the Creative Commons Attribution 4.0 License.
the Creative Commons Attribution 4.0 License.
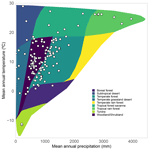
Global transpiration data from sap flow measurements: the SAPFLUXNET database
Víctor Granda
Víctor Flo
Mark A. Adams
Balázs Adorján
David Aguadé
Marcos P. M. Aidar
Scott Allen
M. Susana Alvarado-Barrientos
Kristina J. Anderson-Teixeira
Luiza Maria Aparecido
M. Altaf Arain
Ismael Aranda
Heidi Asbjornsen
Robert Baxter
Eric Beamesderfer
Z. Carter Berry
Daniel Berveiller
Bethany Blakely
Johnny Boggs
Gil Bohrer
Paul V. Bolstad
Damien Bonal
Rosvel Bracho
Patricia Brito
Jason Brodeur
Fernando Casanoves
Jérôme Chave
Cesar Cisneros
Kenneth Clark
Edoardo Cremonese
Hongzhong Dang
Jorge S. David
Teresa S. David
Nicolas Delpierre
Ankur R. Desai
Frederic C. Do
Michal Dohnal
Jean-Christophe Domec
Sebinasi Dzikiti
Colin Edgar
Rebekka Eichstaedt
Tarek S. El-Madany
Jan Elbers
Cleiton B. Eller
Eugénie S. Euskirchen
Brent Ewers
Patrick Fonti
Alicia Forner
David I. Forrester
Helber C. Freitas
Marta Galvagno
Omar Garcia-Tejera
Chandra Prasad Ghimire
Teresa E. Gimeno
John Grace
André Granier
Anne Griebel
Yan Guangyu
Mark B. Gush
Paul J. Hanson
Niles J. Hasselquist
Ingo Heinrich
Virginia Hernandez-Santana
Valentine Herrmann
Teemu Hölttä
Friso Holwerda
James Irvine
Supat Isarangkool Na Ayutthaya
Paul G. Jarvis
Hubert Jochheim
Carlos A. Joly
Julia Kaplick
Hyun Seok Kim
Leif Klemedtsson
Heather Kropp
Fredrik Lagergren
Patrick Lane
Petra Lang
Andrei Lapenas
Víctor Lechuga
Minsu Lee
Christoph Leuschner
Jean-Marc Limousin
Juan Carlos Linares
Maj-Lena Linderson
Anders Lindroth
Pilar Llorens
Álvaro López-Bernal
Michael M. Loranty
Dietmar Lüttschwager
Cate Macinnis-Ng
Isabelle Maréchaux
Timothy A. Martin
Ashley Matheny
Nate McDowell
Sean McMahon
Patrick Meir
Ilona Mészáros
Mirco Migliavacca
Patrick Mitchell
Meelis Mölder
Leonardo Montagnani
Georgianne W. Moore
Ryogo Nakada
Furong Niu
Rachael H. Nolan
Richard Norby
Kimberly Novick
Walter Oberhuber
Nikolaus Obojes
A. Christopher Oishi
Rafael S. Oliveira
Jean-Marc Ourcival
Teemu Paljakka
Oscar Perez-Priego
Pablo L. Peri
Richard L. Peters
Sebastian Pfautsch
William T. Pockman
Yakir Preisler
Katherine Rascher
George Robinson
Humberto Rocha
Alain Rocheteau
Alexander Röll
Bruno H. P. Rosado
Lucy Rowland
Alexey V. Rubtsov
Santiago Sabaté
Yann Salmon
Roberto L. Salomón
Elisenda Sánchez-Costa
Karina V. R. Schäfer
Bernhard Schuldt
Alexandr Shashkin
Clément Stahl
Marko Stojanović
Juan Carlos Suárez
Ge Sun
Justyna Szatniewska
Fyodor Tatarinov
Miroslav Tesař
Frank M. Thomas
Pantana Tor-ngern
Josef Urban
Fernando Valladares
Christiaan van der Tol
Ilja van Meerveld
Andrej Varlagin
Holm Voigt
Jeffrey Warren
Christiane Werner
Willy Werner
Gerhard Wieser
Lisa Wingate
Stan Wullschleger
Roman Zweifel
Kathy Steppe
Maurizio Mencuccini
Jordi Martínez-Vilalta
Plant transpiration links physiological responses of vegetation to water supply and demand with hydrological, energy, and carbon budgets at the land–atmosphere interface. However, despite being the main land evaporative flux at the global scale, transpiration and its response to environmental drivers are currently not well constrained by observations. Here we introduce the first global compilation of whole-plant transpiration data from sap flow measurements (SAPFLUXNET, https://sapfluxnet.creaf.cat/, last access: 8 June 2021). We harmonized and quality-controlled individual datasets supplied by contributors worldwide in a semi-automatic data workflow implemented in the R programming language. Datasets include sub-daily time series of sap flow and hydrometeorological drivers for one or more growing seasons, as well as metadata on the stand characteristics, plant attributes, and technical details of the measurements. SAPFLUXNET contains 202 globally distributed datasets with sap flow time series for 2714 plants, mostly trees, of 174 species. SAPFLUXNET has a broad bioclimatic coverage, with woodland/shrubland and temperate forest biomes especially well represented (80 % of the datasets). The measurements cover a wide variety of stand structural characteristics and plant sizes. The datasets encompass the period between 1995 and 2018, with 50 % of the datasets being at least 3 years long. Accompanying radiation and vapour pressure deficit data are available for most of the datasets, while on-site soil water content is available for 56 % of the datasets. Many datasets contain data for species that make up 90 % or more of the total stand basal area, allowing the estimation of stand transpiration in diverse ecological settings. SAPFLUXNET adds to existing plant trait datasets, ecosystem flux networks, and remote sensing products to help increase our understanding of plant water use, plant responses to drought, and ecohydrological processes. SAPFLUXNET version 0.1.5 is freely available from the Zenodo repository (https://doi.org/10.5281/zenodo.3971689; Poyatos et al., 2020a). The “sapfluxnetr” R package – designed to access, visualize, and process SAPFLUXNET data – is available from CRAN.
- Article
(10145 KB) - Full-text XML
-
Supplement
(1680 KB) - BibTeX
- EndNote
Terrestrial vegetation transpires ca. 45 000 km3 of water per year (Schlesinger and Jasechko, 2014; Wang-Erlandsson et al., 2014; Wei et al., 2017), a flux that represents 40 % of global land precipitation and 70 % of total land evapotranspiration (Oki and Kanae, 2006), and is comparable in magnitude to global annual river discharge (Rodell et al., 2015). For most terrestrial plants, transpiration is an inevitable water loss to the atmosphere because they need to open stomata to allow CO2 diffusion into the leaves for photosynthesis. Latent heat from transpiration represents 30 %–40 % of surface net radiation globally (Schlesinger and Jasechko, 2014; Wild et al., 2015). Transpiration is therefore a key process coupling land–atmosphere exchange of water, carbon, and energy, determining several vegetation–atmosphere feedbacks, such as land evaporative cooling or moisture recycling. Regulation of transpiration in response to fluctuating water availability and/or evaporative demand is a key component of plant functioning and one of the main determinants of a plant's response to drought (Martin-StPaul et al., 2017; Whitehead, 1998). Despite its relevance for earth functioning, transpiration and its spatiotemporal dynamics are poorly constrained by available observations (Schlesinger and Jasechko, 2014) and not well represented in models (Fatichi et al., 2016; Mencuccini et al., 2019). An improved understanding of transpiration and its regulation along environmental gradients and across species is thus needed to predict future trajectories of land evaporative fluxes and vegetation functioning under increased drought conditions driven by global change.
Conceptually, transpiration can be quantified at different organizational scales: leaves, branches, and whole plants; ecosystems; and watersheds. In practice, transpiration is relatively easy to isolate from the bulk evaporative flux, evapotranspiration, when measuring in a dry canopy, at the leaf or the plant level. However, in terrestrial ecosystems, evapotranspiration includes evaporation from the soil and from water-covered surfaces, including plants. Transpiration measurements on individual leaves or branches with gas exchange systems are difficult to upscale to the plant level (Jarvis, 1995). Likewise, transpiration measurements using whole-plant chambers (e.g. Pérez-Priego et al., 2010) or gravimetric methods (e.g. weighing lysimeters) in the field are still challenging. At the ecosystem scale and beyond, evapotranspiration is generally determined using micrometeorological methods, catchment water budgets, or remote sensing approaches (Shuttleworth, 2007; Wang and Dickinson, 2012). In some cases, isotopic methods and different algorithms applied to measured ecosystem fluxes can provide an estimation of transpiration at the ecosystem scale (Kool et al., 2014; Stoy et al., 2019).
Transpiration drives water transport from roots to leaves in the form of sap flow through the plant's xylem pathway (Tyree and Zimmermann, 2002), and this sap flow affects heat transport in the xylem. Taking advantage of this, thermometric sap flow methods were first developed in the 1930s (Huber, 1932) and further refined over the following decades (Čermák et al., 1973; Marshall, 1958) to provide operational measurements of plant water use. These methods have become widely used in plant ecophysiology, agronomy, and hydrology (Poyatos et al., 2016), especially after the development of simple, easily replicable methods (e.g. Granier, 1985, 1987). Whole-plant measurements of water use obtained with thermometric sap flow methods provide estimates of water flow through plants from sub-daily to interannual timescales and have been mostly applied in woody plants, although several studies have measured sap flow in herbaceous species (Baker and Van Bavel, 1987; Skelton et al., 2013) and non-woody stems (e.g. Lu et al., 2002). Xylem sap flow can be upscaled to the whole plant, obtaining a near-continuous quantification of plant water use, keeping in mind that stem sap flow typically lags behind canopy transpiration (Schulze et al., 1985). Multiple sap flow sensors can be deployed, in almost any terrestrial ecosystem, to determine the magnitude and temporal dynamics of transpiration across species, environmental conditions, or experimental treatments. All sap flow methods are subject to methodological and scaling issues, which may affect the quantification of absolute water use in some circumstances (Čermák et al., 2004; Köstner et al., 1998; Smith and Allen, 1996; Vandegehuchte and Steppe, 2013). Nevertheless, all methods are suitable for the assessment of the temporal dynamics of transpiration and of its responses to environmental changes or to experimental treatments (Flo et al., 2019).
The generalized application of sap flow methods in ecological and hydrological research in the last 30 years has thus generated a large volume of data, with an enormous potential to advance our understanding of the spatiotemporal patterns and the ecological drivers of plant transpiration and its regulation (Poyatos et al., 2016). However, these data need to be compiled and harmonized to enable global syntheses and comparative studies across species and regions. Across-species data syntheses using sap flow data have mostly focused on maximum values extracted from publications (Kallarackal et al., 2013; Manzoni et al., 2013; Wullschleger et al., 1998). Multi-site syntheses have focused on the environmental sensitivity of sap flow, using site means of plant-level sap flow or sap-flow-derived stand transpiration (Poyatos et al., 2007; Tor-ngern et al., 2017). Because data sharing is only incipient in plant ecophysiology, sap flow datasets have not been traditionally available in open data repositories. Open data practices are now being implemented in databases, which fosters collaboration across monitoring networks in research areas relevant to plant functional ecology (Falster et al., 2015; Gallagher et al., 2020; Kattge et al., 2020) and ecosystem ecology (Bond-Lamberty and Thomson, 2010). The success of the data sharing and data re-use policies within the FLUXNET global network of ecosystem-level fluxes has shown how these practices can contribute to scientific progress (Bond-Lamberty, 2018).
Here we introduce SAPFLUXNET, the first global database of sap flow measurements built from individual community-contributed datasets. We implemented this compilation in a data structure designed to accommodate time series of sap flow and the main hydrometeorological drivers of transpiration, together with metadata documenting different aspects of each dataset. We harmonized all datasets and performed basic semi-automated quality assurance and quality control (QC) procedures. We also created a software package that provides access to the database, allows easy visualization of the datasets, and performs basic temporal aggregations. We present the ecological and geographic coverage of SAPFLUXNET version 0.1.5 (Poyatos et al., 2020a), followed by a discussion of potential applications of the database, its limitations, and a perspective of future developments.
2.1 An overview of sap flow measurements
The main characteristics of sap flow methods have been reviewed elsewhere (Čermák et al., 2004; Smith and Allen, 1996; Swanson, 1994; Vandegehuchte and Steppe, 2013). Given the already broad scope of the paper, here we only provide a brief methodological overview, without delving into the details of the individual methods. Sap flow sensors track the fate of heat applied to the plant's conducting tissue, or sapwood, using temperature sensors (thermocouples or thermistors), usually deployed in the plant's main stem. Both heating and temperature sensing can be done either internally, by inserting needle-like probes containing electrical resistors (or electrodes for some methods) and temperature sensors into the sapwood (Vandegehuchte and Steppe, 2013), or externally; these latter systems are especially designed for small stems and non-lignified tissues (Clearwater et al., 2009; Helfter et al., 2007; Sakuratani, 1981). Depending on how the heat is applied and the principles underlying sap flow calculations, sap flow sensors can be classified into three major groups: heat dissipation methods, heat pulse methods, and heat balance methods (Flo et al., 2019). Heat dissipation and heat pulse methods estimate sap flow per unit sapwood area, and they have been called “sap flux density methods” (Vandegehuchte and Steppe, 2013); heat balance methods directly yield sap flow for the entire stem or for a sapwood section. Heat dissipation methods include the constant heat dissipation (HD; Granier, 1985, 1987), the transient (or cyclic) heat dissipation (CHD; Do and Rocheteau, 2002), and the heat deformation (HFD; Nadezhdina, 2018) methods. Heat pulse methods include the compensation heat pulse (CHP; Swanson and Whitfield, 1981), heat ratio (HR; Burgess et al., 2001), heat pulse T-max (HPTM; Cohen et al., 1981), and sapflow+ (Vandegehuchte and Steppe, 2012) methods. Heat balance methods include the trunk sector heat balance (TSHB; Čermák et al., 1973) and the stem heat balance (SHB; Sakuratani, 1981) methods. The suitability of a certain method in a given application largely depends on plant size and the flow range of interest (Flo et al., 2019), but heat dissipation and compensation heat pulse are the most widely used (Flo et al., 2019; Poyatos et al., 2016). Apart from these different methodologies, within each sap flow method sensor design (Davis et al., 2012) and data processing (Peters et al., 2018) can vary, resulting in relatively high levels of methodological variability comparable to those in other areas of plant ecophysiology.
The output from sap flow sensors is automatically recorded by data loggers at hourly or even higher temporal resolution. This output relates to heat transport in the stem and needs to be converted to meaningful quantities of water transport, such as sap flow per plant or per unit sapwood area. How this conversion is achieved varies greatly across methods, with some relying on empirical calibrations and others being more physically based and requiring the estimation of wood thermal properties and other parameters (Čermák et al., 2004; Smith and Allen, 1996; Vandegehuchte and Steppe, 2013). Depending on the method and the specific sensor design, sap flow measurements can be representative of single points, linear segments along the sapwood, sapwood area sections, or entire stems. Except for stem heat balance methods, which typically measure entire stems or large sapwood sections, most sap flow measurements need to be spatially integrated to account for radial (Berdanier et al., 2016; Cohen et al., 2008; Nadezhdina et al., 2002; Phillips et al., 1996) and azimuthal (Cohen et al., 2008; Lu et al., 2000; Oren et al., 1999a) variation of sap flow within the stem to obtain an estimate of whole-plant water use (Čermák et al., 2004). At a minimum, an estimate of sapwood area is needed to upscale the measurements to whole-plant sap flow rates. Sap flow rates can thus be expressed per individual (i.e. plant or tree), per unit sapwood area (normalizing by water-conducting area), and per unit leaf area (normalizing by transpiring area).
Here we will use the term “sap flow” when referring, in general, to the rate at which water moves through the sapwood of a plant and, more specifically, when we refer to sap flow per plant (i.e. water volume per unit time, Edwards et al., 1996). We acknowledge that the term “sap flux” has also been proposed for this quantity (Lemeur et al., 2009), but more generally “sap flux density” (e.g. Vandegehuchte and Steppe, 2013) or just “sap flux” are used to refer to “sap flow per unit sapwood area”. Since here we include methods natively measuring sap flow per plant or per sapwood area, throughout this paper we will use the more general term “sap flow”, and, when necessary, we will indicate explicitly the reference area used: “sap flow per (unit) sapwood area”, “sap flow per (unit) leaf area”, or “sap flow per (unit) ground area”.
2.2 Data compilation
SAPFLUXNET was conceived as a compilation of published and unpublished sap flow datasets (Appendix, Table A1), and thus the ultimate success of the initiative critically depended on the contribution of datasets by the sap flow community. An expression of interest showed that a critical mass of datasets with a wide geographic distribution could potentially be contributed, and the results of this survey were used to raise the interest of the sap flow community (Poyatos et al., 2016). The data contribution stage was open between July 2016 and December 2017, although a few additional datasets were updated during the data quality control process and contain more recent data.
All contributed datasets had to meet some minimum criteria before they were accepted, in terms of both content and format. We required that all datasets contained sub-daily, processed sap flow data, representative of whole-plant water use under different hydrometeorological conditions. This meant that both the processing from raw temperature data to sap flow quantities and the scaling from single-point measurements to whole-plant data had been performed by the data contributor responsible for each dataset. Time series of sap flow data and hydrometeorological drivers were required to be representative of one growing season, setting, as a broad reference, a minimum duration of 3 months. Sap flow could be expressed as total flow rate either per plant or per unit sapwood area. Contributors also needed to provide metadata on relevant ecological information of the site, stand, species, and measured plants as well as on basic technical details of the sap flow and hydrometeorological time series. Datasets had to be formatted using a documented spreadsheet template (cf. “sapfluxnet_metadata_template.xlsx” in the Supplement) and uploaded to a dedicated server at CREAF, Spain, using an online form.
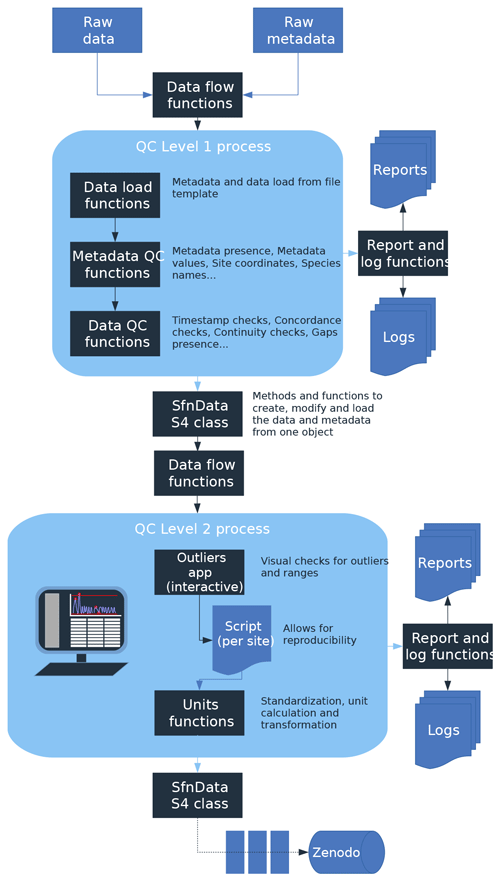
Figure 1Overview of the SAPFLUXNET data workflow. Data files are received from data contributors and undergo several quality-control processes (QC1 and QC2). Both QC1 and QC2 produce an .RData object of the custom-designed sfn_data S4 class storing all data, metadata, and data flags for each dataset. The progress and results of the QC processes are monitored through individual reports and log files. The final outcome is stored in a folder structure with a either single .RData file for each dataset or a set of seven csv files for each dataset.
2.3 Data harmonization and quality control: QC1
Once datasets were received, they were stored and entered a process of data harmonization and quality control (Fig. 1, Supplement Fig. S1). This process combined automatic data checks with human supervision, and the entire workflow was governed by functions and scripts in the R language (R Core Team, 2019), including other related tools, such as R markdown documents and Shiny applications. All R code involved in this QC process was implemented in the sapfluxnetQC1 package (Granda et al., 2016); see the package vignettes for a detailed description (https://github.com/sapfluxnet/sapfluxnetQC1/tree/master/vignettes, last access: 8 June 2021). To aid in the detection of potential data issues throughout the entire process (Figs. 1, S1), we implemented several elements of control: (1) automatic log files tracking the output of each QC function applied, (2) automatic creation and update of status files tracking the QC level reached by each dataset, (3) automatic QC summary reports in the form of R markdown documents, (4) interactive Shiny applications for data visualization, (5) documentation of manual changes applied to the datasets using manually edited text files, (6) storage of manual data cleaning operations in text files, and (7) automatic data quality flagging associated with each dataset. All these items ensure a robust, transparent, reproducible, and scalable data workflow. Example files for (2), (3), and (6) can be found in the Supplement.
The first stage of the data QC (QC1) performed several data checks (Supplement Table S1) on received spreadsheet files and produced an interactive report in an R markdown document, which signalled possible inconsistencies in the data and warned of potential errors. These data issues were addressed, with the help of data contributors if needed. Once no errors remained, the dataset was converted into an object of the custom-designed “sfn_data” class (Fig. S2; see also Sect. 2.5), which contained all data and metadata for a given dataset (Tables A2–A6 list all variable names and units). Data and metadata belonging to all Level 1 datasets were further visually inspected using an interactive R Shiny application, and, if no major issues were detected, they were subjected to the second QC process, QC2.
2.4 Data harmonization and quality control: QC2
Datasets entering QC2 underwent several data cleaning and data harmonization processes (Table S2). We first ran outlier detection and out-of-range checks; these checks did not delete or modify the data but only warned about any suspicious observation (“outlier” and “range” warnings). The outlier detection algorithm was based on a Hampel filter, which also estimates a replacement value for a candidate outlier (Hampel, 1974). For the range checks, we defined minimum and maximum allowed values for all the time series variables, based on published values of extreme weather records and maximum transpiration rates (Cerveny et al., 2007; Manzoni et al., 2013). The outcome of outlier and range checks were visually inspected on the actual time series being evaluated using an interactive R Shiny application (Fig. S3). Following expert knowledge, visually confirmed outliers were replaced by the values estimated by the Hampel filter. Similarly, we replaced out-of-range values with “NA” if the variable was out of its physically allowed range (Fig. S3). Outlier and out-of-range “warnings” for each observation (e.g. for each variable and times step) were documented in two data flags tables, with the same dimensions as the corresponding data tables (Fig. S2). Likewise, those observations with confirmed problematic values, which were removed or replaced, were also flagged; further information can be found in the “data flags” vignettes in the “sapfluxnetr” package (Granda et al., 2019).
Final data harmonization processes in QC2 involved unit transformations and the calculation of derived variables (Table S2). When plant sapwood area was provided by data contributors, we interconverted between sap flow rate per plant and per unit sapwood area. If leaf area was supplied, we also calculated sap flow per unit leaf area, but note that this transformation does not take into account the seasonal variation in leaf area; we document in the metadata for which datasets this information could be available from data contributors. In QC2 we estimated missing environmental variables which could be derived from related variables in the dataset (Appendix, Table A6). We also estimated the apparent solar time and extraterrestrial global radiation from the provided timestamp and geographic coordinates using the R package “solaR” (Perpiñán, 2012). All estimated or interconverted observations were flagged as “CALCULATED” in the “env_flags” or “sap_flags” table (Fig. S2).
2.5 Data structure
One of the major benefits of the SAPFLUXNET data workflow is the encapsulation of datasets in self-contained R objects of the S4 class with a predefined structure. These objects belong to the custom-designed sfn_data class, which displays different slots to store time series of sap flow and environmental data, their associated data flags, and all the metadata (Fig. S2). For further information please see the “sfn_data classes” vignette in the sapfluxnetr package (Granda et al., 2019). The code identifying each dataset was created by the combination of a “country” code; a “site” code; and, if applicable, a “stand” code and a “treatment” code. This means that several stands and/or treatments can be present within one site (Table S3).
At the end of the QC process, we generated a folder structure with a first-level storing datasets as either sfn_data objects or as a set of comma-separated (csv) text files. Within each of these formats, a second-level folder groups datasets according to how sap flow is normalized (per plant, sapwood, or leaf area); note that the same dataset, expressing different sap flow quantities, can be present in more than one folder (e.g. “plant” and “sapwood”). Finally, the third level contains the data files for each dataset: either a single sfn_data object storing all data and metadata or all the individual csv files. More details on the data structure and units can be found in the “sapfluxnetr-quick-guide” and “metadata-and-data-units” vignettes, respectively, in the sapfluxnetr package (Granda et al., 2019).
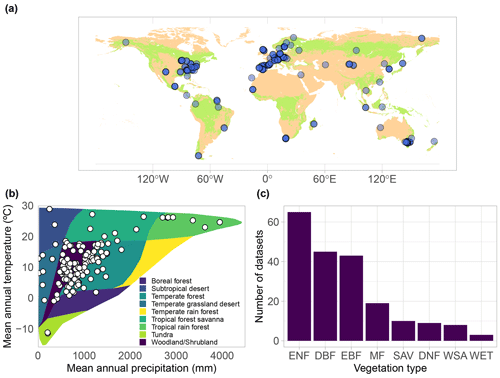
Figure 2(a) Geographic, (b) bioclimatic, and (c) vegetation type distribution of SAPFLUXNET datasets. In panel (a), woodland area from Crowther et al. (2015) is shown in green. In panel (b), we represent the different datasets according to their mean annual temperature and precipitation in a Whittaker diagram showing the classification of the main terrestrial biomes. In panel (c), vegetation types are defined according to the International Geosphere-Biosphere Programme (IGBP) classification (ENF: evergreen needleleaf forest; DBF: deciduous broadleaf forest; EBF: evergreen broadleaf forest; MF: mixed forest; DNF: deciduous needleleaf forest; SAV: savannas; WSA: woody savannas; WET: permanent wetlands).
3.1 Data coverage
The SAPFLUXNET version 0.1.5 database harbours 202 globally distributed datasets (Figs. 2a and S4, Table S3), from 121 geographical locations, with Europe, the eastern USA, and Australia especially well represented. These datasets were represented in the bioclimatic space using the terrestrial biomes delimited by Whittaker (Fig. 2b), but note that, as any bioclimatic classification, it has its limitations. Datasets have been compiled from all terrestrial biomes, except for temperate rain forests, although some tropical montane sites have been included. Woodland/shrubland and temperate forest biomes are the most represented in the database, adding up to 80 % of the datasets (Fig. 2b). However, large forested areas in the tropics and in boreal regions are still not well represented (Fig. 2a and b). Looking at the distribution by vegetation type (Fig. 2c), evergreen needleleaf forest is the most represented vegetation type (65 datasets), followed by deciduous broadleaf forest (47 datasets) and evergreen broadleaf forest (43 datasets).
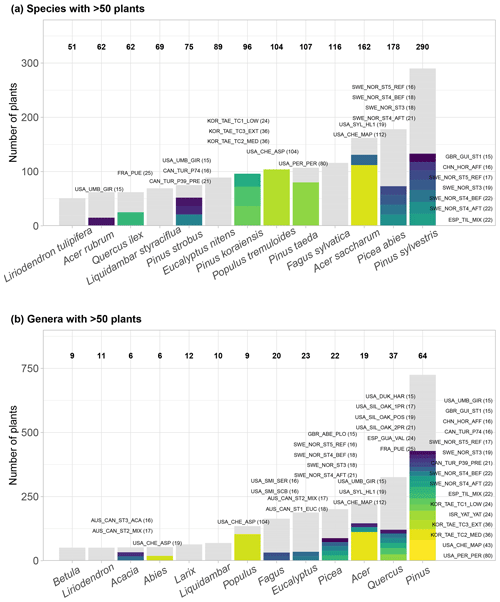
Figure 3Taxonomic distribution of genera and species in SAPFLUXNET, showing (a) species and (b) genera with >50 plants in the database. Total bar height depicts number of plants per species (a) or genus (b). Numbers on top of each bar show the number of datasets where each species (a) or genus (b) is present. Colours other than grey highlight datasets with 15 or more plants of a given species (a) or genus (b). Bar height for a given colour is proportional to the number of plants in the corresponding dataset, which is also shown in parentheses next to the dataset code.
SAPFLUXNET contains sap flow data for 2714 individual plants (1584 angiosperms and 1130 gymnosperms), belonging to 174 species (141 angiosperms and 33 gymnosperms), 95 different genera, and 45 different families (Supplement, Tables S4–S5). All species but one – Elaeis guineensis, a palm – are tree species. Pinus and Quercus are the most represented genera (Fig. 3b). Amongst the gymnosperms, Pinus sylvestris, Picea abies, and Pinus taeda are the three most represented species, with data provided on 290, 178, and 107 trees, respectively (Fig. 3a). For the angiosperms, Acer saccharum, Fagus sylvatica, and Populus tremuloides are the most represented species, with 162, 116, and 104 trees, respectively, although most Acer saccharum data come from a single study with a very large sample size (Fig. 3a). Some species are present in more than 10 datasets: Pinus sylvestris, Picea abies, Fagus sylvatica, Acer rubrum, Liriodendron tulipifera, and Liquidambar styraciflua (Fig. 3a, Table S4).
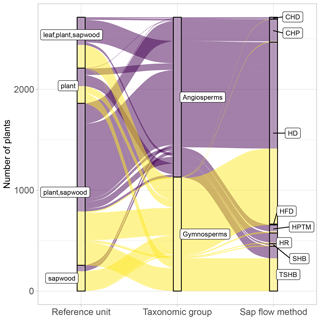
Figure 4Distribution of plants in SAPFLUXNET according to major taxonomic group (angiosperms, gymnosperms), sap flow method (CHD: cycling heat dissipation; CHP: compensation heat pulse; HD: heat dissipation; HFD: heat field deformation: HPTM: heat pulse T-max (HPTM): HRM: heat ratio (HR); SHB: stem heat balance; TSHB: trunk sector heat balance), and reference unit for the expression of sap flow (plant, sapwood area, leaf area). Combinations of reference units imply that data are present in multiple units.
3.2 Methodological aspects
For more than 90 % of the plants, sap flow at the whole-plant level is available (either directly provided by contributors or calculated in the QC process); this is important for upscaling SAPFLUXNET data to the stand level (cf. Sect. 4.2). Because the leaf area of the measured plants is often not available as metadata, sap flow per unit leaf area was estimated for only 18.6 % of the individuals (Fig. 4). The heat dissipation method is the most frequent method in the database (HD, 66.4 % of the plants), followed by the trunk sector heat balance (TSHB, 16.4 %) and the compensation heat pulse method (CHP, 8.4 %) (Fig. 4). This distribution is broadly similar to the use of each method documented in the literature, although the TSHB method is overrepresented here, compared to the current use of this method by the sap flow community (Flo et al., 2019; Poyatos et al., 2016). Some methods, especially those belonging to the heat pulse family and the cyclic (or transient) heat dissipation (CHD) method, are mostly used in angiosperms, while the TSHB and the heat field deformation (HFD) methods are more frequently used in gymnosperms (Fig. 4).
Table 1Number of sap flow times series in SAPFLUXNET depending on whether they were calibrated (species-specific) or non-calibrated, or whether this information was not provided, for the different sap flow methods: cyclic (or transient) heat dissipation (CHD), compensation heat pulse (CHP), heat dissipation (HD), heat field deformation (HFD), heat pulse T-max (HPTM), heat ratio (HR), stem heat balance (SHB), and trunk sector heat balance (TSHB). The percentage of calibrated time series was expressed with respect to the total number of sap flow time series for each method.
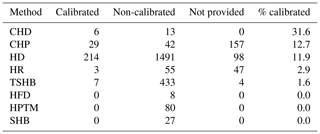
Calibration of sap flow sensors and scaling from point measurements to the whole-plant can be critical steps towards accurate estimates of absolute sap flow rates. In SAPFLUXNET, most of the sap flow time series have not undergone a species-specific calibration, with the CHD method showing the highest percentage of calibrated time series (Table 1). This lack of calibrations may be relevant for the more empirical heat dissipation methods (HD and CHD), which have been shown to consistently underestimate sap flow rates by 40 % on average (Flo et al., 2019; Peters et al., 2018; Steppe et al., 2010). Radial integration of single-point sap flow measurements is more frequent than azimuthal integration (Table 2), except for the CHD method. For a large number of plants measured with the HD method and all plants measured with the HPTM method, there was not any radial integration procedure reported. In contrast, the CHP, HR, SHB, and TSHB methods are those which more frequently addressed radial variation in one way or another (Table 2). Azimuthal integration procedures are also more frequent when the TSHB method is used (Table 2).
Table 2Number of plants in the SAPFLUXNET database using different radial and azimuthal integration approaches for the different sap flow methods: cyclic (or transient) heat dissipation (CHD), compensation heat pulse (CHP), heat dissipation (HD), heat field deformation (HFD), heat pulse T-max (HPTM), heat ratio (HR), stem heat balance (SHB), and trunk sector heat balance (TSHB).
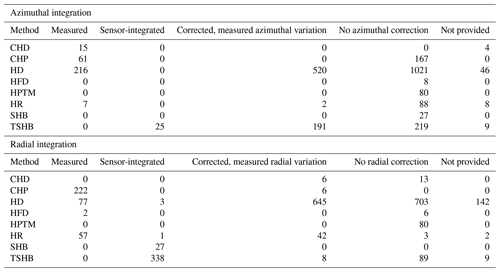
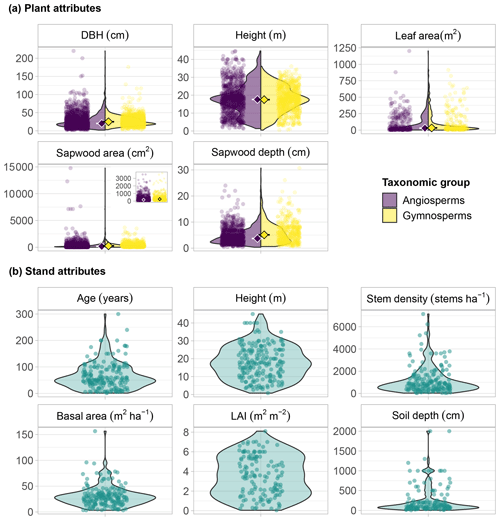
Figure 5Characteristics of trees and stands in the SAPFLUXNET database. Panel (a) shows plant data and kernel density plots of the main plant attributes, coloured by taxonomic group (angiosperms and gymnosperms): diameter at breast height (DBH), plant height, sapwood area, sapwood depth, and leaf area. The inset in the sapwood area panel zooms in on values lower than 5000 cm2. Panel (b) shows stand data and kernel density plots of the main stand attributes: stand age, stand height, stem density, stand basal area, leaf area index (LAI), and soil depth.
3.3 Plant characteristics
Plant-level metadata are almost complete (99.5 % of the individuals) for diameter at breast height (DBH), while sapwood area and sapwood depth, important variables for sap flow upscaling, are not available, or could not be estimated, for 23 % and 47 % of the plants, respectively. Plant height and plant age are missing for 42 % and 62 % of the individuals, respectively. Sap flow data in SAPFLUXNET are representative of a broad range of plant sizes (Fig. 5a). The distribution of DBH showed a median of 25.0 and 20.4 cm for gymnosperms and angiosperms, respectively, with a long tail towards the largest plants, two Mortoniodendron anisophyllum trees from a tropical forest in Costa Rica that measured >200 cm (Fig. 5a). The largest gymnosperm tree in SAPFLUXNET (176 cm in DBH) is a kauri tree (Agathis australis) from New Zealand. The distribution of plant heights is less skewed, with similar medians for angiosperms (17.6 m) and gymnosperms (17.5 m). The tallest plants are located in a tropical forest in Indonesia, where a Pouteria firma tree reached 44.7 m. Remarkably, of the 16 plants taller than 40 m, over 60 % are Eucalyptus species. The tallest gymnosperm (36.2 m) is a Pinus strobus from the northeastern USA.
Plant size metadata in SAPFLUXNET are complemented with plant-level data of sapwood and leaf area, which provide information on the functional areas for water transport and loss (Fig. 5a). Distributions of sapwood and leaf area show highly skewed distributions, with long tails towards the largest values and slightly higher median values for gymnosperms (262 cm2 and 33.0 m2 for sapwood and leaf areas, respectively) compared to angiosperms (168 cm2 and 29.9 m2). Accordingly, median sapwood depth is also higher for gymnosperms (5.1 cm) compared to angiosperms (3.7 cm). The largest trees (Mortoniodendron, Pouteria, Agathis) with deep sapwood (17–24 cm) are also those with largest sapwood areas. Many large angiosperm trees from tropical (CRI_TAM_TOW, IDN_PON_STE, GUF_GUY_ST2; see Table S3 for dataset codes) and temperate forests (Fagus grandifolia, USA_SMIC_SCB) also show large sapwood areas (>5000 cm2), but the plant with the deepest sapwood is a gymnosperm, an Abies pinsapo in Spain with 30.7 cm of sapwood depth.
3.4 Stand characteristics
Stand-level metadata include several variables associated with management, vegetation structure, and soil properties. Half of the datasets originate from naturally regenerated, unmanaged stands, and 13.9 % come from naturally regenerated but managed stands. Plantations add up to 32.2 %, and orchards only represent 4 % of the datasets. Reporting of structural variables is mixed, with stand height, age, density, and basal area showing relatively low missingness (6.4 %, 11.4 %, 12.9 %, and 13.4 %, respectively); in contrast, soil depth and leaf area index (LAI) are missing from 26.7 % and 33.7 % of the datasets.
SAPFLUXNET datasets originate from stands with diverse structural characteristics. Median stand age is 54 years, and there are several datasets coming from >100-year-old forests (Fig. 5b). Stand height shows a similar range and distribution of values compared to individual plant height (Fig. 5a and b). The denser stands correspond to coppiced evergreen oak stands from Mediterranean forests (FRA_PUE, ESP_TIL_OAK), species-rich tropical forests (MDG_SEM_TAL), or relatively young temperate forests (e.g. FRA_HES_HE1_NON, USA_CHE_MAP). The sparsest stands (<200 stems ha−1) correspond to tree–grass savanna systems (Spain, Portugal, Australia, Senegal), dry woodlands (China), or oil palm plantations in Indonesia (IDN_JAM_OIL). Stands with the largest basal areas (>70 m2 ha−1) are mostly dominated by broadleaf species, except for a Picea abies plantation in Sweden (SWE_SKO_MIN).
The distribution of LAI shows a median of 3.5 m2 m−2, with the largest values observed in temperate (CZE_BIK, USA_DUK_HAR, HUN_SIK) and tropical (GUF_GUY_GUY, COL_MAC_SAF_RAD) forests. The stands with the lowest LAI correspond to the sparse woodlands from Mediterranean and semi-arid locations and also those from forests near altitudinal or latitudinal treelines (FIN_PET, AUT_TSC). SAPFLUXNET datasets show a median soil depth of 100 cm, with only a dozen datasets originated from sites with soils deeper than 10 m (Fig. 5b).
The number of plants per dataset is highly variable, with most of the datasets (86 %) containing data for at least 4 trees and 46 % of the datasets having data for at least 10 trees (Fig. 6a; see also Fig. 9).
3.5 Temporal characteristics
The oldest datasets in SAPFLUXNET go back to 1995 (GBR_DEV_CON, GBR_DEV_DRO), while the most recent data reach up to 2018 (datasets from the ESP_MAJ cluster of sites). Several multi-year datasets are present in SAPFLUXNET (Fig. 6), with 50 % of the datasets spanning a period of at least 3 years and some datasets being extraordinarily long (16 years in FRA_PUE). Frequently, the datasets only cover the “growing season” periods, or even shorter periods for some sites which were eventually included because they improved the ecological and geographic coverage of the database (e.g. ARG_MAZ, ARG_TRE as representative of deciduous Nothofagus forest in southern Patagonia). In contrast, a few datasets show continuous records over multiple years (Fig. 6b). Amongst the longest datasets, most of them come from European or North American sites (Fig. 6), except some datasets from Israel (ISR_YAT_YAT, 7 years), Russia (RUS_FYO, 7 years), South Korea (KOR_TAE cluster of sites, 6 years), or New Zealand (NZL_HUA_HUA, 5 years).
SAPFLUXNET provides an unprecedented database to study the detailed temporal dynamics of plant transpiration across species and sites globally. Sub-daily records of sap flow (e.g. at least at hourly time steps) are available for extended periods (Fig. 6b), allowing both seasonal and diel patterns in water-use regulation by trees to be addressed, as well as how these temporal patterns change across species or years across terrestrial biomes, reflecting different phenologies and water-use strategies. For instance, in Mediterranean forests, evergreen species such as Quercus ilex, Arbutus unedo, and Pinus halepensis show moderate sap flow the whole year round, while the deciduous Quercus pubescens shows higher sap flow density during a shorter period and its water use is heavily reduced during a dry year (2012) (Fig. 7a). Temperate forests without water availability limitations show relatively high flows during the growing season and similar diel sap flow patterns amongst species (Fig. 7b). In contrast, tropical forests show moderate to high sap flow rates during the entire year, with different dynamics in the intradaily water-use regulation across species. For example, Inga sp. in a highly diverse wet tropical forest in Costa Rica reduced sap flow during mid-day hours compared to co-existing species (Fig. 7c).
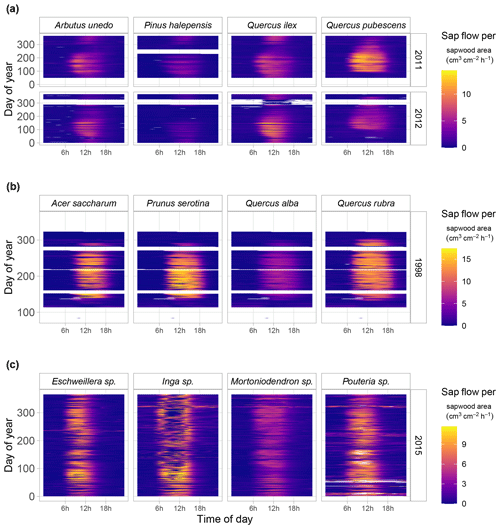
Figure 7Fingerprint plots showing hourly sap flow per unit sapwood area (colour scale) as a function of hour of day (x axis) and day of year (y axis) for a selection of SAPFLUXNET sites with at least four co-occurring species. Panel (a) shows data from a woodland/shrubland forest in NE Spain (ESP_CAN) for an average (2011) and a dry (2012) year. Panel (b) shows data for a mesic temperate forest (USA_WVF), and panel (c) shows data for a tropical forest (CRI_TAM_TOW). For the latter site, only 4 of the 17 measured species are shown, and some of them were only identified at the genus level.
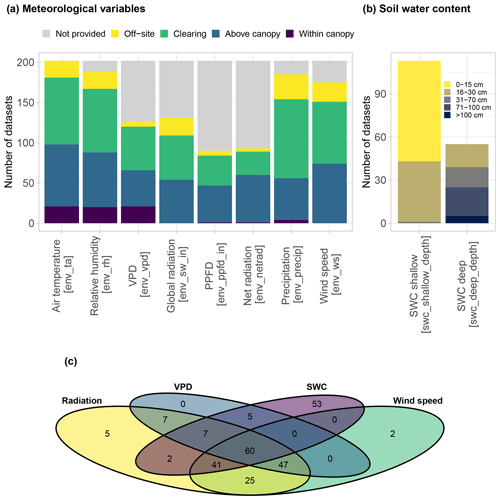
Figure 8Summary of the availability of different environmental variables in SAPFLUXNET datasets. (a) Distribution of meteorological variables according to sensor location (in brackets, names of the variables in the database). (b) Distribution of soil moisture variables according to the measurement depth (in brackets, names of the variables in the database). (c) Venn diagram showing the number of datasets where each combination of different environmental variables are present, grouping shortwave, photosynthetic photon flux density (PPFD), and net radiation under “radiation” variables.
3.6 Availability of environmental data
All SAPFLUXNET datasets contain ancillary time series of the main hydrometeorological drivers of transpiration, accompanied by information on where these variables had been measured (Fig. 8a). Air temperature is available for all datasets. Although vapour pressure deficit (VPD) was originally absent in 38 % of the datasets (Fig. 8a and b), we could estimate it for those sites providing air temperature and relative humidity data (QC Level 2; see Sect. 2.3), and finally only 2 out of the 202 datasets have missing VPD information. For radiation variables, shortwave radiation was most often provided, compared to photosynthetically active and net radiation, which were less provided; only 8 out of 202 datasets do not have any accompanying radiation data. Most of these environmental variables were measured on site, with precipitation being the variable most frequently retrieved from nearby meteorological stations (48 % of the datasets) (Fig. 8a). Soil water content measured at shallow depth, typically between 0 and 30 cm below the soil surface, is provided for 56 % of the datasets, while soil moisture from deep soil layers is available for only 27 % of the datasets.
3.7 Uncertainty estimation and bias correction in sap flow measurements
Uncertainty for the main sap flow density methods could be obtained by using a recent compilation of sap flow calibration data (Flo et al., 2019) (Table B1); these calibrations generally covered the range of sap flow per sapwood area observed in SAPFLUXNET, except for the CHP method (Fig. B1). At low flows, uncertainties were larger for HPTM and, to a lesser extent, for CHP, while they were lowest for HR and HFD. Uncertainties increased steeply with flow particularly for the HPTM, CHP, and HR methods. These patterns were evident when examining sub-daily sap flow measured with the most represented sap flux density methods in SAPFLUXNET (Fig. B2). The analysis of calibration data also showed that HD, the most represented method by far, underestimates water flow, on average, by 40 % (Flo et al., 2019) when using the original calibration (Granier, 1985, 1987). Because plant-level metadata contain information that document the conversion from raw to processed data, a first-order correction for data from uncalibrated HD probes can be applied (Fig. B3a).
Additional uncertainties and corrections by sapwood area estimation and integration of sap flow radial variability must also be considered when upscaling to plant-level sap flow. Uncertainty from sapwood area estimation is expected to be lower than methodological uncertainty given the generally tight relationship between basal area and sapwood area (Fig. B3b and c). Data without an explicit radial integration of sap flow measurements can be adjusted using generic radial sap flow profiles based on wood type (Berdanier et al., 2016). In this case, assuming uniform sap flow along the sapwood usually leads to sap flow overestimation for both ring-porous and diffuse-porous species (Fig. B4).
4.1 Applications in plant ecophysiology and functional ecology
There are multiple potential applications of the SAPFLUXNET database to assess whole-plant water-use rates and their environmental sensitivity, both across species (e.g. Oren et al., 1999b) and at the intraspecific level (Poyatos et al., 2007). SAPFLUXNET will allow disentangling the roles of evaporative demand and soil water content in controlling transpiration at the plant level, complementing recent studies looking at how water supply and demand affect evapotranspiration at the ecosystem level (Anderegg et al., 2018; Novick et al., 2016). The availability of global sap flow data at sub-daily time resolution and spanning entire growing seasons will allow focusing on how maximum water use and its environmental sensitivity vary with plant-level attributes such as stem diameter (Dierick and Hölscher, 2009; Meinzer et al., 2005), tree height (Novick et al., 2009; Schäfer et al., 2000), hydraulic traits (Manzoni et al., 2013; Poyatos et al., 2007), and other plant traits (Grossiord et al., 2020; Kallarackal et al., 2013). SAPFLUXNET thus provides an unprecedented tool to understand how structural and physiological traits coordinate with each other (Liu et al., 2019), how these traits translate to whole-plant regulation of water fluxes (McCulloh et al., 2019), and how this integration determines drought responses (Choat et al., 2018) and post-drought recovery patterns (Yin and Bauerle, 2017). Analyses of the temporal dynamics of plant water use in response to specific drought events, as recently assessed for gross primary productivity (e.g. Schwalm et al., 2017), can also help to quantify drought legacy effects. If combined with water potential measurements, sap flow data can be used to estimate whole-plant hydraulic conductance and study its response to drought (e.g., Cochard et al., 1996), as well as the recovery of the plant hydraulic system after drought.
SAPFLUXNET will allow new insights into within-day patterns and controls in whole-plant water use, which can disclose the fine details of its physiological regulation. Circadian rhythms can modulate stomatal responses to the environment, potentially affecting sap flow dynamics (e.g. de Dios et al., 2015). Hysteresis in diel sap flow relationships with evaporative demand and time lags between transpiration and sap flow are two linked phenomena likely arising from plant capacitance and other mechanisms (O'Brien et al., 2004; Schulze et al., 1985) that also influence diel evapotranspiration dynamics (Matheny et al., 2014; Zhang et al., 2014). A major driver of time lags is the use of stored water to meet the transpiration demand (Phillips et al., 2009), which can now be analysed across species, plant sizes, or drought conditions using time series analyses, simplified electric analogies (Phillips et al., 1997, 2004; Ward et al., 2013), or detailed water transport models (Bohrer et al., 2005; Mirfenderesgi et al., 2016). Night-time water use can be substantial for some species (Forster, 2014; Resco de Dios et al., 2019). However, available syntheses rely on study-specific quantification of what constitutes nocturnal sap flow and do not address possible methodological influences (Zeppel et al., 2014). SAPFLUXNET includes metadata to identify methods (e.g. HRM; Burgess et al., 2001) and data processing approaches (zero-flow determination method in “pl_sens_cor_zero”, Table A5) that can help identify suitable datasets to quantify night-time fluxes.
Sap flow data have been widely employed to assess changes in tree water use after biotic (e.g. Hultine et al., 2010) or abiotic (Oren et al., 1999a) disturbances. Likewise, sap flow data have been used to report changes in species and stand water use following experimental treatments involving resource availability modifications (e.g. Ewers et al., 1999) or density changes (i.e. thinning; Simonin et al., 2007). The SAPFLUXNET database includes datasets with experimental manipulations, applied either at the stand or at the individual level, qualitatively documented in the metadata (Table 3). The main treatments present are related to thinning, water availability changes (irrigation, throughfall exclusion), and wildfire impact (Table 3), potentially facilitating new data syntheses and meta-analyses using these datasets (e.g. Grossiord et al., 2018).
The combination of SAPFLUXNET with other ecophysiological databases can be informative as to the relative sensitivity of different physiological processes in response to drought, for example those related to growth and carbon assimilation (Steppe et al., 2015). Within-day fluctuations of stem diameter can be jointly analysed with co-located sap flow measurements to study the dynamics of stored water use under drought and its contribution to transpiration (e.g. Brinkmann et al., 2016) and to infer parameters on tree hydraulic functioning using mechanistic models of tree hydrodynamics (Salomón et al., 2017; Steppe et al., 2006; Zweifel et al., 2007). These analyses could be carried out for a large number of species by combining SAPFLUXNET with data from the DENDROGLOBAL database (http://78.90.202.92/streess/databases/dendroglobal, last access: 8 June 2021); there are at least 18 SAPFLUXNET datasets with dendrometer data in DENDROGLOBAL. This database and the International Tree-Ring Data Bank (S. Zhao et al., 2019) could also be used with SAPFLUXNET to investigate, at the species level, the link between radial growth and water use, including their environmental sensitivity (Morán-López et al., 2014), and how these two processes comparatively respond to drought (Sánchez-Costa et al., 2015). Moreover, given the tight link between water use and carbon assimilation, combining SAPFLUXNET with water-use efficiency from plant δ13C data could potentially be used to estimate whole-plant carbon assimilation (Hu et al., 2010; Klein et al., 2016; Rascher et al., 2010; Vernay et al., 2020), a quantity that is difficult to measure directly, especially in field-grown, mature trees.
4.2 Applications in ecosystem ecology and ecohydrology
SAPFLUXNET will provide a global look at plant water flows to bridge the scales between plant traits and ecosystem fluxes and properties (Reichstein et al., 2014). Vegetation structure, species composition, and differential water-use strategies amongst and within species scale up to different seasonal patterns of ecosystem transpiration, with a strong influence on ecosystem evapotranspiration and its partitioning. Global controls on evaporative fluxes from vegetation have been mostly addressed using ecosystem (Williams et al., 2012) or catchment evapotranspiration data (Peel et al., 2010). These studies have described global patterns in evapotranspiration driven by different plant functional types or climates, but they cannot be used to quantify and to explain the enormous variation in the regulation of transpiration across and within taxa.
The SAPFLUXNET database will provide a long-demanded data source to be used in ecohydrological research (Asbjornsen et al., 2011). Upscaling individual measurements to the stand level (Čermák et al., 2004; Granier et al., 1996; Köstner et al., 1998) is necessary to quantitatively compare sap-flow-based transpiration with evapotranspiration and transpiration estimates at the ecosystem scale and beyond. Even though SAPFLUXNET was designed to accommodate sap flow data at the plant level, scaling to the ecosystem level is possible for many datasets. For a basic upscaling exercise using SAPFLUXNET data (Poyatos et al., 2020b), whole-plant sap flow can be normalized by individual basal area (as DBH is usually available in the metadata; cf. Sect. 3.3), averaged for a given species, and then scaled to stand-level transpiration using total stand basal area and the fraction of basal area occupied by each measured species (see stand metadata, Table A3). For many datasets, sap flow data are available for the species comprising most of the stand basal area (often even 100 %, Fig. 9), but species-based upscaling may be unfeasible in many tropical sites (Fig. 9b), where size-based scaling could be applied instead (e.g. da Costa et al., 2018). Further refinements of the upscaling procedure could be achieved by using trunk diameter distributions of the sap flow plots (Berry et al., 2018). This information, however, is not readily available in SAPFLUXNET, and other data sources (e.g. forest inventories, LIDAR data) or additional simplifying assumptions (i.e. applying the size distribution of measured individuals in the dataset) would be needed.
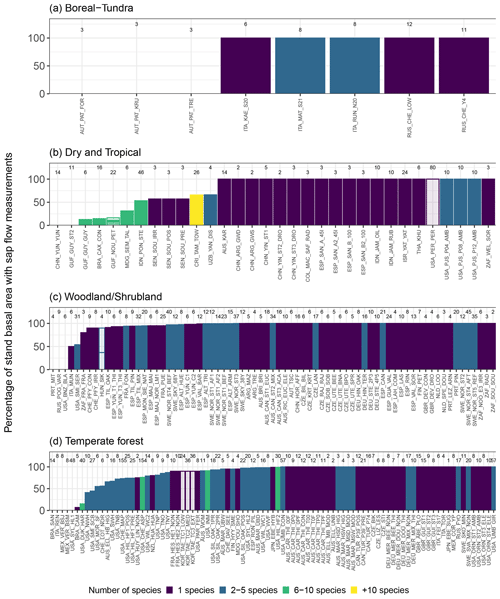
Figure 9Potential for upscaling species-specific plant sap flow to stand-level sap flow using SAPFLUXNET datasets. Datasets are shown using an aggregated biome classification; “dry and tropical” include “subtropical desert”, “temperate grassland desert”, “tropical forest savanna”, and “tropical rain forest”. Each panel shows the percentage of total stand basal area that is covered by sap flow measurements for each species in the dataset. Datasets are also coloured by the number of species present. Numbers on top of each bar depict the total number of plants for a given dataset. Empty bars show datasets for which sap flow data expressed at the plant level were not available.
Stand-level transpiration estimates from a large number of SAPFLUXNET sites can contribute to improve our understanding of the role of forest transpiration in the context of stand water balance and its components at the ecosystem (e.g. Tor-ngern et al., 2018) and catchment levels (Oishi et al., 2010; Wilson et al., 2001). Importantly, SAPFLUXNET can contribute to a better understanding of the global controls on vegetation water use (Good et al., 2017), including the biological and climatic controls on evapotranspiration partitioning into transpiration and evaporation components (Schlesinger and Jasechko, 2014; Stoy et al., 2019). There is some overlap between the FLUXNET network and SAPFLUXNET (47 datasets from FLUXNET sites). Hence, transpiration from SAPFLUXNET can also be used as a “ground-truth” reference for transpiration estimates from remote sensing approaches (Talsma et al., 2018) and from eddy covariance data (Nelson et al., 2020). Extrapolating sap-flow-derived stand transpiration to large spatial scales can be challenging due to landscape-scale variation in forest structure (Ford et al., 2007) or topography (Hassler et al., 2018) and due to the low spatial representativeness of sap flow measurements (Mackay et al., 2010). A promising research avenue to help elucidate the role of vegetation in driving hydrological changes across environmental gradients (Vose et al., 2016) would be to combine species-specific stand transpiration data from SAPFLUXNET with stand structural and compositional data from forest inventories (e.g. sapwood area index; Benyon et al., 2015).
Understanding the patterns and mechanisms underlying species interactions with respect to water use within a community is necessary to predict tree species vulnerability to drought (Grossiord, 2020). Multispecies datasets from SAPFLUXNET (Table S3) can be used to assess competition for water resources amongst species, for example by identifying changes in seasonal water use across co-existing species and hence characterizing the spatiotemporal segregation of their hydrological niches (Silvertown et al., 2015). By providing a detailed seasonal quantification of tree water use, SAPFLUXNET could also complement isotope-based studies and contribute to interpreting the large diversity in root water uptake patterns observed worldwide (Barbeta and Peñuelas, 2017; Evaristo and McDonnell, 2017) and to explaining the different seasonal origin of root-absorbed water across species and environmental gradients (Allen et al., 2019).
Plant water fluxes and hydrodynamics are amongst the most uncertain components of ecosystem and terrestrial biosphere models (Fatichi et al., 2016; Fisher et al., 2018). These models are now incorporating hydraulic traits and processes in their transpiration regulation algorithms (Mencuccini et al., 2019), but multi-site assessments of these algorithms are usually performed against evapotranspiration from eddy flux data (Knauer et al., 2015; Matheny et al., 2014). Model validation against sap flow data has been carried out typically at only one (Kennedy et al., 2019; Williams et al., 2001) or few (Buckley et al., 2012) sites. SAPFLUXNET can thus contribute to assessing the performance of models simulating transpiration of stands or species within stands (e.g. De Cáceres et al., 2021), for a large number of species and under diverse climatic conditions.
5.1 Limitations
Sap flow data processing differs within and amongst methods, because different algorithms, calibrations, or parameters involved in sap flow calculations may be applied. All of these methods contribute to methodological uncertainty (Looker et al., 2016; Peters et al., 2018), and this challenging methodological variability precludes the implementation of a complete, standardized data workflow from raw to processed data within SAPFLUXNET, as it is done for eddy flux data (Vitale et al., 2020; Wutzler et al., 2018). Commercial software for sap flow data processing from multiple methods is available (i.e. http://www.sapflowtool.com/SapFlowToolSensors.html, last access: 8 June 2021), but it has not yet been widely adopted. Freely available data-processing software is only available for the HD method (Oishi et al., 2016; Speckman et al., 2020; Ward et al., 2017). Open-source software also allows a seamless integration of different data processing approaches and the implementation of species-specific calibrations, which can contribute to obtaining more robust estimations of sap flow and facilitate replicability (Peters et al., 2021).
Sap flow measured with thermometric methods provides a precise estimate of the temporal dynamics of water flow through plants (Flo et al., 2019). However, their performance in measuring absolute flows is mixed. While some well-represented methods in SAPFLUXNET, such as CHP, yield accurate estimates (at least for moderate-to-high flows), the HD method, the most represented method by far, can significantly underestimate sap flow. Our suggested bias correction for uncalibrated HD data (cf. Sect. 3.7) can be applied, but, given the unexplained high variability (i.e. by species and wood traits) in the performance of sap flow calibrations (Flo et al., 2019), these corrections should be applied with caution.
SAPFLUXNET has been designed to store whole-plant sap flow data, and therefore sap flow measured at multiple points within an individual is not available in the database. Even though this spatial variation could be useful to describe detailed aspects of plant water transport (Nadezhdina et al., 2009), focusing on plant-level data greatly simplifies the data structure. Hence, SAPFLUXNET only includes data already upscaled to the plant level by the data contributors. The main details of how this upscaling process was done for each dataset are provided together with other plant metadata (Table A5), but these metadata show that within-plant variation in sap flow is often not considered (Table 2). For those datasets without radial integration of point measurements, we show how to implement a radial integration based on generic wood porosity types (cf. Sect. 3.7, Appendix B). The impact of not accounting for radial and circumferential variability when scaling single-point measurements of sap flow to the whole-plant level can be important (Merlin et al., 2020), but the estimation of sapwood area can also cause large errors if it is not accurately determined (Looker et al., 2016). SAPFLUXNET does not provide information on the method employed to quantify sapwood area (e.g. visual estimation with or without the application of dyes, indirect estimation through allometries at species or site levels) or on the accuracy of sapwood area data. This precludes uncertainty estimation at the individual level (Fig. B3). Future developments in the SAPFLUXNET data structure could include this information as metadata to better document the sensor-to-plant scaling process. Overall, this first global compilation of sap flow data will allow addressing uncertainties in sap flow upscaling in space and time in the same way that the development of FLUXNET stimulated the quantification and aggregation of uncertainties for eddy flux data (Richardson et al., 2012).
While SAPFLUXNET makes global sap flow data available for the first time, we note that spatial coverage is still sparse and some forested regions are underrepresented in the database (Fig. 2a). We note especially the relatively small number of datasets for boreal and tropical forests, two important biomes in terms of global water and carbon fluxes (Beer et al., 2010; Schlesinger and Jasechko, 2014). While many geographic gaps are caused by the absence of sap flow studies from such areas, some regions where sap flow studies have been conducted are still not represented in SAPFLUXNET. For example, the recent proliferation of Asian sap flow studies (Peters et al., 2018) has not translated into a high representativity of Asian datasets in SAPFLUXNET yet. Similarly, while the coverage of taxonomic and biometric diversity is unprecedented, SAPFLUXNET lacks data for the extremely tall trees (Ambrose et al., 2010) or for other growth forms such as shrubs (Liu et al., 2011), lianas (Chen et al., 2015), and other non-woody species (Lu et al., 2002).
5.2 Outlook
The public release of SAPFLUXNET has set the stage for the first generation of sap-flow-based data syntheses. The work on these syntheses will fuel new ideas and tools for future improvements of the database, for example new computing approaches for the processing and analysis of sap flow datasets. One example would be the development of robust imputation algorithms to gap-fill time series of sap flow and environmental data, which can take advantage of tools and datasets already developed by the ecosystem flux community (Moffat et al., 2007; Vuichard and Papale, 2015). The dissemination of SAPFLUXNET will encourage the use of machine learning algorithms, only occasionally used to analyse sap flow datasets so far (e.g. Whitley et al., 2013). These approaches can also be used to identify the relative importance of different hydrometeorological drivers of transpiration (W. L. Zhao et al., 2019), or to produce global transpiration maps, by combining SAPFLUXNET with other data (Jung et al., 2019). This upscaling of stand transpiration to large areas will also allow addressing broader questions at the regional and continental scale, such as the role of transpiration in moisture recycling (Staal et al., 2018).
The eventual success of this initiative, in terms of enabling data re-use and contributing to the understanding and modelling of tree water use at local to global scales, will likely encourage the sap flow community to contribute new datasets to future updates of the database. We expect that the development of open-source software for the processing of raw sap flow data (Peters et al., 2021; Speckman et al., 2020), its eventual widespread use by the sap flow community, and the adoption of standardized calibration practices will increase the quality and intercomparability of future sap flow datasets. These new datasets will hopefully expand the temporal, geographical, and ecological representativity of SAPFLUXNET when new data contribution periods can be opened in the future.
In this paper we present SAPFLUXNET version 0.1.5 (Poyatos et al., 2020a), which contains some small metadata improvements on version 0.1.4, the first one to be made publicly available, in March 2020. Both versions supersede version 0.1.3, which was initially released to data contributors in March 2019. The entire database can be downloaded from its hosting web page in the Zenodo repository (https://doi.org/10.5281/zenodo.3971689, Poyatos et al. 2020a). In this repository, we provide the database as separate .csv files and as .RData objects; see Sect. 2.4. for details on data structure. Together with the initial publication of SAPFLUXNET in March 2019, we also released the sapfluxnetr R package, available on CRAN, to enable easy access, selection, temporal aggregation, and visualization of SAPFLUXNET data. Feedback on data quality issues can be forwarded to the SAPFLUXNET initiative email address: sapfluxnet@creaf.uab.cat. All the information about SAPFLUXNET, including the publication of new calls for data contribution, can be found on the project website: http://sapfluxnet.creaf.cat/ (last access: 8 June 2021).
The code to reproduce the figures in this paper is available in the following Zenodo repository: https://doi.org/10.5281/zenodo.4727825 (Poyatos et al., 2021).
The SAPFLUXNET database provides the first global perspective of water use by individual plants at multiple timescales, with important applications in multiple fields, ranging from plant ecophysiology to Earth system science. This database has been built from community-contributed datasets and is complemented with a software package to facilitate data access. Both the database and the software have been implemented following open science practices, ensuring public access and reproducibility. Data sharing has been a key component of the success of the FLUXNET network of ecosystem fluxes (Bond-Lamberty, 2018), and many databases in plant and ecosystem ecology now offer open data (Bond-Lamberty and Thomson, 2010; Falster et al., 2015; Gallagher et al., 2020; Kattge et al., 2020). SAPFLUXNET fully aligns with this philosophy. We expect that this initial data infrastructure will promote data sharing amongst the sap flow community in the future (Dai et al., 2018) and will allow the continued growth of the SAPFLUXNET database.
Table A1SAPFLUXNET dataset codes and DOIs (digital object identifiers) of the publications associated with each dataset. Where no DOI was available, the bibliographic reference is shown. Some datasets may have no associated publication (“unpublished”).

Here we will show examples of uncertainty estimation for sap flow data in the SAPFLUXNET database. We will address three main sources of uncertainty which affect plant-level estimates of sap flow: (i) methodological uncertainty, (ii) sapwood area uncertainty, and (iii) radial integration uncertainty.
Methodological uncertainty was estimated using the data in the global meta-analysis of sap flow calibrations by Flo et al. (2019) as published in Flo et al. (2021). This estimation can be applied for the main sap flow density methods. We predicted the standard error (SE) of sub-daily sap flow density by fitting, for each method, linear mixed models of reference flow (i.e. using a gravimetric method or others employed as reference standards in calibration studies) as a function of measured flow, including the individual calibration as a random intercept factor (Table B1, Fig. B1). This model shows that HPTM presents the highest uncertainty and that this method and CHP are the ones showing larger uncertainties at low flows, while HD and CHD show lower relative uncertainty at high sap flow density (Figs. B1, B2). We also show in Fig. B3a the effect of applying the bias correction factor for uncalibrated heat dissipation probes obtained from the meta-analysis by Flo et al. (2019).
Uncertainty in the determination of sapwood area can arise when allometric relationships are used to estimate sapwood area, because this area is then applied to upscale sap flow density values to the whole plant. This uncertainty can be accounted for if the original data employed to obtain the allometry are available. Using these data for one of the datasets in SAPFLUXNET (ESP_VAL_SOR), we first predicted sapwood area, together with the upper and lower bounds of its 68 % predictive interval (equivalent to 1 SE). Then, we estimated the corresponding mean sap flow and its 68 % uncertainty interval (Fig. B3a). In this case, methodological uncertainty was larger than that caused by sapwood area estimation (Fig. B3b). Total combined uncertainty (i.e. methodological and sapwood) was obtained by adding their squared values and then taking the square root, following error propagation theory (Fig. B3c).
In this example tree, total uncertainty for instantaneous values is around 400–500 cm3 h−1, resulting in a high uncertainty for low flows but low relative uncertainty for higher flows, reaching 13 % at peak flows on 6 June (Fig. B3c). When expressed as daily means, this uncertainty will be reduced as temporal averaging decreases the uncertainty by a factor equal to the inverse of the root square of the number of observations within a day (Richardson et al., 2012). In the same example (Fig. B3c), a day with high daily mean flow will also show lower relative uncertainty (6 June, 1589±45 cm3 h−1, 3 %) compared to one with lower daily mean flow (30 May, 237±45 cm3 h−1, 19 %).
Finally, when no information on the variation of sap flow along the sapwood is available, radial integration of point measurements of sap flow density and associated uncertainty can be obtained by applying generic radial profiles according to wood porosity (Berdanier et al., 2016) as implemented in the R package “sapflux” (https://github.com/berdaniera/sapflux, last access: 8 June 2021). An example application of this procedure shows how different uncertainty bounds can be obtained depending on wood anatomy (Fig. B4). In addition, this application shows how assuming a uniform radial profile for ring-porous or diffuse-porous species can lead to substantial underestimation of whole-plant sap flow, compared to a lower impact for tracheid-bearing species.
Table B1Fixed-effect coefficients from the linear mixed models fitting reference sap flow density as a function of measured sap flow density using the data from the global sap flow calibration meta-analysis by Flo et al. (2019). Models for each method included the individual calibration as a random intercept. Sap flow methods are ranked according to their presence in the SAPFLUXNET database, from most to least present: HD (heat dissipation), CHP (compensation heat pulse), HR (heat ratio), HPTM (heat pulse T-max), CHD (cyclic heat dissipation), and HFD (heat field deformation).
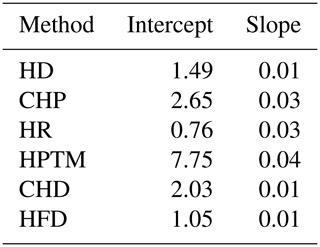
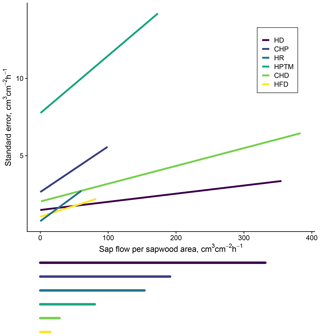
Figure B1Methodological uncertainty estimation in sap flow density measurements based on the data from the global meta-analysis of sap flow calibrations in Flo et al. (2019). The main panel shows predicted standard error based on method-specific linear mixed models of reference flow as a function of measured flow including the individual calibration as a random intercept factor. The span of the horizontal lines below the main panel corresponds to the maximum sap flow density in SAPFLUXNET (estimated as the 99 % quantile of sub-daily measurements) for that specific method.
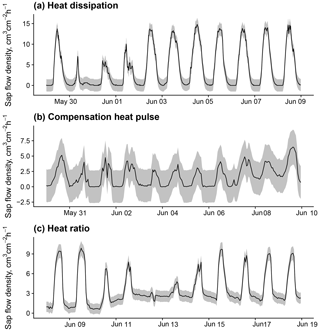
Figure B2Sub-daily time series of sap flow and methodological uncertainty estimations (1 standard error) according to the model in Fig. B1 for 10 d periods in trees measured with (a) heat dissipation, (b) compensation heat pulse, and (c) heat ratio sensors. Data for panel (a) from a Pinus sylvestris tree in dataset ESP_VAL_SOR; data for panel (b) from a Pinus sylvestris tree in GBR_DEV_CON; and data for panel (c) from a Eucalyptus victrix tree in AUS_KAR.
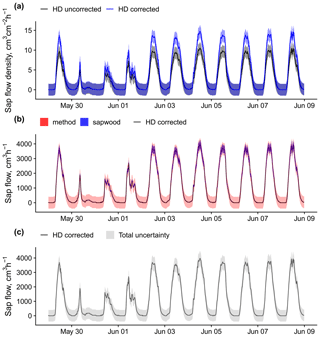
Figure B3An example of sap flow uncertainty estimation and bias correction for a Pinus sylvestris tree (ESP_VAL_SOR_Js_Ps_12) measured using heat dissipation sensors. Panel (a) shows sap flow density HD measurements with and without the application of the bias correction reported in Flo et al. (2019), together with the corresponding uncertainty estimated from the model in Fig. B1. Panel (b) shows corrected sap flow data comparing methodological uncertainty with that derived from the 68 % predictive interval of sapwood area estimation. Panel (c) shows corrected sap flow data together with the combined methodological and sapwood uncertainty.
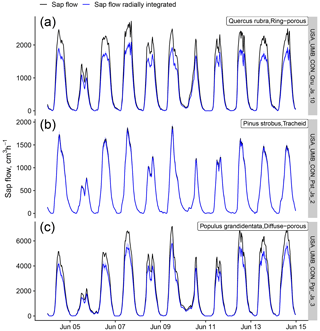
Figure B4Effects of a generic radial integration on sap flow data originally supplied without any radial integration procedure. Radial integration and uncertainty estimation (blue bands show the 68 % prediction interval based on 100 bootstrap samples) were applied using the wood-type-specific radial profiles provided in Berdanier et al. (2016) for a ring-porous species (a), a tracheid-bearing species (b), and a diffuse-porous species (c), all belonging to the USA_UMB_CON dataset.
The supplement related to this article is available online at: https://doi.org/10.5194/essd-13-2607-2021-supplement.
VG, RP, VF, and JMV designed and built the database. RP, VG, and VF summarized the database and drafted the manuscript, with the contribution of JMV, MM, and KS. The rest of the co-authors contributed data to the database and edited the manuscript.
The authors declare that they have no conflict of interest.
This data compilation would have not been possible without the contribution of all the people who supported the construction and maintenance of measurement infrastructures, helped with field data collection, and participated in data processing of individual datasets. We would also like to acknowledge the support of Agustí Escobar, Roberto Molowny-Horas, Marie Sirot, and Guillem Bagaria in building the data infrastructure. We would also like to thank Stan Schymanski and Rob Skelton for their useful feedback during the review process. This paper is dedicated to the memory of our colleague and co-author Niles J. Hasselquist.
This research was supported by the Ministerio de Economía y Competitividad (grant no. CGL2014-55883-JIN), the Ministerio de Ciencia e Innovación (grant no. RTI2018-095297-J-I00), the Ministerio de Ciencia e Innovación (grant no. CAS16/00207), the Agència de Gestió d'Ajuts Universitaris i de Recerca (grant no. SGR1001), the Alexander von Humboldt-Stiftung (Humboldt Research Fellowship for Experienced Researchers (RP)), and the Institució Catalana de Recerca i Estudis Avançats (Academia Award (JMV)). Víctor Flo was supported by the doctoral fellowship FPU15/03939 (MECD, Spain).
This paper was edited by Sibylle K. Hassler and reviewed by Robert Skelton and Stan Schymanski.
Allen, S. T., Kirchner, J. W., Braun, S., Siegwolf, R. T. W., and Goldsmith, G. R.: Seasonal origins of soil water used by trees, Hydrol. Earth Syst. Sci., 23, 1199–1210, https://doi.org/10.5194/hess-23-1199-2019, 2019.
Ambrose, A. R., Sillett, S. C., Koch, G. W., Van Pelt, R., Antoine, M. E., and Dawson, T. E.: Effects of height on treetop transpiration and stomatal conductance in coast redwood (Sequoia sempervirens), Tree Physiol., 30, 1260–1272, https://doi.org/10.1093/treephys/tpq064, 2010.
Anderegg, W. R. L., Konings, A. G., Trugman, A. T., Yu, K., Bowling, D. R., Gabbitas, R., Karp, D. S., Pacala, S., Sperry, J. S., Sulman, B. N., and Zenes, N.: Hydraulic diversity of forests regulates ecosystem resilience during drought, Nature, 561, 538–541, https://doi.org/10.1038/s41586-018-0539-7, 2018.
Asbjornsen, H., Goldsmith, G. R., Alvarado-Barrientos, M. S., Rebel, K., Osch, F. P. V., Rietkerk, M., Chen, J., Gotsch, S., Tobón, C., Geissert, D. R., Gómez-Tagle, A., Vache, K., and Dawson, T. E.: Ecohydrological advances and applications in plant-water relations research: a review, J. Plant Ecol., 4, 3–22, https://doi.org/10.1093/jpe/rtr005, 2011.
Baker, J. M. and Van Bavel, C. H. M.: Measurement of mass flow of water in the stems of herbaceous plants, Plant Cell Environ., 10, 777–782, https://doi.org/10.1111/1365-3040.ep11604765, 1987.
Barbeta, A. and Peñuelas, J.: Relative contribution of groundwater to plant transpiration estimated with stable isotopes, Sci. Rep.-UK, 7, 10580, https://doi.org/10.1038/s41598-017-09643-x, 2017.
Beer, C., Reichstein, M., Tomelleri, E., Ciais, P., Jung, M., Carvalhais, N., Rodenbeck, C., Arain, M. A., Baldocchi, D., Bonan, G. B., Bondeau, A., Cescatti, A., Lasslop, G., Lindroth, A., Lomas, M., Luyssaert, S., Margolis, H., Oleson, K. W., Roupsard, O., Veenendaal, E., Viovy, N., Williams, C., Woodward, F. I., and Papale, D.: Terrestrial Gross Carbon Dioxide Uptake: Global Distribution and Covariation with Climate, Science, 329, 834–838, https://doi.org/10.1126/science.1184984, 2010.
Benyon, R. G., Lane, P. N. J., Jaskierniak, D., Kuczera, G., and Haydon, S. R.: Use of a forest sapwood area index to explain long-term variability in mean annual evapotranspiration and streamflow in moist eucalypt forests, Water Resour. Res., 51, 5318–5331, https://doi.org/10.1002/2015WR017321, 2015.
Berdanier, A. B., Miniat, C. F., and Clark, J. S.: Predictive models for radial sap flux variation in coniferous, diffuse-porous and ring-porous temperate trees, Tree Physiol., 36, 932–941, https://doi.org/10.1093/treephys/tpw027, 2016.
Berry, Z. C., Looker, N., Holwerda, F., Aguilar, G., Rodrigo, L., Ortiz Colin, P., González Martínez, T., and Asbjornsen, H.: Why size matters: the interactive influences of tree diameter distribution and sap flow parameters on upscaled transpiration, Tree Physiol., 38, 263–275, https://doi.org/10.1093/treephys/tpx124, 2018.
Bohrer, G., Mourad, H., Laursen, T. A., Drewry, D., Avissar, R., Poggi, D., Oren, R., and Katul, G. G.: Finite element tree crown hydrodynamics model (FETCH) using porous media flow within branching elements: A new representation of tree hydrodynamics, Water Resour. Res., 41, W11404, https://doi.org/10.1029/2005WR004181, 2005.
Bond-Lamberty, B.: Data Sharing and Scientific Impact in Eddy Covariance Research, J. Geophys. Res.-Biogeo., 123, 1440–1443, https://doi.org/10.1002/2018JG004502, 2018.
Bond-Lamberty, B. and Thomson, A.: A global database of soil respiration data, Biogeosciences, 7, 1915–1926, https://doi.org/10.5194/bg-7-1915-2010, 2010.
Brinkmann, N., Eugster, W., Zweifel, R., Buchmann, N., and Kahmen, A.: Temperate tree species show identical response in tree water deficit but different sensitivities in sap flow to summer soil drying, Tree Physiol., 12, 1508–1519, https://doi.org/10.1093/treephys/tpw062, 2016.
Buckley, T. N., Turnbull, T. L., and Adams, M. A.: Simple models for stomatal conductance derived from a process model: cross-validation against sap flux data, Plant Cell Environ., 35, 1647–1662, https://doi.org/10.1111/j.1365-3040.2012.02515.x, 2012.
Burgess, S. S. O., Adams, M. A., Turner, N. C., Beverly, C. R., Ong, C. K., Khan, A. A. H., and Bleby, T. M.: An improved heat pulse method to measure low and reverse rates of sap flow in woody plants, Tree Physiol., 21, 589–598, https://doi.org/10.1093/treephys/21.9.589, 2001.
Čermák, J., Deml, M., and Penka, M.: A new method of sapflow rate determination in trees, Biol. Plantarum, 15, 171–178, 1973.
Čermák, J., Kučera, J., and Nadezhdina, N.: Sap flow measurements with some thermodynamic methods, flow integration within trees and scaling up from sample trees to entire forest stands, Trees, 18, 529–546, https://doi.org/10.1007/s00468-004-0339-6, 2004.
Cerveny, R. S., Lawrimore, J., Edwards, R., and Landsea, C.: Extreme Weather Records, B. Am. Meteorol. Soc., 88, 853–860, https://doi.org/10.1175/BAMS-88-6-853, 2007.
Chen, Y.-J., Cao, K.-F., Schnitzer, S. A., Fan, Z.-X., Zhang, J.-L., and Bongers, F.: Water-use advantage for lianas over trees in tropical seasonal forests, New Phytol., 205, 128–136, https://doi.org/10.1111/nph.13036, 2015.
Choat, B., Brodribb, T. J., Brodersen, C. R., Duursma, R. A., López, R., and Medlyn, B. E.: Triggers of tree mortality under drought, Nature, 558, 531–539, https://doi.org/10.1038/s41586-018-0240-x, 2018.
Clearwater, M. J., Luo, Z., Mazzeo, M., and Dichio, B.: An external heat pulse method for measurement of sap flow through fruit pedicels, leaf petioles and other small-diameter stems, Plant Cell Environ., 32, 1652–1663, https://doi.org/10.1111/j.1365-3040.2009.02026.x, 2009.
Cochard, H., Bréda, N., and Granier, A.: Whole tree hydraulic conductance and water loss regulation in Quercus during drought: evidence for stomatal control of embolism?, Ann. Sci. Forest., 53, 197–206, 1996.
Cohen, Y., Fuchs, M., and Green, G. C.: Improvement of the heat pulse method for determining sap flow in trees, Plant Cell Environ., 4, 391–397, https://doi.org/10.1111/j.1365-3040.1981.tb02117.x, 1981.
Cohen, Y., Cohen, S., Cantuarias-Aviles, T., and Schiller, G.: Variations in the radial gradient of sap velocity in trunks of forest and fruit trees, Plant Soil, 305, 49–59, https://doi.org/10.1007/s11104-007-9351-0, 2008.
Crowther, T. W., Glick, H. B., Covey, K. R., Bettigole, C., Maynard, D. S., Thomas, S. M., Smith, J. R., Hintler, G., Duguid, M. C., Amatulli, G., Tuanmu, M.-N., Jetz, W., Salas, C., Stam, C., Piotto, D., Tavani, R., Green, S., Bruce, G., Williams, S. J., Wiser, S. K., Huber, M. O., Hengeveld, G. M., Nabuurs, G.-J., Tikhonova, E., Borchardt, P., Li, C.-F., Powrie, L. W., Fischer, M., Hemp, A., Homeier, J., Cho, P., Vibrans, A. C., Umunay, P. M., Piao, S. L., Rowe, C. W., Ashton, M. S., Crane, P. R., and Bradford, M. A.: Mapping tree density at a global scale, Nature, 525, 201–205, https://doi.org/10.1038/nature14967, 2015.
da Costa, A. C. L., Rowland, L., Oliveira, R. S., Oliveira, A. A. R., Binks, O. J., Salmon, Y., Vasconcelos, S. S., Junior, J. A. S., Ferreira, L. V., Poyatos, R., Mencuccini, M., and Meir, P.: Stand dynamics modulate water cycling and mortality risk in droughted tropical forest, Global Change Biol., 24, 249–258, https://doi.org/10.1111/gcb.13851, 2018.
Dai, S.-Q., Li, H., Xiong, J., Ma, J., Guo, H.-Q., Xiao, X., and Zhao, B.: Assessing the Extent and Impact of Online Data Sharing in Eddy Covariance Flux Research, J. Geophys. Res.-Biogeo., 123, 129–137, https://doi.org/10.1002/2017JG004277, 2018.
Davis, T. W., Kuo, C.-M., Liang, X., and Yu, P.-S.: Sap Flow Sensors: Construction, Quality Control and Comparison, Sensors, 12, 954–971, https://doi.org/10.3390/s120100954, 2012.
De Cáceres, M., Mencuccini, M., Martin-StPaul, N., Limousin, J.-M., Coll, L., Poyatos, R., Cabon, A., Granda, V., Forner, A., Valladares, F., and Martínez-Vilalta, J.: Unravelling the effect of species mixing on water use and drought stress in Mediterranean forests: A modelling approach, Agr. Forest Meteorol., 296, 108233, https://doi.org/10.1016/j.agrformet.2020.108233, 2021.
de Dios, V. R., Roy, J., Ferrio, J. P., Alday, J. G., Landais, D., Milcu, A., and Gessler, A.: Processes driving nocturnal transpiration and implications for estimating land evapotranspiration, Sci. Rep.-UK, 5, 10975, https://doi.org/10.1038/srep10975, 2015.
Dierick, D. and Hölscher, D.: Species-specific tree water use characteristics in reforestation stands in the Philippines, Agr. Forest Meteorol., 149, 1317–1326, https://doi.org/10.1016/j.agrformet.2009.03.003, 2009.
Do, F. and Rocheteau, A.: Influence of natural temperature gradients on measurements of xylem sap flow with thermal dissipation probes, 2. Advantages and calibration of a noncontinuous heating system, Tree Physiol., 22, 649–654, https://doi.org/10.1093/treephys/22.9.649, 2002.
Edwards, W. R. N., Becker, P., and Čermák, J.: A unified nomenclature for sap flow measurements, Tree Physiol., 17, 65–67, 1996.
Evaristo, J. and McDonnell, J. J.: Prevalence and magnitude of groundwater use by vegetation: a global stable isotope meta-analysis, Sci. Rep.-UK, 7, 44110, https://doi.org/10.1038/srep44110, 2017.
Ewers, B. E., Oren, R., Albaugh, T. J., and Dougherty, P. M.: Carry-over effects of water and nutrient supply on water use of Pinus taeda, Ecol. Appl., 9, 513–525, https://doi.org/10.1890/1051-0761(1999)009[0513:COEOWA]2.0.CO;2, 1999.
Falster, D. S., Duursma, R. A., Ishihara, M. I., Barneche, D. R., FitzJohn, R. G., Vårhammar, A., Aiba, M., Ando, M., Anten, N., Aspinwall, M. J., Baltzer, J. L., Baraloto, C., Battaglia, M., Battles, J. J., Bond-Lamberty, B., van Breugel, M., Camac, J., Claveau, Y., Coll, L., Dannoura, M., Delagrange, S., Domec, J.-C., Fatemi, F., Feng, W., Gargaglione, V., Goto, Y., Hagihara, A., Hall, J. S., Hamilton, S., Harja, D., Hiura, T., Holdaway, R., Hutley, L. S., Ichie, T., Jokela, E. J., Kantola, A., Kelly, J. W. G., Kenzo, T., King, D., Kloeppel, B. D., Kohyama, T., Komiyama, A., Laclau, J.-P., Lusk, C. H., Maguire, D. A., le Maire, G., Mäkelä, A., Markesteijn, L., Marshall, J., McCulloh, K., Miyata, I., Mokany, K., Mori, S., Myster, R. W., Nagano, M., Naidu, S. L., Nouvellon, Y., O'Grady, A. P., O'Hara, K. L., Ohtsuka, T., Osada, N., Osunkoya, O. O., Peri, P. L., Petritan, A. M., Poorter, L., Portsmuth, A., Potvin, C., Ransijn, J., Reid, D., Ribeiro, S. C., Roberts, S. D., Rodríguez, R., Saldaña-Acosta, A., Santa-Regina, I., Sasa, K., Selaya, N. G., Sillett, S. C., Sterck, F., Takagi, K., Tange, T., Tanouchi, H., Tissue, D., Umehara, T., Utsugi, H., Vadeboncoeur, M. A., Valladares, F., Vanninen, P., Wang, J. R., Wenk, E., Williams, R., de Ximenes, F. A., Yamaba, A., Yamada, T., Yamakura, T., Yanai, R. D., and York, R. A.: BAAD: a Biomass And Allometry Database for woody plants, Ecology, 96, 1445–1446, 2015.
Fatichi, S., Pappas, C., and Ivanov, V. Y.: Modeling plant-water interactions: an ecohydrological overview from the cell to the global scale, WIREs Water, 3, 327–368, https://doi.org/10.1002/wat2.1125, 2016.
Fisher, R. A., Koven, C. D., Anderegg, W. R. L., Christoffersen, B. O., Dietze, M. C., Farrior, C. E., Holm, J. A., Hurtt, G. C., Knox, R. G., Lawrence, P. J., Lichstein, J. W., Longo, M., Matheny, A. M., Medvigy, D., Muller-Landau, H. C., Powell, T. L., Serbin, S. P., Sato, H., Shuman, J. K., Smith, B., Trugman, A. T., Viskari, T., Verbeeck, H., Weng, E., Xu, C., Xu, X., Zhang, T., and Moorcroft, P. R.: Vegetation demographics in Earth System Models: A review of progress and priorities, Global Change Biol., 24, 35–54, https://doi.org/10.1111/gcb.13910, 2018.
Flo, V., Martinez-Vilalta, J., Steppe, K., Schuldt, B., and Poyatos, R.: A synthesis of bias and uncertainty in sap flow methods, Agr. Forest Meteorol., 271, 362–374, https://doi.org/10.1016/j.agrformet.2019.03.012, 2019.
Flo, V., Martínez-Vilalta, J., Steppe, K., Schuldt, B., and Poyatos, R.: Sap flow methods calibrations [data set], Zenodo, https://doi.org/10.5281/zenodo.4559497, 2021.
Ford, C. R., Hubbard, R. M., Kloeppel, B. D., and Vose, J. M.: A comparison of sap flux-based evapotranspiration estimates with catchment-scale water balance, Agr. Forest Meteorol., 145, 176–185, 2007.
Forster, M. A.: How significant is nocturnal sap flow?, Tree Physiol., 34, 757–765, https://doi.org/10.1093/treephys/tpu051, 2014.
Gallagher, R. V., Falster, D. S., Maitner, B. S., Salguero-Gómez, R., Vandvik, V., Pearse, W. D., Schneider, F. D., Kattge, J., Poelen, J. H., Madin, J. S., Ankenbrand, M. J., Penone, C., Feng, X., Adams, V. M., Alroy, J., Andrew, S. C., Balk, M. A., Bland, L. M., Boyle, B. L., Bravo-Avila, C. H., Brennan, I., Carthey, A. J. R., Catullo, R., Cavazos, B. R., Conde, D. A., Chown, S. L., Fadrique, B., Gibb, H., Halbritter, A. H., Hammock, J., Hogan, J. A., Holewa, H., Hope, M., Iversen, C. M., Jochum, M., Kearney, M., Keller, A., Mabee, P., Manning, P., McCormack, L., Michaletz, S. T., Park, D. S., Perez, T. M., Pineda-Munoz, S., Ray, C. A., Rossetto, M., Sauquet, H., Sparrow, B., Spasojevic, M. J., Telford, R. J., Tobias, J. A., Violle, C., Walls, R., Weiss, K. C. B., Westoby, M., Wright, I. J., and Enquist, B. J.: Open Science principles for accelerating trait-based science across the Tree of Life, Nature Ecology & Evolution, 4, 294–303, https://doi.org/10.1038/s41559-020-1109-6, 2020.
Good, S. P., Moore, G. W., and Miralles, D. G.: A mesic maximum in biological water use demarcates biome sensitivity to aridity shifts, Nature Ecology & Evolution, 1, 1883–1888, https://doi.org/10.1038/s41559-017-0371-8, 2017.
Granda, V., Poyatos, R., Flo, V., Sirot, M., and Bagaria, G.: sapfluxnetQC1: R package with functions related to sapfluxnet project, SAPFLUXNET, available at: https://github.com/sapfluxnet/sapfluxnetQC1 (last access: 21 September 2017), 2016.
Granda, V., Poyatos, R., Flo, V., Nelson, J., and Team, S. C.: sapfluxnetr: Working with “Sapfluxnet” Project Data, available at: https://CRAN.R-project.org/package=sapfluxnetr, last access: 14 May 2019.
Granier, A.: Une nouvelle méthode pur la mesure du flux de sève brute dans le tronc des arbres, Ann. Sci. Forest., 42, 193–200, 1985.
Granier, A.: Evaluation of transpiration in a Douglas-fir stand by means of sap flow measurements, Tree Physiol., 3, 309–320, 1987.
Granier, A., Biron, P., Bréda, N., Pontailler, J. Y., and Saugier, B.: Transpiration of trees and forest stands:short and long-term monitoring using sapflow methods, Global Change Biol., 2, 265–274, 1996.
Grossiord, C.: Having the right neighbors: how tree species diversity modulates drought impacts on forests, New Phytol., 228, 42–49, https://doi.org/10.1111/nph.15667, 2020.
Grossiord, C., Sevanto, S., Limousin, J.-M., Meir, P., Mencuccini, M., Pangle, R. E., Pockman, W. T., Salmon, Y., Zweifel, R., and McDowell, N. G.: Manipulative experiments demonstrate how long-term soil moisture changes alter controls of plant water use, Environ. Exp. Bot., 152, 19–27, https://doi.org/10.1016/j.envexpbot.2017.12.010, 2018.
Grossiord, C., Christoffersen, B., Alonso-Rodríguez, A. M., Anderson-Teixeira, K., Asbjornsen, H., Aparecido, L. M. T., Carter Berry, Z., Baraloto, C., Bonal, D., Borrego, I., Burban, B., Chambers, J. Q., Christianson, D. S., Detto, M., Faybishenko, B., Fontes, C. G., Fortunel, C., Gimenez, B. O., Jardine, K. J., Kueppers, L., Miller, G. R., Moore, G. W., Negron-Juarez, R., Stahl, C., Swenson, N. G., Trotsiuk, V., Varadharajan, C., Warren, J. M., Wolfe, B. T., Wei, L., Wood, T. E., Xu, C., and McDowell, N. G.: Precipitation mediates sap flux sensitivity to evaporative demand in the neotropics, Oecologia, 191, 519–530, https://doi.org/10.1007/s00442-019-04513-x, 2019.
Hampel, F. R.: The Influence Curve and its Role in Robust Estimation, J. Am. Stat. Assoc., 69, 383–393, https://doi.org/10.1080/01621459.1974.10482962, 1974.
Hassler, S. K., Weiler, M., and Blume, T.: Tree-, stand- and site-specific controls on landscape-scale patterns of transpiration, Hydrol. Earth Syst. Sci., 22, 13–30, https://doi.org/10.5194/hess-22-13-2018, 2018.
Helfter, C., Shephard, J. D., Martínez-Vilalta, J., Mencuccini, M., and Hand, D. P.: A noninvasive optical system for the measurement of xylem and phloem sap flow in woody plants of small stem size, Tree Physiol., 27, 169–179, https://doi.org/10.1093/treephys/27.2.169, 2007.
Hu, J., Moore, D. J. P., Riveros-Iregui, D. A., Burns, S. P., and Monson, R. K.: Modeling whole-tree carbon assimilation rate using observed transpiration rates and needle sugar carbon isotope ratios, New Phytol., 185, 1000–1015, https://doi.org/10.1111/j.1469-8137.2009.03154.x, 2010.
Huber, B.: Beobachtung und Messung pflanzlicher Sartströme, Ber. Deut. Bot. Ges., 50, 89–109, https://doi.org/10.1111/j.1438-8677.1932.tb00039.x, 1932.
Hultine, K. R., Nagler, P. L., Morino, K., Bush, S. E., Burtch, K. G., Dennison, P. E., Glenn, E. P., and Ehleringer, J. R.: Sap flux-scaled transpiration by tamarisk (Tamarix spp.) before, during and after episodic defoliation by the saltcedar leaf beetle (Diorhabda carinulata), Agr. Forest Meteorol., 150, 1467–1475, https://doi.org/10.1016/j.agrformet.2010.07.009, 2010.
Jarvis, P. G.: Scaling processes and problems, Plant Cell Environ., 18, 1079–1089, https://doi.org/10.1111/j.1365-3040.1995.tb00620.x, 1995.
Jung, M., Koirala, S., Weber, U., Ichii, K., Gans, F., Camps-Valls, G., Papale, D., Schwalm, C., Tramontana, G., and Reichstein, M.: The FLUXCOM ensemble of global land-atmosphere energy fluxes, Sci. Data, 6, 74, https://doi.org/10.1038/s41597-019-0076-8, 2019.
Kallarackal, J., Otieno, D. O., Reineking, B., Jung, E.-Y., Schmidt, M. W. T., Granier, A., and Tenhunen, J. D.: Functional convergence in water use of trees from different geographical regions: a meta-analysis, Trees, 27, 787–799, https://doi.org/10.1007/s00468-012-0834-0, 2013.
Kattge, J., Bönisch, G., Díaz, S., Lavorel, S., Prentice, I. C., Leadley, P., Tautenhahn, S., Werner, G. D. A., Aakala, T., Abedi, M., Acosta, A. T. R., Adamidis, G. C., Adamson, K., Aiba, M., Albert, C. H., Alcántara, J. M., Álcazar, C. C., Aleixo, I., Ali, H., Amiaud, B., Ammer, C., Amoroso, M. M., Anand, M., Anderson, C., Anten, N., Antos, J., Apgaua, D. M. G., Ashman, T.-L., Asmara, D. H., Asner, G. P., Aspinwall, M., Atkin, O., Aubin, I., Baastrup‐Spohr, L., Bahalkeh, K., Bahn, M., Baker, T., Baker, W. J., Bakker, J. P., Baldocchi, D., Baltzer, J., Banerjee, A., Baranger, A., Barlow, J., Barneche, D. R., Baruch, Z., Bastianelli, D., Battles, J., Bauerle, W., Bauters, M., Bazzato, E., Beckmann, M., Beeckman, H., Beierkuhnlein, C., Bekker, R., Belfry, G., Belluau, M., Beloiu, M., Benavides, R., Benomar, L., Berdugo‐Lattke, M. L., Berenguer, E., Bergamin, R., Bergmann, J., Carlucci, M. B., Berner, L., Bernhardt‐Römermann, M., Bigler, C., Bjorkman, A. D., Blackman, C., Blanco, C., Blonder, B., Blumenthal, D., Bocanegra‐González, K. T., Boeckx, P., Bohlman, S., Böhning‐Gaese, K., Boisvert‐Marsh, L., Bond, W., Bond‐Lamberty, B., Boom, A., Boonman, C. C. F., Bordin, K., Boughton, E. H., Boukili, V., Bowman, D. M. J. S., Bravo, S., Brendel, M. R., Broadley, M. R., Brown, K. A., Bruelheide, H., Brumnich, F., Bruun, H. H., Bruy, D., Buchanan, S. W., Bucher, S. F., Buchmann, N., Buitenwerf, R., Bunker, D. E., Bürger, J., Burrascano, S., Burslem, D. F. R. P., Butterfield, B. J., Byun, C., Marques, M., Scalon, M. C., Caccianiga, M., Cadotte, M., Cailleret, M., Camac, J., Camarero, J. J., Campany, C., Campetella, G., Campos, J. A., Cano‐Arboleda, L., Canullo, R., Carbognani, M., Carvalho, F., Casanoves, F., Castagneyrol, B., Catford, J. A., Cavender‐Bares, J., Cerabolini, B. E. L., Cervellini, M., Chacón‐Madrigal, E., Chapin, K., Chapin, F. S., Chelli, S., Chen, S.-C., Chen, A., Cherubini, P., Chianucci, F., Choat, B., Chung, K.-S., Chytrý, M., Ciccarelli, D., Coll, L., Collins, C. G., Conti, L., Coomes, D., Cornelissen, J. H. C., Cornwell, W. K., Corona, P., Coyea, M., Craine, J., Craven, D., Cromsigt, J. P. G. M., Csecserits, A., Cufar, K., Cuntz, M., da Silva, A. C., Dahlin, K. M., Dainese, M., Dalke, I., Fratte, M. D., Dang‐Le, A. T., Danihelka, J., Dannoura, M., Dawson, S., de Beer, A. J., Frutos, A. D., Long, J. R. D., Dechant, B., Delagrange, S., Delpierre, N., Derroire, G., Dias, A. S., Diaz‐Toribio, M. H., Dimitrakopoulos, P. G., Dobrowolski, M., Doktor, D., Dřevojan, P., Dong, N., Dransfield, J., Dressler, S., Duarte, L., Ducouret, E., Dullinger, S., Durka, W., Duursma, R., Dymova, O., E‐Vojtkó, A., Eckstein, R. L., Ejtehadi, H., Elser, J., Emilio, T., Engemann, K., Erfanian, M. B., Erfmeier, A., Esquivel‐Muelbert, A., Esser, G., Estiarte, M., Domingues, T. F., Fagan, W. F., Fagúndez, J., Falster, D. S., Fan, Y., Fang, J., Farris, E., Fazlioglu, F., Feng, Y., Fernandez‐Mendez, F., Ferrara, C., Ferreira, J., Fidelis, A., Finegan, B., Firn, J., Flowers, T. J., Flynn, D. F. B., Fontana, V., Forey, E., Forgiarini, C., François, L., Frangipani, M., Frank, D., Frenette‐Dussault, C., Freschet, G. T., Fry, E. L., Fyllas, N. M., Mazzochini, G. G., Gachet, S., Gallagher, R., Ganade, G., Ganga, F., García‐Palacios, P., Gargaglione, V., Garnier, E., Garrido, J. L., de Gasper, A. L., Gea‐Izquierdo, G., Gibson, D., Gillison, A. N., Giroldo, A., Glasenhardt, M.-C., Gleason, S., Gliesch, M., Goldberg, E., Göldel, B., Gonzalez‐Akre, E., Gonzalez‐Andujar, J. L., González‐Melo, A., González‐Robles, A., Graae, B. J., Granda, E., Graves, S., Green, W. A., Gregor, T., Gross, N., Guerin, G. R., Günther, A., Gutiérrez, A. G., Haddock, L., Haines, A., Hall, J., Hambuckers, A., Han, W., Harrison, S. P., Hattingh, W., Hawes, J. E., He, T., He, P., Heberling, J. M., Helm, A., Hempel, S., Hentschel, J., Hérault, B., Hereş, A.-M., Herz, K., Heuertz, M., Hickler, T., Hietz, P., Higuchi, P., Hipp, A. L., Hirons, A., Hock, M., Hogan, J. A., Holl, K., Honnay, O., Hornstein, D., Hou, E., Hough‐Snee, N., Hovstad, K. A., Ichie, T., Igić, B., Illa, E., Isaac, M., Ishihara, M., Ivanov, L., Ivanova, L., Iversen, C. M., Izquierdo, J., Jackson, R. B., Jackson, B., Jactel, H., Jagodzinski, A. M., Jandt, U., Jansen, S., Jenkins, T., Jentsch, A., Jespersen, J. R. P., Jiang, G.-F., Johansen, J. L., Johnson, D., Jokela, E. J., Joly, C. A., Jordan, G. J., Joseph, G. S., Junaedi, D., Junker, R. R., Justes, E., Kabzems, R., Kane, J., Kaplan, Z., Kattenborn, T., Kavelenova, L., Kearsley, E., Kempel, A., Kenzo, T., Kerkhoff, A., Khalil, M. I., Kinlock, N. L., Kissling, W. D., Kitajima, K., Kitzberger, T., Kjøller, R., Klein, T., Kleyer, M., Klimešová, J., Klipel, J., Kloeppel, B., Klotz, S., Knops, J. M. H., Kohyama, T., Koike, F., Kollmann, J., Komac, B., Komatsu, K., König, C., Kraft, N. J. B., Kramer, K., Kreft, H., Kühn, I., Kumarathunge, D., Kuppler, J., Kurokawa, H., Kurosawa, Y., Kuyah, S., Laclau, J.-P., Lafleur, B., Lallai, E., Lamb, E., Lamprecht, A., Larkin, D. J., Laughlin, D., Bagousse‐Pinguet, Y. L., le Maire, G., le Roux, P. C., le Roux, E., Lee, T., Lens, F., Lewis, S. L., Lhotsky, B., Li, Y., Li, X., Lichstein, J. W., Liebergesell, M., Lim, J. Y., Lin, Y.-S., Linares, J. C., Liu, C., Liu, D., Liu, U., Livingstone, S., Llusià, J., Lohbeck, M., López‐García, Á., Lopez‐Gonzalez, G., Lososová, Z., Louault, F., Lukács, B. A., Lukeš, P., Luo, Y., Lussu, M., Ma, S., Pereira, C.M.R., Mack, M., Maire, V., Mäkelä, A., Mäkinen, H., Malhado, A. C. M., Mallik, A., Manning, P., Manzoni, S., Marchetti, Z., Marchino, L., Marcilio‐Silva, V., Marcon, E., Marignani, M., Markesteijn, L., Martin, A., Martínez‐Garza, C., Martínez‐Vilalta, J., Mašková, T., Mason, K., Mason, N., Massad, T. J., Masse, J., Mayrose, I., McCarthy, J., McCormack, M. L., McCulloh, K., McFadden, I. R., McGill, B. J., McPartland, M. Y., Medeiros, J. S., Medlyn, B., Meerts, P., Mehrabi, Z., Meir, P., Melo, F. P. L., Mencuccini, M., Meredieu, C., Messier, J., Mészáros, I., Metsaranta, J., Michaletz, S. T., Michelaki, C., Migalina, S., Milla, R., Miller, J. E. D., Minden, V., Ming, R., Mokany, K., Moles, A. T., Molnár, A., Molofsky, J., Molz, M., Montgomery, R. A., Monty, A., Moravcová, L., Moreno‐Martínez, A., Moretti, M., Mori, A. S., Mori, S., Morris, D., Morrison, J., Mucina, L., Mueller, S., Muir, C. D., Müller, S. C., Munoz, F., Myers‐Smith, I. H., Myster, R. W., Nagano, M., Naidu, S., Narayanan, A., Natesan, B., Negoita, L., Nelson, A. S., Neuschulz, E. L., Ni, J., Niedrist, G., Nieto, J., Niinemets, Ü., Nolan, R., Nottebrock, H., Nouvellon, Y., Novakovskiy, A., Nystuen, K. O., O'Grady, A., O'Hara, K., O'Reilly‐Nugent, A., Oakley, S., Oberhuber, W., Ohtsuka, T., Oliveira, R., Öllerer, K., Olson, M. E., Onipchenko, V., Onoda, Y., Onstein, R. E., Ordonez, J. C., Osada, N., Ostonen, I., Ottaviani, G., Otto, S., Overbeck, G. E., Ozinga, W. A., Pahl, A. T., Paine, C. E. T., Pakeman, R. J., Papageorgiou, A. C., Parfionova, E., Pärtel, M., Patacca, M., Paula, S., Paule, J., Pauli, H., Pausas, J. G., Peco, B., Penuelas, J., Perea, A., Peri, P. L., Petisco‐Souza, A. C., Petraglia, A., Petritan, A. M., Phillips, O. L., Pierce, S., Pillar, V. D., Pisek, J., Pomogaybin, A., Poorter, H., Portsmuth, A., Poschlod, P., Potvin, C., Pounds, D., Powell, A. S., Power, S. A., Prinzing, A., Puglielli, G., Pyšek, P., Raevel, V., Rammig, A., Ransijn, J., Ray, C. A., Reich, P. B., Reichstein, M., Reid, D. E. B., Réjou‐Méchain, M., de Dios, V. R., Ribeiro, S., Richardson, S., Riibak, K., Rillig, M. C., Riviera, F., Robert, E. M. R., Roberts, S., Robroek, B., Roddy, A., Rodrigues, A. V., Rogers, A., Rollinson, E., Rolo, V., Römermann, C., Ronzhina, D., Roscher, C., Rosell, J.A., Rosenfield, M. F., Rossi, C., Roy, D. B., Royer‐Tardif, S., Rüger, N., Ruiz‐Peinado, R., Rumpf, S. B., Rusch, G. M., Ryo, M., Sack, L., Saldaña, A., Salgado‐Negret, B., Salguero‐Gomez, R., Santa‐Regina, I., Santacruz‐García, A. C., Santos, J., Sardans, J., Schamp, B., Scherer‐Lorenzen, M., Schleuning, M., Schmid, B., Schmidt, M., Schmitt, S., Schneider, J.V., Schowanek, S. D., Schrader, J., Schrodt, F., Schuldt, B., Schurr, F., Garvizu, G. S., Semchenko, M., Seymour, C., Sfair, J. C., Sharpe, J. M., Sheppard, C. S., Sheremetiev, S., Shiodera, S., Shipley, B., Shovon, T. A., Siebenkäs, A., Sierra, C., Silva, V., Silva, M., Sitzia, T., Sjöman, H., Slot, M., Smith, N. G., Sodhi, D., Soltis, P., Soltis, D., Somers, B., Sonnier, G., Sørensen, M. V., Sosinski, E. E., Soudzilovskaia, N. A., Souza, A. F., Spasojevic, M., Sperandii, M. G., Stan, A. B., Stegen, J., Steinbauer, K., Stephan, J. G., Sterck, F., Stojanovic, D. B., Strydom, T., Suarez, M.L., Svenning, J.-C., Svitková, I., Svitok, M., Svoboda, M., Swaine, E., Swenson, N., Tabarelli, M., Takagi, K., Tappeiner, U., Tarifa, R., Tauugourdeau, S., Tavsanoglu, C., te Beest, M., Tedersoo, L., Thiffault, N., Thom, D., Thomas, E., Thompson, K., Thornton, P. E., Thuiller, W., Tichý, L., Tissue, D., Tjoelker, M. G., Tng, D. Y. P., Tobias, J., Török, P., Tarin, T., Torres‐Ruiz, J. M., Tóthmérész, B., Treurnicht, M., Trivellone, V., Trolliet, F., Trotsiuk, V., Tsakalos, J. L., Tsiripidis, I., Tysklind, N., Umehara, T., Usoltsev, V., Vadeboncoeur, M., Vaezi, J., Valladares, F., Vamosi, J., van Bodegom, P. M., van Breugel, M., Cleemput, E. V., van de Weg, M., van der Merwe, S., van der Plas, F., van der Sande, M. T., van Kleunen, M., Meerbeek, K. V., Vanderwel, M., Vanselow, K. A., Vårhammar, A., Varone, L., Valderrama, M. Y. V., Vassilev, K., Vellend, M., Veneklaas, E. J., Verbeeck, H., Verheyen, K., Vibrans, A., Vieira, I., Villacís, J., Violle, C., Vivek, P., Wagner, K., Waldram, M., Waldron, A., Walker, A. P., Waller, M., Walther, G., Wang, H., Wang, F., Wang, W., Watkins, H., Watkins, J., Weber, U., Weedon, J. T., Wei, L., Weigelt, P., Weiher, E., Wells, A. W., Wellstein, C., Wenk, E., Westoby, M., Westwood, A., White, P. J., Whitten, M., Williams, M., Winkler, D. E., Winter, K., Womack, C., Wright, I. J., Wright, S. J., Wright, J., Pinho, B. X., Ximenes, F., Yamada, T., Yamaji, K., Yanai, R., Yankov, N., Yguel, B., Zanini, K. J., Zanne, A. E., Zelený, D., Zhao, Y.-P., Zheng, Jingming, Zheng, Ji, Ziemińska, K., Zirbel, C. R., Zizka, G., Zo‐Bi, I. C., Zotz, G., Wirth, C.: TRY plant trait database – enhanced coverage and open access, Global Change Biol., 26, 119–188, https://doi.org/10.1111/gcb.14904, 2020.
Kennedy, D., Swenson, S., Oleson, K. W., Lawrence, D. M., Fisher, R., Lola da Costa, A. C., and Gentine, P.: Implementing Plant Hydraulics in the Community Land Model, Version 5, J. Adv. Model. Earth Sy., 11, 485–513, https://doi.org/10.1029/2018MS001500, 2019.
Klein, T., Rotenberg, E., Tatarinov, F., and Yakir, D.: Association between sap flow-derived and eddy covariance-derived measurements of forest canopy CO2 uptake, New Phytol., 209, 436–446, https://doi.org/10.1111/nph.13597, 2016.
Knauer, J., Werner, C., and Zaehle, S.: Evaluating stomatal models and their atmospheric drought response in a land surface scheme: A multibiome analysis, J. Geophys. Res.-Biogeo., 120, 1894–1911, https://doi.org/10.1002/2015JG003114, 2015.
Kool, D., Agam, N., Lazarovitch, N., Heitman, J. L., Sauer, T. J., and Ben-Gal, A.: A review of approaches for evapotranspiration partitioning, Agr. Forest Meteorol., 184, 56–70, https://doi.org/10.1016/j.agrformet.2013.09.003, 2014.
Köstner, B., Granier, A., and Cermák, J.: Sapflow measurements in forest stands: methods and uncertainties, Ann. Sci. Forest., 55, 13–27, https://doi.org/10.1051/forest:19980102, 1998.
Lemeur, R., Fernández, J. E., and Steppe, K.: Symbols, SI units and physical quantities within the scope of sap flow studies, Acta Hortic., 846, 21–32, https://doi.org/10.17660/ActaHortic.2009.846.0, 2009.
Liu, B., Zhao, W., and Jin, B.: The response of sap flow in desert shrubs to environmental variables in an arid region of China, Ecohydrology, 4, 448–457, https://doi.org/10.1002/eco.151, 2011.
Liu, H., Gleason, S. M., Hao, G., Hua, L., He, P., Goldstein, G., and Ye, Q.: Hydraulic traits are coordinated with maximum plant height at the global scale, Science Advances, 5, eaav1332, https://doi.org/10.1126/sciadv.aav1332, 2019.
Looker, N., Martin, J., Jencso, K., and Hu, J.: Contribution of sapwood traits to uncertainty in conifer sap flow as estimated with the heat-ratio method, Agr. Forest Meteorol., 223, 60–71, https://doi.org/10.1016/j.agrformet.2016.03.014, 2016.
Lu, P., Müller, W. J., and Chacko, E. K.: Spatial variations in xylem sap flux density in the trunk of orchard-grown, mature mango trees under changing soil water conditions, Tree Physiol., 20, 683–692, https://doi.org/10.1093/treephys/20.10.683, 2000.
Lu, P., Woo, K. C., and Liu, Z. T.: Estimation of whole-transpiration of bananas using sap flow measurements, J. Exp. Bot., 53, 1771–1779, https://doi.org/10.1093/jxb/erf019, 2002.
Mackay, D. S., Ewers, B. E., Loranty, M. M., and Kruger, E. L.: On the representativeness of plot size and location for scaling transpiration from trees to a stand, J. Geophys. Res.-Biogeo., 115, G02016, https://doi.org/10.1029/2009JG001092, 2010.
Manzoni, S., Vico, G., Katul, G., Palmroth, S., Jackson, R. B., and Porporato, A.: Hydraulic limits on maximum plant transpiration and the emergence of the safety-efficiency trade-off, New Phytol., 198, 169–178, https://doi.org/10.1111/nph.12126, 2013.
Marshall, D. C.: Measurement of sap flow in conifers by heat transport, Plant Physiol., 33, 385–396, 1958.
Martin-StPaul, N., Delzon, S., and Cochard, H.: Plant resistance to drought depends on timely stomatal closure, Ecol. Lett., 20, 1437–1447, https://doi.org/10.1111/ele.12851, 2017.
Matheny, A. M., Bohrer, G., Stoy, P. C., Baker, I. T., Black, A. T., Desai, A. R., Dietze, M. C., Gough, C. M., Ivanov, V. Y., Jassal, R. S., Novick, K. A., Schäfer, K. V. R., and Verbeeck, H.: Characterizing the diurnal patterns of errors in the prediction of evapotranspiration by several land-surface models: An NACP analysis, J. Geophys. Res.-Biogeo., 119, 1458–1473, https://doi.org/10.1002/2014JG002623, 2014.
McCulloh, K. A., Domec, J., Johnson, D. M., Smith, D. D., and Meinzer, F. C.: A dynamic yet vulnerable pipeline: Integration and coordination of hydraulic traits across whole plants, Plant Cell Environ., 42, 2789–2807, https://doi.org/10.1111/pce.13607, 2019.
Meinzer, F. C., Bond, B. J., Warren, J. M., and Woodruff, D. R.: Does water transport scale universally with tree size?, Funct. Ecol., 19, 558–565, https://doi.org/10.1111/j.1365-2435.2005.01017.x, 2005.
Mencuccini, M., Manzoni, S., and Christoffersen, B.: Modelling water fluxes in plants: from tissues to biosphere, New Phytol., 222, 1207–1222, https://doi.org/10.1111/nph.15681, 2019.
Merlin, M., Solarik, K. A., and Landhäusser, S. M.: Quantification of uncertainties introduced by data-processing procedures of sap flow measurements using the cut-tree method on a large mature tree, Agr. Forest Meteorol., 287, 107926, https://doi.org/10.1016/j.agrformet.2020.107926, 2020.
Mészáros, I., Kanalas, P., Fenyvesi, A., Kis, J., Nyitrai, B., Szollosi, E., Oláh, V., Demeter, Z., Lakatos, Á., and Ander, I.: Diurnal and seasonal changes in stem radius increment and sap flow density indicate different responses of two co-existing oak species to drought stress, Acta Silvatica et Lignaria Hungarica, 7, 97–108, 2011.
Mirfenderesgi, G., Bohrer, G., Matheny, A. M., Fatichi, S., de Moraes Frasson, R. P., and Schäfer, K. V. R.: Tree level hydrodynamic approach for resolving aboveground water storage and stomatal conductance and modeling the effects of tree hydraulic strategy, J. Geophys. Res.-Biogeo., 121, 1792–1813, https://doi.org/10.1002/2016JG003467, 2016.
Moffat, A. M., Papale, D., Reichstein, M., Hollinger, D. Y., Richardson, A. D., Barr, A. G., Beckstein, C., Braswell, B. H., Churkina, G., Desai, A. R., Falge, E., Gove, J. H., Heimann, M., Hui, D., Jarvis, A. J., Kattge, J., Noormets, A., and Stauch, V. J.: Comprehensive comparison of gap-filling techniques for eddy covariance net carbon fluxes, Agr. Forest Meteorol., 147, 209–232, 2007.
Morán-López, T., Poyatos, R., Llorens, P., and Sabaté, S.: Effects of past growth trends and current water use strategies on Scots pine and pubescent oak drought sensitivity, Eur. J. Forest Res., 133, 369–382, https://doi.org/10.1007/s10342-013-0768-0, 2014.
Nadezhdina, N.: Revisiting the Heat Field Deformation (HFD) method for measuring sap flow, iForest, 11, 118–130, https://doi.org/10.3832/ifor2381-011, 2018.
Nadezhdina, N., Čermák, J., and Ceulemans, R.: Radial patterns of sap flow in woody stems of dominant and understory species: scaling errors associated with positioning of sensors, Tree Physiol., 22, 907–918, 2002.
Nadezhdina, N., Steppe, K., De Pauw, D. J. W., Bequet, R., Cermák, J., and Ceulemans, R.: Stem-mediated hydraulic redistribution in large roots on opposing sides of a Douglas-fir tree following localized irrigation, New Phytol., 184, 932–943, 2009.
Nelson, J. A., Pérez-Priego, O., Zhou, S., Poyatos, R., Zhang, Y., Blanken, P. D., Gimeno, T. E., Wohlfahrt, G., Desai, A. R., Gioli, B., Limousin, J.-M., Bonal, D., Paul-Limoges, E., Scott, R. L., Varlagin, A., Fuchs, K., Montagnani, L., Wolf, S., Delpierre, N., Berveiller, D., Gharun, M., Marchesini, L. B., Gianelle, D., Šigut, L., Mammarella, I., Siebicke, L., Black, T. A., Knohl, A., Hörtnagl, L., Magliulo, V., Besnard, S., Weber, U., Carvalhais, N., Migliavacca, M., Reichstein, M., and Jung, M.: Ecosystem transpiration and evaporation: Insights from three water flux partitioning methods across FLUXNET sites, Global Change Biol., 26, 6916–6930, https://doi.org/10.1111/gcb.15314, 2020.
Novick, K., Oren, R., Stoy, P., Juang, J.-Y., Siqueira, M., and Katul, G.: The relationship between reference canopy conductance and simplified hydraulic architecture, Adv. Water Resour., 32, 809–819, https://doi.org/10.1016/j.advwatres.2009.02.004, 2009.
Novick, K. A., Ficklin, D. L., Stoy, P. C., Williams, C. A., Bohrer, G., Oishi, A. C., Papuga, S. A., Blanken, P. D., Noormets, A., Sulman, B. N., Scott, R. L., Wang, L., and Phillips, R. P.: The increasing importance of atmospheric demand for ecosystem water and carbon fluxes, Nat. Clim. Change, 6, 1023–1027, https://doi.org/10.1038/nclimate3114, 2016.
O'Brien, J. J., Oberbauer, S. F., and Clark, D. B.: Whole tree xylem sap flow responses to multiple environmental variables in a wet tropical forest, Plant Cell Environ., 27, 551–567, 2004.
Oishi, A. C., Oren, R., Novick, K. A., Palmroth, S., and Katul, G. G.: Interannual Invariability of Forest Evapotranspiration and Its Consequence to Water Flow Downstream, Ecosystems, 13, 421–436, https://doi.org/10.1007/s10021-010-9328-3, 2010.
Oishi, A. C., Hawthorne, D. A., and Oren, R.: Baseliner: An open-source, interactive tool for processing sap flux data from thermal dissipation probes, SoftwareX, 5, 139–143, https://doi.org/10.1016/j.softx.2016.07.003, 2016.
Oki, T. and Kanae, S.: Global hydrological cycles and world water resources, Science, 313, 1068–1072, 2006.
Oren, R., Phillips, N., Ewers, B. E., Pataki, D., and Megonigal, J. P.: Sap-flux-scaled transpiration responses to light, vapor pressure deficit, and leaf area reduction in a flooded Taxodium distichum forest, Tree Physiol., 19, 337–347, 1999a.
Oren, R., Sperry, J. S., Katul, G. G., Pataki, D. E., Ewers, B. E., Phillips, N., and Schäfer, K. V. R.: Survey and synthesis of intra- and interspecific variation in stomatal sensitivity to vapour pressure deficit, Plant Cell Environ., 22, 1515–1526, 1999b.
Peel, M. C., McMahon, T. A., and Finlayson, B. L.: Vegetation impact on mean annual evapotranspiration at a global catchment scale, Water Resour. Res., 46, W09508, https://doi.org/10.1029/2009WR008233, 2010.
Pérez-Priego, O., Testi, L., Orgaz, F., and Villalobos, F. J.: A large closed canopy chamber for measuring CO2 and water vapour exchange of whole trees, Environ. Exp. Bot., 68, 131–138, https://doi.org/10.1016/j.envexpbot.2009.10.009, 2010.
Perpiñán, O.: solaR: Solar Radiation and Photovoltaic Systems with R, J. Stat. Softw., 50, 1–32, 2012.
Peters, R. L., Fonti, P., Frank, D. C., Poyatos, R., Pappas, C., Kahmen, A., Carraro, V., Prendin, A. L., Schneider, L., Baltzer, J. L., Baron-Gafford, G. A., Dietrich, L., Heinrich, I., Minor, R. L., Sonnentag, O., Matheny, A. M., Wightman, M. G., and Steppe, K.: Quantification of uncertainties in conifer sap flow measured with the thermal dissipation method, New Phytol., 219, 1283–1299, https://doi.org/10.1111/nph.15241, 2018.
Peters, R. L., Pappas, C., Hurley, A. G., Poyatos, R., Flo, V., Zweifel, R., Goossens, W., and Steppe, K.: Assimilate, process and analyse thermal dissipation sap flow data using the TREX r package, Methods Ecol. Evol., 12, 342–350, https://doi.org/10.1111/2041-210X.13524, 2021.
Phillips, N., Oren, R., and Zimmerman, R.: Radial patterns of sylem sap flow in non-, diffuse- and ring-porous tree species, Plant Cell Environ., 19, 983–990, 1996.
Phillips, N., Nagchaudhuri, A., Oren, R., and Katul, G.: Time constant for water transport in loblolly pine trees estimated from time series of evaporative demand and stem sapflow, Trees, 11, 412–419, https://doi.org/10.1007/s004680050102, 1997.
Phillips, N. G., Oren, R., Licata, J., and Linder, S.: Time series diagnosis of tree hydraulic characteristics, Tree Physiol., 24, 879–890, https://doi.org/10.1093/treephys/24.8.879, 2004.
Phillips, N. G., Scholz, F. G., Bucci, S. J., Goldstein, G., and Meinzer, F. C.: Using branch and basal trunk sap flow measurements to estimate whole-plant water capacitance: comment on Burgess and Dawson (2008), Plant Soil, 315, 315–324, https://doi.org/10.1007/s11104-008-9741-y, 2009.
Poyatos, R., Martínez-Vilalta, J., Čermák, J., Ceulemans, R., Granier, A., Irvine, J., Köstner, B., Lagergren, F., Meiresonne, L., Nadezhdina, N., Zimmermann, R., Llorens, P., and Mencuccini, M.: Plasticity in hydraulic architecture of Scots pine across Eurasia, Oecologia, 153, 245–259, 2007.
Poyatos, R., Granda, V., Molowny-Horas, R., Mencuccini, M., Steppe, K., and Martínez-Vilalta, J.: SAPFLUXNET: towards a global database of sap flow measurements, Tree Physiol., 36, 1449–1455, https://doi.org/10.1093/treephys/tpw110, 2016.
Poyatos, R., Granda, V., Flo, V., Molowny-Horas, R., Steppe, K., Mencuccini, M., and Martínez-Vilalta, J.: SAPFLUXNET: A global database of sap flow measurements, (Version 0.1.5) [Data set], Zenodo, https://doi.org/10.5281/zenodo.3971689, 2020a.
Poyatos, R., Flo, V., Granda, V., Steppe, K., Mencuccini, M., and Martínez-Vilalta, J.: Using the SAPFLUXNET database to understand transpiration regulation of trees and forests, Acta Hortic., 1300, 179–186, https://doi.org/10.17660/ActaHortic.2020.1300.23, 2020b.
Poyatos, R., Granda, V., Flo, V., Mencuccini, M., and Martínez-Vilalta, J.: Code associated to the SAPFLUXNET data paper (v. 0.1.5) (Version v1.0.0) [code], Zenodo, https://doi.org/10.5281/zenodo.4727825, 2021.
Rascher, K. G., Máguas, C., and Werner, C.: On the use of phloem sap δ13C as an indicator of canopy carbon discrimination, Tree Physiol., 30, 1499–1514, https://doi.org/10.1093/treephys/tpq092, 2010.
R Core Team: A Language and Environment for Statistical Computing, R Foundation for Statistical Computing, Vienna, Austria, available at: https://www.R-project.org/ (last access: 8 June 2021), 2019.
Reichstein, M., Bahn, M., Mahecha, M. D., Kattge, J., and Baldocchi, D. D.: Linking plant and ecosystem functional biogeography, P. Natl. Acad. Sci. USA, 111, 13697–13702, https://doi.org/10.1073/pnas.1216065111, 2014.
Resco de Dios, V., Chowdhury, F. I., Granda, E., Yao, Y., and Tissue, D. T.: Assessing the potential functions of nocturnal stomatal conductance in C3 and C4 plants, New Phytol., 223, 1696–1706, https://doi.org/10.1111/nph.15881, 2019.
Richardson, A. D., Aubinet, M., Barr, A. G., Hollinger, D. Y., Ibrom, A., Lasslop, G., and Reichstein, M.: Uncertainty Quantification, in: Eddy Covariance: A Practical Guide to Measurement and Data Analysis, edited by: Aubinet, M., Vesala, T., and Papale, D., Springer, Dordrecht, The Netherlands, 173–209, https://doi.org/10.1007/978-94-007-2351-1_7, 2012.
Rodell, M., Beaudoing, H. K., L'Ecuyer, T. S., Olson, W. S., Famiglietti, J. S., Houser, P. R., Adler, R., Bosilovich, M. G., Clayson, C. A., Chambers, D., Clark, E., Fetzer, E. J., Gao, X., Gu, G., Hilburn, K., Huffman, G. J., Lettenmaier, D. P., Liu, W. T., Robertson, F. R., Schlosser, C. A., Sheffield, J., and Wood, E. F.: The Observed State of the Water Cycle in the Early Twenty-First Century, J. Climate, 28, 8289–8318, https://doi.org/10.1175/JCLI-D-14-00555.1, 2015.
Sakuratani, T.: A Heat Balance Method for Measuring Water Flux in the Stem of Intact Plants, J. Agric. Meteorol., 37, 9–17, https://doi.org/10.2480/agrmet.37.9, 1981.
Salomón, R. L., Limousin, J. M., Ourcival, J. M., Rodríguez-Calcerrada, J., and Steppe, K.: Stem hydraulic capacitance decreases with drought stress: implications for modelling tree hydraulics in the Mediterranean oak Quercus ilex, Plant Cell Environ., 40, 1379–1391, https://doi.org/10.1111/pce.12928, 2017.
Sánchez-Costa, E., Poyatos, R., and Sabaté, S.: Contrasting growth and water use strategies in four co-occurring Mediterranean tree species revealed by concurrent measurements of sap flow and stem diameter variations, Agr. Forest Meteorol., 207, 24–37, https://doi.org/10.1016/j.agrformet.2015.03.012, 2015.
Schäfer, K. V. R., Oren, R., and Tenhunen, J. D.: The effect of tree height on crown level stomatal conductance, Plant Cell Environ., 23, 365–375, 2000.
Schlesinger, W. H. and Jasechko, S.: Transpiration in the global water cycle, Agr. Forest Meteorol., 189–190, 115–117, https://doi.org/10.1016/j.agrformet.2014.01.011, 2014.
Schulze, E.-D., Čermák, J., Matyssek, M., Penka, M., Zimmermann, R., Vasícek, F., Gries, W., and Kučera, J.: Canopy transpiration and water fluxes in the xylem of the trunk of Larix and Picea trees – a comparison of xylem flow, porometer and cuvette measurements, Oecologia, 66, 475–483, https://doi.org/10.1007/BF00379337, 1985.
Schwalm, C. R., Anderegg, W. R. L., Michalak, A. M., Fisher, J. B., Biondi, F., Koch, G., Litvak, M., Ogle, K., Shaw, J. D., Wolf, A., Huntzinger, D. N., Schaefer, K., Cook, R., Wei, Y., Fang, Y., Hayes, D., Huang, M., Jain, A., and Tian, H.: Global patterns of drought recovery, Nature, 548, 202–205, https://doi.org/10.1038/nature23021, 2017.
Shuttleworth, W. J.: Putting the “vap” into evaporation, Hydrol. Earth Syst. Sci., 11, 210–244, https://doi.org/10.5194/hess-11-210-2007, 2007.
Silvertown, J., Araya, Y., and Gowing, D.: Hydrological niches in terrestrial plant communities: a review, J. Ecol., 103, 93–108, https://doi.org/10.1111/1365-2745.12332, 2015.
Simonin, K., Kolb, T., Montes-Helu, M., and Koch, G.: The influence of thinning on components of stand water balance in a ponderosa pine forest stand during and after extreme drought, Agr. Forest Meteorol., 143, 266–276, 2007.
Skelton, R. P., West, A. G., Dawson, T. E., and Leonard, J. M.: External heat-pulse method allows comparative sapflow measurements in diverse functional types in a Mediterranean-type shrubland in South Africa, Functional Plant Biol., 40, 1076–1087, https://doi.org/10.1071/FP12379, 2013.
Smith, D. and Allen, S.: Measurement of sap flow in plant stems, J. Exp. Bot., 47, 1833–1844, 1996.
Speckman, H., Ewers, B. E., and Beverly, D. P.: AquaFlux: Rapid, transparent and replicable analyses of plant transpiration, Methods Ecol. Evol., 11, 44–50, https://doi.org/10.1111/2041-210X.13309, 2020.
Staal, A., Tuinenburg, O. A., Bosmans, J. H. C., Holmgren, M., van Nes, E. H., Scheffer, M., Zemp, D. C., and Dekker, S. C.: Forest-rainfall cascades buffer against drought across the Amazon, Nat. Clim. Change, 8, 539–543, https://doi.org/10.1038/s41558-018-0177-y, 2018.
Steppe, K., De Pauw, D. J., Lemeur, R., and Vanrolleghem, P. A.: A mathematical model linking tree sap flow dynamics to daily stem diameter fluctuations and radial stem growth, Tree Physiol., 26, 257–273, 2006.
Steppe, K., De Pauw, D. J. W., Doody, T. M., and Teskey, R. O.: A comparison of sap flux density using thermal dissipation, heat pulse velocity and heat field deformation methods, Agr. Forest Meteorol., 150, 1046–1056, https://doi.org/10.1016/j.agrformet.2010.04.004, 2010.
Steppe, K., Sterck, F., and Deslauriers, A.: Diel growth dynamics in tree stems: linking anatomy and ecophysiology, Trends Plant Sci., 20, 335–343, https://doi.org/10.1016/j.tplants.2015.03.015, 2015.
Stoy, P. C., El-Madany, T. S., Fisher, J. B., Gentine, P., Gerken, T., Good, S. P., Klosterhalfen, A., Liu, S., Miralles, D. G., Perez-Priego, O., Rigden, A. J., Skaggs, T. H., Wohlfahrt, G., Anderson, R. G., Coenders-Gerrits, A. M. J., Jung, M., Maes, W. H., Mammarella, I., Mauder, M., Migliavacca, M., Nelson, J. A., Poyatos, R., Reichstein, M., Scott, R. L., and Wolf, S.: Reviews and syntheses: Turning the challenges of partitioning ecosystem evaporation and transpiration into opportunities, Biogeosciences, 16, 3747–3775, https://doi.org/10.5194/bg-16-3747-2019, 2019.
Swanson, R. H.: Significant historical developments in thermal methods for measuring sap flow in trees, Agr. Forest Meteorol., 72, 113–132, https://doi.org/10.1016/0168-1923(94)90094-9, 1994.
Swanson, R. H. and Whitfield, D. W. A.: A Numerical Analysis of Heat Pulse Velocity Theory and Practice, J. Exp. Bot., 32, 221–239, https://doi.org/10.1093/jxb/32.1.221, 1981.
Talsma, C. J., Good, S. P., Jimenez, C., Martens, B., Fisher, J. B., Miralles, D. G., McCabe, M. F., and Purdy, A. J.: Partitioning of evapotranspiration in remote sensing-based models, Agr. Forest Meteorol., 260–261, 131–143, https://doi.org/10.1016/j.agrformet.2018.05.010, 2018.
Tor-ngern, P., Oren, R., Oishi, A. C., Uebelherr, J. M., Palmroth, S., Tarvainen, L., Ottosson-Löfvenius, M., Linder, S., Domec, J.-C., and Näsholm, T.: Ecophysiological variation of transpiration of pine forests: synthesis of new and published results, Ecol. Appl., 27, 118–133, https://doi.org/10.1002/eap.1423, 2017.
Tor-ngern, P., Oren, R., Palmroth, S., Novick, K., Oishi, A., Linder, S., Ottosson-Löfvenius, M., and Näsholm, T.: Water balance of pine forests: Synthesis of new and published results, Agr. Forest Meteorol., 259, 107–117, 2018.
Tyree, M. T. and Zimmermann, M. H.: Xylem Structure and the Ascent of Sap, Springer, Berlin, Germany, 284 pp., 2002.
Vandegehuchte, M. W. and Steppe, K.: Sapflow+: a four-needle heat-pulse sap flow sensor enabling nonempirical sap flux density and water content measurements, New Phytol., 196, 306–317, https://doi.org/10.1111/j.1469-8137.2012.04237.x, 2012.
Vandegehuchte, M. W. and Steppe, K.: Sap-flux density measurement methods: working principles and applicability, Funct. Plant Biol., 40, 213–223, https://doi.org/10.1071/FP12233, 2013.
Vernay, A., Tian, X., Chi, J., Linder, S., Mäkelä, A., Oren, R., Peichl, M., Stangl, Z. R., Tor-ngern, P., and Marshall, J. D.: Estimating canopy gross primary production by combining phloem stable isotopes with canopy and mesophyll conductances, Plant Cell Environ., 43, 2124–2142, https://doi.org/10.1111/pce.13835, 2020.
Vitale, D., Fratini, G., Bilancia, M., Nicolini, G., Sabbatini, S., and Papale, D.: A robust data cleaning procedure for eddy covariance flux measurements, Biogeosciences, 17, 1367–1391, https://doi.org/10.5194/bg-17-1367-2020, 2020.
Vose, J. M., Miniat, C. F., Luce, C. H., Asbjornsen, H., Caldwell, P. V., Campbell, J. L., Grant, G. E., Isaak, D. J., Loheide, S. P., and Sun, G.: Ecohydrological implications of drought for forests in the United States, Forest Ecol. Manag., 380, 335–345, https://doi.org/10.1016/j.foreco.2016.03.025, 2016.
Vuichard, N. and Papale, D.: Filling the gaps in meteorological continuous data measured at FLUXNET sites with ERA-Interim reanalysis, Earth Syst. Sci. Data, 7, 157–171, https://doi.org/10.5194/essd-7-157-2015, 2015.
Wang, K. and Dickinson, R. E.: A review of global terrestrial evapotranspiration: Observation, modeling, climatology, and climatic variability, Rev. Geophys., 50, RG2005, https://doi.org/10.1029/2011RG000373, 2012.
Wang-Erlandsson, L., van der Ent, R. J., Gordon, L. J., and Savenije, H. H. G.: Contrasting roles of interception and transpiration in the hydrological cycle – Part 1: Temporal characteristics over land, Earth Syst. Dynam., 5, 441–469, https://doi.org/10.5194/esd-5-441-2014, 2014.
Ward, E. J., Bell, D. M., Clark, J. S., and Oren, R.: Hydraulic time constants for transpiration of loblolly pine at a free-air carbon dioxide enrichment site, Tree Physiol., 33, 123–134, https://doi.org/10.1093/treephys/tps114, 2013.
Ward, E. J., Domec, J.-C., King, J., Sun, G., McNulty, S., and Noormets, A.: TRACC: an open source software for processing sap flux data from thermal dissipation probes, Trees, 31, 1737–1742, https://doi.org/10.1007/s00468-017-1556-0, 2017.
Wei, Z., Yoshimura, K., Wang, L., Miralles, D. G., Jasechko, S., and Lee, X.: Revisiting the contribution of transpiration to global terrestrial evapotranspiration, Geophys. Res. Lett., 44, 2792–2801, https://doi.org/10.1002/2016GL072235, 2017.
Whitehead, D.: Regulation of stomatal conductance and transpiration in forest canopies, Tree Physiol., 18, 633–644, 1998.
Whitley, R., Taylor, D., Macinnis-Ng, C., Zeppel, M., Yunusa, I., O'Grady, A., Froend, R., Medlyn, B., and Eamus, D.: Developing an empirical model of canopy water flux describing the common response of transpiration to solar radiation and VPD across five contrasting woodlands and forests, Hydrol. Process., 27, 1133–1146, https://doi.org/10.1002/hyp.9280, 2013.
Whittaker, R. H.: Communities and ecosystems, Macmillan, New York, NY, USA, 1970.
Wild, M., Folini, D., Hakuba, M. Z., Schär, C., Seneviratne, S. I., Kato, S., Rutan, D., Ammann, C., Wood, E. F., and König-Langlo, G.: The energy balance over land and oceans: an assessment based on direct observations and CMIP5 climate models, Clim. Dynam., 44, 3393–3429, https://doi.org/10.1007/s00382-014-2430-z, 2015.
Williams, C. A., Reichstein, M., Buchmann, N., Baldocchi, D., Beer, C., Schwalm, C., Wohlfahrt, G., Hasler, N., Bernhofer, C., Foken, T., Papale, D., Schymanski, S., and Schaefer, K.: Climate and vegetation controls on the surface water balance: Synthesis of evapotranspiration measured across a global network of flux towers, Water Resour. Res., 48, W06523, https://doi.org/10.1029/2011WR011586, 2012.
Williams, M., Bond, B. J., and Ryan, M. G.: Evaluating different soil and plant hydraulic constraints on tree function using a model and sap flow data from ponderosa pine, Plant Cell Environ., 24, 679–690, 2001.
Wilson, K. B., Hanson, P. J., Mulholland, P. J., Baldocchi, D. D., and Wullschleger, S. D.: A comparison of methods for determining forest evapotranspiration and its components: sap-flow, soil water budget, eddy covariance and catchment water balance, Agr. Forest Meteorol., 106, 153–168, 2001.
Wullschleger, S. D., Meinzer, F. C., and Vertessy, R. A.: A review of whole-plant water use studies in trees, Tree Physiol., 18, 499–512, https://doi.org/10.1093/treephys/18.8-9.499, 1998.
Wutzler, T., Lucas-Moffat, A., Migliavacca, M., Knauer, J., Sickel, K., Šigut, L., Menzer, O., and Reichstein, M.: Basic and extensible post-processing of eddy covariance flux data with REddyProc, Biogeosciences, 15, 5015–5030, https://doi.org/10.5194/bg-15-5015-2018, 2018.
Yin, J. and Bauerle, T. L.: A global analysis of plant recovery performance from water stress, Oikos, 126, 1377–1388, https://doi.org/10.1111/oik.04534, 2017.
Zeppel, M. J. B., Lewis, J. D., Phillips, N. G., and Tissue, D. T.: Consequences of nocturnal water loss: a synthesis of regulating factors and implications for capacitance, embolism and use in models, Tree Physiol., 34, 1047–1055, https://doi.org/10.1093/treephys/tpu089, 2014.
Zhang, Q., Manzoni, S., Katul, G., Porporato, A., and Yang, D.: The hysteretic evapotranspiration – Vapor pressure deficit relation, J. Geophys. Res.-Biogeo., 119, 125–140, https://doi.org/10.1002/2013JG002484, 2014.
Zhao, S., Pederson, N., D'Orangeville, L., HilleRisLambers, J., Boose, E., Penone, C., Bauer, B., Jiang, Y., and Manzanedo, R. D.: The International Tree-Ring Data Bank (ITRDB) revisited: Data availability and global ecological representativity, J. Biogeogr., 46, 355–368, https://doi.org/10.1111/jbi.13488, 2019.
Zhao, W. L., Gentine, P., Reichstein, M., Zhang, Y., Zhou, S., Wen, Y., Lin, C., Li, X., and Qiu, G. Y.: Physics-Constrained Machine Learning of Evapotranspiration, Geophys. Res. Lett., 46, 14496–14507, https://doi.org/10.1029/2019GL085291, 2019.
Zweifel, R., Steppe, K., and Sterck, F. J.: Stomatal regulation by microclimate and tree water relations: interpreting ecophysiological field data with a hydraulic plant model, J. Exp. Bot., 58, 2113–2131, https://doi.org/10.1093/jxb/erm050, 2007.
- Abstract
- Introduction
- The SAPFLUXNET data workflow
- The SAPFLUXNET database
- Potential applications
- Limitations and future developments
- Data availability, access, and feedback
- Code availability
- Conclusions
- Appendix A: References for individual datasets in SAPFLUXNET
- Appendix B: Uncertainty estimation in sap flow measurements in the SAPFLUXNET database
- Author contributions
- Competing interests
- Acknowledgements
- Financial support
- Review statement
- References
- Supplement
sapfluxnetrwill facilitate new data syntheses on the ecological factors driving water use and drought responses of trees and forests.
- Abstract
- Introduction
- The SAPFLUXNET data workflow
- The SAPFLUXNET database
- Potential applications
- Limitations and future developments
- Data availability, access, and feedback
- Code availability
- Conclusions
- Appendix A: References for individual datasets in SAPFLUXNET
- Appendix B: Uncertainty estimation in sap flow measurements in the SAPFLUXNET database
- Author contributions
- Competing interests
- Acknowledgements
- Financial support
- Review statement
- References
- Supplement