the Creative Commons Attribution 4.0 License.
the Creative Commons Attribution 4.0 License.
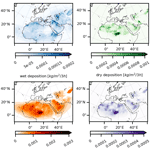
The MONARCH high-resolution reanalysis of desert dust aerosol over Northern Africa, the Middle East and Europe (2007–2016)
Enza Di Tomaso
Jerónimo Escribano
Sara Basart
Paul Ginoux
Francesca Macchia
Francesca Barnaba
Francesco Benincasa
Pierre-Antoine Bretonnière
Arnau Buñuel
Miguel Castrillo
Emilio Cuevas
Paola Formenti
María Gonçalves
Oriol Jorba
Martina Klose
Lucia Mona
Gilbert Montané Pinto
Michail Mytilinaios
Vincenzo Obiso
Miriam Olid
Nick Schutgens
Athanasios Votsis
Ernest Werner
Carlos Pérez García-Pando
One of the challenges in studying desert dust aerosol along with its numerous interactions and impacts is the paucity of direct in situ measurements, particularly in the areas most affected by dust storms. Satellites typically provide column-integrated aerosol measurements, but observationally constrained continuous 3D dust fields are needed to assess dust variability, climate effects and impacts upon a variety of socio-economic sectors. Here, we present a high-resolution regional reanalysis data set of desert dust aerosols that covers Northern Africa, the Middle East and Europe along with the Mediterranean Sea and parts of central Asia and the Atlantic and Indian oceans between 2007 and 2016. The horizontal resolution is 0.1∘ latitude × 0.1∘ longitude in a rotated grid, and the temporal resolution is 3 h. The reanalysis was produced using local ensemble transform Kalman filter (LETKF) data assimilation in the Multiscale Online Nonhydrostatic AtmospheRe CHemistry model (MONARCH) developed at the Barcelona Supercomputing Center (BSC). The assimilated data are coarse-mode dust optical depth retrieved from the Moderate Resolution Imaging Spectroradiometer (MODIS) Deep Blue Level 2 products. The reanalysis data set consists of upper-air variables (dust mass concentrations and the extinction coefficient), surface variables (dust deposition and solar irradiance fields among them) and total column variables (e.g. dust optical depth and load). Some dust variables, such as concentrations and wet and dry deposition, are expressed for a binned size distribution that ranges from 0.2 to 20 µm in particle diameter. Both analysis and first-guess (analysis-initialized simulation) fields are available for the variables that are diagnosed from the state vector. A set of ensemble statistics is archived for each output variable, namely the ensemble mean, standard deviation, maximum and median. The spatial and temporal distribution of the dust fields follows well-known dust cycle features controlled by seasonal changes in meteorology and vegetation cover. The analysis is statistically closer to the assimilated retrievals than the first guess, which proves the consistency of the data assimilation method. Independent evaluation using Aerosol Robotic Network (AERONET) dust-filtered optical depth retrievals indicates that the reanalysis data set is highly accurate (mean bias = −0.05, RMSE = 0.12 and r = 0.81 when compared to retrievals from the spectral de-convolution algorithm on a 3-hourly basis). Verification statistics are broadly homogeneous in space and time with regional differences that can be partly attributed to model limitations (e.g. poor representation of small-scale emission processes), the presence of aerosols other than dust in the observations used in the evaluation and differences in the number of observations among seasons. Such a reliable high-resolution historical record of atmospheric desert dust will allow a better quantification of dust impacts upon key sectors of society and economy, including health, solar energy production and transportation. The reanalysis data set (Di Tomaso et al., 2021) is distributed via Thematic Real-time Environmental Distributed Data Services (THREDDS) at BSC and is freely available at http://hdl.handle.net/21.12146/c6d4a608-5de3-47f6-a004-67cb1d498d98 (last access: 10 June 2022).
- Article
(9175 KB) - Full-text XML
-
Supplement
(5416 KB) - BibTeX
- EndNote
Desert (or mineral) dust is the most abundant aerosol by mass in the global atmosphere (Textor et al., 2006) and plays a key role in the Earth system (Knippertz and Stuut, 2014). It is emitted from the surface by aeolian processes an doriginates predominantly – but not only – from desert regions. Dust affects weather and climate by perturbing the radiative balance directly through scattering and absorption of solar and thermal radiation (Pérez et al., 2006; Boucher et al., 2019; Miller et al., 2014) and indirectly by altering cloud formation and cloud chemistry (Cziczo et al., 2013; Harris et al., 2013; Kiselev et al., 2017). It also contributes to the fertilization of the ocean (Jickels et al., 2005; Kanakidou et al., 2018) and the land (Yu et al., 2015; Rizzolo et al., 2017) through the deposition of iron and phosphorus, thus affecting the global carbon cycle. All in all, the amount of, spatial distribution of and variability in desert dust have implications on climate, the environment, air quality (Rodríguez et al., 2001; Pey et al., 2013; Barnaba et al., 2017) and human health (Mallone et al., 2011; Morman and Plumlee, 2013; Pérez García-Pando et al., 2014; Pandolfi et al., 2014; Terradellas et al., 2015; Stafoggia et al., 2016; Querol et al., 2019), and a variety of socio-economic sectors such as aviation and solar energy production (Schroedter-Homscheidt et al., 2013; Votsis et al., 2020). Due to the nature of its emission and transport and its relatively short lifetime (Gliß et al., 2021), dust varies strongly in space and time, which requires continuous monitoring both in situ and remotely by satellite, airborne and ground-based sensors (Barnaba and Gobbi, 2004; Kaufman et al., 2005; Marticorena et al., 2010; Kim et al., 2011; Mona et al., 2012; Pey et al., 2013; Luo et al., 2015). A major challenge in studying desert dust along with its impacts is the paucity of direct in situ measurements in the regions most affected by dust storms. There are some operational visibility observations providing qualitative estimates of dust presence (Mahowald et al., 2007), but there is a severe lack of routine surface aerosol concentration measurements (Benedetti et al., 2018). In addition to the lack of in situ observations, there is limited information on aerosol speciation, which is essential to distinguish dust from other aerosol types (Rodríguez et al., 2012). Satellites mostly provide column-integrated aerosol information, but spatially and temporally resolved surface dust concentration and deposition estimates are needed to enable detailed impact assessments. Dust observations or retrievals are therefore best exploited in combination with model simulations either to provide optimal initial conditions (analyses) to forecast models (Benedetti et al., 2014) or to monitor current and past states of the atmosphere through the production of reanalyses, i.e. complete and consistent four-dimensional reconstructions of the atmosphere.
There are several available global aerosol reanalyses that include desert dust, such as MERRA-2 (Modern-Era Retrospective analysis for Research and Applications, Version 2; Gelaro et al., 2017; Randles et al., 2017; Buchard et al., 2017) and CAMSRA (Copernicus Atmosphere Monitoring Service Reanalysis; Inness et al., 2019) along with their predecessors MERRAero (Modern-Era Retrospective analysis for Research and Applications Aerosol Reanalysis; Buchard et al., 2015) and MACC-II (Monitoring Atmospheric Composition and Climate-II; Inness et al., 2013; Cuevas et al., 2015), respectively, and the JRAero (Japanese Reanalysis for Aerosol; Yumimoto et al., 2017) and the NAAPS (Navy Aerosol Analysis and Prediction System; Lynch et al., 2016) reanalyses. These global data sets have been produced at relatively coarse spatial resolution and by assimilating total aerosol optical depth (AOD). MERRA-2 is NASA's latest reanalysis. It has been produced at a spatial resolution of 0.58∘ latitude × 0.6258∘ longitude, with 72 hybrid eta layers and by assimilating bias-corrected, neural-network-retrieved AOD from the Moderate Resolution Imaging Spectroradiometer (MODIS) and from the Advanced Very High Resolution Radiometer (AVHRR; over ocean only), as well as AOD from the Multi-angle Imaging SpectroRadiometer (MISR; over bright surfaces only) and from the Aerosol Robotic Network (AERONET) of Sun photometers. The latest reanalysis for atmospheric composition produced by the Copernicus Atmosphere Monitoring Service (CAMS) CAMSRA covers the period January 2003 to 2020 and is extended by adding 1 year each year. It has been produced at a spatial resolution of ∼ 80 km and with 60 hybrid sigma–pressure levels in the vertical, by assimilating Collection 6 MODIS AOD produced with Deep Blue (DB; over land) and Dark Target (over land and ocean) algorithms and by additionally assimilating the Advanced Along-Track Scanning Radiometer (AATSR) AOD from 2003 to March 2012. JRAero is a global 5-year (2011–2015) reanalysis product constructed by the Meteorological Research Institute of the Japan Meteorological Agency. It has been produced assimilating the MODIS 6-hourly Level 3 AOD product provided by the US Naval Research Laboratory (NRL) and the University of North Dakota (UND) for the purpose of aerosol data assimilation and is based on the NASA operational MODIS Level 2 Collection 5 (Dark Target) AOD data set. This same data set has been previously used, together with MISR AOD, by NRL to produce the NAAPS 11-year (2003–2013) global gridded aerosol reanalysis product at a resolution of 1∘ latitude × 1∘ longitude.
At the European level, air quality regional reanalyses (including dust) are produced by nine different operational systems and the associated multi-model ensemble through the CAMS regional services of the Copernicus programme. These models assimilate surface observations of O3, SO2, NO2 and CO and particulate matter (PM2.5 and PM10) operationally, and one of the models additionally assimilates AOD in research mode. These products are restricted to an extended European domain, which excludes major desert dust sources in Northern Africa and the Middle East. These reanalyses are produced as an improved product compared to the daily CAMS analyses, by using the latest validated observations, but we note they may not be consistent over the different production periods as they are not necessarily produced with the same model version.
We present here a regional reanalysis focusing specifically on desert dust aerosols that overcomes some of the potential limitations of existing global and regional reanalysis products. The data set was obtained by combining satellite remote sensing dust retrievals with a dynamical model. It spans a 10-year period, from 2007 to 2016; has a horizontal resolution of 0.1∘ latitude × 0.1∘ longitude in a rotated grid; and has 3-hourly output. It provides a regional reconstruction of past dust conditions across Northern Africa, the Middle East and Europe, including the Mediterranean Sea and parts of central Asia, and the Atlantic and Indian oceans. The reanalysis consists of a set of dust geophysical variables (and their uncertainties) produced with a consistent model and data assimilation scheme, i.e. a frozen version of the code used during the whole simulation period, including emission schemes, input data sets and the retrieval algorithm for the assimilated observations. This ensures the production of a consistent data set avoiding the introduction of spurious trends that could be associated with model or assimilation changes.
We have adopted an ensemble-based data assimilation scheme for the estimation of the dust analysis. The use of ensemble model simulations has allowed for the estimation of flow-dependent background uncertainty, which is otherwise difficult to estimate due to the highly varying nature of dust concentrations. Assimilating AOD may not necessarily constrain individual aerosol components because the aerosol attribution in the analysis increments is typically determined by the model first guess (Tsikerdekis et al., 2021). To at least partly overcome this limitation, we have directly assimilated dust retrievals, namely satellite-derived coarse-mode dust optical depth (DODcoarse) at 550 nm over land surfaces, including bright surfaces such as desert areas. The assimilated retrievals are based on the MODIS DB algorithm (Hsu el al., 2013; Sayer et al., 2013), which uses measurements at different wavelengths with a different contrast between the surface and atmospheric aerosols. In particular, the algorithm capitalizes on the much lower surface reflectance at ultraviolet wavelengths than at longer wavelengths.
This new reanalysis data set can be used to support the provision of climate services and monitoring. It can also contribute to the development of dust impact mitigation strategies. For instance, the design of the reanalysis output fields has been tailored to the specific needs in three socio-economic sectors affected by mineral dust, which are air quality and health, energy production, and transport. In addition to the 3D fields of dust mass concentration, the reanalysis data set includes dust extinction and deposition variables, along with other variables associated with meteorology and radiation. In summary, we present here a regional dust reanalysis at an unprecedented resolution using for the first time specific dust retrievals over dust source regions and including grid-level uncertainty estimates.
The following sections describe the different aspects related to the production of the reanalysis: the dust modelling aspect, including the dust sources and emission schemes is outlined in Sect. 2; the generation of ensemble perturbations to best characterize model uncertainty is explained in Sect. 3; the assimilated dust retrievals and the data assimilation scheme are described in Sects. 4 and 5, respectively. Additionally, Sect. 6 describes the details of the reanalysis simulation settings, while Sect. 7 describes the content and structure of the reanalysis data set. Section 8 provides an evaluation of the column-integrated dust optical depth (DOD) and DODcoarse in terms of geographical distribution, the study of analysis increments, data assimilation inner diagnostics and comparison against independent observations. Information about the data set availability is provided in Sect. 9. Finally, conclusions are drawn in Sect. 10.
Hunt et al., 2007; Schutgens et al., 2010; Di Tomaso et al., 2017(Ginoux et al., 2010, 2012; Pu and Ginoux, 2016)Pérez et al., 2011; Klose et al., 2021(Marticorena and Bergametti, 1995)(Ginoux et al., 2001)(Kok et al., 2014)Kok (2011)(Nonhydrostatic Multi-scale Model on the B grid; Janjic and Gall, 2012)(Dee el al., 2011)(Gelaro et al., 2017)(Hersbach et al., 2020)(Iacono et al., 2008)(Hess et al., 1998)(Gonçalves et al., 2022)(Ferrier et al., 2002)(Janjic, 1994, 1996b)(Ek et al., 2003)(Janjic, 1996a, 2001)(Betts, 1986; Betts and Miller, 1986; Janjic, 1994, 2000)The reanalysis has been produced using the Multiscale Online Nonhydrostatic AtmospheRe CHemistry model (MONARCH; Pérez et al., 2011; Haustein et al., 2012; Jorba et al., 2012; Spada et al., 2013; Badia et al., 2017; Klose et al., 2021), which consists of advanced chemistry and aerosol packages coupled online with the Nonhydrostatic Multiscale Model on the B grid (NMMB; Janjic et al., 2001; Janjic and Gall, 2012). MONARCH is able to work across a wide range of spatial scales thanks to its unified non-hydrostatic dynamical core. In the global setup, MONARCH is run on a latitude–longitude grid, while the regional version used in this work runs on a rotated latitude–longitude grid. Different physics schemes are available in the NMMB to resolve turbulence, convection, soil, radiation and clouds. The exact configuration used in this work is reported in Table 1, where the key configuration settings are summarized for both modelling and data assimilation aspects.
MONARCH represents the atmospheric dust cycle including emission, transport and deposition along with dust–radiation interactions. A variety of dust emission schemes and configurations are available as described in Klose et al. (2021), ranging from strongly simplified to physics-based parameterizations. Dust transport is produced by horizontal advection, solved with the Adams–Bashforth scheme; vertical advection, solved with the Crank–Nicolson scheme; and lateral diffusion, which follows the Smagorinsky non-linear approach. Furthermore, dust is vertically mixed by turbulent diffusion and deep and shallow convection. Sinks include gravitational settling, dry deposition through turbulent diffusion, and in-cloud and below-cloud scavenging from both stratiform and convective clouds. MONARCH follows a sectional approach for dust, i.e. the size distribution is decomposed into small size bins that range from 0.2 to 20 µm in diameter. The particle size distribution (PSD) at emission either can be chosen from a set of pre-defined PSDs or is calculated online, depending on the selected emission scheme. In this work, we have used a PSD of emitted dust over sources derived from Kok (2011).
A more detailed description of the dust module of MONARCH can be found in Pérez et al. (2011) and Klose et al. (2021), with the latter work including also advances developed after the start of the dust reanalysis production. Those recent developments were therefore not yet used in the present work for which a frozen model version is important. Below we provide further details on the configuration of the emission and radiation schemes used in this work.
2.1 Dust emission schemes
MONARCH contains multiple dust emission schemes, of which we used the following three to generate ensemble perturbations for the production of the reanalysis: (i) a scheme based on Marticorena and Bergametti (1995), hereafter called MB95, which is based on saltation flux and soil texture and was combined with the topographic source mask from Ginoux et al. (2001) as described in Pérez et al. (2011); (ii) the GOCART dust emission scheme from Ginoux et al. (2001) based mainly on a topographic source function, hereafter called G01; (iii) a scheme based on brittle fragmentation by saltation as in Kok et al. (2014), hereafter called K14. The location of dust sources is identified by a climatology of frequency of occurrence (FoO) of DOD greater than 0.2 derived from MODIS DB Collection 6 at the resolution of 0.1∘ latitude × 0.1∘ longitude (Hsu et al., 2004; Ginoux et al., 2012 – see their Sect. 4.3.1) with a minimal threshold for FoO equal to 0.05, below which there is no emission. Surface roughness is accounted for in the dust emission calculation using the drag partition parameterization from Marticorena and Bergametti (1995) with input from MODIS Collection 5 monthly leaf area index for the specific year of simulation from 2007 to 2015 and from a climatology for 2016, combined with a static roughness length for arid regions (Prigent et al., 2012) as described in Klose et al. (2021). The X parameter in the Marticorena and Bergametti (1995) drag partition follows Pierre et al. (2014). The USGS climatological database for vegetation is used by the meteorology and land surface scheme. A soil moisture correction is used for MB95 and K14 as in Fecan et al. (1999) with a revised scaling factor as in Klose et al. (2021) and Zender et al. (2003). G01 uses the default GOCART soil moisture correction, which is based on Belly et al. (1964) as described in Ginoux et al. (2001), and a threshold friction velocity as described in Pérez et al. (2011).
2.2 Radiation and dust optical properties
In MONARCH, dust is coupled online with the RRTMG radiation scheme, which accounts for short-wave (SW) absorption and scattering and long-wave (LW) absorption (Iacono et al., 2008). The input dust optical properties (extinction efficiency, single-scattering albedo and asymmetry factor) for each particle size bin and wavelength are based on refractive indices (RIs) that account for the variation in mineralogical composition by size (Perlwitz et al., 2015a, b; Scanza et al., 2015; Pérez García-Pando et al., 2016) in the SW and derived from the OPAC data set (Hess et al., 1998) in the LW. Optical properties are calculated using Mie scattering theory (Mishchenko et al., 2002) assuming that dust is spherical despite its well-known non-sphericity (Kok et al., 2017). Although MONARCH now allows accounting for the effect of dust non-sphericity upon the optical properties (Klose et al., 2021), this option was not ready by the start of the reanalysis production.
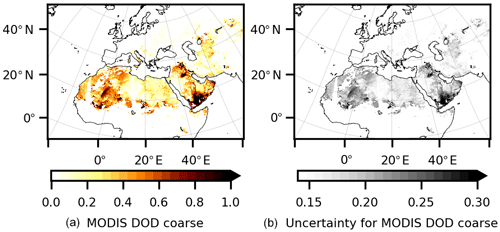
Figure 1Example of assimilated observations for 9 July 2012: DODcoarse retrieved from the Aqua MODIS DB Level 2 products (Collection 6; a) and the associated observation uncertainty used in the assimilation algorithm (b).
To calculate the mineralogy-based size-dependent RIs in the SW, we applied the multi-component Maxwell Garnett theory (Markel, 2016) to internal mixtures of eight dominant dust minerals (Gonçalves et al., 2022) derived from the soil mineralogical atlas of Claquin et al. (1999). The single-mineral RIs were taken from Scanza et al. (2015). The mineral fractions in each size bin are estimated for each of the 28 soil types considered in the atlas based on brittle fragmentation theory (Kok, 2011). For each size bin and wavelength, we finally retain the median real and imaginary RIs across the 28 soil types. In the visible band, the obtained median RIs compare well with recent chamber-based retrievals (Di Biagio et al., 2019) and in situ aircraft measurements (Denjean et al., 2016), as shown in Gonçalves et al. (2022).
The dust–radiation coupling allows the computation of the direct radiative effect at each radiation time step with a simple double-call approach. We also calculate direct normal irradiance (DNI) and global horizontal irradiance (GHI) at the surface, under all-sky conditions, from downward fluxes in ultraviolet–visible–near-infrared bands of the model. While GHI includes direct and diffuse beams collected by a horizontal unit surface, DNI accounts for the direct beam hitting a normal surface. These variables are useful for applications in the context of solar energy production.
We adopted an ensemble-based data assimilation scheme to estimate dust. Hence model uncertainty, expressed as background error covariance in the data assimilation algorithm, is estimated from the realizations of the dust fields in an ensemble of MONARCH model calculations. The use of an ensemble of model simulations allows the estimation of a flow-dependent background uncertainty that would otherwise be difficult to estimate due to the highly variable nature of dust concentrations. We generated a 12-member ensemble using different meteorological initial and boundary conditions and dust emission schemes, along with additional perturbations in the model emission parameters. Such perturbations aim at representing the model uncertainty, mainly in dust emission, which is one of the major contributors to model error (Huneeus et al., 2011), but also in other aspects of the dust cycle where meteorology has a role, such as transport and deposition. The characteristics of each ensemble member are listed in Table S1 in the Supplement and described below.
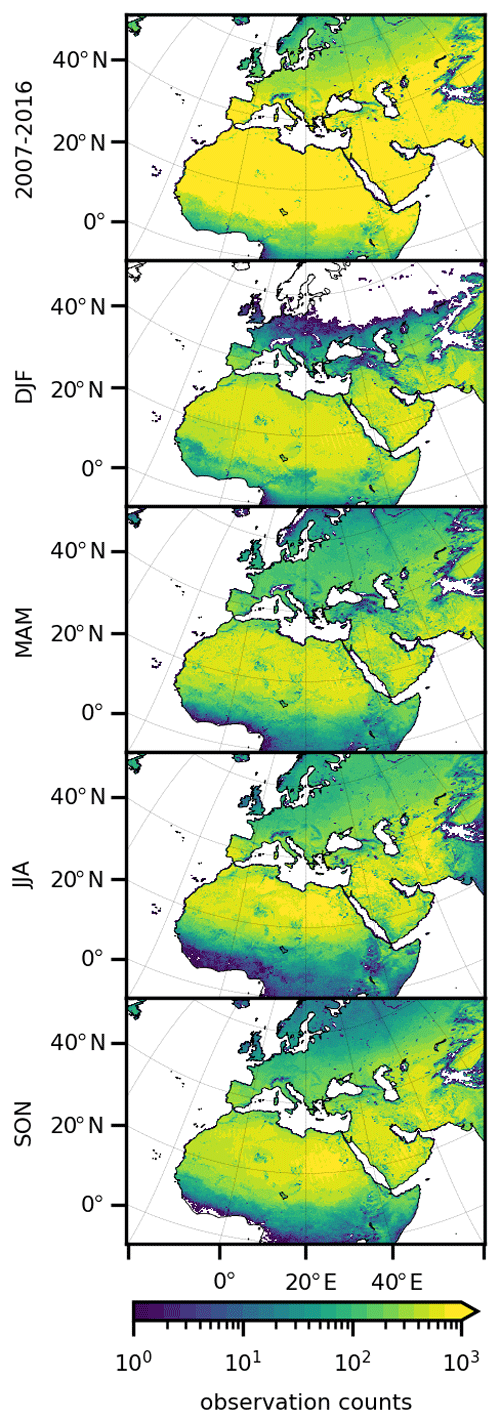
Figure 2Maps of counts of assimilated observations for the whole period (2007–2016; top row) and for the different seasons (DJF, MAM, JJA, SON; rows 2 to 5) of the 10-year period.
The benefit of combining meteorological and aerosol source perturbations is shown in Rubin et al. (2016) and Escribano et al. (2022). The meteorology in our reanalysis is re-initialized every day using global reanalyses. Our ensemble uses two different meteorological reanalyses as initial conditions at the start of every daily run (at 00:00 UTC) and as boundary conditions every 6 h. ERA-Interim (Berrisford et al., 2011; Dee el al., 2011) is used in six ensemble members, and MERRA-2 (Gelaro et al., 2017) together with ERA5 soil information (Hersbach et al., 2020) is used in the remaining six members.
Experiments conducted in Escribano et al. (2021) showed that using different dust emission schemes provides a better characterization of the background covariance than a single scheme with parameter perturbations due to the large variability in the modelled emissions. The ensemble uses three different emission schemes briefly introduced in Sect. 2, namely MB95 (as in Pérez et al., 2011), G01 (as in Ginoux et al., 2001) and K14 (as in Kok et al., 2014). Each emission scheme was used four times (twice in each of the two six-member groups driven by the different meteorological reanalyses). In addition, each of the 12 ensemble members was run with a different value for one or more parameters in the corresponding emission scheme following Di Tomaso et al. (2017). Specifically, we perturbed the threshold friction (or threshold wind velocity for one of the emission schemes), which is soil-moisture-dependent and determines the friction or wind velocity above which soil particles begin to move in saltation, and the dust emission flux across each of the eight dust model bins. The threshold friction or wind velocity was perturbed by drawing a multiplicative random factor from a normal distribution with mean 1 and spread 0.4. The dust emission flux was perturbed imposing a physical constraint. Correlated noise was used across the bins so that noise correlation decreases with increased difference in the normalized cubic radius between the bins; the noise has mean 1 and a standard deviation of 30 % of the unperturbed value in each bin. These emitted size distribution perturbations used here are analogous to those in Fig. 1 in Di Tomaso et al. (2017) but departing from Kok (2011) instead of D'Almeida (1987). The structure of the emission parameter perturbations is temporally and spatially constant.
We have used for assimilation an innovative DOD data set derived from the MODIS DB aerosol products (Collection 6), which covers all cloud-free and snow-free land surfaces. DB aerosol retrievals are available over areas not easily covered by other observational data sets, e.g. very bright reflective surfaces such as deserts, and are therefore particularly relevant for dust applications. The MODIS Dark Target product, for example, has a limited coverage over land since the retrieval algorithm assumes low surface albedo. The DB algorithm uses top-of-the-atmosphere reflectances at 412 and 470 nm, and, in the presence of a heavy dust load, also at 650 nm. It exploits the fact that, over most surfaces, a darker surface and stronger aerosol signal are seen in the blue wavelength range than at longer wavelengths. The quality of the MODIS DB AOD product is improved in Collection 6 compared to previous releases, as shown by the work of Sayer et al. (2014) and Gkikas et al. (2015), based on Level 2 and Level 3 retrievals, respectively. Furthermore, a recent study by Schutgens et al. (2020) showed that DB AOD from MODIS (on board the Aqua satellite) is one of the best products when compared to other satellite products.
More specifically, we have assimilated DODcoarse retrieved from MODIS DB Level 2 aerosol products as described in Ginoux et al. (2010, 2012) and Pu and Ginoux (2016). The generation of the dust retrievals includes the different steps of formatting, dust filtering and retrieval. First, aerosol products such as AOD, single-scattering albedo and the Ångström exponent are interpolated to a regular grid of 0.1∘ latitude × 0.1∘ longitude using the algorithm described by Ginoux et al. (2010). The DOD is then derived from AOD following the methods of Ginoux et al. (2012) with adaptions to MODIS Collection 6 aerosol products. To separate dust from other aerosols, two variables are used: the Ångström exponent, which is highly sensitive to particle size (Ångström, 1929; Eck et al., 1999), and a single-scattering albedo at 412 nm less than 0.95 for dust due to its absorption of solar radiation (Takemura et al., 2002). Subsequently, an empirical continuous function relating the Ångström exponent to fine-mode AOD (Anderson et al., 2005, their Eq. 5) is applied to retrieve the dust fine-mode fraction of optical depth.
Since the retrievals are based on visible reflectances, their availability is limited to the daytime only. The MODIS instrument is on board two NASA polar-orbiting satellites, namely Aqua and Terra. However, we have considered for assimilation only DODcoarse retrievals based on measurements from MODIS on board the Aqua platform. The equatorial crossing local time of the Aqua satellite is at 13:30 in an ascending orbit. In our 3-hourly discretization of the assimilation window, the assimilated observations are associated with the time slot (or interval) centred at 12:00 UTC and, due to the 4D extension of the implemented LETKF scheme, affect the whole assimilation window.
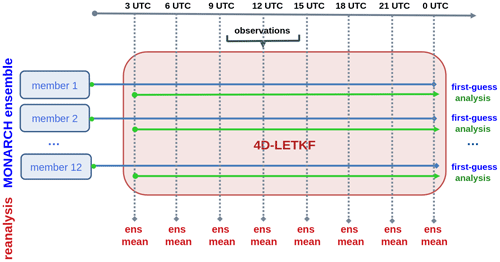
Figure 3Schematic of the 24 h assimilation window for the production of the reanalysis. The ensemble member analyses are used to initialize the corresponding ensemble member first guess in the subsequent simulation/state estimation window.
We have used 0.07 + 0.075 DODcoarse to characterize the observation uncertainty in the assimilated observations, following the linear model of previous studies (Hsu el al., 2013; Sayer et al., 2013) with the coefficients adjusted for our application by inflating the uncertainty for low DODcoarse values, which were otherwise detrimental for the analysis. We have assumed a diagonal observation error covariance matrix, i.e. uncorrelated error between the different retrievals. Observation coordinates were pre-processed to be mapped on the rotated longitude–latitude regional grid of MONARCH. Figure 1 shows an example of the extent of the daily observational coverage on a given date (9 July 2012) together with the associated observational uncertainty.
Maps of observation counts are shown in Fig. 2 for the whole reanalysis period (top row of Fig. 2) and for the different seasons (rows 2 to 5 of Fig. 2), namely the winter seasons represented by December, January and February (DJF); the spring season represented by March, April and May (MAM); the summer season represented by June, July and August (JJA); and the autumn season represented by September, October and November (SON). As expected, there is a higher number of dust retrievals closer to sources than far from them. The total number of retrievals is bigger in the SON and JJA seasons than in the other seasons. During the boreal winter the number of retrievals inland from the Gulf of Guinea increases compared to other times of the year due to transport of dust by northeasterly harmattan winds. The number of dust retrievals decreases in the north of Europe and Asia in the DJF season as MODIS DB covers only snow-free surfaces. Yearly observation counts are consistent throughout the whole period (see Fig. S1 in the Supplement).
The reanalysis was produced using a local ensemble transform Kalman filter (LETKF) data assimilation scheme (Hunt et al., 2007; Miyoshi and Yamane, 2007; Schutgens et al., 2010; Tsikerdekis et al., 2021) coupled to the MONARCH ensemble. We have used an implementation of the LETKF scheme with four-dimensional extension (4D-LETKF) as described in Hunt et al. (2007) in order to estimate the dust analysis over a 24 h assimilation window. The overall scheme implements an iterative approach consisting of a forward simulation of the MONARCH ensemble for 24 h and a state estimation step. The two steps are coupled at each iteration. The state estimation step is an execution of the LETKF which combines information from the dust observations and the model ensemble simulations. The forward simulation of the MONARCH ensemble is named first guess (or background) to indicate a simulation initialized from an analysis and thus incorporates information from past observations. As a result of the estimation step, the analysis is estimated at each assimilation window using both concurrent and past observations.
The LETKF is well suited to computationally demanding calculations such as the estimation of a high-resolution analysis carried out in this work. The analysis at each model grid point can be calculated independently, and at each grid point only observations within a certain distance are assimilated. Furthermore, the use of a dynamic characterization of model background uncertainty, through ensemble forward simulations, is well suited for highly varying dust fields. A detailed description of the scheme can be found in Hunt et al. (2007). Below we discuss the basic concepts behind the LETKF algorithm.
Consider a state vector x of the dynamic variables of a system, in our case the dust mass mixing ratio. The mean analysis increment at a grid point is estimated as a linear combination of the background ensemble perturbations Xb:
where we use the superscripts a and b to denote the analysis and background state vector, respectively, and where the ith column of the matrix Xb is , with k ensemble members (12 in our case), i.e. the difference between the ith ensemble member xb(i) and the ensemble mean . w is termed the “weight” vector, specifying what linear combination of the background ensemble perturbations is added to the background mean to obtain the analysis ensemble. The weight vector is given by
where Yb is the background ensemble perturbation matrix in observation space (or background observation ensemble perturbation matrix); R is the observation error covariance matrix, which we assume is diagonal; I is the identity matrix; yo is the vector of observations; and is the mean background observation ensemble. The background observation ensemble is obtained applying the observation operator h(⋅) to the ensemble members xb(i); i.e. yb(i)=h(xb(i)).
The 4D extension of the algorithm is coded such that background observation means and perturbation matrices Yj are formed at the various time slots j when the observations are available; then they are concatenated to form a combined background observation mean and perturbation matrix Y, where the time slots are the time intervals into which the assimilation window is split. and Y are used for the calculation of a weight vector w using the standard LETKF; i.e. we calculate a single w based on all innovations throughout the day. This same w is then applied to the state vector at different times throughout the assimilation window.
Spatial covariance localization can be applied in the LETKF algorithm through R localization; i.e. the localization is performed in the observation error covariance matrix, making the influence of an observation on the analysis decay gradually towards zero as the distance from the analysis location increases. The use of spatial localization reduces the effect of spurious long-range covariances due to sampling errors produced by a low dimensionality of the ensemble. To achieve this, the observation error is divided by a distance-dependent function that decays to zero with increasing distance: , where dist is the distance in the grid space between an observation and the model grid and l is a horizontal localization factor. The localization factor was set to 15; hence the observation influence practically fades to zero before 30 model grid points away from the observation location (in the horizontal plane).
The control variable is formulated in terms of the total mixing ratio over the five model prognostic variables (corresponding to different dust particle size bins) used to simulate coarse dust in MONARCH. Therefore an observation operator is needed to map the ensemble mean control vector into the observation space. The observation operator has two components: (i) a spatial interpolation of the model simulation to the observation location, which is done at the observation longitude and latitude, and (ii) the calculation of simulated DODcoarse at the wavelength of 550 nm which is calculated using the five coarse model size bins ranging from 1.2 to 20 µm in dust particle diameter. The analysis of the model's fine dust fraction (i.e. the three model size bins from 0.2 to 1.2 µm in dust particle diameter) is estimated proportionally to the change (due to observation assimilation) of the coarse fraction. This choice is motivated by the fact that observations do not carry information about either fine dust particles or particle size distribution. Hereafter, DOD and DODcoarse refer to the wavelength of 550 nm.
This section presents the key settings for the modelling, observational and data assimilation aspects that have been described in Sects. 2 to 5 and that are summarized in Table 1. The reanalysis extends over the period 2007–2016 and covers a regional domain centred around Northern Africa, the Middle East and Europe (hereafter called the NAMEE region) that also includes parts of central Asia and the Atlantic and Indian oceans. The domain has a horizontal resolution of 0.1∘ latitude × 0.1∘ longitude in a rotated grid and 40 hybrid pressure–sigma model layers in the vertical. The model top was set to 5000 Pa. This domain configuration is used operationally to deliver daily forecasts at the World Meteorological Organization Barcelona Dust Regional Center (https://dust.aemet.es/, last access: 8 April 2022).
Table 2List of reanalysis variables. For each variable the following ensemble statistics are calculated and archived: ensemble mean, standard deviation, max and median. n/a – not applicable.
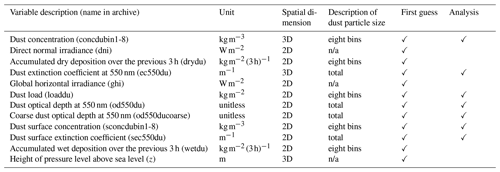
Table 3Averaged DOD of first guess (fg), analysis (an) and analysis increments (an-fg) for the full period (2007–2016), for different seasons (DJF, MAM, JJA, SON) and for individual years.
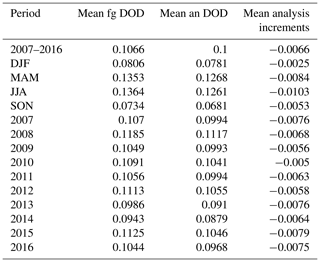
The model runs were conducted using a dynamics time step of 20 s. Lateral diffusion is called every time step; advection every 2 time steps; turbulence, surface layer, dust emission, sedimentation and dry deposition routines every 4 time steps; moist convection, microphysics and wet deposition every eight time steps; and short- and long-wave radiation routines every 180 time steps. The MONARCH ensemble of forward simulations was run daily at 00:00 UTC during 24 h, which was used as the first guess for the data assimilation. Simulation outputs are provided every 3 h (03:00, 06:00, 09:00, 12:00, 15:00, 18:00, 21:00 and 00:00 UTC), which is also the time resolution of the reanalysis product. Figure 3 shows the scheme of the 24 h assimilation window for the production of the reanalysis where each ensemble member forward simulation is initialized at 00:00 UTC using the dust analysis produced in the previous window.
Simulations were run without inflating the background or analysis covariance errors during the assimilation cycle. A quality control has been applied as in Di Tomaso et al. (2017) that rejects observations by a first-guess departure check (observations further than 1.4, in DODcoarse, from the first guess are rejected). This quality control is applied since the observations have not been corrected before assimilation for possible systematic biases. After the estimation of total dust coarse mixing ratio analysis, the analysis increments are partitioned among the dust coarse size bins according to their fractional contribution to the total coarse mixing ratio in the forward simulation step (i.e. before assimilation).
A spin-up period was necessary for the soil variables that need a longer period to adjust. We have run a 1-year spin-up with a two-member experiment, each of them initialized using either MERRA-2 or ERA-Interim meteorology with ERA5 soil information. Furthermore, a 2-month spin-up period was needed for the ensemble without data assimilation, to have a good representation of the ensemble spread everywhere in the atmospheric domain.
6.1 Ensemble calibration
MONARCH uses a globally uniform, empirically constrained tuning (or calibration) factor for the total emitted dust mass, referred to as dcal. This factor varies according to the specified configuration settings for the simulation. In particular, it depends on the emission scheme and the meteorological initial and boundary conditions used to initialize the simulation. We calibrated six free-running experiments, which cover all the different combinations between the emission scheme and meteorological conditions. The calibration factors were obtained by rescaling initial values for the calibration factors, namely dcal(m)old, by the ratio between the MODIS DB mean DODcoarse and the ensemble free-run mean DODcoarse calculated over the whole domain; i.e.
where m indicates an ensemble member. We have repeated the estimation twice where the second simulation re-run has used the calibration factors estimated from the first run. The final estimated calibration factors for each of six ensemble members are reported in Table S2.
The reanalysis data set consists of three-dimensional (3D) and two-dimensional (2D) variables (see Table 2). The 3D, or upper-air, variables include dust mass concentration [kg m−3] for each dust size bin, the dust extinction coefficient at 550 nm [m−1] integrated over all size bins and the height of the pressure level above sea level [m]. The 2D variables are either surface fields or total column fields. The 2D variables for each dust size bin include dry and wet accumulated dust deposition over the previous 3 h [] and instantaneous total column dust load [kg m−2], dust mass surface concentration [kg m−3], DOD [unitless] and DODcoarse [unitless] at 550 nm. The set of archived 2D variables is completed by the surface extinction coefficient at 550 nm [m−1], direct normal irradiance [W m−2] and global horizontal irradiance [W m−2]. These variables have been used to produce dust-relevant information for different sectors (Votsis et al., 2020, 2021) and related validation exercises (Mytilinaios et al., 2022a, b). For example, a dust-PM10 field has been derived from the 2D, bin-resolved dust mass surface concentration for air quality applications. This field will be used to evaluate the ability of the reanalysis to reproduce dust concentration values at the ground (Barnaba et al., 2022a). Over Europe, the latter will be extracted from measured PM10 values following a procedure similar to that described (Barnaba et al., 2022b). Furthermore, visibility data from 3D dust-extinction coefficient fields have been used for aviation applications (Basart et al., 2021), while soiling index based on wet and dry dust deposition has been used to develop products for solar energy production (Rautio et al., 2022).
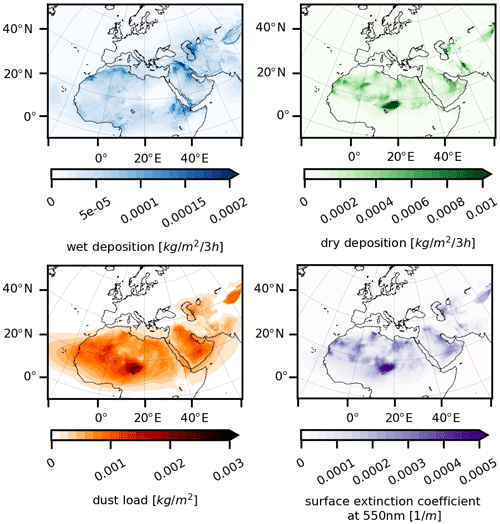
Figure 4Maps of mean 3-hourly first guess of wet and dry accumulated (over the previous 3 h) dust deposition [] and analysis of total column dust load [kg m−2] and of the dust surface extinction coefficient at 550 nm [m−1] calculated for the whole period (2007–2016). Model fields are the ensemble mean.
Both analysis and first-guess fields are available for the variables that are diagnosed from the state vector. As mentioned earlier, the first guesses are model forward simulations initialized with an analysis. When available, the analysis field is the recommended output for that variable. A set of ensemble statistics is calculated and archived for each output variable, namely the ensemble mean, standard deviation, maximum and median. The spread among the ensemble members, represented by the standard deviation with respect to the ensemble mean, can be interpreted as a measure for the uncertainty in the mean estimates. Figure 4 shows the ensemble mean over the whole reanalysis period for the analysis or first guess of some of the 2D variables. While model fields have been produced on 40 vertical levels, the data are stored on 15 standard pressure levels between 1000 and 100 hPa (i.e. 1000, 975, 900, 850, 750, 700, 600, 500, 400, 350, 300, 250, 175, 150, 100 hPa), which were defined taking into account regulatory standards in the aviation sector (in view of end-user products developed from the reanalysis in this sector; Votsis et al., 2020). In that way we reduced storage space while easing the use of the vertical information.
The reanalysis data set is structured into individual Network Common Data Form (NetCDF) files per variable and type of ensemble statistics. Further details on the file structure of the data set are reported in Sect. 9, while the naming convention for the data set files and folders is explained in Appendix A.
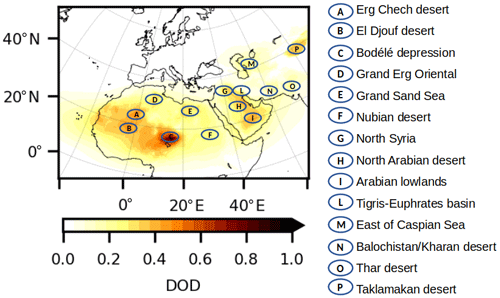
Figure 5A number of desert, arid and semi-arid regions of interest for the description of the dust reanalysis. The underlying dust field is the mean 3-hourly DOD analysis calculated for the whole reanalysis period (2007–2016).
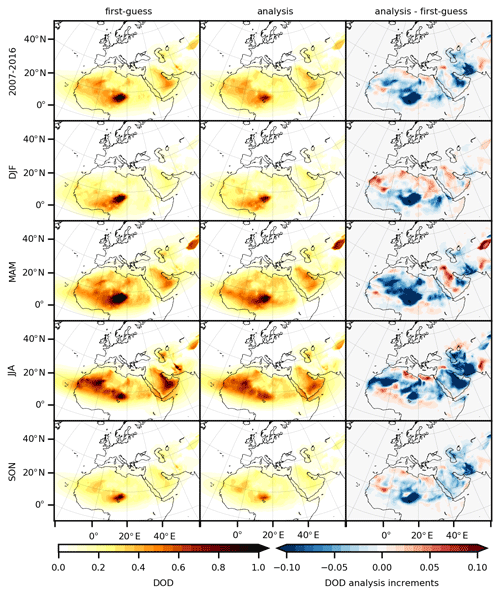
Figure 6Maps of mean 3-hourly DOD first guess (first column), analysis (second column) and analysis increments (third column) calculated for the whole period (2007–2016; top row) and for different seasons (DJF, MAM, JJA, SON; rows 2 to 5). Model fields are the ensemble mean.
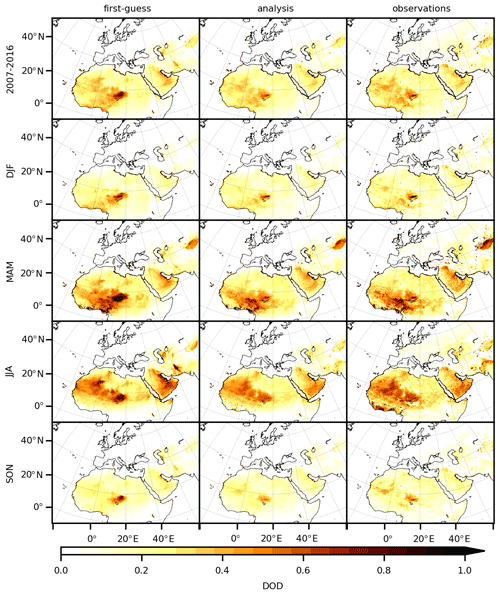
Figure 7Maps of mean 3-hourly DODcoarse first guess (first column), analysis (second column) and MODIS DB assimilated observations (third column) calculated for the whole period (2007–2016; top row) and for different seasons (DJF, MAM, JJA, SON; rows 2 to 5). Model fields are the ensemble mean and are collocated with the observations.
In this section we validate the reanalysis DOD or DODcoarse in terms of data assimilation inner diagnostics (analysis increments and statistics of departures from assimilated observations) and verify it against independent ground-based observations. We also discuss the DOD spatial and temporal patterns over the reanalysis domain and period. Figure 5 highlights the location of major dust source areas that will be used in the discussion. The verification of DOD and DODcoarse against long-term ground-based observations across the domain is a first step towards a more comprehensive evaluation of the reanalysis data set that is planned in follow-up papers (Barnaba et al., 2022a; Di Tomaso et al., 2022; Mytilinaios et al., 2022a, b), which include the comparison against independent sets of in situ, column-based and profile retrievals.
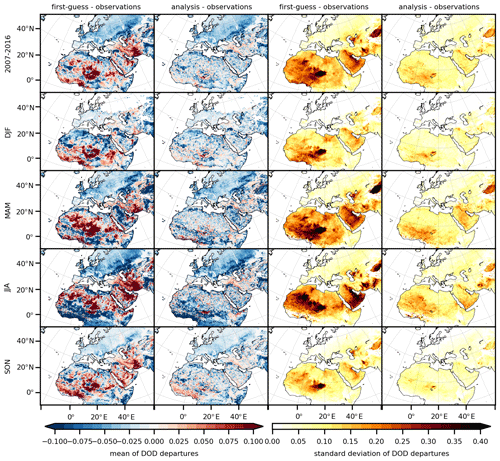
Figure 8Maps of mean 3-hourly DODcoarse for first-guess departures (first column), analysis departures (second column), standard deviation of first-guess departures (third column) and standard deviation of analysis departures (fourth column) calculated for the whole period (2007–2016; top row) and for different seasons (DJF, MAM, JJA, SON; rows 2 to 5). Model fields are the ensemble mean and are collocated with the observations.
8.1 DOD geographical distribution
Figure 6 shows the ensemble annual and seasonal mean DOD for the first guess (left column) and the analysis (central column) during the whole reanalysis period. In agreement with observations, the highest DOD values are placed over the major emission areas of the domain, in particular in the Bodélé Depression in Chad, the Erg Chech in Algeria, and the El Djouf between Mauritania and Mali, followed by the Arabian Desert; the Taklamakan Desert in northwest China; and the smaller areas of the Grand Erg Oriental in Algeria, the Grand Sand Sea between Libya and Egypt; and the Kharan Desert in southwestern Pakistan. Table 3 reports the averaged DOD of first guess, analysis and analysis minus first guess (analysis increments) when calculated for the whole domain for the full period, for different seasons (DJF, MAM, JJA, SON) and for individual years.
The decadal mean analysis DOD (top row of Fig. 6) is generally smaller than the first-guess DOD except in the Taklamakan and Thar deserts and in areas where the mean DOD is below 0.3. Therefore, on average, MONARCH emissions are likely too strong for the configurations used, although a potentially too weak deposition cannot be discarded. The latter is strongly dependent upon the emitted size distribution that evolves during transport.
Seasonal changes in the geographical distribution of the analysis mean DOD (rows 2 to 5 of Fig. 6) are consistent with well-known patterns (Prospero et al., 2002; Ginoux et al., 2012): (i) dust peaks everywhere during spring and summer, in particular, across the Taklamakan Desert during spring when more dust-generating cold fronts arrive in the area; (ii) dust from the south Sahara and Sahel is preferentially transported by northeasterly harmattan winds towards the Gulf of Guinea in winter and spring; (iii) the dust plume that originated in western Africa and is transported across the tropical North Atlantic is shifted towards northern latitudes in summer along with the Intertropical Convergence Zone (ITCZ; Moulin et al., 1997); (iv) dust is strongly mobilized on the Arabian Peninsula and in the Tigris–Euphrates Basin in summer by the north-northwesterly shamal winds; (v) the lowest overall DOD is simulated everywhere in autumn.
8.2 DOD analysis increments
Figure 6 also shows the difference between DOD analysis and first guess (namely analysis increments; right column) averaged over the full reanalysis period (top row). Non-zero systematic analysis increments are to be interpreted as systematic corrections to the model simulations and can serve as a proxy for model bias. By applying these corrections, the analysis improves the underlying model. The patterns of these systematic corrections vary with season and geographical location. While over the entire domain the mean analysis and first guess are comparable, the biggest systematic negative corrections (removing mass from the atmosphere) are linked to overestimation of sources' strengths in the Bodélé Depression in Chad; in the Saudi Arabia lowlands; and in the Balochistan region of southwestern Asia that extends over Iran, Afghanistan and Pakistan and contains, for example, the Kharan Desert. Negative mean increments are also present but to a lesser extent in other arid and semi-arid areas such as the Erg Chech in Algeria, the Great Sand Sea in Libya, the Nubian Desert in Sudan and eastwards of the Caspian Sea. Positive mean increments calculated for the whole reanalysis period are less widespread than the negative increments. The strongest values are over the Thar Desert, in the northern part of Syria, over a long stretch inland from the Mediterranean Sea in the north of Africa, and in the desert of El Djouf between Mauritania and Mali. All in all, as expected, the largest positive or negative analysis increments correspond to areas with more dust load, i.e. to source regions and their vicinity.
The patterns of the mean increments depend upon the season (see rows 2 to 5 of Fig. 6). These patterns are clearly linked to the seasonal changes in dust activities in the different regions, as mean increments are, in absolute value, higher in the presence of high mean DODs compared to low DOD values. The areas that show the strongest seasonality with respect to the analysis increments are the Bodélé Depression and the Arabian and Taklamakan deserts. The overestimation of the Bodélé source strength in the first guess is more pronounced in winter and spring. In spring the emissions from the Taklamakan Desert, Syria and the northern part of the Arabian Desert are clearly underestimated, while in summer strong negative increments are present all over the Arabian Desert. Wide areas in the Sahara are affected by negative increments in the spring and summer. The Balochistan region and the Thar Desert show negative and positive increments, respectively, throughout the year, but their magnitudes are greater in spring and summer.
The patterns of the increments are consistent among the different years (Figs. S2 and S3) and vary mostly in the amplitude of the mean corrections, although there are some exceptions. Positive increments over the Thar Desert, northern Syria and the north of the Arabian Desert mainly appear in the first part of the reanalysis, between 2007 and 2012, in contrast to the small positive or even negative increments in the case of the Arabian Desert in the subsequent years. Strong negative increments east of the Caspian Sea are applied mainly through 2007 to 2010. Those yearly differences suggest changes, for example in land use, that are not captured by the model. Negative corrections in the west of the Sahara are more widespread in 2007 and 2008 than in other years due to the higher mean DOD during those 2 years.
8.3 Statistics of departures from assimilated observations
We compare here the reanalysis DODcoarse with the assimilated observations. Figure 7 shows the DODcoarse for the observation-collocated ensemble mean first guess and analysis and for the assimilated observations averaged over the full reanalysis period (top row of Fig. 7) and over the DJF, MAM, JJA and SON seasons (from the second to the fifth row of Fig. 7). Table 4 reports the corresponding values averaged over the whole domain for the full period, for different seasons and for individual years. By visual inspection, the analysis is closer to the assimilated observations in all the time periods considered, which constitutes a good sanity check for the assimilation scheme. This is also confirmed when the averages are calculated for individual years of the reanalysis period (Figs. S4 and S5). The seasonality in the model simulations closely resembles that in the observations, with MAM and JJA being the most active dust seasons.
Table 4Averaged DODcoarse of observation-collocated first guess (fg), observation-collocated analysis (an) and assimilated MODIS DB retrievals for the full period (2007–2016), for different seasons (DJF, MAM, JJA, SON) and for individual years.
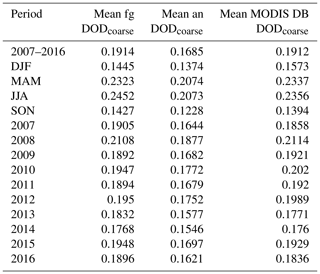
Table 5Statistics (mean and SD) of departures of DODcoarse first guess (fg) and analysis (an) from assimilated observations calculated for the full period (2007–2016), for different seasons (DJF, MAM, JJA, SON) and for individual years. The number of observation counts is also reported.
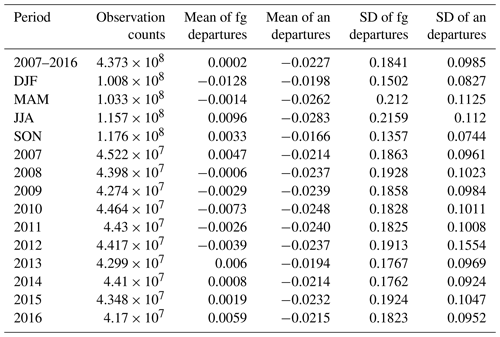
Figure 8 shows the mean (first and second column of Fig. 8) and standard deviation (third and fourth column of Fig. 8) of the first-guess and analysis DODcoarse departures (respectively) from assimilated observations averaged over the full reanalysis period (top row of Fig. 8) and over the different four seasons (from the second to the fifth row of Fig. 8). The corresponding values averaged over the whole domain are reported in Table 5, together with the number of observation counts and statistics calculated for individual years. The departure statistics, in particular the reduction in the standard deviation of the analysis departures compared to the first guess everywhere in the domain of interest, prove the consistency of our assimilation procedure. This is also the case on a seasonal and yearly basis (Figs. S6 and S7). With respect to the mean departures, the positive mean departures (model simulation minus observations) decrease considerably in the analysis compared to the first guess, while some of the negative mean departures remain unchanged in specific regions or seasons. The latter is the case for example in Europe and Russia, when considering the full reanalysis period or the different seasons. The aforementioned regions see on average much lower DODcoarse values than the rest of the domain and are analysed less efficiently. This is likely due to the ensemble not having a sufficient spread for low simulated concentrations. A similar issue was previously identified in other assimilation systems (see Benedetti et al., 2009, their Sect. 4) and attributed to the fact that aerosol mass is a positive definite variable, which intrinsically deviates from the assumed Gaussian conditions in the prior in the analysis step. Negative mean departures are present in the sub-Sahel region and in particular over a stretch along the Gulf of Guinea in the summer season, with respect to both the first guess and the analysis. This might be due to the contamination of aerosols other than dust in the observational data set, which might be of anthropogenic or natural origin, e.g. aerosol produced by biomass burning in central Africa advected northwards (Haslett et al., 2019). Due to the above regional or seasonal issues, the total bias (i.e. mean departure from the observations calculated over the entire domain) is higher in the analysis compared to the first guess.
8.4 Verification of DOD and DODcoarse against AERONET
We compare here the reanalysis DOD and DODcoarse with independent observations that have not been used in the assimilation process. We employed products from the Aerosol Robotic Network (AERONET) of ground-based Sun photometers (Holben et al., 1998; O'Neill et al., 2003; Giles et al., 2019).
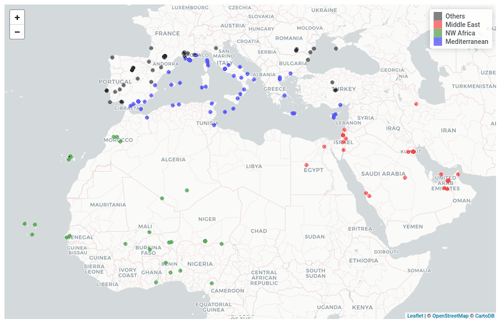
Figure 9Spatial distribution of the AERONET sites used in this study. The different colours indicate the sub-regions considered in the discussion of the results: northwestern Africa (green), the Middle East (red) and the Mediterranean (blue). When validating the NAMEE region, all the sites are considered, including the ones labelled “Others” (black). © OpenStreetMap contributors 2022. Distributed under the Open Data Commons Open Database License (ODbL) v1.0; © CARTO (https://carto.com/attribution/, last access: 8 June 2022).
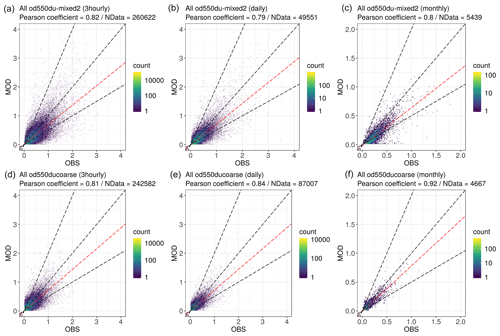
Figure 10Density scatter plots of the reanalysis (MOD) DOD and DODcoarse versus AERONET (OBS) dust-filtered AOD, DOD-mixed2 (a–c) and DODcoarse (d–f), during the whole reanalysis period (2007–2016). The results are calculated for the NAMEE domain and for different time basis: 3-hourly (a, d), daily (b, e) and monthly (c, f). The dust filters applied to the AERONET observations are described in Table 6. The bin size is 0.01.
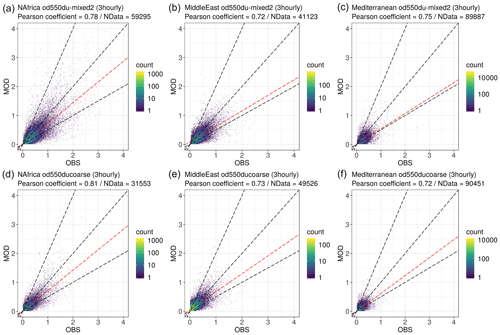
Figure 11Density scatter plots of the reanalysis (MOD) DOD and DODcoarse versus AERONET (OBS) dust-filtered AOD, DOD-mixed2 (a–c) and DODcoarse (d–f), during the whole reanalysis period (2007–2016) and on a 3-hourly basis. The results are calculated for three different sub-regions of the reanalysis domain: northwestern Africa (a, d), the Middle East (b, e) and the Mediterranean (c, f). The dust filters applied to the AERONET observations are described in Table 6. The bin size is 0.01.
Table 7Verification statistics (r, RMSE, MB and MFB) and number of samples (NDATA) for the reanalysis versus AERONET AODs for the entire period (2007–2016) and NAMEE region and for northwestern Africa, the Middle East and Mediterranean regions. AERONET version 3, cloud-screened, 3-hourly, dust-filtered AOD and AODcoarse comprise the reference. The definition of each of the DOD filters is in Table 6.
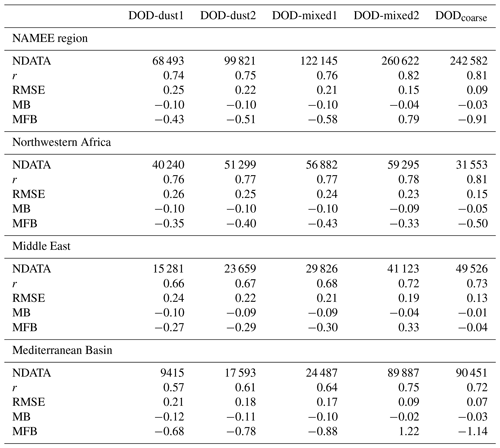
8.4.1 Verification methodology
We used AERONET version 3 quality-assured data. On the one hand, the modelled DODcoarse at 550 nm is compared with coarse-mode AOD retrievals at 500 nm from the spectral de-convolution algorithm (SDA; O'Neill et al., 2003). In general, AODcoarse is dominated by maritime/oceanic aerosols and desert dust. However, sea salt is usually associated with low AOD (< 0.03; Dubovik et al., 2002) and mainly affects coastal stations, and therefore inland high AODcoarse values can be assumed to be mineral dust. On the other hand, the modelled DOD at 550 nm is compared with dust-filtered AOD values from the direct-Sun algorithm (Giles et al., 2019). We used direct-Sun AOD retrievals between 440 and 870 nm to obtain the AOD at 550 nm using the Ångström law. Dust-dominated conditions are identified using a specific set of dust filters based on the AERONET Ångström exponent (AE). The AE is inversely related to the average size of the particles: the smaller the particles, the larger the AE. The AE ranges normally from 4, corresponding to pure molecular extinction, down to close to null values, corresponding to extinction dominated by coarse-mode aerosols (sea salt and mineral dust), producing a spectral neutral AOD (O'Neill et al., 2003). Values of AE > 1.2 typically indicate a significant presence of fine-mode particles (biomass burning or urban aerosols; Basart et al., 2009). Quantitative evaluations of the modelled DOD are conducted for dust-dominated conditions based on four different AE filters (Table 6) where AE ranges from typical desert dust source values (AE < 0.4) to values characteristic of dust long-range transport conditions (AE < 0.75). Additionally, for one of the filter methods (namely DOD-mixed2), DOD is assumed to be 0 when the AE is greater than 1.2. These dust filters roughly represent “pure” desert dust conditions (i.e. DOD-dust1, DOD-dust2 and DODcoarse) and long-range transport (i.e. mixed) dust conditions (i.e. DOD-mixed1 and DOD-mixed2).
We focus our verification on the NAMEE region. We used data from 140 AERONET stations (Fig. 9; see also Tables S3 to S8 for the list of AERONET sites used), which include all the available AERONET sites in the NAMEE domain providing observations during the period of 2007–2016, with the exception of those sites that are at high altitudes (i.e. altitudes greater than about 1850 m above sea level). Results are presented for different sub-regions, namely the Middle East, northwestern Africa and the Mediterranean, and for all available AERONET stations including those sites outside the three above-mentioned regions, and they are depicted in Fig. 9. Model values are ensemble mean analysis fields.
AERONET measurements are nominally taken at 15 min intervals. Here we average observations within ± 30 min of the 3-hourly model output times. These averaged observations are used to evaluate the model on a 3-hourly, daily and monthly basis. For the daily and monthly average evaluation, only coincident 3-hourly model output and observations are used. We use verification statistics such as the Pearson correlation coefficient (r), mean bias (MB), root mean square error (RMSE) and mean fractional bias (MFB) (see Appendix B) to measure the skill of the model when performing diagnostic analyses of DOD and DODcoarse where AERONET sites are located.
8.4.2 Comparison with 3-hourly, daily and monthly reference data
Overall, the dust reanalysis can reproduce the 3-hourly, daily and monthly observed variability with Pearson correlation coefficients ranging from 0.74 and 0.82, depending on the dust filter, for 3-hourly DOD to up to 0.92 for monthly DODcoarse. The reanalysis tends to underestimate the DOD and DODcoarse compared to AERONET observations (see Fig. 10). The model results are dominated by the results in northwestern Africa, and the largest relative underestimations are observed in the Mediterranean and the Middle East (Fig. 11), likely because of marine aerosols at these sites. Therefore some model underestimation is expected, in particular in proximity to coastal stations or when mixtures of aerosols are present (Basart et al., 2009).
Table 8Same as Table 7 but using daily reanalysis values and AERONET dust-filtered AOD and AODcoarse averaged on a daily basis as reference.
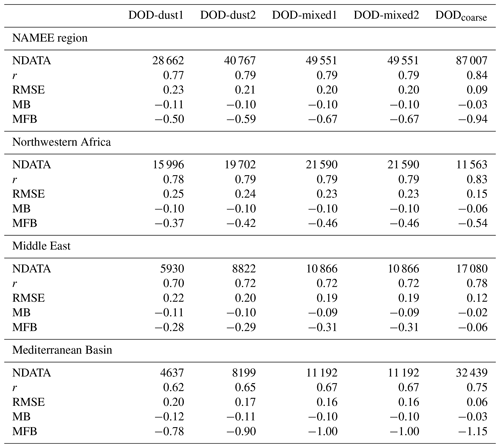
Table 9Same as Table 7 but using monthly reanalysis values and AERONET dust-filtered AOD and AODcoarse averaged on a monthly basis as reference.
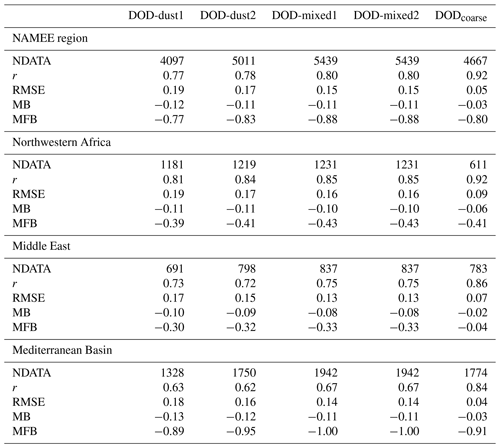
Tables 7 to 9 present the verification statistics on a 3-hourly, daily and monthly basis when calculated using the five dust-filtered reference data sets. The stricter the dust filter, the lower the correlation coefficient.
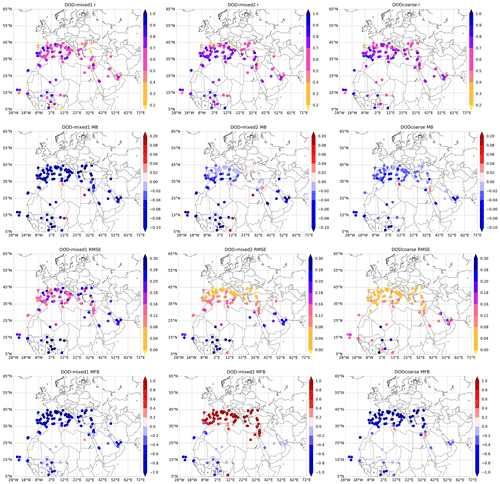
Figure 12Maps of verification statistics (r, MB, RMSE, MFB, from top to bottom) of the analysis DOD (first and second columns) and DODcoarse (third column) versus AERONET dust-filtered AOD (Table 6): DOD-mixed1 (left), DOD-mixed2 (middle) and DODcoarse (right) calculated for the whole period (2007–2016). The results are obtained using 3-hourly collocated reanalysis and observation values (see also Table 7).
The verification results calculated using the DOD-mixed2 dust filter are comparable to those obtained with the DODcoarse reference data set in terms of correlation (0.82 versus 0.81 for the entire region) and MB (−0.04 versus −0.03). When considering regional results, the use of the DOD-mixed2 dust filter shows a reduction in the MB together with an increase in MFB in the Mediterranean region (Fig. 12). This is directly related to the assumption DOD = 0 for AE > 1.2 (Table 6), which increases the number of collocations particularly in the Mediterranean, where the presence of dust is sporadic. This is confirmed by the comparison with the results obtained with the DOD-mixed1 filter where this condition is neglected (see Fig. 12). The RMSE obtained with DOD-mixed2 and DODcoarse reference data shows a clear north-to-south gradient that scales with dust concentrations with maximum values over sources (in northwestern Africa and the Middle East, RMSE > 0.12) and minimum values in the Mediterranean (RMSE < 0.12).
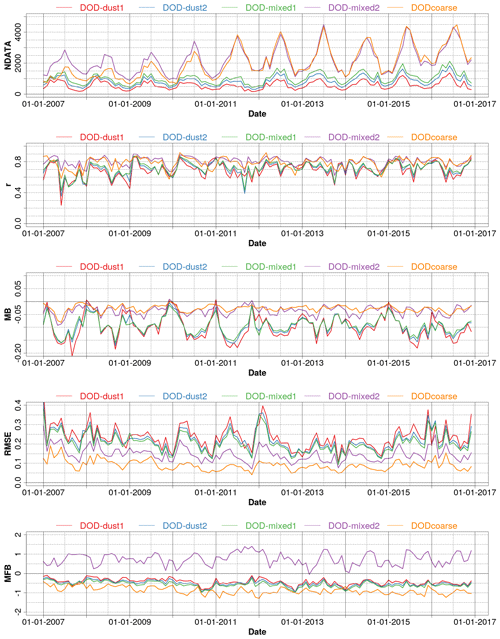
Figure 13Time series of monthly verification statistics (r, MB, RMSE, MFB) and number of samples (NDATA) for the reanalysis DOD and DODcoarse versus dust-filtered AERONET observations for the period 2007–2016 for the NAMEE domain. Different colours are associated with the results obtained with the different dust filters: DOD-dust1, DOD-dust2, DOD-mixed1, DOD-mixed2 and DODcoarse. The definition of each dust filter is reported in Table 6. The results are obtained using 3-hourly collocated model and observation values.
Monthly DOD and DODcoarse verification statistics are sensitive to the number of AERONET observations, as shown in Fig. 13. A clear seasonal trend is identified with lower performance in the cloudy winter season than in summer when clear skies are more frequent. Time series of the verification statistics for DODcoarse show a change after 2011, with reductions in MB and RMSE in comparison to previous years. Also the MFB is closer to the MFB from the different dust filters (see Fig. 13). This change is associated with a decrease in DODcoarse in the Mediterranean region (not shown here) that is captured by the reanalysis. Underestimations are observed in northwestern Africa and the Mediterranean regions when the DOD-mixed2 and DODcoarse data are used as reference. In summertime in northwestern Africa, we find the largest underestimations (monthly MB < −0.10 for DOD-mixed2 and DODcoarse). These underestimations are likely related to strong dust outbreaks associated with mesoscale convective systems (called haboobs) that the model is not able to capture. In the Middle East, the model shows a systematic underestimation when compared to DOD-mixed2 and DODcoarse reference data, although some overestimation in particular years (2011–2012) is observed. The observed DOD underestimations in comparison with AERONET in the Middle East can be partly attributed to a poor representation of small-scale emission processes such as the wind peak associated with the breakdown of the nocturnal low-level jet, the meteorological effects of orography, sea breezes and cold pools (Basart et al., 2016).
Overall, the comparison with AERONET observations shows a good performance of the reanalysis in reproducing the spatial and temporal distribution of mineral dust aerosols over the entire domain and for the 10-year period.
The reanalysis data set (Di Tomaso et al., 2021) is distributed via Thematic Real-time Environmental Distributed Data Services (THREDDS) at BSC and is made freely available at http://hdl.handle.net/21.12146/c6d4a608-5de3-47f6-a004-67cb1d498d98 (last access: 10 June 2022). The data set (78 TB in size) is structured into individual NetCDF files per geophysical variable and type of ensemble statistics (ensemble mean, standard deviation, maximum and median). Each individual file covers the time period of one assimilation window (24 h) and contains 8 time steps at a 3-hourly time frequency starting at 03:00 UTC. The files are organized into folders, where each folder contains the files relative to the whole reanalysis period (10 years) for a given variable and type of statistics.
A regional dust reanalysis has been produced using the MONARCH chemical weather prediction system and satellite retrievals of DODcoarse based on MODIS Aqua DB AOD at 550 nm. The reanalysis data set spans the period 2007–2016 at a horizontal resolution of 0.1∘ latitude × 0.1∘ longitude in a rotated grid and a temporal resolution of 3 h. The reanalysis covers a regional domain centred around Northern Africa, the Middle East and Europe (NAMEE region) that also includes parts of central Asia and the Atlantic and Indian oceans. This paper describes the modelling, observational and assimilation aspects related to the production of the reanalysis, whose unprecedentedly high resolution has required the use of advanced archiving and computing strategies, which are also described in the paper (see Appendix C). The assimilated observations have provided a total column optical constraint on the coarse fraction of dust particles over land, in cloud- and snow-free conditions, and in the daytime, with one satellite overpass per day. Analysis increments were estimated over the whole assimilation window through the 4D implementation of the assimilation algorithm and, to a certain extent (limited by the observation radius of influence), also over sea according to the model background spatial covariance. Re-partitions of analysis increments in the vertical dimension of the model control vector and across the individual model coarse size bins have relied on the model background.
The seasonal changes in the spatial distribution of the dust reanalysis are well characterized and follow well-known, region-dependent dust cycle features controlled by seasonal changes in meteorology (mainly surface winds but also precipitation) and in vegetation cover. The most prominent seasonal features that stand out in the reanalysis are the mobilization of dust during the so-called Asian dust events in the Taklamakan region in spring and by north-northwesterly shamal winds on the Arabian Peninsula and in the Tigris–Euphrates Basin during summer, the transport of south Saharan dust southwest towards the Gulf of Guinea by northeasterly harmattan trade winds during winter and spring, and the northward shift of the plume extending from western Africa over the tropical Atlantic during summer due to movements of the ITCZ.
Diagnostics based on departures of first guess and analysis from assimilated observations provided a sanity check for the quality of our assimilation procedure. As expected, the analysis is statistically closer to the assimilated observations than the first guess. The mean departures are larger in the analysis than in the first guess only in specific regions and seasons, which can be explained by the contamination of aerosols other than dust in the observational data set (for example, biomass burning aerosols produced by fires in central Africa that are advected further north during summer) or by the presence of fairly low DODcoarse values (mainly over Europe and western Russia) that are not analysed as efficiently by the assimilation scheme as the higher DODcoarse values. Mean analysis increments suggest seasonally dependent model biases that follow seasonal dust changes. By applying these corrections, the analysis improves the underlying model. Overall, the spatial distribution of the analysis increments over source regions, as well as in their proximity, highlights the pivotal role of the MODIS DB retrievals in providing an observational constraint over the most critical regions, confirming what previous studies have shown (Di Tomaso et al., 2017; Benedetti et al., 2019).
The reanalysis DOD and DODcoarse have been validated with highly accurate ground-truth measurements from AERONET on a 3-hourly, daily and monthly basis and with the application of specific dust filters to the reference products or the use of the coarse-mode AOD product. When the latter is used as reference, a Pearson correlation coefficient as high as 0.81 with a MB of −0.05 and a RMSE of 0.12 are estimated when considering the whole reanalysis period and 3-hourly AERONET retrievals. This confirms the good accuracy of the reanalysis data set and its suitability to be used in specific air quality/health and climate service applications. By extending the existing observation-based information intended for mineral dust monitoring, this reanalysis will allow a better quantification of dust impacts upon key sectors of society and the economy. This makes the data set a potentially useful tool in support of climate research and service, including the support to operational early warning systems and to the development of mitigation strategies.
This desert dust reanalysis data set is intended to be the first major endeavour towards the production of BSC aerosol reanalyses over regional or global domains. Extensions of the data set are planned for the near future. A series of companion papers will provide a more comprehensive evaluation of the reanalysis, an analysis of inter-annual variability and trends, and a description of the data set's application in dust-tailored services.
As described in Sect. 9, the reanalysis data set is structured into individual files per variable and type of ensemble statistics (e.g. an individual file contains the ensemble mean analysis of DOD at 550 nm). The filenames include the following terms separated by the underscore sign: the short name of the variable (as reported in Table 2); the initial date and time of the data included in the file; a suffix from among av, max, median and std indicating the ensemble mean, max, median and standard deviation, respectively, for that variable and optionally the label an for the variables for which an analysis field is produced. When the latter label is not present, the fields are model first guess. The filenames end with the extension suffix nc identifying NetCDF files. Each individual file contains eight time steps at a 3-hourly time frequency starting at 03:00 UTC. Therefore, for example, the filename for the ensemble mean analysis of DOD at 550 nm for a given date is od550du_YYYYMMDDHH_av_an.nc, where od550du is the variable short name and YYYYMMDDHH can take values from 2007010103 to 2016123103. The files are organized into folders containing the whole 10-year period for a given variable and type of statistics. Each folder is named with the variable short name followed by the hyphen sign and the suffix indicating the type of ensemble statistics and optionally by the label an preceded by the underscore sign for the variables for which there is an analysis field. Hence the folder containing the files of the example above is named od550du-av_an, while the corresponding ensemble mean first guess data are stored in the folder named od550du-av. To follow on with the same example, the ensemble mean analysis of DOD at 550 nm for 9 July 2012 can be found in the file path od550du-av_an/od550du_2012070903_av_an.nc.
The definitions of the verification metrics used in this study are reported in Table B1.
The reanalysis has been run on the BSC high-performance computing (HPC) infrastructure using the Autosubmit workflow manager (Manubens et al., 2016; Uruchi et al., 2021), a Python-based tool to create, manage and monitor experiments running on one or multiple remote computing clusters or HPC via the Secure Shell protocol. Scripts and templates to use Autosubmit were developed specifically for the reanalysis to be able to easily run and monitor long simulations by using the BSC HPC resources and store their results in the BSC archive. Autosubmit handles the job submission of the different workflow steps automatically, taking into account interruptions and failures. A functionality to wrap the 12 daily model simulations (each using 768 computing cores) and the data assimilation calculations (using 576 cores) was used to minimize the queuing times. This allows processing a number of days in a row and increasing the parallelism since a single job allocates the total sum of computing nodes required (i.e. 9792 cores were reserved by each wrapper). The number of computing cores for each job has been estimated to balance the execution time of a model simulation and of the assimilation for these jobs to share the computing resources. Additionally, the jobs of two post-processing steps were also wrapped together with the simulation and data assimilation jobs. The post-processing steps are needed to compress and reduce the original model and assimilation output and for the calculation of some basic ensemble statistics (to be provided to the users as main output of the reanalysis) before the final output is transferred to a long-term archive. The job wrapper has been designed with a crossing-date strategy to run two different starting dates within the same experiment. This is done so that the model ensemble simulations from the first starting date can be run in parallel with the assimilation job of the second starting date. This choice of design was made since model ensemble simulations and data assimilation calculations from the same date cannot be run simultaneously due to the obvious dependency of the data assimilation job on the model output. This novel workflow design was developed specifically for the production of the dust reanalysis and has proven successful for such highly computationally intensive calculations.
The supplement related to this article is available online at: https://doi.org/10.5194/essd-14-2785-2022-supplement.
CPGP, SB, EDT, OJ and JE designed the study and discussed the main results. FBa, EC, PF, LM, MM, AV, EW, MK and MG contributed to the discussions. EDT, JE, FM and NS developed and maintained the data assimilation code. PG prepared the assimilated observations. OJ led the MONARCH developments with contributions from CPGP, MG, MK, VO, JE and EDT. SB and CPGP carried out the independent validation, while EDT and JE performed the validation against assimilated observations. FM, MC, GMP, EDT and MO contributed to the development of the computing workflow. FM, AB, GMP and EDT ran the simulations. AB, SB, EDT, PAB and FBe performed the data set quality check and storage. EDT, CPGP and SB wrote the manuscript. All authors commented on the manuscript.
The contact author has declared that neither they nor their co-authors have any competing interests.
Publisher's note: Copernicus Publications remains neutral with regard to jurisdictional claims in published maps and institutional affiliations.
The BSC co-authors acknowledge PRACE (eDUST, eFRAGMENT1 and eFRAGMENT2) and RES (AECT-2019-3-0001, AECT-2020-1-0007, AECT-2020-3-0013) for awarding access to MareNostrum at the BSC and for providing technical support. The authors thank all the principal investigators and their staff for establishing and maintaining the NASA and PHOTONS AERONET sites and the MODIS mission scientists and associated NASA personnel for the production of the data used in this study. The authors also thank John P. Dunne, Julie Letertre-Danczak and the anonymous referee, who provided constructive comments that improved the manuscript.
This research has been supported by the DustClim project, which is part of ERA4CS, an ERA-NET programme co-funded by the European Union's Horizon 2020 research and innovation programme (grant no. 690462); the European Research Council (FRAGMENT (grant no. 773051)); grant no. RYC-2015-18690 funded by MCIN/AEI/10.13039/501100011033 and ESF Investing in your future; grant no. CGL2017-88911-R funded by MCIN/AEI/10.13039/501100011033 and ERDF A way of making Europe; the AXA Research Fund (AXA Chair on Sand and Dust Storms); the European Commission, Horizon 2020 Framework Programme (grant no. 792103 (SOLWARIS)); and ATMO-ACCESS (Access to Atmospheric Research Facilities) funded in the frame of the programme H2020-EU.1.4.1.2 (grant no. 101008004, 1 April 2021–31 March 2025). Jerónimo Escribano and Martina Klose have received funding from the European Union's Horizon 2020 research and innovation programme under the Marie Skłodowska-Curie grant agreements H2020-MSCA-COFUND-2016-754433 and H2020-MSCA-IF-2017-789630, respectively. Martina Klose received further support through the Helmholtz Association's Initiative and Networking Fund (grant no. VH-NG-1533). This work has been partially funded by the contribution agreement between AEMET and BSC to carry out development and improvement activities of the products and services supplied by the World Meteorological Organization (WMO) Barcelona Dust Regional Center (i.e. the WMO Sand and Dust Storm Warning Advisory and Assessment System (SDS-WAS) Regional Center for Northern Africa, the Middle East and Europe).
This paper was edited by Nellie Elguindi and reviewed by Julie Letertre-Danczak and one anonymous referee.
Anderson, T. L., Wu, Y., Chu, D. A., Schmid, B., Redemann, J., and Dubovik, O.: Testing the MODIS satellite retrieval of aerosol fine-mode fraction, J. Geophys. Res. Atmos., 110, D18204, https://doi.org/10.1029/2005JD005978, 2005. a
Ångström, A.: On the Atmospheric Transmission of Sun Radiation and on Dust in the Air, Geogr. Ann., 11, 156–166, https://doi.org/10.1080/20014422.1929.11880498, 1929. a
Badia, A., Jorba, O., Voulgarakis, A., Dabdub, D., Pérez García-Pando, C., Hilboll, A., Gonçalves, M., and Janjic, Z.: Description and evaluation of the Multiscale Online Nonhydrostatic AtmospheRe CHemistry model (NMMB-MONARCH) version 1.0: gas-phase chemistry at global scale, Geosci. Model Dev., 10, 609–638, https://doi.org/10.5194/gmd-10-609-2017, 2017. a
Barnaba, F. and Gobbi, G. P.: Aerosol seasonal variability over the Mediterranean region and relative impact of maritime, continental and Saharan dust particles over the basin from MODIS data in the year 2001, Atmos. Chem. Phys., 4, 2367–2391, https://doi.org/10.5194/acp-4-2367-2004, 2004. a
Barnaba, F., Bolignano, A., Di Liberto, L., Morelli, M., Lucarelli, F., Nava, S., Perrino, C., Canepari, S., Basart, S., Costabile, F., Dionisi, D., Ciampichetti, S., Sozzi, R., and Gobbi, G. P.: Desert dust contribution to PM10 loads in Italy: Methods and recommendations addressing the relevant European Commission Guidelines in support to the Air Quality Directive 2008/50, Atmos. Environ., 161, 288–305, https://doi.org/10.1016/j.atmosenv.2017.04.038, 2017. a
Barnaba F., Basart, S., Di Tomaso, E., Mytilinaios, M., Mona, L., Votsis, A., Werner, E., and Pérez García-Pando, C.: A pan European evaluation of the desert-dust impact on air quality employing model and observation-based approaches, in preparation, 2022a. a, b
Barnaba F., Alvan Romero, N., Bolignano, A., Basart, S., Renzi, M., and Stafoggia, M.: Multiannual assessment of the desert dust impact on air quality in Italy combining PM10 data with physics-based and geostatistical models, Environ. Int., 163, 107204, https://doi.org/10.1016/j.envint.2022.107204, 2022b. a
Basart, S., Pérez, C., Cuevas, E., Baldasano, J. M., and Gobbi, G. P.: Aerosol characterization in Northern Africa, Northeastern Atlantic, Mediterranean Basin and Middle East from direct-sun AERONET observations, Atmos. Chem. Phys., 9, 8265–8282, https://doi.org/10.5194/acp-9-8265-2009, 2009. a, b
Basart, S., Vendrell, L., and Baldasano, J. M.: High-resolution dust modelling over complex terrains in West Asia, Aeolian Res., 23, 37–50, https://doi.org/10.1016/j.aeolia.2016.09.005, 2016. a
Basart, S., Votsis, A., Rautio, T., Chouta, K., Barnaba, F., Di Tomaso, E., Mona, L., Mytilinaios, M., Formenti, P., Werner, E., and Pérez García-Pando, C.: Operating in risky sand and dust storm environments in Northern Africa, the Middle East and Europe: a portfolio of aviation climate services, EGU General Assembly 2021, online, 19–30 April 2021, EGU21-14490, https://doi.org/10.5194/egusphere-egu21-14490, 2021. a
Belly, P.-Y.: Sand movement by wind, Technical Memorandum No. 1, US Army Coastal Engineering Research Center, Washington, DC, USA, 1964. a
Benedetti, A., Morcrette, J.-J., Boucher, O., Dethof, A., Engelen, R. J., Fisher, M., Flentje, H., Huneeus, N., Jones, L., Kaiser, J. W., Kinne, S., Mangold, A., Razinger, M., Simmons, A. J., and Suttie, M.: Aerosol analysis and forecast in the European Centre for Medium-Range Weather Forecasts Integrated Forecast System: . Data assimilation, J. Geophys. Res., 114, D13205, https://doi.org/10.1029/2008JD011115, 2009. a
Benedetti, A., Baldasano, J. M., Basart, S., Benincasa, F., Boucher, O., Brooks, M. E., Chen, J.-P., Colarco, P. R., Gong, S., Huneeus, N., Jones, L., Lu, S., Menut, L., Morcrette, J.-J., Mulcahy, J., Nickovic, S., Pérez García-Pando, C., Reid, J. S., Sekiyama, T. T., Tanaka, T. Y., Terradellas, E., Westphal, D. L., Zhang, X.-Y, and Zhou C.-H.: Operational dust prediction, in: Mineral Dust: A Key Player in the Earth System, edited by: Knippertz, P. and Stuut, J.-B. W., Springer Netherlands, Dordrecht, https://doi.org/10.1007/978-94-017-8978-3_10, pp. 223–265, 2014. a
Benedetti, A., Reid, J. S., Knippertz, P., Marsham, J. H., Di Giuseppe, F., Rémy, S., Basart, S., Boucher, O., Brooks, I. M., Menut, L., Mona, L., Laj, P., Pappalardo, G., Wiedensohler, A., Baklanov, A., Brooks, M., Colarco, P. R., Cuevas, E., da Silva, A., Escribano, J., Flemming, J., Huneeus, N., Jorba, O., Kazadzis, S., Kinne, S., Popp, T., Quinn, P. K., Sekiyama, T. T., Tanaka, T., and Terradellas, E.: Status and future of numerical atmospheric aerosol prediction with a focus on data requirements, Atmos. Chem. Phys., 18, 10615–10643, https://doi.org/10.5194/acp-18-10615-2018, 2018. a
Benedetti, A., Di Giuseppe, F., Jones, L., Peuch, V.-H., Rémy, S., and Zhang, X.: The value of satellite observations in the analysis and short-range prediction of Asian dust, Atmos. Chem. Phys., 19, 987–998, https://doi.org/10.5194/acp-19-987-2019, 2019. a
Berrisford, P., Dee, D. P., Poli, P., Brugge, R., Fielding, M., Fuentes, M., Kållberg, P. W., Kobayashi, S., Uppala, S., and Simmons, A.: The ERA-Interim archive Version 2.0, ERA Report Series 1, ECMWF, Shinfield Park, Reading, https://www.ecmwf.int/node/8174 (last access: 8 April 2022), 2011. a
Betts, A. K.: A new convective adjustment scheme. Part 1: Observational and theoretical basis, Q. J. Roy. Meteor. Soc., 112, 677–691, https://doi.org/10.1002/qj.49711247307, 1986. a
Betts, A. K. and Miller, M. J.: A new convective adjustment scheme. Part 2: Single column tests using GATE wave, BOMEX, ATEX and arctic air-mass data sets, Q. J. Roy. Meteor. Soc., 112, 693–709, https://doi.org/10.1002/qj.49711247308, 1986. a
Boucher, O., Randall, D., Artaxo, P., Bretherton, C., Feingold, G., Forster, P., Kerminen, V.-M., Kondo, Y., Liao, H., Lohmann, U., Rasch, P., Satheesh, S. K., Sherwood, S., Stevens, B., and Zhang, X. Y.: Clouds and Aerosols, in: Climate Change 2013: The Physical Science Basis. Contribution of Working Group I to the Fifth Assessment Report of the Intergovernmental Panel on Climate Change, edited by: Stocker, T. F., Quin, D., Plattner, G.-K., Tignor, M., Allen, S. K., Boschung, J., Nauels, A., Xia, Y., Bex, V., and Midgley, P. M., Cambridge University Press, Cambridge, UK and New York, NY, USA, 2013–2019, 2019. a
Buchard, V., da Silva, A. M., Colarco, P. R., Darmenov, A., Randles, C. A., Govindaraju, R., Torres, O., Campbell, J., and Spurr, R.: Using the OMI aerosol index and absorption aerosol optical depth to evaluate the NASA MERRA Aerosol Reanalysis, Atmos. Chem. Phys., 15, 5743–5760, https://doi.org/10.5194/acp-15-5743-2015, 2015. a
Buchard V., Randles C. A., da Silva A . M., Darmenov A., Colarco P. R., Govindaraju R., Ferrare R., Hair J., Beyersdorf A. J., Ziemba L. D., and Yu H.: The MERRA-2 Aerosol Reanalysis, 1980 Onward. Part II: Evaluation and Case Studies, J Climate, 30, 6851–6872, https://doi.org/10.1175/jcli-d-16-0613.1, 2017. a
Claquin, T., Schulz, M., and Balkanski, Y. J.: Modeling the mineralogy of atmospheric dust sources, J. Geophys. Res., 104, 22243–22256, https://doi.org/10.1029/1999JD900416, 1999. a
Cuevas, E., Camino, C., Benedetti, A., Basart, S., Terradellas, E., Baldasano, J. M., Morcrette, J. J., Marticorena, B., Goloub, P., Mortier, A., Berjón, A., Hernández, Y., Gil-Ojeda, M., and Schulz, M.: The MACC-II 2007–2008 reanalysis: atmospheric dust evaluation and characterization over northern Africa and the Middle East, Atmos. Chem. Phys., 15, 3991–4024, https://doi.org/10.5194/acp-15-3991-2015, 2015. a
Cziczo, D. J., Froyd K. D., Hoose C., Jensen, E. J., Diao, M., Zondlo, M. A., Smith, J. B., Twohy, C. H., and Murphy D. M.: Clarifying the dominant sources and mechanisms of cirrus cloud formation, Science, 340, 1320–1324, https://doi.org/10.1126/science.1234145, 2013. a
D'Almeida, D. A.: On the variability of desert aerosol radiative characteristics, J. Geophys. Res., 92, https://doi.org/10.1029/JD092iD03p03017, 1987. a
Dee, D. P., Uppala, S. M., Simmons, A. J., Berrisford, P., Poli, P., Kobayashi, S., Andrae, U., Balmaseda, M. A., Balsamo, G., Bauer, P., Bechtold, P., Beljaars, A. C. M., van de Berg, L., Bidlot, J., Bormann, N., Delsol, C., Dragani, R., Fuentes, M., Geer, A. J., Haimberger, L., Healy, S. B., Hersbach, H., Hólm, E. V., Isaksen, L., Kållberg, P., Köhler, M., Matricardi, M., McNally, A. P., Monge-Sanz, B. M., Morcrette, J.-J., Park, B.-K., Peubey, C., de Rosnay, P., Tavolato, C., Thépaut, J.-N., and Vitart, F.: The ERA-Interim reanalysis: configuration and performance of the data assimilation system, Q. J. Roy. Meteor. Soc., 137, 553–597, https://doi.org/10.1002/qj.828, 2011. a, b
Denjean, C., Cassola, F., Mazzino, A., Triquet, S., Chevaillier, S., Grand, N., Bourrianne, T., Momboisse, G., Sellegri, K., Schwarzenbock, A., Freney, E., Mallet, M., and Formenti, P.: Size distribution and optical properties of mineral dust aerosols transported in the western Mediterranean, Atmos. Chem. Phys., 16, 1081–1104, https://doi.org/10.5194/acp-16-1081-2016, 2016. a
Di Biagio, C., Formenti, P., Balkanski, Y., Caponi, L., Cazaunau, M., Pangui, E., Journet, E., Nowak, S., Andreae, M. O., Kandler, K., Saeed, T., Piketh, S., Seibert, D., Williams, E., and Doussin, J.-F.: Complex refractive indices and single-scattering albedo of global dust aerosols in the shortwave spectrum and relationship to size and iron content, Atmos. Chem. Phys., 19, 15503–15531, https://doi.org/10.5194/acp-19-15503-2019, 2019. a
Di Tomaso, E., Schutgens, N. A. J., Jorba, O., and Pérez García-Pando, C.: Assimilation of MODIS Dark Target and Deep Blue observations in the dust aerosol component of NMMB-MONARCH version 1.0, Geosci. Model Dev., 10, 1107–1129, https://doi.org/10.5194/gmd-10-1107-2017, 2017. a, b, c, d, e
Di Tomaso, E., Escribano, J., Basart, S., Macchia, F., Benincasa, F., Bretonnière, P.-A., Buñuel, A., Castrillo, M., Gonçalves, M., Jorba, O., Klose, M., Montané Pinto, G., Olid, M., and Pérez García-Pando, C.: MONARCH high-resolution reanalysis data set of desert dust aerosol over Northern Africa, the Middle East and Europe, BSC, THREDDS, http://hdl.handle.net/21.12146/c6d4a608-5de3-47f6-a004-67cb1d498d98 (last access: 10 June 2022), 2021. a, b
Di Tomaso, E., Escribano, J., Basart, S., Ginoux, P., Macchia, F., Barnaba, F., Benincasa, F., Bretonnière, P.-A., Buñuel, A., Castrillo, M., Cuevas, E., Formenti, P., Gonçalves, M., Jorba, O., Klose, M., Mona, L., Montané Pinto, G., Mytilinaios, M., Obiso, V., Olid, M., Schutgens, N., Votsis, A., Werner, E., and Pérez García-Pando, C.: MONARCH regional reanalysis of desert dust aerosols: an initial assessment, in: Air pollution modeling and its application XXVIII, edited by: Mensink C. and Jorba O., Springer, in preparation, 2022. a
Dubovik, O., Holben, B., Eck, T. F., Smirnov, A., Kaufman, Y. J., King, M. D., Tanré, D., and Slutsker, I.: Variability of Absorption and Optical Properties of Key Aerosol Types Observed in Worldwide Locations, J. Atmos. Sci., 59, 590–608, https://doi.org/10.1175/1520-0469(2002)059<0590:VOAAOP>2.0.CO;2, 2002. a
Eck, T. F., Holben, B. N., Reid, J. S., Dubovik, O., Smirnov, A.,O'Neill, N. T., Slutsker, I., and Kinne, S.: Wavelength dependence of the optical depth of biomass burning, urban, and desert dust aerosols, J. Geophys. Res., 104, 31333–31349, https://doi.org/10.1029/1999JD900923, 1999. a
Ek, M. B., Mitchell, K. E., Lin, Y., Rogers, E., Grunmann, P., Koren, V., Gayno, G., and Tarpley, J. D.: Implementation of Noah land surface model advances in the National Centers for Environmental Prediction operational mesoscale Eta model, J. Geophys. Res.-Atmos., 108, 8851, https://doi.org/10.1029/2002JD003296, 2003. a
Escribano, J., Pérez García-Pando, C., Di Tomaso, E., Jorba, O., Klose, M., Macchia, F., and Montané, G.: Ensemble generation for the assimilation of dust aerosol observations, EGU General Assembly 2021, online, 19–30 April 2021, EGU21-10328, https://doi.org/10.5194/egusphere-egu21-10328, 2021. a
Escribano, J., Di Tomaso, E., Jorba, O., Klose, M., Gonçalves, M., Macchia, F., and Pérez García-Pando, C.: Emission and meteorological ensembles in the assimilation of dust optical depth, in preparation, 2022. a
Fecan, F., Marticorena, B., and Bergametti, G.: Parametrization of the increase of the aeolian erosion threshold wind friction velocity due to soil moisture for arid and semi-arid areas, Ann. Geophys., 17, 149–157, https://doi.org/10.1007/s00585-999-0149-7, 1999. a
Ferrier, B. S., Jin, Y., Lin, Y., Black, T., Rogers, E., and DiMego, G.: Implementation of a new grid-scale cloud and precipitation scheme in the NCEP Eta Model, in: Proc. 15th Conf. on Numerical Weather Prediction, 12–16 August 2002, San Antonio, TX, Amer. Meteor. Soc., pp. 280–283, 2002. a
Gelaro, R., McCarty, W., Suárez, M. J., Todling, R., Molod, A., Takacs, L., Randles, C., Darmenov, A., Bosilovich, M. G., Reichle, R., Wargan, K., Coy, L., Cullather, R., Draper, C., Akella, S., Buchard, V., Conaty, A., da Silva, A., Gu, W., Kim, G. K., Koster, R, Lucchesi, R., Merkova D., Nielsen, J. E., Partyka, G., Pawson, S., Putman, W., Rienecker, M., Schubert, S. D., Sienkiewicz, M., and Zhao, B.: The Modern-Era Retrospective Analysis for Research and Applications, Version 2 (MERRA-2), J. Climate, 30, 5419–5454. https://doi.org/10.1175/JCLI-D-16-0758.1, 2017. a, b, c
Giles, D. M., Sinyuk, A., Sorokin, M. G., Schafer, J. S., Smirnov, A., Slutsker, I., Eck, T. F., Holben, B. N., Lewis, J. R., Campbell, J. R., Welton, E. J., Korkin, S. V., and Lyapustin, A. I.: Advancements in the Aerosol Robotic Network (AERONET) Version 3 database – automated near-real-time quality control algorithm with improved cloud screening for Sun photometer aerosol optical depth (AOD) measurements, Atmos. Meas. Tech., 12, 169–209, https://doi.org/10.5194/amt-12-169-2019, 2019. a, b
Ginoux, P., Chin, M., Tegen, I., Prospero, J. M., Holben, B., Dubovik, O., and Lin, S.-J.: Sources and distributions of dust aerosols simulated with the GOCART model, J. Geophys. Res., 106, 20 255–20 273, https://doi.org/10.1029/2000JD000053, 2001. a, b, c, d, e
Ginoux, P., Garbuzov, D., and Hsu, N. C.: Identification of anthropogenic and natural dust sources using Moderate Resolution Imaging Spectroradiometer (MODIS) Deep Blue level 2 data, J. Geophys. Res., 115, D05204, https://doi.org/10.1029/2009JD012398, 2010. a, b, c
Ginoux, P., Prospero, J. M., Gill, T. E., Hsu, N. C., and Zhao, M.: Global-scale attribution of anthropogenic and natural dust sources and their emission rates based on Modis Deep Blue aerosol products, Rev. Geophys., 50, RG3005, https://doi.org/10.1029/2012rg000388, 2012. a, b, c, d, e
Gkikas, A., Basart, S., Korras-Carraca, M., Papadimas, C., Hatzianastassiou, N., Sayer, A., Hsu, C., and Baldasano, J. M.: Intercomparison of MODIS-Aqua C051 and C006 Level 3 Deep Blue AOD and Ångström exponent retrievals over the Sahara desert and the Arabian Peninsula during the period 2002–2014, Geophys. Res. Abstracts, 17, EGU2015-13537, 2015. a
Gkikas, A., Basart, S., Hatzianastassiou, N., Marinou, E., Amiridis, V., Kazadzis, S., Pey, J., Querol, X., Jorba, O., Gassó, S., and Baldasano, J. M.: Mediterranean intense desert dust outbreaks and their vertical structure based on remote sensing data, Atmos. Chem. Phys., 16, 8609–8642, https://doi.org/10.5194/acp-16-8609-2016, 2016.
Gliß, J., Mortier, A., Schulz, M., Andrews, E., Balkanski, Y., Bauer, S. E., Benedictow, A. M. K., Bian, H., Checa-Garcia, R., Chin, M., Ginoux, P., Griesfeller, J. J., Heckel, A., Kipling, Z., Kirkevåg, A., Kokkola, H., Laj, P., Le Sager, P., Lund, M. T., Lund Myhre, C., Matsui, H., Myhre, G., Neubauer, D., van Noije, T., North, P., Olivié, D. J. L., Rémy, S., Sogacheva, L., Takemura, T., Tsigaridis, K., and Tsyro, S. G.: AeroCom phase III multi-model evaluation of the aerosol life cycle and optical properties using ground- and space-based remote sensing as well as surface in situ observations, Atmos. Chem. Phys., 21, 87–128, https://doi.org/10.5194/acp-21-87-2021, 2021. a
Gonçalves, M., Obiso, V., Jorba, O., Formenti, P., Di Biagio, C., Miller, R., Schulz, M., and Pérez García-Pando, C.: Sensitivity of the fast climate response over North Africa to dust mineralogy, in preparation, 2022. a, b, c
Harris, E., Sinha, B., van Pinxteren, D., Tilgner, A., Wadinga Fomba, K., Schneider, J., Anja Roth, Gnauk, T., Fahlbusch, B., Mertes, S., Lee, T., Collett, J., Foley5, s., Borrmann, S., Hoppe, P., and Herrmann, H.: Enhanced Role of Transition Metal Ion Catalysis During In-Cloud Oxidation of SO2, Science, 340, 727–730, https://doi.org/10.1126/science.1230911, 2013. a
Haslett, S. L., Taylor, J. W., Evans, M., Morris, E., Vogel, B., Dajuma, A., Brito, J., Batenburg, A. M., Borrmann, S., Schneider, J., Schulz, C., Denjean, C., Bourrianne, T., Knippertz, P., Dupuy, R., Schwarzenböck, A., Sauer, D., Flamant, C., Dorsey, J., Crawford, I., and Coe, H.: Remote biomass burning dominates southern West African air pollution during the monsoon, Atmos. Chem. Phys., 19, 15217–15234, https://doi.org/10.5194/acp-19-15217-2019, 2019. a
Haustein, K., Pérez, C., Baldasano, J. M., Jorba, O., Basart, S., Miller, R. L., Janjic, Z., Black, T., Nickovic, S., Todd, M. C., Washington, R., Müller, D., Tesche, M., Weinzierl, B., Esselborn, M., and Schladitz, A.: Atmospheric dust modeling from meso to global scales with the online NMMB/BSC-Dust model – Part 2: Experimental campaigns in Northern Africa, Atmos. Chem. Phys., 12, 2933–2958, https://doi.org/10.5194/acp-12-2933-2012, 2012. a
Hersbach, H., Bell, B., Berrisford, P., Hirahara, S., Horányi, A., Muñoz-Sabater, J., Nicolas, J., Peubey, C., Radu, R., Schepers, D., Simmons, A., Soci, C., Abdalla, S., Abellan, X., Balsamo, G., Bechtold, P., Biavati, G., Bidlot, J., Bonavita, M., De Chiara, G., Dahlgren, P., Dee, D., Diamantakis, M., Dragani, R., Flemming, J., Forbes, R., Fuentes, M., Geer, A., Haimberger, L., Healy, S., Hogan, R. J., Hólm, E., Janisková, M., Keeley, S., Laloyaux, P., Lopez, P., Lupu, C., Radnoti, G., de Rosnay, P., Rozum, I., Vamborg, F., Villaume, S., and Thépaut, J.-N.: The ERA5 global reanalysis, Q. J. Roy. Meteor. Soc., 146, 1999–2049, https://doi.org/10.1002/qj.3803, 2020. a, b
Hess, M., Koepke, P., and Schult, I.: Optical properties of aerosols and clouds: the software package OPAC, B. Am. Meteorol. Soc., 79, 831–844, https://doi.org/10.1175/1520-0477(1998)079<0831:OPOAAC>2.0.CO;2, 1998. a, b
Holben, B. N., Eck, T. F., Slutsker, I., Tanré, D., Buis, J. P., Setzer, A., Vermote, E., Reagan, J. A., Kaufman, Y. J., Nakajima, T., Lavenu, F., Jankowiak, I., and Smirnov, A.: AERONET-A federated instrument network and data archive for aerosol characterization, Remote Sens. Environ., 66, 1–16, https://doi.org/10.1016/S0034-4257(98)00031-5, 1998. a
Hsu, N. C., Tsay, S.-C., King, M., and Herman, J. R.: Aerosol properties over bright-reflecting source regions, IEEE T. Geosci. Remote, 42, 557–569, https://doi.org/10.1109/TGRS.2004.824067, 2004. a
Hsu, N. C., Jeong, M.-J., Bettenhausen, C., Sayer, A., Hansell, R. A., Seftor, C. S., Huang, J., and Tsay, S.-C.: Enhanced deep blue aerosol retrieval algorithm: The second generation, J. Geophys. Res.-Atmos., 118, 9296–9315, https://doi.org/10.1002/jgrd.50712, 2013. a, b
Huneeus, N., Schulz, M., Balkanski, Y., Griesfeller, J., Prospero, J., Kinne, S., Bauer, S., Boucher, O., Chin, M., Dentener, F., Diehl, T., Easter, R., Fillmore, D., Ghan, S., Ginoux, P., Grini, A., Horowitz, L., Koch, D., Krol, M. C., Landing, W., Liu, X., Mahowald, N., Miller, R., Morcrette, J.-J., Myhre, G., Penner, J., Perlwitz, J., Stier, P., Takemura, T., and Zender, C. S.: Global dust model intercomparison in AeroCom phase I, Atmos. Chem. Phys., 11, 7781–7816, https://doi.org/10.5194/acp-11-7781-2011, 2011. a
Hunt, B. R., Kostelich, E. J., and Szunyogh, I.: Efficient data assimilation for spatiotemporal chaos: A local ensemble transform Kalman filter, Physica D, 230, 112–126, https://doi.org/10.1016/j.physd.2006.11.008, 2007. a, b, c, d
Iacono, M. J., Delamere, J. S., Mlawer, E. J., Shephard, M. W., Clough, S. A., and Collins, W. D.: Radiative forcing by long-lived greenhouse gases: Calculations with the AER radiative transfer models, J. Geophys. Res., 113, D13103, https://doi.org/10.1029/2008JD009944, 2008. a, b
Inness, A., Baier, F., Benedetti, A., Bouarar, I., Chabrillat, S., Clark, H., Clerbaux, C., Coheur, P., Engelen, R. J., Errera, Q., Flemming, J., George, M., Granier, C., Hadji-Lazaro, J., Huijnen, V., Hurtmans, D., Jones, L., Kaiser, J. W., Kapsomenakis, J., Lefever, K., Leitão, J., Razinger, M., Richter, A., Schultz, M. G., Simmons, A. J., Suttie, M., Stein, O., Thépaut, J.-N., Thouret, V., Vrekoussis, M., Zerefos, C., and the MACC team: The MACC reanalysis: an 8 yr data set of atmospheric composition, Atmos. Chem. Phys., 13, 4073–4109, https://doi.org/10.5194/acp-13-4073-2013, 2013. a
Inness, A., Ades, M., Agustí-Panareda, A., Barré, J., Benedictow, A., Blechschmidt, A.-M., Dominguez, J. J., Engelen, R., Eskes, H., Flemming, J., Huijnen, V., Jones, L., Kipling, Z., Massart, S., Parrington, M., Peuch, V.-H., Razinger, M., Remy, S., Schulz, M., and Suttie, M.: The CAMS reanalysis of atmospheric composition, Atmos. Chem. Phys., 19, 3515–3556, https://doi.org/10.5194/acp-19-3515-2019, 2019. a
Janjic, Z. I.: The step-mountain eta coordinate model: further developments of the convection, viscous sublayer, and turbulence closure schemes, Mon. Weather. Rev., 122, 927–945, https://doi.org/10.1175/1520-0493(1994)122<0927:TSMECM>2.0.CO;2, 1994. a, b
Janjic, Z. I.: The Mellor-Yamada level 2.5 scheme in the NCEP Eta Model, 11th Conference on Numerical Weather Prediction, 19–23 August 1996, Norfolk, USA, 333–334, 1996a. a
Janjic, Z. I.: The Surface Layer in the NCEP Eta Model, 11th Conference on Numerical Weather Prediction, 19–23 August 1996, Norfolk, USA, 354–355, 1996b. a
Janjic, Z. I.: Comments on “Development and evaluation of a convection scheme for use in climate models”, J. Atmos. Sci., 57, 3686, https://doi.org/10.1175/1520-0469(2000)057<3686:CODAEO>2.0.CO;2, 2000. a
Janjic, Z. I.: Nonsingular implementation of the Mellor-Yamada Level 2.5 scheme in the NCEP Meso model, Office Note, no. 437, National Centers for Environmental Prediction, https://www.emc.ncep.noaa.gov/officenotes/newernotes/on437.pdf (last access: 8 April 2022), 2001. a
Janjic, Z. I. and Gall, R.: Scientific documentation of the NCEP Nonhydrostatic Multiscale Model on the B grid (NMMB). Part 1 Dynamics, NCAR Technical Note NCAR/TN-489+STR, University Corporation for Atmospheric Research, https://doi.org/10.5065/D6WH2MZX, 2012. a, b
Janjic, Z. I., Gerrity Jr., J. P., and Nickovic, S.: An alternative approach to nonhydrostatic modeling, Mon. Weather Rev., 129, 1164–1178, https://doi.org/10.1175/1520-0493(2001)129<1164:AAATNM>2.0.CO;2, 2001. a
Jickels, T. D., An, Z. S., Andersen, K. K., Baker, A. R., Bergametti, G., Brooks, N., Cao, J. J., Boyd, P. W., Duce, R. A., Hunter, K. A., Kawahata, H., Kubilay, N., LaRoche, J., Liss, P. S., Mahowald, N., Prospero, J. M., Ridgwell, A. J., Tegen, I., and Torres, R.: Global Iron Connections Between Desert Dust, Ocean Biogeochemistry, and Climate, Science, 308, 67–71, https://doi.org/10.1126/science.1105959, 2005. a
Jorba, O. Dabdub, D., Blaszczak-Boxe, C., Pérez, C., Janjic, Z., Baldasano, J. M., Spada, M., Badia, A., and Gonçalves, M.: Potential significance of photoexcited NO2 on global air quality with the NMMB/BSC chemical transport model, J. Geophys. Res., 117, D13301, https://doi.org/10.1029/2012JD017730, 2012. a
Kanakidou, M., Myriokefalitakis, S., and Tsigaridis, K.: Aerosols in atmospheric chemistry and biogeochemical cycles of nutrients, Environ. Res. Lett., 13, 063004, https://doi.org/10.1088/1748-9326/aabcdb, 2018. a
Kaufman, Y. J., Koren, I., Remer, L. A., Tanre, D., Ginoux, P., and Fan, S.: Dust transport and deposition observed from the Terra-Moderate Resolution Imaging Spectroradiometer spacecraft over the Atlantic Ocean, J. Geophys. Res., 110, D0S12, https://doi.org/10.1029/2003JD004436, 2005. a
Kim, D., Chin, M., Yu, H., Eck, T. F., Sinyuk, A., Smirnov, A., and Holben, B. N.: Dust optical properties over North Africa and Arabian Peninsula derived from the AERONET dataset, Atmos. Chem. Phys., 11, 10733–10741, https://doi.org/10.5194/acp-11-10733-2011, 2011. a
Kiselev, A., Bachmann, F., Pedevilla, P., Cox, S. J., Michaelides, A., Gerthsen, D., and Leisner, T.: Active sites in heterogeneous ice nucleation – the example of K-rich feldspars, Science, 355, 367–371, https://doi.org/10.1126/science.aai8034, 2017. a
Klose, M., Jorba, O., Gonçalves Ageitos, M., Escribano, J., Dawson, M. L., Obiso, V., Di Tomaso, E., Basart, S., Montané Pinto, G., Macchia, F., Ginoux, P., Guerschman, J., Prigent, C., Huang, Y., Kok, J. F., Miller, R. L., and Pérez García-Pando, C.: Mineral dust cycle in the Multiscale Online Nonhydrostatic AtmospheRe CHemistry model (MONARCH) Version 2.0, Geosci. Model Dev., 14, 6403–6444, https://doi.org/10.5194/gmd-14-6403-2021, 2021. a, b, c, d, e, f, g
Knippertz, P. and Stuut, J.-B. W. (Eds.): Mineral Dust: A Key Player in the Earth System, Springer Science, Dordrecht, the Netherlands, 509 p., 2014. a
Kok, J. F.: A scaling theory for the size distribution of emitted dust aerosols suggests climate models underestimate the size of the global dust cycle, P. Natl. Acad. Sci. USA, 108, 1016–1021, https://doi.org/10.1073/pnas.1014798108, 2011. a, b, c, d
Kok, J. F., Mahowald, N. M., Fratini, G., Gillies, J. A., Ishizuka, M., Leys, J. F., Mikami, M., Park, M.-S., Park, S.-U., Van Pelt, R. S., and Zobeck, T. M.: An improved dust emission model – Part 1: Model description and comparison against measurements, Atmos. Chem. Phys., 14, 13023–13041, https://doi.org/10.5194/acp-14-13023-2014, 2014. a, b, c
Kok, J. F., Ridley, D. A., Zhou, Q., Miller, R. L., Zhao, C., Heald, C. L., Ward, D. S., Albani, S., and Haustein, K.: Smaller desert dust cooling effect estimated from analysis of dust size and abundance, Nat. Geosci., 10, 274–278, https://doi.org/10.1038/ngeo2912, 2017. a
Luo, T., Wang, Z., Zhang, D., Liu, X., Wang, Y., and Yuan, R.: Global dust distribution from improved thin dust layer detection using A-train satellite lidar observations, Geophys. Res. Lett., 42, 620–628, https://doi.org/10.1002/2014GL062111, 2015. a
Lynch, P., Reid, J. S., Westphal, D. L., Zhang, J., Hogan, T. F., Hyer, E. J., Curtis, C. A., Hegg, D. A., Shi, Y., Campbell, J. R., Rubin, J. I., Sessions, W. R., Turk, F. J., and Walker, A. L.: An 11-year global gridded aerosol optical thickness reanalysis (v1.0) for atmospheric and climate sciences, Geosci. Model Dev., 9, 1489–1522, https://doi.org/10.5194/gmd-9-1489-2016, 2016. a
Mahowald, N. M., Ballantine, J. A., Feddema, J., and Ramankutty, N.: Global trends in visibility: implications for dust sources, Atmos. Chem. Phys., 7, 3309–3339, https://doi.org/10.5194/acp-7-3309-2007, 2007. a
Mallone, S., Stafoggia, M., Faustini, A., Gobbi, G. P., Marconi, A., and Forastiere, F.: Saharan dust and associations between particulate matter and daily mortality in Rome, Italy, Environ. Health Persp., 119, 1409–1414, https://doi.org/10.1289/ehp.1003026, 2011. a
Manubens-Gil, D., Vegas-Regidor, J., Prodhomme, C., Mula-Valls, O., and Doblas-Reyes, F. J.: Seamless management of ensemble climate prediction experiments on HPC platforms, 2016 International Conference on High Performance Computing and Simulation (HPCS), Innsbruck, 18–22 July 2016, https://doi.org/10.1109/HPCSim.2016.7568429, 2016. a
Markel, V. A.: Introduction to the Maxwell Garnett approximation: tutorial, J. Opt. Soc. Am. A, 33, 1244–1256, https://doi.org/10.1364/JOSAA.33.001244, 2016. a
Marticorena, B. and Bergametti, G.: Modeling the atmospheric dust cycle: 1. design of a soil-derived dust emission scheme, J. Geophys. Res., 100, 16415–16430, https://doi.org/10.1029/95JD00690, 1995. a, b, c, d
Marticorena, B., Chatenet, B., Rajot, J. L., Traoré, S., Coulibaly, M., Diallo, A., Koné, I., Maman, A., NDiaye, T., and Zakou, A.: Temporal variability of mineral dust concentrations over West Africa: analyses of a pluriannual monitoring from the AMMA Sahelian Dust Transect, Atmos. Chem. Phys., 10, 8899–8915, https://doi.org/10.5194/acp-10-8899-2010, 2010. a
Miller, R. L., Knippertz, P., García-Pando, C. P., Perlwitz, J. P., and Tegen, I.: Impact of dust radiative forcing upon climate, in: Mineral Dust, edited by: Knippertz, P. and Stuut, J.-B. W., Springer, Netherlands, 327–357, 2014. a
Mishchenko, M. I., Travis, L. D., and Lacis, A. A.: Scattering, Absorption, and Emission of Light by Small Particles, Cambridge University Press, Cambridge, UKm 2002. a
Miyoshi T. and Yamane, S.: Local ensemble transform Kalman filtering with an AGCM at a T159/L48 resolution, Mon. Weather Rev., 135, 3841–3861, https://doi.org/10.1175/2007MWR1873.1, 2007. a
Mona, L., Liu, Z., Mü!ller, D., Omar, A., Papayannis, A., Pappalardo, G., Sugimoto, N., and Vaughan, M.: Lidar measurements for desert dust characterization: an overview, Adv. Meteorol., 2012, 356265, https://doi.org/10.1155/2012/356265, 2012. a
Morman, S. A. and Plumlee, G. S.: The role of airborne mineral dusts in human disease, Aeolian Res., 9, 203–212, https://doi.org/10.1016/j.aeolia.2012.12.001, 2013. a
Moulin, C., Lambert, C. E., Dulac, F., and Dayan, U.: Control of atmospheric export of dust from North Africa by the North Atlantic Oscillation, Nature, 387, 691–694, https://doi.org/10.1038/42679, 1997. a
Mytilinaios, M., Ciamprone, S., Trippetta, S., Basart, S., Di Tomaso, E., Jorba, O., Pérez García-Pando, C., Formenti, P., Cuesta, J., Gkikas, A., Kahn, R., and Mona, L.: Evaluation of the MONARCH reanalysis dust and coarse dust optical depth over Northern Africa, Middle East and Europe, using MODIS, MISR, IASI and AERONET observations, in preparation, 2022a. a, b
Mytilinaios, M., Ciamprone, S., Trippetta, S., Basart, S., Di Tomaso, E., Jorba, O., Pérez García-Pando, C., Proestakis E., Marinou E., Amiridis V., Cuesta, J., and Mona, L.: Evaluation of the MONARCH reanalysis dust extinction profiles over Northern Africa, Middle East and Europe, using CALIPSO, IASI and EARLINET observations, in preparation, 2022b. a, b
O'Neill, N. T., Eck, T. F., Smirnov, A., Holben, B. N., and Thulasiraman, S.: Spectral discrimination of coarse and fine model optical depth, J. Geophys. Res.-Atmos., 108, D17, https://doi.org/10.1029/2002JD002975, 2003. a, b, c
Pandolfi, M., Tobias, A., Alastuey, A., Sunyer, J., Schwartz, J., Lorente, J., Pey, J., and Querol, X.: Effect of atmospheric mixing layer depth variations on urban air quality and daily mortality during Saharan dust outbreaks, Sci. Total Environ., 1, 283–289, https://doi.org/10.1016/j.scitotenv.2014.07.004, 2014. a
Pérez, C., Nickovic, S., Pejanovic, G., Baldasano, J. M., and Özsoy, E.: Interactive dust-radiation modeling: a step to improve weather forecasts, J. Geophys. Res., 111, D16206, https://doi.org/10.1029/2005JD006717, 2006. a
Pérez, C., Haustein, K., Janjic, Z., Jorba, O., Huneeus, N., Baldasano, J. M., Black, T., Basart, S., Nickovic, S., Miller, R. L., Perlwitz, J. P., Schulz, M., and Thomson, M.: Atmospheric dust modeling from meso to global scales with the online NMMB/BSC-Dust model – Part 1: Model description, annual simulations and evaluation, Atmos. Chem. Phys., 11, 13001–13027, https://doi.org/10.5194/acp-11-13001-2011, 2011. a, b, c, d, e, f
Pérez García-Pando, C., Stanton, M. C., Diggle, P. J., Trzaska, S., Miller, R. L., Perlwitz, J. P., Baldasano, J. M., Cuevas, E., Ceccato, P., Yaka, P., and Thomson, M. C.: Soil Dust Aerosols and Wind as Predictors of Seasonal Meningitis Incidence in Niger, Environ. Health Persp., 112, 679–686, https://doi.org/10.1289/ehp.1306640, 2014. a
Pérez García-Pando, C., Miller, R. L., Perlwitz, J. P., Rodríguez, S., and Prospero, J. M.: Predicting the mineral composition of dust aerosols: Insights from elemental composition measured at the Izaña Observatory. Geophys. Res. Lett., 43, 10520–10529, https://doi.org/10.1002/2016GL069873, 2016. a
Pey, J., Querol, X., Alastuey, A., Forastiere, F., and Stafoggia, M.: African dust outbreaks over the Mediterranean Basin during 2001–2011: PM10 concentrations, phenomenology and trends, and its relation with synoptic and mesoscale meteorology, Atmos. Chem. Phys., 13, 1395–1410, https://doi.org/10.5194/acp-13-1395-2013, 2013. a, b
Perlwitz, J. P., Pérez García-Pando, C., and Miller, R. L.: Predicting the mineral composition of dust aerosols – Part 1: Representing key processes, Atmos. Chem. Phys., 15, 11593–11627, https://doi.org/10.5194/acp-15-11593-2015, 2015a. a
Perlwitz, J. P., Pérez García-Pando, C., and Miller, R. L.: Predicting the mineral composition of dust aerosols – Part 2: Model evaluation and identification of key processes with observations, Atmos. Chem. Phys., 15, 11629–11652, https://doi.org/10.5194/acp-15-11629-2015, 2015b. a
Pierre, C., Bergametti, G., Marticorena, B., AbdourhamaneTouré, A., Rajot, J.-L., and Kergoat, L.: Modeling wind erosion flux and its seasonality from a cultivated sahelian surface: A case study in Niger, Catena, 122, 61–71, https://doi.org/10.1016/j.catena.2014.06.006, 2014. a
Prigent, C., Jiménez, C., and Catherinot, J.: Comparison of satellite microwave backscattering (ASCAT) and visible/near-infrared reflectances (PARASOL) for the estimation of aeolian aerodynamic roughness length in arid and semi-arid regions, Atmos. Meas. Tech., 5, 2703–2712, https://doi.org/10.5194/amt-5-2703-2012, 2012. a
Prospero, J. M., Ginoux, P., Torres, O., Nicholson, S. E., and Gill, T. E.: Environmental characterization of global sources of atmospheric soil dust identified with the nimbus 7 total ozone mapping spectrometer (TOMS) absorbing aerosol product, Rev. Geophys., 40, 1002, https://doi.org/10.1029/2000RG000095, 2002. a
Pu, B. and Ginoux, P.: The impact of the Pacific Decadal Oscillation on springtime dust activity in Syria, Atmos. Chem. Phys., 16, 13431–13448, https://doi.org/10.5194/acp-16-13431-2016, 2016. a, b
Querol, X., Tobías, A., Pérez, N., Karanasiou, A., Amato, F., Stafoggia, M., Pérez García-Pando, C., Ginoux, P., Forastiere, F., Gumy, S., Mudu, P., and Alastuey, A.: Monitoring the impact of desert dust outbreaks for air quality for health studies, Environ. Int., 130, 104867, https://doi.org/10.1016/j.envint.2019.05.061, 2019. a
Randles C. A., da Silva A. M., Buchard V., Colarco P. R., Darmenov A., Govindaraju R., Smirnov A., Holben B., Ferrare R., Hair J., Shinozuka Y., and Flynn C. J.: The MERRA-2 Aerosol Reanalysis, 1980 – onward, Part I: System Description and Data Assimilation Evaluation, J Climate, 30, 6823–6850, https://doi.org/10.1175/JCLI-D-16-0609.1, 2017. a
Rautio, T., Votsis, A., Lindfors, A. V., Pérez García-Pando, C., Di Tomaso, E., Werner Hidalgo, E., Barnaba, F., Mona, L., Wang, Y., and Basart, S.: Impact assessment of sand and dust storms on solar energy production: a high-resolution climatological approach for Northern Africa, the Middle East and Europe, in preparation, 2022. a
Rizzolo, J. A., Barbosa, C. G. G., Borillo, G. C., Godoi, A. F. L., Souza, R. A. F., Andreoli, R. V., Manzi, A. O., Sá, M. O., Alves, E. G., Pöhlker, C., Angelis, I. H., Ditas, F., Saturno, J., Moran-Zuloaga, D., Rizzo, L. V., Rosário, N. E., Pauliquevis, T., Santos, R. M. N., Yamamoto, C. I., Andreae, M. O., Artaxo, P., Taylor, P. E., and Godoi, R. H. M.: Soluble iron nutrients in Saharan dust over the central Amazon rainforest, Atmos. Chem. Phys., 17, 2673–2687, https://doi.org/10.5194/acp-17-2673-2017, 2017. a
Rodríguez, S., Querol, X., Alastuey, A., Kallos, G., and Kakaliagou, O.: Saharan dust contributions to PM10 and TSP levels in Southern and Eastern Spain, Atmos. Environ., 35, 2433–2447, https://doi.org/10.1016/S1352-2310(00)00496-9, 2001. a
Rodríguez S., Alastuey, A., and Querol, X.: A review of methods for long term in-situ characterization of aerosol dust, Aeolian Res., 6, 55–74, https://doi.org/10.1016/j.aeolia.2012.07.004, 2012. a
Rubin, J. I., Reid, J. S., Hansen, J. A., Anderson, J. L., Collins, N., Hoar, T. J., Hogan, T., Lynch, P., McLay, J., Reynolds, C. A., Sessions, W. R., Westphal, D. L., and Zhang, J.: Development of the Ensemble Navy Aerosol Analysis Prediction System (ENAAPS) and its application of the Data Assimilation Research Testbed (DART) in support of aerosol forecasting, Atmos. Chem. Phys., 16, 3927–3951, https://doi.org/10.5194/acp-16-3927-2016, 2016. a
Sayer, A. M., Hsu, N. C., Bettenhausen, C., and Jeong, M.-J.: Validation and uncertainty estimates for MODIS Collection 6 “Deep Blue” aerosol data, J. Geophys. Res.-Atmos., 118, 7864–7873, https://doi.org/10.1002/jgrd.50600, 2013. a, b
Sayer, A. M., Munchak, L. A., Hsu, N. C., Levy, R. C., Bettenhausen, C., and Jeong, M.-J.: MODIS Collection 6 aerosol products: Comparison between Aqua's e-Deep Blue, Dark Target, and “merged” data sets, and usage recommendations, J. Geophys. Res.-Atmos., 119, 13, https://doi.org/10.1002/2014JD022453, 2014. a
Scanza, R. A., Mahowald, N., Ghan, S., Zender, C. S., Kok, J. F., Liu, X., Zhang, Y., and Albani, S.: Modeling dust as component minerals in the Community Atmosphere Model: development of framework and impact on radiative forcing, Atmos. Chem. Phys., 15, 537–561, https://doi.org/10.5194/acp-15-537-2015, 2015. a, b
Schroedter-Homscheidt, M., Oumbe, A., Benedetti, A., and Morcrette, J. J.: Aerosols for concentrating solar electricity production forecasts: requirement quantification and ECMWF/MACC aerosol forecast assessment, B. Am. Meteorol. Soc., 94, 903–914, https://doi.org/10.1175/BAMS-D-11-00259.1, 2013. a
Schutgens, N. A. J., Miyoshi, T., Takemura, T., and Nakajima, T.: Applying an ensemble Kalman filter to the assimilation of AERONET observations in a global aerosol transport model, Atmos. Chem. Phys., 10, 2561–2576, https://doi.org/10.5194/acp-10-2561-2010, 2010. a, b
Schutgens, N., Sayer, A. M., Heckel, A., Hsu, C., Jethva, H., de Leeuw, G., Leonard, P. J. T., Levy, R. C., Lipponen, A., Lyapustin, A., North, P., Popp, T., Poulsen, C., Sawyer, V., Sogacheva, L., Thomas, G., Torres, O., Wang, Y., Kinne, S., Schulz, M., and Stier, P.: An AeroCom–AeroSat study: intercomparison of satellite AOD datasets for aerosol model evaluation, Atmos. Chem. Phys., 20, 12431–12457, https://doi.org/10.5194/acp-20-12431-2020, 2020. a
Spada, M., Jorba, O., Pérez García-Pando, C., Janjic, Z., and Baldasano, J. M.: Modeling and evaluation of the global sea-salt aerosol distribution: sensitivity to size-resolved and sea-surface temperature dependent emission schemes, Atmos. Chem. Phys., 13, 11735–11755, https://doi.org/10.5194/acp-13-11735-2013, 2013. a
Stafoggia, M., Zauli-Sajani, S., Pey, J., Samoli, E., Alessandrini, E., Basagaña, X., Cernigliaro, A., Chiusolo, M., Demaria, M., Díaz, J., Faustini, A., Katsouyanni, K., Kelessis, A. G., Linares, C., Marchesi, S., Medina, S., Pandolfi, P., Pérez, N., Querol, X., Randi, G., Ranzi, A., Tobias, A., Forastiere, F., and the MED-PARTICLES Study Group: Desert Dust Outbreaks in Southern Europe: Contribution to Daily PM10 Concentrations and Short-Term Associations with Mortality and Hospital Admissions, Environ. Health Persp., 124, 413–419, https://doi.org/10.1289/ehp.1409164, 2016. a
Takemura, T., Nakajima, T., Dubovik, O., Holben, B., and Kinne, S.: Single-Scattering Albedo and Radiative Forcing of Various Aerosol Species with a Global Three-Dimensional Model, J. Climate, 15, 333–352, https://doi.org/10.1175/1520-0442(2002)015<0333:SSAARF>2.0.CO;2, 2002. a
Terradellas, E., Nickovic, S., and X. Zhang: Airborne dust: a hazard to human health, environment and society, WMO Bulletin, 64, 42–46, 2015. a
Textor, C., Schulz, M., Guibert, S., Kinne, S., Balkanski, Y., Bauer, S., Berntsen, T., Berglen, T., Boucher, O., Chin, M., Dentener, F., Diehl, T., Easter, R., Feichter, H., Fillmore, D., Ghan, S., Ginoux, P., Gong, S., Grini, A., Hendricks, J., Horowitz, L., Huang, P., Isaksen, I., Iversen, I., Kloster, S., Koch, D., Kirkevåg, A., Kristjansson, J. E., Krol, M., Lauer, A., Lamarque, J. F., Liu, X., Montanaro, V., Myhre, G., Penner, J., Pitari, G., Reddy, S., Seland, Ø., Stier, P., Takemura, T., and Tie, X.: Analysis and quantification of the diversities of aerosol life cycles within AeroCom, Atmos. Chem. Phys., 6, 1777–1813, https://doi.org/10.5194/acp-6-1777-2006, 2006. a
Tsikerdekis, A., Schutgens, N. A. J., and Hasekamp, O. P.: Assimilating aerosol optical properties related to size and absorption from POLDER/PARASOL with an ensemble data assimilation system, Atmos. Chem. Phys., 21, 2637–2674, https://doi.org/10.5194/acp-21-2637-2021, 2021. a, b
Uruchi, W., Castrillo, M., and Beltrán, D.: Autosubmit GUI: A Javascript-based graphical user interface to monitor experiments workflow execution, Journal of Open Source Software, 6, 3049, https://doi.org/10.21105/joss.03049, 2021. a
Votsis, A., Barnaba, F., Basart, S., Di Tomaso, E., Formenti, P., Lindfors, A., Mona, L., Rautio, T., Wang, Y., and Werner, E. H.: Operational risks of sand and dust storms in aviation and solar energy: the DustClim approach, FMI's Climate Bulletin: Research Letters 1/2020, https://doi.org/10.35614/ISSN-2341-6408-IK-2020-02-RL, 2020. a, b, c
Votsis, A., Basart, S., Barnaba, F., Di Tomaso, E., Lindfors, A., Mona, L., Mytilinaios, M., Formenti, P., Rautio, T., Wang, Y., Werner, E., and Pérez García-Pando, C.: Addressing the impacts of sand and dust storms in North Africa, the Middle East and Europe for air quality, aviation and solar energy: the DustClim approach to climate services, EGU General Assembly 2021, online, 19–30 April 2021, EGU21-12819, https://doi.org/10.5194/egusphere-egu21-12819, 2021. a
Yu, H., Chin, M., Yuan, T., Bian, H., Remer, L. A., Prospero, J. M., Omar, A., Winker, D., Yang, Y., Zhang, Y., Zhang, Z., and Zhao, C.: The fertilizing role of African dust in the Amazon rainforest: A first multiyear assessment based on data from Cloud-Aerosol Lidar and Infrared Path finder Satellite Observations, Geophys. Res. Lett., 42, 1984–1991, https://doi.org/10.1002/2015GL063040, 2015. a
Yumimoto, K. and Takemura, T.: Direct radiative effect of aerosols estimated using ensemble-based data assimilation in a global aerosol climate model, Geophys. Res. Lett., 28, L21802, https://doi.org/10.1029/2011GL049258, 2011.
Yumimoto, K., Tanaka, T. Y., Oshima, N., and Maki, T.: JRAero: the Japanese Reanalysis for Aerosol v1.0, Geosci. Model Dev., 10, 3225–3253, https://doi.org/10.5194/gmd-10-3225-2017, 2017. a
Zender, C. S., Bian, H., and Newman, D., Mineral Dust Entrainment and Deposition (DEAD) model: Description and 1990s dust climatology, J. Geophys. Res., 108, D14, https://doi.org/10.1029/2002JD002775, 2003. a
- Abstract
- Introduction
- MONARCH modelling system
- Generation of ensemble perturbations
- Assimilated observations
- Data assimilation algorithm
- Domain, resolution and other simulation settings
- Reanalysis product description
- DOD evaluation
- Data availability
- Conclusions and further perspectives
- Appendix A: Folder and file naming convention of the reanalysis data set
- Appendix B: Verification metrics
- Appendix C: Simulation workflow
- Author contributions
- Competing interests
- Disclaimer
- Acknowledgements
- Financial support
- Review statement
- References
- Supplement
- Abstract
- Introduction
- MONARCH modelling system
- Generation of ensemble perturbations
- Assimilated observations
- Data assimilation algorithm
- Domain, resolution and other simulation settings
- Reanalysis product description
- DOD evaluation
- Data availability
- Conclusions and further perspectives
- Appendix A: Folder and file naming convention of the reanalysis data set
- Appendix B: Verification metrics
- Appendix C: Simulation workflow
- Author contributions
- Competing interests
- Disclaimer
- Acknowledgements
- Financial support
- Review statement
- References
- Supplement