiency, , as an input. While the need for a parameterization for W is a disadvantage for the method, the inclusion of W adds a bound to P and R.
In total, five partitioning methods were implemented: flux-variance similarity (FVS) (Scanlon and Sahu, 2008; Scanlon and Kustas, 2010; Scanlon et al., 2019), modified relaxed eddy accumulation (MREA) (Thomas et al., 2008), conditional eddy covariance (CEC) (Zahn et al., 2022), modified CEC that also utilizes water use efficiency (CECw) (Zahn et al., 2024), and conditional eddy accumulation (CEA) (Zahn et al., 2024). The advantage of these models is that they mostly rely on high-frequency eddy-covariance observations, requiring no or few input parameters. While MREA, CEC, and CEA are based solely on statistics computed from the high-frequency data, FVS and CECw additionally require water use efficiency as an input.
Three of the methods, FVS, MREA, and CEC, have been investigated extensively in previous studies (Thomas et al., 2008; Klosterhalfen et al., 2019; Scanlon et al., 2019; Zahn et al., 2022). More recently, these three methods were tested by Zahn et al. (2024) using large-eddy simulations; they also formulated and tested the CECw and CEA approaches to probe the assumptions and performance of all the methods. Their simulation-based analyses indicated that all the methods result in reliable results for a range of flux combinations that are expected in real ecosystems. While that study was not able to pinpoint conditions under which the accuracy of the results in field experiments can be guaranteed, it delineated flux combinations that may result in higher uncertainties, it quantified the impact of errors in W on the results of FVS and CECw, and it identified the most consequential assumptions in the various methods. The broad recommendations of that study are as follows:
-
The measurement height z, i.e., the height where the EC system is placed, should be as close as possible to the mean canopy height, h, to better distinguish between the soil and plant signals. However, while is a good recommendation, we note that the performance also depends on other factors such as canopy density and the specific method, as some approaches were found to perform well even outside this region.
-
All flux components from the soil (evaporation and respiration) and from the vegetation (transpiration and photosynthesis) should be non-negligible. This criterion arises from the water vapor–CO2 coupling, which needs the CO2 signal to determine the origin of the water vapor.
-
The (anti)correlation between CO2 and water vapor, ρc,q, should not be perfect, i.e., . Perfect correlation or anticorrelation indicates that turbulence fully mixed the scalars from the soil and from the canopy. In this case, the framework of all five partitioning models is no longer valid. Most often, high correlation is seen when measurements are too far from the canopy top or when one of the flux components is negligible. Thus, this condition should be satisfied if points 1 and 2 are addressed first.
Overall, by comparing the outputs of the five models and identifying periods or sites where they consistently agree, we can increase our confidence in the results obtained, even without a “true” value for comparison. In addition, the range of predictions from the various methods can be used to quantify the uncertainty in the overall partitioning.
3.2 Data preprocessing
We downloaded and processed high-frequency eddy-covariance turbulent exchange (ECTE) data using our in-house Python routines. The following variables were extracted from the original datasets for each site: the mixing ratios of CO2 and water vapor (rc and rq); the three components of the velocity field in the x, y, and z directions (u, v, and w); the sonic temperature (Ts); and the atmospheric pressure (P). In addition, sensor flags describing the quality of the measurements and signal were used for quality control (see Table 2 for a description of the variables used). Our algorithm was implemented following the preprocessing of daily files as follows:
-
Instantaneous measurements that were assigned 1 (poor quality) by any of the flags described in Table 2 were discarded.
-
CO2 and H2O mixing ratio observations were discarded if the signal strength indicator was smaller than 0.7.
-
Air density (kg m−3) and mass concentrations of CO2 and H2O (mg m−3 and g m−3, respectively) were computed.
-
Daily files with less than 50 % raw high-frequency data were discarded, with very few cases falling into this category. Files that passed this test were split into half-hour blocks of 36 000 points each (at 20 Hz measurements).
-
Instantaneous observations were checked for physical plausibility, such as positive scalar concentrations.
-
Outliers were removed using a despiking algorithm (Zahn et al., 2016).
-
The velocity field was rotated using double-coordinate rotation.
-
CO2 and H2O mass concentrations, c and q, were lag-corrected, since the LI7200 is a closed-path sensor with tubing, using the cross-correlation technique (Rebmann et al., 2012).
-
Turbulent fluctuations were computed for all the quantities by removing the linear trends for all the respective variables.
The outcomes of these steps are 30 min time series comprising cleaned turbulence data. In the next section we describe how these data were used to partition ET and Fc fluxes following the five methods described in Sect. 3.1.
3.3 Implementation of the partitioning methods
Since all partitioning methods are based on turbulence transport and similarity, their implementation requires the computation of turbulent statistics such as covariances and correlation coefficients. Details of their implementation can be found in Zahn et al. (2022, 2024). These statistics are computed for each 30 min block. Using these turbulence statistics, we first implement the three methods that do not require extra inputs, i.e., CEC, CEA, and MREA. For each partitioning method, we thus obtain Tpart, Epart, Ppart, and Rpart, where the subscript “part” represents any of the three methods. For instance, for CEC we have TCEC, ECEC, PCEC, and RCEC.
The implementation of FVS and CECw additionally requires an estimate of the water use efficiency W. Note that, in this context, W is defined as the ratio between net photosynthesis and transpiration, which again differs from other definitions. This quantity was also estimated for each 30 min block using the following expression:
where and are the H2O and CO2 atmospheric mean concentrations near the canopy, while and are the mean intercellular concentrations. The near-canopy concentrations ( and ) are obtained using the logarithmic profile and mean concentrations from EC data, while qs is calculated under the assumptions of stomatal saturation (at air temperature) and a well-coupled leaf. , on the other hand, is the most challenging variable to obtain. Following previous studies (Wagle et al., 2021; Zahn et al., 2022), we implemented five models for , giving a total of five estimates of W. These parameterizations are summarized in Table 3. For each 30 min period, FVS and CECw were thus implemented five times, one for each parameterization of W. As a result, each flux component is estimated five times; for instance, following the nomenclature presented in Table 3, after implementing FVS we obtain , , , , and . These five estimates are then averaged to when at least one of the five outputs is available. The same averaging is done to obtain , , and . Likewise, the same procedure is applied to CECw, which also takes W as an input. Our final dataset includes the outputs from all five models as well as the averaged values, as described in the next section.
Scanlon et al. (2019)Table 3Descriptions of all five parameterizations adopted for W. More details can be found in Wagle et al. (2021) and Zahn et al. (2022). All five parameterizations were used to partition fluxes by FVS and CECw, thus resulting in five partitioning results for each partitioning approach.
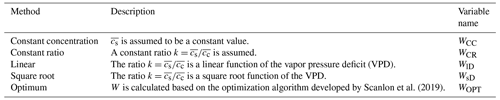
3.4 Data postprocessing
3.4.1 Data cleaning and flux rescaling
The last section described how all five partitioning methods were implemented to obtain estimates of soil and canopy fluxes at a 30 min time interval. In postprocessing, we first eliminated unrealistic high flux magnitudes and counter-gradient fluxes by discarding periods when any of the following conditions were identified:
-
Tpart<0 W m−2 or Epart<0 W m−2;
-
Ppart>0 mg m−2 s−1 or Rpart<0 mg m−2 s−1;
-
W m−2; and
-
mg m−2 s−1.
As previously described, all the partitioning methods relied on high-frequency time series for turbulent statistics and flux computation. Consequently, the resulting flux components lacked certain eddy-covariance corrections commonly applied to the final fluxes, including high-frequency spectral adjustments. To address this, we rescaled our fluxes based on those obtained from the NEON bundled data processed by eddy4R. We first computed correction factors for the ET and Fc fluxes as
where ETNEON and are the evapotranspiration and net CO2 fluxes downloaded directly from NEON, while ETpart and are the fluxes computed by our routines using the high-frequency data following the preprocessing described in Sect. 3.2. Note that the total fluxes, and , are the same for all five partitioning methods. The corrections were then applied as
By rescaling our fluxes based on this comparison, we ensure that our fluxes are consistent with NEON products, mitigating potential discrepancies arising from variations in data processing methodologies. For simplicity, we drop the subscript “cor” in the remainder of the text and in the data files.
Additionally, we utilized NEON's bundled eddy-covariance products to assess the quality of both evapotranspiration and CO2 flux data. Although our initial data preprocessing removed numerous periods with poor quality, we refrained from conducting further tests, such as assessing data stationarity, integral turbulence characteristics, and spectral analysis. Consequently, we integrated the flags as outlined in Metzger et al. (2022) into our final dataset. These flags are classified into two categories: 0, indicating compliance with all the quality criteria and rendering the data valid and reliable for analysis, and 1, indicating failure to meet one or more quality tests and requiring cautious utilization or possible exclusion. Retaining the original flags in our dataset facilitates the identification of periods characterized by high-quality data. Nonetheless, we opted not to automatically discard data based solely on these flags, leaving such decisions to the discretion of subsequent users.
3.4.2 Gap-filling
As a consequence of the inherent challenges associated with eddy-covariance measurements – such as sensor malfunction, data transmission, or even external factors such as rain and dust collection – flux time series are often incomplete. For our dataset, Table 1 shows the availability of ET fluxes during the 5-year period for each site. Considering only daytime periods (selected based on incoming solar radiation greater than 10 W m2), this reveals that some sites have available fluxes only 34 % of the time (BARR), while others have daytime ET estimates for up to 77 % of the 5-year record (KONA).
Due to mathematical constraints and/or invalidity of physical assumptions, partitioning methods are not guaranteed to converge to a solution for every 30 min time series, leading to further gaps in the record of flux components. As a consequence, the dataset generated as described in the previous sections contains missing periods, with the length of these gaps depending on the site and method. Table 4 illustrates that the methods with the lowest convergence rates – defined as the fraction of solutions found per available period – range from least to most solutions found as follows: FVS, CECw, MREA, CEA, and CEC. The lowest rate of convergence for the FVS method (on average 50 %) is not surprising and has been discussed in previous studies (Wagle et al., 2021; Zahn et al., 2022, 2024). Additionally, the low rate of solutions for FVS and CECw when combined with WOPT is due to the model for this water use efficiency itself not always converging (i.e., WOPT was not available).
Table 4Success rate of each partitioning approach in finding a solution, expressed as a percentage of the available half-hour periods (i.e., only considering cases when the time series was available and the partitioning methods were implemented). The table shows the average and standard deviation across all the sites before and after gap-filling. For FVS and CECw, the convergence using each water use efficiency parameterization is also shown.
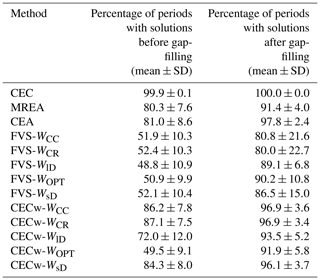
To increase the availability of periods with valid partitioning estimates for each method, we implemented XGBoost (Chen and Guestrin, 2016) for regression to predict transpiration. By training the algorithm to partition ET into E and T based on selected environmental features, the goal is to apply the trained algorithm to estimate T only when ET is available but a given partitioning method did not yield a valid estimate. That is, an XGBoost model is developed for each partitioning approach independently and then applied to fill the gaps in the time series of that approach. Additional machine learning algorithms, including neural networks and k-means clustering, were investigated but yielded similar or inferior results compared to XGBoost. Previous studies focusing on gap-filling eddy-covariance data also observed superior performance by XGBoost (Huang and Hsieh, 2020; Irvin et al., 2021). These findings are corroborated by research indicating that tree-based models outperform deep learning for tabular data used in regression tasks (Grinsztajn et al., 2022). Thus, the selected algorithm is robust and a very suitable choice for the problem at hand.
Only daytime conditions were considered, a constraint imposed by selecting periods when the incoming solar radiation was >10 W m2. In addition, we ensured that only cases with positive ET were included. For regression purposes, we further filtered out periods where one or more input features were unavailable. As a result of these criteria, not every segment of the time series was suitable for gap-filling. Nonetheless, this approach maximizes the data availability of T and E, in particular during daytime, expanding the use of this dataset for future studies. Note that gap-filling was not applied to the Fc components. This decision was made due to the noisier nature of CO2 fluxes. Furthermore, as discussed later, estimates for respiration and photosynthesis are less reliable across methods (larger variance between methods), and thus gap-filling for these components was not deemed as robust as with ET components.
To maximize the periods with available data features, we only selected meteorological variables with short periods of missing data and high quality. Following tests on different meteorological variables, the following features were retained: ET, air temperature (TAIR), photosynthetically active radiation (PAR), relative humidity (RH), vapor pressure deficit (VPD), mean wind speed (U), sensible heat flux (H), and friction velocity (u*). In addition, daily and seasonal cycles were represented as
where Xdiurnal and Xyearly represent the diurnal and yearly cycles corresponding to the hour of the day (in local time) and the Julian day, respectively. While there are other variables that could be relevant for this problem, they were excluded due to poor data quality or availability. Nonetheless, using only the listed variables resulted in excellent performance metrics.
For each site and partitioning method – including the different combinations of the FVS, CECw, and water use efficiency models taken individually – the dataset was divided into training (60 %), validation (20 %), and testing (20 %) sets. Across the different cases, the size of the training set varied between 1700 and 12 000 points. To monitor the performance of the algorithm, we used the coefficient of determination, R2, defined as
where y represents the observed values with average , while represents the predictions. This metric indicates how well the independent variables explain the variance in the dependent variable. Thus, the upper bound is expected only if the selected features explain 100 % of the variance.
After experimenting with different hyperparameters, the same values for the learning rate (0.01), maximum depth of a tree (5), and number of trees (1000) were used to train all the sites and methods individually. Finally, gap-filling was only performed for a particular case, when its respective R2 on the test set was greater than 0.7. A few cases had an R2 below this threshold, which was found to correspond most often to datasets combining FVS and WCC or WCR over shrubland or tundra sites, combinations which resulted in the lowest number of valid partitioning estimates (smallest training sets). Excluding these few exceptions, the majority of the cases featured R2>0.8 (and bias < 1 W m−2) and were deemed reliable for gap-filling. The final metrics for all the models and sites can be found in the Supplement. All auxiliary data used to implement the gap-filling algorithm are also available in the final dataset.
As expected, the algorithm identified ET as the most relevant variable across all the cases (approximately 80 %). Note that, in this context, we do not use ET to explain the variability in E or T in the sense of an environmental forcing. Instead, ET is used to teach the model how to partition the fluxes, effectively functioning as a machine-learning-based partitioning method. In Sect. 6, we briefly explore feature importance for T and in the context of environmental forcings, where only environmental variables are included as features (excluding ET).
While the variability in feature importance was small across the vegetation types, a larger variability was observed between the methods. Notably, FVS combined with the water use efficiency models WCC and WCR often attributed a lower importance to ET (as low as 27 %) and a higher importance to VPD (as high as 25 %). Previous work (Wagle et al., 2023) has shown that these two water use efficiency models are very sensitive to the choice of parameters and greatly overestimate W compared to the other models, especially early in the morning. While investigating the possible causes of this discrepancy across water use efficiency models is beyond the scope of this paper, deeper analyses will be enabled by this dataset.
Following the algorithm evaluation, transpiration was estimated for the missing data periods and defined as , where the superscript “xgb” refers to the gap-filled variable using XGBoost. Only half-hour periods with were gap-filled. Evaporation was then estimated as . For FVS and CECw, we also computed the average across their different water use efficiency outputs, corresponding to and .
The convergence rate to valid solutions after gap-filling is shown in the last column of Table 4. Most notably, gap-filling increased the presence of estimates from the FVS method for 80 % to 90 % of the periods when partitioning was applied. To further illustrate the completeness of the flux-partitioning record in the 5-year dataset, Table 1 shows the availability of flux components as a fraction of the total number of half-hour periods in the record. Overall, all the methods cover a similar temporal distribution of flux partitioning and are potential candidates for ensemble averaging.
The final dataset is available for download at https://doi.org/10.5281/zenodo.12191876 (Zahn and Bou-Zeid, 2024). It is organized into different folders for each site, with each site containing a .csv file for each method. This format is selected to be user-friendly and accessible in various programming languages and software packages. For FVS and CECw, in addition to their ensemble averages for different water use efficiency parameterizations, we include each individual output. This allows future users to compare the impact of water use efficiency with the partitioning output.
All the results are in UTC, where this represents the start time of measurements for a half-hour period (i.e., starting at minute “00” or “30”). Each file contains all fluxes in continuous time stamps of 30 min. In cases where a specific variable was unavailable, the corresponding cell in the .csv file was left blank. A Python script showing how to read the final data files is included with the dataset.
In each file, the following variables are included:
-
Tpart
, transpiration [W m−2]; -
Tpart_xgb
, transpiration after gap-filling [W m−2]; -
Ppart
, plant net photosynthesis [mg m−2 s−1]; -
Epart
, below-canopy evaporation [W m−2]; -
Epart_xgb
, below-canopy evaporation after gap-filling [W m−2]; -
Rpart
, below-canopy respiration [mg m−2 s−1]; -
Fc
, net CO2 flux [mg m−2 s−1]; -
ET
, evapotranspiration [W m−2]; -
qfFinalCO2
, final NEON quality flag for the CO2 flux (0:Fc
passed all quality assurance tests; 1:Fc
was flagged by one or more tests); and -
qfFinalH2O
, final NEON quality flag for theET
flux (0:ET
passed all quality assurance tests; 1:ET
was flagged by one or more quality assurance tests).
For the flux components, “part” represents any of the methods. In addition, a separate file, WUEinput.csv, contains the water use efficiency values from all five parameterizations, defined as follows:
-
const_ppm
[kg(kg]; -
const_ratio
[kg(kg]; -
linear
[kg(kg]; -
sqrt
[kg(kg]; and -
opt
[kg(kg].
Finally, a file named MeteorologicalVariables.csv is also included, which contains all the auxiliary meteorological variables used for gap-filling and feature importance analysis. This file is synchronized with the partitioning data file to contain the same records. The following variables are available:
-
SoilMoisture
, soil moisture measured at the second level below the surface (depth varies by site) [m3 m−3]; -
AirTemperature
, air temperature [°C]; -
SolarRadIn
, incoming solar radiation measured at the top of the tower [W m−2]; -
RH
, relative humidity (%); -
Rain
, accumulated precipitation at the top of the tower during the 30 min period [mm]; -
PAR
, photosynthetically active radiation measured at the top of the tower [µmolec. m−2 s−1]; -
fluxTemp
, sensible heat flux at the top of the tower [W m−2]; -
ustar
, friction velocity at the top of the tower [m s−1]; -
WindSpeed
, mean wind speed at the top of the tower [m s−1]; and -
vpd
, vapor pressure deficit [kPa].
A comparison of across all the seasons for different types of vegetation is presented in Fig. 3. The averages were computed over half-hour periods obtained between 06:00 and 18:00 LT (local time). For all the vegetation types, the average ratios range from 0.5 in winter to 0.7 in summer. Except for the results over the tundra locations, there is a notable similarity across the five methods, particularly during spring and fall. In contrast, the dissimilarity across the methods for tundra is possibly linked to the heterogeneity of these sites, which breaks down the assumptions of the partitioning methods more often. A surprising result is that good agreement across the methods was observed regardless of the measurement height. Even above the agricultural sites, where the ratio can be as large as 30, good agreement was observed for many locations.
Given the underlying uncertainties with the partitioning algorithms, one route for verifying the reliability of partitioning estimates is to compare different methods. In Table 5, we compare the different transpiration estimates obtained by CEC, FVS, CEA, and CECw, where the slope α of the linear regression is shown. The results for MREA were similar to those for CEC and are not shown. To help interpret these results, they were separated into four groups: agreement in the 10 % range, i.e., ; slopes in the range ; slopes in the range ; and disagreement greater than 30 %, i.e., α<0.7 or α>1.3.
Table 5Slopes of the linear regression for transpiration for all the methods across all the sites. Only half-hour periods between 06:00 and 18:00 LT, without gap-filling, were included. For FVS and CECw, their ensemble average across the water use efficiency options was used. The sites were grouped according to the dominant vegetation type representing the tower footprint. To facilitate visual inspection of the results, slopes α in the 10 % range () are shown in bold, while slopes in the 20 % range () appear in italic.
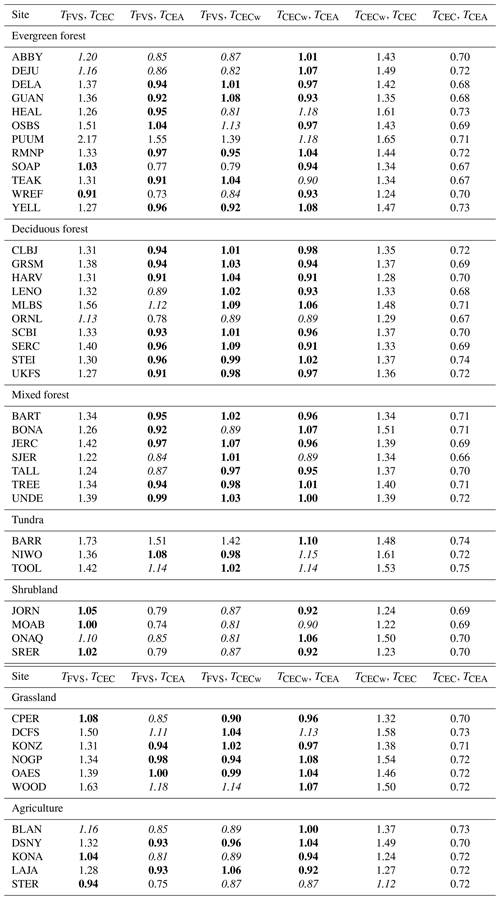
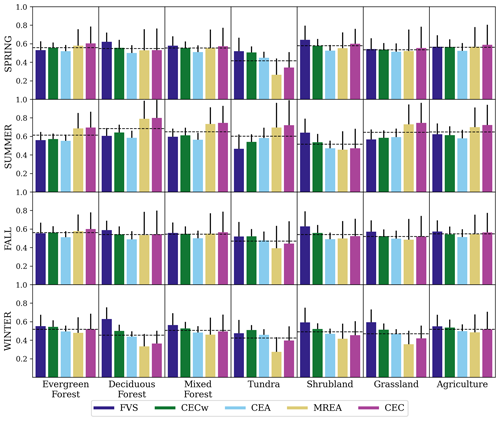
Figure 3 ratios averaged over all the half-hour periods across seasons and sites with similar vegetation types. Only half-hour periods during the day (06:00 and 18:00 LT) were included, and only when solutions for all the methods were available. For FVS and CECw, the ensemble averages of outputs with different water use efficiencies were used. For each season or vegetation type, the black dashed line indicates the average across all the methods.
Overall, TFVS and TCEA are in very good agreement, with differences often within the 10 % range across various sites. Only at two sites (PUUM and BARR) were the slopes found to be greater than 1.3 (1.55 and 1.39, respectively). Similarly good agreement is seen for the pairs TFVS and TCECw as well as TCEA and TCECw. The close agreement for these three methods was also observed in their average diurnal cycles, in particular between 09:00 and 15:00 LT (Fig. A1), and even under conditions when . Nonetheless, note that the diurnal cycles for FVS exhibit higher values early in the morning and late in the afternoon. Previous studies have highlighted significant variability among the five W options, with differences that can span 2 orders of magnitude during the early hours (Wagle et al., 2023), which inevitably influences the output of the FVS method. Therefore, without additional information on the actual water use efficiency, we cannot explain this behavior with certainty.
The good agreement between FVS and CEA is particularly encouraging given that the CEA method does not require water use efficiency as an input, using only turbulence information. Moreover, we showed that the outputs of FVS using WCR and WCC were the ones that differed most from CEA, the remaining three FVS outputs being more similar; WOPT produced the estimates closest to CEA (Fig. A2). Finally, transpiration estimates obtained by CEC tend to disagree more often with other methods (similar trends were observed for MREA; data not shown). Nonetheless, increased agreement is observed when the data are separated by seasons, as shown in Figs. 3 and A1.
The main challenge of flux partitioning is verifying the accuracy of these results, so we cannot initially assume that CEC's results are inherently inaccurate or incorrect. However, previous numerical experiments (Zahn et al., 2024) found that CEC and MREA are more restrictive than the other approaches, being stricter in terms of measurement height and canopy density. Thus, it is possible that the denser canopy during summer will directly affect these assumptions, decreasing these two methods' performance. They were also more prone to larger errors when the ratios and were more dissimilar. Unfortunately, a metric to determine the “goodness” of partitioning is not feasible since in experiments the true answer is never known. Only estimates of these partitioned fluxes are available since even the sap flow, isotope, and flux chamber methods have their limitations.
Given this uncertainty, a prudent recommendation is to implement the ensemble averages of T and E for all the methods in future studies. Ideally, the results should converge regardless of the partitioning methods used. However, depending on the specific NEON site or analyses of interest, it may be advantageous to prioritize the FVS, CEA, and CECw methods under certain circumstances given their close agreement, potentially adding either CEC or MREA since these two methods give similar results and should thus be treated as one member of the ensemble. To this end, the slopes shown in Table 5 offer a reference.
Table 6Slopes of the linear regression for net primary productivity (P) for all the methods across all the sites. Only half-hour periods between 06:00 and 18:00 LT were included. For FVS and CECw, their ensemble averages across the water use efficiency options were used. The sites were grouped according to the dominant vegetation type. To facilitate visual inspection of the results, slopes α in the 10 % range () are shown in bold, while slopes in the 20 % range () appear in italic.
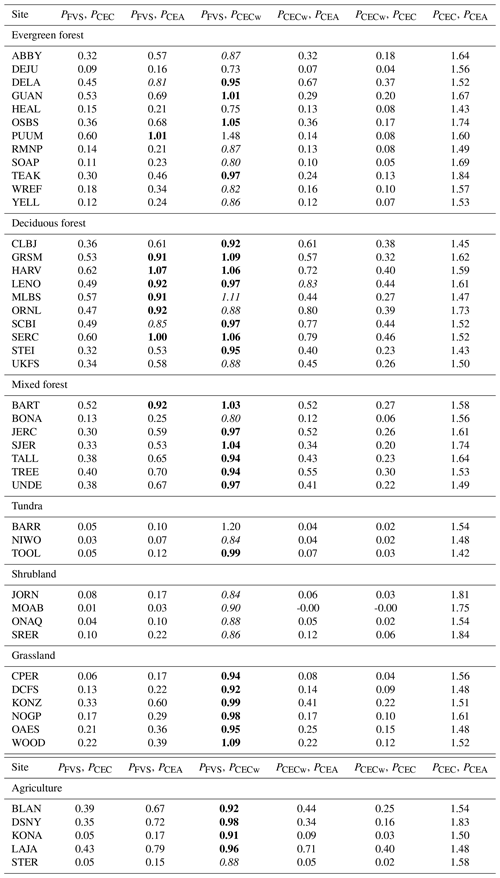
In contrast to ET flux partitioning, the estimated CO2 flux components showed more disagreement across the methods (Table 6). Generally, methods that do not require water use efficiency (CEC, CEA, and MREA) underestimated P and R compared to FVS and CECw, where most slopes indicated less than 10 % agreement. Our previous findings using large-eddy simulations to test all the methods (Zahn et al., 2024) found larger errors for CO2 components with CEC, CEA, and MREA. We found that, while CO2 is essential as a tracer for H2O in these methods' formulations, it cannot be reliably partitioned between soil and canopy components given the lack of an upper bound on Fc components, which combine a positive (respiration) flux and a negative (photosynthesis) flux. In contrast, FVS and CECw limit the magnitudes of these components by imposing , thus adding a constraint to the magnitude of . Note, however, that P (and R) will be subjected to uncertainties present in the water use efficiency estimate. Overall, these findings indicate that CO2 flux partitioning is more challenging, and care must be taken when using and interpreting these results. At present, for CO2 flux components, we thus recommend taking the ensemble average of CECw and FVS (across all five W estimates), potentially adding CEA as a third member of the ensemble for the selected sites, in particular above deciduous forests following Table 6.
The final dataset will enable hydrologists and ecologists to investigate a variety of research questions related to flux partitioning. Considering the dataset's breadth of coverage, its spatial and temporal distributions enable comparison across diverse conditions, encompassing various forest structures, water availability, climate and weather patterns, and more. Due to remaining gaps in the final flux time series, evaluation of long-term budgets over the period of 5 years is not recommended without additional work to complete the temporal coverage. Nonetheless, budgets over smaller periods and for specific NEON sites are possible and can be explored. In this case, for nighttime periods, when most partitioning results are unavailable, researchers might fill gaps by assuming zero plant fluxes (e.g., for T and P), E=ET, and R=Fc.
One relevant research question regarding ecosystem functioning revolves around the main drivers of plant and soil fluxes. To briefly explore this question, we implemented XGBoost to estimate the importance of a few environmental variables for T and , where we first averaged the output from all five partitioning models (excluding gap-filled periods). Note that this analysis is different from the procedure used for gap-filling, where ET was used as a predictor. Here, we simply investigate the importance of each environmental variable as a driver of plant fluxes, and thus ET is not used as an input. The selected features were air temperature, photosynthetically active radiation, relative humidity, vapor pressure deficit, mean wind speed, sensible heat flux, and friction velocity.
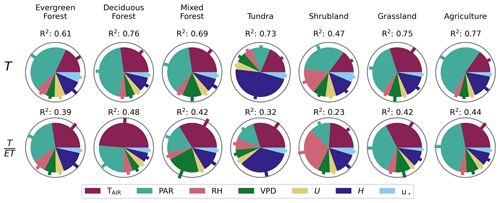
Figure 4Importance of seven environmental variables as drivers of transpiration (top-row panels) and (bottom-row panels) across the vegetation types. From left to right, the legend includes air temperature (TAIR), photosynthetically active radiation (PAR), relative humidity (RH), vapor pressure deficit (VPD), mean wind velocity (U), sensible heat flux (H), and friction velocity (u*). The pie charts display the average over all sites with the same vegetation type, where bars represent the standard deviation of feature importance across the sites ( %). As a reference, the outer circle represents a standard deviation of 20 %.
The importance of each environmental variable is shown in Fig. 4 for T (top row) and (bottom row) averaged across sites with the same vegetation type. The standard deviation was also computed across the sites and is represented by the bars in the figure (the outer circle represents a standard deviation of 20 %). Notably, these seven environmental variables can explain up to 77 % (R2=0.77 over the agricultural sites) of the variability in transpiration. With the exception of the tundra sites, where sensible heat was the most important variable, photosynthetically active radiation, followed by air temperature, was the most relevant feature. Intersite variability was also observed: for instance, the standard deviation of PAR and TAIR was as large as 20 % for evergreen and mixed forests. The variability across the methods is not included in this analysis since the ensemble average was used; nonetheless, similar to our discussion regarding gap-filling, we observed that FVS combined with WCC and WCR often differed from the other methods (results not shown). This discrepancy is also left to future studies.
The ratio , on the other hand, is more complex and dependent on additional variables not included in this analysis. Across the vegetation types, these seven features were able to explain as little as 23 % (shrublands) or no more than 48 % (deciduous forests). While relevant environmental variables such as soil moisture and accumulated rainfall were not included in our analyses due to availability and/or data quality, these variables can be included for selected sites and/or smaller temporal records. By exploring different environmental forcings, this dataset presents an opportunity to address specific inquiries, such as the impact of heat waves, droughts, and other extreme events on plant transpiration. Research endeavors of this nature are vital, as they contribute to a deeper comprehension of how present and future climate conditions affect local water and energy budgets, together with plant water use efficiency (Hatfield and Dold, 2019).
The link between fluxes and canopy structure features another set of critical scientific questions that can be addressed using this dataset. Previous work (Wang et al., 2014; Wei et al., 2017) compiled results from several case studies and summarized the correlation between and leaf area index (LAI), which has been turned into a simple regression model often used in other studies (Nelson et al., 2020). Future investigations could expand this study and utilize NEON products or satellite imagery to derive seasonal variations in LAI, thereby enhancing our understanding of how vegetation dynamics influence transpiration. By combining the most important meteorological features and vegetation characteristics, simpler and more accurate partitioning models can be derived and applied more broadly.
In addition to observational studies, modeling or hybrid studies (Rafi et al., 2019; Kozii et al., 2020) can also benefit from the dataset compiled here. This approach can be explored to develop models for transpiration partitioning or modeling (Bright et al., 2022), where observations can validate the model or help determine relevant parameters, such as plant conductance. For instance, Schreiner‐McGraw et al. (2022) used transpiration data from FVS to optimize parameters for ParFlow.CLM, a hydrological model coupling groundwater flow to a land surface model (Maxwell and Miller, 2005). This methodology is particularly useful for evaluating the performance of land surface models, such as NOAH-MP (Niu et al., 2011; Li et al., 2021) and the Community Land Model (Lawrence et al., 2007), thereby improving assessments of their impact on weather prediction and climate projections (Berg and Sheffield, 2019; Dong et al., 2022).
Future studies can also expand this dataset by including additional partitioning models, including the products derived from the CO2 partitioning algorithm proposed by Reichstein et al. (2005) and Lasslop et al. (2010). In terms of ET partitioning, for instance, different methods have been proposed that take GPP as an input (Perez-Priego et al., 2018; Zhou et al., 2016; Nelson et al., 2018); such models were compared by Nelson et al. (2020) across FLUXNET sites. By comparing different algorithms, we can further explore their uncertainties and focus on model improvement. Finally, as more data become available, other options can be used to train machine learning algorithms, focusing on gap-filling.
The dataset is available at https://doi.org/10.5281/zenodo.12191876 (Zahn and Bou-Zeid, 2024). In addition to all the flux components, it contains the auxiliary meteorological inputs used to implement the Extreme Gradient Boosting algorithm for gap-filling and feature importance analysis. The scripts used to implement all five partitioning methods can be found at https://doi.org/10.5281/zenodo.13244979 (Zahn, 2024).
This paper described the first dataset of flux partitioning across all NEON eddy covariance sites from 2019 to 2023. Estimates of transpiration, evaporation, soil respiration, and plant net CO2 assimilation were obtained using five partitioning approaches. These methods had undergone extensive testing in both experimental and numerical settings in prior studies (Zahn et al., 2022, 2024), aiding in understanding their limitations and reliability.
Overall, ET components exhibited notable consistency across the methods, which was particularly evident in FVS, CEA, and CECw, thereby enhancing confidence in these estimates. In contrast, the CO2 components are more challenging, and care must be taken when interpreting these estimates. The final dataset, available at https://doi.org/10.5281/zenodo.12191876 (Zahn and Bou-Zeid, 2024), can be used to address numerous research questions. We recommend utilizing an ensemble average of the methods available for a particular site. However, based on the comparison presented in this study, a combination of the methods might be adopted for specific analyses or NEON sites. In any case, the user should keep in mind the following considerations:
-
None of the methods can estimate plant respiration and gross photosynthesis separately: only an estimate of their difference and plant net photosynthesis (i.e., plant net fluxes) is computed. Similarly, below-canopy respiration – which we assume to be mostly from the soil – also includes respiration from roots and litter.
-
Evaporation from canopy-intercepted water is neglected.
-
While nighttime fluxes are also included, we do not recommend the inclusion of nighttime periods, since CO2 assimilation is not expected at night for C3 and C4 plants, thus breaking the assumption of CO2–H2O coupling.
-
Uncertainties in W will be transferred to the partitioning estimates for FVS and CECw.
-
Given the differences in the method frameworks and their assumptions, the data gaps vary. Thus, selecting the periods of interest and the methods to average must be done with care to avoid biases.
This dataset opens up the many research pathways that we outlined above and that can be the basis for other derived datasets. The partitioning approaches can also be applied to other periods of NEON observations or implemented in the NEON workflow so that the partitioned fluxes are part of the standard NEON outputs.
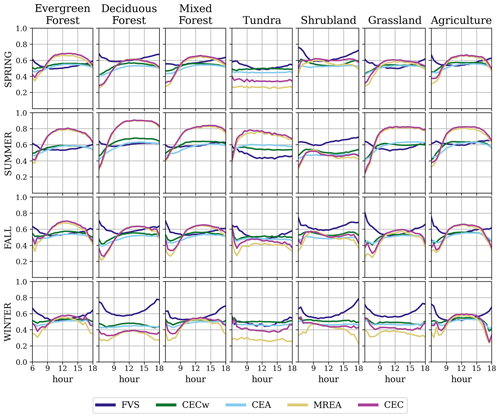
Figure A1Diurnal cycle of the partitioning ratio for all the methods across season and vegetation type. Half-hourly estimates during the daytime hours (06:00 to 18:00 LT) are only included when all the methods have valid solutions. For FVS and CECw, the ensemble average across the water use efficiency options was used.
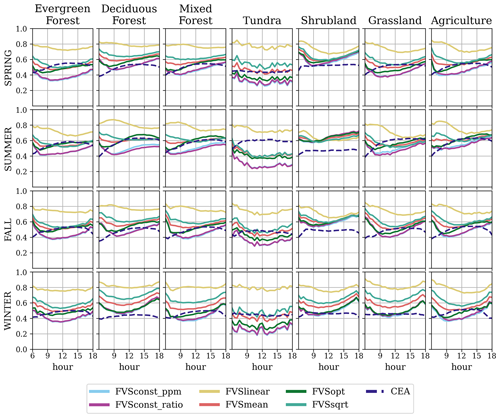
Figure A2Diurnal cycle of the partitioning ratio comparing the FVS output from different water use efficiency models (and their ensemble average) and the CEA method (dashed line). Half-hourly estimates during the daytime hours (06:00 to 18:00 LT) are only included when all the methods have valid solutions.
The supplement related to this article is available online at: https://doi.org/10.5194/essd-16-5603-2024-supplement.
EZ and EBZ conceptualized the study and designed the methodology. EZ processed the data. EZ wrote the original draft. EZ and EBZ edited and revised the manuscript.
The contact author has declared that neither of the authors has any competing interests.
Publisher's note: Copernicus Publications remains neutral with regard to jurisdictional claims made in the text, published maps, institutional affiliations, or any other geographical representation in this paper. While Copernicus Publications makes every effort to include appropriate place names, the final responsibility lies with the authors.
This work was supported by the Moore Charitable Foundation's Science-to-Action Fund of the School of Engineering and Applied Science at Princeton University.
This paper was edited by Tobias Gerken and reviewed by two anonymous referees.
Baslam, M., Mitsui, T., Hodges, M., Priesack, E., Herritt, M. T., Aranjuelo, I., and Sanz-Saez, A.: Photosynthesis in a Changing Global Climate: Scaling Up and Scaling Down in Crops, Front. Plant Sci., 11, 882, https://doi.org/10.3389/fpls.2020.00882, 2020. a
Berg, A. and Sheffield, J.: Evapotranspiration Partitioning in CMIP5 Models: Uncertainties and Future Projections, J. Climate, 32, 2653==2671, https://doi.org/10.1175/jcli-d-18-0583.1, 2019. a
Bright, R. M., Miralles, D. G., Poyatos, R., and Eisner, S.: Simple Models Outperform More Complex Big‐Leaf Models of Daily Transpiration in Forested Biomes, Geophys. Res. Lett., 49, e2022GL100100, https://doi.org/10.1029/2022gl100100, 2022. a
Chen, T. and Guestrin, C.: XGBoost: A Scalable Tree Boosting System, in: Proceedings of the 22nd ACM SIGKDD International Conference on Knowledge Discovery and Data Mining, KDD'16, ACM, 13–17 August 2016, San Francisco, California, USA, https://doi.org/10.1145/2939672.2939785, 2016. a
Dong, J., Lei, F., and Crow, W. T.: Land transpiration-evaporation partitioning errors responsible for modeled summertime warm bias in the central United States, Nat. Commun., 13, 336, https://doi.org/10.1038/s41467-021-27938-6, 2022. a
Dusenge, M. E., Duarte, A. G., and Way, D. A.: Plant carbon metabolism and climate change: elevated CO2 and temperature impacts on photosynthesis, photorespiration and respiration, New Phytol., 221, 32–49, https://doi.org/10.1111/nph.15283, 2019. a
Fiorella, R. P., Good, S. P., Allen, S. T., Guo, J. S., Still, C. J., Noone, D. C., Anderegg, W. R. L., Florian, C. R., Luo, H., Pingintha‐Durden, N., and Bowen, G. J.: Calibration Strategies for Detecting Macroscale Patterns in NEON Atmospheric Carbon Isotope Observations, J. Geophys. Res.-Biogeo., 126, e2020JG005862, https://doi.org/10.1029/2020jg005862, 2021. a
Good, S. P., Soderberg, K., Guan, K., King, E. G., Scanlon, T. M., and Caylor, K. K.: δ2H isotopic flux partitioning of evapotranspiration over a grass field following a water pulse and subsequent dry down, Water Resour. Res., 50, 1410–1432, https://doi.org/10.1002/2013wr014333, 2014. a
Grinsztajn, L., Oyallon, E., and Varoquaux, G.: Why do tree-based models still outperform deep learning on typical tabular data?, in: Thirty-sixth Conference on Neural Information Processing Systems Datasets and Benchmarks Track, https://openreview.net/forum?id=Fp7__phQszn (last access: 15 September 2024), 2022. a
Hatfield, J. L. and Dold, C.: Water-Use Efficiency: Advances and Challenges in a Changing Climate, Front. Plant Sci., 10, 103, https://doi.org/10.3389/fpls.2019.00103, 2019. a
Huang, I.-H. and Hsieh, C.-I.: Gap-Filling of Surface Fluxes Using Machine Learning Algorithms in Various Ecosystems, Water, 12, 3415, https://doi.org/10.3390/w12123415, 2020. a
Irvin, J., Zhou, S., McNicol, G., Lu, F., Liu, V., Fluet-Chouinard, E., Ouyang, Z., Knox, S. H., Lucas-Moffat, A., Trotta, C., Papale, D., Vitale, D., Mammarella, I., Alekseychik, P., Aurela, M., Avati, A., Baldocchi, D., Bansal, S., Bohrer, G., Campbell, D. I., Chen, J., Chu, H., Dalmagro, H. J., Delwiche, K. B., Desai, A. R., Euskirchen, E., Feron, S., Goeckede, M., Heimann, M., Helbig, M., Helfter, C., Hemes, K. S., Hirano, T., Iwata, H., Jurasinski, G., Kalhori, A., Kondrich, A., Lai, D. Y., Lohila, A., Malhotra, A., Merbold, L., Mitra, B., Ng, A., Nilsson, M. B., Noormets, A., Peichl, M., Rey-Sanchez, A. C., Richardson, A. D., Runkle, B. R., Schäfer, K. V., Sonnentag, O., Stuart-Haëntjens, E., Sturtevant, C., Ueyama, M., Valach, A. C., Vargas, R., Vourlitis, G. L., Ward, E. J., Wong, G. X., Zona, D., Alberto, M. C. R., Billesbach, D. P., Celis, G., Dolman, H., Friborg, T., Fuchs, K., Gogo, S., Gondwe, M. J., Goodrich, J. P., Gottschalk, P., Hörtnagl, L., Jacotot, A., Koebsch, F., Kasak, K., Maier, R., Morin, T. H., Nemitz, E., Oechel, W. C., Oikawa, P. Y., Ono, K., Sachs, T., Sakabe, A., Schuur, E. A., Shortt, R., Sullivan, R. C., Szutu, D. J., Tuittila, E.-S., Varlagin, A., Verfaillie, J. G., Wille, C., Windham-Myers, L., Poulter, B., and Jackson, R. B.: Gap-filling eddy covariance methane fluxes: Comparison of machine learning model predictions and uncertainties at FLUXNET-CH4 wetlands, Agr. Forest Meteorol., 308–309, 108528, https://doi.org/10.1016/j.agrformet.2021.108528, 2021. a
Kirschbaum, M. U. F. and McMillan, A. M. S.: Warming and Elevated CO2 Have Opposing Influences on Transpiration. Which is more Important?, Curr. Forest. Rep., 4, 51–71, https://doi.org/10.1007/s40725-018-0073-8, 2018. a
Klosterhalfen, A., Graf, A., Brüggemann, N., Drüe, C., Esser, O., González-Dugo, M. P., Heinemann, G., Jacobs, C. M. J., Mauder, M., Moene, A. F., Ney, P., Pütz, T., Rebmann, C., Ramos Rodríguez, M., Scanlon, T. M., Schmidt, M., Steinbrecher, R., Thomas, C. K., Valler, V., Zeeman, M. J., and Vereecken, H.: Source partitioning of H2O and CO2 fluxes based on high-frequency eddy covariance data: a comparison between study sites, Biogeosciences, 16, 1111–1132, https://doi.org/10.5194/bg-16-1111-2019, 2019. a
Kool, D., Agam, N., Lazarovitch, N., Heitman, J., Sauer, T., and Ben-Gal, A.: A review of approaches for evapotranspiration partitioning, Agr. Forest Meteorol., 184, 56==70, https://doi.org/10.1016/j.agrformet.2013.09.003, 2014. a, b
Kozii, N., Haahti, K., Tor-ngern, P., Chi, J., Hasselquist, E. M., Laudon, H., Launiainen, S., Oren, R., Peichl, M., Wallerman, J., and Hasselquist, N. J.: Partitioning growing season water balance within a forested boreal catchment using sap flux, eddy covariance, and a process-based model, Hydrol. Earth Syst. Sci., 24, 2999–3014, https://doi.org/10.5194/hess-24-2999-2020, 2020. a
Lasslop, G., Reichstein, M., Papale, D., Richardson, A. D., Arneth, A., Barr, A., Stoy, P., and Wohlfahrt, G.: Separation of net ecosystem exchange into assimilation and respiration using a light response curve approach: critical issues and global evaluation, Global Change Biol., 16, 187–208, https://doi.org/10.1111/j.1365-2486.2009.02041.x, 2010. a, b
Lawrence, D. M., Thornton, P. E., Oleson, K. W., and Bonan, G. B.: The Partitioning of Evapotranspiration into Transpiration, Soil Evaporation, and Canopy Evaporation in a GCM: Impacts on Land–Atmosphere Interaction, J. Hydrometeorol., 8, 862–880, https://doi.org/10.1175/jhm596.1, 2007. a
Lesk, C., Coffel, E., Winter, J., Ray, D., Zscheischler, J., Seneviratne, S. I., and Horton, R.: Stronger temperature–moisture couplings exacerbate the impact of climate warming on global crop yields, Nat. Food, 2, 683–691, https://doi.org/10.1038/s43016-021-00341-6, 2021. a
Li, L., Yang, Z., Matheny, A. M., Zheng, H., Swenson, S. C., Lawrence, D. M., Barlage, M., Yan, B., McDowell, N. G., and Leung, L. R.: Representation of Plant Hydraulics in the Noah‐MP Land Surface Model: Model Development and Multiscale Evaluation, J. Adv. Model. Earth Syst., 13, e2020MS002214, https://doi.org/10.1029/2020ms002214, 2021. a
Lunch, C., Laney, C., Mietkiewicz, N., Sokol, E., Cawley, K., and NEON: neonUtilities: Utilities for Working with NEON Data, version 2.4.2, CRAN, https://doi.org/10.32614/CRAN.package.neonUtilities, 2024. a
Maxwell, R. M. and Condon, L. E.: Connections between groundwater flow and transpiration partitioning, Science, 353, 377–380, https://doi.org/10.1126/science.aaf7891, 2016. a
Maxwell, R. M. and Miller, N. L.: Development of a Coupled Land Surface and Groundwater Model, J. Hydrometeorol., 6, 233–247, https://doi.org/10.1175/jhm422.1, 2005. a
Mengis, N., Keller, D. P., Eby, M., and Oschlies, A.: Uncertainty in the response of transpiration to CO2 and implications for climate change, Environ. Res. Lett., 10, 094001, https://doi.org/10.1088/1748-9326/10/9/094001, 2015. a
Metzger, S., Durden, D., Sturtevant, C., Luo, H., Pingintha-Durden, N., Sachs, T., Serafimovich, A., Hartmann, J., Li, J., Xu, K., and Desai, A. R.: eddy4R 0.2.0: a DevOps model for community-extensible processing and analysis of eddy-covariance data based on R, Git, Docker, and HDF5, Geosci. Model Dev., 10, 3189–3206, https://doi.org/10.5194/gmd-10-3189-2017, 2017. a
Metzger, S., Ayres, E., Durden, D., Florian, C., Lee, R., Lunch, C., Luo, H., Pingintha-Durden, N., Roberti, J. A., SanClements, M., Sturtevant, C., Xu, K., and Zulueta, R. C.: From NEON Field Sites to Data Portal: A Community Resource for Surface–Atmosphere Research Comes Online, B. Am. Meteorol. Soc., 100, 2305–2325, https://doi.org/10.1175/BAMS-D-17-0307.1, 2019. a
Metzger, S., Durden, D., Xu, K., Pingintha-Durden, N., Luo, H., and Florian, C.: NEON Algorithm Theoretical Basis Document (ATBD): Eddy-Covariance Data Products Bundle, Technical Report NEON.DOC.004571, NEON – National Ecological Observatory Network, http://data.neonscience.org/data-product-view?dpCode=DP4.00200.001 (last access: 12 September 2024), 2022. a
Nelson, J. A., Carvalhais, N., Cuntz, M., Delpierre, N., Knauer, J., Ogée, J., Migliavacca, M., Reichstein, M., and Jung, M.: Coupling Water and Carbon Fluxes to Constrain Estimates of Transpiration: The TEA Algorithm, J. Geophys. Res.-Biogeo., 123, 3617–3632, https://doi.org/10.1029/2018JG004727, 2018. a
Nelson, J. A., Pérez-Priego, O., Zhou, S., Poyatos, R., Zhang, Y., Blanken, P. D., Gimeno, T. E., Wohlfahrt, G., Desai, A. R., Gioli, B., Limousin, J.-M., Bonal, D., Paul-Limoges, E., Scott, R. L., Varlagin, A., Fuchs, K., Montagnani, L., Wolf, S., Delpierre, N., Berveiller, D., Gharun, M., Belelli Marchesini, L., Gianelle, D., Šigut, L., Mammarella, I., Siebicke, L., Andrew Black, T., Knohl, A., Hörtnagl, L., Magliulo, V., Besnard, S., Weber, U., Carvalhais, N., Migliavacca, M., Reichstein, M., and Jung, M.: Ecosystem transpiration and evaporation: Insights from three water flux partitioning methods across FLUXNET sites, Global Change Biol., 26, 6916–6930, https://doi.org/10.1111/gcb.15314, 2020. a, b
NEON – National Ecological Observatory Network: Bundled data products – eddy covariance (DP4.00200.001), https://doi.org/10.48443/J9PT-M241, 2024a. a
NEON – National Ecological Observatory Network: Bundled data products – eddy covariance (DP4.00200.001), https://data.neonscience.org/data-products/DP4.00200.001 (last access: 2 October 2024), 2024b. a
NEON – National Ecological Observatory Network: Photosynthetically active radiation (PAR) (DP1.00024.001), https://data.neonscience.org/data-products/DP1.00024.001 (last access: 2 October 2024), 2024c. a
NEON – National Ecological Observatory Network: Photosynthetically active radiation (PAR) (DP1.00024.001), https://doi.org/10.48443/VMJD-YY64, 2024d. a
NEON – National Ecological Observatory Network: Relative humidity (DP1.00098.001), https://data.neonscience.org/data-products/DP1.00098.001 (last access: 2 October 2024), 2024e. a
NEON – National Ecological Observatory Network: Relative humidity (DP1.00098.001), https://doi.org/10.48443/K9VK-5K27, 2024f. a
NEON – National Ecological Observatory Network: Triple aspirated air temperature (DP1.00003.001), https://data.neonscience.org/data-products/DP1.00003.001 (last access: 2 October 2024), 2024g. a
NEON – National Ecological Observatory Network: Triple aspirated air temperature (DP1.00003.001), https://doi.org/10.48443/PDE7-K607, 2024h. a
NEON – National Ecological Observatory Network: Shortwave and longwave radiation (net radiometer) (DP1.00023.001), https://data.neonscience.org/data-products/DP1.00023.001 (last access: 2 October 2024), 2024i. a
NEON – National Ecological Observatory Network: Shortwave and longwave radiation (net radiometer) (DP1.00023.001), https://doi.org/10.48443/9QPC-5V70, 2024j. a
NEON – National Ecological Observatory Network: 2D wind speed and direction (DP1.00001.001), https://doi.org/10.48443/YEX7-7Z81, 2024k. a
NEON – National Ecological Observatory Network: 2D wind speed and direction (DP1.00001.001), https://data.neonscience.org/data-products/DP1.00001.001 (last access: 2 October 2024), 2024l. a
Niu, G.-Y., Yang, Z.-L., Mitchell, K. E., Chen, F., Ek, M. B., Barlage, M., Kumar, A., Manning, K., Niyogi, D., Rosero, E., Tewari, M., and Xia, Y.: The community Noah land surface model with multiparameterization options (Noah-MP): 1. Model description and evaluation with local-scale measurements, J. Geophys. Res., 116, D12109, https://doi.org/10.1029/2010jd015139, 2011. a
Pastorello, G., Trotta, C., Canfora, E., Chu, H., Christianson, D., Cheah, Y.-W., Poindexter, C., Chen, J., Elbashandy, A., Humphrey, M., Isaac, P., Polidori, D., Reichstein, M., Ribeca, A., van Ingen, C., Vuichard, N., Zhang, L., Amiro, B., Ammann, C., Arain, M. A., Ardö, J., Arkebauer, T., Arndt, S. K., Arriga, N., Aubinet, M., Aurela, M., Baldocchi, D., Barr, A., Beamesderfer, E., Marchesini, L. B., Bergeron, O., Beringer, J., Bernhofer, C., Berveiller, D., Billesbach, D., Black, T. A., Blanken, P. D., Bohrer, G., Boike, J., Bolstad, P. V., Bonal, D., Bonnefond, J.-M., Bowling, D. R., Bracho, R., Brodeur, J., Brümmer, C., Buchmann, N., Burban, B., Burns, S. P., Buysse, P., Cale, P., Cavagna, M., Cellier, P., Chen, S., Chini, I., Christensen, T. R., Cleverly, J., Collalti, A., Consalvo, C., Cook, B. D., Cook, D., Coursolle, C., Cremonese, E., Curtis, P. S., D'Andrea, E., da Rocha, H., Dai, X., Davis, K. J., Cinti, B. D., Grandcourt, A. D., Ligne, A. D., De Oliveira, R. C., Delpierre, N., Desai, A. R., Di Bella, C. M., Tommasi, P. d., Dolman, H., Domingo, F., Dong, G., Dore, S., Duce, P., Dufrêne, E., Dunn, A., Dušek, J., Eamus, D., Eichelmann, U., ElKhidir, H. A. M., Eugster, W., Ewenz, C. M., Ewers, B., Famulari, D., Fares, S., Feigenwinter, I., Feitz, A., Fensholt, R., Filippa, G., Fischer, M., Frank, J., Galvagno, M., Gharun, M., Gianelle, D., Gielen, B., Gioli, B., Gitelson, A., Goded, I., Goeckede, M., Goldstein, A. H., Gough, C. M., Goulden, M. L., Graf, A., Griebel, A., Gruening, C., Grünwald, T., Hammerle, A., Han, S., Han, X., Hansen, B. U., Hanson, C., Hatakka, J., He, Y., Hehn, M., Heinesch, B., Hinko-Najera, N., Hörtnagl, L., Hutley, L., Ibrom, A., Ikawa, H., Jackowicz-Korczynski, M., Janoš, D., Jans, W., Jassal, R., Jiang, S., Kato, T., Khomik, M., Klatt, J., Knohl, A., Knox, S., Kobayashi, H., Koerber, G., Kolle, O., Kosugi, Y., Kotani, A., Kowalski, A., Kruijt, B., Kurbatova, J., Kutsch, W. L., Kwon, H., Launiainen, S., Laurila, T., Law, B., Leuning, R., Li, Y., Liddell, M., Limousin, J.-M., Lion, M., Liska, A. J., Lohila, A., López-Ballesteros, A., López-Blanco, E., Loubet, B., Loustau, D., Lucas-Moffat, A., Lüers, J., Ma, S., Macfarlane, C., Magliulo, V., Maier, R., Mammarella, I., Manca, G., Marcolla, B., Margolis, H. A., Marras, S., Massman, W., Mastepanov, M., Matamala, R., Matthes, J. H., Mazzenga, F., McCaughey, H., McHugh, I., McMillan, A. M. S., Merbold, L., Meyer, W., Meyers, T., Miller, S. D., Minerbi, S., Moderow, U., Monson, R. K., Montagnani, L., Moore, C. E., Moors, E., Moreaux, V., Moureaux, C., Munger, J. W., Nakai, T., Neirynck, J., Nesic, Z., Nicolini, G., Noormets, A., Northwood, M., Nosetto, M., Nouvellon, Y., Novick, K., Oechel, W., Olesen, J. E., Ourcival, J.-M., Papuga, S. A., Parmentier, F.-J., Paul-Limoges, E., Pavelka, M., Peichl, M., Pendall, E., Phillips, R. P., Pilegaard, K., Pirk, N., Posse, G., Powell, T., Prasse, H., Prober, S. M., Rambal, S., Rannik, U., Raz-Yaseef, N., Rebmann, C., Reed, D., Dios, V. R. D., Restrepo-Coupe, N., Reverter, B. R., Roland, M., Sabbatini, S., Sachs, T., Saleska, S. R., Sánchez-Cañete, E. P., Sanchez-Mejia, Z. M., Schmid, H. P., Schmidt, M., Schneider, K., Schrader, F., Schroder, I., Scott, R. L., Sedlák, P., Serrano-Ortíz, P., Shao, C., Shi, P., Shironya, I., Siebicke, L., Šigut, L., Silberstein, R., Sirca, C., Spano, D., Steinbrecher, R., Stevens, R. M., Sturtevant, C., Suyker, A., Tagesson, T., Takanashi, S., Tang, Y., Tapper, N., Thom, J., Tomassucci, M., Tuovinen, J.-P., Urbanski, S., Valentini, R., van der Molen, M., van Gorsel, E., van Huissteden, K., Varlagin, A., Verfaillie, J., Vesala, T., Vincke, C., Vitale, D., Vygodskaya, N., Walker, J. P., Walter-Shea, E., Wang, H., Weber, R., Westermann, S., Wille, C., Wofsy, S., Wohlfahrt, G., Wolf, S., Woodgate, W., Li, Y., Zampedri, R., Zhang, J., Zhou, G., Zona, D., Agarwal, D., Biraud, S., Torn, M., and Papale, D.: The FLUXNET2015 dataset and the ONEFlux processing pipeline for eddy covariance data, Sci. Data, 7, 225, https://doi.org/10.1038/s41597-020-0534-3, 2020. a
Perez-Priego, O., Katul, G. G., Reichstein, M., El-Madany, T. S., Ahrens, B., Carrara, A., Scanlon, T. M., and Migliavacca, M.: Partitioning Eddy Covariance Water Flux Components Using Physiological and Micrometeorological Approaches, J. Geophys. Res.-Biogeo., 123, 3353–3370, https://doi.org/10.1029/2018JG004637, 2018. a
Rafi, Z., Merlin, O., Le Dantec, V., Khabba, S., Mordelet, P., Er-Raki, S., Amazirh, A., Olivera-Guerra, L., Ait Hssaine, B., Simonneaux, V., Ezzahar, J., and Ferrer, F.: Partitioning evapotranspiration of a drip-irrigated wheat crop: Inter-comparing eddy covariance-, sap flow-, lysimeter- and FAO-based methods, Agr. Forest Meteorol., 265, 310–326, https://doi.org/10.1016/j.agrformet.2018.11.031, 2019. a
Rebmann, C., Kolle, O., Heinesch, B., Queck, R., Ibrom, A., and Aubinet, M.: Data acquisition and flux calculations, in: Eddy Covariance: A Practical Guide to Measurement and Data Analysis, edited by: Aubinet, M., Vesala, T., and Papale, D., Springer, Dordrecht, Heidelberg, London, New York, 59–83, https://doi.org/10.1007/978-94-007-2351-1, 2012. a
Reichstein, M., Falge, E., Baldocchi, D., Papale, D., Aubinet, M., Berbigier, P., Bernhofer, C., Buchmann, N., Gilmanov, T., Granier, A., Grünwald, T., Havránková, K., Ilvesniemi, H., Janous, D., Knohl, A., Laurila, T., Lohila, A., Loustau, D., Matteucci, G., Meyers, T., Miglietta, F., Ourcival, J.-M., Pumpanen, J., Rambal, S., Rotenberg, E., Sanz, M., Tenhunen, J., Seufert, G., Vaccari, F., Vesala, T., Yakir, D., and Valentini, R.: On the separation of net ecosystem exchange into assimilation and ecosystem respiration: review and improved algorithm, Global Change Biol., 11, 1424–1439, https://doi.org/10.1111/j.1365-2486.2005.001002.x, 2005. a, b
Scanlon, T. M. and Kustas, W. P.: Partitioning carbon dioxide and water vapor fluxes using correlation analysis, Agr. Forest Meteorol., 150, 89–99, https://doi.org/10.1016/j.agrformet.2009.09.005, 2010. a
Scanlon, T. M. and Sahu, P.: On the correlation structure of water vapor and carbon dioxide in the atmospheric surface layer: A basis for flux partitioning, Water Resour. Res., 44, W10418, https://doi.org/10.1029/2008WR006932, 2008. a
Scanlon, T. M., Schmidt, D. F., and Skaggs, T. H.: Correlation-based flux partitioning of water vapor and carbon dioxide fluxes: Method simplification and estimation of canopy water use efficiency, Agr. Forest Meteorol., 279, 107732, https://doi.org/10.1016/j.agrformet.2019.107732, 2019. a, b, c
Schreiner‐McGraw, A. P., Ajami, H., Anderson, R. G., and Wang, D.: Integrating partitioned evapotranspiration data into hydrologic models: Vegetation parameterization and uncertainty quantification of simulated plant water use, Hydrol. Process., 36, e14580, https://doi.org/10.1002/hyp.14580, 2022. a
Stoy, P. C., El-Madany, T. S., Fisher, J. B., Gentine, P., Gerken, T., Good, S. P., Klosterhalfen, A., Liu, S., Miralles, D. G., Perez-Priego, O., Rigden, A. J., Skaggs, T. H., Wohlfahrt, G., Anderson, R. G., Coenders-Gerrits, A. M. J., Jung, M., Maes, W. H., Mammarella, I., Mauder, M., Migliavacca, M., Nelson, J. A., Poyatos, R., Reichstein, M., Scott, R. L., and Wolf, S.: Reviews and syntheses: Turning the challenges of partitioning ecosystem evaporation and transpiration into opportunities, Biogeosciences, 16, 3747–3775, https://doi.org/10.5194/bg-16-3747-2019, 2019. a
Thomas, C. K., Martin, J., Goeckede, M., Siqueira, M., Foken, T., Law, B., Loescher, H., and Katul, G. G.: Estimating daytime subcanopy respiration from conditional sampling methods applied to multi-scalar high frequency turbulence time series, Agr. Forest Meteorol., 148, 1210–1229, https://doi.org/10.1016/j.agrformet.2008.03.002, 2008. a, b
Wagle, P., Skaggs, T. H., Gowda, P. H., Northup, B. K., Neel, J. P. S., and Anderson, R. G.: Evaluation of Water Use Efficiency Algorithms for Flux Variance Similarity-Based Evapotranspiration Partitioning in C3 and C4 Grain Crops, Water Resour. Res., 57, e2020WR028866, https://doi.org/10.1029/2020WR028866, 2021. a, b, c
Wagle, P., Raghav, P., Kumar, M., and Gunter, S. A.: Influence of water use efficiency parameterizations on flux variance similarity-based partitioning of evapotranspiration, Agr. Forest Meteorol., 328, 109254, https://doi.org/10.1016/j.agrformet.2022.109254, 2023. a, b
Wang, K., Bastos, A., Ciais, P., Wang, X., Rodenbeck, C., Gentine, P., Chevallier, F., Humphrey, V. W., Huntingford, C., O'Sullivan, M., Seneviratne, S. I., Sitch, S., and Piao, S.: Regional and seasonal partitioning of water and temperature controls on global land carbon uptake variability, Nat. Commun., 13, 3469, https://doi.org/10.1038/s41467-022-31175-w, 2022. a
Wang, L., Good, S. P., and Caylor, K. K.: Global synthesis of vegetation control on evapotranspiration partitioning, Geophys. Res. Lett., 41, 6753–6757, https://doi.org/10.1002/2014gl061439, 2014. a
Wei, Z., Yoshimura, K., Wang, L., Miralles, D. G., Jasechko, S., and Lee, X.: Revisiting the contribution of transpiration to global terrestrial evapotranspiration, Geophys. Res. Lett., 44, 2792–2801, https://doi.org/10.1002/2016gl072235, 2017. a
Wohlfahrt, G. and Gu, L.: The many meanings of gross photosynthesis and their implication for photosynthesis research from leaf to globe, Plant Cell Environ., 38, 2500–2507, https://doi.org/10.1111/pce.12569, 2015. a
Wohlfahrt, G., Brilli, F., HÖRtnagl, L., Xu, X., Bingemer, H., Hansel, A., and Loreto, F.: Carbonyl sulfide (COS) as a tracer for canopy photosynthesis, transpiration and stomatal conductance: potential and limitations, Plant Cell Environ., 35, 657–667, https://doi.org/10.1111/j.1365-3040.2011.02451.x, 2011. a
Zahn, E.: einaraz/PartitioningMethods: Processing Eddy-Covariance Data: Five Evapotranspiration Flux Partitioning Methods (v1.0.1) [Software], Zenodo [code], https://doi.org/10.5281/zenodo.11510363, 2024. a
Zahn, E. and Bou-Zeid, E.: Partitioning of water and CO2 fluxes at NEON sites into soil and plant components: a five-year dataset for spatial and temporal analysis [dataset], Zenodo [data set], https://doi.org/10.5281/zenodo.12191876, 2024. a, b, c, d
Zahn, E., Chor, T. L., and Dias, N. L.: A Simple Methodology for Quality Control of Micrometeorological Datasets, Am. J. Environ. Eng., 6, 135–142, 2016. a
Zahn, E., Bou-Zeid, E., Good, S. P., Katul, G. G., Thomas, C. K., Ghannam, K., Smith, J. A., Chamecki, M., Dias, N. L., Fuentes, J. D., Alfieri, J. G., Kwon, H., Caylor, K. K., Gao, Z., Soderberg, K., Bambach, N. E., Hipps, L. E., Prueger, J. H., and Kustas, W. P.: Direct partitioning of eddy-covariance water and carbon dioxide fluxes into ground and plant components, Agr. Forest Meteorol., 315, 108790, https://doi.org/10.1016/j.agrformet.2021.108790, 2022. a, b, c, d, e, f, g
Zahn, E., Ghannam, K., Chamecki, M., Moene, A. F., Kustas, W. P., Good, S., and Bou-Zeid, E.: Numerical Investigation of Observational Flux Partitioning Methods for Water Vapor and Carbon Dioxide, J. Geophys. Res.-Biogeo., 129, e2024JG008025, https://doi.org/10.1029/2024JG008025, 2024. a, b, c, d, e, f, g, h
Zhou, S., Yu, B., Zhang, Y., Huang, Y., and Wang, G.: Partitioning evapotranspiration based on the concept of underlying water use efficiency, Water Resour. Res., 52, 1160–1175, https://doi.org/10.1002/2015WR017766, 2016. a
- Abstract
- Introduction
- Study area and input data
- Methods
- Description of the final dataset
- Comparison of the partitioning approaches
- Exploring research opportunities with a flux-partitioning dataset
- Code and data availability
- Conclusions
- Appendix A: Diurnal cycle of
- Author contributions
- Competing interests
- Disclaimer
- Acknowledgements
- Review statement
- References
- Supplement
- Abstract
- Introduction
- Study area and input data
- Methods
- Description of the final dataset
- Comparison of the partitioning approaches
- Exploring research opportunities with a flux-partitioning dataset
- Code and data availability
- Conclusions
- Appendix A: Diurnal cycle of
- Author contributions
- Competing interests
- Disclaimer
- Acknowledgements
- Review statement
- References
- Supplement