the Creative Commons Attribution 4.0 License.
the Creative Commons Attribution 4.0 License.
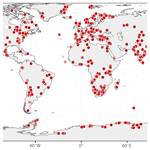
The secret life of garnets: a comprehensive, standardized dataset of garnet geochemical analyses integrating localities and petrogenesis
Morgan Gabor
Isabella Lupini
Randolph Rutledge
Julia Ann Nord
Shuang Zhang
Asmaa Boujibar
Emma S. Bullock
Michael J. Walter
Kerstin Lehnert
Frank Spear
Shaunna M. Morrison
Robert M. Hazen
Integrating mineralogy with data science is critical to modernizing Earth materials research and its applications to geosciences. Data were compiled on 95 650 garnet sample analyses from a variety of sources, ranging from large repositories (EarthChem, RRUFF, MetPetDB) to individual peer-reviewed literature. An important feature is the inclusion of mineralogical “dark data” from papers published prior to 1990. Garnets are commonly used as indicators of formation environments, which directly correlate with their geochemical properties; thus, they are an ideal subject for the creation of an extensive data resource that incorporates composition, locality information, paragenetic mode, age, temperature, pressure, and geochemistry. For the data extracted from existing databases and literature, we increased the resolution of several key aspects, including petrogenetic and paragenetic attributes, which we extended from generic material type (e.g., igneous, metamorphic) to more specific rock-type names (e.g., diorite, eclogite, skarn) and locality information, increasing specificity by examining the continent, country, area, geological context, longitude, and latitude. Likewise, we utilized end-member and quality index calculations to help assess the garnet sample analysis quality. This comprehensive dataset of garnet information is an open-access resource available in the Evolutionary System of Mineralogy Database (ESMD) for future mineralogical studies, paving the way for characterizing correlations between chemical composition and paragenesis through natural kind clustering (Chiama et al., 2022; https://doi.org/10.48484/camh-xy98). We encourage scientists to contribute their own unpublished and unarchived analyses to the growing data repositories of mineralogical information that are increasingly valuable for advancing scientific discovery.
- Article
(2664 KB) - Full-text XML
-
Supplement
(505 KB) - BibTeX
- EndNote
As scientific discovery becomes increasingly dependent on the internet, older publications are disappearing from the scientific record. Mineral analyses published prior to 1990 are recorded in documents (hard-copy journals, books, scanned PDFs, and photographs) that are difficult to convert to a digital format. Without efforts to collect and preserve these data, their value will be lost to the scientific community and become “dark data”, information that is not currently accessible in existing geochemical databases or is not represented in the supplementary data of peer-reviewed literature (Hazen et al., 2019; Prabhu et al., 2020). This project emphasizes accumulating dark data with large datasets which both prevents the loss of scientific material and expands the availability of mineralogical data (Hazen, 2014; Hazen et al., 2019; Wilkinson et al., 2016).
The aim of this project is to compile a dataset of geochemical, temporal, and spatial properties pertaining to the garnet mineral group as a means for data-driven discovery in mineralogy and petrology. Gathering data from existing literature and presenting the results in an easily accessible manner with tabulated numeric and categorical data provide opportunities for inductive inference (Hazen et al., 2019; Wilkinson et al., 2016) and abductive discovery (Hazen, 2014). Dark data were collected and tabulated along with information from established geochemical databases and recent publications to create a comprehensive and standardized dataset (Chassé et al., 2018; Deer et al., 1982; Gatewood et al., 2015; Hazen et al., 2019; Jochum et al., 2007; Lehnert et al., 2000; Locock, 2008; Spear et al., 2009; Wilkinson et al., 2016). The resultant garnet dataset consists of 95 650 sample analyses from peer-reviewed literature published between 1949 and 2019. The dataset incorporates 186 diverse attributes pertaining to locality information, petrogenetic and paragenetic mode, major element oxides, trace elements, isotopic ratios, and rare earth elements (REEs) as well as additional information when available, such as zonation, color, age, temperature, and pressure. The creation of this dataset required a series of definitions and assumptions to maximize the amount of information recorded for each sample without losing the standardization. Specific information regarding each attribute can be found in the Methods section (Sect. 2). This newly compiled dataset offers researchers the opportunity to explore the spatial and temporal history of garnet formation and related geologic processes by using multiple statistical and machine learning techniques, specifically in the evolutionary system of mineralogy and natural kind clustering (Hazen et al., 2019; Morrison et al., 2020).
1.1 Data integration
Integrating mineralogy with data science is an important step to modernize the field of earth science. Mineral informatics relies on robust and cohesive mineral databases (Hazen et al., 2019; Lafuente et al., 2015; Lehnert et al., 2000; Morrison et al., 2020; Prabhu et al., 2020, 2022; Spear et al., 2009). Typical examples of existing open-access databases in the mineralogical community include Mindat, EarthChem, MetPetDB, PetDB, the RRUFF project, the Mineral Evolution Database (MED), GeoRoc, and GeoReM (Mindat: https://www.mindat.org, last access: 21 September 2023; EarthChem Portal: http://www.earthchem.org, last access: 21 September 2023; PetDB: https://search.earthchem.org, last access: 21 September 2023; The RRUFF Project: https://rruff-2.geo.arizona.edu, last access: 21 September 2023; GeoRoc: http://georoc.mpch-mainz.gwdg.de/georoc/Start.asp, last access: 21 September 2023; GeoReM: http://georem.mpch-mainz.gwdg.de/, last access: 21 September 2023; Golden, 2019; Jochum et al., 2007; Lafuente et al., 2015; Lehnert et al., 2000; Spear et al., 2009). As instrumentation improves, high-resolution spatial geochemical data are being continuously produced, and additional efforts are often needed to integrate these new data into the existing databases. Moreover, robust metadata relating to geochemical analyses, such as temporal and spatial information, are not recorded in the same format across publications and studies, but those metadata will increase the value of and return on data science in future research. Further, introducing unambiguous location data, such as detailed categorical locality information combined with specific longitude and latitude coordinates, will increase reliability and standardization. Therefore, a standardized approach to storing data will solve reproducibility issues that stem from a lack of documentation and improper representation. Metadata standards in reporting location and spatial data were adopted from EarthChem as they allow for the seamless integration of metadata from PetDB, GeoRoc, MetPetDB, and GeoReM (Lehnert et al., 2000). Further, there are several efforts underway to produce data standards across the various geochemical and earth science data types, including IUGS/CGI (https://cgi-iugs.org/, last access: 21 September 2023), OneGeochemistry (Lehnert and Wyborn, 2019), OneGeology (Jackson, 2008), and OneStratigraphy (Wang et al., 2021).
Due to limited digital documentation, older publications and data are disappearing from the scientific record to become dark data. According to Hazen et al. (2019), dark data in mineralogy consist of “information on mineral compositions, localities, and other data that are available only through hard-copy publications, proprietary corporate documents (notably companies in the natural resources industry), or privately held research records”. For example, garnet sample analyses published prior to 1990 are recorded in scanned PDFs that are difficult to convert to an Excel spreadsheet by automated means. These sources of data are not easy to manipulate and often disappear from scientific records with time. Thus, a primary purpose of this study is to record dark data in a standardized format that is readily accessible, which prevents both the loss of scientific material and continues to expand the availability of mineralogical data.
Standardization of data within the mineralogical community needs to be firmly established. For example, color characteristic names vary dramatically among projects and are subject to the authors' interpretations. Deer et al. (1982) featured descriptive, yet ambiguous, color labels for samples such as “parrot green”, which is difficult to integrate into a dataset. In some applications, specialized systems of color classification have been proposed. For example, the Gemological Institute of America (GIA) has developed a set of standards with descriptive language as well as virtual codes for characterizing specific gem colors (http://gemologyproject.com/wiki/index.php?title=Color_ grading, last access: 10 October 2020; Web Colors, 2020). In regard to geochemical research, using categorized descriptive terms would allow scientists to convey their data in a more precise and accurate manner. Implementing standardization practices also enables data from disparate sources to be easily accessed for future evaluation or comparison with other databases.
The findable, accessible, interoperable, and reusable (FAIR) initiative, while new within the geological community, has been instrumental in bolstering data preservation throughout the physical sciences (Wilkinson et al., 2016). The FAIR principles for database curation encourage proper data management as well as stewardship across a broad range of disciplines to benefit the entire academic community (Lehnert et al., 2021; Wilkinson et al., 2016; FAIR principles: https://www.force11.org/fairprinciples; last access: 21 September 2023). Currently, EarthChem and MetPetDB are advancing data science in geosciences by providing an open-access repository with rich datasets (Lehnert et al., 2000; Spear et al., 2009).
1.2 Garnets
Garnets were selected for this dataset, owing to their vast informative properties, such as geochemical characteristics, physical attributes, wide range of paragenetic modes, distribution throughout geological time, resistance to weathering, and resilience during diagenetic processes (Alizai et al., 2016; Chen et al., 2015; Čopjaková et al., 2005; Deer et al., 1982; Hazen et al., 2008; Kotková and Harley, 2010; Morton et al., 2004; Yang et al., 2013). This section will summarize some relevant information pertaining to garnets and their applicability for a comprehensive dataset incorporating localities, petrogenesis and paragenesis, and geochemical data.
Garnets are good indicators of formational environments as they contain distinct age, temperature, and pressure information indicative of the protolith chemistry as well as mineral evolution throughout geological time (Baxter et al., 2017; Baxter and Scherer, 2013; Chen et al., 2015; Deer et al., 1982; Hazen et al., 2008; Kotková and Harley, 2010). For instance, the high-pressure garnet majorite (Mg3[MgSi]Si3O12) was formed during the era of planetary accretion (>4.56–4.55 Ga) through impact transformations of pyroxene and, subsequently, through igneous and metamorphic processes in earth's mantle. Grossular (Ca3Al2Si3O12) and andradite (Ca3Fe2Si3O12) emerged from the secondary thermal alteration of chondrites and achondrites, potentially very early in our solar system history (∼4.56 to 4.55 Ga; Fagan et al., 2005; Hazen et al., 2008). Also reported are rare instances of goldmanite (Ca3VSi3O12), eringaite (Ca3Sc2Si3O12), and rubinite (Ca3Ti2Si3O12), occurring in chondrite meteorites (Hazen et al., 2008; Grew et al., 2013; Morrison and Hazen, 2020). Both grossular and andradite are characteristic of carbonate-bearing metamorphic material; however, formation of andradite depends on the availability of Al3+ and Fe3+ during metamorphism (Nesse, 2013). Earth's differentiation, volcanic activity, and plate tectonics gave rise to new garnet species (Hazen et al., 2008). Pyrope (Mg3Al2Si3O12) potentially formed through early volcanic processes on earth's surface from 4.55 to 4.0 Ga (Hazen et al., 2008). Further, pyrope is formed in magnesium-rich, high-grade metamorphic and ultramafic igneous environments and is also commonly found in eclogite and serpentinite (Deer et al., 1982; Nesse, 2013). Almandine (Fe3Al2Si3O12), possibly first formed around 4.4 to 3.3 Ga as it is indicative of felsic igneous environments, occurs in medium- to low-grade metamorphic terrains and is typically found in pegmatites, granite, mica schist, or gneiss (Deer et al., 1982; Nesse, 2013; Zhong et al., 2023). A transition from stagnant-lid tectonics to present-day, active-lid plate tectonics occurred between 4.4–2.5 Ga (Cawood et al., 2022). The appearance of spessartine (Mn3Al2Si3O12), which occurs in uplifted regional metamorphic environments, most likely occurred around 3.6–2.5 Ga during which lateral tectonics initiated and the lithosphere went from variable to uniformly rigid (Hazen et al., 2008; Bauer et al., 2020; Hawkesworth et al., 2020; Cawood et al., 2022). Spessartine and almandine-spessartine varieties are also common in felsic igneous rocks such as granite and pegmatites in addition to manganese-rich metamorphic rocks (Deer et al., 1982; Makrygina and Suvorova, 2011; Nesse, 2013). Uvarovite is rare and occurs in chromite-rich metasomatic or hydrothermal environments (Deer et al., 1982; Farré-de-Pablo et al., 2022; Melcher et al., 1997; Nesse, 2013). The complex story of garnet mineral evolution and diverse formational environments provides an excellent case study to investigate the relationship between paragenetic modes, geochemical data, and location information through natural kind clusters (Boujibar et al., 2021; Hazen, 2019; Hazen and Morrison, 2020, 2021; Hazen et al., 2008, 2020; Morrison and Hazen, 2020, 2021; Nesse, 2013).
In addition to a diverse story of mineral evolution, garnets are often used as geochronometers, geothermometers, and geobarometers (Baxter et al., 2017). Similar to zircons, garnets are effective in establishing the chronology of geological events by using radiogenic parent / child isotopic ratios, such as Sm / Nd, U / Pb, and Rb / Sr (Baxter and Scherer, 2013; Kotková and Harley, 2010). Garnet phase equilibria and mineral–mineral element exchange reactions also provide thermometric and thermobarometric information for a wide range of rock types including during regional metamorphism in crustal protoliths (Baxter and Scherer, 2013; Chen et al., 2015) and in mafic and ultramafic mantle rocks (Nickel and Green, 1985; Nimis and Grutter, 2010; Wu and Zhao, 2011). The majorite content of garnet inclusions provide the only reliable information on the depth of formation in sublithospheric diamonds (Thomson et al., 2021). Garnets often undergo crystal rotation, complex zonation, and deformation, which can be used to distinguish specific grain kinematic histories and shearing planes in metamorphic rocks (Rosenfeld, 1970; Spear and Daniel, 2001; Whitney and Seaton, 2010).
In nature, garnets close to ideal end-member compositions are rare. Therefore, natural samples are often expressed as percentages of several idealized end-members calculated from the major oxides or oxygen cation ratios (Deer et al., 1982; Geiger, 2016; Grew et al., 2013; Nesse, 2013). According to the list of approved mineral species from the International Mineralogical Association's (IMA's) Commission on New Minerals, Nomenclature and Classification (CNMNC) (https://rruff.info/ima/; last access: 5 October 2020), the garnet supergroup contains 37 structural garnet species, while the silicate garnet group consists of 6 major end-member species and 14 minor species classified by their idealized chemical formula: X3Y2Si3O12 (Deer et al., 1982; Grew et al., 2013). The two main garnet series are pyralspite and ugrandite, both of which form continuous solid–solution series (Deer et al., 1982; Nesse, 2013). Pyralspite consists of pyrope, almandine, and spessartine which require aluminum in the Y site, while ugrandite includes uvarovite, grossular, and andradite which requires calcium in the X site (Deer et al., 1982; Nesse, 2013). Historically, it was thought that a miscibility gap exists between the pyralspite and ugrandite series; however, it is now known that uncommon intermediate compositions between the two series exist (Deer et al., 1982; Geiger, 2016; Nesse, 2013). Additionally, there is some contention about whether these series should be used as they exclude high-pressure garnet species, such as majorite, which are prevalent in the transition zone of the mantle (Geiger, 2016).
The detailed garnet solid–solution series from major oxides (SiO2, TiO2, MgO, MnO, FeO, Fe2O3, Al2O3, CaO, Cr2O3, NiO, K2O) are classified based on several rules regarding chemical composition. However, the goal of understanding the evolutionary system of garnet group minerals requires a paragenetic context for mineral classification – one that is based on each specimen's formational conditions, as well as its composition. Recognizing distinct types of garnets thus requires natural kind clustering, which relies on the complex, multivariate correlations among all of the major, minor, and trace element constituents of garnet samples to determine their paragenetic relationships (Hazen et al., 2019; Morrison and Hazen, 2020). To that end, we initiated this study to establish an extensive, reliable, open-access data resource of garnet sample analyses across a multitude of resources for data pertaining to geochemistry, localities, and petrogenetic and paragenetic modes.
We compiled a dataset of 95 650 garnet analyses across a total of 186 attributes (https://doi.org/10.48484/camh-xy98, Chiama et al., 2022). The dataset includes 61 294 analyses from EarthChem (https://doi.org/10.26022/IEDA/112171, Chiama et al., 2021b; 64 from the North American Volcanic and Intrusive Rock Database (NAVDAT), 47 591 from GeoRoc, and 13 639 from PetDB), 12 781 from Chassé et al. (2018), 10 380 almandine point analyses from the supplementary data in Gatewood et al. (2015), 6787 samples from MetPetDB (https://doi.org/10.26022/IEDA/112173, Chiama et al., 2021a), 4162 assorted samples from peer-reviewed literature and other datasets such as the RRUFF project, and finally 246 original electron microprobe analyses (EMPAs). All of the samples compiled were collected from English-language literature and repositories. Peer-reviewed literature was compiled in Zotero, and sample analyses were converted from PDF documents to Excel using Tabula (https://tabula.technology/; last access: 27 September 2020) or by manual entry, depending on the quality of the PDF. This section will examine the methods and assumptions behind the formation of the dataset as well as the methods employed to analyze nine original garnet samples.
2.1 Dataset formation
The primary attributes incorporated in the dataset include locality information, petrogenesis and paragenesis, and major oxides. Secondary attributes include the sample age, temperature, pressure, trace elements (e.g., REEs), and isotopes when provided by the source material. Each of the attributes are identified in a detailed system while maintaining the ability to cluster and identify patterns within the dataset. A data schema is included in Table 1 to define each of the attributes in order of appearance in the dataset.
Data were compiled from multiple resources to create this dataset. The data were extracted from the EarthChem Portal database, which provides a central access point to mineral composition data from PetDB, GeoRoc, and NAVDAT by querying for all garnet analyses available (“analyzed material” = “garnet”) and retrieving all available variables (date downloaded: 13 August 2019). Data from MetPetDB were compiled from a search for chemical analyses of garnet and a search for samples that contain garnet. The two searches were then cross-correlated by the original sample ID so that each garnet analysis could be annotated with location, rock type, and other metadata (date downloaded: 24 December 2020). Majorite samples are from the compilation of Walter et al. (2022). All other samples were compiled by undertaking a literature review of garnet sample analyses which provided geochemical data, geologic formation environment, and/or location information. The data from the data repositories and literature were standardized for common attributes to form the structure of this dataset.
We created an identification system to maintain as much information as possible from original sources and additional references. Each sample was given a unique “Project ID” which is indicated by a line number to identify the total number of samples examined. The “Individual Project ID” indicates where the major data repositories' sample information originated from (i.e., EarthChem employs a line number followed by EC_GARNET) or the initials of the author who compiled the samples from peer-reviewed literature. Multiple sources did not provide the International Generic Sample Number (IGSN, 2020); however, the original EMPA garnet sample analyses performed in this study were assigned IGSNs. The “Origin ID” attribute was created to label sample analyses based on their respective original sample identification.
A detailed reference section was embedded in the dataset for future researchers to quickly locate the original source of samples. This section was split into three separate attributes: “Title”, “Journal”, and “Reference”. The “Reference” attribute lists the authors and year of publication while maintaining the formatting for the samples originating from the EarthChem and MetPetDB repositories. The “Title” and “Journal” attributes were adopted to prevent confusion because some authors published multiple papers on garnet samples in the same year; for example, Chassé et al. (2018) reported samples from Griffin et al. (1999a and b). This multi-attribute reference and identification system was adopted to quickly identify any additional information regarding specific samples not already included in the dataset. Reference formats from EarthChem and MetPetDB were maintained to simplify cross-referencing.
2.1.1 Mineral species
Regarding the IMA classification of garnet species, there are 37 minerals within the garnet structural group, 14 garnets within the silicate group, and 6 common end-member species (https://rruff.info/ima/; last access: 5 October 2020). As it is not within the scope of this paper to apply the IMA classification of composition for each sample, we simply assigned a dominant garnet species name if one was reported. Often, many literature sources and data repositories (EarthChem and MetPetDB) will not classify a garnet sample by a specific species as garnets are typically chemically zoned. We indicated all unidentified samples as “Garnet” which dominates the dataset (82 558 analyses). Samples reported as a combination of end-members were listed as both (i.e., “Almandine-Spessartine”; Yang et al., 2013). There are a total of 39 possible variations of mineral species in the database (including the unknown “Garnet” flag) defined by 6 end-members, 6 silicate group garnets, 21 different combinations of end-members, and 4 structural garnet species (bitikleite, elbrusite, henritermierite, and toturite). When an additional varietal species or minor species was provided in the literature, it was recorded in the “Varietal Name” attribute (i.e., “Chromian Andradite” or “Titanian Melanite”; Deer et al., 1982; Ghosh and Morishita, 2011). Further, hydrated garnets were denoted with a “1”, while unhydrated garnets are represented with “0” in the “Hydrated Garnet” attribute. It is important to note that we recorded samples as hydrous only when samples were denoted as such in the literature.
2.1.2 Zonation
Garnets are often highly chemically zoned throughout each grain, and the zonation can be used to understand the changing environmental conditions, such as temperature and pressure, over time (Javanmard et al., 2018; Yang et al., 2013). Although there is debate about the complexity and style of zonation within garnet samples, it is not within the scope of this paper to address zonation in detail. This section will address different types of zonation leading to a discussion about how to use the “Zone” attribute in the dataset.
Classically, zonation for garnets is measured concentrically from the core to rim of the grain (Javanmard et al., 2018; Yang et al., 2013). Polycrystalline garnets, though less common, can record the changing mechanisms and chemical conditions by combining 2 to 30 plus crystallites within one garnet grain (Whitney and Seaton, 2010). The major divalent cations in garnets (Fe, Mg, Mn, and Ca) can feature different styles of zonation within individual polycrystals (Spear and Daniel, 2001; Whitney and Seaton, 2010). This style of zonation leads to classification issues in a dataset format, such as identifying specific styles of zonation across multiple studies and classifying them with limited information. For example, polycrystalline zonation is identified by polycrystal number, while concentric zonation is classically identified by zone number originating from the core and increasing in numerical value towards the rim (Whitney and Seaton, 2010).
We intended to maintain as much information as possible about the individual samples without over-complicating the dataset through the zonation classification process. Yet, many authors and databases did not report zonation or only reported core, middle, and rim of each grain and did not interpret polycrystalline zonation. Therefore, while zonation is crucial to identifying the mechanisms and paragenetic conditions of garnet formation, we cannot identify polycrystalline or complex zonation from limited data. Ultimately, the “Zone” of each sample analysis was classified simply by the core (c), middle (m), and rim (r) of each grain. For samples that were unclear or did not report zonation, this field was intentionally left blank. Ideally, a standardized system of zonation representation should be adopted to limit the subjectivity and interpretation of zones. The clarity would have allowed us to adopt a dual-attribute system identifying the style of zonation (e.g., concentric, polycrystalline) in one attribute for each point analysis and the polycrystal or concentric zone number in a second attribute. This system would provide an in-depth analysis of compositional evolution across complex zonation styles.
2.1.3 Locality
Locality information from the literature and repositories varies dramatically in specificity. In order to maintain continuity, the location information was classified into four categories: Continent, Country, Area, and Geological Context. In the cases where a country or regional area has politically dissolved, the original published nomenclature for each sample was maintained in either the “Location” or “Country” attribute to prevent confusion over historical borders. For example, Deer et al. (1982) references former countries such as the USSR and Czechoslovakia. The three extraterrestrial samples are recorded by the location they were discovered (Continent, Country, and Area) and are designated as extraterrestrial material in the petrogenetic attributes. The regional “Area” encompasses provinces, states, districts, counties, and cities, while the attribute “Geological Context” focuses more specifically on the geological location information such as metamorphic terranes, kimberlite fields, and mining sites. Some sources provided a further in-depth description or information that did not fit into these designated categories (Deer et al., 1982). To prevent oversimplification, any additional information was denoted in the “Location” attribute. Latitude and longitude were converted from degrees, minutes, and seconds to decimal degrees for ease of use.
2.1.4 Petrogenetic attributes
The categorization of geological and mineralogical formation environments was a key component in the formation of this dataset. We define petrogenesis as the origin and formational conditions of the host rock and paragenesis as a characteristic rock-type name associated with the origin and formation conditions of minerals based on definitions obtained from Mindat.org (https://www.mindat.org/; last access: 30 December 2020). Because petrogenesis and paragenesis are reported differently between studies, a standardized system was required to adequately categorize this information in a dataset format. Due to a large percentage of the garnet samples originating from EarthChem (61 294 out of 95 650) and in an effort to maintain data continuity, we adopted their petrographic classification. All of the sample analyses were identified by a series of petrogenetic attributes such as the following: a detailed geologic “Formation” environment, general parent “Material”, “Type” and “Composition” of parent material, and finally a general “Paragenesis”. These attributes were chosen such that petrogenetic and paragenetic clusters can be examined with different degrees of resolution. The goal of the petrogenetic attribute classification system was to organize data for resolution-dependent cluster analysis.
The detailed “Formation environment” is different for nearly every sample as it was extracted verbatim from the peer-reviewed literature; thus, this attribute has the highest resolution. In contrast, the “Material” attribute offers the lowest resolution as it was simplified to detrital, igneous, metamorphic, extraterrestrial, metasomatic, and unknown material from which the samples originated. “Type” describes the type of material from which samples originated. For example, the type of igneous material was identified to be volcanic or plutonic, whereas the type of metamorphic material examined metamorphic facies such as amphibolite, greenschist, and eclogite facies. The “Composition” focused on the dominant mineral assemblages primarily related to igneous and metasomatic materials, such as felsic, mafic, ultramafic, carbonate, and calc-silicate. Therefore, the “Composition” attribute was simplified to represent information that can be identified across most peer-reviewed literature. Because not all studies reported specific mineral assemblages, it is not within the scope of this paper to assign and classify the associated minerals by locality. Regarding the “Paragenesis” attribute, a majority of previous publications classify paragenesis as a detailed mineral formation process which does not translate to a dataset format that can be clustered. Thus, the attribute “Paragenesis” was simplified to the rock-type name; a one- or two-word term that adequately represents the sample. Rock-type definitions and classifications were taken verbatim from the literature as well as Mindat.org as it is a well-accepted resource for mineralogy (https://www.mindat.org/; last access: 30 December 2020).
This petrogenetic attribute reporting system offers the opportunity for resolution-dependent cluster analysis. Material is the lowest resolution attribute containing only six categories, while “Paragenesis” is the highest resolution attribute representing 161 different paragenetic modes. We recommend examining each of the petrogenetic attributes collectively as well as individually to best characterize the data with cluster analysis. It should also be noted that how each of the attributes are classified remains a subject of debate as they are highly subjective and vary over time and between authors. For example, the distinction between igneous and metamorphic rocks can be arbitrary when various mantle processes at various depths can be responsible for a specific rock's mineralogy and texture.
2.1.5 Age, pressure, and temperature
Samples that reported age (Ma), pressure (kbar), and/or temperature (∘C) of formation were recorded in the dataset, including uncertainty, when provided. Each of these parameters included attribute columns with standardized units for the minimum, average, and maximum value. Despite garnets being excellent environmental indicators, few sources reported a specific formation temperature, pressure, or age for individual sample analyses. Rather than directly analyzing the garnet grains, most studies and datasets (i.e., EarthChem) conflate the age, pressure, and temperature of parageneses with those of the garnet grain. Additionally, due to the complexity of many natural systems, which tend to not experience a singular unaltered event, some studies had inconclusive age, temperature, and pressure results. The term “age” is a matter of interpretation as various geologic processes can be dated such as crystallization age, metamorphic age, and cooling age, and the different studies within the dataset used the term in the context of their studies' focus. Therefore, when using the age, pressure, and temperature data in this dataset it is recommended to reference the context of each analysis used. These sample ages were not further modified within the dataset as our goal was to preserve the raw data. Sources that reported detailed age information often reported average values without uncertainty or employed unclear terminology. For example, Parthasarathy et al. (1999) reported ages in terms of epochs or periods which were instead denoted as maximum and minimum dates to maintain consistency in the dataset.
2.1.6 Geochemical data
A major component of the dataset consists of geochemical information for major oxides and trace elements which account for 129 attributes of the total 186 represented. Major oxides were recorded in weight percent (wt %), whereas trace elements were recorded in parts per million (ppm) to maintain consistency. Generally, older publications reported major oxides to cation numbers based on 24, 12, or 8 oxygen atoms and/or mole percent end-member species (Deer et al., 1982). We chose to exclude the oxygen cation data and end-member calculations from this dataset as both can be calculated from the major oxides. Additionally, a few sources provided information on isotopes which were included in the dataset. As some sources did not have a field for the sum of the total oxides, we added an attribute named “Our Calc (wt %)” which is a summation of all the major oxides to address this issue. This attribute helps identify problematic samples with an abnormally high or low total wt %, which could be misrepresented due to a typographical error, miscalculation, or experimental error.
Additionally, during the acquisition of data, many dark data sources could not be automatically converted to Excel spreadsheets; therefore, the data were entered manually. Data from Deer et al. (1982) were poorly converted in Tabula (https://tabula.technology/; last access: 27 September 2020) with decimal places replaced by multiplication symbols or values transposed throughout the resulting spreadsheet. Manual entry aimed to prevent data corruption, but this also introduced the opportunity for typographical errors. Data entered manually were double-checked for errors using the “Our Calc (wt %)” column as a summation of the major oxides.
2.1.7 Iron
Iron can be found in garnets as Fe2+ in the X site of the mineral structure, Fe3+ in the Y site, or in both depending on the garnet species (Deer et al., 1982; Nesse, 2013). However, without applying the flank method (Höfer et al., 2000), EMPAs cannot measure the two valences concurrently (Droop, 1987). Instead, most authors assumed all iron to be one chosen valence, resulting in it being recorded as either FeOT (total) when it was all calculated as Fe2+ or Fe2O3T (total) when all the iron was calculated as Fe3+. Very few studies conducted post-EMPA calculations in order to find both iron oxides for their samples. Additionally, many of the databases presented their iron data in a way that made it unclear if this calculation was performed as they labeled all their analyses as one of the iron oxides yet did not mention the other (Chassé et al., 2018; Gatewood et al., 2015; MetPetDB). As a result, we included four separate columns for iron: “FeO”, “FeOT”, “Fe2O3”, and “Fe2O3T”. However, it was difficult to compare garnets across four attributes for two iron oxides (FeO and Fe2O3).
In order to evaluate our original EMPA samples, we utilized a spreadsheet created by Locock (Andrew J. Locock, personal communication, 2020), based on the work of Droop (1987), to calculate both FeO and Fe2O3 from FeOT. The spreadsheet applies the ideal cation : oxygen ratio of garnets (8:12) and the major oxide results (including FeO) to estimate FeO wt %, Fe2O3 wt %, a new analysis total, and the added amount of oxygen from the presence of Fe3+ (which is included in the “Notes” column of the dataset). This spreadsheet was not applied to the entire dataset for a couple of reasons. First, many of the analyses did not include finite values and reported the concentration as below the detection limit using “<” or one of several abbreviations for absent or non-detected oxides and trace elements. The spreadsheet cannot interpret these abbreviations; therefore, they had to be removed. One approach to make these data readable by the spreadsheet would be to replace these abbreviations with absolute values; however, this would misrepresent the true values of the data and potentially bias the results. This concept is further described in Sect. 2.1.12. Secondly, the calculation is not suitable for hydrogarnets, which have variable numbers of oxygen atoms per anhydrous formula unit (Droop, 1987). Thus, the recalculation was only applied to the original EMPA analyses performed in this study.
2.1.8 End-member classification and quality index
Since 82 558 of the 95 650 total sample analyses are simply labeled as garnet and mainly originate from the EarthChem repository, an additional 16 attributes were added to the dataset in order to further classify them while preserving the original mineral identifier. This was done by utilizing a combination of the Grew et al. (2013) and Locock (2008) spreadsheets designed to guide the determination of species. The columns “Group”, “Species”, “Hypothetical End-Member”, and “Check Data” originate from the Grew et al. (2013) spreadsheet, while the remaining columns (“Analytical Total”, “Proportions Dodecahedral”, “Proportions Octahedral”, “Proportions Tetrahedral”, “Oct Si”, “Charge Balance”, “Analytical Total Check”, “Proportions Check”, “Oct Si Check”, “Charge Balance Check”, “Subtotal”, “Quality Index”) originate from the Locock (2008) spreadsheet. The “Group” column divides the garnet supergroup into six groups (henritermierite, bitikleite, schorlomite, garnet, berzeliite, and ungrouped) based on symmetry and the total charge at the tetrahedral site. The “Species” and “Hypothetical End-Member” columns classify the analyses into 32 IMA-approved garnet species and 16 end-members, respectively, based on the principal cations present within the charge-balanced formula, with the latter column utilized in the few cases where an approved species is not found for an analysis. If no result is returned for these two columns, then an appeal to check the data will be recorded in the “Check Data” column. The remaining 12 additional columns make up the “Quality Index” created and employed by Locock (2008). It considers the “Analytical Total”, the deviation in the ideal cation proportions (“Proportions Dodecahedral”, “Proportions Octahedral”, “Proportions Tetrahedral”), the presence of unnecessary octahedral Si (“Oct Si”), and the “Charge Balance” of each analysis. Identical to the original Locock (2008) spreadsheet, a point is added to each column (i.e., “Analytical Total Check”, “Proportions Check”, “Oct Si Check”, “Charge Balance Check”) that is not ideal. For example, if the “Analytical Total” is not within 97 %–101 %, a point is added. For more information on each component of the Quality Index calculation, refer to Table 1 or Locock (2008). The “Subtotal” column sums the points allotted, and the “Quality Index” columns reports whether the analysis is superior (0 points), excellent (1 point), good (2 points), fair (3 points), or poor (4 points). If an analysis returns a poor or fair classification, then the data and/or presence of possible analytical difficulties should be studied. For the 17 973 analyses that reported no major oxide data but only trace element data, these 16 columns were left blank as the calculation could not be done. Analyses with greater than one major oxide recorded were input into the end-member and quality index spreadsheet; however, we caution the validity of the results of these data as the Locock (2008) and Grew et al. (2013) spreadsheets were not designed to work with such limited raw data. Some analyses, including those with only one major oxide, would return no results; in these cases, we recorded N/A in the “Group”, “Species”, and “Quality Index” columns and an appeal to check the data in the “Check Data” column.
2.1.9 Duplicate samples
Because garnet data were derived from individual studies as well as databases, there was a potential for overlap. Repeated samples were identified by their “Origin ID”, original references, and identical geochemical information. Only 7.57 % of samples (7240 total) are repeated in the overall dataset. The major sources of sample overlap occur with Chassé et al. (2018) and EarthChem. The major difference between these sources is that Chassé et al. (2018) reported categorical location information, whereas EarthChem provided only longitude and latitude. To maintain relevant information, the attribute “Repeat” was created to list the first iteration of samples as “0” and the second iteration of samples, or duplicates, as “1” such that samples marked by “1” are excluded from further analysis.
2.1.10 Color
Color classification is ambiguous because color definitions are subjective between different authors. Color was the most diverse descriptor of all attributes within our dataset. For example, Deer et al. (1982) reported color in a plethora of different designations such as “Dark Peach-Tan” or “Hyacinth Red”. The method used to standardize the “Color” column into a cluster-able format was adopted from GIA's (Gemological Institute of America's) color grading system, specifically The Gemology Project (http://gemologyproject.com/wiki/index.php?title=Color_grading; last access: 10 October 2020). This system assigns abbreviations to hues and employs numbers to indicate the strength of the tone and saturation for the colors. When saturation or tone were not given as descriptive labels, neutral values were chosen to represent the sample. Typical notation for the sample is indicated as “hue tone/saturation”. For example, “bright green” would be “slyG 5/6”. However, for this dataset, each of the three descriptors were separated into individual columns. Because color descriptions are open to interpretation, adapting them to the GIA format without access to the specimens introduces significant room for error. Establishing a universal or standardized color code would be beneficial for conveying exact colors in a non-visual format. We propose a more specific method of characterizing and defining color through virtual color codes, such as hex, HTML, CMYK color codes or HSL (hue, saturation, lightness) or RGB values (https://htmlcolorcodes.com/; last access: 10 October 2020). Virtual color codes are an internationally recognized and accessible format for color grading to limit ambiguity and interpretation error. In our circumstance, we did not have access to the original samples and thus could not identify colors with specific labels.
2.1.11 Notes
The “Notes” column is dedicated to any important sample information that is not regularly reported in established databases or peer-reviewed literature. For example, the presence of birefringence, inclusions, twinning, crystal shape, and original color designations are noted for the respective sample when provided. Additionally, the original references are recorded in this section if a larger, more encompassing, paper or database was the main reference cited. For example, Deer et al. (1982) is a compilation of sources, so references to the original literature were listed in our “Notes” column. This approach is also employed by Chassé et al. (2018) and EarthChem, which contain samples compiled across multiple sources and indicate the original authors.
2.1.12 Analysis method and minimum detection limit
Information about instrumentation used in geochemical analyses of garnet samples was recorded in order to avoid interlaboratory biases generated by systematic differences between various equipment (Hazen, 2014). Due to the range in analytical methods, certain terms were used for absent or non-detected oxides and trace elements. The terms found in literature include the following: below detection limit (bdl, b.d.l.), not detected (nd, n.d., nd., n. d.), not applicable/analyzed (na, n.a.), no value (–, . , nil), trace (tr, t.r., tr.), and “<[VALUE]”. Terms were standardized (e.g., from “b.d.l.” to “bdl”) to maintain consistency in the dataset. Standardized terms in the dataset include below detection limit (bdl), not detected or not applicable (na), trace (tr), and “<[VALUE]”. Because each one of these abbreviations has a separate definition, we did not significantly alter these terms to prevent misrepresenting the data. For example, “bdl” could not be replaced with a zero or removed, as it does not explicitly say the oxide or element was not found but simply that it was below the detection limit. Trace values were treated similarly, as standardization of these abbreviations would also not be conducive to representing information from the original sources accurately.
Other concerns included the minimum detection limit for each analysis method. Initially, we examined the minimum detection limit, which ranged in numerical value and varied dramatically among the instrumentation used and the year when various studies were conducted. This information was not included as it could not be standardized nor applied to the entire dataset without altering or potentially skewing the dataset to a particular value.
2.2 Electron microprobe analyses
In addition to samples compiled in the dataset, major elements from nine garnet samples (almandine, andradite, two samples of grossular, spessartine, uvarovite, and three unknown samples of garnet) donated by George Mason University were measured using a JEOL JXA-8530F field emission electron microprobe (EMPA) at the Carnegie Institution for Science's Earth and Planets Laboratory in Washington, DC. The microprobe was standardized using albite, TiO2, MgCr2O4, orthoclase, spessartine-almandine, pyrope-almandine, and augite. The acceleration voltage was 15 kV with a probe current of 20 nA and a 5 µm diameter beam. Samples were analyzed for their concentration of Na, Si, Ti, Ca, Mg, Al, Cr, K, Fe, and Mn and were reported in their oxide form in the dataset. Oxygen was determined by stoichiometry. Each point analysis is identified with an IGSN in the dataset. Additionally, the “Origin ID” for each analysis was provided to help delineate zonation identified in the samples. Specifically, we identified inclusions within two samples (uvarovite and almandine) that potentially exhibit complex rather than concentric zonation. The individual sample IDs employ A, B, and C to denote the different regions/inclusions measured in these point analyses. However, to maintain consistency with the rest of the dataset, the “Zone” attribute identifies the location of point analyses in the core, middle, and rim of the grain, while inclusion information was classified in the “Notes” attribute. A total of 275 point analyses were performed with a minimum of 25 points for each sample. In the case of uvarovite, which exhibited concentric zonation visible to the naked eye, an additional 24 point analyses were performed in a linear path from the core to the rim of the grain to confirm the complexity of zonation. The 29 point analyses that exhibited visible inclusions and had geochemical data indicative of minerals other than garnet were excluded from the dataset. A detailed evaluation of the 246 point analyses included in the dataset is in the Supplement Sect. A, and a summary of the average major oxide concentrations is in the Supplement Sect B.
The analysis of our dataset examines the representation of mineral species, classification of garnet end-members, locality information, and petrogenetic attributes while considering the possibility for errors or bias. The purpose is to visualize the compiled data through single attribute-based diagrams. The mineral species, locality information, and petrogenesis results may be biased due to the sources of compiled data. Additionally, all analyses were categorized into their likely garnet group and subspecies, and their quality was assessed based on the end-member and quality index spreadsheet based on the work from Grew et al. (2013) and Locock (2008).
3.1 Mineral species
This dataset includes the IMA nomenclature to identify the dominant “Mineral” species for sample analyses. There are 37 IMA-recognized structural garnet species and 14 silicate garnets; however, there are 32 categories of mineral names within the dataset which include the combination of end-members such as “Almandine-Grossular” and “Almandine-Pyrope” for samples near 50 / 50 in composition as well as the simplified term “Garnet” for unidentified samples. For samples that reported a near 50 / 50 composition, we standardized the naming convention to one category. For example, sample analyses that reported “Pyrope-Almandine” are included in “Almandine-Pyrope” for simplicity.
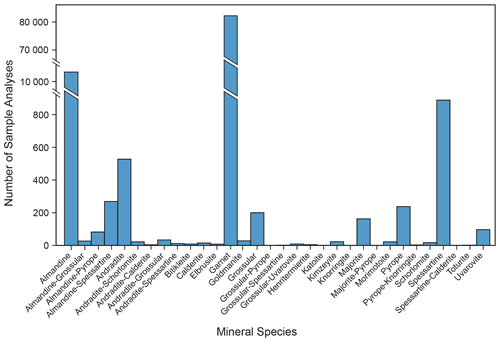
Figure 1Representation of all the sample analyses across the 32 different “Mineral” categories including garnet end-members, end-member combinations, silicate garnets, and structural garnets present in the dataset. There are two breaks in the scale to include 10 681 Almandine and 82 256 general garnet sample analyses without obscuring the distribution of other categories present. There are 889 spessartine, 528 andradite, and 267 almandine-spessartine analyses as well as 1029 analyses accommodated by the remaining 27 categories.
The representation of 32 different variations of mineral species in the dataset was plotted by counts of unique categories with two breaks in the scale to prevent the large number of almandine and general garnet samples from obscuring the distribution of the other species present (Fig. 1). Of the 95 650 total sample analyses in the dataset, 82 256 are categorized as general garnet, while 13 394 contain more specific silicate and structural garnet species or end-member combination names. The 82 256 unidentified “Garnet” samples originate from 61 294 EarthChem samples, 12 781 samples from Chassé et al. (2018), 6787 from MetPetDB, and other compiled peer-reviewed literature which did not provide specific garnet species names due to the common chemical zonation of garnets. There are 10 681 samples categorized as almandine, of which 10 380 analyses are from 10 garnet grains described as “dominantly almandine (XFe=0.52–0.78), with subordinate amounts of pyrope (XMg=0.03–0.12), spessartine (XMn=0.00–0.25), and grossular (XCa=0.12–0.21)” by Gatewood et al. (2015). These samples were grouped as general almandine because the primary focus of the dataset was to report raw data not to further examine the IMA mineral classifications. The remaining 2713 sample analyses in the dataset consist of 889 spessartine, 528 andradite, 269 almandine-spessartine, and 1027 analyses distributed across 27 other silicate and structural garnets as well as end-member name combinations (Fig. 1). While this distribution is not representative of garnet species in nature, it is significant for the dataset to include as many garnet sample analyses as possible. It is important to note that the majority of sample analyses are tabulated under the general “Garnet” flag and originate from the EarthChem repository.
3.2 End-member classification and quality index
In addition to recording the reported mineral species classification from the literature and respective data repositories, we classified the garnet sample analyses by their end-members based on their major oxide composition. It is important to keep in mind during the following discussion of the end-member classification and quality index that the original purpose of the Grew et al. (2013) and Locock (2008) spreadsheets was to help guide the determination of the garnet species. The cation assignments to each site in these spreadsheets are rigid, following a strict sequence, and may not be in accord with actual experimental determinations (Andrew J. Locock, personal communication, 2023). This is observable in the 3110 samples whose literature name does not match the name provided by the “End-member Classification and Quality Index” spreadsheet. This number includes analyses assigned N/A (1186) and ungrouped (287) by the spreadsheet. Some papers classify the garnets as a combination of end-members (i.e., “Almandine-Spessartine”; Yang et al., 2013); in these instances, as long as one of these end-members is reported as the dominant species according to the “End-member Classification and Quality Index” spreadsheet, then we counted the names as matching in the above count. According to the spreadsheet, the dominant mineral group represented in the dataset is garnet with 76 051 analyses, followed by 125 schorlomite, 20 bitikleite, 5 henritermierite, and 2 berzeliite. In Fig. 2a, the largest garnet species represented is pyrope with 47 994 analyses, of which 37 135 are from EarthChem and 9392 are from Chassé et al. (2018). There are 21 145 samples classified as almandine, a little less than half of which (9753) are from the 10 garnet grains analyzed by Gatewood et al. (2015) (Fig. 2a). The remaining major species represented include the following: 2565 majorite, 1131 andradite, and 832 grossular (Fig. 2a). There were 469 analyses where an approved species within the spreadsheet was not found and a hypothetical end-member was assigned instead; these included 381{ Mg3} [Fe2](Si3)O12, 65{Ca3} [TiMg](Si3)O12, 12{ Ca3} [Ti2](SiAl2)O12, 8{ Fe3} [Fe2](Si3)O12, and 3{ Na2Ca} [Ti2](Si3)O12. The end-member classification and quality index were unable to assign a group or species to 287 samples. These ungrouped samples originate from the following: 153 from Chassé et al. (2018), 106 from EarthChem, 16 from MetPetDB, 9 from Gatewood et al. (2015), and 3 other compiled peer-reviewed literature. A majority of these ungrouped samples (205) report little to no SiO2 and mostly appear to be rich in titanium and iron, indicating they may represent iron-rich ilmenite inclusions, while some are rich in chromium, indicating they may be chromite inclusions. These samples were not removed from the dataset as one of the main goals of this project was to maintain data continuity; however, these 16 end-member classification and quality index columns were added to aid in identifying low-quality data. It is not a standalone solution as the Grew et al. (2013) and Locock (2008) spreadsheets were not designed to determine whether an analysis is or is not a garnet; therefore, it is unlikely to label all inclusion analyses as ungrouped, especially if the inclusion is a silicate mineral. Based on the quality index calculation, 52.5 % of our samples (not including samples that had no major oxide data, were ungrouped, or N/A) were rated as excellent, 16.5 % as superior, 20.9 % as good, 5.7 % as fair, and 4.2 % as poor, as shown in Fig. 2b. Only 1186 samples, not including those labeled N/A, have requests for the data to be checked.
3.3 Locality information
Locality information within the dataset consists of six attributes of increasing resolution: Continent, Country, Area, Geological Context, Latitude, and Longitude. Of the total 95 650 sample analyses in the dataset, up to 33 313 report some form of categorical location information (continent, country, area, or geological context), and 67 846 report numerical data (longitude and latitude), while only 7972 report both categorical and numerical location data. All sources provided either categorical or numerical location information except for Locock (2008), which did not contain location data. Thus, a dual system of categorical and numerical location data was created to best represent the entire distribution of sample localities.
There are 33 313 sample analyses that report an origin from one of the seven continents and 32 837 analyses which indicate a specific country of origin. There are 702 unique regional areas represented by 29 077 sample analyses and 396 unique geological contexts for 30 697 sample analyses. The regional area and the geological context attributes include specific locality information as descriptive as “60 km NW of Kimberley, Cape Province,” and “Markt Kimberlite, Subcontinental lithospheric mantle, Rehoboth Subprovince”, respectively, to increase reproducibility and availability of data (Chassé et al., 2018; Deer et al., 1982). Further, the three analyses with an extraterrestrial origin can be identified by the “Material” attribute and are listed by the continent and country in which they were discovered. The remaining analyses in the dataset (62 337 continent, 62 813 country, 66 573 area, and 64 953 geological context) did not report location information and are designated as unknown. The distribution of samples from each continent and country were plotted by counts of unique categories (Figs. 2 and 3). The regional area and geological context attributes were not plotted due to the vast quantity of unique categories. The 67 846 samples that report latitude and longitude were plotted to visualize the global distribution of samples in the dataset which represent 1691 unique locations (Fig. 4). Ocean floor samples were not represented in the categorical location data; however, they can be identified in the map of samples by longitude and latitude (Fig. 4). The majority of the unknown samples pertaining to categorical localities consist of ∼99 % of the 61 294 analyses donated from the EarthChem repository; however, these data points report precise latitude and longitude for every analysis instead.
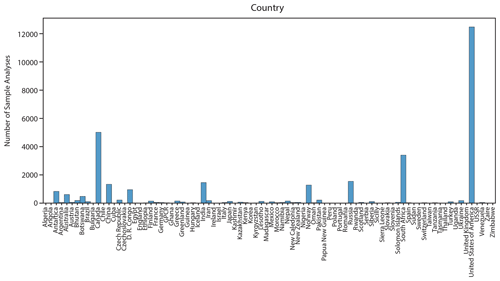
Figure 4Representation of all sample analyses across different countries. There are sample analyses from 88 total countries represented in the dataset. The most prominent sample localities are 5019 sample analyses from Canada, 1426 from India, 1288 from Norway, 1544 from Russia, 3403 from South Africa, and 12 489 from the United States of America. There are 62 836 samples which do not indicate a country of origin and are listed as unknown. Along the x axis, D.R. Congo indicates the Democratic Republic of the Congo; GPCR is an abbreviation for sample analyses that originated from a combined location listed as Germany, Poland, and the Czech Republic; and the USSR indicates samples originating from within the historic borders of the Soviet Union.
The distribution of samples from different continents and countries is depicted in Figs. 3 and 4. The highest concentration of garnet analyses is located in North America with 17 692 samples, followed by Asia with 6028 samples, Africa with 5266 samples, and Europe with 2476 samples (Fig. 2). The dataset contains 87 different countries of origin for garnet samples (Fig. 3). The most prominent sample countries are Canada (5019 sample analyses), Russia (1547), South Africa (3403), and the United States of America (12 479). There are 62 813 samples which do not indicate a country of origin and are listed as “Unknown”. It is important to note that of the 12 479 samples from the United States, 10 380 are sample analyses from Townshend Dam, Vermont (Gatewood et al., 2015), which introduces a significant bias in the dataset. It was not our intention to represent the overall natural occurrence of garnets but rather to record the data found in the literature and list locations for samples when they were provided.
Despite the bias towards the United States from the categorical data, there is a diverse distribution of samples around the world based on the map of longitude and latitude in Fig. 5. There are 1691 unique locations represented by 67 846 samples (Fig. 5). Samples originate from every major continent as well as Greenland, Iceland, New Zealand, and a handful of Pacific islands. These samples primarily originate from the EarthChem and MetPetDB repositories; however, some of the compiled peer-reviewed literature label specific longitude and latitude for each analysis, which are also included in this map (Alizai et al., 2016; Ghosh et al., 2017; Inglis et al., 2017; Javanmard et al., 2018; Kotková and Harley, 2010; Korinevsky, 2015; Krippner et al., 2016; Manton et al., 2017; Parthasarathy et al., 1999; Patranabis-Deb et al., 2009; Schönig et al., 2018; Sieck et al., 2019; Suwa et al., 1996). Thus, despite the compilation of samples from solely English literature and repositories and the bias of samples from North America, the distribution of sample localities around the world is diverse based on the reported longitude and latitude data. The distribution of sample analyses based on longitude and latitude captures the natural occurrence of garnets better than the categorical data.
3.4 Petrogenetic attributes
The petrogenetic attributes were chosen with increasing resolution within the dataset and adopted the format and classifications of the EarthChem repository to maintain data continuity. Of these attributes, only “Material”, “Type”, “Composition”, and “Paragenesis” were examined further because the attribute “Formation” contains detailed geologic descriptions taken verbatim from literature, which cannot be clustered into specific groups, unlike the other four attributes. When only the geologic “Formation” environment was provided, terms were determined based on descriptions from the literature and rock-type definitions from Mindat (https://www.mindat.org, last access: 21 September 2023) for each of the petrogenetic attributes. Therefore, all 95 650 sample analyses contain terms for each of the petrogenetic attributes or were recorded as unknown if unidentified. Each of the petrogenetic attributes were plotted by counts of unique categories to examine the representation of attributes within the dataset (Figs. 6, 7, 8, 9). Table 2 includes an abbreviated summary of the most prominent categories within each petrogenetic attribute and the number of sample analyses that are represented by each category. Much like the categorical locality data, the petrogenesis data should not be used to represent the overall natural occurrence of garnets.
Table 2Abbreviated summary of category totals for the “Petrogenetic” attributes (material, type, composition, paragenesis). There are 6 total categories for the Material attribute, 56 Types of material, 19 Compositions, and finally 161 unique paragenetic modes. All of the 95 650 sample analyses have assigned categories in the dataset. The most prevalent categories and the number of sample analyses represented by each category are listed for the Type, Composition, and Paragenesis attributes, respectively. Plots of these attributes are depicted in Fig. 6. See the dataset in the Evolutionary System of Mineralogy Database (ESMD; http://odr.io/ESMD, last access: 21 September 2023) for the detailed petrogenetic attributes.
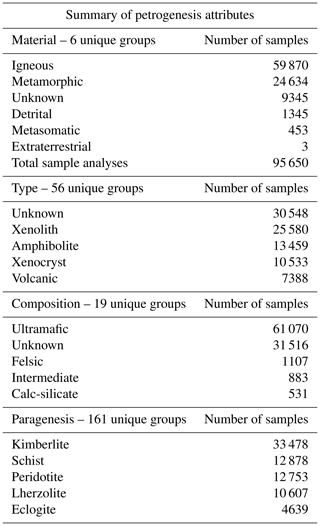
Beginning with “Material”, this attribute offers the lowest resolution across six categories: extraterrestrial, igneous, metamorphic, metasomatic, detrital, and unknown (Fig. 6). The extraterrestrial material contains garnet grains obtained from meteorites. The igneous material (both intrusive and extrusive) consists of garnets from volcanic provinces, while the metamorphic material contains garnets from a diverse set of metamorphic terranes due to the MetPetDB data. The metasomatic material is dominated by skarn deposits. The detrital material consists of garnet grains found in sedimentary deposits without an associated host rock. Finally, the unknown material consists of sample analyses without any associated information. The most common parent material represented in the dataset is igneous with 59 870 analyses followed by 24 634 metamorphic, 9345 unknown, 1345 detrital, 453 metasomatic, and 3 extraterrestrial sample analyses (Fig. 6; Table 2). As garnets are most commonly found within metamorphic rocks, this was an unexpected result. It is possible that the dataset may be significantly biased towards garnets of igneous origin because the samples from the EarthChem repository constitute a substantial proportion of the igneous sample analyses in the overall dataset, potentially due to the prevalence of kimberlite exploration studies.
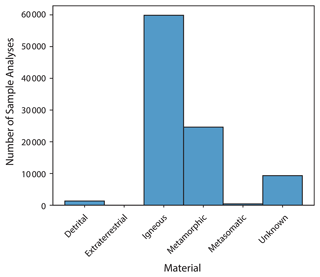
Figure 6Representation of the parent “Material” in the dataset. There are six categories for Material represented by igneous, metamorphic, unknown, detrital, metasomatic, and extraterrestrial sample analyses. See Table 2 for the total number of analyses per category.
The “Type” of parent material is represented by 56 categories in the dataset which are plotted based on the number of samples per category in Fig. 7. The five most reported material types include 30 548 unknown analyses followed by xenoliths with 25 580 analyses largely originating from EarthChem, as well as 13 459 amphibolite analyses, 10 533 xenocrysts, and finally 7388 volcanic analyses (Table 2). These five categories account for ∼91 % of the overall dataset. The total number of samples for each of the other 55 types of material categories feature a substantially lower count. This is most likely a result of biases from the sources collected to construct the dataset rather than the distribution of garnets represented in nature.
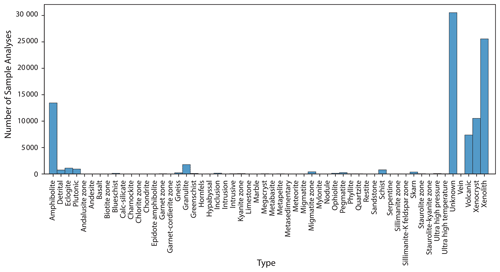
Figure 7Representation of the “Type” of parent material in the dataset. There are 56 possible categories for the Type of parent material which are largely represented by unknown, xenolith, amphibolite, xenocryst, and finally volcanic sample analyses. See Table 2 for an abbreviated summary of the total number of analyses per category.
The “Composition” of parent material is expressed by 19 different categories throughout the dataset (Fig. 8). There are 61 070 ultramafic and 31 516 unknown compositions which dominate the distribution (Fig. 8; Table 2). Despite these large values, the next two most prevalent categories of composition include 1107 felsic and 883 intermediate samples. These main compositions of the parent material account for the large number of igneous samples recorded from the EarthChem repository.
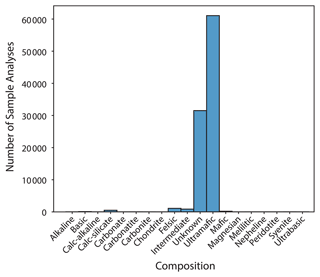
Figure 8Representation of the “Composition” of parent material in the dataset. There are 19 possible Compositions which are heavily biased by ultramafic and unknown compositions, followed by felsic and intermediate sample analyses. See Table 2 for an abbreviated summary of the total number of analyses per category.
The “Paragenesis” of sample analyses is the highest resolution attribute and presents a total 161 possible paragenetic modes of specific rock-type names derived from the literature and data repositories. We maintained as much of the terminology used to describe each sample as possible to minimize oversimplification. For example, orthogneiss and paragneiss are recorded as such rather than being lumped into the general category of gneiss. Nevertheless, some sources were more descriptive than others, which created a wide range of categories in this attribute from a vague classification of “igneous” to an “orthopyroxenite”. The distribution of the 161 categories within Paragenesis is plotted in Fig. 9. The majority of samples originate from 33 478 kimberlite analyses in the EarthChem repository, which contributes to the large number of classified igneous material samples as well (Fig. 9; Table 2). Other significant paragenetic modes include 12 878 schist, 12 753 peridotite, 10 607 lherzolite, and 4656 eclogite sample analyses (Fig. 9; Table 2). These five most common paragenetic modes represent 77.7 % of the entire dataset. As with the other petrogenetic attributes, these data are most likely biased based on the chosen locality of these samples, the specific scientific investigation of certain studies, or the compiled literature across all data repositories and peer-reviewed literature.
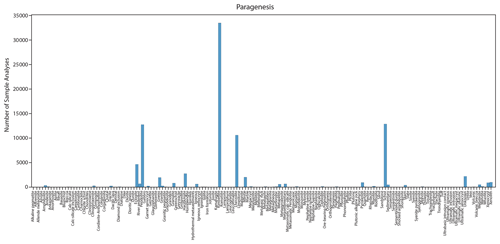
Figure 9Representation of the “Paragenesis” of sample analyses in the dataset. There are 161 categories for Paragenesis in the dataset. The most common paragenetic modes include kimberlite, peridotite, schist, lherzolite, eclogite, and unknown sample analyses. See Table 2 for an abbreviated summary of the total number of analyses per category.
3.5 Dataset applications and limitations
This dataset offers a wide range of garnet sample analyses across data sources in the literature, large data repositories, and maintains sample analyses published prior to 1990 to prevent the loss of dark data. These sample analyses measure garnets with unusual compositions, end-members, and/or high concentrations of one or more elements (e.g., uranium). The localization of garnet sample analyses to one dataset offers a plethora of possible research applications. For example, this dataset is useful for both geological and archeological provenance evaluations as well as natural kind clustering and multivariate analysis in geochemical and mineralogical research (Hazen et al., 2008; Hazen et al., 2012; Hazen, 2014; Hazen et al., 2014; Hazen 2019; Hazen et al., 2019; Hazen and Morrison, 2020; Hazen et al., 2020; Morrison et al., 2020; Hazen and Morrison, 2021; Prabhu et al., 2023).
Nevertheless, despite the diversity of sample analyses, there are some key limitations that should be kept in mind when considering applications of this dataset. First, this dataset includes all garnet samples, including rare or unusual compositions, across all possible formation environments. Thus, there are certain distinctions between igneous and metamorphic garnets that must be considered regarding their composition. Garnets that form in metamorphic environments will exhibit compositional zoning from core to rim that vary due to the temperature and pressure changes during formation (Wang et al., 2023). A single grain can have drastically different compositions and presence of trace elements throughout zonation layers (Spear and Daniel, 2001; Whitney and Seaton, 2010). Additionally, if a garnet underwent secondary metamorphism, high temperatures would modify the zonation and the composition could be affected by partial decomposition, dissolution, and regrowth in the form of accretion of new garnet during subsequent metamorphic processes (Wang et al., 2023). In contrast, igneous garnets in plutonic rocks will form from the equilibrium phases in the residual melt while volcanic garnets cannot be assumed to be in equilibrium during magmatic crystallization. Therefore, when applying this dataset to paragenesis and petrogenesis of garnets, the formation history and zonation of a garnet must be considered in addition to the geochemical data.
Second, there are some limitations regarding the classifications of the petrogenetic and paragenetic attributes within the dataset – these distinctions are simplified and could be subjective to each authors interpretation. For example, within the “Type” category of “Xenoliths”, these rock fragments could consist of different formation processes (such as fragments of amphibolite/granulite/eclogite facies) that were captured in a volcanic sequence. Thus, their Type as a Xenolith would not represent the individual formation processes of the garnets within the host rock.
Third, some classifications of paragenesis do not contain compositional information. For example, a “Schist” does not consider the compositional origin of the parent rock and therefore could be a peridotite with a foliated texture.
Finally, these classifications and distinctions were adopted from the EarthChem repository to maintain data continuity. Therefore, this dataset provides the original classifications applied to the data donated to the repository – presumably from the original authors themselves, although this cannot be guaranteed. For example, while Peridotite is listed as a category within paragenesis so are Lherzolite and Harzburgite which are types of peridotites. We recommend that these categories be grouped together when analyzing this dataset further. Ideally, a system of properly representing the rock-type origin and individual mineral formation processes should be developed to prevent misinterpretation of samples within large datasets such as this one.
There could be other limitations other than the specific examples mentioned here. We recommend that any researchers using this dataset for their own work carefully consider the petrogenetic and paragenetic categories as well as the original sources of the data.
Future work with cluster analysis will focus on dividing garnet samples into different groups that correspond to their paragenetic modes (such as igneous or metamorphic types), formational environment (different tectonic settings), or temperature–pressure conditions which is consistent with natural kind clustering. For example, pyrope is known to occur in mantle-derived ultramafic rocks, including eclogite and kimberlite, as well as in amphibole and biotite schists (Deer et al., 1982). Similarly, andradite is frequently encountered in both contact metamorphic environments as well as in alkali igneous rocks. We suggest that multivariate and cluster analysis will reveal discrete combinations of compositions and other attributes for these contrasting igneous and metamorphic parageneses for pyrope and andradite. Compared with defining garnet groups based on chemical compositions, these future paths might have further implications for understanding the formation of the garnets, identifying source lithologies for detrital garnets, and documenting the co-evolution of garnet with earth's environment.
This database aims to incorporate future studies and sample analyses, after publication, in the Evolutionary System of Mineralogy Database (ESMD). Ultimately, we intend to develop a system in which researchers can upload their samples to this database for continuous documentation and expansion of garnet mineralogical data.
These data are freely available from the Evolutionary System of Mineralogy Database (ESMD; https://doi.org/10.48484/camh-xy98) (Chiama et al., 2022).
In a society increasingly dependent on the internet and open-access data resources, it is imperative to maintain the accessibility, reproducibility, and interoperability of data in accordance with the FAIR guiding principles. Thus, the data science goals of this study were to record dark data for garnet group minerals in a standardized format that is readily accessible and to combine those dark data with current databases, which facilitates the access to valuable scientific information while continuing to expand the availability of mineralogical data for future studies. We encourage scientists to contribute to these large and growing data repositories of mineralogical information, which are proving invaluable in the advancement of scientific discovery.
Supplement Sect. A: a detailed analysis of the 275 original EMPA point analyses performed for the dataset. Supplement Sect. B: a summary of the average oxide totals for the 275 original EMPA point analyses. Supplement Sect. C: a list of references for the data presented in the dataset. The supplement related to this article is available online at: https://doi.org/10.5194/essd-15-4235-2023-supplement.
RMH conceptualized the project idea. RMH, SZ, SMM, ESB AB, and JAN mentored and provided advice. RMH, JAN, MJW, KL, and FS provided resources of garnet samples. KC, MG, IL, and RR performed data curation, development of dataset methodology, formal analysis of data, investigation, and manuscript preparation and finalization. KC, MG, IL, RR, ESB, and AB prepared samples and analyzed their compositions. IL and MG preformed investigations for the “End-member Classification and Quality Index” spreadsheet. KC created visualizations for individual attributes in the dataset. RMH, JAN, SZ, SMM, AB, MJW, KL, and FS reviewed and edited the manuscript.
The contact author has declared that none of the authors has any competing interests.
Publisher’s note: Copernicus Publications remains neutral with regard to jurisdictional claims in published maps and institutional affiliations.
We thank the Carnegie Institution for Science's Earth and Planets Laboratory and the 4D Deep-Time Data Driven Initiative (4d.carnegiescience.edu) for supporting us during this research project. We thank Julia A. Nord and Robert M. Hazen for their donation of garnet samples for EMPA analysis as well as Kerstin Lehnert and Frank Spear for their donation of garnet sample analyses included in the EarthChem and MetPetDB repositories, respectively. We also thank Michael Walter, the deputy director of the Earth and Planets Laboratory, for donating his personal dataset of majorite samples for this project. We would like to thank Emma Bullock for arranging time to operate the SEM and EMPA to evaluate our samples (as well as for advice and guidance with sample analyses), Guiseppina Kysar for advice and feedback on the manuscript, Rebecca Schmidt for help with compiling garnet analyses from the literature, Andrew Locock for his help on combining the two spreadsheets used in this paper, Matthew Endries for coding and Excel advice and edits, and Michael Naylor Hudgins for manuscript advice and figure preparation. We thank the reviewers for their thoughtful and insightful feedback that made this paper stronger.
This research was supported by the NASA Astrobiology Institute (Cycle 8) ENIGMA: Evolution of Nanomachines In Geospheres and Microbial Ancestors (grant number 80NSSC18M0093) and the John Templeton Foundation.
This paper was edited by Attila Demény and reviewed by Shoji Arai and two anonymous referees.
Alizai, A., Clift, P. D., and Still, J.: Indus Basin sediment provenance constrained using garnet geochemistry, J. Asian Earth Sci., 126, 29–57, https://doi.org/10.1016/j.jseaes.2016.05.023, 2016.
Bauer, A. M., Reimink, J. R., Chacko, T., Foley, B. J., Shirey, S. B., and Pearson, D. G.: Hafnium isotopes in zircons document the gradual onset of mobile-lid tectonics, Geochemical Perspectives Letters, 14, 1–6, https://doi.org/10.7185/geochemlet.2015, 2020.
Baxter, E. F. and Scherer, E. E.: Garnet geochronology: Timekeeper of tectonometamorphic processes, Elements, 9, 433–438, https://doi.org/10.2113/gselements.9.6.433, 2013.
Baxter, E. F., Caddick, M. J., and Dragovic, B.: Garnet: A rock-forming mineral petrochronometer, Rev. Mineral. Geochem., 83, 469–533, https://doi.org/10.2138/rmg.2017.83.15, 2017.
Boujibar, A., Howell, S., Zhang, S., Hystad, G., Prabhu, A., Liu, N., Stephan, T., Narkar, S., Eleish, A., Morrison, S. M., Hazen, R. M., and Nittler, L. R.: Cluster analysis of presolar silicon carbide grains: evaluation of their classification and astrophysical implications, Astrophys. J. Lett., 907, L39, https://doi.org/10.3847/2041-8213/abd102, 2021.
Cawood, P., Chowdhury, P., Mulder, J., Hawkesworth, C., Capitanio, F., Gunawardana, P., and Nebel, O.: Secular Evolution of Continents and the Earth System, Rev. Geophys., 60, e2022RG000789, https://doi.org/10.1029/2022RG000789, 2022.
Chassé, M., Griffin, W. L., Alard, O., O'Reilly, S. Y., and Calas, G.: Insights into the mantle geochemistry of scandium from a meta-analysis of garnet data, Lithos, 310–311, 409–421, https://doi.org/10.1016/j.lithos.2018.03.026, 2018.
Chen, Y.-X., Zhou, K., Zheng, Y.-F., Chen, R.-X., and Hu, Z.: Garnet geochemistry records the action of metamorphic fluids in ultrahigh-pressure dioritic gneiss from the Sulu orogen, Chem. Geol., 398, 46–60, https://doi.org/10.1016/j.chemgeo.2015.01.021, 2015.
Chiama, K., Gabor, M., Lupini, I., Rutledge, R., Nord, J. A., Zhang, S., Boujibar, A., Bullock, E. S., Walter, M. J., Lehnert, K., Spear, F., Morrison, S., and Hazen, R. M.: Garnet mineral geochemistry data download from the MetPetDB (re3data.org) August 2019, Version 1.0., Interdisciplinary Earth Data Alliance (IEDA) [data set], https://doi.org/10.26022/IEDA/112173, 2021a.
Chiama, K., Gabor, M., Lupini, I., Rutledge, R., Nord, J. A., Zhang, S., Boujibar, A., Bullock, E. S., Walter, M. J., Lehnert, K., Spear, F., Morrison, S., and Hazen, R. M.: Garnet mineral geochemistry data download from the EarthChem Portal August 2019, Version 1.0., Interdisciplinary Earth Data Alliance (IEDA) [data set], https://doi.org/10.26022/IEDA/112171, 2021b.
Chiama, K., Gabor, M., Lupini, I., Rutledge, R., Nord, J. A., Zhang, S., Boujibar, A., Bullock, E. S., Walter, M. J., Lehnert, K., Spear, F., Morrison, S. M., and Hazen, R. M.: ESMD – Garnet Dataset, Open Data Repository [data set], https://doi.org/10.48484/camh-xy98, 2022.
Čopjaková, R., Sulovský, P., and Paterson, B.A.: Major and trace elements in pyrope–almandine garnets as sediment provenance indicators of the Lower Carboniferous Culm sediments, Drahany Uplands, Bohemian Massif, Lithos, 82, 51–70, https://doi.org/10.1016/j.lithos.2004.12.006, 2005.
Deer, W. A., Howie, R. A., and Zussman, J.: Rock-Forming Minerals: Volume 1A Orthosilicates, Second Edition, New York: Longman, 1982.
Droop, G.: A general equation for estimating Fe3 concentrations in ferromagnesian silicates and oxides from microprobe analyses, using stoichiometric criteria, Mineral. Mag., 51, 431–435, https://doi.org/10.1180/minmag.1987.051.361.10, 1987.
EarthChem Portal: EarthChem Portal [data set], https://www.earthchem.org, last access: 22 September 2023.
Fagan, T. J., Guan, Y., MacPherson, G. J., and Huss, G. R.: Al-Mg isotopic evidence for separate nebular and parent-body alteration events in two allende CAls, Lunar and Planetary Sciences, https://www.lpi.usra.edu/meetings/lpsc2005/pdf/1820.pdf (last access: 21 September 2023), 2005.
Farré-de-Pablo, J., Proenza, J. A., González-Jiménez, J. M., Aiglsperger, T., Torro, L., Domenech, C., and Garcia-Casco, A.: Low-temperature hydrothermal Pt mineralization in uvarovite-bearing ophiolitic chromitites from the Dominican Republic, Miner. Deposita, 57, 955–976, https://doi.org/10.1007/s00126-021-01079-8, 2022.
Gatewood, M. P., Dragovic, B., Stowell, H. H., Baxter, E. F., Hirsch, D. M., and Bloom, R.: Evaluating chemical equilibrium in metamorphic rocks using major element and Sm–Nd isotopic age zoning in garnet, Townshend Dam, Vermont, USA, Chem. Geol., 401, 151–168, https://doi.org/10.1016/j.chemgeo.2015.02.017, 2015.
Geiger, C. A: A tale of two garnets: The role of solid solution in the development toward a modern mineralogy, Am. Mineral., 101, 1735–1749, https://doi.org/10.2138/am-2016-5522, 2016.
GeoReM: Geological and Environmental [data set], http://georem.mpch-mainz.gwdg.de/, last access: 21 September 2023.
GeoRoc: Geochemistry of Rocks of the Oceans and Continents [data set], http://georoc.mpch-mainz.gwdg.de/georoc/Start.asp, last access: 21 September 2023
Ghosh, B. and Morishita, T.: Andradite-uvarovite solid solution from hydrothermally altered podiform chromite, Rutland ophiolite, Andaman, India, Can. Mineral., 49, 573–580, https://doi.org/10.3749/canmin.49.2.573, 2011.
Ghosh, B., Morishita, T., Ray, J., Tamura, A., Mizukami, T., Soda, Y., and Ovung, T. N.: A new occurrence of titanian (hydro)andradite from the Nagaland ophiolite, India: Implications for element mobility in hydrothermal environments, Chem. Geol., 457, 47–60, https://doi.org/10.1016/j.chemgeo.2017.03.012, 2017.
Golden, J. J.: Mineral Evolution Database: Data Model for Mineral Age Associations, M.S. Thesis, University of Arizona, Tucson AZ, 2019.
Grew, E. S., Locock, A. J., Mills, S. J., Galuskina, I. O., Galuskin, E. V., and Hålenius, U.: Nomenclature of the garnet supergroup, Am. Mineral., 98, 785–811, https://doi.org/10.2138/am.2013.4201, 2013.
Griffin, W. L., Fisher, N. I., Friedman, J. H., Ryan, C. G., and O'Reilly, S. Y.: Cr-pyrope garnets in the lithospheric mantle. I. Compositional systematics and relations to tectonic settings, J. Petrol., 40, 679–704, https://doi.org/10.1093/petroj/40.5.679, 1999a.
Griffin, W. L., Shee, S. R., Ryan, C. G., Win, T. T., and Wyatt, B. A.: Harzburgite to lherzolite and back again: metasomatic processes in ultramafic xenoliths from the Wesselton Kimberlite, Kimberley, South Africa, Contrib. Mineral. Petr., 134, 232–250, https://doi.org/10.1007/s004100050481, 1999b.
Hawkesworth, C. J., Cawood, P. A., and Dhuime, B.: The Evolution of the Continental Crust and the Onset of Plate Tectonics, Front. Earth Sci., 8, 326, https://doi.org/10.3389/feart.2020.00326, 2020.
Hazen, R. M.: Data-driven abductive discovery in mineralogy, Am. Mineral., 99, 2165–2170, https://doi.org/10.2138/am-2014-4895, 2014.
Hazen, R. M.: An evolutionary system of mineralogy: Proposal for a classification of planetary materials based on natural kind clustering, Am. Mineral., 104, 810–816, https://doi.org/10.2138/am-2019-6709CCBYNCND, 2019.
Hazen, R. M. and Morrison, S. M.: An evolutionary system of mineralogy, Part I: stellar mineralogy (>13 to 4.6 Ga), Am. Mineral., 105, 627–651, https://doi.org/10.2138/am-2020-7173, 2020.
Hazen, R. M. and Morrison, S. M.: An evolutionary system of mineralogy, Part V: Aqueous and thermal alteration of planetesimals (∼4565 to 4550 Ma), Am. Mineral., 106, 1388–1419, https://doi.org/10.2138/am-2021-7760, 2021.
Hazen, R. M., Papineau, D., Bleeker, W., Downs, R. T., Ferry, J. M., McCoy, T. J., Sverjensky, D. A., and Yang, H.: Mineral evolution, Am. Mineral., 93, 1693–1720, https://doi.org/10.2138/am.2008.2955, 2008.
Hazen, R. M., Golden, J., Downs, R. T., Hystad, G., Grew, E. S., Azzolini, D., and Sverjensky, D. A.: Mercury (Hg) mineral evolution: A mineralogical record of supercontinent assembly, changing ocean geochemistry, and the emerging terrestrial biosphere, Am. Mineral., 97, 1013–1042, https://doi.org/10.2138/am.2012.3922, 2012.
Hazen, R. M., Liu, X.-M., Downs, R. T., Golden, J., Pires, A. J., Grew, E. S., Hystad, G., Estrada, C., and Sverjensky, D. A.: Mineral Evolution: Episodic Metallogenesis, the Supercontinent Cycle, and the Coevolving Geosphere and Biosphere, Econ. Geol. Special Publication, 18, 1–15, 2014.
Hazen, R. M., Downs, R. T., Eleish, A., Fox, P., Gagné, O. C., Golden, J. J., Grew, E. S., Hummer, D. R., Hystad, G., Krivovichev, S. V., Li, C., Liu, C., Ma, X., Morrison, S. M., Pan, F., Pires, A. J., Prabhu, A., Ralph, J., Runyon, S. E., and Zhong, H.: Data-driven discovery in mineralogy: Recent advances in data resources, analysis, and visualization, Engineering, 5, 397–405, https://doi.org/10.1016/j.eng.2019.03.006, 2019.
Hazen, R. M., Morrison, S. M., and Prabhu, A.: An evolutionary system of mineralogy, Part III: Primary chondrule mineralogy (4566 to 4561 Ma), Am. Mineral., 106, 325–350, https://doi.org/10.2138/am-2020-7564, 2020.
Höfer, H. E., Weinbrunch, S., McCammon, C. A., and Brey, G. P.: Comparison of two electron probe microanalysis techniques to determine ferric iron in synthetic wustite samples, Eur. J. Mineral, 12 , 63–71, https://doi.org/10.1127/0935-1221/2000/0012-0063, 2000.
International Generic Sample Number (IGSN): https://www.igsn.org/, last access: 27 September 2020.
Inglis, J. D., Hefferan, K., Samson, S. D., Admou, H., and Saquaque, A.: Determining Age of Pan African Metamorphism using Sm-Nd Garnet-Whole Rock Geochronology and Phase Equilibria Modeling in the Tasriwine Ophiolite, Sirwa, Anti-Atlas Morocco, J. Afr. Earth Sci., 127, 88–98, https://doi.org/10.1016/j.jafrearsci.2016.06.021, 2017.
Jackson, I.: OneGeology: from concept to reality, Episodes Journal of International Geoscience, 31, 344–345, 2008.
Javanmard, S. R., Tahmasbi, Z., Ding, X., Khalaji, A. A., and Hetherington, C. J.: Geochemistry of garnet in pegmatites from the Boroujerd Intrusive Complex, Sanandaj-Sirjan Zone, western Iran: implications for the origin of pegmatite melts, Miner. Petrol., 112, 837–856, https://doi.org/10.1007/s00710-018-0591-x, 2018.
Jochum, K. P., Nohl, U., Herwig, K, Lammel, E., Stoll, B., and Hofmann, A. W.: GeoReM: A New Geochemical Database for Reference Materials and Isotopic Standards, Geostand. Geoanal. Res., 29, 333–338, https://doi.org/10.1111/j.1751-908X.2005.tb00904.x, 2007.
Korinevsky, V. G.: Spessartine-Andradite In Scapolite Pegmatite, Ilmeny Mountains, Russia, Can. Mineral., 53, 623–632, https://doi.org/10.3749/canmin.4354, 2015.
Kotková, J. and Harley, S. L.: Anatexis during High-pressure Crustal Metamorphism: Evidence from Garnet–Whole-rock REE Relationships and Zircon–Rutile Ti–Zr Thermometry in Leucogranulites from the Bohemian Massif, J. Petrol., 51, 1967–2001, https://doi.org/10.1093/petrology/egq045, 2010.
Krippner, A., Meinhold, G., Morton, A. C., Schönig, J., and Von Eynatten, H.: Heavy minerals and garnet geochemistry of stream sediments and bedrocks from the Almklovdalen area, Western Gneiss Region, SW Norway: Implications for provenance analysis, Sediment. Geol., 336, 96–105, https://doi.org/10.1016/j.sedgeo.2015.09.009, 2016.
Lafuente B., Downs R. T., Yang H., and Stone, N.: The power of databases: the RRUFF project, in: Highlights in Mineralogical Crystallography, edited by: Armbruster, T. and Danisi, R. M., Berlin, Germany, W. De Gruyter, 1–30, https://doi.org/10.1515/9783110417104-003, 2015.
Lehnert, K. and Wyborn, L. A.: OneGeochemistry: Toward a global network of geochemical data, AGU Fall Meeting 2019, AGU, 2019.
Lehnert, K., Su, Y., Langmuir, C., Sarbas, B., and Nohl, U.: A global geochemical database structure for rocks, Geochem. Geophy. Geosy. 1, 1012, https://doi.org/10.1029/1999GC000026, 2000.
Lehnert, K., Wyborn, L., Bennett, V. C., Hezel, D., McInnes, B. I. A., Plank, T., and Rubin, K.: OneGeochemistry: Towards an Interoperable Global Network of FAIR Geochemical Data, CODATA: Towards Next-Generation Data-Driven Science September 2019 (CODATA2019), Beijing, China, Zenodo, https://doi.org/10.5281/zenodo.5767950, 2021.
Locock, A. J.: An Excel spreadsheet to recast analyses of garnet into end-member components, and a synopsis of the crystal chemistry of natural silicate garnets, Comput. Geosci., 34, 1769–1780, https://doi.org/10.1016/j.cageo.2007.12.013, 2008.
Makrygina, V. A. and Suvorova, L. F.: Spessartine in the greenschist facies: Crystallization conditions, Geochem. Int., 49, 299–308, https://doi.org/10.1134/S0016702911030074, 2011.
Manton, R. J., Buckman, S., Nutman, A. P., Bennett, V. C., and Belousova, E. A.: U-Pb-Hf-REE-Ti zircon and REE garnet geochemistry of the Cambrian Attunga eclogite, New England Orogen, Australia: Implications for continental growth along eastern Gondwana: Orogens and Oceanic Terranes, Tectonics, 36, 1580–1613, https://doi.org/10.1002/2016TC004408, 2017.
Melcher, F., Grum, W., Simon G., Thalhammer, T. V., and Stumpfl, E. F.: Petrogenesis of the Ophiolitic Giant Chromite Deposits of Kempirsai, Kazakhstan: a Study of Solid and Fluid Inclusions in Chromite, J. Petrol., 38, 1419–1458, https://doi.org/10.1093/petroj/38.10.1419, 1997.
MetPetDB: editing status 2019-02-01; – Registry of Research Data Repositories [data set], https://doi.org/10.17616/R3ZN2S, last access: 15 October 2020.
Mindat: Mindat.org [data set], https://www.mindat.org, last access: 21 September 2023.
Morrison, S. M. and Hazen, R. M.: An evolutionary system of mineralogy. Part II: Interstellar and solar nebula primary condensation mineralogy (>4.565 Ga), Am. Mineral., 105, 1508–1535, https://doi.org/10.2138/am-2020-7447, 2020.
Morrison, S. M. and Hazen, R. M.: An evolutionary system of mineralogy, Part IV: Planetesimal differentiation and impact mineralization (4566 to 4560 Ma), Am. Mineral., 106, 730–761, https://doi.org/10.2138/am-2021-7632, 2021.
Morrison, S. M., Buongiorno, J., Downs, R. T., Eleish, A., Fox, P., Giovannelli, D., Golden, J. J., Hummer, D. R., Hystad, G., Kellogg, L. H., Kreylos, O., Krivovichev, S. V., Liu, C., Prabhu, A., Ralph, J., Runyon, S. E., Zahirovic, S., and Hazen, R. M.: Exploring carbon mineral systems: Recent advances in C mineral evolution, mineral ecology, and network analysis, Front. Earth Sci., 8, 1–12, https://doi.org/10.3389/feart.2020.00208, 2020.
Morton, A., Hallsworth, C., and Chalton, B.: Garnet compositions in Scottish and Norwegian basement terrains: a framework for interpretation of North Sea sandstone provenance, Mar. Petrol. Geol., 21, 393–410, https://doi.org/10.1016/j.marpetgeo.2004.01.001, 2004.
Nesse, W. D.: Introduction to Optical Mineralogy, 4th edition, Oxford University Press, New York, NY, USA, 253–255, 2013.
Nickel, K. G. and Green, D. H.: Empirical geothermobarometry for garnet peridotites and implications for the nature of the lithosphere, kimberlites and diamonds, Earth Planet. Sc. Lett., 73, 158–170, https://doi.org/10.1016/0012-821x(85)90043-3, 1985.
Nimis, P. and Grutter, H.: Internally consistent geothermometers for garnet peridotites and pyroxenites, Contrib. Mineral. Petr., 159, 411–427, https://doi.org/10.1007/s00410-009-0455-9, 2010.
Parthasarathy, G., Balaram, V., and Srinivasan, R.: Characterization of green garnets from an Archean calc-silicate rock, Bandihalli, Karnataka, India: Evidence for a continuous solid solution between uvarovite and grandite, J. Asian Earth Sci., 17, 345–352, https://doi.org/10.1016/S0743-9547(98)00064-6, 1999.
Patranabis-Deb, S., Schieber, J., and Basu, A.: Almandine garnet phenocrysts in a ∼1 Ga rhyolitic tuff from central India, Geol. Mag., 146, 133–143, https://doi.org/10.1017/S0016756808005293, 2009.
PetDB: EarthChem PetDB Search [data set], http://www.earthchem.org/petdb, last access: 21 September 2023.
Prabhu, A., Morrison, S., Eleish, A., Zhong, H., Huang, F., Golden, J., Perry, S., Hummer, D., Runyon, S., Fontaine, K., Krivovichev, S., Downs, R., Hazen, R. M., and Fox, P.: Global Earth mineral inventory: A data legacy, Geosci. Data J., 1, 1–16, https://doi.org/10.1002/gdj3.106, 2020.
Prabhu, A., Morrison, S. M., Fox, P. A., Ma, X., Wong, M. L., Williams, J., McGuinness, K. N., Krivovichev, S., Lehnert, K., Ralph, J., Lafuente, B., Downs, R. T., Walter, M. J., and Hazen, R. M.: What is mineral informatics?, Am. Mineral., 108, 1242–1257, https://doi.org/10.2138/am-2022-8613, 2023.
Rosenfeld, J.: Rotated garnets in metamorphic rocks, Geological Society of America, Boulder, Colorado, https://doi.org/10.1130/SPE129, 1970.
Schönig, J., Meinhold, G., Von Eynatten, H., and Lünsdorf, N. K.: Provenance information recorded by mineral inclusions in detrital garnet, Sediment. Geol., 376, 32–49, https://doi.org/10.1016/j.sedgeo.2018.07.009, 2018.
Sieck, P., López-Doncel, R., Dávila-Harris, P., Aguillón-Robles, A., Wemmer, K., and Maury, R. C.: Almandine garnet-bearing rhyolites associated to bimodal volcanism in the Mesa Central of Mexico: Geochemical, petrological and geochronological evolution, J. S. Am. Earth Sci., 92, 310–328, https://doi.org/10.1016/j.jsames.2019.03.018, 2019.
Spear, F. S. and Daniel, C. G.: Diffusion control of garnet growth, Harpswell Neck, Maine, USA, J. Metamorph. Geol., 19, 179–195, https://doi.org/10.1046/j.0263-4929.2000.00306.x, 2001.
Spear, F. S., Hallett, B., Pyle, J. M., Adalı, S., Szymanski, B. K., Waters, A., Linder, Z., Pearce, S. O., Fyffe, M., Goldfarb, D., Glickenhouse, N., and Buletti, H.: MetPetDB: A database for metamorphic geochemistry, Geochem. Geophys. Geosy., 10, Q12005, https://doi.org/10.1029/2009GC002766, 2009.
Suwa, K., Suzuki, K., and Agata, T.: Vanadium grossular from the Mozambique metamorphic rocks, south Kenya, J. Southe. Asian Earth, 14, 299–308, https://doi.org/10.1016/S0743-9547(96)00066-9, 1996.
The Gemology Project Color Grading: Color Grading, http://gemologyproject.com/wiki/index.php?title=Color_grading, last access: 10 October 2020.
The RRUFF Project: The RRUFF Project, https://rruff-2.geo.arizona.edu, last access: 21 September 2023.
Thomson, A. R., Kohn, S. C., Prabhu, A., and Walter, M. J.: Evaluating the Formation Pressure of Diamond-Hosted Majoritic Garnets: A Machine Learning Majorite Barometer, J. Geophys. Res.-Sol. Ea., 126, e2020JB02060, https://doi.org/10.1029/2020jb020604, 2021.
Walter, M. J., Thomson, A. R., and Smith, E.: Geochemistry of silicate and oxide inclusions in sub-lithospheric diamonds, Reviews in Mineralogy and Geochemistry, Mineralogical Society of America, 88, 393–450, https://doi.org/10.2138/rmg.2022.88.07, 2022.
Wang, C., Hazen, R. M., Cheng, Q., Stephenson, M. H., Zhou, C., Fox, P., Shen, S.-Z., Oberhänsli, R., Hou, Z., Ma, X., Feng, Z., Fan, J., Ma, C., Hu, X., Luo, B., Wang, J., and Schiffries,C. M.: The Deep-Time Digital Earth program: data-driven discovery in geosciences, Nat. Sci. Rev., 8, nwab027, https://doi.org/10.1093/nsr/nwab027, 2021.
Wang, D., Mitchell, R. N., Guo, J., and Liu, F.: Exhumation of an Archean Granulite Terrane by Paleoproterozoic Orogenesis: Evidence from the North China Craton, J. Petrol., 64, egad035, https://doi.org/10.1093/petrology/egad035, 2023.
Web Colors: Web Colors, https://en.wikipedia.org/wiki/Web_colors, last access: 16 October 2020.
Whitney, D. L. and Seaton, N. C.A .: Garnet polycrystals and the significance of clustered crystallization, Contrib. Mineral. Petr., 160, 591–607, https://doi.org/10.1007/s00410-010-0495-1, 2010.
Wilkinson, M. D., Dumontier, M., Aalbersberg, I. J., Appleton, G., Axton, M., Baak, A., Blomberg, N., Boiten, J. W., da Silva Santos, L. B., Bourne, P. E., Bouwman, J., Brookes, A. J., Clark, T., Crosas, M., Dillo, I., Dumon, O., Edmunds, S., Evelo, C. T., Finkers, R., Gonzalez-Beltran, A., Gray, A. J., Groth, P., Goble, C., Grethe, J. S., Heringa, J., 't Hoen, P. A., Hooft, R., Kuhn, T., Kok, R., Kok, J., Lusher, S. J., Martone, M. E., Mons, A., Packer, A. L., Persson, B., Rocca-Serra, P., Roos, M., van Schaik, R., Sansone, S. A., Schultes, E., Sengstag, T., Slater, T., Strawn, G., Swertz, M. A., Thompson, M., van der Lei, J., van Mulligen, E., Velterop, J., Waagmeester, A., Wittenburg, P., Wolstencroft, K., Zhao, J., and Mons, B.: The FAIR Guiding Principles for scientific data management and stewardship, Sci. Data, 3, 160018, https://doi.org/10.1038/sdata.2016.18, 2016.
Wu, C. M. and Zhao, G. C.: The applicability of garnet-orthopyroxene geobarometry in mantle xenoliths, Lithos, 125, 1–9, https://doi.org/10.1016/j.lithos.2011.02.018, 2011.
Yang, J., Peng, J., Hu, R., Bi, X., Zhao, J., Fu, Y., and Shen, N.-P.: Garnet geochemistry of tungsten-mineralized Xihuashan granites in South China, Lithos, 177, 79–90, https://doi.org/10.1016/j.lithos.2013.06.008, 2013.
Zhong, S., Li, S., Liu, Y., Cawood, P. A., and Seltmann, R.: I-type and S-type granites in the Earth's earliest continental crust, Commun. Earth Environ., 4, 61, https://doi.org/10.1038/s43247-023-00731-7, 2023.