the Creative Commons Attribution 4.0 License.
the Creative Commons Attribution 4.0 License.
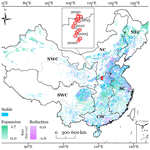
CIrrMap250: annual maps of China's irrigated cropland from 2000 to 2020 developed through multisource data integration
Ling Zhang
Yanhua Xie
Xiufang Zhu
Qimin Ma
Luca Brocca
Accurate maps of irrigation extent and dynamics are crucial for studying food security and its far-reaching impacts on Earth systems and the environment. While several efforts have been made to map irrigated area in China, few have provided multiyear maps, incorporated national land surveys, addressed data discrepancies, and considered the fractional coverage of cropland within coarse-resolution pixels. Here, we addressed these important gaps and developed new annual maps of China's irrigated cropland from 2000 to 2020, named CIrrMap250 (China's irrigation map with a 250 m resolution). We harmonized irrigation statistics and surveys and reconciled them with remote sensing data. The refined estimates of irrigated area were then integrated with multiple remote sensing data (i.e. vegetation indices, hybrid cropland products, and paddy field maps) and an irrigation suitability map by means of a semi-automatic training approach. We evaluated our CIrrMap250 maps using ∼ 20 000 reference samples, high-resolution irrigation water withdrawal data, and existing local to nationwide maps. Our CIrrMap250 maps demonstrated an overall accuracy of 0.79–0.88 for the years 2000, 2010, and 2020 and outperformed currently available maps. The CIrrMap250-estimated irrigation area explained 50 %–60 % of the variance in irrigation water withdrawal across China. CIrrMap250 revealed that China's irrigation area increased by about 180 000 km2 (or 25 %) from 2000 to 2020, with the majority (61 %) occurring in the water-unsustainable regions facing severe to extreme water stress. Moreover, our product unveiled a noticeable northward shift of China's irrigation area, attributed to substantial expansions in irrigated cropland across northeastern and northwestern China. The accurate representation of irrigation extent in CIrrMap250 will greatly support hydrologic, agricultural, and climate studies in China, aiding in improved water and land resources management. CIrrMap250 can be accessed at https://doi.org/10.6084/m9.figshare.24814293.v2 (Zhang et al., 2023a).
- Article
(11271 KB) - Full-text XML
-
Supplement
(2354 KB) - BibTeX
- EndNote
Irrigation is becoming increasingly important as an adaption strategy in response to climate change (Zaveri and Lobell, 2019; Bhattarai et al., 2023) and plays a vital role in ensuring food security by reducing both water and heat stresses of crops (Zhu and Burney, 2022; Zhu et al., 2022). Covering 20 % of global croplands, irrigated agriculture contributes to 40 % of global food production (UNESCO World Water Assessment Programme, 2019). However, it uses 60 %–70 % of total freshwater withdrawals and 80 %–90 % of consumptive water uses (Qin et al., 2022; Wu et al., 2022). The extensive use of irrigation water intensifies water management and drives a myriad of Earth system and environmental impacts (McDermid et al., 2021, 2023). These impacts include changes in hydroclimatic and biogeochemical cycling (Kang and Eltahir, 2018; Mishra et al., 2020; Thiery et al., 2020; Guo and Zhou, 2022; Yang et al., 2023), depletion of aquifers and surface waterbodies (Cheng et al., 2014; Noori et al., 2021), freshwater salinization (Thorslund et al., 2021), and landslides (Lacroix et al., 2020). Given the vital importance of irrigation, knowing its precise location and dynamics is essential. However, this proves to be challenging due to the hidden nature of irrigation signals and the frequent confusion between irrigated and rainfed fields (Ozdogan and Gutman, 2008; L. Zhang et al., 2022; Chen et al., 2023).
Remote sensing provides significant opportunities for cost-effective and spatially explicit mapping of land surfaces (Potapov et al., 2021). Over the past decade, there has been growing interest in using Earth observations to map irrigation extent (Massari et al., 2021). The existing remote sensing methods for irrigation mapping are generally based on three indicators: vegetation greenness, soil moisture, and integrated vegetation–soil moisture. Vegetation indices derived from optical sensors, such as the normalized difference vegetation index (NDVI) (Rouse et al., 1974), green index (GI) (Gitelson, 2005), and normalized difference water index (NDWI) (Gao, 1996; McFeeters, 1996), have been widely employed to detect irrigated areas based on the underlying fact that irrigated fields typically exhibit higher productivity and greenness compared to adjacent rainfed ones, especially under drought conditions. Techniques used include threshold splitting (Ozdogan et al., 2010; Zhu et al., 2014; Esmaeili et al., 2023; Wang et al., 2023), spectral matching (Ozdogan and Gutman, 2008; Lu et al., 2021), decision trees (Ozdogan and Gutman, 2008; Shahriar Pervez et al., 2014; Ambika et al., 2016; Xiong et al., 2017), and supervised classification (Deines et al., 2017, 2019; Xie et al., 2019). The soil-moisture-based approach utilizes remotely sensed soil moisture signals from microwave and optical sensors to detect irrigated areas by using similar techniques like threshold splitting (Yao et al., 2022) and supervised or unsupervised classification (Gao et al., 2018; Dari et al., 2021). The rationale behind this approach is that irrigation alters soil moisture, leading to distinct spatiotemporal dynamics compared to adjacent rainfed areas. The vegetation–soil moisture integration approach combines vegetation indices with soil moisture for irrigation detection. This approach has gained attention and achieved success in recent years (Pun et al., 2017; Elwan et al., 2022; Longo-Minnolo et al., 2022; Zuo et al., 2023), leveraging the strengths of both vegetation- and soil-moisture-based methods for more accurate irrigation mapping.
Despite significant advancements, broad-scale mapping of irrigated areas (e.g. national and global levels) remains challenging due to substantial variations in irrigation practices, landscapes, and climatic characteristics (Salmon et al., 2015; L. Zhang et al., 2022). This challenge is further compounded by the lack of sufficient ground reference data (Xie et al., 2019; Xie and Lark, 2021). Consequently, high-quality irrigation maps are still missing in most countries (Chen et al., 2023; Mpakairi et al., 2023). In recent years, researchers have sought to address the challenges of large-scale irrigation mapping by integrating remote sensing data, agricultural statistics, existing irrigation maps, and other relevant datasets such as those of irrigation suitability (Meier et al., 2018; Xie et al., 2021; C. Zhang et al., 2022a; L. Zhang et al., 2022). Researchers have successfully generated new irrigation maps at the global and national scales, featuring higher spatial resolutions and mapping accuracy compared to existing products. These efforts underscore the great potential of multisource data fusion techniques for large-scale irrigation mapping.
China is a big agricultural country with the largest irrigated area in the world (International Commission on Irrigation and Drainage, 2018). With only 8 % of the world's cropland, China feeds 20 % of the global population and has a tight connection with the food supply chain of other nations. Therefore, developing reliable maps of irrigated cropland is particularly important for sustainable food production in China. Despite this, less attention has been devoted to mapping irrigated areas in China compared to in other countries with extensive irrigation, such as the United States and India (Zhu et al., 2014; L. Zhang et al., 2022). It is only in recent years that maps of irrigated cropland specifically tailored for China have emerged, facilitated by the integration of multisource data, including remote sensing, reported statistics, and existing land use and/or cover maps (Xiang et al., 2020; Bai et al., 2022; C. Zhang et al., 2022b, c; L. Zhang et al., 2022).
While previous studies have considerably improved our understanding of the spatial distribution of irrigated cropland in China, limitations remain. First, few studies have provided annual irrigation maps, hindering the spatiotemporal analysis of China's irrigated areas. As a result, it remains unclear where the changes in irrigation area are water-sustainable (e.g. irrigation expansion in places without water stress) (Mehta et al., 2024). Second, irrigation area data from official statistical bureaus, collected through field-sampling surveys and bottom-up aggregation, have been extensively utilized to constrain the overall extent of irrigated cropland in previous studies. Besides statistical data, the National Land Surveys conducted by the State Council of China also provide estimates of irrigated cropland acreage. The surveys involve many investigators and rely on high-resolution satellite remote sensing imagery and advanced survey techniques (Chen et al., 2022). Harmonizing irrigation statistics with the National Land Surveys could potentially help to reduce biases and uncertainties in each data source (Yu et al., 2021), but this has rarely been taken into account. Third, the majority of farms in China are small and fragmented, with the average farmland size being smaller than 1 ha (Teluguntla et al., 2018). This leads to the widespread presence of mixed pixels where cropland and other land use and/or cover types coexist. However, in most previous studies, binary cropland masks were used for irrigation mapping. These masks classify each pixel as either cropland or non-cropland, neglecting the fractional coverage of cropland within coarse-resolution pixels. This may lead to the overestimation or underestimation of irrigation extent. Finally, it is worth noting that, apart from Zhang et al. (2022a), many studies assessed their irrigation maps with a limited number of reference samples, potentially compromising the reliability of their evaluation results (Zhu et al., 2014; Xiang et al., 2020; Bai et al., 2022; L. Zhang et al., 2022). Obtaining sufficient reference samples is crucial for robust evaluations of national-scale irrigated-cropland maps, a task that is, however, challenging due to the substantial cost and time involved.
Building on our previous work (L. Zhang et al., 2022, 2024), this study aims to bridge these gaps and create new annual maps of irrigated cropland in China (2000–2020) by integrating remote sensing data (i.e. vegetation indices, hybrid cropland maps, and paddy field maps), reported statistics and surveys, and an irrigation suitability map. The newly developed maps (CIrrMap250) feature a spatial resolution of 250 m at an annual frequency from 2000 to 2020. Our maps show the percentage of each 250 m × 250 m pixel that is covered by irrigated cropland (i.e. pixel value = irrigated areapixel area × 100). Other objectives of this study are as follows: (i) assessing the accuracy of CIrrMap250 using ∼ 20 000 reference samples and high-resolution irrigation water withdrawal data; (ii) comparing the performance of CIrrMap250 with four existing local to nationwide irrigation maps, including IrriMap_CN (C. Zhang et al., 2022a), IAAA (Siddiqui et al., 2016), GFSAD (Thenkabail et al., 2016), and OPTRAM30 (Yao et al., 2022); and (iii) investigating the spatiotemporal dynamics of China's irrigation extent and quantifying the water sustainability of changes in irrigated area.
2.1 Remote sensing data
We collected the Terra Moderate Resolution Imaging Spectroradiometer (MODIS) MOD13Q1 vegetation indices, i.e. NDVI and enhanced vegetation index (EVI) (Huete et al., 1997), from NASA's Earth Science Data Systems (https://www.earthdata.nasa.gov/, last access: 6 November 2024). These indices are generated every 16 d with a 250 m spatial resolution. Meanwhile, the MODIS band-4 (545–565 nm) surface reflectance from the MOD09A1 product was used and resampled from the original 500 to 250 m using the nearest-neighbour interpolation method (Debeurs and Townsend, 2008). The resampled data were then used together with the 250 m and 8 d band-1 (620–670 nm) surface reflectance from MOD09Q1 to derive the greenness index (GI) (Table S1 in the Supplement). We extracted MODIS data for all cropland pixels in China, using only high-quality data on cloud- and snow- or ice-free pixels (Hilker et al., 2012). Low-quality MODIS data were excluded based on the quality band and were interpolated using high-quality data from the nearest neighbouring cropland pixels.
We created a new 30 m resolution hybrid cropland product for China (CCropLand30) by fusing state-of-the-art remote sensing land use and/or cover products with the latest national land surveys (Zhang et al., 2024). CCropLand30 was generated at a 5-year interval from 2000 to 2020 and exhibited a higher accuracy compared to existing products (Zhang et al., 2024). Building upon CCropLand30, we developed 250 m resolution cropland layers for the years 2000, 2005, 2010, 2015, and 2020, which show the cropland proportion within each 250 m grid. Additionally, we extracted paddy fields from China's land use and/or cover dataset (CLUD) for the years 2000, 2005, 2010, 2015, and 2020 (Liu et al., 2014; Xu et al., 2018). Paddy fields, which include cultivated land where rice and lotus roots are grown and supported by water and irrigation facilities, were considered to be part of irrigated cropland with high confidence (C. Zhang et al., 2022c).
2.2 Irrigation statistics and surveys
2.2.1 Harmonization of irrigation statistics and surveys
We collected annual irrigation area data (2000–2020) from various statistical yearbooks provided by the National Bureau of Statistics of China and by local statistical bureaus. These yearbooks include the Provincial Statistical Yearbook, the Rural Statistical Yearbook, the China Statistical Yearbook for Regional Economy, and the China Water Statistical Yearbook. The data were sourced from the China Economic and Social Big Data Research Platform (https://data.cnki.net/, last access: 6 November 2024). We compiled county-level irrigation data for over 80 % of the provinces and prefecture-level data for the rest (L. Zhang et al., 2022), which provide more irrigation information for China than earlier studies (Zhu et al., 2014; Xiang et al., 2020; C. Zhang et al., 2022b).
In addition to statistical data, we utilized land survey data to obtain more detailed and reliable information on irrigated areas for select years. Currently, China has conducted three rounds of National Land Surveys (1980s, 2010, and 2020). The surveys engaged a significant number of surveyors and utilized high-resolution satellite remote sensing imagery, along with advanced survey techniques like mobile internet, cloud computing, and drones (Chen et al., 2022). Due to national security concerns, the land survey maps were not publicly available. However, the Ministry of Natural Resources recently released the county-level survey results of the second and third National Land Surveys, including data on cropland and its sub-categories (dryland, irrigated land, and paddy field) (https://www.mnr.gov.cn/, last access: 6 November 2024). Within the dataset, the surveyed irrigated land and paddy fields reflect the extent of irrigated cropland, covering the periods 2009–2016 and 2019–2022. For the years with survey data, irrigation statistics were harmonized with the survey data at the county scale using Eq. (1). The data harmonization was based on two assumptions: (1) the maximum value between statistical and surveyed irrigation area should be more reliable, and (2) irrigation area should be smaller than the total cropland area. The first assumption accounts for the underestimation tendency of both statistical and survey data due to possible insufficient and non-representative field sampling (C. Zhang et al., 2022a) and the prevalence of fragmented and small crop fields (Teluguntla et al., 2018). Alternative harmonization methods, such as mean and minimum values, were also tested but performed worse than the maximum harmonization approach. For years without survey data, the irrigation area was estimated by adjusting the harmonized data from adjacent survey years using relative-change information derived from the irrigation statistics (Eq. 2). This method preserved the interannual changes observed in statistical irrigation area while enhancing data consistency across years.
In the above, Aharm, Astat, and Asurv represent the harmonized, statistical, and surveyed irrigation area, respectively; CA is the surveyed area of cropland; and ts and t2 indicate the year with and without land surveys, respectively.
2.2.2 Reconciliation between statistical/survey data and remote sensing data
Cropland area statistics and survey data are inherently incompatible with remote sensing data due to different measurement techniques. While statistical and survey data measure the net area of cropland, remote sensing data represent the gross area of cropland, including subpixels; non-cropland features such as field ridges; linear elements; and scattered features like roads, ponds, and rural houses (Zhang et al., 2024). As a result, statistical and surveyed cropland areas exhibit a negative and systematic bias compared to those derived from remote sensing data (Zhang et al., 2021; L. Zhang et al., 2022). Similarly, as a subset of cropland, irrigated cropland is also reported in statistics and surveys as a net area that is different from in remote sensing data. Directly using the statistical or surveyed irrigation acreage to constrain remote-sensing-based irrigated cropland would likely result in underestimating the irrigation extent (Schepaschenko et al., 2015). To address this discrepancy, we adjusted the harmonized irrigation area (Sect. 2.2.1) to reconcile the statistical and survey data with remote sensing data, as shown in Eq. (3). This adjustment was performed based on the assumption that the proportion of irrigated cropland remains consistent in the statistical and survey data and the remote-sensing-derived maps. For instance, if statistical or survey data indicate that 99 % of the cropland in a given county is irrigated, the remote-sensing-derived irrigation proportion should also be approximately 99 %.
In the above, and are the reconciled and harmonized irrigation area, respectively, for the year t; is the remote-sensing-derived cropland area estimated from our hybrid cropland product (Zhang et al., 2024); is the surveyed cropland area; and denotes the bias ratio of remote-sensing-derived cropland area relative to surveys. This ratio was estimated for each county and constrained to the median value of all counties in its agricultural zones (C. Zhang et al., 2022c) to exclude extreme bias ratios and to ensure a conservative adjustment. In years lacking survey data, the bias ratio was estimated using a straightforward nearest-neighbour interpolation method.
2.3 Auxiliary data
This study utilized various auxiliary datasets (Table S2 in the Supplement), including meteorological and environmental variables, irrigation water withdrawal, water scarcity index, and administrative boundaries. Daily meteorological observations such as precipitation, relative humidity, air temperature, and pressure were collected from approximately 2400 meteorological stations across China, provided by the National Meteorological Information Center (NMIC, http://data.cma.cn/, last access: 6 November 2024). These datasets were combined with the MCD43A3 albedo product to compute daily potential evapotranspiration (PET) using the Priestley–Taylor method (Priestley and Taylor, 1972). The daily PET values were aggregated to annual values for the period from 2000 to 2020, which were then used to derive the aridity index, defined as the ratio of precipitation to PET. The environmental variables included elevation, slope, cropping intensity, soil type, and distance to waterbodies. Elevation data were sourced from the Shuttle Radar Topography Mission digital elevation model (SRTM DEM), and the slope map was generated from the SRTM DEM data using the slope function in ArcGIS. The distance to waterbodies was calculated based on the spatial distribution of waterbodies (rivers, lakes, reservoirs, canals, and ponds) using the Euclidean distance tool in ArcGIS. The above auxiliary data were partly obtained from the National Tibetan Plateau (https://data.tpdc.ac.cn/, last access: 6 November 2024), and the remaining data were obtained from the Resource and Environment Science and Data Center (https://www.resdc.cn/Default.aspx, last access: 6 November 2024).
Additionally, the prefecture-level irrigation water withdrawal data for 2009–2011 and 2018–2020 were compiled from provincial water resource departments and local statistical bureaus. The prefecture-level data on water scarcity index (WSI) for 2010–2020 were extracted from our previous study (Zhang et al., 2023b). WSI is defined as the ratio of total water use to water availability, as shown in Table S2 in the Supplement. Total water use encompasses both groundwater and surface water withdrawals for irrigation, industry, domestic purposes, forestry, livestock, and fishery. Water availability refers to the total surface water and groundwater generated by precipitation.
In this study, we created annual maps of irrigated cropland in China by integrating multisource data through a semi-automatic training approach (Xie et al., 2019; L. Zhang et al., 2022). After acquiring and processing the data, our methodology started with the creation of training samples, as depicted in Fig. 1. This step involves three major processes: (i) generating intermediate irrigation maps through a threshold calibration method, (ii) establishing a training pool (i.e. potential training data) via overlay analysis of the intermediate maps, and (iii) generating training samples through random sampling from the training pool. Using these training samples, we classified irrigated and rainfed cropland in each county annually using the random forest algorithm (Breiman, 2001). The resulting county-level maps were then mosaicked and post-processed to produce the annual maps of irrigated cropland in China, referred to as CIrrMap250. Subsequently, we evaluated the accuracy of CIrrMap250 and compared it with existing products. Finally, we examined the spatiotemporal changes in irrigated croplands and quantified the water sustainability of irrigation expansion by relating them to water stress areas.
3.1 Generation of training samples
We applied a threshold calibration method to automatically generate the training pool, following previous studies by Xie et al. (2019, 2021) and L. Zhang et al. (2022). With this method, cropland pixels with annual peak vegetation greenness exceeding an optimized threshold were classified as “irrigated”. The threshold was individually calibrated for each county and year using available irrigation statistics and surveys. Based on the calculated optimized thresholds, intermediate irrigation maps were generated at the county level. Pixels consistently classified as irrigated in all intermediate maps were identified as irrigation candidates, while those classified as non-irrigated were considered to be potential non-irrigated samples.
In this study, we first calculated the peak values of vegetation indices (NDVI, EVI, and GI) for cropland grids in each year and adjusted them by irrigation suitability. A static irrigation suitability map was created based on the elevation, slope, and aridity index of cropland. These factors play a crucial role in shaping the spatial distribution of irrigated cropland in China, as demonstrated by Liu et al. (2022). Cropland areas characterized by lower elevation, gentler slopes, and higher aridity indices were hypothesized to exhibit greater irrigation suitability and potential, in line with previous studies (Worqlul et al., 2015, 2017; Li and Chen, 2020; L. Zhang et al., 2022). Specifically, the irrigation suitability map was derived by combining irrigation suitability values of elevation, slope, and aridity index, as in Eq. (4):
where is the irrigation suitability for cropland cell i in county jof province k; w is the weight of the influencing factors, which was determined through a trial-and-error procedure; SElev, SSlope, and SArid are the irrigation suitability values of elevation, slope, and aridity index, respectively (Table S3 in the Supplement). The peak vegetation index was subsequently adjusted by irrigation suitability (Eq. 5), with the assumption that irrigated cropland, being greener and more productive, is also more suitable for irrigation compared to rainfed cropland.
In the above, SVI denotes the irrigation-suitability-adjusted peak vegetation index; VI denotes the vegetation index value; and g and t represent the growth period and year, respectively.
We then generated three intermediate irrigation maps annually from 2000 to 2020 utilizing the SVI (i.e. irrigation-suitability-adjusted peak NDVI, EVI, and GI) and the paddy field maps. This was achieved through a threshold-splitting method (Pervez and Brown, 2010; Zhu et al., 2014; Meier et al., 2018). Specifically, the SVI values for all cropland pixels within each county were ranked in a descending order, and the cumulative irrigated area was sequentially calculated. The accumulated area was then compared with the reconciled irrigation area. The SVI value at which the cumulative irrigated area closely matched the reconciled irrigated area was identified as the optimal threshold. Notably, for paddy fields, the SVI value was set to the maximum SVI among croplands within a county, prioritizing these areas as irrigated. The cropland grids were finally classified into irrigated and rainfed categories using Eq. (6).
The intermediate irrigation maps were finally overlaid to identify pixels consistently classified as irrigated or rainfed cropland across these maps. These pixels were designated as potential training samples, forming the training pool for each county and year. From the training pool, we randomly selected 200 rainfed pixels and 200 irrigated pixels to train the random forest model. This selection ensures a balance between the need for an adequate number of samples and the computational efficiency of the classification algorithm (Xie et al., 2019; L. Zhang et al., 2022).
3.2 Classification of irrigated cropland using random forest
We employed the random forest algorithm (Breiman, 2001) to classify irrigated and rainfed cropland using the random samples extracted from the training pool. The hyperparameters of our model were optimized through a trial-and-error process, including the number of trees, the minimum number of observations per node, and the number of variables randomly sampled at each decision split (Table S4 in the Supplement). The input features of our model encompassed both time-varying variables (i.e. vegetation indices, precipitation, temperature, PET, and aridity index) and stable environmental variables (i.e. latitude, longitude, cropping intensity, elevation, distance to waterbodies, slope, and soil type). The classification was conducted independently for each county per year from 2000 to 2020. After that, we merged the annual, county-level mapping results to generate preliminary binary irrigation maps in China (i.e. 1 for irrigated and 0 for non-irrigated).
We then employed a spatial filtering to remove isolated irrigation pixels and to identify potentially omitted irrigated croplands. Specifically, we first calculated the irrigation proportion within a 7 × 7 pixel window for each preliminary irrigation pixel. Then, all cropland pixels within the moving window were classified as non-irrigated if the calculated ratio fell below 5 %. Conversely, if the ratio exceeded 95 %, we assumed all cropland pixels within the moving window to be irrigated. The spatial-filtering operation preserved the original spatial resolution of the maps (250 m).
Finally, we multiplied the binary, spatially filtered irrigation maps by their corresponding cropland mask layers to generate annual irrigation maps for China. The final product, CIrrMap250, represents the percentage of a 250 m pixel covered by irrigated croplands (i.e. pixel value = irrigated areapixel area × 100). Unlike simple binary maps, our product considers the fractional coverage of croplands within coarse-resolution MODIS pixels, thereby enhancing the accuracy of irrigation area estimates in China, where farms are typically small and fragmented.
3.3 Accuracy assessment and intercomparison
3.3.1 Assessment with reference points
We assessed the accuracy of CIrrMap250 using three independent sets of validation samples. The first validation dataset was for the year 2000 (Fig. 2a), obtained from Zhu et al. (2014), primarily derived from the crop growth and soil moisture dataset provided by the China Meteorological Data Sharing Service System (https://data.cma.cn/, last access: 6 November 2024). The second validation dataset for the year 2020 (Fig. 2c) was acquired from Chen et al. (2023), showing the global location of centre pivot irrigation systems (CPISs). We extracted the CPIS polygons across China (mainly distributed in northern China) and compared them with our product. In addition, we retrieved the validation samples for the year circa 2010 (Fig. 2b) from the provincial land use maps of China's second National Land Survey (https://www.mnr.gov.cn/, last access: 6 November 2024). We georeferenced these land use maps using the georeferencing tool in ArcGIS. A total of 234 control points were selected from high-resolution images and provincial administrative boundaries for the georeferencing process (Fig. S1 in the Supplement). The irrigation samples were randomly extracted from irrigated lands and paddy fields, while non-irrigated samples were taken from dryland patches. As shown in Fig. 2d, we obtained a total of 20 720 reference samples. The performance of CIrrMap250 was evaluated quantitatively using the overall accuracy (OA), F1 score, producer's accuracy (PA), and user's accuracy (UA) (Table S5 in the Supplement).
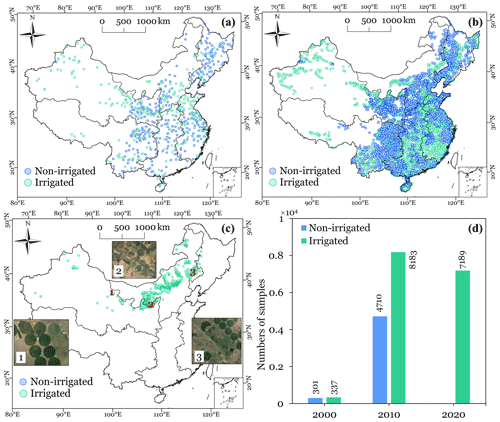
Figure 2Spatial distribution of validation samples. (a) Spatial distribution of the third-party samples in 2000. (b) Spatial distribution of the samples in 2010 retrieved from provincial land use maps of China's second National Land Survey. (c) Spatial distribution of the third-party samples in 2020. (d) Numbers of irrigated and non-irrigated samples for different years.
3.3.2 Assessment with irrigation water withdrawal data
We further assessed the performance of CIrrMap250 by comparing its irrigation area with prefecture-level irrigation water withdrawal for the years circa 2010 and 2020. Since irrigated area is a dominate driver of irrigation water withdrawal (Lamb et al., 2021; Puy et al., 2021), irrigation water withdrawal can indirectly evaluate the accuracy of irrigation maps (C. Zhang et al., 2022a). High-accuracy irrigation maps are expected to better explain the variations in irrigation water withdrawal compared to low-accuracy maps. The explanatory power of the irrigation area estimates was assessed by the coefficient of determination (R2) from a linear regression model fitted to the irrigation water withdrawal data using the least-squares method.
3.3.3 Comparison with existing products
We evaluated CIrrMap250 using three existing irrigation maps covering the entirety of China, including IrriMap_CN (C. Zhang et al., 2022a), IAAA (Siddiqui et al., 2016), and GFSAD (Thenkabail et al., 2016). IrriMap_CN provides annual irrigation maps across China for the years from 2000 to 2019 at a 500 m resolution and was developed using MODIS data and machine learning (C. Zhang et al., 2022a). The IAAA irrigation maps cover Asia and Africa for the years 2000 and 2010 at a 500 m resolution. These maps were created based on seasonal vegetation variations captured in MODIS data (Siddiqui et al., 2016). The 2010 global irrigation map, GFSAD, has a spatial resolution of 1000 m and was generated by overlaying dominant crops with remote-sensing-derived irrigated and rainfed cropland maps (Thenkabail et al., 2016).
Additionally, we evaluated our maps for the Hexi Corridor using a field-scale irrigation map specifically created for the region (Yao et al., 2022). The map, OPTRAM30, has a 30 m resolution and demonstrates an accuracy close to 100 % when validated against in situ datasets. In addition to assessing CIrrMap250, we also evaluated IrriMap_CN, IAAA, and GFSAD using OPTRAM30.
3.4 Irrigation area change and its correlation with water stress areas
We examined the irrigation trends in a spatially explicit manner using our new irrigation maps from 2000 to 2020. The trends were quantified as the slope of the regression line fitted to the time series irrigation data at the pixel scale using the least-squares method. Furthermore, we adopted the concept of “centre of gravity” to track the spatial dynamics of irrigated areas (Zeng and Ren, 2022). The gravity centre of the irrigated area (X, Y) is represented as follows:
where IrrArea denotes the irrigated area in grid i; xi and yi are the longitude and latitude of grid i, respectively; n is the number of irrigated cropland grids; and t is year.
In addition, we quantified the water sustainability of irrigation changes across China. To do so, we first identified the expansion and decline in irrigated areas between 2000 and 2020 at a 5 km resolution following previous studies (Deines et al., 2019; Xie and Lark, 2021). Subsequently, we compared the changes with a prefecture-level water stress map derived from the mean values of WSI over the period 2010–2020. WSI denotes the fraction of available water resources appropriated by humans and is employed to categorize water stress into four levels: low (WSI ≤ 0.2), moderate (0.2 < WSI ≤ 0.4), severe (0.4 < WSI ≤ 1.0), and extreme (WSI > 1) (Zhang et al., 2023b). Irrigation expansion under severe to extreme water stress was designated as unsustainable due to the potential of exacerbating the depletion of surface water and groundwater (Mehta et al., 2024). Conversely, the expansion of irrigation under low to moderate water stress and the shrinkage of irrigation under severe to extreme stress were deemed to be sustainable.
4.1 Accuracy assessment
4.1.1 Pixel-scale assessment
As shown in Fig. 3 and Table S6 in the Supplement, CIrrMap250 attains an OA and F1 score of 0.79 and 0.78, respectively, for the year 2000, surpassing the performance of IrriMap_CN and IAAA. In the year 2010, CIrrMap250 achieves a high OA of 0.79 and an F1 score of 0.71, whereas the existing maps attain OA values below 0.66 and F1 scores under 0.63. For the year 2020, CIrrMap250 detects 88 % of centre pivot irrigated fields, while IrriMap_CN identifies only 20 % (Fig. 3c and Fig. S2 in the Supplement). Note that both CIrrMap250 and IrriMap_CN achieve a perfect user's accuracy for the irrigation class in 2020 because all the reference points are irrigated samples (Sect. 3.3.1 and Table S7 in the Supplement). For irrigated samples, CIrrMap250 has a significantly higher producer's accuracy in 2000, 2010, and 2020 compared to the existing products. CIrrMap250 and IrriMap_CN perform similarly in terms of user's accuracy. For non-irrigated samples, the producer's accuracy of CIrrMap250 is slightly lower than that of IrriMap_CN, but the user's accuracy of CIrrMap250 is significantly higher than that of IrriMap_CN. In terms of the producer's accuracy and user's accuracy, both CIrrMap250 and IrriMap_CN outperform IAAA and GFSAD.
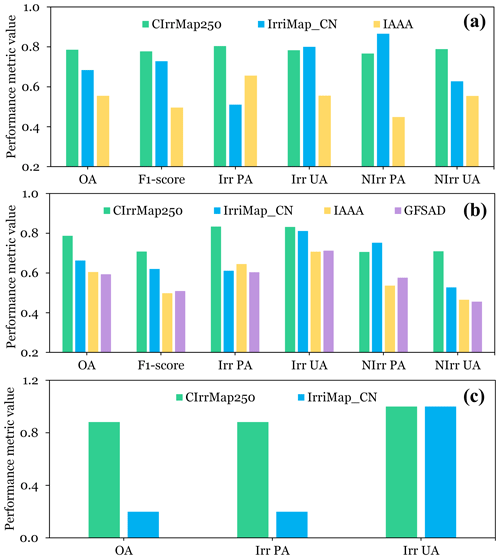
Figure 3Performance of CIrrMap250 and existing irrigation maps (IrriMap_CN, IAAA, GFSAD). Panels (a), (b), and (c) show the results for 2000, 2010, and 2020, respectively. OA, PU, and UA represent overall accuracy, producer's accuracy, and user's accuracy, respectively. Irr and NIrr indicate irrigated and non-irrigated samples, respectively.
4.1.2 Nationwide and regional comparison with existing products
Figure 4 shows the spatial distribution of irrigated cropland from different maps. At the national scale, CIrrMap250 and IrriMap_CN, specifically developed for China, capture similar irrigation patterns. They both show some irrigation hotspots (e.g. North China Plain and northwestern China) and well-known irrigation districts like Hetao, Baojixia, Dujiangyan, Qingtongxia, and Fenhe. However, CIrrMap250 shows more widespread irrigation than IrriMap_CN in most areas of China (Fig. S3 in the Supplement). IrriMap_CN estimates irrigation proportion (i.e. the ratio of irrigated cropland area to total cropland area) to be 0.47, 0.37, and 0.61 for China, northern China, and the Xinjiang Uygur Autonomous Region, respectively (Fig. S4 in the Supplement). In comparison, the values derived from CIrrMap250 are 0.58, 0.70, and 0.96, respectively, which align more closely with the official reports (https://gtdc.mnr.gov.cn/, last access: 6 November 2024). Nevertheless, CIrrMap250 tends to yield lower estimates of irrigation area in northeastern China (NEC) when compared to IrriMap_CN, possibly due to inaccurate statistical and survey data in this region. In contrast to CIrrMap250 and IrriMap_CN, IAAA notably underestimates irrigated croplands in northwestern China (NWC) and northern China (NC) but overestimates it in NEC and southwestern China (SWC). This could be explained by the fact that IAAA was developed using unsupervised classification (Siddiqui et al., 2016), limiting its ability to characterize the spatial heterogeneity of irrigation in China (Tian et al., 2024). GFSAD shows overestimations of irrigated area in the Dujiangyan district and the North China Plain but exhibits evident omission errors in sparsely distributed irrigation regions like NWC and southern China (SC). The large bias of GFSAD is understandable as it is not an irrigation-specific product and only covers five irrigated crops (Thenkabail et al., 2016; Xie et al., 2021).
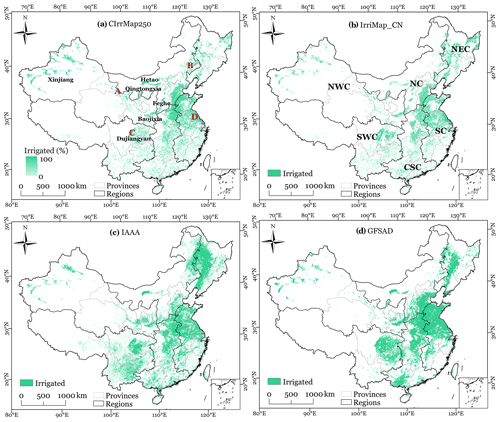
Figure 4Spatial distribution of irrigated cropland from different maps for the year 2010. NEC, NC, NWC, SWC, SC, and CSC represent northeastern China, northern China, northwestern China, southwestern China, southern China, and central southern China, respectively.
We further compared CIrrMap250 with existing maps in four heavily irrigated zones (A–D; locations are shown in Fig. 4a). Zones A and B are situated in arid regions where crop growth is not possible without irrigation, while zones C and D are in humid regions where paddy rice is widespread and relies heavily on supplemental irrigation. As shown in Fig. 5, CIrrMap250 accurately portrays the actual distribution of irrigated cropland in these zones. In contrast, IrriMap_CN underestimates irrigation extent in zones A and B and lacks detailed information in zones C and D. IAAA significantly underestimates irrigation area in zone A, incorrectly identifies it in zone B, and overestimates irrigated cropland in region C. The GFSAD product, with a relatively coarse resolution of 1 km, shows the lowest agreement with other maps.
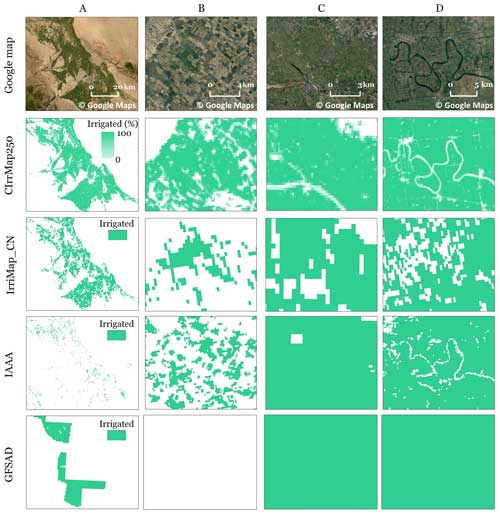
Figure 5Visual comparison of CIrrMap250 with existing maps. The five rows from top to bottom correspond to the Google map, CIrrMap250, IrriMap_CN, IAAA, and GFSAD, respectively. Locations of the four selected zones are presented in Fig. 4a.
When examining the Hexi Corridor (Fig. 6), CIrrMap250 exhibits a high agreement with OPTRAM30. While IrriMap_CN captures the general patterns, it tends to underestimate the overall irrigation extent, as demonstrated in zones I and II of the region (Fig. 6d). The IAAA product struggles to identify irrigated cropland in this area, displaying significant omission and commission errors. Similarly, GFSAD has a limited ability to accurately depict irrigated areas in the Hexi Corridor.
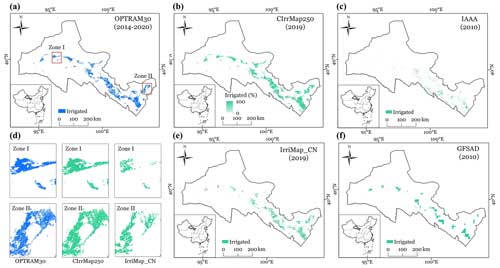
Figure 6Comparison of large-scale irrigation maps with the field-scale remote sensing irrigation map (OPTRAM30) in the Hexi Corridor of northwestern China. Panels (a), (b), (c), (e), and (f) depict the distribution of irrigated cropland in OPTRAM30, CIrrMap250, IAAA, IrriMap_CN, and GFSAD, respectively. Panel (d) shows the comparisons of CIrrMap250 and IrriMap_CN with OPTRAM30 in two local zones.
4.1.3 Comparison with irrigation water use data
As illustrated in Fig. 7, the CIrrMap250-estimated irrigation areas exhibit a notable correlation with irrigation water withdrawals. Irrigation area changes derived from CIrrMap250 account for approximately 50 % and 60 % of the variance in irrigation water withdrawals circa 2010 and 2020, respectively. In contrast, variations in irrigated area obtained from IrriMap_CN can only explain 40 % and 48 % of the variance in irrigation water withdrawals for 2010 and 2020, respectively. As shown in Fig. 7c and f, the irrigated area estimates from the other two maps (i.e. IAAA and GFSAD) demonstrate limited explanatory power, explaining only 12 % and 20 % of the variation in irrigation withdrawals for the year 2010. These results indirectly imply a superior performance of CIrrMap250 over existing maps.
4.2 Spatiotemporal changes in irrigated croplands
As depicted in Fig. 8, our CIrrMap250 revealed that irrigation area expanded significantly in NEC and NWC from 2000 to 2020. Conversely, notable decreases in irrigated areas were identified in the northern parts of SC and CSC, the northeastern part of SWC, and the southern parts of CSC and NC. The decline in irrigated areas tended to be concentrated in populous areas, attributable to the rapid urban expansion on cropland (Zhang et al., 2024). The gravity centre of irrigation was situated on the border of NC and CSC and exhibited a noticeable northward shift during the study period. This northward trend is likely to exacerbate the water crisis in northern China (Li et al., 2023), which has only 20 % of China's water resources but supports more than half of its population. The gravity centre showed clear trends in NWC, NEC, and NC but was insignificant in the remaining subregions (Fig. S5 in the Supplement). In NWC, irrigation significantly shifted to the northwest, while in NEC, it significantly shifted to the northeast. Meanwhile, there was a northward spatial trend in irrigation in NC.
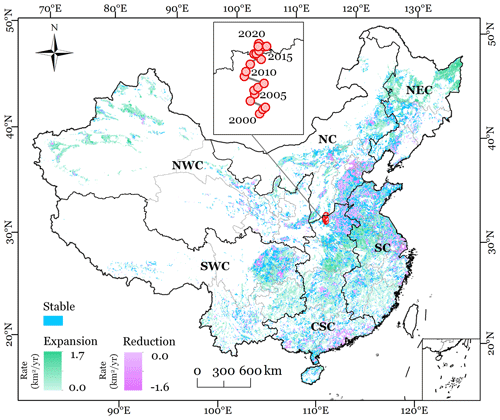
Figure 8Spatiotemporal changes in irrigated area from 2000 to 2020. Pixels exhibiting significant interannual trends (p<0.05) in irrigated area were labelled as “expansion” or “reduction”, while those with insignificant changes are denoted as “stable”. Pixels with less than 5 % irrigated croplands were excluded from the map. The inset panel on the top of the figure depicts the centre-of-gravity movement (spatial trend) of China's irrigated areas at the national scale.
As shown in Fig. 9, our annual irrigation maps indicated that all subregions exhibited an increasing trend in irrigated area from 2000 to 2020, with NEC expanding significantly faster than the other subregions. More specifically, China's irrigation area increased from about 760 000 to 940 000 km2 at an annual rate of 10 000 km2 (or 1.29 % yr−1). Despite the overall upward trend, changes in the proportion of irrigated area varied by subregion, with upward trends in NEC and NWC and decreasing trends in CSC, SC, and NC. SC accounted for the largest proportion of irrigated cropland in China (26 %–29 %), followed by CSC (22 %–24 %), NC (16 %–17 %), NWC (12 %–14 %), SWC (11 %), and NEC (7 %–11 %).
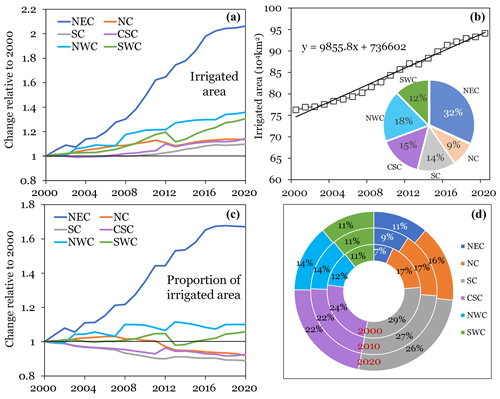
Figure 9Changes in irrigated area across the six subregions of China during 2000–2020. (a) Relative changes in irrigated area. (b) Changes in China's total irrigated area, with the contribution of different subregions depicted in the inserted pie chart. (c) Relative changes in proportion of irrigated area. (d) Proportion of irrigated area for the years 2000, 2010, and 2020.
4.3 Irrigation changes under different water stress levels
Figure 10 shows irrigation changes under different water stress levels. We found a gross irrigation expansion of ∼ 250 000 km2 in China from 2000 to 2020, of which 64 % was unsustainable from the perspective of water resources and was in regions with severe to extreme water stress. The expansion of irrigated area was mainly situated in NWC, NEC, NC, and the northern parts of CSC and SC. The gross reduction was about 70 000 km2, of which 72 % was in regions with severe to extreme water stress and could be considered to be sustainable. This sustainable reduction was primarily located in NC, CSC, and SC, which partly mitigated the unsustainable expansion in the regions. The net expansion of irrigated area was about 180 000 km2, of which 61 % was water-unsustainable. The subregions of NEC and NWC had a larger proportion of unsustainably expanded irrigated area compared to other subregions, accounting for about 70 % of China's net unsustainable irrigation expansion. In contrast, the subregions of CSC and SWC had a greater proportion of sustainable expansion than in other subregions due to the abundance of water resources and lower water stress there.
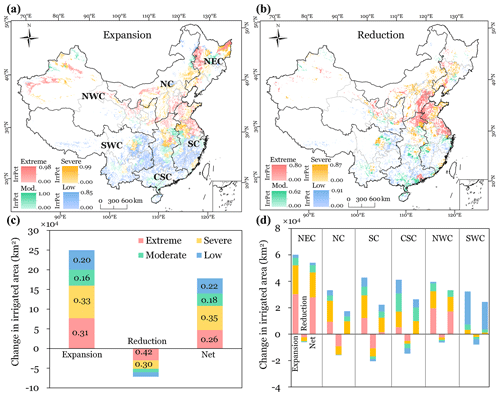
Figure 10Changes in irrigated area between 2000 and 2020 under different water stress levels. Panels (a) and (b) present the spatial distribution of gross expansions and reductions in irrigated area under four categories of water stress (i.e. low, moderate, severe, and extreme). Panel (c) shows the gross and net changes in irrigated area by water stress category for China, while panel (d) presents the results for the six subregions.
5.1 Improvement of CIrrMap250 over existing products
Our CIrrMap250 product provides annual maps of China's irrigated cropland from 2000 to 2020, exhibiting higher accuracy compared to existing products. The improved performance of CIrrMap250 can be attributed to several key factors. First, CIrrMap250 has digested unprecedentedly detailed irrigation statistics and reliable national land surveys and, at the same time, has reconciled the discrepancies between statistical and survey data and remote sensing data. We compiled county-level statistical data for 80 % of provinces in China, along with prefecture-level data for the remaining provinces. These datasets, for the first time, were harmonized with China's National Land Surveys, greatly reducing the errors and uncertainties in reported statistics. The harmonized irrigated-area data were further adjusted to reconcile the statistical and survey data with remote sensing data to account for their inconsistencies. Without data harmonization and reconciliation, the irrigation extent would be significantly underestimated, leading to a decrease in irrigation-mapping accuracy of 8 %–26 % (Fig. S6 in the Supplement).
Furthermore, CIrrMap250 considered the fractional coverage of cropland within coarse-resolution pixels rather than using binary cropland masks in most existing products. The majority of farms in China are small and fragmented. We observed that 37 % of China's cropland grids had cropland proportions below 50 % for the year 2020, and only 40 % of cropland grids showed cropland proportions above 90 %. Therefore, it becomes crucial to consider the fraction coverage of cropland in cropland masks for irrigation mapping. To underscore this necessity, we conducted an additional experiment, wherein we adopted the 250 m cropland masks that described cropland distribution in a binary manner (i.e. each pixel was classified as either cropland or non-cropland) for irrigation mapping. As depicted in Fig. S7 in the Supplement, a substantial portion of irrigated cropland would be overlooked if the fractional coverage of cropland were removed, particularly in southern China. The accuracy of the final irrigation maps would decrease by approximately 5 %–6 % if we used such binary cropland masks (Fig. S8 in the Supplement).
Lastly, CIrrMap250 has incorporated an irrigation suitability analysis based on the premise that irrigated cropland should not only be greener and more productive but also more suitable for irrigation compared to rainfed cropland. To demonstrate the importance of integrating irrigation suitability into the irrigation-mapping process, we randomly generated 250 sets of weights (assigned to the influencing factors) for all provinces in China, resulting in 250 distinct irrigation suitability maps. Based on these maps, we then created 250 different irrigated cropland maps for the year 2010 using the method proposed in this study. As shown in Fig. S9 in the Supplement, regardless of the choice of irrigation suitability maps, these irrigation maps consistently outperform the baseline irrigation map, which disregarded irrigation suitability during the mapping process. Furthermore, there is a narrow range (0.75–0.77) in the overall accuracy of these irrigation maps, implying the robustness (low sensitivity) of the mapping method in relation to the use of different irrigation suitability maps.
5.2 Uncertainties, limitations, and potential applications of CIrrMap250
Despite the advancements of CIrrMap250 compared to existing products, we acknowledge several uncertainties and limitations associated with the product. CIrrMap250 was developed by integrating data from multiple sources using a semi-automatic training method, leveraging joint information related to irrigation in each data source. However, each data source inherently presents uncertainties and deficiencies (Shahriar Pervez et al., 2014; Tian et al., 2024). Irrigation area statistics, in particular, can contain significant uncertainties due to technical and political factors, such as variations in statistical methods and administrative divisions (Thenkabail et al., 2009; Meier et al., 2018), which have not been well characterized. These biases and uncertainties would manifest in CIrrMap250 since our training samples were derived from these statistics-constrained irrigation maps. In this study, we addressed this issue by merging reported irrigation statistics with independent survey results. Nonetheless, uncertainties related to irrigated areas may remain unresolved in certain regions. For instance, we found considerable discrepancies between the statistical and surveyed irrigation areas in SC and NEC (Fig. S10a in the Supplement), implying greater uncertainties in these subregions compared to others. Furthermore, the irrigation statistics and surveys were reconciled with remote sensing data to address inconsistencies between the two sources. However, the bias ratio may be inaccurately estimated in the reconciliation process, introducing additional uncertainties into the results.
Cropland mask layers used to distinguish croplands from non-croplands are another source of uncertainty. These layers were constructed using our hybrid cropland product (Zhang et al., 2024), which integrates five state-of-the-art remote sensing land use and/or cover products. This hybrid product significantly reduced uncertainties associated with cropland distributions in China. However, remote-sensing-derived cropland data show large uncertainties in southern China. As illustrated in Fig. S10b in the Supplement, on average, only 27 % of croplands in SWC, SC, and CSC are consistently identified by remote sensing products compared to 39 % in the northern subregions (NEC, NC, and NWC). These uncertainties are reflected in our hybrid cropland product, which shows greater accuracy in the northern subregions than in the southern ones (Fig. S10c in the Supplement). Meanwhile, the temporal resolution of the cropland layers is 5 years, which may not accurately capture changes in cropland distribution in regions experiencing rapid changes. The uncertainties and errors in the cropland mask layer, particularly in southern China, could propagate into CIrrMap250.
An additional source of uncertainty is the MODIS-derived vegetation indices (i.e. NDVI, EVI, and GI). These indices are prone to data gaps due to cloud and cloud shadow contaminations. In this study, we filled the data gaps by using a simple nearest-neighbour interpolation method, which may introduce uncertainties into CIrrMap250. Additionally, irrigated croplands in humid southern China are more sparsely distributed and show weaker contrasts with rainfed fields compared to northern China. This makes the peak vegetation indices less effective and more uncertain in distinguishing irrigated from rainfed cropland (Xie et al., 2019; C. Zhang et al., 2022a). Consequently, our CIrrMap250 product exhibits higher accuracy in the NEC, NWC, and NC subregions than in the SC, CSC, and SWC subregions (Fig. S10d in the Supplement).
Lastly, CIrrMap250 has the limitation of a relatively coarse spatial resolution of 250 m and does not fully address the mixed-pixel problem. While CIrrMap250 offers a higher spatial resolution than many existing large-scale irrigation maps, it may not be suitable for local applications, such as field or irrigation district levels. The mixed-pixel problem significantly affects the precision of cropland masks (Zhang et al., 2024) and weakens the distinction between vegetation indices for irrigated and rainfed cropland. Even though CIrrMap250 considers the fractional coverage of cropland, it does not differentiate between irrigated and rainfed croplands at subpixel scales, like many other existing irrigation maps. There are many small and fragmented croplands in the mountainous regions of southern China. CIrrMap250 should be used with caution in these areas due to the prevalence of mixed pixels. Additionally, CIrrMap250 was created at an annual frequency and therefore does not provide monthly and seasonal irrigation information. A pixel is classified as irrigated if it has been irrigated at any point during the year, regardless of the specific month or season. While a growth-season-based irrigation product could be more desirable, it faces significant challenges, such as the lack of high-resolution crop type information (e.g. rice, wheat, maize) and high-quality training samples with sufficient temporal resolution.
Despite these limitations, CIrrMap250 makes a valuable contribution to the field of irrigation mapping and is poised to significantly support agricultural, hydrological, and climate studies, as well as water resource management in China. Ongoing efforts to address these limitations and to explore potential enhancements will undoubtedly improve the accuracy and utility of our irrigation maps in the future. One of the major applications of CIrrMap250 will be estimating irrigation water use or requirements, considering the fact that irrigated area is a dominate driver of irrigation water withdrawal (Ozdogan and Gutman, 2008; Puy et al., 2021). Secondly, the spatial detail provided by CIrrMap250 can be integrated into crop, hydrological, and climate models to improve the simulations of water uses and land–atmosphere interactions (Uniyal and Dietrich, 2021; McDermid et al., 2023; Yang et al., 2023). This integration will advance our understanding of how irrigation practices influence crop yield and of hydrological and climatic processes from local to nationwide scales. Lastly, CIrrMap250 provides insights into irrigation changes and can assist in optimizing the spatial distribution of irrigated croplands (Rosa et al., 2020a, b), thereby supporting more informed decisions for sustainable water and land use.
The annual maps of China's irrigated cropland from 2000 to 2020 (named CIrrMap250) can be accessed at https://doi.org/10.6084/m9.figshare.24814293.v2 (Zhang et al., 2023a). All maps are presented in GeoTIFF format, with the geographic coordinate of WGS84. The pixel size is 0.00225 × 0.00225 degrees (∼ 250 m × 250 m at the Equator). Our maps show the percentage of each 250 m pixel covered by irrigated cropland (i.e. pixel value = irrigated areapixel area × 100). Note that our product accounts for the fractional coverage of croplands within coarse-resolution MODIS pixels but does not differentiate between irrigated and rainfed croplands at subpixel scales. For example, if a pixel has 50 % cropland coverage, all cropland within that pixel would be classified as either irrigated or non-irrigated.
China, as a big agricultural country with extensive irrigation, underscores the critical importance of developing reliable irrigation maps for sustainable land–water–food nexus management. This study presented new annual maps of irrigated cropland in China spanning from 2000 to 2020, referred to as CIrrMap250. These maps were developed by integrating multisource data, including remote sensing data, reported statistics and surveys, and an irrigation suitability map. Validation against 20 720 reference samples demonstrated that our irrigation maps achieved high accuracy and outperformed the currently available products covering the entirety of China. The superiority of our product over existing maps was further confirmed through the assessments using irrigation water withdrawal data and local-scale visual comparisons. Based on the 21 years of data, we found a clear upward trend and northward shift in China's irrigation area. The irrigation expansion is particularly notable in water-scarce regions like northeastern China and northwestern China, potentially exacerbating water scarcity concerns. CIrrMap250 will significantly enhance agricultural, hydrological, and climate studies, as well as water resource management in China.
The supplement related to this article is available online at: https://doi.org/10.5194/essd-16-5207-2024-supplement.
LZ conceived the research, carried out the experiments, analysed the results, and prepared the paper with contributions from all the co-authors. YX analysed the results, provided the technical support, reviewed and edited the paper, and supervised the research. XZ and QM collected the validation dataset. LB reviewed and edited the paper and supervised the research.
The contact author has declared that none of the authors has any competing interests.
Publisher's note: Copernicus Publications remains neutral with regard to jurisdictional claims made in the text, published maps, institutional affiliations, or any other geographical representation in this paper. While Copernicus Publications makes every effort to include appropriate place names, the final responsibility lies with the authors. Regarding the maps used in this paper, please note that Figs. 2, 4, 6, 8, and 10 contain disputed territories.
We greatly appreciate the Ministry of Natural Resources of the People's Republic of China for data provision.
This study is supported by the National Natural Science Foundation of China (grant no. 42271286), the Youth Innovation Promotion Association of the Chinese Academy of Sciences (grant no. 2023454), and the Key Research Program of Gansu Province (grant no. 23ZDKA0004).
This paper was edited by Peng Zhu and reviewed by Kunlun Xiang and two anonymous referees.
Ambika, A. K., Wardlow, B., and Mishra, V.: Remotely sensed high resolution irrigated area mapping in India for 2000 to 2015, Scientific Data, 3, 160118, https://doi.org/10.1038/sdata.2016.118, 2016.
Bai, M., Zhou, S., and Tang, T.: A Reconstruction of Irrigated Cropland Extent in China from 2000 to 2019 Using the Synergy of Statistics and Satellite-Based Datasets, Land, 11, 1686, https://doi.org/10.3390/land11101686, 2022.
Bhattarai, N., Lobell, D. B., Balwinder, S., Fishman, R., Kustas, W. P., Pokhrel, Y., and Jain, M.: Warming temperatures exacerbate groundwater depletion rates in India, Science Advance, 9, eadi1401, https://doi.org/10.1126/sciadv.adi1401, 2023.
Breiman, L.: Random Forests, Machine Learning, 45, 5–32, https://doi.org/10.1023/A:1010933404324, 2001.
Chen, F., Zhao, H., Roberts, D., Van de Voorde, T., Batelaan, O., Fan, T., and Xu, W.: Mapping center pivot irrigation systems in global arid regions using instance segmentation and analyzing their spatial relationship with freshwater resources, Remote Sens. Environ., 297, 113760, https://doi.org/10.1016/j.rse.2023.113760, 2023.
Chen, X., Yu, L., Du, Z., Liu, Z., Qi, Y., Liu, T., and Gong, P.: Toward sustainable land use in China: A perspective on China's national land surveys, Land Use Policy, 123, 106428, https://doi.org/10.1016/j.landusepol.2022.106428, 2022.
Cheng, G., Li, X., Zhao, W., Xu, Z., Feng, Q., Xiao, S., and Xiao, H.: Integrated study of the water–ecosystem–economy in the Heihe River Basin, Natl. Sci. Rev., 1, 413-428, 2014.
Dari, J., Quintana-Seguí, P., José Escorihuela, M., Stefan, V., Brocca, L., and Morbidelli, R.: Detecting and mapping irrigated areas in a Mediterranean environment by using remote sensing soil moisture and a land surface model, J. Hydrol., 596, 126129, https://doi.org/10.1016/j.jhydrol.2021.126129, 2021.
Debeurs, K. and Townsend, P.: Estimating the effect of gypsy moth defoliation using MODIS, Remote Sens. Environ., 112, 3983–3990, https://doi.org/10.1016/j.rse.2008.07.008, 2008.
Deines, J. M., Kendall, A. D., and Hyndman, D. W.: Annual Irrigation Dynamics in the U.S. Northern High Plains Derived from Landsat Satellite Data, Geophys. Res. Lett., 44, 9350–9360, https://doi.org/10.1002/2017GL074071, 2017.
Deines, J. M., Kendall, A. D., Crowley, M. A., Rapp, J., Cardille, J. A., and Hyndman, D. W.: Mapping three decades of annual irrigation across the US High Plains Aquifer using Landsat and Google Earth Engine, Remote Sens. Environ., 233, 111400, https://doi.org/10.1016/j.rse.2019.111400, 2019.
Elwan, E., Le Page, M., Jarlan, L., Baghdadi, N., Brocca, L., Modanesi, S., Dari, J., Quintana Seguí, P., and Zribi, M.: Irrigation Mapping on Two Contrasted Climatic Contexts Using Sentinel-1 and Sentinel-2 Data, Water, 14, 804, https://doi.org/10.3390/w14050804, 2022.
Esmaeili, P., Vazifedoust, M., Rahmani, M., and Pakdel, H.: A simple rule-based algorithm in Google Earth Engine for operational discrimination of rice paddies in Sefidroud Irrigation Network, Arab. J. Geosci., 16, 649, https://doi.org/10.1007/s12517-023-11770-x, 2023.
Gao, B.-c.: NDWI – A normalized difference water index for remote sensing of vegetation liquid water from space, Remote Sens. Environ., 58, 257–266, https://doi.org/10.1016/S0034-4257(96)00067-3, 1996.
Gao, Q., Zribi, M., Escorihuela, M., Baghdadi, N., and Segui, P.: Irrigation Mapping Using Sentinel-1 Time Series at Field Scale, Remote Sensing, 10, 1495, https://doi.org/10.3390/rs10091495, 2018.
Gitelson, A. A.: Remote estimation of canopy chlorophyll content in crops, Geophys. Res. Lett., 32, L08403, https://doi.org/10.1029/2005GL022688, 2005.
Guo, Q. and Zhou, X.: Irrigated cropland expansion exacerbates the urban moist heat stress in northern India, Environ. Res. Lett., 17, 054013, https://doi.org/10.1088/1748-9326/ac64b6, 2022.
Hilker, T., Lyapustin, A. I., Tucker, C. J., Sellers, P. J., Hall, F. G., and Wang, Y.: Remote sensing of tropical ecosystems: Atmospheric correction and cloud masking matter, Remote Sens. Environ., 127, 370–384, https://doi.org/10.1016/j.rse.2012.08.035, 2012.
Huete, A. R., Liu, H. Q., Batchily, K., and van Leeuwen, W.: A comparison of vegetation indices over a global set of TM images for EOS-MODIS, Remote Sens. Environ., 59, 440–451, https://doi.org/10.1016/S0034-4257(96)00112-5, 1997.
International Commission on Irrigation and Drainage: World Irrigated Area-2018, 1–6, https://www.icid.org/world-irrigated-area.pdf (last access: 6 November 2024), 2018.
Kang, S. and Eltahir, E. A. B.: North China Plain threatened by deadly heatwaves due to climate change and irrigation, Nat. Commun., 9, 2894, https://doi.org/10.1038/s41467-018-05252-y, 2018.
Lacroix, P., Dehecq, A., and Taipe, E.: Irrigation-triggered landslides in a Peruvian desert caused by modern intensive farming, Nat. Geosci., 13, 56–60, https://doi.org/10.1038/s41561-019-0500-x, 2020.
Lamb, S. E., Haacker, E. M. K., and Smidt, S. J.: Influence of Irrigation Drivers Using Boosted Regression Trees: Kansas High Plains, Water Resour. Res., 57, e2020WR028867, https://doi.org/10.1029/2020WR028867, 2021.
Li, H. and Chen, Y.: Assessing potential land suitable for surface irrigation using groundwater data and multi-criteria evaluation in Xinjiang inland river basin, Comput. Electron. Agr., 168, 105079, https://doi.org/10.1016/j.compag.2019.105079, 2020.
Li, X., Zhang, Y., Ma, N., Zhang, X., Tian, J., Zhang, L., McVicar, T. R., Wang, E., and Xu, J.: Increased Grain Crop Production Intensifies the Water Crisis in Northern China, Earth's Future, 11, e2023EF003608, https://doi.org/10.1029/2023EF003608, 2023.
Liu, J., Kuang, W., Zhang, Z., Xu, X., Qin, Y., Ning, J., Zhou, W., Zhang, S., Li, R., Yan, C., Wu, S., Shi, X., Jiang, N., Yu, D., Pan, X., and Chi, W.: Spatiotemporal characteristics, patterns, and causes of land-use changes in China since the late 1980s, J. Geogr. Sci., 24, 195–210, https://doi.org/10.1007/s11442-014-1082-6, 2014.
Liu, Y., Li, Q., and Wu, W.: Analysis of feature slection for mapping irrigated cropland in northern China, Chinese Journal of Agricultural Resources and Regional Planning, 42, 27–35, 2022 (In Chinese).
Longo-Minnolo, G., Consoli, S., Vanella, D., Ramírez-Cuesta, J. M., Greimeister-Pfeil, I., Neuwirth, M., and Vuolo, F.: A stand-alone remote sensing approach based on the use of the optical trapezoid model for detecting the irrigated areas, Agr. Water Manage., 274, 107975, https://doi.org/10.1016/j.agwat.2022.107975, 2022.
Lu, Y., Song, W., Lü, J., Chen, M., Su, Z., Zhang, X., and Li, H.: A pixel-based spectral matching method for mapping high-resolution irrigated areas using EVI time series, Remote Sens. Lett., 12, 169–178, https://doi.org/10.1080/2150704X.2020.1837987, 2021.
Massari, C., Modanesi, S., Dari, J., Gruber, A., De Lannoy, G. J. M., Girotto, M., Quintana-Seguí, P., Le Page, M., Jarlan, L., Zribi, M., Ouaadi, N., Vreugdenhil, M., Zappa, L., Dorigo, W., Wagner, W., Brombacher, J., Pelgrum, H., Jaquot, P., Freeman, V., Volden, E., Fernandez Prieto, D., Tarpanelli, A., Barbetta, S., and Brocca, L.: A Review of Irrigation Information Retrievals from Space and Their Utility for Users, Remote Sensing, 13, 4112, https://doi.org/10.3390/rs13204112, 2021.
McDermid, S., Nocco, M., Lawston-Parker, P., Keune, J., Pokhrel, Y., Jain, M., Jägermeyr, J., Brocca, L., Massari, C., Jones, A. D., Vahmani, P., Thiery, W., Yao, Y., Bell, A., Chen, L., Dorigo, W., Hanasaki, N., Jasechko, S., Lo, M.-H., Mahmood, R., Mishra, V., Mueller, N. D., Niyogi, D., Rabin, S. S., Sloat, L., Wada, Y., Zappa, L., Chen, F., Cook, B. I., Kim, H., Lombardozzi, D., Polcher, J., Ryu, D., Santanello, J., Satoh, Y., Seneviratne, S., Singh, D., and Yokohata, T.: Irrigation in the Earth system, Nature Reviews Earth & Environment, 4, 435–453, https://doi.org/10.1038/s43017-023-00438-5, 2023.
McDermid, S. S., Mahmood, R., Hayes, M. J., Bell, J. E., and Lieberman, Z.: Minimizing trade-offs for sustainable irrigation, Nat. Geosci., 14, 706–709, https://doi.org/10.1038/s41561-021-00830-0, 2021.
McFeeters, S. K.: The use of the Normalized Difference Water Index (NDWI) in the delineation of open water features, Int. J. Remote Sens., 17, 1425–1432, https://doi.org/10.1080/01431169608948714, 1996.
Mehta, P., Siebert, S., Kummu, M., Deng, Q., Ali, T., Marston, L., Xie, W., and Davis, K. F.: Half of twenty-first century global irrigation expansion has been in water-stressed regions, Nature Water, 2, 254–261, https://doi.org/10.1038/s44221-024-00206-9, 2024.
Meier, J., Zabel, F., and Mauser, W.: A global approach to estimate irrigated areas – a comparison between different data and statistics, Hydrol. Earth Syst. Sci., 22, 1119–1133, https://doi.org/10.5194/hess-22-1119-2018, 2018.
Mishra, V., Ambika, A. K., Asoka, A., Aadhar, S., Buzan, J., Kumar, R., and Huber, M.: Moist heat stress extremes in India enhanced by irrigation, Nat. Geosci., 13, 722–728, https://doi.org/10.1038/s41561-020-00650-8, 2020.
Mpakairi, K. S., Dube, T., Sibanda, M., and Mutanga, O.: Fine-scale characterization of irrigated and rainfed croplands at national scale using multi-source data, random forest, and deep learning algorithms, ISPRS J. Photogramm., 204, 117–130, https://doi.org/10.1016/j.isprsjprs.2023.09.006, 2023.
Noori, R., Maghrebi, M., Mirchi, A., Tang, Q., Bhattarai, R., Sadegh, M., Noury, M., Torabi Haghighi, A., Kløve, B., and Madani, K.: Anthropogenic depletion of Iran's aquifers, P. Natl. Acad. Sci. USA, 118, e2024221118, https://doi.org/10.1073/pnas.2024221118, 2021.
Ozdogan, M. and Gutman, G.: A new methodology to map irrigated areas using multi-temporal MODIS and ancillary data: An application example in the continental US, Remote Sens. Environ., 112, 3520–3537, https://doi.org/10.1016/j.rse.2008.04.010, 2008.
Ozdogan, M., Yang, Y., Allez, G., and Cervantes, C.: Remote Sensing of Irrigated Agriculture: Opportunities and Challenges, Remote Sensing, 2, 2274–2304, https://doi.org/10.3390/rs2092274, 2010.
Pervez, M. S. and Brown, J. F.: Mapping Irrigated Lands at 250 m Scale by Merging MODIS Data and National Agricultural Statistics, Remote Sensing, 2, 2388–2412, https://doi.org/10.3390/rs2102388, 2010.
Potapov, P., Turubanova, S., Hansen, M. C., Tyukavina, A., Zalles, V., Khan, A., Song, X.-P., Pickens, A., Shen, Q., and Cortez, J.: Global maps of cropland extent and change show accelerated cropland expansion in the twenty-first century, Nature Food, 3, 19–28, https://doi.org/10.1038/s43016-021-00429-z, 2021.
Priestley, C. H. B. and Taylor, R. J.: On the Assessment of Surface Heat Flux and Evaporation Using Large-Scale Parameters, Mon. Weather Rev., 100, 81–92, https://doi.org/10.1175/1520-0493(1972)100<0081:OTAOSH>2.3.CO;2, 1972.
Pun, M., Mutiibwa, D., and Li, R.: Land Use Classification: A Surface Energy Balance and Vegetation Index Application to Map and Monitor Irrigated Lands, Remote Sensing, 9, 1256, https://doi.org/10.3390/rs9121256, 2017.
Puy, A., Borgonovo, E., Lo Piano, S., Levin, S. A., and Saltelli, A.: Irrigated areas drive irrigation water withdrawals, Nat. Commun., 12, 4525, https://doi.org/10.1038/s41467-021-24508-8, 2021.
Qin, Y., Hong, C., Zhao, H., Siebert, S., Abatzoglou, J. T., Huning, L. S., Sloat, L. L., Park, S., Li, S., Munroe, D. K., Zhu, T., Davis, S. J., and Mueller, N. D.: Snowmelt risk telecouplings for irrigated agriculture, Nat. Clim. Change, 12, 1007–1015, https://doi.org/10.1038/s41558-022-01509-z, 2022.
Rosa, L., Chiarelli, D. D., Rulli, M. C., Dell Angelo, J., and D Odorico, P.: Global agricultural economic water scarcity, Science Advances, 6, eaaz6031, https://doi.org/10.1126/sciadv.aaz6031, 2020a.
Rosa, L., Chiarelli, D. D., Sangiorgio, M., Beltran-Peña, A. A., Rulli, M. C., D Odorico, P., and Fung, I.: Potential for sustainable irrigation expansion in a 3 °C warmer climate, P. Natl. Acad. Sci. USA, 117, 29526–29534, https://doi.org/10.1073/pnas.2017796117, 2020b.
Rouse, J. W., Haas, R. H., Schell, J. A., and Deering, D. W.: Monitoring vegetation systems in the Great Plains with ERTS, in: Third Earth Resources Technology Satellite-l Symposium. Volume I: Technical Presentations Section A. Paper A-20, Goddard Space Flight Center: Washington, D.C, 10–14 December 1973, National Aeronautics and Space Administration: Washington, D.C., 309–317, 1974.
Salmon, J. M., Friedl, M. A., Frolking, S., Wisser, D., and Douglas, E. M.: Global rain-fed, irrigated, and paddy croplands: A new high resolution map derived from remote sensing, crop inventories and climate data, Int. J. Appl. Earth Obs., 38, 321–334, https://doi.org/10.1016/j.jag.2015.01.014, 2015.
Schepaschenko, D., See, L., Lesiv, M., McCallum, I., Fritz, S., Salk, C., Moltchanova, E., Perger, C., Shchepashchenko, M., Shvidenko, A., Kovalevskyi, S., Gilitukha, D., Albrecht, F., Kraxner, F., Bun, A., Maksyutov, S., Sokolov, A., Dürauer, M., Obersteiner, M., Karminov, V., and Ontikov, P.: Development of a global hybrid forest mask through the synergy of remote sensing, crowdsourcing and FAO statistics, Remote Sens. Environ., 162, 208–220, https://doi.org/10.1016/j.rse.2015.02.011, 2015.
Shahriar Pervez, M., Budde, M., and Rowland, J.: Mapping irrigated areas in Afghanistan over the past decade using MODIS NDVI, Remote Sens. Environ., 149, 155–165, https://doi.org/10.1016/j.rse.2014.04.008, 2014.
Siddiqui, S., Cai, X., and Chandrasekharan, K.: Irrigated Area Map Asia and Africa. International Water Management Institute, https://waterdata.iwmi.org/applications/irri_area/ (last access: 6 November 2024), 2016.
Teluguntla, P., Thenkabail, P. S., Oliphant, A., Xiong, J., Gumma, M. K., Congalton, R. G., Yadav, K., and Huete, A.: A 30-m landsat-derived cropland extent product of Australia and China using random forest machine learning algorithm on Google Earth Engine cloud computing platform, ISPRS J. Photogramm., 144, 325–340, https://doi.org/10.1016/j.isprsjprs.2018.07.017, 2018.
Thenkabail, P., Knox, J., Ozdogan, M., Gumma, M., Congalton, R., Wu, Z., Milesi, C., Finkral, A., Marshall, M., Mariotto, I., You, S., Giri, C., and Nagler, P.: NASA Making Earth System Data Records for Use in Research Environments (MEaSUREs) Global Food Security Support Analysis Data (GFSAD) Crop Dominance 2010 Global 1 km V001, NASA EOSDIS Land Processes Distributed Active Archive Center [data set], https://doi.org/10.5067/MEaSUREs/GFSAD/GFSAD1KCD.001, 2016.
Thenkabail, P. S., Biradar, C. M., Noojipady, P., Dheeravath, V., Li, Y., Velpuri, M., Gumma, M., Gangalakunta, O. R. P., Turral, H., Cai, X., Vithanage, J., Schull, M. A., and Dutta, R.: Global irrigated area map (GIAM), derived from remote sensing, for the end of the last millennium, Int. J. Remote Sens., 30, 3679–3733, https://doi.org/10.1080/01431160802698919, 2009.
Thiery, W., Visser, A. J., Fischer, E. M., Hauser, M., Hirsch, A. L., Lawrence, D. M., Lejeune, Q., Davin, E. L., and Seneviratne, S. I.: Warming of hot extremes alleviated by expanding irrigation, Nat. Commun., 11, 290, https://doi.org/10.1038/s41467-019-14075-4, 2020.
Thorslund, J., Bierkens, M. F. P., Oude Essink, G. H. P., Sutanudjaja, E. H., and van Vliet, M. T. H.: Common irrigation drivers of freshwater salinisation in river basins worldwide, Nat. Commun., 12, 4232, https://doi.org/10.1038/s41467-021-24281-8, 2021.
Tian, X., Dong, J., Chen, X., Zhou, J., Gao, M., Wei, L., Kang, X., Zhao, D., Zhang, H., Crow, W. T., Huang, R., Shao, W., and Zhou, H.: County-Level Evaluation of Large-Scale Gridded Data Sets of Irrigated Area Over China, J. Geophys. Res.-Atmos., 129, e2023JD040333, https://doi.org/10.1029/2023JD040333, 2024.
UNESCO World Water Assessment Programme: The United Nations world water development report 2019: leaving no one behind. Paris, UNESCO, https://unesdoc.unesco.org/ark:/48223/pf0000367306 (last access: 6 November 2024), 2019.
Uniyal, B. and Dietrich, J.: Simulation of Irrigation Demand and Control in Catchments ? A Review of Methods and Case Studies, Water Resour. Res., 57, e2020WR029263, https://doi.org/10.1029/2020WR029263, 2021.
Wang, C., Chen, J., Gu, L., Wu, G., Tong, S., Xiong, L., and Xu, C.-Y.: A pathway analysis method for quantifying the contributions of precipitation and potential evapotranspiration anomalies to soil moisture drought, J. Hydrol., 621, 129570, https://doi.org/10.1016/j.jhydrol.2023.129570, 2023.
Worqlul, A. W., Collick, A. S., Rossiter, D. G., Langan, S., and Steenhuis, T. S.: Assessment of surface water irrigation potential in the Ethiopian highlands: The Lake Tana Basin, Catena, 129, 76–85, https://doi.org/10.1016/j.catena.2015.02.020, 2015.
Worqlul, A. W., Jeong, J., Dile, Y. T., Osorio, J., Schmitter, P., Gerik, T., Srinivasan, R., and Clark, N.: Assessing potential land suitable for surface irrigation using groundwater in Ethiopia, Appl. Geogr., 85, 1–13, https://doi.org/10.1016/j.apgeog.2017.05.010, 2017.
Wu, B., Tian, F., Zhang, M., Piao, S., Zeng, H., Zhu, W., Liu, J., Elnashar, A., and Lu, Y.: Quantifying global agricultural water appropriation with data derived from earth observations, J. Clean. Prod., 358, 131891, https://doi.org/10.1016/j.jclepro.2022.131891, 2022.
Xiang, K., Yuan, W., Wang, L., and Deng, Y.: An LSWI-Based Method for Mapping Irrigated Areas in China Using Moderate-Resolution Satellite Data, Remote Sensing, 12, 4181, https://doi.org/10.3390/rs12244181, 2020.
Xie, Y. and Lark, T. J.: Mapping annual irrigation from Landsat imagery and environmental variables across the conterminous United States, Remote Sens. Environ., 260, 112445, https://doi.org/10.1016/j.rse.2021.112445, 2021.
Xie, Y., Lark, T. J., Brown, J. F., and Gibbs, H. K.: Mapping irrigated cropland extent across the conterminous United States at 30 m resolution using a semi-automatic training approach on Google Earth Engine, ISPRS J. Photogramm., 155, 136–149, https://doi.org/10.1016/j.isprsjprs.2019.07.005, 2019.
Xie, Y., Gibbs, H. K., and Lark, T. J.: Landsat-based Irrigation Dataset (LANID): 30 m resolution maps of irrigation distribution, frequency, and change for the US, 1997–2017, Earth Syst. Sci. Data, 13, 5689–5710, https://doi.org/10.5194/essd-13-5689-2021, 2021.
Xiong, J., Thenkabail, P. S., Gumma, M. K., Teluguntla, P., Poehnelt, J., Congalton, R. G., Yadav, K., and Thau, D.: Automated cropland mapping of continental Africa using Google Earth Engine cloud computing, ISPRS J. Photogramm., 126, 225–244, https://doi.org/10.1016/j.isprsjprs.2017.01.019, 2017.
Xu, X., Liu, J., Zhang, S., Li, R., Yan, C., and Wu, S.: Remote sensing-based monitoring dataset of land use and cover change over multiple periods in China (CNLUCC), Resource and Environmental Science Data Center [data set], https://doi.org/10.12078/2018070201, 2018 (in Chinese).
Yang, Y., Jin, Z., Mueller, N. D., Driscoll, A. W., Hernandez, R. R., Grodsky, S. M., Sloat, L. L., Chester, M. V., Zhu, Y.-G., and Lobell, D. B.: Sustainable irrigation and climate feedbacks, Nature Food, 4, 654–663, https://doi.org/10.1038/s43016-023-00821-x, 2023.
Yao, Z., Cui, Y., Geng, X., Chen, X., and Li, S.: Mapping Irrigated Area at Field Scale Based on the OPtical TRApezoid Model (OPTRAM) Using Landsat Images and Google Earth Engine, IEEE T. Geosci. Remote, 60, 1–11, https://doi.org/10.1109/TGRS.2022.3148274, 2022.
Yu, Z., Jin, X., Miao, L., and Yang, X.: A historical reconstruction of cropland in China from 1900 to 2016, Earth Syst. Sci. Data, 13, 3203–3218, https://doi.org/10.5194/essd-13-3203-2021, 2021.
Zaveri, E. and B. Lobell, D.: The role of irrigation in changing wheat yields and heat sensitivity in India, Nat. Commun., 10, 4144, https://doi.org/10.1038/s41467-019-12183-9, 2019.
Zeng, R. and Ren, W.: The spatiotemporal trajectory of US agricultural irrigation withdrawal during 1981–2015, Environ. Res. Lett., 17, 104027, https://doi.org/10.1088/1748-9326/ac91e2, 2022.
Zhang, C., Dong, J., and Ge, Q.: IrriMap_CN: Annual irrigation maps across China in 2000–2019 based on satellite observations, environmental variables, and machine learning, Remote Sens. Environ., 280, 113184, https://doi.org/10.1016/j.rse.2022.113184, 2022a.
Zhang, C., Dong, J., and Ge, Q.: Mapping 20 years of irrigated croplands in China using MODIS and statistics and existing irrigation products, Scientific Data, 9, 407, https://doi.org/10.1038/s41597-022-01522-z, 2022b.
Zhang, C., Dong, J., Xie, Y., Zhang, X., and Ge, Q.: Mapping irrigated croplands in China using a synergetic training sample generating method, machine learning classifier, and Google Earth Engine, Int. J. Appl. Earth Obs., 112, 102888, https://doi.org/10.1016/j.jag.2022.102888, 2022c.
Zhang, F., Zhang, T., Li, C., and Li, Z.: Cultivated land in China, China Agricultural University Press, ISBN 9787565525551, 2021 (in Chinese).
Zhang, L., Zhang, K., Zhu, X., Chen, H., and Wang, W.: Integrating remote sensing, irrigation suitability and statistical data for irrigated cropland mapping over mainland China, J. Hydrol., 613, 128413, https://doi.org/10.1016/j.jhydrol.2022.128413, 2022.
Zhang, L., Xie, Y., Zhu, X., Ma, Q., and Brocca, L.: CIrrMap250: Annual maps of China's irrigated cropland from 2000 to 2020, Figshare [data set], https://doi.org/10.6084/m9.figshare.24814293.v2, 2023a.
Zhang, L., Ma, Q., Zhao, Y., Chen, H., Hu, Y., and Ma, H.: China's strictest water policy: Reversing water use trends and alleviating water stress, J. Environ. Manage., 345, 118867, https://doi.org/10.1016/j.jenvman.2023.118867, 2023b.
Zhang, L., Wang, W., Ma, Q., Hu, Y., and Zhao, Y.: CCropLand30: High-resolution hybrid cropland maps of China created through the synergy of state-of-the-art remote sensing products and the latest national land survey, Comput. Electron. Agr., 218, 108672, https://doi.org/10.1016/j.compag.2024.108672, 2024.
Zhu, P. and Burney, J.: Untangling irrigation effects on maize water and heat stress alleviation using satellite data, Hydrol. Earth Syst. Sci., 26, 827–840, https://doi.org/10.5194/hess-26-827-2022, 2022.
Zhu, P., Burney, J., Chang, J., Jin, Z., Mueller, N. D., Xin, Q., Xu, J., Yu, L., Makowski, D., and Ciais, P.: Warming reduces global agricultural production by decreasing cropping frequency and yields, Nat. Clim. Change, 12, 1016–1023, https://doi.org/10.1038/s41558-022-01492-5, 2022.
Zhu, X., Zhu, W., Zhang, J., and Pan, Y.: Mapping Irrigated Areas in China From Remote Sensing and Statistical Data, IEEE J. Sel. Top. Appl., 7, 4490–4504, https://doi.org/10.1109/JSTARS.2013.2296899, 2014.
Zuo, W., Mao, J., Lu, J., Zheng, Z., Han, Q., Xue, R., Tian, Y., Zhu, Y., Cao, W., and Zhang, X.: Mapping Irrigated Areas Based on Remotely Sensed Crop Phenology and Soil Moisture, Agronomy, 13, 1556, https://doi.org/10.3390/agronomy13061556, 2023.