the Creative Commons Attribution 4.0 License.
the Creative Commons Attribution 4.0 License.
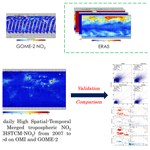
The global daily High Spatial–Temporal Coverage Merged tropospheric NO2 dataset (HSTCM-NO2) from 2007 to 2022 based on OMI and GOME-2
Hongrui Gao
Xuancen Liu
Pravash Tiwari
Remote sensing based on satellites can provide long-term, consistent, and global coverage of NO2 (an important atmospheric air pollutant) as well as other trace gases. However, satellites often miss data due to factors including but not limited to clouds, surface features, and aerosols. Moreover, as one of the longest continuous observational platforms of NO2, the Ozone Monitoring Instrument (OMI) has suffered from missing data over certain rows since 2007, significantly reducing its spatial coverage. This work uses the OMI-based tropospheric NO2 (OMNO2) product as well as a NO2 product from the Global Ozone Monitoring Experiment-2 (GOME-2) in combination with machine learning (eXtreme Gradient Boosting – XGBoost) and spatial interpolation (data-interpolating empirical orthogonal function – DINEOF) methods to produce the 16-year global daily High Spatial–Temporal Coverage Merged tropospheric NO2 dataset (HSTCM-NO2; https://doi.org/10.5281/zenodo.10968462; Qin et al., 2024), which increases the average global spatial coverage of NO2 from 39.5 % to 99.1 %. The HSTCM-NO2 dataset is validated using upward-looking observations of NO2 (multi-axis differential optical absorption spectroscopy – MAX-DOAS), other satellites (the Tropospheric Monitoring Instrument – TROPOMI), and reanalysis products. The comparisons show that HSTCM-NO2 maintains a good correlation with the magnitudes of other observational datasets, except for under heavily polluted conditions (> 6 × 1015 ). This work also introduces a new validation technique to validate coherent spatial and temporal signals (empirical orthogonal function – EOF) and confirms that HSTCM-NO2 is not only consistent with the original OMNO2 data but in some parts of the world also effectively fills in missing gaps and yields a superior result when analyzing long-range atmospheric transport of NO2. The few differences are also reported to be related to areas in which the original OMNO2 signal was very low, which has been shown elsewhere but not from this perspective, further confirming that applying a minimum cutoff to retrieved NO2 data is essential. The reconstructed data product can effectively extend the utilization value of the original OMNO2 data, and the data quality of HSTCM-NO2 can meet the needs of scientific research.
- Article
(15660 KB) - Full-text XML
- BibTeX
- EndNote
The sum of nitrogen dioxide (NO2) and nitrogen oxide, hereafter referred to as nitrogen oxides (NOx), plays several important roles in tropospheric chemistry (Eriksson, 1952; Levy, 1972; Crutzen, 1973, 1979; Fishman et al., 1979; Logan et al., 1981), specifically with respect to tropospheric ozone (Sillman et al., 1990) and nitrate aerosol (X. Lu et al., 2021), which indirectly influence radiative forcing by scattering downward-propagating visible light (Richter et al., 2005) and enhancing absorption of black carbon aerosols (Tiwari et al., 2023), and the concentration of tropospheric OH indirectly influences both methane and carbon monoxide (Lu and Khalil, 1993; Spivakovsky et al., 2000). During the daytime, under low-pollution and low-cloud conditions, the photochemical cycle of NOx can be scaled somewhat stably to NO2, allowing observations of NO2 to be an indicator of NOx concentrations (D. Schaub et al., 2006). Under more heavily polluted conditions, such a relationship can also be established, although it is found to vary in space and month by month (Qin et al., 2023; Li et al., 2023). Due to its rapid reactivity with water vapor, NOx forms nitric acid and contributes directly to acid rain (Wang et al., 2024). Additionally, NOx has been shown to have adverse effects on human health (Liu et al., 2016), specifically as an irritant of the respiratory system and via impacts on respiratory diseases when inhaled at high levels (Manisalidis et al., 2020).
The differential optical absorption spectroscopy (DOAS) method is used extensively to retrieve total column amounts of trace gases such as NO2 and others based on UV–Vis measurements of satellite spectrometers (Eskes and Boersma, 2003). The DOAS technique is based on the wavelength-dependent absorption of light over a specified light path, and it leads to the application of continuous monitoring of tropospheric pollution levels from space (Platt and Stutz, 2008). Initially applied to ground-based upward-looking instruments (i.e., multi-axis differential optical absorption spectroscopy – MAX-DOAS, Wagner et al., 2004), nowadays satellite-based measurements are proven to offer reliable inversions of column NO2 when compared with ground-based measurements (Bauer et al., 2012; Wang et al., 2017; Ialongo et al., 2020), with the errors commonly within a 20 % bound and nearly always within a 40 % bound (Boersma et al., 2004; Irie et al., 2012; Wang et al., 2017; Compernolle et al., 2020; Pinardi et al., 2020; C. Wang et al., 2020; Verhoelst et al., 2021).
Satellite observations offer the advantages of wide spatial and long-term temporal coverage (Streets et al., 2013), which can help fill spatial gaps between ground-based observations, and they do so using a single platform without the need to calibrate multiple individual machines (Kolle et al., 2021). Starting nearly 2 decades ago, and continuing today, an array of different satellites has been monitoring global tropospheric NO2 distributions, including GOME (from 1995 to 2003) aboard ERS-2, SCIAMACHY (from 2002 to 2012) aboard Envisat, the Ozone Monitoring Instrument (OMI) (from 2004) aboard EOS-AURA, the Global Ozone Monitoring Experiment-2 (GOME-2) (from 2006) aboard MetOp, and the Tropospheric Monitoring Instrument (TROPOMI) (from 2017) aboard Sentinel-5P (Bovensmann et al., 1999; Laan et al., 2001; Richter and Burrows, 2002; Veefkind et al., 2012; Munro et al., 2016). As a result, there have been useful products relating to estimating surface or near-surface NO2 emissions (Wang et al., 2012; Li et al., 2021) and detecting the long-term or short-term change in NO2 (van der A et al., 2006; Cooper et al., 2022).
NOx is emitted any time there is a high-temperature reaction that occurs within the air (Echterhof and Pfeifer, 2011). For this reason, most sources are related to anthropogenic combustion of fossil fuels, biomass, and even forests, as well as a small amount from natural sources induced by lightning (Sun et al., 2018; Z. Lu et al., 2021; Li et al., 2022). Emissions are frequently computed using a bottom-up approach, where economic, population, and other factors are merged with an activity coefficient associated with each parameter and applied on average over space and time (Li et al., 2017; Xu et al., 2024). Recent work has looked at using the satellite observations of NO2 above and applying them on a grid-by-grid and day-by-day basis to attribute emissions to different types of industrial sources, population centers, power generation, transportation, residential uses, agriculture, and natural sources (Li et al., 2023; Qin et al., 2023). The current best estimates vary by considerable amounts from each other in space and time (Wang et al., 2021) and account for both natural (Deng et al., 2021) and human-based factors (according to EDGAR and MEIC; Crippa et al., 2023). There is controversy about the amounts that lightning and microbial activity may or may not contribute (Logan, 1983).
Vertical column densities (VCDs) of tropospheric NO2 retrieved from satellite-based instruments provide plentiful data under relatively clean and clear atmospheric conditions but have many missing pixels in both time and space due to a variety of factors, including very bright surfaces, clouds, and aerosols (Lin et al., 2014; Xia and Jia, 2022). One of the underlying sources of error is related to the air mass factor (AMF), which allows conversion from a slant column to a vertical column that is highly sensitive to cloud and aerosol layer height (Leitão et al., 2010), aerosol absorption (Lin et al., 2014; Cooper et al., 2019), and the spatial and temporal distribution of NOx emissions (Qin et al., 2023; Li et al., 2023), which can lead to both uncertainties and biases in retrieval (Bousserez, 2014). For these reasons, pixels known to be impacted by clouds are usually filtered before analysis. However, other impacted pixels may not be properly filtered, leading to other issues. Similarly, for some older satellites, due to their orbit and swath width, this requires 3, 6, and 1.5 d for GOME, SCIAMACHY, and GOME-2, respectively, to cover the whole globe, with additional missing pixels on a day-by-day basis. OMI, which is carried on a near-polar, Sun-synchronous satellite, is the world's first sensor with daily global coverage of NO2 since 2004. However, in 2007, a reduction in OMI's spatial coverage occurred due to an equipment malfunction, called the row anomaly (RA), which began affecting just two rows of data in June 2007 but gradually worsened over time (Torres et al., 2018). The absence of data presently affects both short-term estimation of air quality and long-term quantitative analysis (Duncan et al., 2013; van Geffen et al., 2020), although OMI is still useful for the detection of extreme events (S. Wang et al., 2020, 2021; Deng et al., 2021). Due to 19 years of continuous observations, OMI is a very widely used sensor in the field of atmospheric trace gas research, and finding ways to comprehensively and reasonably fill these missing pixels would allow its usefulness to be extended into other fields (de Hoogh et al., 2019; He et al., 2020; Wu et al., 2021; Wei et al., 2022; Shao et al., 2023; J. Liu et al., 2024).
There are many existing approaches to fill missing data from satellite-based platforms, including interpolation techniques – geostatistical (e.g., kriging), deterministic (e.g., inverse-distance-weighted, thin-plate splines), and hybrid (e.g., regression kriging) (Abdulmanov et al., 2021; Achite et al., 2024) – and machine learning techniques such as random forest (Sanabria et al., 2013). As there is a strong correlation in terms of both the geospatial relationships and the retrieval approaches used to determine the VCDs of the tropospheric NO2 obtained by different sensors (Park et al., 2020), issues of spatial–temporal correlation need to be carefully taken into consideration, something that these previous approaches may not have fully considered. In this work, machine learning and data-interpolating empirical orthogonal function (DINEOF) methods are selected to carry out the reconstruction, which takes advantage of both machine learning and pattern recognition in tandem, as demonstrated by previous studies reconstructing satellite chlorophyll-a data (Wang and Liu, 2013; Chang et al., 2017; Hilborn and Costa, 2018; Park et al., 2020), filling in missing parts of both sea and land surface temperature data (Alvera-Azcárate et al., 2009; Zhou et al., 2017) and analyzing sea surface salinity data (Alvera-Azcárate et al., 2016; Chen et al., 2022), and Jiang et al. (2022) used DINEOF to reconstruct the XCO2 data of OCO-2 and OCO-3 by fusing the two, effectively improving the spatiotemporal coverage of XCO2 products.
This research aims to accurately and precisely reconstruct the tropospheric NO2 VCD at a daily time resolution and a grid-by-grid spatial resolution using OMI for 2007–2022. With the support of the global daily High Spatial–Temporal Coverage Merged tropospheric NO2 dataset (HSTCM-NO2), model validation, spatial distribution analysis, and temporal change monitoring can be carried out. Also, HSTCM-NO2 can be an ideal tool for improving numerical prediction of air quality and the AMF, contributing to a better understanding of typical chemical and dynamic processes in the atmosphere and future remote sensing retrieval improvements.
2.1 Tropospheric NO2 products
2.1.1 OMI tropospheric NO2 (OMNO2)
OMI is a UV–Vis charge-coupled device (CCD) spectrometer aboard the Aura satellite, which was launched on 15 July 2004 into a Sun-synchronous orbit with a local Equator-crossing time of approximately 13:45 local time. OMI covers a spectrum of 270–500 nm with a spectral resolution between 0.42 and 0.63 nm and a nominal spatial resolution of 13 km × 24 km at nadir (Boersma et al., 2008; Foret et al., 2014), providing coverage over 740 wavelength bands along the satellite track and global coverage via 14 orbits per day.
OMI data are processed and archived at NASA's Goddard Earth Sciences Data and Information Services Center (GES DISC). This work specifically uses the daily L3 global gridded data product that corresponds to the OMNO2 standard product, and the adopted L3 grid is a 0.25° × 0.25° grid in latitude and longitude.
2.1.2 GOME-2 tropospheric NO2
GOME-2 is an optical spectrometer aboard the MetOp satellites. MetOp-A was launched on 19 October 2006, MetOp-B was launched on 17 September 2012, and MetOp-C was launched on 7 November 2018. GOME-2 senses backscattered and reflected radiance in the UV–Vis part of the spectrum from 240 to 790 nm, with a high spectral resolution between 0.26 and 0.51 nm covering 4096 spectral points from four detector channels (Fioletov et al., 2013). The spatial resolution varies from 80 km × 40 km to 40 km × 40 km and provides daily near-global coverage at the Equator (S. Liu et al., 2019).
The GOME Data Processor version 4.8 is used for MetOp-A and MetOp-B, while version 4.9 is used for MetOp-C. Datasets were resampled at a uniform gridding of 0.25° × 0.25° using the HARP (https://github.com/stcorp/harp, last access: 12 November 2024) tool.
2.1.3 TROPOMI NO2
TROPOMI was launched on 13 October 2017 aboard the polar-orbiting Sentinel-5P satellite. It measures solar radiation reflected by and emitted from Earth and provides measurements of atmospheric traces, including NO2, O3, SO2, HCHO, CH4, and CO, as well as cloud and aerosol properties. NO2 retrieval is performed using the visible band (400–496 nm), which has a spectral resolution and sampling of 0.54 and 0.20 nm. The instrument operates in a push-broom configuration with a swath width of approximately 2600 km on Earth's surface. The typical pixel size (near nadir) for NO2 is 7 km × 3.5 km, which was reduced to 5.5 km × 3.5 km in 2019 (Ialongo et al., 2020; Ludewig et al., 2020). This work specifically uses the level-2 NO2 data products based on version 1.4 and an applied quality filter of qa_value > 0.75 (van Geffen et al., 2019). The TROPOMI data products are also resampled to a spatial resolution of 0.25° × 0.25° using the HARP tool.
2.2 Auxiliary data
2.2.1 Land cover type data
The Moderate Resolution Imaging Spectroradiometer (MODIS) land cover type (MCD12Q1) provides data that map global land cover at 500 m spatial resolution annually derived from six different classification schemes. The maps were created from classifications of spectra-temporal features derived using the BIOME-Biogeochemical Cycles approach described by Running (1993).
2.2.2 MAX-DOAS data
MAX-DOAS is a passive-DOAS ground-based remote sensing observation technology using solar scattering as its light source. MAX-DOAS technology can be used to detect trace gases in the troposphere and has been widely applied in related fields. This instrument can observe scattered sunlight from different perspectives, thus showing high sensitivity to trace gases in the troposphere, specifically using low-elevation observations as the measurement intensity and zenith measurements as the reference intensity. The Beer–Lambert law can be used to determine the total molecular amount of specific gas categories along the optical path (subtracting zenith concentrations from non-zenith measurements), which is known as differential slant column concentration. The tropospheric vertical column density is inverted using a radiative transfer model. This work specifically adopts the QA4ECV NO2 MAX-DOAS reference datasets, which include 10 sites. The sites are categorized into three types (suburban, urban, and rural) based on their location, and three sites of different types are used here. The information on the three sites is listed in Table 2.
2.2.3 Reanalysis meteorological data
Reanalysis combines model data with observations from across the world into a globally complete and consistent dataset using a model of the atmosphere based on the laws of physics and chemistry. For this reason, this work uses the fifth-generation ECMWF reanalysis (ERA5) for 12 specific meteorological parameters as given in Table 1. The dataset used has an hourly temporal resolution and a 0.25° × 0.25° spatial resolution. The meteorological products in this work are used at the following pressure levels: 100, 200, 500, 700, 850, 925, and 1000 hPa. The actual weightings used in this work are computed using principal component analysis (PCA) and rely nearly fully on the data from 850 hPa and below at roughly equal weights.
This study also uses the fourth-generation ECMWF reanalysis (EAC4), specifically its modeled NO2 column values, which are used as a means of comparison against the NO2 fields generated in this work. EAC4 data have a spatial resolution of 0.25° × 0.25° and a temporal resolution of 3 h. In this work, a vertical column density of tropospheric NO2 is derived from EAC4 and is used for comparison.
2.3 XGBoost (eXtreme Gradient Boosting) algorithm
A gradient-boosting framework is used by the decision-tree-based ensemble machine learning approach known as XGBoost (Chen and Guestrin, 2016). This method employs a more regularized model formalization than other techniques (Cisty and Soldanova, 2018; Zhang et al., 2018), with greater control against overfitting compared with gradient-boosting decision tree (GBDT) approaches (Dong et al., 2022). Similarly to the random forest algorithm, XGBoost needs its hyperparameters tuned (Kapoor and Perrone, 2021). It has a more intricate structure and adds regularization components to the loss function to prevent overfitting so that it can handle complicated data better. Therefore, XGBoost is a better option for working with vast volumes of data and multidimensional affecting factors like NO2 gap-filling. Additionally, XGBoost has been used to estimate pollutants, and its results outperform those of certain other statistical and machine learning methods (Reid et al., 2015; Just et al., 2018; Zhai and Chen, 2018; Fan et al., 2020). Table 1 shows the data used in this research, which are input into the machine learning model.
2.4 SHAP (SHapley Additive exPlanation) values
SHAP values quantitatively represent the conditional expected value function of the machine learning model, implying the average contribution of a feature to a prediction (Lloyd Shapley, 1952). The use of a blackbox model, such as XGBoost in this work, necessitates an explanatory model, in contrast to interpretable algorithms (i.e., Cohen et al., 2011). According to each feature's marginal contribution, SHAP distributes the overall gain in terms of both negative and positive contributions. In this work, SHAP values are used to quantify the importance of features, as shown in Fig. 2.
2.5 DINEOF
DINEOF is used in this work to reconstruct the missing points in the spatiotemporal field of NO2. This method relies on an empirical orthogonal function (EOF) decomposition in space and a principal component (PC) decomposition in time that identifies spatial–temporal domains of maximal variation following Cohen (2014). The method allows the assignment of a prediction under conditions in time and/or space that are missing observational data. By using the weighted EOFs and PCs in an iterative manner, missing data points can be re-synthesized based on a weighting of the various underlying orthogonal basis functions. The number of iterations which minimize the cross-validation error is used to obtain the best-reconstructed data. For a more detailed description of the overall approach, see Beckers and Rixen (2003) and Alvera-Azcárate et al. (2009). In this work, the number of data filled using this approach range from 27 % to 35 % on a year-by-year basis, as given in Table 3.
2.6 Validation strategy
In order to analyze the performance of the reconstructed dataset, this work not only uses cross-validation based on the original data themselves but also refers to the observations from TROPOMI, MAX-DOAS, and the EAC4 reanalysis product mentioned above. The root mean square error (RMSE), Pearson correlation coefficient (R), normalized mean bias (NMB), and mean absolute error (MAE) are all used in the validation process.
Also, as an important and innovative approach, EOF decomposition is performed on the three-dimensional observed and HSTCM-NO2 fields. These values are compared to ensure that the maximum changes in the spatial and temporal signals are consistent with the original observations. EOF is an exploratory technique for multivariate data, which is in essence an eigenvalue problem aiming at explaining and interpreting the variability in the data. Till now, EOF has been introduced into data analysis of satellite-based remote sensing to estimate the spatiotemporal distribution characteristics of pollutants such as HCHO (Kim et al., 2014), CO (Baek and Kim, 2011), aerosols (Cohen et al., 2017), and NO2 (Li et al., 2023).
2.7 Method selection
The goal of this work is to use all available day-by-day and pixel-by-pixel NO2 column data from both OMI and GOME-2 in tandem to reconstruct a consistent global NO2 column product with the highest possible coverage. Machine learning used in this work can only predict OMNO2 data, which also have GOME-2 data at corresponding positions in space and time. For this reason, this work introduces DINEOF to reconstruct data in locations where both OMI and GOME-2 have no values but where data exist at other times or in nearby locations in space.
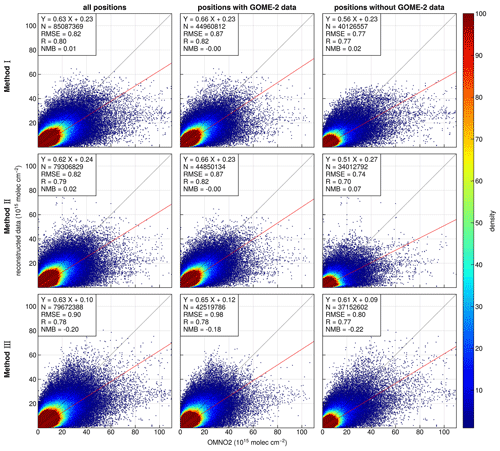
Figure 1Cross-validation of the three methods between the reconstructed data and masked OMNO2 in 2007.
Since DINEOF and machine learning have not previously been used in tandem for this type of issue, a critical component of the methodology is to quantify the impact of using the two approaches individually, in tandem, and, if in tandem, in what order. To first determine which sets of methods are best suited for this work, a subset of data from 2007 is selected. Furthermore, due to the issue of the row anomaly, a second comparison dataset from 2013 is used as a mask. In this way, data from 2007 which are masked by data from 2013 will be separated for validation, and the missing data will be the major difference assuming that the changes in the climatology are not significant. Therefore, the following methods are applied, as shown in Fig. 1:
- I.
First, XGBoost is used to predict OMNO2 data based on GOME-2 data. Subsequently, DINEOF is applied to fill the remaining gaps.
- II.
DINEOF is first used to fill the gaps in GOME-2 data. This is then followed by XGBoost prediction based on the reconstructed GOME-2 dataset.
- III.
DINEOF is used solely to fill in gaps in OMNO2.
The reconstructed dataset is evaluated based on comparison between the masked data from 2007 and the results. Additionally, in order to verify whether and how the absence of GOME-2 values affects the prediction accuracy, further partitioning of the dataset based on the presence or absence of GOME-2 values is performed. All the results are given in Fig. 1, where the row is the method and the column is the amount of GOME-2 data used.
As shown in Fig. 1, the complete or partial datasets reconstructed by Method (I) all have a maximum R value and minimum RMSE and NMB in the same scenario. Meanwhile, by comparing Column 2 and Column 3, it is obvious that the presence of GOME-2 observations can greatly improve the accuracy of the reconstruction and have an impact on the fitted slopes (especially in the cases of Methods I and II). From Row 3, it can be seen that DINEOF has universality but does not have an outstanding performance. Therefore, it is only necessary to use machine learning for prediction in positions with values obtained from GOME-2 and DINEOF for filling in positions that do not contain GOME-2 data. In conclusion, in order to obtain optimal results, Method (I) will be chosen as the reconstruction scheme in this work, which is consistent with the idea that using the highest amount of actual observational data possible best supports the machine learning approach.
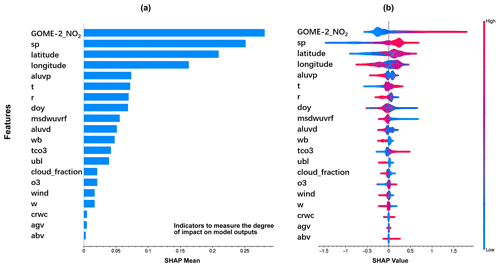
Figure 2Feature importance ranking (a) and scatterplot of feature density for each parameter of XGBoost (b), represented as a bee swarm. Specifically, each row represents a feature, and the order of arrangement is determined by the importance of the feature calculated in the previous step. The horizontal coordinate is the SHAP value, where the sign of the value indicates the direction of the contribution of that feature. Each point in each row represents a single sample, and the color of the point indicates the magnitude of the feature value (high in red and low in blue).
3.1 Reconstruction process and model evaluation
3.1.1 Quantifying the importance of individual features
The results of the SHAP value and its statistics are given in Fig. 2 based on global training data from February 2019 as an example.
The 20 features with the highest contributions are provided. Data from GOME-2_NO2 have both the highest overall mean contribution and the largest absolute contribution (up to 1.8), which are larger than the absolute values of all other contributing factors and the only significant source in terms of positive contribution (greater than 0.6). This result is consistent with the fact that GOME-2_NO2 is the base observation upon which machine learning acts. The second most significant driving feature is the surface pressure, which has both the second highest mean and the second largest absolute contribution (down to −1.5) of any factor. This is consistent with the fact that human settlements tend to be at lower elevations in general and that changes in pressure tend to accompany changes in the rates of transport and chemical activity of NO2 in situ (S. Wang et al., 2020; Li et al., 2023). Below this there are some interesting patterns in which some species contribute more to the mean SHAP value but not necessarily to the extreme SHAP value, meaning that the global and local contribution factors are different in different locations. As expected, latitude, longitude, day of year, and downwelling UV radiation are all relatively important in different areas, which is consistent with the highly heterogenous nature of NO2 emissions, different driving forces which impact the ratio of NO to NO2 emissions within NOx, issues of geospatial change, and processing once NO2 is in the atmosphere. These factors are sufficient for capturing the presence of pollution sources within specific pixels, and therefore it is necessary to not only be able to predict the long-term signal but also to account for short-term changes of a sudden nature.
3.1.2 Separation of models over ocean and land
Globally, the distribution of NO2 observed by satellites is not balanced, due to the fact that NO2 has a relatively short lifetime and the vast majority of its emissions occur over land in and around areas of anthropogenic disturbance. Furthermore, if major shipping lanes and areas of significant downwind transport are excluded, NO2 generally has lower values over the sea compared to land. On top of this, the surface absorption profile over the oceans is different from land, which may further contribute to differences in the column interpretation. This section quantitatively explores the impact of separating those pixels over the sea from those over land in terms of training the machine learning model and works to quantify any reduction in the overall error rate of the models between the separated and unified approaches.
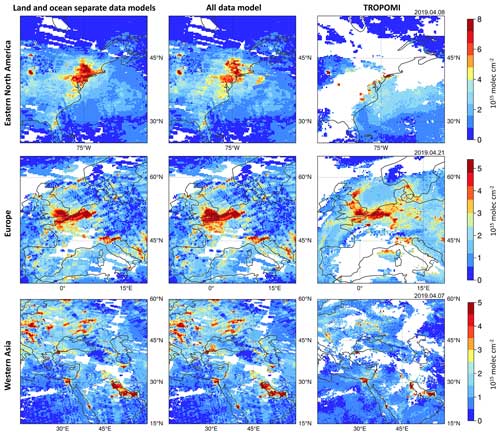
Figure 3Prediction results with and without prior knowledge versus TROPOMI observations over three regions (eastern North America, Europe, and western Asia).
The effects of separating land from the ocean models are demonstrated clearly over April 2019 in Fig. 3. First, the high values of NO2 observed over the western Atlantic Ocean found in all the data models are no longer observed in the land and separate ocean data models, which is consistent with TROPOMI NO2 observations. Over western Europe, the high values off Scotland as confirmed by TROPOMI still remain in the land and separate ocean data model cases, while the unusually high values in all the data model cases are reduced to more reasonable values compared to the observations from TROPOMI over the areas between Spain and France and between the UK and France. Even with the separation, there are still erroneously high values between the UK and Ireland, and in the eastern Atlantic these are not resolved. The third row shows the distribution of NO2 concentrations in western Asia. In the TROPOMI observations, high values are only observed on the southwestern side of the Arabian Peninsula over land and mostly on land over northern Türkiye, except for the Bosporus Strait, which is consistent with what is understood and what the separate land and ocean data models are able to capture, while all the data models misrepresent these values as being higher than the observations suggest. Overall, there is a considerable improvement observed over the near-sea areas in terms of retaining enhancement, where this is justified, and reducing enhancement, where this is not justified, by using the separately trained models. However, there are still inconsistencies which are not resolved.
3.1.3 Evaluation of machine learning
After applying XGBoost and the prior knowledge mentioned above, Fig. 4 demonstrates the reconstructed results and compares them with the original data on a pixel-by-pixel basis in 2007.
Of the results predicted by XGBoost, the MAE and RMSE of the results located over water are slightly lower than those located over land. The predicted results over East Asia, Europe, and eastern North America show a higher correlation with the observations, indicating that the variability is captured better over regions where the vertical column density of NO2 is larger. For these reasons, the machine learning model is trained separately over both land and the ocean, with training done on a month-by-month basis. The results of this fitting are given in Fig. 5, demonstrating the time series of the statistics from 2013 to 2015.
As demonstrated, the RMSE and MAE of the ocean model are both always higher than and less temporally variable than those of the land model. Also, unlike the land model, which shows improved performance during winter, the ocean model does not experience this seasonal improvement. This indicates that these errors scale in terms of both the magnitude, which is higher over land, and the response to the retrieval algorithms themselves, which have different amounts of error over bright and dark surfaces. Additionally, the abundance of surface-based measurements over land initially enhanced the accuracy of these retrievals. The correlation over time of the land model is slightly higher than that of the ocean model, indicating that the data predicted by the land model may have a lower uncertainty that is possibly due to better a priori data, a better-defined AMF over land, or better overall retrieval over land as compared with water (Richter et al., 2011; Streets et al., 2013; Lamsal et al., 2021).
The quality of the land model fit shows a strong decrease over a period of 1 to 3 months every year, experiencing both interannual and intra-annual variations, while the ocean model shows a weaker decrease in the fit for a few months in two of the years and no change in the other years. This indicates that there must be a few different forces acting on the fits, including some that are clearly seasonal in nature with only a small variation (air temperature), while others are more variable (UV radiation or absorption aerosol optical depth (AAOD), which is consistent with the results of S. Wang et al. (2020) and Li et al. (2023). On the one hand, the UV intensity is generally lowest in December and January, leading to an increase in the residence time of NO2 in the atmosphere, and is generally highest in May and June, leading to a decrease in the residence time. However, the UV radiation itself is also modified by the effects of both clouds and absorbing aerosols. Cloud coverage tends to affect a larger percentage of the ocean surface compared to land. However, absorbing aerosols have a more significant impact over land, which contributes to the findings mentioned earlier. The effects of temperature tend to peak differently from those of UV radiation, but these effects tend to be climatologically more similar from year to year, given that the years analyzed do not contain any El Niño or La Niña types of patterns. In addition to this, the vertical column density of NO2 itself also changes from month to month, with the peak values over land occurring in December and January and the magnitudes of the peak and peak month varying from year to year. This allows for a greater amount of differentiation between the heavily polluted and cleaner regions during this time, especially over land. As discussed previously, such high variability may lead to additional machine learning fitting issues. On the other hand, there is generally less cloud during winter, meaning more observations on a day-by-day basis and more atmospheric stability in winter and leading to less vertical and long-range transport of pollutants away from their source regions. The combination of all of these things enables the model to make more accurate predictions.
3.1.4 Reconstruction process and accuracy analysis of DINEOF
The EOF separates the data into their primary basis functions, of which there are spatial and temporal components. To test the efficacy of the EOF procedure as a function of the time length of the data used, this work has run the procedure over different time periods from a minimum of 1 month of data to a maximum of 3 years of data. The annual data, as shown in Table 3, yield the lowest overall standard deviation. This is consistent with the above results, which show that there is a clear annual peak in the NO2 columns occurring each winter and indicate that this amount of variability drives the model more than the smaller year-to-year changes in the peak or overall characteristics of NO2. This result is consistent with a year (intra-annual variability) that tends to be smaller than year-to-year variability, unless a very long time series is considered (minimum of 20–30 years) (Chowdhury, 2022) and a known extreme such as El Niño or La Niña is captured. (Deng et al., 2021). Based on the timing chosen and the results below, this work will rely on applying the DINEOF reconstruction of the dataset on a year-by-year basis.
Table 4 shows the DINEOF results for each year, with most years achieving convergence after 12 to 29 iterations. The standard deviation is shown to be lowest when analyzing data one year at a time. Interestingly, the year 2009 saw the most data loss, with more than one-third of the total data (34.3 %) lost. This indicates that both the geospatial nature of the data and the range of column loading values are important factors, in addition to the absolute amount of data reconstructed.
3.1.5 Overall analysis
The performance of reconstruction can be tested by simulating the known RA issue, wherein OMI started to lose access to specific camera angles on a swath-by-swath basis starting in 2007, leading to the appearance of missing lines of data. Since the data are otherwise in good order, a well-conditioned filling method should be able to produce data to cover these well-known and geometrically simple gaps. Five regions (East Asia, Europe, eastern North America, southern Africa, and South Asia) are used to demonstrate the effectiveness of the procedure in filling these gaps on different days in 2007.
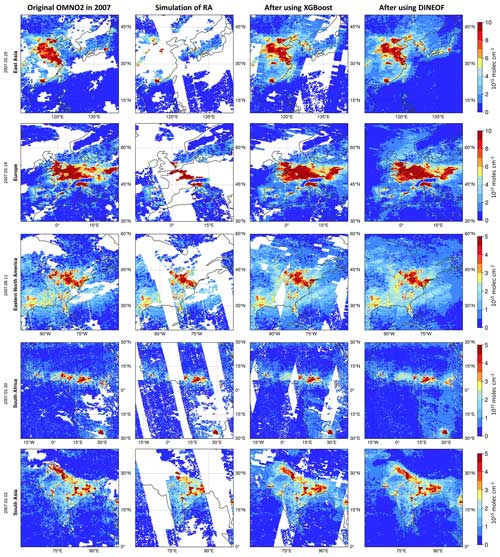
Figure 6Results of stepwise reconstruction of masked data over five regions (East Asia, Europe, eastern North America, southern Africa, and South Asia).
As shown in Fig. 6, the first column shows the original OMNO2, and the second column shows the data distribution after simulating the effect of the RA. Machine learning reconstructs the image of GOME-2 at positions with values, keeps the original observations, and only reconstructs the missing parts. After reconstruction by XGBoost, the image elements that are still missing are reconstructed using DINEOF to obtain a dataset with more than 99 % coverage. Comparing the reconstructed data with the original data, it can be found that the reconstructed results are basically consistent with the distribution of the original OMNO2, with the following two exceptions: some very high pixels observed in the EU and USA have been removed and replaced with lower-valued pixels in the reconstruction, while some moderate- and low-valued pixels in China and India have been replaced with high-valued pixels in the reconstruction. In general, the overall shapes are reasonably similar, and the transition from high to low values seems to make sense based on the values from the original OMNO2.
3.1.6 Coverage statistics
The spatial coverage of the original OMNO2 declined from about 50 % in 2007 to 35 %–40 % after 2009 due to the RA phenomenon and cloud occlusion, and it improved slightly in late 2012 (while not recovering to its previous levels). The reconstructed data however have a daily coverage of over 90 %. As shown in Fig. 7a, the original data have more gaps when the cloud volume is higher and fewer gaps when the cloud volume is smaller. The reconstructed data also show such a trend, although with a much smaller difference between the high-cloud and low-cloud periods of time, indicating that some fraction of the cloud-covered data can be reconstructed successfully, while some other amount has so much data lost that even the technique used in this work cannot fully reconstruct them.
Figure 7b shows the comparison between the original OMNO2 and HSTCM-NO2 in terms of spatial distribution. OMNO2 in the eastern part of North America, the northwestern part of South America, Europe, and the southeastern part of Asia is obviously missing, although after reconstruction all the data in the above locations are reconstructed. The reliability of their HSTCM-NO2 is verified over such land-based and near-land areas. There are a few exceptions, such as perpetually cloud-covered areas in the North Pacific and along the Equator, but in these cases there is likely no possible solution since they are covered for days in a row over huge spatial areas. Globally, on average, the 39.5 % coverage of OMNO2 increases to a 99.1 % coverage of HSTCM-NO2.
3.2 Multisource validation of HSTCM-NO2
3.2.1 Comparison with MAX-DOAS data
The original OMNO2 and HSTCM-NO2 were validated against MAX-DOAS, and the following results were obtained. The three sites used in this work are Xianghe, Uccle and Observatoire de Haute-Provence (OHP), which are classified separately as suburban, urban, and rural.
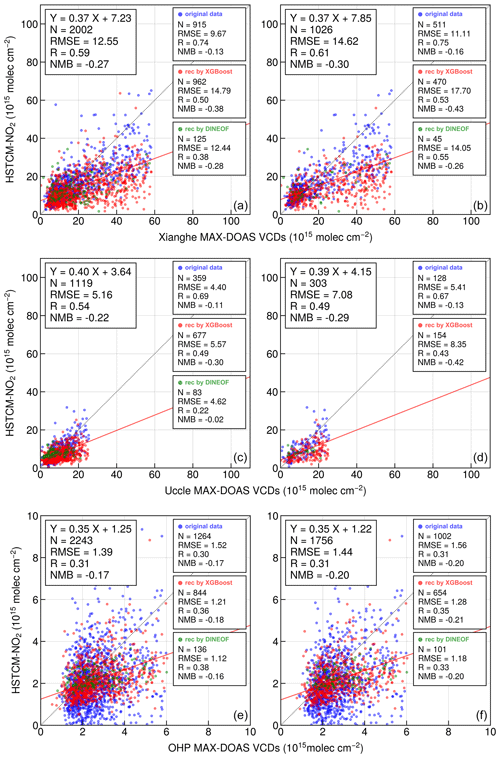
Figure 8Scatterplots of comparison between MAX-DOAS observations (Xianghe, Uccle, and OHP) and HSTCM-NO2. The figures in the left panels (a, c, and e) all use all observations of MAX-DOAS, while those in the right panels (b, d, and f) are filtered-out extreme cases. The boxes in the upper left corner summarize the statistical comparisons, while the boxes to the right of each subfigure represent the statistics of each individual reconstruction step.
Comparisons between the various different products and MAX-DOAS are shown in Fig. 8. Due to the small amount of data, there is a missing box which corresponds to a result that did not pass the p test. In all the cases, there is a sufficient number of data points to consider the fits under all-data and extreme-event-filtered-data conditions. At the sites with very high NO2 column loading (Xianghe) and moderately high NO2 column loading (Uccle), the results using both XGBoost and DINEOF together are still less good than those of the original OMI data, regardless of whether the data were filtered or unfiltered. In Xianghe this difference is even larger than in Uccle, confirming that the approaches employed here do not work very well when a substantial number of data are located at or above 6 × 1015 . However, it is clear even at these high-elevation sites that using both XGBoost and DINEOF together yields a final product that is more representative of OMI than when using only one method independently. In the case of Xianghe, using all data with XGBoost alone or using filtered data with either XGBoost or DINEOF alone yields similar results that are worse than when applying both XGBoost and DINEOF in tandem. At Uccle, applying XGBoost on its own always yields a result with a much higher R coefficient and a lower RMSE coefficient than when DINEOF is applied on its own, which is consistent across both filtered and unfiltered data. Interestingly, under the relatively cleaner conditions found at OHP, the results from applying both XGBoost and DINEOF together yield a result that is better than the result of OMI in terms of RMSE and similar in terms of R. The application of either XGBoost or DINEOF independently at this location yields results that are quite good when compared with OMI. This set of results makes it clear that, under cleaner conditions, the use of one or both of XGBoost and/or DINEOF yields benefits and can be considered trustworthy, while their combination yields a large amount of additional data and still works well. Clearly the benefits of the gap-filling and prediction are consistent with the observations under these conditions, allowing the conclusions observed above under different polluted conditions to be confirmed further.
3.2.2 Comparison with TROPOMI
Compared with OMI, TROPOMI has a higher spatial resolution and a wider swath angle, allowing improved spatial observation of tropospheric NO2, with the caveat that higher resolution may mean that some pixels are cloud-covered, whereas at lower resolution this may not be the case. For these reasons, TROPOMI NO2 is used as an external data source to allow comparison with the various products and to serve as a means of ensuring that the derived products are reasonable.
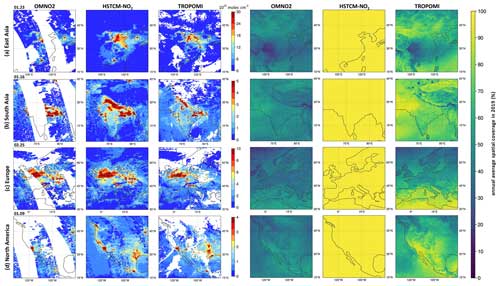
Figure 9Distribution and coverage statistics of OMI, HSTCM-NO2, and TROPOMI over East Asia (a), South Asia (b), Europe (c), and North America (d) in 2019.
The spatial distribution on 4 specific days in 2019 and the annual average coverage over East Asia, South Asia, Europe, and North America are used to compare OMNO2, HSTCM-NO2, and TROPOMI NO2, as shown in Fig. 9.
As shown in Fig. 9a, the coastal areas of China are severely affected by the RA, leading to a significant portion of the data missing in OMNO2. The reconstructed results of HSTCM-NO2 are similar to those of TROPOMI on average and in particular in Hebei, Henan, Shanxi, Shaanxi, parts of industrial Inner Mongolia, the Pearl River Delta, and even the transport corridors between China and South Korea in the East China Sea. However, there are some regions in Shandong, southern Jiangsu, Wuhan, and Shanghai where the average characteristics may be acceptable but where high and low values are too smoothed over and extremes are not well predicted by HSTCM-NO2 as compared with TROPOMI. Due to the effects of cloud cover, both OMNO2 and TROPOMI show no data over the megacities of Chongqing and Chengdu, while HSTCM-NO2 effectively solves this problem in terms of large-scale spatial averaging, with a coverage of almost 100 %. However, the fine-scale centers of the two cities are not clear in this case.
In Fig. 9b, again due to the RA, OMNO2 lacks data over New Delhi, Lahore, and other cities in central and western India. The reconstructed HSTCM-NO2 products fill this part of the data well, and the NO2 distribution shown in HSTCM-NO2 is similar to that of TROPOMI, with the major issue being that heavily polluted areas are more diffuse than in TROPOMI. In particular, the areas of northeastern India which are known to have seasonal fires at this time of the year are reflected well in HSTCM-NO2 but not in TROPOMI, possibly indicating that the information from the morning provided by GOME-2 identifies information which is missed by TROPOMI in the afternoon. The special geographical environment of the Qinghai–Tibetan Plateau has led to both high cloud cover and significant surface reflection in the region. As a result, the coverage of the OMI and TROPOMI products in the Qinghai–Tibetan Plateau region is relatively low, and HSTCM-NO2 is able to provide some amount of geospatial information, likely again from GOME-2, while filling the climatological gap.
As shown in Fig. 9c, due to the influence of the marine climate, high cloud coverage often occurs in the European region, which causes significant interference in satellite observations. The coverage of both the OMNO2 and TROPOMI products in the European region is relatively low on this day. HSTCM-NO2 has effectively reconstructed missing data in the UK from Scotland through London, most of central France, and even into Algeria and Tunisia while greatly increasing data coverage throughout Europe as a whole.
As shown in Fig. 9d, missing data in areas such as the western coast of North America, Texas, and Oklahoma have been reconstructed well. Due to the impact of the RA, the spatial coverage of OMNO2 is lower than that of TROPOMI, and the coverage of both is not ideal in high-latitude and high-altitude regions. Through the comparison of the four regions, it can be seen that HSTCM-NO2 solves this problem and has high consistency with TROPOMI NO2. It works particularly well along the western coast from San Francisco up through Vancouver, energy-producing areas from Texas through New Mexico, and in general around urban and energy-producing areas along the eastern edge of the Rockies.
As shown in Fig. 10, each day and grid which contain a value of both HSTCM-NO2 and TROPOMI NO2 are compared for 2019. The comparison consists of a total of 171 297 320 pixels and shows a reasonable fit globally, with an RMSE of 0.64, R of 0.75, and NMB of 0.09. As pointed out elsewhere in this work, at values higher than 6 × 1015 and especially at values higher than 20 × 1015 , there are some small differences in the overall shape.
3.2.3 Comparison with EAC4 data
Global results as well as results over three regions with a sufficient number of pixels with high NO2 vertical column densities (East Asia, North America, and Europe) were selected to compare the reconstruction results with EAC4 data for February 2008. The reconstructed data at a global scale contain more than 2.6 million points and have an RMSE of 1.02, R of 0.77, and NMB of −0.25. Of the three regions, East Asia has the validation results with the highest R and the lowest NMB, followed by North America and Europe, as shown in Fig. 11.
3.3 Results of EOF analysis
In order to verify the performance of HSTCM-NO2, the temporal and spatial patterns are expected to match the observed variability. Specifically, analysis was done over the time period from 2019 to 2021. The first three modes contribute 7.6 %, 2.2 %, and 2.0 % of the total original OMNO2, while they contribute 26.1 %, 4.0 %, and 3.2 % for HSTCM-NO2. This indicates that a spatial and temporal comparison using the first mode is sufficient to demonstrate the ability of HSTCM-NO2 to reproduce OMNO2, given the fact that they both contribute more than the approximated global background 5 % of the error associated with the NO2 retrieval itself. The contribution of HSTCM-NO2's first mode to the total variance indicates that the reconstructed data are missing many finer modes of variability. However, as demonstrated below, the good spatial and temporal match shows that it is able to reproduce the signal reasonably well in actuality, with the major sources of this difference being regions north of 40° N and south of 40° S, all of which tend to be relatively clean and owe the majority of their variability to noise in the retrievals themselves, which is not explicitly considered by the methods employed herein.
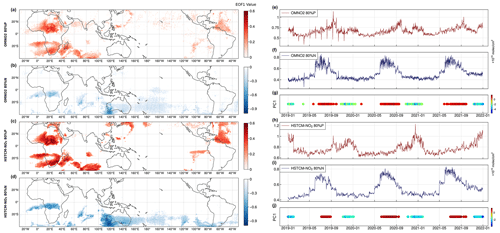
Figure 12Spatial and temporal patterns after EOF variance maximization is performed on both OMNO2 and HSTCM-NO2. EOF1 is given for OMNO2 positive (a), OMNO2 negative (b), HSTCM-NO2 positive (c), and HSTCM-NO2 negative (d). The temporal mean values of OMNO2 over the EOF1 positive region and EOF1 negative region are given in panels (e) and (f), respectively, while PC1 is given in panel (g), where red and blue represent the peaks of the positive and negative factors, respectively. Panels (h)–(j) are similar to panels (e)–(g), except when applied to HSTCM-NO2.
Figure 12 shows the spatial and temporal patterns after EOF variance maximization is performed on both OMNO2 and HSTCM-NO2. First and foremost, the EOFs represent a few general patterns, seeming to capture a combination of biomass burning (across Africa, South America, and Australia), urbanization (across southern Africa, northeastern China, and Japan), energy-producing regions in the southern USA and northern Mexico, and transport regions from the Mediterranean Sea to the Indian Ocean. This includes the large areas of pollution transported downwind over the various oceans and the uncertainty associated with clouds, sea salt, and low signal strengths near where the Southern Ocean intrudes into the cleaner areas of the Indian and Pacific oceans, respectively. The overall patterns look reasonable in both space and time.
A more detailed analysis clearly demonstrates that three such examples are consistently represented between the original OMNO2 and HSTCM-NO2. First, the negative mode of EOF1 representing biomass burning over Congo and its subsequent transport over the South Atlantic Ocean and the positive mode of EOF1 representing biomass burning and urbanization over the respective parts of southern Africa are interpolated well and are line-filled by the respective negative and positive modes of the HSTCM-NO2 EOF1 (Du et al., 2020). Second, the wildfires off southwestern Australia and the subsequent transport into the Southern Ocean are clearly shown by the negative mode of EOF1, while the negative mode of EOF1 of HSTCM-NO2 expands these observations into the Indian Ocean and all the way to New Zealand while narrowing the band and reducing the error due to the mixing from the Southern Ocean, which is consistent with the observations (Wenig et al., 2003). Third, the positive region of EOF1 loosely picks up the transported wave trains from East Asia to North America, while HSTCM-NO2 is able to clearly pick up the entire wave train that clearly originates in industrial regions of Japan and spreads some of the time to Luzon and other times to the USA (Wang et al., 2023). In terms of time, it is clear that the negative EOF1 regions in both plots are well represented by the positive PC1 values. All three peaks demonstrated are clearly observed in the average values of NO2 over the negative EOF1 regions. There are four large peaks and two small peaks represented in the negative PC1 values, all of which are picked up well in the average values of NO2 over the positive EOF1 regions. All of the peak times are represented in the time series using different colors.
While the distribution of the HSTCM-NO2 EOF is more smeared spatially than the OMNO2 product in some regions, this is not unexpected. In some cases, this makes the story consistent by filling in missing data, especially in cases of long-range-transported plumes which are otherwise missing and where the known variation is observed over Henan and Shandong. However, some of the smearing is also noise, as identified over the low-NO2-concentration regions near where the Indian and Pacific oceans intersect with the Southern Ocean.
This analysis shows that the HSTCM-NO2 product does a decent job at representing the temporal and spatial extremes in the original OMNO2 dataset. While this test is not frequently done in the community (Cohen, 2014; Z. Liu et al., 2024), this clearly demonstrates in an objective manner a new and additional way of testing the goodness of the final product, in that it requires the product to match not only observed mean conditions in space and time but also observed extreme conditions. The fact that there is spatial smearing in some aspects is good, in that this fills in missing long-range transport events that are missed between swaths or are due to clouds in situ. In other aspects, this may extend the actual signals too far in space. For these reasons, care must be taken when applying the results. We hope that this section will set a gold standard by which future big-data products are more carefully compared with and validated against underlying data.
The global daily High Spatial–Temporal Coverage Merged tropospheric NO2 dataset (HSTCM-NO2) from 2007 to 2022 based on OMI and GOME-2 can be accessed directly at https://doi.org/10.5281/zenodo.10968462 (Qin et al., 2024).
In order to improve the spatial coverage of OMNO2 due to data loss caused by cloud occlusion, the row anomaly, high retrieval noise, and other issues, this study proposes an effective method of reconstruction consisting of machine learning (XGBoost) and gap-filling (DINEOF) to produce a new reconstructed product (HSTCM-NO2).
First, the process of applying XGBoost first, followed by DINEOF, yields the highest correlation and the lowest RMSE between OMNO2 and HSTCM-NO2. One reason for this is that XGBoost requires the presence of GOME-2 data, allowing for additional observational support in the final reconstructed product. This is consistent with the fact that GOME-2 has a very high SHAP value. There are a few qualifiers, however: first, cases without prior knowledge perform less well than cases with prior knowledge, and second, locations with a lower column loading of OMNO2 work better than locations with a higher column loading of OMNO2. Since the majority of the global data points are biased towards lower (i.e., non-polluted) areas, comparison with additional datasets and use of different approaches are essential.
Second, external observations from MAX-DOAS and TROPOMI together with reanalysis data from EAC4 are used to validate HSTCM-NO2 on a column-by-column, large-area basis. HSTCM-NO2 shows good correlations with all of the observations above, especially when the VCDs are below 6 × 1015 . Specific issues in terms of spatial distribution mismatches and issues reproducing very high VCDs are explained in detail in the paper. There are a few exceptions to this, specifically over Wuhan and the Yangtze River from Wuhan up to Nanjing, and specific urban parts of India (such as New Delhi) are reasonably well represented.
Third, additional analysis to verify the goodness of HSTCM-NO2 in terms of being able to capture extreme events observed in the OMNO2 data is performed. In this case, variance maximization is used to decompose the OMNO2 data into standing spatial (EOF) and temporal (PC) signals. A similar analysis is performed on the HSTCM-NO2 data, with the resulting signals compared. It is shown that, in addition to generally matching in terms of space and time, data after gap-filling observed by HSTCM-NO2, especially downwind from high-pollution areas over various oceans (South Atlantic, Indian, South Pacific, and North Pacific), are improved. Interestingly, some of the strongest signals, including biomass burning from central and northern Africa (including in Algeria and including pixels over a value of 6 × 1015 ) are also well represented in terms of the magnitude and spatial and temporal extremes.
This combination of findings indicates that the new HSTCM-NO2 product works well in terms of representing both grid-by-grid and climatological mean conditions as well as extreme events, with the caveats that first there is some a priori knowledge and second that the original OMNO2 data have a VCD below 6 × 1015 (i.e., are not heavily polluted). In the future, related work will focus on how to enhance the application of datasets in polluted scenarios. Separating low and high values for training might be an effective approach, since it is known that there are different retrieval assumptions and impacts that occur under polluted and non-polluted conditions (Boersma et al., 2007; Chimot et al., 2016; Lorente et al., 2018; M. Liu et al., 2019; Zhou et al., 2024). Presently the criteria for demarcation and the sets of impacting variables are still under discussion by the community and are not yet agreed upon. Whether there are better methods or combinations of methods that can be applied across the full range of scenarios at the same time is also something that needs to be considered.
KQ is responsible for the conceptualization, funding acquisition, investigation, project administration, resources, supervision, and editing. JBC is responsible for the methodology refinement, supervision, validation, results review, and original draft editing. HG is responsible for the data curation, formal analysis, software, visualization, and original draft writing. XL is responsible for the data curation and visualization. QH is responsible for the original draft editing and the visualization. PT is responsible for the draft editing and the additional formal analysis during the response phase. All the authors contributed to the final paper.
The contact author has declared that none of the authors has any competing interests.
Publisher's note: Copernicus Publications remains neutral with regard to jurisdictional claims made in the text, published maps, institutional affiliations, or any other geographical representation in this paper. While Copernicus Publications makes every effort to include appropriate place names, the final responsibility lies with the authors.
This research has been supported by the National Natural Science Foundation of China (grant no. 42375125).
This paper was edited by Jing Wei and reviewed by two anonymous referees.
Abdulmanov, R., Miftakhov, I., Ishbulatov, M., Galeev, E., and Shafeeva, E.: Comparison of the effectiveness of GIS-based interpolation methods for estimating the spatial distribution of agrochemical soil properties, Environ. Technol. Innov., 24, 101970, https://doi.org/10.1016/j.eti.2021.101970, 2021.
Achite, M., Katipoğlu, Okan Mert, Javari, M., and Caloiero, T.: Hybrid interpolation approach for estimating the spatial variation of annual precipitation in the Macta basin, Algeria, Theor. Appl. Climatol., 155, 1139–1166, https://doi.org/10.1007/s00704-023-04685-w, 2024.
Alvera-Azcárate, A., Barth, A., Sirjacobs, D., and Beckers, J.-M.: Enhancing temporal correlations in EOF expansions for the reconstruction of missing data using DINEOF, Ocean Sci., 5, 475–485, https://doi.org/10.5194/os-5-475-2009, 2009.
Alvera-Azcárate, A., Barth, A., Parard, G., and Beckers, J.-M.: Analysis of SMOS sea surface salinity data using DINEOF, Remote Sens. Environ., 180, 137–145, https://doi.org/10.1016/j.rse.2016.02.044, 2016.
Baek, K. and Kim, J.: Analysis of Characteristics of Satellite-derived Air Pollutant over Southeast Asia and Evaluation of Tropospheric Ozone using Statistical Methods, J. Korean Soc. Atmos. Environ., 27, 650–662, https://doi.org/10.5572/kosae.2011.27.6.650, 2011.
Bauer, R., Rozanov, A., McLinden, C. A., Gordley, L. L., Lotz, W., Russell III, J. M., Walker, K. A., Zawodny, J. M., Ladstätter-Weißenmayer, A., Bovensmann, H., and Burrows, J. P.: Validation of SCIAMACHY limb NO2 profiles using solar occultation measurements, Atmos. Meas. Tech., 5, 1059–1084, https://doi.org/10.5194/amt-5-1059-2012, 2012.
Beckers, J.-M. and Rixen, M.: EOF Calculations and Data Filling from Incomplete Oceanographic Datasets, J. Atmos. Ocean. Tech., 20, 1839–1856, https://doi.org/10.1175/1520-0426(2003)020<1839:ECADFF>2.0.CO;2, 2003.
Boersma, K. F., Eskes, H. J., and Brinksma, E. J.: Error analysis for tropospheric NO2 retrieval from space, J. Geophys. Res., 109, D04311, https://doi.org/10.1029/2003JD003962, 2004.
Boersma, K. F., Eskes, H. J., Veefkind, J. P., Brinksma, E. J., van der A, R. J., Sneep, M., van den Oord, G. H. J., Levelt, P. F., Stammes, P., Gleason, J. F., and Bucsela, E. J.: Near-real time retrieval of tropospheric NO2 from OMI, Atmos. Chem. Phys., 7, 2103–2118, https://doi.org/10.5194/acp-7-2103-2007, 2007.
Boersma, K. F., Jacob, D. J., Eskes, H. J., Pinder, R. W., Wang, J., and van der A, R. J.: Intercomparison of SCIAMACHY and OMI tropospheric NO2 columns: Observing the diurnal evolution of chemistry and emissions from space, J. Geophys. Res., 113, D16S26, https://doi.org/10.1029/2007JD008816, 2008.
Bousserez, N.: Space-based retrieval of NO2 over biomass burning regions: quantifying and reducing uncertainties, Atmos. Meas. Tech., 7, 3431–3444, https://doi.org/10.5194/amt-7-3431-2014, 2014.
Bovensmann, H., Burrows, J. P., Buchwitz, M., Frerick, J., Noël, S., Rozanov, V. V., Chance, K. V., and Goede, A. P. H.: SCIAMACHY: Mission Objectives and Measurement Modes, J. Atmos. Sci., 56, 127–150, https://doi.org/10.1175/1520-0469(1999)056<0127:SMOAMM>2.0.CO;2, 1999.
Chang, N., Bai, K., and Chen, C.: Integrating multisensor satellite data merging and image reconstruction in support of machine learning for better water quality management, J. Environ. Manage., 201, 227–240, https://doi.org/10.1016/j.jenvman.2017.06.045, 2017.
Chen, T. and Guestrin, C.: XGBoost: A Scalable Tree Boosting System, in: Proceedings of the 22nd ACM SIGKDD International Conference on Knowledge Discovery and Data Mining, San Francisco, California, USA, August 2016, 785–794, https://doi.org/10.1145/2939672.2939785, 2016.
Chen, Z., Wang, P., Bao, S., and Zhang, W.: Rapid reconstruction of temperature and salinity fields based on machine learning and the assimilation application, Front. Mar. Sci., 9, https://doi.org/10.3389/fmars.2022.985048, 2022.
Chimot, J., Vlemmix, T., Veefkind, J. P., de Haan, J. F., and Levelt, P. F.: Impact of aerosols on the OMI tropospheric NO2 retrievals over industrialized regions: how accurate is the aerosol correction of cloud-free scenes via a simple cloud model?, Atmos. Meas. Tech., 9, 359–382, https://doi.org/10.5194/amt-9-359-2016, 2016.
Chowdhury, M. R.: Overview of Weather, ENSO, and Climate Scale, in: Seasonal Flood Forecasts and Warning Response Opportunities: ENSO Applications in Bangladesh, Springer, Cham, 59–74, https://doi.org/10.1007/978-3-031-17825-2, 2022.
Cisty, M. and Soldanova, V.: Flow Prediction Versus Flow Simulation Using Machine Learning Algorithms, in: Machine Learning and Data Mining in Pattern Recognition, vol. 10935, edited by: Perner, P., Springer, Cham, 369–382, https://doi.org/10.1007/978-3-319-96133-0_28, 2018.
Cohen, J. B.: Quantifying the occurrence and magnitude of the Southeast Asian fire climatology, Environ. Res. Lett., 9, 114018, https://doi.org/10.1088/1748-9326/9/11/114018, 2014.
Cohen, J. B., Prinn, R. G., and Wang, C.: The impact of detailed urban-scale processing on the composition, distribution, and radiative forcing of anthropogenic aerosols, Geophys. Res. Lett., 38, L10808, https://doi.org/10.1029/2011gl047417, 2011.
Cohen, J. B., Lecoeur, E., and Hui Loong Ng, D.: Decadal-scale relationship between measurements of aerosols, land-use change, and fire over Southeast Asia, Atmos. Chem. Phys., 17, 721–743, https://doi.org/10.5194/acp-17-721-2017, 2017.
Compernolle, S., Verhoelst, T., Pinardi, G., Granville, J., Hubert, D., Keppens, A., Niemeijer, S., Rino, B., Bais, A., Beirle, S., Boersma, F., Burrows, J. P., De Smedt, I., Eskes, H., Goutail, F., Hendrick, F., Lorente, A., Pazmino, A., Piters, A., Peters, E., Pommereau, J.-P., Remmers, J., Richter, A., van Geffen, J., Van Roozendael, M., Wagner, T., and Lambert, J.-C.: Validation of Aura-OMI QA4ECV NO2 climate data records with ground-based DOAS networks: the role of measurement and comparison uncertainties, Atmos. Chem. Phys., 20, 8017–8045, https://doi.org/10.5194/acp-20-8017-2020, 2020.
Cooper, M. J., Martin, R. V., Hammer, M. S., and McLinden, C. A.: An Observation-Based Correction for Aerosol Effects on Nitrogen Dioxide Column Retrievals Using the Absorbing Aerosol Index, Geophys. Res. Lett., 46, 8442–8452, https://doi.org/10.1029/2019GL083673, 2019.
Cooper, M. J., Martin, R. V., Hammer, M. S., Levelt, P. F., Veefkind, P., Lamsal, L. N., Krotkov, N. A., Brook, J. R., and McLinden, C. A.: Global fine-scale changes in ambient NO2 during COVID-19 lockdowns, Nature, 601, 380–387, https://doi.org/10.1038/s41586-021-04229-0, 2022.
Crutzen, P. J.: Gas-Phase Nitrogen and Methane Chemistry in the Atmosphere, in: Physics and Chemistry of Upper Atmosphere, edited by: McCormac, B. M., Astrophysics and Space Science Library, vol. 35, Springer, Dordrecht, 110–124, https://doi.org/10.1007/978-94-010-2542-3_12, 1973.
Crutzen, P. J.: The Role of NO and NO2 in the Chemistry of the Troposphere and Stratosphere, Annu. Rev. Earth Pl. Sc., 7, 443–472, https://doi.org/10.1146/annurev.ea.07.050179.002303, 1979.
de Hoogh, K., Saucy, A., Shtein, A., Schwartz, J., West, E. A., Strassmann, A., Puhan, M., Röösli, M., Stafoggia, M., and Kloog, I.: Predicting Fine-Scale Daily NO2 for 2005–2016 Incorporating OMI Satellite Data Across Switzerland, Environ. Sci. Technol., 53, 10279–10287, https://doi.org/10.1021/acs.est.9b03107, 2019.
Deng, W., Cohen, J. B., Wang, S., and Lin, C.: Improving the understanding between climate variability and observed extremes of global NO2 over the past 15 years, Environ. Res. Lett., 16, 054020, https://doi.org/10.1088/1748-9326/abd502, 2021.
Dong, J., Chen, Y., Yao, B., Zhang, X., and Zeng, N.: A neural network boosting regression model based on XGBoost, Appl. Soft. Comput., 125, 109067, https://doi.org/10.1016/j.asoc.2022.109067, 2022.
Du, M., Chen, L., Lin, J., Liu, Y., Feng, K., Liu, Q., Liu, Y., Wang, J., Ni, R., Zhao, Y., Si, W., Li, Y., Kong, H., Weng, H., Liu, M., and Adeniran, J. A.: Winners and losers of the Sino–US trade war from economic and environmental perspectives, Environ. Res. Lett., 15, 094032, https://doi.org/10.1088/1748-9326/aba3d5, 2020.
Duncan, B. N., Yoshida, Y., de Foy, B., Lamsal, L. N., Streets, D. G., Lu, Z., Pickering, K. E., and Krotkov, N. A.: The observed response of Ozone Monitoring Instrument (OMI) NO2 columns to NOx emission controls on power plants in the United States: 2005–2011, Atmos. Environ., 81, 102–111, https://doi.org/10.1016/j.atmosenv.2013.08.068, 2013.
Echterhof, T. and Pfeifer, H.: Nitrogen Oxide Formation in the Electric Arc Furnace—Measurement and Modeling, Metall. Mater. Trans. B, 43, 163–172, https://doi.org/10.1007/s11663-011-9564-8, 2011.
Eriksson, E.: Composition of Atmospheric Precipitation, Tellus, 4, 280–303, https://doi.org/10.3402/tellusa.v4i4.8813, 1952.
Eskes, H. J. and Boersma, K. F.: Averaging kernels for DOAS total-column satellite retrievals, Atmos. Chem. Phys., 3, 1285–1291, https://doi.org/10.5194/acp-3-1285-2003, 2003.
Crippa, M., Guizzardi, D., and Schaaf, E., Monforti-Ferrario, F., and Quadrelli, R.: GHG emissions of all world countries, European Commission, Joint Research Centre, Publications Office of the European Union, https://doi.org/10.2760/953322, 2023.
Fan, Z., Zhan, Q., Yang, C., Liu, H., and Bilal, M.: Estimating PM2.5 Concentrations Using Spatially Local Xgboost Based on Full-Covered SARA AOD at the Urban Scale, Remote Sens.-Basel, 12, 3368, https://doi.org/10.3390/rs12203368, 2020.
Fioletov, V. E., McLinden, C. A., Krotkov, N., Yang, K., Loyola, D. G., Valks, P., Theys, N., Van Roozendael, M., Nowlan, C. R., Chance, K., Liu, X., Lee, C., and Martin, R. V.: Application of OMI, SCIAMACHY, and GOME-2 satellite SO2 retrievals for detection of large emission sources, J. Geophys. Res.-Atmos., 118, 11, 399–11, 418, https://doi.org/10.1002/jgrd.50826, 2013.
Fishman, J., Solomon, S., and Crutzen, P. J.: Observational and theoretical evidence in support of a significant in-situ photochemical source of tropospheric ozone, Tellus, 31, 432–446, https://doi.org/10.1111/j.2153-3490.1979.tb00922.x, 1979.
Foret, G., Eremenko, M., Cuesta, J., Sellitto, P., Barré, J., Gaubert, B., Coman, A., Dufour, G., Liu, X., Joly, M., Doche, C., and Beekmann, M.: Ozone pollution: What can we see from space? A case study, J. Geophys. Res.-Atmos., 119, 8476–8499, https://doi.org/10.1002/2013JD021340, 2014.
He, Q., Qin, K., Cohen, J. B., Loyola, D., Li, D., Shi, J., and Xue, Y.: Spatially and temporally coherent reconstruction of tropospheric NO2 over China combining OMI and GOME-2B measurements, Environ. Res. Lett., 15, 125011, https://doi.org/10.1088/1748-9326/abc7df, 2020.
Hilborn, A. and Costa, M.: Applications of DINEOF to Satellite-Derived Chlorophyll-a from a Productive Coastal Region, Remote Sens.-Basel, 10, 1449, https://doi.org/10.3390/rs10091449, 2018.
Ialongo, I., Virta, H., Eskes, H., Hovila, J., and Douros, J.: Comparison of TROPOMI/Sentinel-5 Precursor NO2 observations with ground-based measurements in Helsinki, Atmos. Meas. Tech., 13, 205–218, https://doi.org/10.5194/amt-13-205-2020, 2020.
Irie, H., Boersma, K. F., Kanaya, Y., Takashima, H., Pan, X., and Wang, Z. F.: Quantitative bias estimates for tropospheric NO2 columns retrieved from SCIAMACHY, OMI, and GOME-2 using a common standard for East Asia, Atmos. Meas. Tech., 5, 2403–2411, https://doi.org/10.5194/amt-5-2403-2012, 2012.
Jiang, Y., Gao, Z., He, J., Wu, J., and Christakos, G.: Application and Analysis of XCO2 Data from OCO Satellite Using a Synthetic DINEOF–BME Spatiotemporal Interpolation Framework, Remote Sens.-Basel, 14, 4422, https://doi.org/10.3390/rs14174422, 2022.
Just, A. C., Margherita, C., Shtein, A., Dorman, M., Lyapustin, A., and Kloog, I.: Correcting Measurement Error in Satellite Aerosol Optical Depth with Machine Learning for Modeling PM2.5 in the Northeastern USA, Remote Sens.-Basel, 10, 803, https://doi.org/10.3390/rs10050803, 2018.
Kapoor, S. and Perrone, V.: A Simple and Fast Baseline for Tuning Large XGBoost Models, arXiv (Cornell University), https://doi.org/10.48550/arxiv.2111.06924, 2021.
Kim, J., Baek, K., and Kim, S.: Validation of OMI HCHO with EOF and SVD over Tropical Africa, Korean J. Remote Sensing, 30, 417–430, https://doi.org/10.7780/kjrs.2014.30.4.1, 2014.
Kolle, O., Kalthoff, N., Kottmeier, C., and William, M. J.: Ground-Based Platforms, in: Springer Handbook of Atmospheric Measurements, edited by: Foken, T., Springer International Publishing, Cham, 155–182, https://doi.org/10.1007/978-3-030-52171-4_6, 2021.
Laan, E., de Winter, D., de Vries, J., Levelt, P. F., van den Oord, G. H., Malkki, A., Leppelmeier, G. W., and Hilsenrath, E.: Toward the use of the Ozone Monitoring Instrument (OMI), P. SPIE, 4540, 270–277, 2001.
Lamsal, L. N., Krotkov, N. A., Vasilkov, A., Marchenko, S., Qin, W., Yang, E.-S., Fasnacht, Z., Joiner, J., Choi, S., Haffner, D., Swartz, W. H., Fisher, B., and Bucsela, E.: Ozone Monitoring Instrument (OMI) Aura nitrogen dioxide standard product version 4.0 with improved surface and cloud treatments, Atmos. Meas. Tech., 14, 455–479, https://doi.org/10.5194/amt-14-455-2021, 2021.
Leitão, J., Richter, A., Vrekoussis, M., Kokhanovsky, A., Zhang, Q. J., Beekmann, M., and Burrows, J. P.: On the improvement of NO2 satellite retrievals – aerosol impact on the airmass factors, Atmos. Meas. Tech., 3, 475–493, https://doi.org/10.5194/amt-3-475-2010, 2010.
Levy, H.: Photochemistry of the lower troposphere, Planet. Space Sci., 20, 919–935, https://doi.org/10.1016/0032-0633(72)90177-8, 1972.
Li, D., Qin, K., Cohen, J. B., He, Q., Wang, S., Li, D., Zhou, X., Ling, X., and Xue, Y.: Combing GOME-2B and OMI Satellite Data to Estimate Near-Surface NO2 of Mainland China, IEEE J. Sel. Top. Appl., 14, 10269–10277, https://doi.org/10.1109/JSTARS.2021.3117396, 2021.
Li, M., Liu, H., Geng, G., Hong, C., Liu, F., Song, Y., Tong, D., Zheng, B., Cui, H., Man, H., Zhang, Q., and He, K.: Anthropogenic emission inventories in China: a review, Natl. Sci. Rev., 4, 834–866, https://doi.org/10.1093/nsr/nwx150, 2017.
Li, M., Mao, J., Chen, S., Bian, J., Bai, Z., Wang, X., Chen, W., and Yu, P.: Significant contribution of lightning NOx to summertime surface O3 on the Tibetan Plateau, Sci. Total Environ., 829, 154639, https://doi.org/10.1016/j.scitotenv.2022.154639, 2022.
Li, X., Cohen, J. B., Qin, K., Geng, H., Wu, X., Wu, L., Yang, C., Zhang, R., and Zhang, L.: Remotely sensed and surface measurement-derived mass-conserving inversion of daily NOx emissions and inferred combustion technologies in energy-rich northern China, Atmos. Chem. Phys., 23, 8001–8019, https://doi.org/10.5194/acp-23-8001-2023, 2023.
Lin, J.-T., Martin, R. V., Boersma, K. F., Sneep, M., Stammes, P., Spurr, R., Wang, P., Van Roozendael, M., Clémer, K., and Irie, H.: Retrieving tropospheric nitrogen dioxide from the Ozone Monitoring Instrument: effects of aerosols, surface reflectance anisotropy, and vertical profile of nitrogen dioxide, Atmos. Chem. Phys., 14, 1441–1461, https://doi.org/10.5194/acp-14-1441-2014, 2014.
Liu, F., Zhang, Q., van der A, R. J., Zheng, B., Tong, D., Yan, L., Zheng, Y., and He, K.: Recent reduction in NOx missions over China: synthesis of satellite observations and emission inventories, Environ. Res. Lett., 11, 114002, https://doi.org/10.1088/1748-9326/11/11/114002, 2016.
Liu, J., Cohen, J. B., He, Q., Tiwari, P., and Qin, K.: Accounting for NOx emissions from biomass burning and urbanization doubles existing inventories over South, Southeast and East Asia, Commun. Earth Environ., 5, 255, https://doi.org/10.1038/s43247-024-01424-5, 2024.
Liu, M., Lin, J., Boersma, K. F., Pinardi, G., Wang, Y., Chimot, J., Wagner, T., Xie, P., Eskes, H., Van Roozendael, M., Hendrick, F., Wang, P., Wang, T., Yan, Y., Chen, L., and Ni, R.: Improved aerosol correction for OMI tropospheric NO2 retrieval over East Asia: constraint from CALIOP aerosol vertical profile, Atmos. Meas. Tech., 12, 1–21, https://doi.org/10.5194/amt-12-1-2019, 2019.
Liu, S., Valks, P., Pinardi, G., De Smedt, I., Yu, H., Beirle, S., and Richter, A.: An improved total and tropospheric NO2 column retrieval for GOME-2, Atmos. Meas. Tech., 12, 1029–1057, https://doi.org/10.5194/amt-12-1029-2019, 2019.
Liu, Z., Cohen, J. B., Wang, S., Wang, X., Tiwari, P., and Qin, K.: Remotely sensed BC columns over rapidly changing Western China show significant decreases in mass and inconsistent changes in number, size, and mixing properties due to policy actions, npj Clim. Atmos. Sci., 7, 124, https://doi.org/10.1038/s41612-024-00663-9, 2024.
Logan, J. A.: Nitrogen oxides in the troposphere: Global and regional budgets, J. Geophys. Res., 88, 10785, https://doi.org/10.1029/jc088ic15p10785, 1983.
Logan, J. A., Prather, M. J., Wofsy, S. C., and McElroy, M. B.: Tropospheric chemistry: A global perspective, J. Geophys. Res., 86, 7210, https://doi.org/10.1029/jc086ic08p07210, 1981.
Lorente, A., Boersma, K. F., Stammes, P., Tilstra, L. G., Richter, A., Yu, H., Kharbouche, S., and Muller, J.-P.: The importance of surface reflectance anisotropy for cloud and NO2 retrievals from GOME-2 and OMI, Atmos. Meas. Tech., 11, 4509–4529, https://doi.org/10.5194/amt-11-4509-2018, 2018.
Lu, X., Ye, X., Zhou, M., Zhao, Y., Weng, H., Kong, H., Li, K., Gao, M., Zheng, B., Lin, J., Zhou, F., Zhang, Q., Wu, D., Zhang, L., and Zhang, Y.: The underappreciated role of agricultural soil nitrogen oxide emissions in ozone pollution regulation in North China, Nat. Commun., 12, 5021, https://doi.org/10.1038/s41467-021-25147-9, 2021.
Lu, Y. and Khalil, M. A. K.: Methane and carbon monoxide in OH chemistry: The effects of feedbacks and reservoirs generated by the reactive products, Chemosphere, 26, 641–655, https://doi.org/10.1016/0045-6535(93)90450-J, 1993.
Lu, Z., Liu, X., Zaveri, R. A., Easter, R. C., Tilmes, S., Emmons, L. K., Vitt, F., Singh, B., Wang, H., Zhang, R., and Rasch, P. J.: Radiative Forcing of Nitrate Aerosols From 1975 to 2010 as Simulated by MOSAIC Module in CESM2-MAM4, J. Geophys. Res.-Atmos., 126, e2021JD034809, https://doi.org/10.1029/2021jd034809, 2021.
Ludewig, A., Kleipool, Q., Bartstra, R., Landzaat, R., Leloux, J., Loots, E., Meijering, P., van der Plas, E., Rozemeijer, N., Vonk, F., and Veefkind, P.: In-flight calibration results of the TROPOMI payload on board the Sentinel-5 Precursor satellite, Atmos. Meas. Tech., 13, 3561–3580, https://doi.org/10.5194/amt-13-3561-2020, 2020.
Manisalidis, I., Stavropoulou, E., Stavropoulos, A., and Bezirtzoglou, E.: Environmental and Health Impacts of Air Pollution: A Review, Front. Public Health, 8, 1–13, https://doi.org/10.3389/fpubh.2020.00014, 2020.
Munro, R., Lang, R., Klaes, D., Poli, G., Retscher, C., Lindstrot, R., Huckle, R., Lacan, A., Grzegorski, M., Holdak, A., Kokhanovsky, A., Livschitz, J., and Eisinger, M.: The GOME-2 instrument on the Metop series of satellites: instrument design, calibration, and level 1 data processing – an overview, Atmos. Meas. Tech., 9, 1279–1301, https://doi.org/10.5194/amt-9-1279-2016, 2016.
Park, J., Kim, H., Bae, D., and Jo, Y.: Data Reconstruction for Remotely Sensed Chlorophyll-a Concentration in the Ross Sea Using Ensemble-Based Machine Learning, Remote Sens.-Basel, 12, 1898, https://doi.org/10.3390/rs12111898, 2020.
Pinardi, G., Van Roozendael, M., Hendrick, F., Theys, N., Abuhassan, N., Bais, A., Boersma, F., Cede, A., Chong, J., Donner, S., Drosoglou, T., Dzhola, A., Eskes, H., Frieß, U., Granville, J., Herman, J. R., Holla, R., Hovila, J., Irie, H., Kanaya, Y., Karagkiozidis, D., Kouremeti, N., Lambert, J.-C., Ma, J., Peters, E., Piters, A., Postylyakov, O., Richter, A., Remmers, J., Takashima, H., Tiefengraber, M., Valks, P., Vlemmix, T., Wagner, T., and Wittrock, F.: Validation of tropospheric NO2 column measurements of GOME-2A and OMI using MAX-DOAS and direct sun network observations, Atmos. Meas. Tech., 13, 6141–6174, https://doi.org/10.5194/amt-13-6141-2020, 2020.
Platt, U. and Stutz, J.: Differential Optical Absorption Spectroscopy: Principles and Applications, Springer Berlin Heidelberg, Berlin, Heidelberg, https://doi.org/10.1007/978-3-540-75776-4_6, 2008.
Qin, K., Lu, L., Liu, J., He, Q., Shi, J., Deng, W., Wang, S., and Cohen, J. B.: Model-free daily inversion of NOx emissions using TROPOMI (MCMFE-NOx) and its uncertainty: Declining regulated emissions and growth of new sources, Remote Sens. Environ., 295, 113720, https://doi.org/10.1016/j.rse.2023.113720, 2023.
Qin, K., Gao, H., Liu, X., He, Q., and Cohen, J. B.: HSTCM-NO2 [Data set]. Zenodo. https://doi.org/10.5281/zenodo.10968462, 2024.
Reid, C. E., Jerrett, M., Petersen, M. L., Pfister, G. G., Morefield, P. E., Tager, I. B., Raffuse, S. M., and Balmes, J. R.: Spatiotemporal Prediction of Fine Particulate Matter During the 2008 Northern California Wildfires Using Machine Learning, Environ. Sci. Technol., 49, 3887–3896, https://doi.org/10.1021/es505846r, 2015.
Richter, A. and Burrows, J. P.: Tropospheric NO2 from GOME measurements, Adv. Space Res., 29, 1673–1683, https://doi.org/10.1016/S0273-1177(02)00100-X, 2002.
Richter, A., Burrows, J. P., Nüß, H., Granier, C., and Niemeier, U.: Increase in tropospheric nitrogen dioxide over China observed from space, Nature, 437, 129–132, https://doi.org/10.1038/nature04092, 2005.
Richter, A., Begoin, M., Hilboll, A., and Burrows, J. P.: An improved NO2 retrieval for the GOME-2 satellite instrument, Atmos. Meas. Tech., 4, 1147–1159, https://doi.org/10.5194/amt-4-1147-2011, 2011.
Running, S. W.: Generalization of a forest ecosystem process model for other biomes, BIOME-BGC, and an application for global-scale models, Scaling Physiological Processes Leaf to Globe, 1993, 141–158, https://doi.org/10.1016/B978-0-12-233440-5.50014-2, 1993.
Sanabria, L. A., Qin, X., Li, J., Cechet, R. P., and Lucas, C.: Spatial interpolation of McArthur's Forest Fire Danger Index across Australia: Observational study, Environ. Modell. Softw., 50, 37–50, https://doi.org/10.1016/j.envsoft.2013.08.012, 2013.
Schaub, D., Boersma, K. F., Kaiser, J. W., Weiss, A. K., Folini, D., Eskes, H. J., and Buchmann, B.: Comparison of GOME tropospheric NO2 columns with NO2 profiles deduced from ground-based in situ measurements, Atmos. Chem. Phys., 6, 3211–3229, https://doi.org/10.5194/acp-6-3211-2006, 2006.
Shao, Y., Zhao, W., Liu, R., Yang, J., Liu, M., Fang, W., Hu, L., Adams, M., Bi, J., and Ma, Z.: Estimation of daily NO2 with explainable machine learning model in China, 2007–2020, Atmos. Environ., 314, 120111, https://doi.org/10.1016/j.atmosenv.2023.120111, 2023.
Shapley, L. S.: A Value for N-Person Games, RAND Corporation, Santa Monica, CA, https://doi.org/10.7249/P0295, 1952.
Sillman, S., Logan, J. A., and Wofsy, S. C.: The sensitivity of ozone to nitrogen oxides and hydrocarbons in regional ozone episodes, J. Geophys. Res., 95, 1837, https://doi.org/10.1029/jd095id02p01837, 1990.
Spivakovsky, C. M., Logan, J. A., Montzka, S. A., Balkanski, Y. J., Foreman-Fowler, M., Jones, D. B. A., Horowitz, L. W., Fusco, A. C., Brenninkmeijer, C. A. M., Prather, M. J., Wofsy, S. C., and McElroy, M. B.: Three-dimensional climatological distribution of tropospheric OH: Update and evaluation, J. Geophys. Res., 105, 8931–8980, https://doi.org/10.1029/1999jd901006, 2000.
Streets, D. G., Canty, T., Carmichael, G. R., de Foy, B., Dickerson, R. R., Duncan, B. N., Edwards, D. P., Haynes, J. A., Henze, D. K., Houyoux, M. R., Jacob, D. J., Krotkov, N. A., Lamsal, L. N., Liu, Y., Lu, Z., Martin, R. V., Pfister, G. G., Pinder, R. W., Salawitch, R. J., and Wecht, K. J.: Emissions estimation from satellite retrievals: A review of current capability, Atmos. Environ., 77, 1011–1042, https://doi.org/10.1016/j.atmosenv.2013.05.051, 2013.
Sun, W., Shao, M., Granier, C., Liu, Y., Ye, C., and Zheng, J.: Long-Term Trends of Anthropogenic SO2, NOx, CO, and NMVOCs Emissions in China, Earths Future, 6, 1112–1133, https://doi.org/10.1029/2018ef000822, 2018.
Tiwari, P., Cohen, J. B., Wang, X., Wang, S., and Qin, K.: Radiative forcing bias calculation based on COSMO (CoreShell Mie model Optimization) and AERONET data, npj Clim. Atmos. Sci., 6, 193, https://doi.org/10.1038/s41612-023-00520-1, 2023.
Torres, O., Bhartia, P. K., Jethva, H., and Ahn, C.: Impact of the ozone monitoring instrument row anomaly on the long-term record of aerosol products, Atmos. Meas. Tech., 11, 2701–2715, https://doi.org/10.5194/amt-11-2701-2018, 2018.
van der A, R. J., Peters, D. H. M. U., Eskes, H., Boersma, K. F., Van Roozendael, M., De Smedt, I., and Kelder, H. M.: Detection of the trend and seasonal variation in tropospheric NO2 over China, J. Geophys. Res., 111, 1–10, https://doi.org/10.1029/2005JD006594, 2006.
van Geffen, J., Eskes, H. J., Boersma, K. F., Maasakkers, J. D., and Veefkind, J. P.: TROPOMI ATBD of the total and tropospheric NO2 data products, Tech. Rep., KNMI, S5P-KNMI-L2-0005-RP, https://sentinel.esa.int/documents/247904/2476257/Sentinel-5P-TROPOMI-ATBD-NO2-data-products.pdf (last access: 12 November 2024), 2019.
van Geffen, J., Boersma, K. F., Eskes, H., Sneep, M., ter Linden, M., Zara, M., and Veefkind, J. P.: S5P TROPOMI NO2 slant column retrieval: method, stability, uncertainties and comparisons with OMI, Atmos. Meas. Tech., 13, 1315–1335, https://doi.org/10.5194/amt-13-1315-2020, 2020.
Veefkind, J. P., Aben, I., McMullan, K., Förster, H., de Vries, J., Otter, G., Claas, J., Eskes, H. J., de Haan, J. F., Kleipool, Q., van Weele, M., Hasekamp, O., Hoogeveen, R., Landgraf, J., Snel, R., Tol, P., Ingmann, P., Voors, R., Kruizinga, B., and Vink, R.: TROPOMI on the ESA Sentinel-5 Precursor: A GMES mission for global observations of the atmospheric composition for climate, air quality and ozone layer applications, Remote Sens. Environ., 120, 70–83, https://doi.org/10.1016/j.rse.2011.09.027, 2012.
Verhoelst, T., Compernolle, S., Pinardi, G., Lambert, J.-C., Eskes, H. J., Eichmann, K.-U., Fjæraa, A. M., Granville, J., Niemeijer, S., Cede, A., Tiefengraber, M., Hendrick, F., Pazmiño, A., Bais, A., Bazureau, A., Boersma, K. F., Bognar, K., Dehn, A., Donner, S., Elokhov, A., Gebetsberger, M., Goutail, F., Grutter de la Mora, M., Gruzdev, A., Gratsea, M., Hansen, G. H., Irie, H., Jepsen, N., Kanaya, Y., Karagkiozidis, D., Kivi, R., Kreher, K., Levelt, P. F., Liu, C., Müller, M., Navarro Comas, M., Piters, A. J. M., Pommereau, J.-P., Portafaix, T., Prados-Roman, C., Puentedura, O., Querel, R., Remmers, J., Richter, A., Rimmer, J., Rivera Cárdenas, C., Saavedra de Miguel, L., Sinyakov, V. P., Stremme, W., Strong, K., Van Roozendael, M., Veefkind, J. P., Wagner, T., Wittrock, F., Yela González, M., and Zehner, C.: Ground-based validation of the Copernicus Sentinel-5P TROPOMI NO2 measurements with the NDACC ZSL-DOAS, MAX-DOAS and Pandonia global networks, Atmos. Meas. Tech., 14, 481–510, https://doi.org/10.5194/amt-14-481-2021, 2021.
Wagner, T., Dix, B., Friedeburg, C. V., Frieß, U., Sanghavi, S., Sinreich, R., and Platt, U.: MAX-DOAS O4 measurements: A new technique to derive information on atmospheric aerosols—Principles and information content, J. Geophys. Res.-Atmos., 109, D22205, https://doi.org/10.1029/2004jd004904, 2004.
Wang, C., Wang, T., Wang, P., and Rakitin, V.: Comparison and Validation of TROPOMI and OMI NO2 Observations over China, Atmosphere, 11, 636, https://doi.org/10.3390/atmos11060636, 2020.
Wang, S., Cohen, J. B., Lin, C., and Deng, W.: Constraining the relationships between aerosol height, aerosol optical depth and total column trace gas measurements using remote sensing and models, Atmos. Chem. Phys., 20, 15401–15426, https://doi.org/10.5194/acp-20-15401-2020, 2020.
Wang, S., Cohen, J. B., Deng, W., Qin, K., and Guo, J.: Using a New Top-Down Constrained Emissions Inventory to Attribute the Previously Unknown Source of Extreme Aerosol Loadings Observed Annually in the Monsoon Asia Free Troposphere, Earths Future, 9, e2021EF002167, https://doi.org/10.1029/2021ef002167, 2021.
Wang, S., Ma, X., Zhou, S., Wu, L., Wang, H., Tang, Z., Xu, G., Jing, Z., Chen, Z., and Gan, B.: Extreme atmospheric rivers in a warming climate, Nat. Commun., 14, 3219, https://doi.org/10.1038/s41467-023-38980-x, 2023.
Wang, S., Cohen, J. B., Guan, L., Tiwari, P., and Qin, K.: Classifying and quantifying decadal changes in wet deposition over Southeast and East Asia using EANET, OMI, and GPCP, Atmos. Res., 304, 107400, https://doi.org/10.1016/j.atmosres.2024.107400, 2024.
Wang, S. W., Zhang, Q., Streets, D. G., He, K. B., Martin, R. V., Lamsal, L. N., Chen, D., Lei, Y., and Lu, Z.: Growth in NOx emissions from power plants in China: bottom-up estimates and satellite observations, Atmos. Chem. Phys., 12, 4429–4447, https://doi.org/10.5194/acp-12-4429-2012, 2012.
Wang, Y. and Liu, D.: Reconstruction of satellite chlorophyll-a data using a modified DINEOF method: a case study in the Bohai and Yellow seas, China, Int. J. Remote Sens., 35, 204–217, https://doi.org/10.1080/01431161.2013.866290, 2013.
Wang, Y., Beirle, S., Lampel, J., Koukouli, M., De Smedt, I., Theys, N., Li, A., Wu, D., Xie, P., Liu, C., Van Roozendael, M., Stavrakou, T., Müller, J.-F., and Wagner, T.: Validation of OMI, GOME-2A and GOME-2B tropospheric NO2, SO2 and HCHO products using MAX-DOAS observations from 2011 to 2014 in Wuxi, China: investigation of the effects of priori profiles and aerosols on the satellite products, Atmos. Chem. Phys., 17, 5007–5033, https://doi.org/10.5194/acp-17-5007-2017, 2017.
Wang, Z., Wang, G., Guo, X., Bai, Y., Xu, Y., and Dai, M.: Spatial reconstruction of long-term (2003–2020) sea surface pCO2 in the South China Sea using a machine-learning-based regression method aided by empirical orthogonal function analysis, Earth Syst. Sci. Data, 15, 1711–1731, https://doi.org/10.5194/essd-15-1711-2023, 2023.
Wei, J., Li, Z., Li, K., Dickerson, R. R., Pinker, R. T., Wang, J., Liu, X., Sun, L., Xue, W., and Cribb, M.: Full-coverage mapping and spatiotemporal variations of ground-level ozone (O3) pollution from 2013 to 2020 across China, Remote Sens. Environ., 270, 112775, https://doi.org/10.1016/j.rse.2021.112775, 2022.
Wenig, M., Spichtinger, N., Stohl, A., Held, G., Beirle, S., Wagner, T., Jähne, B., and Platt, U.: Intercontinental transport of nitrogen oxide pollution plumes, Atmos. Chem. Phys., 3, 387–393, https://doi.org/10.5194/acp-3-387-2003, 2003.
Wu, Y., Di, B., Luo, Y., Grieneisen, M. L., Zeng, W., Zhang, S., Deng, X., Tang, Y., Shi, G., Yang, F., and Zhan, Y.: A robust approach to deriving long-term daily surface NO2 levels across China: Correction to substantial estimation bias in back-extrapolation, Environ. Int., 154, 106576, https://doi.org/10.1016/j.envint.2021.106576, 2021.
Xia, M. and Jia, K.: Reconstructing Missing Information of Remote Sensing Data Contaminated by Large and Thick Clouds Based on an Improved Multitemporal Dictionary Learning Method, IEEE T. Geosci. Remote, 60, 1–14, https://doi.org/10.1109/TGRS.2021.3095067, 2022.
Xu, R., Tong, D., Xiao, Q., Qin, X., Chen, C., Yan, L., Cheng, J., Cui, C., Hu, H., Liu, W., Yan, X., Wang, H., Liu, X., Geng, G., Lei, Y., Guan, D., He, K., and Zhang, Q.: MEIC-global-CO2: A new global CO2 emission inventory with highly-resolved source category and sub-country information, Sci. China Earth Sci., 67, 450–465, https://doi.org/10.1007/s11430-023-1230-3, 2024.
Zhai, B. and Chen, J.: Development of a stacked ensemble model for forecasting and analyzing daily average PM2.5 concentrations in Beijing, China, Sci. Total Environ., 635, 644–658, https://doi.org/10.1016/j.scitotenv.2018.04.040, 2018.
Zhang, W., Quan, H., and Srinivasan, D.: Parallel and reliable probabilistic load forecasting via quantile regression forest and quantile determination, Energy, 160, 810–819, https://doi.org/10.1016/j.energy.2018.07.019, 2018.
Zhou, W., Peng, B., and Shi, J.: Reconstructing spatial–temporal continuous MODIS land surface temperature using the DINEOF method, J. Appl. Remote Sens., 11, 1–15, https://doi.org/10.1117/1.JRS.11.046016, 2017.
Zhou, W., Qin, K., He, Q., Wang, L., Luo, J., Xie, W.: Comparison and Optimization of Ground-Level NO2 Concentration Estimation in China Based on TROPOMI and OMI, Acta Opt. Sin., 44, 0601010, https://doi.org/10.3788/AOS231013, 2024.