the Creative Commons Attribution 4.0 License.
the Creative Commons Attribution 4.0 License.
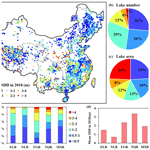
A Landsat-derived annual inland water clarity dataset of China between 1984 and 2018
Hui Tao
Ge Liu
Qiang Wang
Zhidan Wen
Pierre-Andre Jacinthe
Xiaofeng Xu
Jia Du
Yingxin Shang
Sijia Li
Zongming Wang
Lili Lyu
Junbin Hou
Xiang Wang
Dong Liu
Kun Shi
Baohua Zhang
Hongtao Duan
Water clarity serves as a sensitive tool for understanding the spatial pattern and historical trend in lakes' trophic status. Despite the wide availability of remotely sensed data, this metric has not been fully explored for long-term environmental monitoring. To this end, we utilized Landsat top-of-atmosphere reflectance products within Google Earth Engine in the period 1984–2018 to retrieve the average Secchi disk depth (SDD) for each lake in each year. Three SDD datasets were used for model calibration and validation from different field campaigns mainly conducted during 2004–2018. The red blue band ratio algorithm was applied to map SDD for lakes (>0.01 km2) based on the first SDD dataset, where R2=0.79 and relative RMSE (rRMSE) =61.9 %. The other two datasets were used to validate the temporal transferability of the SDD estimation model, which confirmed the stable performance of the model. The spatiotemporal dynamics of SDD were analyzed at the five lake regions and individual lake scales, and the average, changing trend, lake number and area, and spatial distribution of lake SDDs across China were presented. In 2018, we found the number of lakes with SDD <2 m accounted for the largest proportion (80.93 %) of the total lakes, but the total areas of lakes with SDD of <0.5 and >4 m were the largest, both accounting for about 24.00 % of the total lakes. During 1984–2018, lakes in the Tibetan–Qinghai Plateau region (TQR) had the clearest water with an average value of 3.32±0.38 m, while that in the northeastern region (NLR) exhibited the lowest SDD (mean 0.60±0.09 m). Among the 10 814 lakes with SDD results for more than 10 years, 55.42 % and 3.49 % of lakes experienced significant increasing and decreasing trends, respectively. At the five lake regions, except for the Inner Mongolia–Xinjiang region (MXR), more than half of the total lakes in every other region exhibited significant increasing trends. In the eastern region (ELR), NLR and Yungui Plateau region (YGR), almost more than 50 % of the lakes that displayed increase or decrease in SDD were mainly distributed in the area range of 0.01–1 km2, whereas those in the TQR and MXR were primarily concentrated in large lakes (>10 km2). Spatially, lakes located in the plateau regions generally exhibited higher SDD than those situated in the flat plain regions. The dataset is freely available at the National Tibetan Plateau Data Center (https://doi.org/10.11888/Hydro.tpdc.271571, Tao et al., 2021).
- Article
(11817 KB) - Full-text XML
-
Supplement
(459 KB) - BibTeX
- EndNote
Lakes and reservoirs are important aquatic habitats and serve as freshwater water sources for drinking, industrial and agricultural uses (Pekel et al., 2016; Tranvik et al., 2009; Wetzel, 2001). More than 26 000 lakes (with area >0.01 km2) and 78 000 reservoirs are distributed across China (Song et al., 2018a), providing multiple ecosystem services (S. L. Feng et al., 2019; Lehner and Doll, 2004; Tranvik et al., 2009; Yang and Lu, 2014). Over the last 4 decades, China has made considerable achievements with respect to socio-economic development but has also faced increasing water pollution challenges due to, among other contributing factors, agricultural nonpoint pollution, wastewater discharge, urban expansion and increased water consumption (Han et al., 2016; Qin et al., 2010; Tong et al., 2017). Eutrophication and algal bloom proliferation are the clearest manifestations of these water quality problems, and major efforts have been made (afforestation, conversion of cropland to grassland or wetland) to mitigate these impacts and restore the ecological integrity of inland water systems (Huang et al., 2016; Ma et al., 2020; Tong et al., 2020).
Across the country, the number of stations dedicated to the monitoring of water quality in lakes (59) and reservoirs (52) is very limited in comparison to the national inventory of lakes and reservoirs (SOEE, 2018). Water resource managers in China clearly need better assessment tools to monitor inland water quality (Rosenzweig et al., 2011). Commonly expressed as the Secchi disk depth (SDD) (Carlson, 1977), water clarity provides both a practical and a comprehensive measure of the trophic state of aquatic ecosystems (Olmanson et al., 2008; Richardson et al., 2010). However, traditional SDD measurements are limited in terms of their suitability for monitoring large water bodies exhibiting strong spatiotemporal dynamics (Kloiber et al., 2002; Song et al., 2020). Although a Secchi disk apparatus is easy to operate in the field, water clarity monitoring in lakes or reservoirs (herein lakes) located in remote areas can be nearly impossible without aquatic vehicles and may not yield data with sufficient spatial and temporal frequency for trend analysis (Kloiber et al., 2002; Olmanson et al., 2008).
The abundance of optically active constituents (OACs; phytoplankton, non-algal particles and CDOM) is related to the trophic status of aquatic ecosystems and also contributes to water clarity and water surface reflectance, which can be captured by spaceborne sensors (Gordon et al., 1983; Lee et al., 2015). Remote sensing has been widely used for monitoring the spatiotemporal dynamics of SDD at regional and national scales. Available methods for SDD estimation using remote sensing data can be grouped into three categories: analytical, semi-analytical and empirical algorithms (Doron et al., 2007; Lee et al., 2015; G. Liu et al., 2020; McCullough et al., 2013; Olmanson et al., 2008, 2011). The first two methods are difficult to apply to large-scale studies (provincial and national scales) due to the complex theoretical models and parameterization processes and expensive equipment required (Cao et al., 2017; Giardino et al., 2007). The last group of methods is widely used to retrieve SDD at multiple scales due to its simplicity and operability (Duan et al., 2009; L. Feng et al., 2019; McCullough et al., 2012; Olmanson et al., 2011; Shen et al., 2020).
In the past, we faced the challenge of how to handle and analyze big data at national or global scales, like remote sensing datasets from different satellites. Since 2010, Google has hosted a big geo-data platform based on cloud computing, named Google Earth Engine (GEE), which is time-saving for users, who can conduct scientific research online (into topics such as vegetation, agriculture, hydrology and land cover) without downloading these satellite images (Amani et al., 2020). The GEE platform mainly comprises datasets of remote sensing, geophysics and meteorology. The remote sensing datasets contain Landsat (1972–present), Moderate Resolution Imaging Spectrometer (MODIS; 2000–present) and Sentinel (2014–present) (https://code.earthengine.google.com/, last access: last access: 5 January 2022). Remote sensing images are selectively used to estimate SDD for specific regions according to their spatial and temporal resolutions, among which the Landsat images can be used to not only examine the long-term (3–4 decades) spatiotemporal variation in SDD but also monitor lakes ranging from small to large with its higher spatial resolution (30 m). Therefore, the GEE platform is an optimal choice to quickly map SDD long-time-series dynamics based on Landsat observation across China.
In recent years, a few studies have examined the spatiotemporal dynamics of SDD in lakes across China, but they have mainly focused on the large lakes and reservoirs (area >10 km2) (D. Liu et al., 2020; S. Wang et al., 2020; Zhang et al., 2021). Smaller lakes (area <10 km2) are widely distributed across the country, but our understanding of their ecological status remains limited. For example, D. Liu et al. (2020) used an empirical model and the MODIS red and green bands (2000–2018) within GEE to study SDD variation in 412 large lakes (area >20 km2) across China. S. Wang et al. (2020) applied water color parameters (Forel–Ule index and hue angle) to MODIS data (2000–2017) and obtained SDD data for 153 lakes (>25 km2) across China. Zhang et al. (2021) built a simple power function model based on the Landsat red band (2016–2018) to investigate the spatial distribution of SDD in 641 lakes (≥10 km2) across China. In addition, other investigations of the spatiotemporal variations in SDD have been made using MODIS data for lakes in the Yangtze Plain (50 lakes, >10 km2; L. Feng et al., 2019) and in the Tibetan Plateau (64 lakes, >50 km2; Pi et al., 2020). In these studies, the empirical models exhibited better ability than other models to estimate SDD at large scales.
In this study, we tuned a recently developed SDD empirical model which has been demonstrated as effective to map the spatial–temporal dynamics of SDD in surface waters based on atmospherically corrected Landsat reflectance products in GEE (Song et al., 2020). The overall purpose of this study was to map the spatiotemporal variation in SDD in lakes (>0.01 km2) across China from 1984 to 2018. Specifically, the objectives were to (1) built a lake SDD estimation model across China based on extensive in situ measurements, (2) derive SDD of lakes across China using Landsat data embedded in GEE, and (3) analyze the interannual variability in SDD at the lake regions' scale and the individual lake scale. Such research provides valuable information regarding water quality conditions and can inform future water resource planning and management.
China is a vast and physiographically diverse country endowed with a large number of lakes. Based on broad regional variations of landforms and climate characteristics, the lakes in China have been grouped into five regions (Ma et al., 2011) (Fig. 1a). The Inner Mongolia–Xinjiang lake region (MXR) and Tibetan–Qinghai Plateau lake region (TQR) are located in arid or semiarid climates, while the northeastern lake region (NLR), Yungui Plateau lake region (YGR) and eastern lake region (ELR) are situated in the Asian monsoon climate zone. The MXR and TQR have lower annual precipitation, lower temperature and a higher evaporation level than other three lake regions. Regionally, the lakes' distribution sourced from Song et al. (2020) is as follows (in decreasing order): 49 % in the ELR, 22 % in the NLR, 18 % in the YGR, 8 % in the MXR and 4 % in the TQR (Fig. 1b). However, on the basis of lake surface area, regional distribution is slightly different and is in the following order: TQR (41 %) > ELR (30 %) > MXR (14 %) > NLR (10 %) > ELR (6 %) (Fig. 1b). The lakes in the plateau region with higher elevation are less affected by human activities and generally exhibit better ecological conditions than lakes in the other regions (Zhang et al., 2019). In contrast, the lakes in the plain regions are frequently influenced by anthropogenic activities, such as urbanization, population growth, agricultural fertilizer and wastewater discharge (L. Feng et al., 2019; Tong et al., 2020).
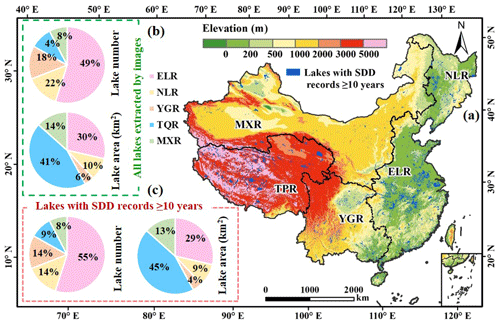
Figure 1The geographical distribution of lakes with water clarity (SDD) records of more than 10 years (lake area >0.01 km2; N=11 336) in different lake regions across China (a). The percentage distribution of lakes, based on the number of lakes and lake surface area in the five lake regions, is shown in the pie charts. The left one (green box) is based on all lakes extracted from Landsat images (b), while the lower left corner one (red box) is based on lakes with SDD records of more than 10 years (c).
3.1 Water body mask
Following Song et al. (2020), the lake boundaries (lakes and reservoirs) with area >0.01 km2 across China were derived from Landsat 8 Operational Land Imager (OLI) images mainly acquired in 2016, and detailed description of boundary extraction is available in that study. However, some lakes in China have changed substantially over time. These lakes were dealt with separately to obtain their boundaries in each year during the period 1984–2018. To obtain the information of lake area variation (e.g., size and year), we referred to an analysis on multi-decadal lake area (≥1 km2 in size) changes in China from the 1960s to 2015 (Zhang et al., 2019) (Fig. S1 in the Supplement). The datasets of lake boundaries (1960s–2020) have been released by the National Tibetan Plateau Data Centre. As for the reservoirs, we mainly viewed and compared the Landsat natural color images on the website of Earthdata Search (https://search.earthdata.nasa.gov/, last access: 5 January 2022) and historical images embedded in Google Earth to confirm the changing region, respectively. For the small lakes with area <1 km2 obtained from the study of Song et al. (2020), we assumed their boundaries remained unchanged during the study period.
We extracted the boundaries of these changing lakes using Landsat images during 1984–2018. The cloudless top-of-atmosphere (TOA) image of each path and row was downloaded from the GEE platform and processed to obtain the modified normalized difference water index (MNDWI) as follows:
where Rrc, Green and Rrc, SWIR are the Rayleigh scattering reflectance in the green band and shortwave infrared (SWIR) band, respectively. First, we used the MNDWI, combined with tasseled cap transformation (TC) and a density slicing with multi-threshold approach, to build a decision tree for extracting water body boundaries using the ENVI software package (Rokni et al., 2014; Xu, 2006). Then, Landsat images acquired during 1984–2018 were classified into water and non-water areas (Feyisa et al., 2014; X. Wang et al., 2020). The extracted water bodies were subsequently converted into polygons with contiguous pixels and stored in shape file format using ArcGIS 10.4 (Esri Inc., Redlands, CA, USA). According to the shoreline features, we divided water bodies into lakes, reservoirs and rivers. By referring to the Global Reservoir and Dam database (Lehner et al., 2011), Chinese Reservoirs and Dams database (Song et al., 2018b), and high-resolution images from Google Earth, we distinguished rivers and reservoirs from water bodies mainly by visual interpretation. The shape file of lakes and reservoirs (herein lakes) was used as a water mask to extract the SDD map derived from the Landsat imageries (Fig. 1a).
The impact of land contamination on water remains a challenge in terms of accurately retrieving water quality parameters (Jensen, 2006; Hou et al., 2017; D. Liu et al., 2020; S. Wang et al., 2020). Jensen (2006) pointed out that the different surface objects have different reflectances to the NIR band. For instance, land and vegetation can largely reflect the NIR band strongly absorbed by water, especially for shallow lakes or reservoirs. In our study, a 1-pixel (2-pixel) buffer inward of water boundary was removed for lakes with an area ≤1 km2 (>1 km2) in order to avoid the influence of adjacent land on water bodies that can result in mixed land–water pixels. The determination of the number of pixels buffered was referenced to the method proposed in the study of Wang et al. (2018), who made a comparison of water-leaving reflectance in the transects selected from the land–water boundaries to identify a suitable buffer distance. This method has been demonstrated to be effective in other studies related to SDD estimation (D. Liu et al., 2020; S. Wang et al., 2020).
3.2 SDD in situ data collection across China
We used three SDD datasets for model calibration and validation (Fig. 2a). To assemble the first dataset (IGA-04-19), we conducted 37 field campaigns from April 2004 to September 2018; surveyed 361 water bodies; and collected 2293 samples from lakes and reservoirs across China (Table S1 in the Supplement), most of which were collected in late summer and early autumn. The second dataset was assembled from field campaigns (2007–2009) conducted by researchers from the Nanjing Institute of Geography and Limnology, Chinese Academy of Sciences. The third dataset (229 samples) was collected by different research groups during the 1980s–1990s and included records for which the data collection date was not available. The spatial distribution of these three groups samples is shown in Fig. 2a. At each station, Secchi disk depth (SDD, in cm) was determined to represent water clarity and was taken as the depth from the water surface where a black–white Secchi disk can no longer be seen under water. For the first two datasets, SDD data derived from field surveys (2004–2018) were matched with the TOA reflectance data collected by Landsat satellites overpassing a lake/reservoir within 7 d of a field site visit, and the average reflectance of pixels within a 3×3 window matching a sampling point was extracted for bands 1–5 (Kloiber et al., 2002). After matching the in situ SDD with images, altogether, 1301 and 340 pairs of data were obtained based on the first and second SDD datasets, respectively. For the third dataset, the cloud-free TOA images whose dates were closest to the time recorded on the lake survey reports were selected to match the measured SDD, which were between May and October during the period of field survey. Finally, 229 matchups were found by expanding the time window between the third dataset of SDD and images.
3.3 Acquisition and processing of Landsat imagery data
To track the dynamics of lake SDD in the past 35 years, all available Landsat Thematic Mapper (TM)/Enhanced Thematic Mapper Plus (ETM+)/OLI images of TOA across China were used in this study (∼82 000 images, >60 TB of data) via the GEE platform. The number of images used for the SDD estimate in a specific year spanned a large range, from 371 in 1984 to 4784 in 2018 (Fig. 2b), with more images available when two satellites operated simultaneously in space to acquire Landsat imagery. In this study, based on the GEE platform, the TOA images were mainly collected during the ice-free season (May to October) from 1984 to 2018 in the TQR, MXR, NLR and ELR but not in the YGR (from January to December) due to lack of good-quality images. The pixel_qa band, as a pixel quality control band generated from the CFMask algorithm, was selected to mask out the land and snow/ice and to remove cloud contamination (cloud cover >60 %) on the GEE platform, thus minimizing the potential impact of cloud on SDD estimation accuracy. Landsat imagery atmospheric correction is a key step for water quality inversion (Wang et al., 2009), particularly for monitoring of temporal variation at a large scale. The TOA products within GEE were produced using the equations developed by Chander et al. (2009), and the function of these equations was to convert calibrated digital numbers to absolute units of TOA reflectance. A description of Landsat TOA products is available on the GEE platform (https://developers.google.com/earth-engine/datasets/catalog/landsat, last access: 5 January 2022). More than 98.35 % of the pixels within China had a total of qualified observations >35 in the past 35 years, and the majority of images had more than three scenes of good observations for each year.
3.4 Model for SDD estimation and mapping in GEE
Model development was a key step in this study. For the first matchup dataset, i.e., 1301 pairs of in situ SDD and TOA, we divided the valid data into four groups, with three groups used to calibrate the model (N=976) and one group (N=325) used for model validation. Based on a previous investigation, the red and blue (or green) band ratio was found to improve the performance of reflectance-based water quality models in terms of both their spatial and their temporal transferability (Kloiber et al., 2002; Olmanson et al., 2008; Song et al., 2020). Thus, by trying the band combination, the red blue band ratio algorithm using the first matched dataset was employed in this study to map SDD of water bodies and was mathematically expressed as
Then, combining the aforementioned image-processing methods, Eq. (1) was applied to the TOA images from 1984 to 2018 to estimate the SDD in the lakes with an area >0.01 km2 over China via the GEE platform. The annual mean SDDs at the pixel scale were obtained by averaging all available estimated results, and then the lake-based annual mean SDDs were further worked out. During the calculations, we only took into consideration lakes with SDD results of more than 10 years. Finally, 10 814 lakes (size >0.01 km2) were examined for the interannual dynamics of SDD (Fig. 1c).
3.5 Statistical analysis
The SDD estimation model performance was assessed using the determination coefficient (R2), RMSE, relative RMSE (rRMSE) and mean absolute error (MAE).
where N refers to the number of water samples, i refers to the current water sample number, Yobserved, i refers to the in situ SDD measurements, refers to the average of observed Y and Yestimated, i refers to the estimated SDD from the Landsat data.
Once the annual mean SDD maps were generated, the average SDD for each pixel within a lake was calculated for the observation period (1984–2018). For each lake region and individual lake, the spatiotemporal dynamics in SDD were analyzed, including the variations in the average, changing trend, number of lakes and lake surface area. The interannual changing trend was assessed at the 5 % significance level and the slope from linear regression analysis between SDD values and years. These analyses were conducted with the IBM SPSS software. Based on the analysis of interannual change trend in SDD, the lakes in China were divided into three types – lakes with SDD showing significant increasing (Type I, p<0.05 and slope >0), significant decreasing (Type II, p<0.05 and slope <0) and non-significant (Type III, p>0.05) trends from 1984–2018.
The estimation model of lake SDD across China was built using three-quarters of the first matched dataset (976 samples), for which the R2, RMSE, rRMSE and MAE were 0.79, 100.3 cm, 61.9 % and 57.7 cm, respectively (Fig. 3a). Then, we used 325 samples (one-quarter of the first matched dataset) to validate the model, and the validation results indicated stable performance by showing comparative errors (R2=0.80; RMSE =92.7 cm; rRMSE =57.6 %; MAE =54.9 cm; Fig. 3b). Further, the second and the third datasets were both used to validate model performance with a major focus on testing the temporal transferability of the model (Fig. 3c, d). The second dataset (340 samples), collected as part of the Chinese lakes survey conducted by the Nanjing Institute of Geography and Limnology, also indicated a good model performance (R2=0.78; RMSE =74.7 cm; rRMSE =59.1 %; MAE =42.6 cm; Fig. 3c). The third dataset (229 samples) was assembled by the first lake surveys conducted in the 1980s and was used to validate the model performance for SDD derived from historical remotely sensed data. Our results also demonstrated a stable performance for lake SDD before the 1990s (R2=0.81; RMSE =61.8 cm; rRMSE =50.6 %; MAE =40.3 cm; Fig. 3d). Comparison of validation results for these different periods and datasets demonstrated the stable performance of the SDD model (Fig. 3). Therefore, the estimation of SDD using images acquired by Landsat series of sensors provides a reliable method to examine historical trends in SDD through time series analysis.
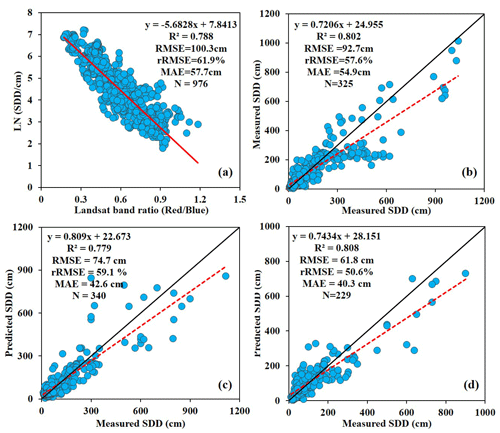
Figure 3Model calibration and validation for SDD estimation with the Landsat TOA reflectance product acquired by different Landsat sensors: (a) model calibration with three-quarters of the total number of samples from the first dataset, (b) model validated with one-quarter of the total number of samples from the first dataset, (c) model validated with the second dataset independently collected during the limnological survey (2007–2009) and (d) model validated with the third dataset collected in the first lake environmental survey during 1985–1990.
Figure 4a shows the spatial distribution of the annual mean SDD of lakes across China in 2018, demonstrating remarkable spatial variation, with lakes in the plateau regions generally exhibiting higher SDD than those situated in the flat plain regions. Based on their mean SDD, all lakes across China in 2018 were divided into six levels, i.e., <0.5, 0.5–1, 1–2, 2–3, 3–4 and >4 m, with 26.4 %, 25.7 %, 28.8 %, 12.5 %, 4.3 % and 2.3 % of lakes in each SDD level, respectively (Fig. 4b). Although the number of lakes with SDD <2 m was more numerous (80.9 % of lakes), the total area of lakes with SDD of <0.5 and >4 m was the largest, accounting for 24 % and 24.3 % of the total area in each category, respectively (Fig. 4c).
Regarding the annual mean SDD in the five lake regions, the top three regions were the TQR (3.37 m), YGR (2.35 m) and MXR (1.92 m), followed by the ELR (1.50 m) and NLR (0.69 m) (Fig. 4d). Except for the YGR, lakes with SDD <2 m were most common, accounting for 96 % (NLR), 82.8 % (ELR), 80.5 % (MXR) and 77.6 % (TQR) of all lakes in the other regions, respectively (Fig. 4e). In the YGR, the lakes with SDD in the 1–3 m range had a wide distribution, and the total proportion of lakes with SDD <3 m was 85.4 % in this region (Fig. 4e). Spatially, the lakes were widely scattered over the ELR, except for the northern and western sections of that region (i.e., northern and southern of Hebei province, northeast of Henan province, northwest of Shandong province, and west of Hubei and Hunan provinces). The lakes in the NLR were located in the northwest and southwest of the region. In the YGR, the lakes were clustered in the southern and northeast of the region (i.e., mid-east of Sichuan province and most of Yunnan and Guangxi provinces). A large number of lakes were inventoried in the TQR, including a collection of large lakes situated in the mid-west and eastern sections of the region, particularly in northwest Tibet and in the western and eastern sections of Qinghai province. In the MXR, the lakes were mainly distributed in the mid-east and mid-west of Inner Mongolia and parts of the western and northern Xinjiang Uygur Autonomous Region.
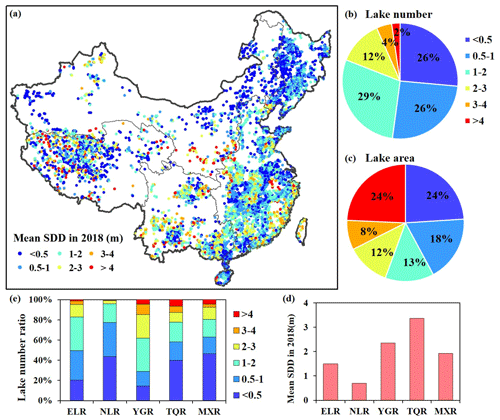
Figure 4Annual mean SDD of lakes (>0.01 km2) across China in 2018. (a) Spatial distribution of lakes with SDD values. (b) Proportion of the lake number with SDD values for six levels (i.e., <0.5, 0.5–1, 1–2, 2–3, 3–4 and >4 m). (c) Proportion of the lake area for six SDD levels. (d) Annual mean SDDs in the five lake regions. (e) Proportion of the lake number at different SDD levels in the five lake regions.
6.1 Temporal average and trend in lake SDD
Similarly to the spatial pattern of SDD estimates obtained in 2018, the multi-year average SDD values in each lake region also revealed similar trends; i.e., the lakes located in the plateau region were more transparent than lakes from other physiographic regions (Fig. 5a). During 1984–2018, the lakes in the NLR exhibited the lowest SDD (mean 0.60±0.09 m), followed by the ELR (mean 1.23±0.17 m). The MXR showed intermediate SDD values (mean 1.63±0.38 m), and the YGR exhibited higher SDD (mean 2.35±0.21 m). Lakes in the TQP had the clearest water (mean SDD 3.32±0.38 m; Fig. 5a). As shown in Fig. 5a, mean annual SDD estimates in the five lake regions were in agreement with in situ-measured SDD.
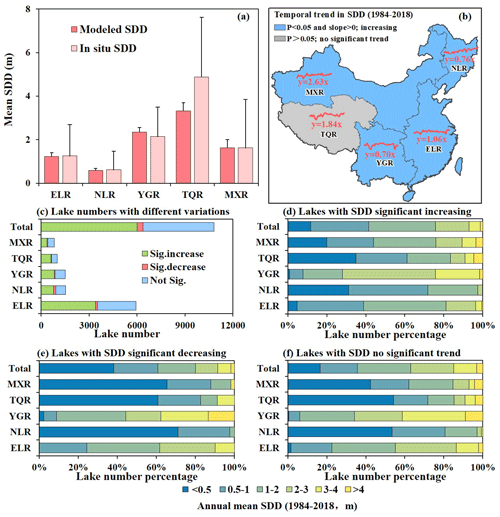
Figure 5The interannual dynamics of lake SDDs in China during 1984–2018. (a) Multi-year average SDD values of the modeled and in situ SDDs in the five lake regions. (b) Interannual trends of mean lake SDDs in five lake regions based on the 5 % significant level and slope representing the coefficient of simple linear regression. (c) Number of lakes with SDD showing statistically significant (p<0.05) increasing (Type I) and decreasing (Type II) trends and non-significant (Type III) trends. Proportions of lake numbers with different SDD values (<0.5, 0.5–1, 1–2, 2–3, 3–4 and >4 m) for (d) lakes with SDD showing significant increasing trend, (e) lakes with SDD showing significant decreasing trend and (f) lakes with SDD showing no significant trend.
Regarding the interannual change trend, with the exception of the TQR, results for the other four lake regions indicated a significant (p<0.05) increasing trend in SDD during the study period (Fig. 5b). At the scale of individual lakes, 55.4 % (5993 out of 10 814) and 3.5 % (377 out of 10 814) of lakes experienced statistically significant (p<0.05) increasing and decreasing trends, respectively, and the remaining lakes (41.1 %, 4444 out of 10 814) displayed no significant change (Fig. 5c). Among the five lake regions, except for the MXR, more than half of all lakes exhibited significant increasing trends (Fig. 5c). Ranked by the total number of lakes exhibiting a significant increase in SDD, the lake regions can be ordered as follows: TQR (61.7 %, 618 out of 1002), ELR (57.1 %, 3396 out of 5943), YGR (54.6 %, 829 out of 1517) and NLR (51.3 %, 784 out of 1528). As for the lakes with decreasing SDD values, the NLR had the highest number of such lakes (8.4 %, 128 out of 1528) followed by the MXR (7 %, 58 out of 824) (Fig. 5c).
Among the three types of lake – lakes with SDD showing significant increasing (Type I), significant decreasing (Type II) and non-significant (Type III) trends from 1984 to 2018, the lake SDDs in Type I, Type II and Type III were mainly concentrated in 0.5–3, 0–2 and 0–3 m, respectively; the corresponding proportions were 81.11 % (4861 out of 5993), 80.11 % (302 out of 377) and 85.13 % (3783 out of 4444) of the total number of lakes, respectively (Fig. 5d–f). At the five lake regions' scale, regardless of the lake type, the distributions of lake SDDs in the NLR, TQR and MXR appeared similar, while those in the ELR and YGR differed from these three lake regions. The former group was mainly distributed in 0–2 m; the latter ranged 0.5–3 m (ELR) and 1–4 m (YGR), respectively (Fig. 5d–f).
6.2 Lake SDDs versus lake sizes in China
The annual mean SDD and lake area were both separated into six levels, and the proportions of lakes with different areas in each SDD category are shown in Fig. 6. In terms of the number of different lake areas in the five lake regions, the lakes with annual mean SDD values in the ELR, NLR and YGR were dominated by the area range of 0.01–1 km2, followed by that of 1–10 km2. In the MXR, the lakes were mainly dominated by the area range of 1–10 km2, followed by that of 0.01–1 km2 (Fig. 6a–f). In the TQR, when the SDDs were <2 m, the lakes covering the area range of 1–10 km2 were in the majority (Fig. 6a–c); when the SDDs were >2 m, the lakes with the area range >10 km2 occupied a dominant position, especially for lakes with the area range of 10–50 and 100–500 km2 (Fig. 6d–f).
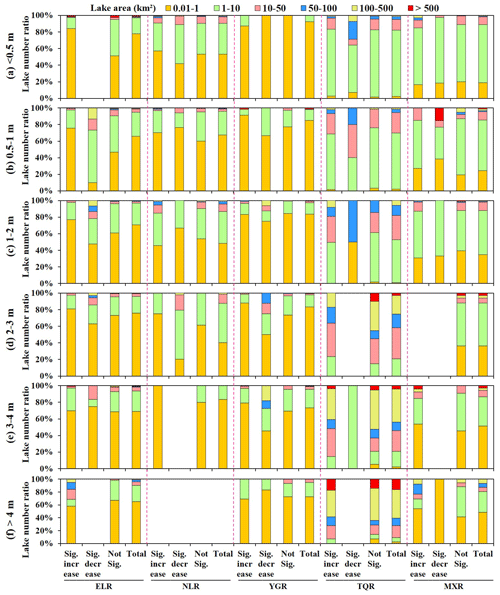
Figure 6Proportions of lake numbers in different areas in the six SDD categories. The six SDD categories are (a) <0.5 m, (b) 0.5–1 m, (c) 1–2 m, (d) 2–3 m, (e) 3–4 m and (f) >4 m. The SDD values are the average of estimated results in each lake during 1984–2018. In the five lake regions, the lakes are further divided into three types – lakes with SDD showing significant increasing (Type I), significant decreasing (Type II) and non-significant (Type III) trends during 1984–2018.
Among the three types of lake in each SDD category, there is a similarity in the distribution of lakes with different sizes between Type I and Type III, while that of Type II was differentiated from these two types of lake (Fig. 6). In the ELR, NLR and YGR, more than 50 % of the lakes ranged 0.01–1 km2 among the lakes of Type I and Type III. The lakes of Type II, located in the three lake regions and with SDD values of 0.5–1 m in the ELR and of <0.5 and 2–3 m in the NLR, were dominated by the area size of 1–10 km2, while the remaining lakes were mostly with the area range of 0.01–1 km2 (Fig. 6a–f). In the MXR, the number of lakes covering the area range of 1–10 km2 across the three types of lake was much larger than that of other sizes among the lakes with SDDs in the range 0–3 m (Fig. 6a–d). When the lake SDDs were >3 m in this lake region, most of the three types of lake were dominated by the lakes covering the area range of 0.01–1 km2, apart from the lakes of Type III with SDD values >4 m, where the proportion of lakes with the area range of 1–10 km2 was slightly higher than that with the area range of 0.01–1 km2 (Fig. 6e–f).
The distribution of the three types of lake with different lake sizes in the TQR differed from those in the other four lake regions. For the lakes of Type I and Type III in the TQR, when the SDDs ranged 0–2 m, the proportions of lakes covering the area range of 1–10 km2 were the largest, somewhere between 49.64 % and 81.12 % (Fig. 6a–c). When the SDDs ranged 2–3 m, the lakes with the area range of 10–50 km2 in Type I and of 100–500 km2 in Type III had the largest proportions of numbers, accounting for 40.43 % and 35.00 %, respectively (Fig. 6d). When the SDDs exceeded 3 m, the lakes covering the area range of 100–500 km2 were dominant in the two types of lake, followed by the area range of 10–50 km2 (Fig. 6e–f). For the lakes of Type II in the TQR, the lakes with SDDs in the <0.5 m category were distributed in the area range of 10–50 km2, followed by that of 50–100 km2 (Fig. 6a). When SDDs were in the 0.5–1 m category, the numbers of lakes with the area range of 1–10 and 10–50 km2 were the largest, where the corresponding percentages were 40.00 % (Fig. 6b). When SDDs were in the 1–2 m category, there were two kinds of lake whose areas were in the range of 0.01–1 and 50–100 km2, and their numbers were the same (Fig. 6c). When SDDs were in the 3–4 m category, only the lakes with the area range of 1–10 km2 existed (Fig. 6e).
6.3 Spatial distribution of lakes with different SDD values
The spatial distributions of lakes and the number of lakes and areas of the three types of lake in five lake regions are presented in Fig. 7. In the SDD of <0.5 m category (Fig. 7a), the NLR had the largest lake numbers and areas of the three types of lake, accounting for 34.51 % and 33.20 % in Type I, 63.19 % and 48.17 % in Type II, and 44.46 % and 34.38 % in Type III of the number of lakes and areas in the lake region, respectively. Spatially, the lakes in Type I and Type III were mainly distributed in the central section of the ELR, the western section of the NLR, the mid-west of the TQR and the mid-east of the MXR, while those in Type II were concentrated on the western section of the NLR and eastern section of the MXR.
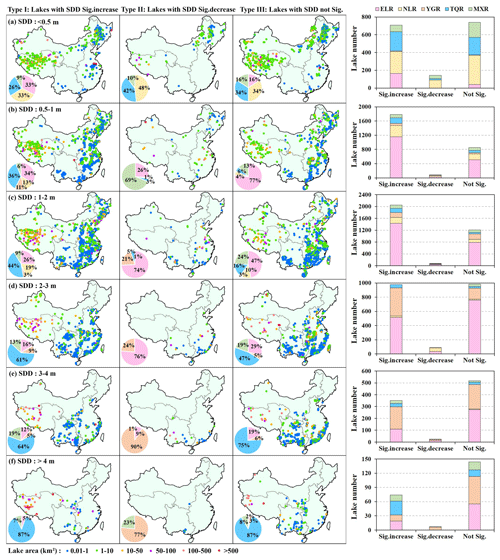
Figure 7Spatial distribution of lakes with multi-year average SDD values during 1984–2018. The SDD values were divided into six levels: (a) <0.5 m, (b) 0.5–1 m, (c) 1–2 m, (d) 2–3 m, (e) 3–4 m and (f) >4 m. The lakes were separated into three types of lake – lakes with SDD showing significant increasing (Type I), significant decreasing (Type II) and non-significant (Type III) trends during 1984–2018. Proportions of total lake area and lake number in each lake region are shown in the pie charts and histograms, respectively.
In the SDD of the 0.5–3 m categories (Fig. 7b–d), the lakes of Types I and III were the most numerous in the ELR, but the largest total lake areas of the five lake regions were different between these two types of lake. Specifically, in the lakes of Type I, the total lake areas in the TQR were the largest, with percentages of 36.38 % (SDD 0.5–1 m), 44.14 % (SDD 1–2 m) and 61.03 % (SDD 2–3 m), respectively (Fig. 7b–d). Regarding the lakes of Type III, the ELR (TQR) had the largest proportion of lake area when SDD was 0.5–2 m (2–3 m). The percentages of lake area when SDD was 0.5–2 m in the ELR were 76.80 % (SDD 0.5–1 m) and 46.90 % (SDD 1–2 m), while that in the TQR was 46.65 % (SDD 2–3 m) (Fig. 7b–d). For the lakes of Type II, the region that had the largest proportions of lake number and area was inconsistent in each SDD category (0.5–3) m. When the SDDs were in the range of 0.5–1 m, the NLR had the largest lake number, while the MXR had the highest percentage of lake area (Fig. 7b). When the SDDs ranged from 1–2 m, the number of lakes and area in the ELR were the largest (Fig. 7c). When the SDDs were around 2–3 m, the lake number in the NLR was the largest and the total lake area in the ELR was the largest (Fig. 7d). Spatially, lake distributions of Types I and III with the SDD range of 0.5–2 m were concentrated in most places of the ELR, the northwest and southeast of the NLR, the southern section of the YGR, the mid-west of the TQR, and the mid-east and the northern section of the MXR (Fig. 7b–c). When these two types of lake SDD were in the range of 2–3 m, they were distributed in the central and southeast coast of the ELR, the central and southwest of the YGR, and the western section of the TQR (Fig. 7d). For Type II of lakes with SDD falling in the range 0.5–3 m, their distributions were scattered over part of the central and southeast coast of the ELR and southwest of the YGR (Fig. 7b–d).
In the SDD of 3–4 m category (Fig. 7e), the regions that had the most lakes for each of the three types of lake were the YGR (Type I, 53.56 %), ELR (Type II, 48.00 %) and ELR (Type III, 53.19 %), respectively. The regions that had the largest lake area were the TQR (Type I, 63.51 %), YGR (Type II, 90.06 %) and TQR (Type III, 75.22 %), respectively. Spatially, the lakes of Types I and III were concentrated at the junction of the ELR, YGR and MXR; the southeast coast of the ELR; the southern section of the YGR; and the western section of the TQR. The lakes of Type III were mainly distributed in the part of the southeast coast of the ELR and the southern section of the YGR.
Regarding the SDD of >4 m category (Fig. 7f), the TQR had the largest lake number and area in the lakes of Type I, accounting for 39.19 % of the number of lakes and 87.34 % of the total lake area. For the lakes of Type II, a few lakes existed in the MXR and YGR. For the lakes of Type III, the YGR had the most lakes and the TQR had the largest total lake area, accounting for 40.28 % of the number of lakes and 87.00 % of the total lake area, respectively. Spatially, the distributions of these lakes were similar to those of the lakes with an SDD range of 3–4 m.
Several past studies have examined the spatiotemporal variation in SDD in lakes across China (or parts of China), but these investigations were mainly based on MODIS images to estimate SDD in large lakes (>10 km2) and primarily focused on the period after 2000 (L. Feng et al., 2019; D. Liu et al., 2020; Pi et al., 2020; S. Wang et al., 2020). Therefore, it becomes a challenge to compare these past results with the results of the present study due to differences in the period of interest, the resolution of the satellite images and lake size (>0.01 km2 in our study). Zhang et al. (2021) adopted an empirical model to retrieve the SDD of lakes (>10 km2) across China based on Landsat surface reflectance products (2016–2018) within GEE. Because of the similarity of methods and images used in Zhang et al. (2021) and the present study, there is a unique opportunity to compare lake SDD estimation models across China proposed by these two studies. To that end, we used available in situ SDD data (2019–2020) collected at monitoring stations in Lake Taihu and Lake Dianchi to assess the accuracy of the two models. As shown in Fig. 8 and demonstrated by statistical parameters (higher R2, lower RMSE, rRMSE and MAE), the estimation model proposed by our study exhibited better performance to retrieve SDD in both Lake Taihu (Fig. 8c) and Lake Dianchi (Fig. 8d).
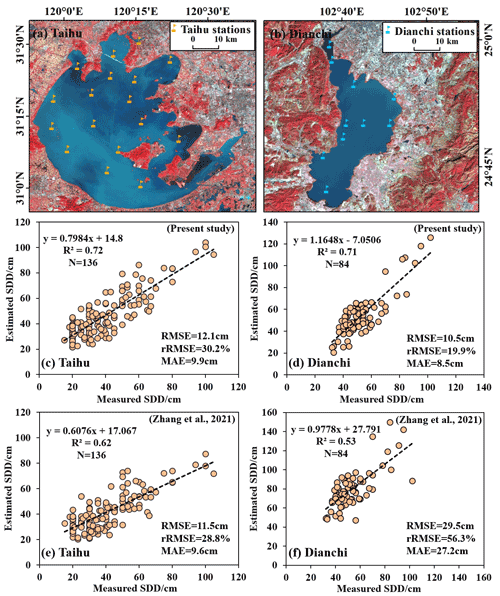
Figure 8Comparison of different SDD estimation models based on Landsat images within GEE. (a, b) Spatial distribution of monitoring stations located in Lake Taihu and Lake Dianchi, respectively. The Landsat 8 OLI images used in these two panels come from the Geospatial Data Cloud site, Computer Network Information Center, Chinese Academy of Sciences (http://www.gscloud.cn, last access: 5 January 2022). (c–f) The regression line between the measured SDD in Lake Taihu (N=136) and Lake Dianchi (N=84) during 2019–2020 and estimated SDD values that were obtained from the estimation models developed in this study and Zhang et al. (2021), respectively.
While previous studies have demonstrated the application of Landsat series data (Landsat 5 TM/Landsat 7 ETM+/Landsat 8 OLI) and the proposed model can provide accurate long-term coverage of the SDD of lakes in China (Zhang et al., 2021; Song et al., 2020; Deutsch et al., 2018; Bonansea et al., 2015; McCullough et al., 2013), several systemic errors in SDD results could not be avoided. On the one hand, the SDD estimation model proposed in this study contained some errors, and the model validation yielded the following results: R2=0.80; RMSE =92.7 cm; rRMSE =57.6 %; MAE =54.9 cm. On the other hand, different atmospheric correction methods can have diverse effects on the Landsat images (Bonansea et al., 2015; Lee et al., 2016). The calibrated TOA reflectance products within GEE were produced using the equations developed by Chander et al. (2009). Nevertheless, these systemic errors do not significantly affect the overall trends of the SDD of lakes in China (Bonansea et al., 2015; Deutsch et al., 2018; Zhang et al., 2021). In addition, under the influence of climate change or human activities, such as floods and droughts, urbanization, and farmland reclamation, the boundaries for some small lakes (<1 km2) may vary greatly, which could cause the uncertainty in SDD estimation (Yang and Huang, 2021; Zhang et al., 2019). This is a limitation of the assumption for small lakes with static boundaries. In the future, further research on the relationship between the area of small lakes and the accuracy of SDD simulation would aid in addressing this limitation.
The dataset of the water clarity of lakes developed in this study consists of one shapefile containing the annual mean values of water clarity in each lake (size >0.01 km2) during 1990–2018, with a temporal resolution of 5 years. The dataset can now be accessed through the website of the National Tibetan Plateau Data Center (http://data.tpdc.ac.cn/en/, last access: 5 January 2022) at https://doi.org/10.11888/Hydro.tpdc.271571 (Tao et al., 2021).
As a comprehensive indicator of water eutrophication, encompassing nutrient enrichment, algal abundance and suspended sediment, water clarity can serve as a valuable index for tracking the ecological health of aquatic ecosystems and guiding the actions of water resource managers. Although field measurement of water clarity can easily be made with a Secchi disk apparatus, this approach is not suitable for long-term time series measurements of lake water clarity at regional and national scales. This information is highly valuable and can be extracted from archived satellite data. In situ water clarity data collected in lakes across China during 2004–2018 were used to calibrate and validate SDD models that incorporate top-of-atmosphere reflectance products and Google Earth Engine to map the spatiotemporal dynamics of SDD over a 35-year time span (1984–2018). The SDD model was validated using different datasets, and results confirmed the stable performance and temporal transferability of the SDD estimation model. Derived SDD estimates were analyzed at the lake region and at the individual lake scales. During the study period (1984–2018), annual mean SDD values in the TQR, YGR, MXR, ELR and NLR were 3.32±0.38, 2.35±0.21, 1.63±0.38, 1.23±0.17 and 0.60±0.09 m, respectively. Among the 10 814 lakes with >10 years of SDD results, 55.4 % and 3.5 % experienced statistically significant (p<0.05) increasing and decreasing trends of water clarity, respectively. The remaining lakes (41.1 %) displayed no significant trends. With the exception of the MXR, more than half of lakes in all the other regions exhibited a significant trend of increasing water clarity. In the ELR, NLR and YGR, most of the lakes displaying either an increase or a decrease in SDD tended to be of 0.01–1 km2 in size, whereas in the TQR and MXR, lakes exhibiting clear trends in SDD were mostly large lakes (>10 km2). Spatially, the lakes in the plateau regions (TQR, YGR) generally exhibited higher SDD than those situated in the flat plain region. The time series of water clarity information presented in this study could aid local, regional and national decision-making on policies and management for protecting/improving inland water quality in China. The research approach implemented could also potentially be used to map water clarity in lakes at the global scale, an effort that could provide useful information for evaluating decadal trends in surface water quality resulting from the adoption of pollution control policies.
The supplement related to this article is available online at: https://doi.org/10.5194/essd-14-79-2022-supplement.
KaS, HT, GL and HD designed the study; KaS, GL and HT performed the research; KaS, HT, GL, QW, ZhW, ZoW, DL, KS, BZ and XW collected and analyzed the data; KaS, HT, GL, PAJ and HD wrote the paper. All authors contributed to the interpretation of findings, helped revise the manuscript and approved the final manuscript for submission.
The contact author has declared that neither they nor their co-authors have any competing interests.
Publisher’s note: Copernicus Publications remains neutral with regard to jurisdictional claims in published maps and institutional affiliations.
This article is part of the special issue “Extreme environment datasets for the three poles”. It is not associated with a conference.
The authors wish to thank Ying Zhao, Jianhang Ma and Ming Wang for their capable assistance in the field sampling and laboratory measurements.
This research was jointly supported by the National Natural Science Foundation of China (grant nos. 41730104, 42001311, 42171385), China Postdoctoral Science Foundation (grant no. 2020M681056), Strategic Priority Research Program of the Chinese Academy of Sciences (grant no. XDA19070501), Research Instrument and Equipment Development Project of the Chinese Academy of Sciences (grant no. YJKYYQ20190044), and National Earth System Science Data Center of China (http://www.geodata.cn/, last access: 5 January 2022).
This paper was edited by Min Feng and reviewed by two anonymous referees.
Amani, M., Ghorbanian, A., Ahmadi, S. A., Kakooei, M., Moghimi, A., Mirmazloumi, S. M., Moghaddam, S. H. A., Mahdavi, S., Ghahremanloo, M., Parsian, S., Wu, Q., and Brisco, B.: Google Earth Engine Cloud Computing Platform for Remote Sensing Big Data Applications: A Comprehensive Review, IEEE J. Sel. Top. Appl., 13, 5326–5350, https://doi.org/10.1109/jstars.2020.3021052, 2020.
Bonansea, M., Ledesma, C., Rodriguez, C., Pinotti, L., and Antunes, M. H.: Effects of atmospheric correction of Landsat imagery on lake water clarity assessment, Adv. Space Res., 56, 2345–2355, https://doi.org/10.1016/j.asr.2015.09.018, 2015.
Cao, Z., Duan, H., Feng, L., Ma, R., and Xue, K.: Climate- and human-induced changes in suspended particulate matter over Lake Hongze on short and long timescales, Remote Sens. Environ., 192, 98–113, https://doi.org/10.1016/j.rse.2017.02.007, 2017.
Carlson, R. E.: A Trophic State Index for Lakes, Limnol. Oceanogr., 22, 361–369, https://doi.org/10.4319/lo.1977.22.2.0361, 1977.
Chander, G., Markham, B. L., and Helder, D. L.: Summary of current radiometric calibration coefficients for Landsat MSS, TM, ETM+, and EO-1 ALI sensors, Remote Sens. Environ., 113, 893–903, https://doi.org/10.1016/j.rse.2009.01.007, 2009.
Deutsch, E. S., Alameddine, I., and El-Fadel, M.: Monitoring water quality in a hypereutrophic reservoir using Landsat ETM plus and OLI sensors: how transferable are the water quality algorithms?, Environ. Monit. Assess., 190, 141, https://doi.org/10.1007/s10661-018-6506-9, 2018.
Doron, M., Babin, M., Mangin, A., and Hembise, O.: Estimation of light penetration, and horizontal and vertical visibility in oceanic and coastal waters from surface reflectance, J. Geophys. Res., 112, C06003, https://doi.org/10.1029/2006jc004007, 2007.
Duan, H., Ma, R., Zhang, Y., and Zhang, B.: Remote-sensing assessment of inland lake water clarity in northeast China, Limnology, 10, 135–141, https://doi.org/10.1007/s10201-009-0263-y, 2009.
Feng, L., Hou, X. J., and Zheng, Y.: Monitoring and understanding the water transparency changes of fifty large lakes on the Yangtze Plain based on long-term MODIS observations, Remote Sens. Environ., 221, 675–686, https://doi.org/10.1016/j.rse.2018.12.007, 2019.
Feng, S. L., Liu, S. G., Huang, Z. H., Jing, L., Zhao, M. F., Peng, X., Yan, W. D., Wu, Y. P., Lv, Y. H., Smith, A. R., McDonald, M. A., Patil, S. D., Sarkissian, A. J., Shi, Z. H., Xia, J., and Ogbodo, U. S.: Inland water bodies in China: Features discovered in the long-term satellite data, P. Natl. Acad. Sci. USA, 116, 25491–25496, https://doi.org/10.1073/pnas.1910872116, 2019.
Feyisa, G. L., Meilby, H., Fensholt, R., and Proud, S. R.: Automated Water Extraction Index: A new technique for surface water mapping using Landsat imagery, Remote Sens. Environ., 140, 23–35, https://doi.org/10.1016/j.rse.2013.08.029, 2014.
Giardino, C., Brando, V. E., Dekker, A. G., Strombeck, N., and Candiani, G.: Assessment of water quality in Lake Garda (Italy) using Hyperion, Remote Sens. Environ., 109, 183–195, https://doi.org/10.1016/j.rse.2006.12.017, 2007.
Gordon, H. R., Clark, D. K., Brown, J. W., Brown, O. B., Evans, R. H., and Broenkow, W. W.: Phytoplankton pigment concentrations in the middle Atlantic Bight–comparison of ship determinations and CZCS estimates, Appl. Optics, 22, 20–36, https://doi.org/10.1364/ao.22.000020, 1983.
Han, D., Currell, M. J., and Cao, G.: Deep challenges for China's war on water pollution, Environ. Pollut., 218, 1222–1233, https://doi.org/10.1016/j.envpol.2016.08.078, 2016.
Hou, X., Feng, L., Duan, H., Chen, X., Sun, D., and Shi, K.: Fifteen-year monitoring of the turbidity dynamics in large lakes and reservoirs in the middle and lower basin of the Yangtze River, China, Remote Sens. Environ., 190, 107–121, https://doi.org/10.1016/j.rse.2016.12.006, 2017.
Huang, J. C., Gao, J. F., and Zhang, Y. J.: Eutrophication Prediction Using a Markov Chain Model: Application to Lakes in the Yangtze River Basin, China, Environ. Model. Assess., 21, 233–246, https://doi.org/10.1007/s10666-015-9472-4, 2016.
Jensen, J.: Remote Sensing of the Environment: An Earth Resource Perspective, Prentice Hall, Upper Saddle River, New Jersey, 2006.
Kloiber, S. N., Brezonik, P. L., Olmanson, L. G., and Bauer, M. E.: A procedure for regional lake water clarity assessment using Landsat multispectral data, Remote Sens. Environ., 82, 38–47, https://doi.org/10.1016/s0034-4257(02)00022-6, 2002.
Lee, Z., Shang, S., Hu, C., Du, K., Weidemann, A., Hou, W., Lin, J., and Lin, G.: Secchi disk depth: A new theory and mechanistic model for underwater visibility, Remote Sens. Environ., 169, 139–149, https://doi.org/10.1016/j.rse.2015.08.002, 2015.
Lee, Z., Shang, S. L., Qi, L., Yan, J., and Lin, G.: A semi-analytical scheme to estimate Secchi-disk depth from Landsat-8 measurements, Remote Sens. Environ., 177, 101–106, https://doi.org/10.1016/j.rse.2016.02.033, 2016.
Lehner, B. and Doll, P.: Development and validation of a global database of lakes, reservoirs and wetlands, J. Hydrol., 296, 1–22, https://doi.org/10.1016/j.jhydrol.2004.03.028, 2004.
Lehner, B., Liermann, C. R., Revenga, C., Voeroesmarty, C., Fekete, B., Crouzet, P., Doell, P., Endejan, M., Frenken, K., Magome, J., Nilsson, C., Robertson, J. C., Roedel, R., Sindorf, N., and Wisser, D.: High-resolution mapping of the world's reservoirs and dams for sustainable river-flow management, Front. Ecol. Environ., 9, 494–502, https://doi.org/10.1890/100125, 2011.
Liu, D., Duan, H., Loiselle, S., Hu, C., Zhang, G., Li, J., Yang, H., Thompson, J. R., Cao, Z., Shen, M., Ma, R., Zhang, M., and Han, W.: Observations of water transparency in China's lakes from space, Int. J. Appl. Earth Obs. Geoinf., 92, 102187, https://doi.org/10.1016/j.jag.2020.102187, 2020a.
Liu, G., Li, L., Song, K., Li, Y., Lyu, H., Wen, Z., Fang, C., Bi, S., Sun, X., Wang, Z., Cao, Z., Shang, Y., Yu, G., Zheng, Z., Huang, C., Xu, Y., and Shi, K.: An OLCI-based algorithm for semi-empirically partitioning absorption coefficient and estimating chlorophyll a concentration in various turbid case-2 waters, Remote Sens. Environ., 239, 111648, https://doi.org/10.1016/j.rse.2020.111648, 2020b.
Ma, R., Yang, G., Duan, H., Jiang, J., Wang, S., Feng, X., Li, A., Kong, F., Xue, B., Wu, J., and Li, S.: China's lakes at present: Number, area and spatial distribution, Sci. China-Earth Sci., 54, 283–289, https://doi.org/10.1007/s11430-010-4052-6, 2011.
Ma, T., Zhao, N., Ni, Y., Yi, J. W., Wilson, J. P., He, L. H., Du, Y. Y., Pei, T., Zhou, C. H., Song, C., and Cheng, W. M.: China's improving inland surface water quality since 2003, Sci. Adv., 6, eaau3798, https://doi.org/10.1126/sciadv.aau3798, 2020.
McCullough, I. M., Loftin, C. S., and Sader, S. A.: Combining lake and watershed characteristics with Landsat TM data for remote estimation of regional lake clarity, Remote Sens. Environ., 123, 109–115, https://doi.org/10.1016/j.rse.2012.03.006, 2012.
McCullough, I. M., Loftin, C. S., and Sader, S. A.: Landsat imagery reveals declining clarity of Maine's lakes during 1995–2010, Freshw. Sci., 32, 741–752, https://doi.org/10.1899/12-070.1, 2013.
Olmanson, L. G., Bauer, M. E., and Brezonik, P. L.: A 20-year Landsat water clarity census of Minnesota's 10,000 lakes, Remote Sens. Environ., 112, 4086–4097, https://doi.org/10.1016/j.rse.2007.12.013, 2008.
Olmanson, L. G., Brezonik, P. L., and Bauer, M. E.: Evaluation of medium to low resolution satellite imagery for regional lake water quality assessments, Water Resour. Res., 47, W09515, https://doi.org/10.1029/2011wr011005, 2011.
Pekel, J.-F., Cottam, A., Gorelick, N., and Belward, A. S.: High-resolution mapping of global surface water and its long-term changes, Nature, 540, 418–422, https://doi.org/10.1038/nature20584, 2016.
Pi, X., Feng, L., Li, W., Zhao, D., Kuang, X., and Li, J.: Water clarity changes in 64 large alpine lakes on the Tibetan Plateau and the potential responses to lake expansion, ISPRS J. Photogramm. Remote Sens., 170, 192–204, https://doi.org/10.1016/j.isprsjprs.2020.10.014, 2020.
Qin, B., Zhu, G., Gao, G., Zhang, Y., Li, W., Paerl, H. W., and Carmichael, W. W.: A Drinking Water Crisis in Lake Taihu, China: Linkage to Climatic Variability and Lake Management, Environ. Manage., 45, 105–112, https://doi.org/10.1007/s00267-009-9393-6, 2010.
Richardson, T. L., Lawrenz, E., Pinckney, J. L., Guajardo, R. C., Walker, E. A., Paerl, H. W., and MacIntyre, H. L.: Spectral fluorometric characterization of phytoplankton community composition using the Algae Online Analyser®, Water Res., 44, 2461–2472, https://doi.org/10.1016/j.watres.2010.01.012, 2010.
Rokni, K., Ahmad, A., Selamat, A., and Hazini, S.: Water Feature Extraction and Change Detection Using Multitemporal Landsat Imagery, Remote Sens., 6, 4173–4189, https://doi.org/10.3390/rs6054173, 2014.
Rosenzweig, B. R., Smith, J. A., Baeck, M. L., and Jaffe, P. R.: Monitoring Nitrogen Loading and Retention in an Urban Stormwater Detention Pond, J. Environ. Qual., 40, 598–609, https://doi.org/10.2134/jeq2010.0300, 2011.
Shen, M., Duan, H., Cao, Z., Xue, K., Qi, T., Ma, J., Liu, D., Song, K., Huang, C., and Song, X.: Sentinel-3 OLCI observations of water clarity in large lakes in eastern China: Implications for SDG 6.3.2 evaluation, Remote Sens. Environ., 247, 111950, https://doi.org/10.1016/j.rse.2020.111950, 2020.
SOEE: Report on the State of the Ecology and Environment of China in 2018, Environmental Publishing House, Beijing, 13 pp., 2018.
Song, K., Wen, Z., Shang, Y., Yang, H., Lyu, L., Liu, G., Fang, C., Du, J., and Zhao, Y.: Quantification of dissolved organic carbon (DOC) storage in lakes and reservoirs of mainland China, J. Environ. Manage., 217, 391–402, 2018a.
Song, K., Wen, Z., Xu, Y., Hong, Y., Lyu, L., Ying, Z., Chong, F., Shang, Y.,Jia, D.: Dissolved carbon in a large variety of lakes across five limnetic regions in China, J. Hydrol., 563, 143–154, 2018b.
Song, K., Liu, G., Wang, Q., Wen, Z., Lyu, L., Du, Y., Sha, L., and Fang, C.: Quantification of lake clarity in China using Landsat OLI imagery data, Remote Sens. Environ., 243, 111800, https://doi.org/10.1016/j.rse.2020.111800, 2020.
Tao, H., Song, K., Liu, G., Wang, Q., and Wen, Z.: Water clarity annual dynamics dataset across China (1990–2018), National Tibetan Plateau Data Center [data set], https://doi.org/10.11888/Hydro.tpdc.271571, CSTR: 18406.11.Hydro.tpdc.271571, 2021 (data vailable at: http://data.tpdc.ac.cn/en/, last access: 5 January 2022).
Tong, Y., Zhang, W., Wang, X., Couture, R.-M., Larssen, T., Zhao, Y., Li, J., Liang, H., Liu, X., Bu, X., He, W., Zhang, Q., and Lin, Y.: Decline in Chinese lake phosphorus concentration accompanied by shift in sources since 2006, Nat. Geosci., 10, 507–511, https://doi.org/10.1038/ngeo2967, 2017.
Tong, Y., Wang, M., Penuelas, J., Liu, X., Paerl, H. W., Elser, J. J., Sardans, J., Couture, R. M., Larssen, T., Hu, H., Dong, X., He, W., Zhang, W., Wang, X., Zhang, Y., Liu, Y., Zeng, S., Kong, X., Janssen, A. B. G., and Lin, Y.: Improvement in municipal wastewater treatment alters lake nitrogen to phosphorus ratios in populated regions, P. Natl. Acad. Sci. USA, 117, 11566–11572, https://doi.org/10.1073/pnas.1920759117, 2020.
Tranvik, L. J., Downing, J. A., Cotner, J. B., Loiselle, S. A., Striegl, R. G., Ballatore, T. J., Dillon, P., Finlay, K., Fortino, K., Knoll, L. B., Kortelainen, P. L., Kutser, T., Larsen, S., Laurion, I., Leech, D. M., McCallister, S. L., McKnight, D. M., Melack, J. M., Overholt, E., Porter, J. A., Prairie, Y., Renwick, W. H., Roland, F., Sherman, B. S., Schindler, D. W., Sobek, S., Tremblay, A., Vanni, M. J., Verschoor, A. M., von Wachenfeldt, E., and Weyhenmeyer, G. A.: Lakes and reservoirs as regulators of carbon cycling and climate, Limnol. Oceanogr., 54, 2298–2314, https://doi.org/10.4319/lo.2009.54.6_part_2.2298, 2009.
Wang, M., Son, S., and Shi, W.: Evaluation of MODIS SWIR and NIR-SWIR atmospheric correction algorithms using SeaBASS data, Remote Sens. Environ., 113, 635–644, https://doi.org/10.1016/j.rse.2008.11.005, 2009.
Wang, S., Li, J., Zhang, B., Lee, Z., Spyrakos, E., Feng, L., Liu, C., Zhao, H., Wu, Y., Zhu, L., Jia, L., Wan, W., Zhang, F., Shen, Q., Tyler, A. N., and Zhang, X.: Changes of water clarity in large lakes and reservoirs across China observed from long-term MODIS, Remote Sens. Environ., 247, 111949, https://doi.org/10.1016/j.rse.2020.111949, 2020.
Wang, S. L., Li, J. S., Zhang, B., Spyrakos, E., Tyler, A. N., Shen, Q., Zhang, F. F., Kutser, T., Lehmann, M. K., Wu, Y. H., and Peng, D. L.: Trophic state assessment of global inland waters using a MODIS-derived Forel-Ule index, Remote Sens. Environ., 217, 444–460, https://doi.org/10.1016/j.rse.2018.08.026, 2018.
Wang, X., Guo, X., Yang, C., Liu, Q., Wei, J., Zhang, Y., Liu, S., Zhang, Y., Jiang, Z., and Tang, Z.: Glacial lake inventory of high-mountain Asia in 1990 and 2018 derived from Landsat images, Earth Syst. Sci. Data, 12, 2169–2182, https://doi.org/10.5194/essd-12-2169-2020, 2020.
Wetzel, R. G.: Limnology: Lake and River Ecosystems, 3rd edn., Academic Press, San Diego, USA, 2001.
Xu, H.: Modification of normalised difference water index (NDWI) to enhance open water features in remotely sensed imagery, Int. J. Remote Sens., 27, 3025–3033, https://doi.org/10.1080/01431160600589179, 2006.
Yang, J. and Huang, X.: The 30 m annual land cover dataset and its dynamics in China from 1990 to 2019, Earth Syst. Sci. Data, 13, 3907–3925, https://doi.org/10.5194/essd-13-3907-2021, 2021.
Yang, X. and Lu, X.: Drastic change in China's lakes and reservoirs over the past decades, Sci. Rep.-UK, 4, 6041, https://doi.org/10.1038/srep06041, 2014.
Zhang, G., Yao, T., Chen, W., Zheng, G., Shum, C. K., Yang, K., Piao, S., Sheng, Y., Yi, S., Li, J., O'Reilly, C. M., Qi, S., Shen, S. S. P., Zhang, H., and Jia, Y.: Regional differences of lake evolution across China during 1960s-2015 and its natural and anthropogenic causes, Remote Sens. Environ., 221, 386–404, https://doi.org/10.1016/j.rse.2018.11.038, 2019.
Zhang, Y., Zhang, Y., Shi, K., Zhou, Y., and Li, N.: Remote sensing estimation of water clarity for various lakes in China, Water Res., 192, 116844–116844, https://doi.org/10.1016/j.watres.2021.116844, 2021.
- Abstract
- Introduction
- Study area
- Methods
- Validation of SDD estimation model
- Spatial distribution of SDD in lakes in 2018
- Interannual dynamics of lake SDD during 1984–2018
- Comparison with past studies and uncertainties
- Data availability
- Conclusions
- Author contributions
- Competing interests
- Disclaimer
- Special issue statement
- Acknowledgements
- Financial support
- Review statement
- References
- Supplement
- Abstract
- Introduction
- Study area
- Methods
- Validation of SDD estimation model
- Spatial distribution of SDD in lakes in 2018
- Interannual dynamics of lake SDD during 1984–2018
- Comparison with past studies and uncertainties
- Data availability
- Conclusions
- Author contributions
- Competing interests
- Disclaimer
- Special issue statement
- Acknowledgements
- Financial support
- Review statement
- References
- Supplement