the Creative Commons Attribution 4.0 License.
the Creative Commons Attribution 4.0 License.
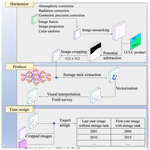
Retrieval of dominant methane (CH4) emission sources, the first high-resolution (1–2 m) dataset of storage tanks of China in 2000–2021
Fang Chen
Lei Wang
Yu Wang
Haiying Zhang
Ning Wang
Pengfei Ma
Bo Yu
Methane (CH4) is a significant greenhouse gas in exacerbating climate change. Approximately 25 % of CH4 is emitted from storage tanks. It is crucial to spatially explore the CH4 emission patterns from storage tanks for efficient strategy proposals to mitigate climate change. However, due to the lack of publicly accessible storage tank locations and distributions, it is difficult to ascertain the CH4 emission spatial pattern over a large-scale area. To address this problem, we generated a storage tank dataset (STD) by implementing a deep learning model with manual refinement based on 4403 high-spatial-resolution images (1–2 m) from the Gaofen-1, Gaofen-2, Gaofen-6, and Ziyuan-3 satellites over city regions in China with officially reported numerous storage tanks in 2021. STD is the first storage tank dataset for over 92 typical city regions in China. The dataset can be accessed at https://doi.org/10.5281/zenodo.10514151 (Chen et al., 2024). It provides a detailed georeferenced inventory of 14 461 storage tanks wherein each storage tank is validated and assigned the construction year (2000–2021) by visual interpretation of the collected high-spatial-resolution images, historical high-spatial-resolution images of Google Earth, and field survey. The inventory comprises storage tanks with various distribution patterns in different city regions. Spatial consistency analysis with the CH4 emission product shows good agreement with storage tank distributions. The intensive construction of storage tanks significantly induces CH4 emissions from 2005 to 2020, underscoring the need for more robust measures to curb CH4 release and aid in climate change mitigation efforts. Our proposed dataset, STD, will foster the accurate estimation of CH4 released from storage tanks for CH4 control and reduction and ensure more efficient treatment strategies are proposed to better understand the impact of storage tanks on the environment, ecology, and human settlements.
- Article
(9846 KB) - Full-text XML
- BibTeX
- EndNote
The Industrial Revolution witnessed a continuous increase in greenhouse gases, resulting in global climate warming (Zhang et al., 2021). Methane (CH4) is the second-most-dominant anthropogenic greenhouse gas affecting global climate warming, with a contribution of 20 % (Kirschke et al., 2013), after carbon dioxide (CO2). Meanwhile, CH4 is more effective in trapping heat, with 85 times more climate warming potency than CO2 for the past decade or two (Stocker, 2014). The atmospheric lifetime of CH4 is approximately 10 years, which is shorter than most other greenhouse gases; thus, reducing CH4 emissions is more cost-effective for lowering the climate warming potential impact (Lin et al., 2021; Montzka et al., 2011). CH4 is emitted mainly from energy-related activities and petrochemical processes (Ding et al., 2017; Fan et al., 2023). Storage tanks, defined as large containers of crude oil or other petroleum, and industrial materials, such as alcohols, gases, or liquids, are among the most significant sources of CH4 emitted (Im et al., 2022; Johnson et al., 2022). Without an adequate control or management strategy, large amounts of CH4 will escape into the atmosphere (Im et al., 2022). From a greenhouse gas control standpoint, it is of great interest to examine the distribution patterns of the storage tanks. With a detailed and comprehensive storage tank inventory, we can effectively estimate the spatial pattern of CH4 emissions and reduce the risk of CH4 emission by installing recovery units (Johnson et al., 2022) to promote sustainable development goals. However, it is challenging to access detailed distribution records for storage tanks from the public records in China.
Given the advances in remotely sensed technology (Chen et al., 2023; Yu et al., 2023a, b), the ready availability of high-spatial-resolution remote sensing images via the Gaofen series satellites and the Ziyuan-3 satellite provides means to extract remote sensing data for large-scale storage tanks. Numerous studies on the use of automatic methods to extract storage tanks from high-spatial-resolution remote sensing images have been performed (Fan et al., 2023; Wu et al., 2022; Yu et al., 2021), including the Hough transform (Yuen et al., 1990), image saliency enhancement (Zhang and Liu, 2019), support vector machines (Xia et al., 2018), and Res2-Unet+ deep convolutional networks (Yu et al., 2021). The focus of the works above is primarily spatially limited, and the images collected for extraction are mostly pre-subtracted from regions known to contain storage tanks. The transferability and the practical applicability of the proposed methods remain to be clarified. To our knowledge, there are limited publicly available datasets on storage tanks. Northeast Petroleum University–Oil Well Object Detection Version 1.0 (NEPU–OWOD V1.0) covers 1192 oil storage tanks within Daqing (Wang et al., 2021). This dataset covers the boundary boxes for each storage tank but lacks details on the storage tank inventory. Another two datasets, the Oil and Gas Tank Dataset (Rabbi et al., 2020) and the Oil Storage Tank Dataset (Airbusgeo, 2019), acquired via the Kaggle platform, have been released without georeferenced information and lack detail regarding the contour shapes. The datasets are generally proposed to improve the performance of algorithms in storage tank extraction. Currently, most studies are concentrated on algorithm development for storage tank extraction rather than exploring the spatial distribution of storage tanks in large-scale areas and the impact of storage tank construction on CH4 emission in different areas over the years. The spatial distributions of storage tanks in China have not yet been investigated and recorded. The lack of storage tank datasets makes it impossible to estimate the impact of anthropogenic energy-related activities on CH4 emission and air pollution.
To foster the control and reduction in CH4 emissions to mitigate climate change and provide researchers with free access to detailed and georeferenced storage tank inventory to monitor the corresponding potential impact on the atmosphere and residential environment over typical city regions in China, we compiled a storage tank inventory based on high-spatial-resolution images of the Gaofen-1, Gaofen-2, Gaofen-6, and Ziyuan-3 satellites for city regions with intensive storage tanks over China. The city regions are listed by the Ministry of Ecology and Environment of China, with intensive storage tanks and prominent fugitive emissions and inadequate monitoring and control of treatment measures (Wang et al., 2022). There are 92 city regions in total, mainly located in mid-eastern China. Given that large storage tanks may emit significant levels of CH4, storage tanks with a footprint of ≥ 500 m2 were selected as the main target to control the reduction in CH4 in the proposed inventory. To this end, we generated a complete inventory of storage tanks with a footprint of ≥ 500 m2 for the 92 city regions in China with intensive storage tanks, which were subject to the implementation of CH4 reduction measures.
In this study, firstly, we collected high-spatial-resolution images to cover the entire study area. We pre-processed them to synchronize the pixel intensities of ground objects in different images from different imaging sensors and study areas. Secondly, we proposed a semantic segmentation framework to construct the storage tank extraction model based on the training samples of Ningbo, Tangshan, and Dongying city regions. Thirdly, the constructed model is applied to extract storage tanks in all the other city regions to generate extraction results. Fourthly, the extracted storage tank result images are converted to vectors, revised, and assigned the corresponding construction year by visual interpretation with reference to the historical high-spatial-resolution images from Google Earth, high-spatial-resolution images collected, and field survey. Fifthly, we explored the spatial distribution pattern of storage tanks in typical city regions in China. Sixthly, we further explored the consistency of storage tank spatial patterns and CH4 emission in the atmosphere and the impact of storage tank construction on time series CH4 emission change from 2005 to 2020. Finally, the uncertainties, limitations, and implications of our proposed dataset, STD, are discussed for studying climate change and air pollution. This new database represents the first inventory to provide a detailed distribution of the locations, the boundaries of the storage tanks, and the corresponding construction year of each storage tank. The inventory documents the spatial and temporal distribution of storage tanks with different footprints, and, hopefully, this work will facilitate the development of environment-friendly regulatory proposals for more effective CH4 emission control and energy resource management.
Storage tank extraction from high-spatial-resolution images has been of interest for many years for its significant role in storage and greenhouse gas emission. Generally, the methods for extracting storage tanks are grouped into three categories. Circle detection by Hough transformation (O'Duda, 1972) and template matching (Hou et al., 2019); machine learning model construction by morphological, spectral, and textual feature engineering (Xia et al., 2018); and deep learning model construction by continuous convolutional operations (Fan et al., 2023). Deep learning methods have been extensively used to map storage tanks due to their strong feature learning capability and higher model transferability.
Semantic segmentation is a widely employed deep learning framework in object extraction by assigning each pixel in the image a semantic label (Chen et al., 2022; Yu et al., 2022b). Fully convolutional network (FCN) (Long et al., 2015) is a basic framework of semantic segmentation with three components: the backbone feature learning, the convolutional feature learning with skip architecture, and an upsampling layer to resample the learned feature map to the size of the input image. Based on FCN, numerous frameworks have been inspired, such as SegNet (Badrinarayanan et al., 2017), PSPNet (Zhao et al., 2017), Unet (Ronneberger et al., 2015), DeepLabv2 (Chen et al., 2017b), and DeepLabv3 (Chen et al., 2017a). Unet has a widespread use for its easy implementation and high efficiency. The proposal of Res2-Unet+ framework for storage tank extraction (Yu et al., 2021; Zalpour et al., 2020) integrates the Res2Net module (Gao et al., 2019) to Unet. The Res2Net module is proposed to learn multi-scale features by learning at a more granular level. It has shown strong applicability in extracting storage tanks from images of different imaging sensors (Yu et al., 2022a). However, many storage tank pixels are still omitted due to their similar spectral characteristics with neighboring ground objects. To resist the shortage, we have proposed a new semantic segmentation framework based on Res2-Unet+ and enlarged the variability in storage tank training samples to build a more robust and accurate extraction model.
3.1 Study area
The study area covers 92 typical city regions (as shown in Fig. 1) with intensive storage tanks over China, assigned by the Ministry of Ecology and Environment of China (Wang et al., 2022). The typical city regions lack detailed monitoring and control of prominent fugitive emissions, whose effective measurements in CH4 reduction emission are urgently demanded and required. The 92 city regions tend to be located in mid-eastern China. Many of the city regions are coastal cities. Synthesized with a digital elevation model (DEM) from the product of the Shuttle Radar Topography Mission (SRTM) (Yang et al., 2011), we can recognize that most city regions are plains. As is acknowledged, plains are densely populated. The large population numbers will bring more frequent human activities, triggering more pollutant and greenhouse gas emissions. The lack of efficient measurements in CH4 emissions will result in a more direct impact on the populations in the residential area. Therefore, exploring the spatial distribution pattern of storage tanks relative to CH4 emission is significant in order to seek more effective solutions for CH4 reduction.
3.2 High-spatial-resolution images
The high-spatial-resolution images used for extracting storage tanks in the 92 city regions were collected from four satellites: the Gaofen-1, Gaofen-2, Gaofen-6, and Ziyuan-3 satellites in 2021. The images collected are of between June and August with the least cloud coverage (< 10 %) from the four satellites, when different ground objects have more pronounced spectral differences, which makes it easier to distinguish storage tanks from background objects. As listed in Table 1, the images for the Gaofen-1, Gaofen-6, and Ziyuan-3 satellites have a spatial resolution of 2 m, and those for the Gaofen-2 have a spatial resolution of 1 m after fusion of the multispectral image and the panchromatic image. Referring to Table 1, we can recognize that 4403 images were collected. The places covered with multiple images are manually screened to choose one image with the best imaging quality and least cloud proportion. Based on the screened high-spatial-resolution images, multiple image pre-processing steps are performed to synchronize the ground objects in different images of different sensors for different study areas, comprising atmospheric correction, radiation correction, geometric precision correction, image fusion, image projection, uniform color processing, and image mosaicking.
3.3 Land use land cover product
Given that storage tanks are constructed mainly in urban area due to the high expense of transportation of pipelines, a 10 m land use land cover (LULC) product of ESRI Land Cover for 2021 (Karra et al., 2021) is used for subtracting the study area to minimize the impact of complex background objects in the high-spatial-resolution images following the workflow as shown in Fig. 2. The land use product of the ESRI Land Cover is generated based on the Sentinel-2 images from the European Space Agency (ESA), with an overall accuracy of 75 % (Venter et al., 2022), which has been updated every year since 2017. It comprises nine ground object categories: water, trees, flooded vegetation, bare ground, crops, snow/ice, clouds, rangeland, and built area. Since storage tanks are mostly constructed in urban areas, the categories of built area and bare ground are recognized as potential areas for constructing storage tanks. Consequently, the corresponding ground object category products of built area and bare ground are subtracted from the LULC 2021 product and used to mask the high-spatial-resolution images of the 92 city regions, as demonstrated in Fig. 2. The masked high-spatial-resolution images of the 92 city regions are further used for storage tank extraction.
3.4 CH4 product image
As storage tanks are a dominant source of CH4 emission, we have collected CH4 emission products to explore the spatial consistency of CH4 with the density of storage tanks and the impact of storage tank construction over time on CH4 emission. There have been many CH4 emission product images proposed, including the Community Emissions Data System (CEDS) (Hoesly et al., 2018), the product from Peking University (Peng et al., 2016), the Emissions Database for Global Atmospheric Research (EDGAR) (Crippa et al., 2019), the Regional Emission Inventory in Asia (REAS) (Kurokawa et al., 2013), and the Greenhouse Gas and Air Pollution Interactions and Synergies (GAINS) (Amann et al., 2011). Since our collected high-spatial-resolution remote sensing images were taken in the year 2021, the spatial consistency and the impact of storage tank construction on CH4 emission are explored using the CH4 emission product of GAINS, which offers a comprehensive series of data accessible to the public (Lin et al., 2021). The dataset of GAINS was selected over the other four products because the four products lacked continuous updates with limited temporal coverage until 2015.
We adopted the estimated CH4 emission from energetic activities product of the ECLIPSE V6b Baseline scenario from GAINS. It is a global annual product with a spatial resolution of 0.5° and a temporal coverage of 1990–2050 at an interval of 5 years. For the estimated CH4 emission from GAINS in the years 1990–2018, the product is generated from statistics of the International Energy Agency (IEA), and the years 2019–2050 are from the outlook of the IEA World Energy Outlook (IEA, 2018). To synchronize with the temporal scope of storage tank construction from 2000 to 2021, the CH4 emission products of 2005, 2010, 2015, and 2020 are collected.
As demonstrated in Fig. 3, the emission of CH4 in 2020 varies remarkably in different areas. There are many clusters of CH4 emission in the study area, with the highest being 5160.62 Tg CH4 yr−1. CH4 in the atmosphere of city regions located in southeastern China is generally higher than that of city regions in northwestern China in 2020.
As depicted in Fig. 4, the workflow of generating a storage tank dataset consists of three sections: harmonizing the pixel intensities of different ground objects across high-spatial-resolution images captured by different sensors in different study areas; producing a storage tank dataset by constructing a storage tank extraction model based on the harmonized high-spatial-resolution images; and assigning the construction year of each storage tank by multiple experts through visual interpretation of the historical high-spatial-resolution images from Google Earth, high-spatial-resolution images collected, and filed survey.
4.1 Image harmonizing
Pixel intensities for ground objects are standardized to ensure consistency across the high-spatial-resolution images collected. This harmonization process mitigates the effects of atmospheric variations and discrepancies between imaging sensors captured at different times. The standardization includes atmospheric correction, radiometric calibration, geometric alignment, image fusion, reprojection, and color normalization. In terms of atmospheric correction, the widely used radiation transfer model of the second simulation of the satellite signal in the solar spectrum (6S) (Vermote et al., 1997) is adopted to determine the atmospheric correction coefficient and eliminate the absorption and scattering impact of atmospheric molecules and aerosols for all the collected high-spatial-resolution images. The strategy of local histogram matching (Shen, 2007) is used to correct radiation differences in the same ground object category in different high-spatial-resolution images. To improve the geometric precision of the high-spatial-resolution images collected, we automatically generated 1000 ground control points by a widely used key point detector of scale-invariant feature transform (SIFT) for each city. We calculated the parameters for affine transformation with reference to the world imagery of the Environmental Systems Research Institute (ESRI) (Hou et al., 2021). Pixel-wise image fusion is conducted on images collected from each high-spatial-resolution satellite since they consist of multispectral images with a coarser spatial resolution than the panchromatic image, as demonstrated in Table 1. To optimize the utilization of the gathered images, we leveraged the wavelet transform (Sahu and Sahu, 2014) for the automatic fusion of multispectral and panchromatic images. To address discrepancies in the projections of the varied high-resolution images we collected, we standardized all the images to the Universal Transverse Mercator (UTM) projection using bilinear interpolation for consistency. To maintain visual consistency across images from different sensors or regions, it is crucial to standardize the color representation of identical ground objects. In this study, we implemented a nonlinear stretching technique to modify pixel intensity distribution. This was accomplished by constructing a color lookup table (Majumder et al., 2010) to ensure uniformity in spectral intensities across the various images.
The harmonized high-spatial-resolution images were further mosaicked to large image patches to integrate overlapping areas from adjacent high-resolution images, ensuring comprehensive coverage and continuity of the observed regions. Referring to the LULC product of the ESRI Land Cover product in 2021, the mosaicked image patches were subtracted with the ground object category of built area and bare ground, both identified as potential areas with storage tank constructions. Finally, for storage tank extraction, the subtracted images were cropped to a size of 512×512 pixels, a size compatible with the computational limits of our GPU hardware.
4.2 Production of storage tank dataset
4.2.1 Proposed framework for storage tank extraction
Stemming from the recently developed semantic segmentation framework for storage tank extraction, Res2-Unet+ (Yu et al., 2021), we proposed a new network structure, Res2-UnetA, to build a storage tank extraction model. As shown in Fig. 5a, our proposed framework integrates the Res2Net module (Fig. 5b) and channel–spatial attention module (Fig. 5c) to enhance the features significant for multi-scale storage tank extraction. During the process of feature map down-scaling, the Res2Net module can learn the multi-scale features from multiple sub-networks and concatenate the multi-scale features to enlarge the visual perception capability. In the stage of feature map upsampling, our proposed channel–spatial attention module adopted after each feature map concatenation operation can increase the feature learning efficiency and enlarge the feature learning scale by synthesizing channel-wise and spatial attention feature learning modules. Detailed calculations of channel-wise and spatial attention modules can be found in Eqs. (1)–(7).
Spatial average pooling (sa) and spatial maximum pooling (sm) operations are calculated as the average value and maximum value of input feature map f with a size of m×n, as described in Eqs. (1) and (2). Correspondingly, the channel-wise average (ca) and maximum pooling (cm) operations are the average feature values of all the h channels and the maximum feature values of all the channels in Eqs. (3) and (4). The output feature maps of the spatial attention module (SA) and channel attention module (CA) are calculated according to Eqs. (5) and (6), respectively, and the synthesis of the feature maps from the channel and spatial attention modules is realized by multiplication, as illustrated in Eq. (7). Through multi-scale feature enhancement by our proposed Res2-UnetA framework, it can learn the multi-scale storage tank features hierarchically and comprehensively from the high-spatial-resolution images of the different imaging sensors.
4.2.2 Storage tank model construction and dataset generation
Based on our proposed framework Res2-UnetA, the pre-processed high-spatial-resolution images for the city regions of Ningbo, Tangshan, and Dongying are used to train the storage tank extraction model. Ningbo, Tangshan, and Dongying are three typical city regions in China, with large densities of storage tanks, so they can provide large quantities of training samples with high spectral and textual feature variety in different sizes. The storage tanks in the collected high-spatial-resolution images for the training dataset are interpreted visually by three experts in a related field. The model is fine-tuned based on the optimized model from Res2-Unet+ (Yu et al., 2021), with a learning rate of 0.01. It converges to the optimum at iteration 69.
With the optimized model, the storage tanks for the remaining city regions are extracted accordingly and vectorized to the shapefile. While the enhanced model for extracting storage tanks generally performs well, it's not infallible. Some tanks are inadvertently missed, and other objects with similar spectral or textural characteristics are occasionally mistakenly identified as tanks. Therefore, each vectorized shapefile is further refined manually by visual interpretation of the high-spatial-resolution images. Due to the inconsistent spectral intensities for the storage tanks in the images, triggered by shadows and different viewing angles, the vectorized storage tanks in the inventory take different shapes. To synchronize the storage tanks in the inventory taking on a round shape, we re-construct a circle for each extracted storage tank according to the radius calculated in the inventory, and the inventory is updated with the re-constructed circle. To facilitate the dating of each storage tank's construction year, the reconstructed circle for each extracted storage tank has been manually validated and refined by six experienced experts through visual interpretation based on our collected high-spatial-resolution images and field survey.
4.3 Construction year assignment
In the STD we developed, a team of six experts determined the construction year for each storage tank by conducting visual assessments using high-resolution historical images available on Google Earth, with the cutoff date for this process being 1 January 2024. The intermittent availability of historical high-resolution images on Google Earth poses a challenge in determining the precise construction years for many storage tanks, especially when images from successive years are missing. We documented the most recent year when a storage tank was absent (last-year image, without the storage tank) and the earliest year when it was first observed (first-year image, with the storage tank) in the historical imagery, as illustrated in Fig. 4. The actual construction year lies within this time frame. For analysis simplicity, we have designated each tank's initial observed year to be the construction year.
Since the high-resolution images used to compile the storage tank dataset were captured in 2021, it is presumed that all tanks were constructed no later than this year. However, due to the absence of updated high-resolution imagery on Google Earth, 488 tanks remain undetected in the historical records. For these, the year of construction has been inferred as 2021 following thorough visual confirmation using the high-resolution images we have acquired. The considerable lapses in historical high-resolution imagery on Google Earth necessitate assigning a provisional construction year, 2021, to 630 storage tanks. The year 2021 marks the earliest documented evidence of these tanks' existence in the high-resolution images we collected, beyond which no prior images are available. For the storage tanks built before 2000, they are recorded with the first-year image, with a storage tank, in the shapefile, but lacking the last-year image, without a storage tank, in our proposed dataset, STD, due to the limited accessibility of high-spatial-resolution images before 2000 from Google Earth.
5.1 Spatial distribution of storage tanks
Following the workflow in Fig. 4, the storage tanks in the 92 typical city regions of China are extracted based on the high-spatial-resolution images using the trained semantic segmentation model. Given that large-capacity storage tanks are known to release significant levels of CH4, resulting in climate warming, the proposed inventory focuses on storage tanks with an area of no less than 500 m2. 14 461 storage tanks are extracted from the 92 city regions with areas ranging from 500 m2 to 18 583.15 m2. As shown in Fig. 6, the storage tanks are distributed unevenly in different city regions and reflect different footprints and spatial distribution patterns. To explore the different distribution patterns, the storage tanks are categorized into three groups according to the area: 500–1000, 1000–10 000, and ≥ 10 000 m2. The accumulated number of storage tanks of different footprints for all the city regions is compiled as shown in Fig. 7. It may be seen that there are more storage tanks of 500–1000 m2 than those with larger footprints. The relatively smaller storage tanks are more widely used in industry. Due to the high cost of construction, considering all the city regions, the maximum number of large storage tanks with a footprint of ≥ 10 000 m2 is found to be seven, for the city of Tangshan. Notably, there are few city regions with storage tanks of 10 000 m2 in footprint.
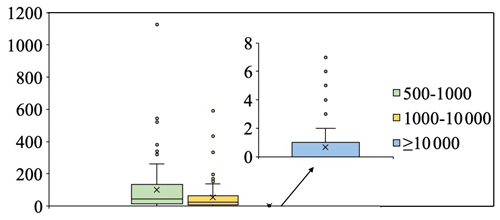
Figure 7Box plot of storage tank distribution for the different footprint categories (in m2) for the 92 city regions.
About the 92 city regions examined, 38 city regions have storage tanks with an accumulated number of ≥ 100, as shown in Fig. 8a. Dongying has the largest accumulated number, 1719, about twice that of Ningbo, the second-highest-ranked city with 981 storage tanks. Weifang and Panjin are next in rank, with more than 500 storage tanks. The number of storage tanks with a footprint of 500–1000 m2 is greater than that for tanks with a footprint of 1000–10 000 and ≥ 10 000 m2 for most city regions. This finding indicates the widespread use of smaller storage tanks in different industries. Furthermore, there are 36 city regions with an accumulated number of tanks of < 50 (Fig. 8b). Among the 36 city regions, Hebi is the only city with four storage tanks of ≥ 10 000 m2 in footprint. The other city regions, except Tangshan, do not have storage tanks that large. No storage tanks with a footprint of ≥ 500 m2 are observed for the city regions of Tai'an, Weihai, and Zigong.
5.2 Spatial consistency with CH4 emission
To explore whether the distribution patterns of storage tanks significantly influence CH4 emissions, we explored the spatial consistency between estimated CH4 from energy emission products in the year 2020 and the density of storage tanks in our proposed dataset, STD, over the study area. Given the coarser spatial resolution of the CH4 emission product at 0.5°, which is less detailed than that of the high-spatial-resolution images used for generating our storage tank dataset, we have calculated storage tank density to align with each pixel grid of the CH4 data. The density is defined by the total storage tank area ratio within each corresponding 3025 km2 pixel grid area (55 km × 55 km), where 55 km is an approximation of 0.5° latitude or longitude at the Equator.
The storage tank density is calculated for each grid pixel of the CH4 emission product and is demonstrated in Fig. 9. We can recognize that large-scale areas with high CH4 emissions in the atmosphere generally cluster large densities of storage tanks (clustered cases of A, B, C, and D). The sparsely distributed storage tanks with a high density are mostly accompanied by a higher CH4 emission than that of the neighborhood (as shown in cases of E). There are also some city regions, especially coastal cities, with a high density of storage tanks and low CH4 emission estimation, as in the cases of F. This could be attributed to the coastal air currents, which likely disperse CH4 emissions more effectively. It also needs to be pointed out that for the city regions marked as G in Fig. 9, the estimated CH4 emission is relatively high but the density of storage tanks is low. One possible reason is the unrestrained leakage of CH4 from the storage tanks, highlighting the urgent need for effective control measures. Alternatively, other high-energy activities within these regions might be significant CH4 contributors, suggesting a need for comprehensive investigation into broader mitigation strategies.
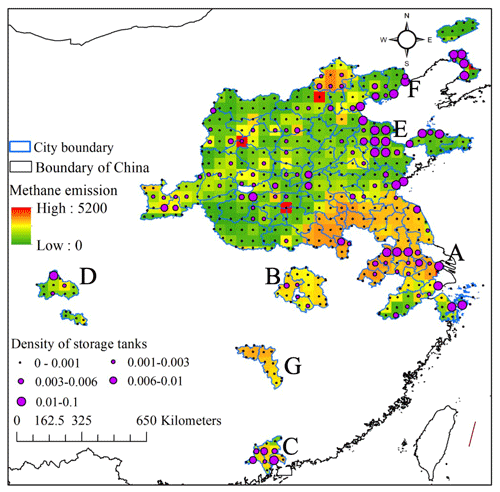
Figure 9Spatial distribution pattern of different densities of storage tank areas with different CH4 emissions in the atmosphere.
To objectively explore the spatial consistency of storage tank distribution and CH4 emission from energetic activities, we randomly selected 4000 storage tank pixels and 4000 background object pixels to evaluate the significance of the impact of storage tanks on CH4 emission. Referring to Fig. 3, the value of CH4 emission varies by a large margin between 0.000055 and 5160.32 Tg CH4 yr−1. The large value gap of CH4 emission causes bias in the differential significance test. We generated the quantity distribution of pixels with different CH4 emission value gaps (as shown in Fig. 10a) and found that 99.83 % of pixels have a CH4 emission value of < 100 Tg CH4 yr−1. Therefore, the 4000 storage tank pixels and 4000 background object pixels are randomly selected from pixels with a CH4 emission value of < 100 Tg CH4 yr−1. As shown in Fig. 10b, the CH4 emission values of storage tank pixels are statistically significantly larger than that of background object pixels at a confidence level of p=0.05. It indicates storage tanks are significant energetic sources of CH4 emission. With our proposed dataset, STD, it is possible to monitor the greenhouse gas emissions from storage tanks to take effective measurements for potential climate warming reduction in time.
5.3 Temporal impact on CH4 emission
Given the constraints of historical high-resolution imagery on Google Earth, the earliest ascertainable construction year for storage tanks is set to 2000, with the latest capped at 2021, as depicted in Fig. 11. Therefore, our dataset, STD, includes storage tanks constructed in years of 2000–2021. It is noted that storage tanks were largely constructed in 2009, 2010, 2012, 2013, and 2014, while fewer were constructed in 2000 and 2001, with the quantity being approximately 20. To align with the construction temporal range of storage tanks in the dataset, CH4 emission products of 2005, 2010, 2015, and 2020 are utilized, as these emission products are updated every 5 years. To explore the impact of storage tank construction on CH4 emission, the storage tanks are grouped by the product year of CH4, as listed in Table 2. Storage tanks built in the years 2000 and 2021 are excluded from the impact analysis due to the extent of the corresponding temporal impact range of CH4 emission.
Table 2Correspondence between the year of CH4 emission product and group of construction years of storage tanks.
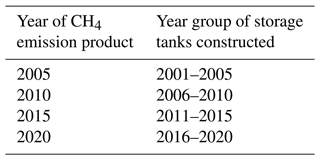
It is noted that the spatial resolution of the CH4 emission product is coarser than the images we used to generate our proposed dataset, STD; similarly to the works in spatial consistency exploration, the storage tanks constructed in different groups of years are gridded by the CH4 emission product, and the density of storage tanks is calculated for each grid. We conducted a correlation analysis to explore the statistical significance of the impact of storage tank construction on CH4 emission over 2005–2020 at levels of p=0.05 and p=0.1, respectively. Moreover, the rate of CH4 emission change and newly constructed storage tank density for every 5 years are calculated according to Eq. (8) and demonstrated accordingly in Fig. 12.
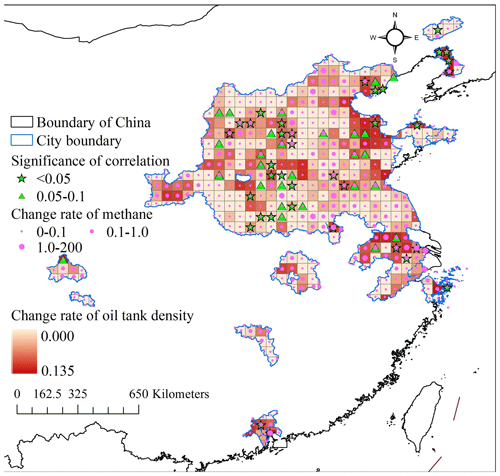
Figure 12Significance of correlation between change rate of storage tank density and CH4 emission change.
Both CH4 emission and newly constructed storage tank density increased from 2005 to 2020, with positive rates in Fig. 12. In the 92 city regions in this study, storage tanks are constantly being constructed to meet the industrial demand, but CH4 emission is on a continuous increase too. The storage tanks in city regions such as Yingkou, Panjin, Dongying, Binzhou, Yantai, Weifang, Tangshan, Linyi, Rizhao, Puyang, Xi'an, Pingdingshan, Huainan, Nanjing, Maanshan, Changzhou, Wuxi, Chengdu, Foshan, Dongguan, and Guangzhou are constructed with a higher rate than in other city regions. CH4 from energetic activities is emitted at a highly increasing rate in multiple city regions, such as Beijing, Yingkou, Zhenjiang, Nanjing, Maanshan, Changzhou, Wuxi, Shijiazhuang, Huainan, and Dongguan. Grids showing a statistically significant correlation (p<0.1) between storage tank density and CH4 emissions typically display a notable rise in the rate of storage tank density, particularly in grids with a confidence level of p=0.05. This trend suggests that areas with active storage tank construction may contribute significantly to increased CH4 emissions. Some grids exhibit high increasing rates of CH4 emission but low increasing rates of storage tank density. This pattern suggests that while storage tank construction significantly contributes to CH4 emissions, other sources related to energy production, such as the extraction and transport of coal, oil, and natural gas, are also major contributors to CH4 release. However, regarding the 92 typical city regions with intensive storage tank distribution and construction, the impact of storage tank construction on CH4 emission from energetic activities is largely statistically significant, especially in areas with a high rate of new storage tank construction. Therefore, it is necessary to propose effective measurements to mitigate CH4 emissions from the continuously constructed storage tanks.
6.1 Comparison with published datasets
To the best of our knowledge, limited research has been published concerning remote sensing datasets on storage tanks. The dataset NEPU–OWOD V1.0 is a recently proposed oil storage tank dataset featuring 1192 oil storage tanks from 432 images of Google Earth. It covers the city of Daqing on a limited scale. However, the dataset lacks georeferenced information and hence has had difficulty in finding support by governmental agencies and academic groups for further research on various subjects, such as air pollution control and energy consumption balance studies (Wang et al., 2021).
The Oil and Gas Tank Dataset, which is similar to the NEPU–OWOD V1.0 dataset and comprises 760 image patches with a size of 512×512 pixels, has also been proposed (Rabbi et al., 2020). The images are taken at a spatial resolution of 30 cm, and the annotations are boundary boxes rather than details on the exact shape. To assess the national energy demand, an oil storage tank dataset is released on the platform Kaggle (Airbusgeo, 2019). However, the images are collected from Google Earth without georeferenced information. Only 100 image patches with a size of 512×512 pixels are included in the dataset.
Publication of datasets on oil storage tanks is generally developed to improve automatic methods for the detection of storage tanks rather than further environmental analysis based on the combination and synthesis with datasets of other domains, such as air pollution products. Therefore, the proposed dataset, STD, is the first storage tank inventory that provides a detailed distribution of storage tanks of diverse footprints in 92 city regions in China. Each storage tank in the dataset has undergone rigorous verification by six experts. Additionally, the dataset meticulously logs the construction year for each tank. This allows for an analysis of the temporal evolution of storage tank distribution and its combined effects with CH4 emissions on the climate. Such insights pave the way for developing more effective energy management and climate change mitigation strategies, serving as a valuable resource for research in atmospheric science, environmental studies, and sustainable development.
6.2 Uncertainties, limitations, and implications
The Storage Tank Dataset (STD) we have compiled for 92 city regions in China serves as a valuable tool for climate change research despite certain limitations. The extraction process from high-resolution images is subject to inaccuracies due to shadows and the inherent limitations of representing three-dimensional tanks as two-dimensional circles, potentially leading to slight positional errors (Fig. 13a). Additionally, the variance in perspective between our collected high-spatial-resolution images and Google Earth historical images can cause deviations in visual refinement in the tanks' vectorized outlines (as shown in Fig. 13b). To mitigate these issues, expert analysis is employed to ensure tank identification and location precision, referring to the collected high-spatial-resolution images.
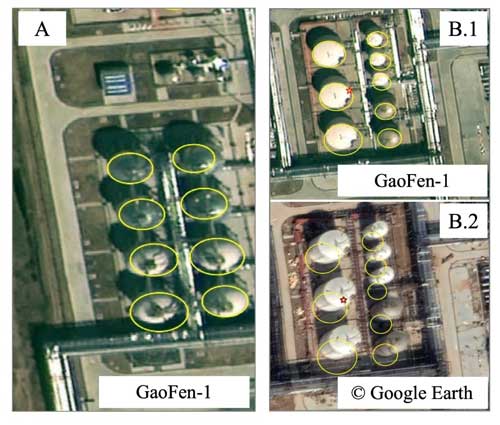
Figure 13Example cases of our proposed STD: (a) cases with shifted circles due to cast by shadow and (b) cases with largely deviated circles in different images due to different viewing angles.
The pioneering dataset, STD, encompasses georeferenced storage tank shapes for 92 key Chinese city regions crafted from high-resolution images. For each storage tank, the corresponding construction year is assigned, referring to the high-resolution historical images of Google Earth. It's a versatile resource, with spatial and temporal distribution patterns for not only mapping CH4 and other emissions but also for aiding the development of infrastructural strategies across various industries. However, the dataset currently lacks volumetric data due to the absence of height measurements for the tanks. Future enhancements aim to incorporate height data through advanced remote sensing technologies like synthetic-aperture radar (SAR) imagery, enriching the dataset with three-dimensional accuracy and providing a more comprehensive understanding of storage tank capacities.
The dataset, STD, is publicly available as a repository at https://doi.org/10.5281/zenodo.10514151 (Chen et al., 2024). The dataset is provided in a shapefile, wherein a polygon with an area attribute in units of square meters represents each storage tank and two attributes of years, year_1 and year_2, indicating the most recent year when a storage tank was absent (last-year image, without the storage tank) and the earliest year when it was first observed (first-year image, with the storage tank), respectively. The inventory is intended to be used to further analyze the impact on CH4 emissions, devise and implement more efficient energy management strategies. Moreover, our approach represents a powerful new source to improve automatic methods for storage tank extraction from high-spatial-resolution images, given that it represents a comprehensive and state-of-the-art inventory with tens of thousands of storage tanks georeferenced in 92 typical city regions over China.
In support of CH4 emission control to mitigate climate warming, the STD is proposed by providing a meticulously georeferenced inventory of storage tanks larger than 500 m2 across 92 key city regions of China in the years 2000–2021. Leveraging a novel semantic segmentation framework, Res2-UnetA, and rigorous visual interpretation based on the collected high-spatial-resolution images, historical high-spatial-resolution images from Google Earth, and field survey, the dataset not only details the spatial distribution of large storage tanks but also includes their construction years. Based on the STD dataset, the spatial distribution pattern of the storage tanks of different footprints was analyzed in 92 city regions. We also explored the impact of storage tank construction on CH4 emission from energetic activities during 2005–2020. Compared with the published datasets for storage tanks, the STD is the first inventory that compiles georeferenced storage tanks in 92 city regions with detailed shape boundaries and construction years. In general, publicly available datasets on storage tanks typically cover only part of a city, without georeferenced information and detailed shape boundaries. It is, therefore, difficult to objectively explore the extent and patterns of environmental impact and the energy management of the storage tanks on a large scale. The STD enables a large-scale environmental impact analysis of storage tanks and their correlation with CH4 emissions. It demonstrates strong spatial consistency with CH4 emissions in 92 typical Chinese city regions, highlighting the substantial increase in CH4 emissions due to storage tank construction. The storage tank dataset, STD, can contribute significantly to supporting energy management strategies and sustainability development studies while giving direct support to academic research and government agencies.
FC and LW designed the study and conducted the experiments. YW, HZ, NW, PM, and BY compiled the dataset. FC, LW, and BY wrote the paper; all authors reviewed and approved the paper.
The contact author has declared that none of the authors has any competing interests.
Publisher’s note: Copernicus Publications remains neutral with regard to jurisdictional claims made in the text, published maps, institutional affiliations, or any other geographical representation in this paper. While Copernicus Publications makes every effort to include appropriate place names, the final responsibility lies with the authors. Regarding the maps used in this paper, please note that Figs. 1, 3, 6, 9, and 12 contain disputed territories.
The authors would like to thank the editors and the reviewers for their useful feedback that improved this paper.
This work was supported by the National Key R&D Program of China (grant no. 2022YFC3800700), the Youth Innovation Promotion Association of CAS (grant no. 2022122), and the China-ASEAN Big Earth Data Platform and Applications (grant no. guikeAA20302022).
This paper was edited by Peng Zhu and reviewed by three anonymous referees.
Airbusgeo: Airbus oil storage detection, https://www.kaggle.com/datasets/airbusgeo/airbus-oil-storage-detection-dataset (last access: 19 July 2024), 2019.
Amann, M., Bertok, I., Borken-Kleefeld, J., Cofala, J., Heyes, C., Höglund-Isaksson, L., Klimont, Z., Nguyen, B., Posch, M., and Rafaj, P.: Cost-effective control of air quality and greenhouse gases in Europe: Modeling and policy applications, Environ. Model. Softw., 26, 1489–1501, https://doi.org/10.1016/j.envsoft.2011.07.012, 2011.
Badrinarayanan, V., Kendall, A., and Cipolla, R.: Segnet: A deep convolutional encoder-decoder architecture for image segmentation, IEEE T. Pattern Anal., 39, 2481–2495, https://doi.org/10.1109/TPAMI.2016.2644615, 2017.
Chen, F., Wang, N., Yu, B., and Wang, L.: Res2-Unet, a new deep architecture for building detection from high spatial resolution images, IEEE J. Sel. Top. Appl., 15, 1494–1501, https://doi.org/10.1109/JSTARS.2022.3146430, 2022.
Chen, F., Wang, J., Li, B., Yang, A., and Zhang, M.: Spatial variability in melting on Himalayan debris-covered glaciers from 2000 to 2013, Remote Sens. Environ., 291, 113560, https://doi.org/10.1016/j.rse.2023.113560, 2023.
Chen, F., Wang, L., Wang, Y., Zhang, H., Wang, N., Ma, P., and Yu, B.: Retrieval of dominant methane (CH4) emission sources, the first high resolution (1–2 m) dataset of storage tank in China in 2021, Zenodo [data set], https://doi.org/10.5281/zenodo.10514151, 2024.
Chen, L.-C., Papandreou, G., Schroff, F., and Adam, H.: Rethinking atrous convolution for semantic image segmentation, arXiv [preprint], https://doi.org/10.48550/arXiv.1706.05587, 2017a.
Chen, L.-C., Papandreou, G., Kokkinos, I., Murphy, K., and Yuille, A. L.: Deeplab: Semantic image segmentation with deep convolutional nets, atrous convolution, and fully connected crfs, IEEE T. Pattern Anal., 40, 834–848, https://doi.org/10.1109/TPAMI.2017.2699184, 2017b.
Crippa, M., Oreggioni, G., Guizzardi, D., Muntean, M., Schaaf, E., Lo Vullo, E., Solazzo, E., Monforti-Ferrario, F., Olivier, J. G., and Vignati, E.: Fossil CO2 and GHG emissions of all world countries – 2019 Report, Publication Office of the European Union, Luxemburg, https://doi.org/10.2760/687800, 2019.
Ding, T., Ning, Y., and Zhang, Y.: Estimation of greenhouse gas emissions in China 1990–2013, Greenh. Gases, 7, 1097–1115, https://doi.org/10.1002/ghg.1718, 2017.
Fan, L., Chen, X., Wan, Y., and Dai, Y.: Comparative Analysis of Remote Sensing Storage Tank Detection Methods Based on Deep Learning, Remote Sens., 15, 2460, https://doi.org/10.3390/rs15092460, 2023.
Gao, S.-H., Cheng, M.-M., Zhao, K., Zhang, X.-Y., Yang, M.-H., and Torr, P.: Res2net: A new multi-scale backbone architecture, IEEE T. Pattern Anal., 43, 652–662, https://doi.org/10.1109/TPAMI.2019.2938758, 2019.
Hoesly, R. M., Smith, S. J., Feng, L., Klimont, Z., Janssens-Maenhout, G., Pitkanen, T., Seibert, J. J., Vu, L., Andres, R. J., Bolt, R. M., Bond, T. C., Dawidowski, L., Kholod, N., Kurokawa, J.-I., Li, M., Liu, L., Lu, Z., Moura, M. C. P., O'Rourke, P. R., and Zhang, Q.: Historical (1750–2014) anthropogenic emissions of reactive gases and aerosols from the Community Emissions Data System (CEDS), Geosci. Model Dev., 11, 369–408, https://doi.org/10.5194/gmd-11-369-2018, 2018.
Hou, B., Ren, Z., Zhao, W., Wu, Q., and Jiao, L.: Object detection in high-resolution panchromatic images using deep models and spatial template matching, IEEE T. Geosci. Remote, 58, 956–970, https://doi.org/10.1109/TGRS.2019.2942103, 2019.
Hou, D., Miao, Z., Xing, H., and Wu, H.: Two novel benchmark datasets from ArcGIS and bing world imagery for remote sensing image retrieval, Int. J. Remote Sens., 42, 240–258, https://doi.org/10.1080/01431161.2020.1804090, 2021.
IEA: World Energy Outlook 2018, IEA, Paris, https://www.iea.org/reports/world-energy-outlook-2018 (last access: 19 July 2024), 2018.
Im, S., Mostafa, A., Lim, K.-H., Kim, I., and Kim, D.-H.: Automatic temperature rise in the manure storage tank increases methane emissions: Worth to cool down!, Sci. Total Environ., 823, 153533, https://doi.org/10.1016/j.scitotenv.2022.153533, 2022.
Johnson, D., Clark, N., Heltzel, R., Darzi, M., Footer, T. L., Herndon, S., and Thoma, E. D.: Methane emissions from oil and gas production sites and their storage tanks in West Virginia, Atmos. Environ., 16, 100193, https://doi.org/10.1016/j.aeaoa.2022.100193, 2022.
Karra, K., Kontgis, C., Statman-Weil, Z., Mazzariello, J. C., Mathis, M., and Brumby, S. P.: Global land use/land cover with Sentinel 2 and deep learning, IEEE International Geoscience and Remote Sensing Symposium IGARSS, 4704–4707, https://doi.org/10.1109/IGARSS47720.2021.9553499, 2021.
Kirschke, S., Bousquet, P., Ciais, P., Saunois, M., Canadell, J. G., Dlugokencky, E. J., Bergamaschi, P., Bergmann, D., Blake, D. R., Bruhwiler, L., Cameron-Smith, P., Castaldi, S., Chevallier, F., Feng, L., Fraser, A., Heimann, M., Hodson, E. L., Houweling, S., Josse, B., Fraser, P. J., Krummel, P. B., Lamarque, J.-F., Langenfelds, R. L., Le Quéré, C., Naik, V., O'Doherty, S., Palmer, P. I., Pison, I., Plummer, D., Poulter, B., Prinn, R. G., Rigby, M., Ringeval, B., Santini, M., Schmidt, M., Shindell, D. T., Simpson, I. J., Spahni, R., Steele, L. P., Strode, S. A., Sudo, K., Szopa, S., van der Werf, G. R., Voulgarakis, A., van Weele, M., Weiss, R. F., Williams, J. E., and Zeng, G.: Three decades of global methane sources and sinks, Nat. Geosci., 6, 813–823, https://doi.org/10.1038/ngeo1955, 2013.
Kurokawa, J., Ohara, T., Morikawa, T., Hanayama, S., Janssens-Maenhout, G., Fukui, T., Kawashima, K., and Akimoto, H.: Emissions of air pollutants and greenhouse gases over Asian regions during 2000–2008: Regional Emission inventory in ASia (REAS) version 2, Atmos. Chem. Phys., 13, 11019–11058, https://doi.org/10.5194/acp-13-11019-2013, 2013.
Lin, X., Zhang, W., Crippa, M., Peng, S., Han, P., Zeng, N., Yu, L., and Wang, G.: A comparative study of anthropogenic CH4 emissions over China based on the ensembles of bottom-up inventories, Earth Syst. Sci. Data, 13, 1073–1088, https://doi.org/10.5194/essd-13-1073-2021, 2021.
Long, J., Shelhamer, E., and Darrell, T.: Fully convolutional networks for semantic segmentation, Proceedings of the IEEE Conference on Computer Vision and Pattern Recognition, 3431–3440, https://doi.org/10.1109/CVPR.2015.7298965, 2015.
Majumder, A., He, Z., Towles, H., and Welch, G.: Achieving color uniformity across multi-projector displays, Proceedings Visualization 2000, VIS 2000 (Cat. No. 00CH37145), 117–124, https://doi.org/10.1109/VISUAL.2000.885684, 2000.
Montzka, S. A., Dlugokencky, E. J., and Butler, J. H.: Non-CO2 greenhouse gases and climate change, Nature, 476, 43–50, https://doi.org/10.1145/361237.361242, 2011.
O'Duda, R.: Use of Hough transformation to detect lines and curves in picture, Commun. ACM, 15, 11–15, 1972.
Peng, S., Piao, S., Bousquet, P., Ciais, P., Li, B., Lin, X., Tao, S., Wang, Z., Zhang, Y., and Zhou, F.: Inventory of anthropogenic methane emissions in mainland China from 1980 to 2010, Atmos. Chem. Phys., 16, 14545–14562, https://doi.org/10.5194/acp-16-14545-2016, 2016.
Rabbi, J., Ray, N., Schubert, M., Chowdhury, S., and Chao, D.: Small-Object Detection in Remote Sensing Images with End-to-End Edge-Enhanced GAN and Object Detector Network, Remote Sens., 12, 1432, ttps://doi.org/10.3390/rs12091432, 2020.
Ronneberger, O., Fischer, P., and Brox, T.: U-net: Convolutional networks for biomedical image segmentation, Medical Image Computing and Computer-Assisted Intervention–MICCAI 2015, 234–241, https://doi.org/10.1007/978-3-319-24574-4_28, 2015.
Sahu, V. and Sahu, D.: Image fusion using Wavelet Transform: A Review, Global Journal of Computer Science and Technology, 14, 21–28, 2014.
Shen, D.: Image registration by local histogram matching, Pattern Recogn., 40, 1161–1172, https://doi.org/10.1016/j.patcog.2006.08.012, 2007.
Stocker, T.: Climate change 2013 – the physical science basis, Working Group I contribution to the Fifth assessment report of the Intergovernmental Panel on Climate Change, Cambridge university press, https://doi.org/10.1017/CBO9781107415324, 2014.
Venter, Z. S., Barton, D. N., Chakraborty, T., Simensen, T., and Singh, G.: Global 10 m Land Use Land Cover Datasets: A Comparison of Dynamic World, World Cover and Esri Land Cover, Remote Sens., 14, 4101, https://doi.org/10.3390/rs14164101, 2022.
Vermote, E. F., Tanré, D., Deuze, J. L., Herman, M., and Morcette, J.-J.: Second simulation of the satellite signal in the solar spectrum, 6S: An overview, IEEE T. Geosci. Remote, 35, 675–686, https://doi.org/10.1109/36.581987, 1997.
Wang, H., Sun, S., Nie, L., Zhang, Z., Li, W., and Hao, Z.: A review of whole-process control of industrial volatile organic compounds in China, J. Environ. Sci., 123, 127–139, https://doi.org/10.1016/j.jes.2022.02.037, 2022.
Wang, Z., Bai, L., Song, G., Zhang, J., Tao, J., Mulvenna, M. D., Bond, R. R., and Chen, L.: An Oil Well Dataset Derived from Satellite-Based Remote Sensing, Remote Sens., 13, 1132, https://doi.org/10.3390/rs13061132, 2021.
Wu, Q., Zhang, B., Xu, C., Zhang, H., and Wang, C.: Dense Oil Tank Detection and Classification via YOLOX-TR Network in Large-Scale SAR Images, Remote Sens., 14, 3246, https://doi.org/10.3390/rs14143246, 2022.
Xia, X., Liang, H., RongFeng, Y., and Kun, Y.: Oil tank extraction in high-resolution remote sensing images based on deep learning, 2018 26th International Conference on Geoinformatics, 1–6, https://doi.org/10.1109/GEOINFORMATICS.2018.8557161, 2018.
Yang, L., Meng, X., and Zhang, X.: SRTM DEM and its application advances, Int. J. Remote Sens., 32, 3875–3896, https://doi.org/10.1080/01431161003786016, 2011.
Yu, B., Chen, F., Wang, Y., Wang, N., Yang, X., Ma, P., Zhou, C., and Zhang, Y.: Res2-Unet+, a Practical Oil Tank Detection Network for Large-Scale High Spatial Resolution Images, Remote Sens., 13, 4740, https://doi.org/10.3390/rs13234740, 2021.
Yu, B., Xu, C., Chen, F., Wang, N., and Wang, L.: HADeenNet: A hierarchical-attention multi-scale deconvolution network for landslide detection, Int. J. Appl. Earth Obs., 111, 102853, https://doi.org/10.1016/j.jag.2022.102853, 2022a.
Yu, B., Yang, A., Chen, F., Wang, N., and Wang, L.: SNNFD, spiking neural segmentation network in frequency domain using high spatial resolution images for building extraction, Int. J. Appl. Earth Obs., 112, 102930, https://doi.org/10.1016/j.jag.2022.102930, 2022b.
Yu, B., Chen, F., Wang, N., Wang, L., and Guo, H.: Assessing changes in nighttime lighting in the aftermath of the Turkey-Syria earthquake using SDGSAT-1 satellite data, The Innovation, 4, 100419, https://doi.org/10.1016/j.xinn.2023.100419, 2023a.
Yu, B., Chen, F., Ye, C., Li, Z., Dong, Y., Wang, N., and Wang, L.: Temporal expansion of the nighttime light images of SDGSAT-1 satellite in illuminating ground object extraction by joint observation of NPP-VIIRS and sentinel-2A images, Remote Sens. Environ., 295, 113691, https://doi.org/10.1016/j.rse.2023.113691, 2023b.
Yuen, H., Princen, J., Illingworth, J., and Kittler, J.: Comparative study of Hough transform methods for circle finding, Image Vision Comput., 8, 71–77, https://doi.org/10.1016/0262-8856(90)90059-E, 1990.
Zalpour, M., Akbarizadeh, G., and Alaei-Sheini, N.: A new approach for oil tank detection using deep learning features with control false alarm rate in high-resolution satellite imagery, Int. J. Remote Sens., 41, 2239–2262, https://doi.org/10.1080/01431161.2019.1685720, 2020.
Zhang, L. and Liu, C.: Oil tank extraction based on joint-spatial saliency analysis for multiple SAR images, IEEE Geosci. Remote Sens. Lett., 17, 998–1002, https://doi.org/10.1109/LGRS.2019.2937355, 2019.
Zhang, Z., Hu, S., and Jing, Y.: China achieving carbon neutral in 2060, fossil energy to fossil resource era, Modern Chem. Ind., 41, 1–5, https://doi.org/10.16606/j.cnki.issn0253-4320.2021.06.001, 2021.
Zhao, H., Shi, J., Qi, X., Wang, X., and Jia, J.: Pyramid scene parsing network, Proc. CVPR. CSFW., 6230–6239, https://doi.org/10.1109/CVPR.2017.660, 2017.