the Creative Commons Attribution 4.0 License.
the Creative Commons Attribution 4.0 License.
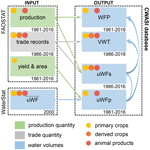
Virtual water trade and water footprint of agricultural goods: the 1961–2016 CWASI database
Marta Tuninetti
Irene Soligno
Francesco Laio
To support national and global assessments of water use in agriculture, we build a comprehensive database of country-specific water footprint and virtual water trade (VWT) data for 370 agricultural goods. The water footprint, indicating the water needed for the production of a good including rainwater and water from surface water and groundwater bodies, is expressed as a volume per unit weight of the good (or unit water footprint, uWF) and is here estimated at the country scale for every year in the period 1961–2016. The uWF is also differentiated, where possible, between production and supply, referring to local production and to a weighted mean of local production and import, respectively. The VWT data, representing the amount of water needed for the production of a good and virtually exchanged with the international trade, are provided for each commodity as bilateral trade matrices, between origin and destination countries, for every year in the period 1986–2016. The database, developed within the CWASI project, improves upon earlier datasets because it takes into account the annual variability of the uWF of crops, it accounts for both produced and imported goods in the definition of the supply-side uWF, and it traces goods across the international trade up to the origin of goods' production. The CWASI database is available on the Zenodo repository at https://doi.org/10.5281/zenodo.4606794 (Tamea et al., 2020), and it welcomes contributions and improvements from the research community to enable analyses specifically accounting for the temporal evolution of the uWF.
- Article
(2290 KB) - Full-text XML
- BibTeX
- EndNote
There has been a booming interest in the concept of water footprint (WF) since its introduction about 15 years ago (Hoekstra and Chapagain, 2007, 2008). The water footprint offers a common approach, language, and method to a wide range of analyses and multidisciplinary studies, and it is appreciated for its capability to convey environmental messages to the public. The WF identifies the freshwater needed for the production of goods along the full supply chain, separating rainfall and water from surface water/groundwater bodies. The WF assessment provides a quantitative framework to analyze the volume of water embedded in agricultural goods and the efficiency of water use, when the metric is computed per unit weight of the good (hereafter referred to as the unit water footprint, or uWF). The term unit water footprint is here introduced to unify the current terminology which includes “water footprint”, used indifferently for volumes and for volumes per unit weight; “crop water footprint” which excludes livestock products; or “virtual water content” mainly used within the context of trade (see, e.g., Hoekstra et al., 2011; Konar et al., 2011; Dalin et al., 2012; Tuninetti et al., 2015). Also, the concept of virtual water, originally proposed by J. A. Allan (1998) and from which the WF originated, has been growing in popularity among both the scientific community and the general public. Virtual water is the volume of water needed to produce a certain good that is virtually traded as a factor of production when the good is exchanged among countries. Such virtual flow defines the international virtual water trade (VWT) and represents a metric that is suitable to analyze environmental aspects related to the global trade of agricultural goods, to the water management and to the agricultural policy.
Assessment of WF and VWT requires a relatively large amount of data, including production and trade data (in metric tons, t) and unit water footprint data (in m3 t−1). The first remarkable database of uWF data has been prepared and shared by the Water Footprint Network, which published a large open-access dataset of uWF for several primary and processed agricultural goods, of crop and animal origin (Mekonnen and Hoekstra, 2010a, b). This database, named WaterStat, includes average values over the period 1996–2005 and has been the basis of the water footprint assessment as presented, e.g., in Hoekstra et al. (2011). Other uWF datasets exist, which are based on spatially distributed models coupling the soil water balance with vegetation growth (see, e.g., Tuninetti et al., 2015, and references therein); such databases mostly refer to a single year or a period or to long-term averages. Other datasets, referring to blue water or to scarcity-weighted indicators, are also available from the literature related to the life cycle assessment (e.g., Pfister et al., 2011, 2016). The temporal variability of uWF has been seldom considered. Few examples include water scarcity indexes (e.g., Pfister and Bayer, 2014) or annual time series of uWF in the EORA database, based on assumptions about the economic growth of different production sectors (Lenzen et al., 2013). Recently, Tuninetti et al. (2017) proposed a fast-track method to estimate annual uWF values from WaterStat using agricultural yield data.
International trade statistics of agricultural goods are organized and shared by, e.g., the Food and Agriculture Organization (FAOSTAT) and the United Nations (UN-COMTRADE). Early publications by the Water Footprint Network (e.g., Hoekstra and Chapagain, 2008) are based on the combination of such trade databases and WaterStat to produce WF assessments. Trade data are also organized and shared as input–output tables, tracing supply chains across sectors and countries, whose worldwide dimension is captured by global multi-regional input–output (MRIO) tables (see Tukker and Dietzenbacher, 2013, for a review). In such a framework, some MRIO databases offer specific water-related extensions, quantifying water volumes associated with international trade (e.g., Geschke and Hadjikakou, 2017). Two relevant examples are the EORA database (Lenzen et al., 2013) and EXIOBASE (Stadler et al., 2108), both including a water assessment distinguishing between green and blue water and including the temporal variability, although product categories and geographical regions are more aggregated than in the present study. Supply chains and trade of specific products, with their impact on the local environment and the water resources, are also the objectives of the TRASE project developed by the Stockholm Environment Institute and the Global Canopy Programme (SEI, 2019). Such a project focuses on a limited set of products, although accurately investigating their supply chain and environmental effects.
Methodologies for VWT and WF assessment can be classified in two approaches: the bottom-up approach and the top-down approach. The bottom-up approach refers to a process-based analysis, with a detailed description of production processes and associated water volumes. Within such an approach, the uWF of each good is multiplied by the (produced or traded) quantity of the good, and resulting water volumes are then summed across goods. WaterStat is the main example of a bottom-up approach The top-down approach aims at tracing full supply chains throughout economic sectors and different countries. Input–output analyses, frequently used in economics for environmental assessments, belong to this approach (Duarte and Yang, 2011). Bottom-up approaches do not consider the entire supply chain of goods and can be affected by truncation errors when used to assess the water footprint of final consumption (Feng et al., 2011). At the same time, bottom-up techniques can offer high commodity resolution considering the water associated with the production of a large variety of single (agricultural) products. A major problem affecting bottom-up approaches is the identification of the geographic origin of produced goods (Hubacek and Feng, 2016). In many cases, product re-export disconnects producing and consuming countries, now allowing a correct identification of dependencies and externalities. In the present work, we improve the traditional bottom-up approach by identifying the origin of produced goods and reconstructing the supply chain of agricultural goods, implementing the method proposed in Kastner et al. (2011). With such improvement, the VWT quantified in this study aims both at best estimating the water embodied in bilateral trade and at providing accurate estimates of the total virtual water embedded in final consumption (Feng et al., 2011; Lenzen et al., 2013).
In this publication, we present an open-access database of virtual water trade, including the annual trade matrices (years 1986–2016) and the annual virtual water export (years 1961–2016) associated with a large number of agricultural products, as well as their unit water footprint in all countries (years 1961–2016), referring to the sum of green water (originating from rainfall) and blue water (originating from surface water and groundwater bodies). Starting from the uWF dataset in Mekonnen and Hoekstra (2010a, b), we extend it to provide annual statistics of uWF. Improvements also include the differentiation between the production side and supply side of uWF. The new time-varying uWFs are applied to the FAOSTAT datasets of agricultural production and trade. The results of this analysis constitute the CWASI database.
The database addresses several needs: (i) the need for a comprehensive database of uWF, WF, and VWT; (ii) the need to adopt unit water footprints that vary in time, as recently pointed out by D'Odorico et al. (2019); (iii) the need to disentangle the production side and the supply side uWF to coherently assess the WF of production and consumption; and (iv) the need for ready-to-use detailed trade matrices, accurately tracing goods' trade and origin, suitable for network analyses. The uWF dataset may also be useful for other methodologies of WF and VWT assessments, such as those based on input–output matrices or the one proposed in the ISO standardization (ISO, 2014).
The present database has been developed within the EU-funded CWASI project “Coping with WAter Scarcity In a globalized world”, and it is shared through an online open-access repository (Tamea et al., 2020). In a relatively recent overview of the field, the research lines that originated from the concept of WF were identified (Hoekstra, 2017). These are the role of trade and globalization in goods production and consumption and how they affect local water issues, the comparison of water requirements with water availability and renewability, and the supply-chain approach applied to water management. With the CWASI database we aim at contributing to these research lines and provide all researchers with an up-to-date and ready-to-use starting point for their research. The database will welcome additions and external contributions that may possibly become available in the future and will represent an open and shared source of data on water footprint and virtual water trade.
From FAOSTAT, the statistical database of the Food and Agriculture Organization (FAO), we collected 31 years (1986–2016) of trade data of agricultural goods (FAO, 2019b). Data originate from national accounting and are available as records containing the following information: reporting country (with FAO code), type of trade (import or export), partner country reported within the trade record (with FAO code), year, commodity (with FAO code), unit of measure, and quantity. From FAOSTAT, we also collected 56 years of agricultural production data including crop-based and animal-based commodities, containing the following information: production country (with FAO code), year, commodity (with FAO code), unit of measure, and quantity (FAO, 2019a, 2020a, b, c, d). From the same source, data of agricultural yield and harvested area were also collected for each considered crop, country, and year in the period 1961–2016 (FAO, 2019a). Reference unit water footprint values for every commodity and country, averaged around the year 2000 (1996–2005 period), are taken from WaterStat (Mekonnen and Hoekstra, 2010a, b), as well as the product fraction and the value fraction needed for the computation of the uWF of processed crops. A detailed summary of data sources has been arranged in Table 1.
2.1 Commodities
Production and trade data collected from FAOSTAT include crops, processed crops, primary livestock, processed livestock, and live animals. The commodities currently included in the CWASI database are 370 and have been identified as those whose FAO code or name or description could be associated with a WaterStat database entry (commodities are listed in the Appendix, Table A1). Commodities includes all products in the “Crop” production statistics of FAO, many processed crops with the exception of feed products (such as bran), animals, and animal-based products for most relevant species. Among all commodities, some appear in both trade and production data, some appear only in trade, and some others appear only in production. Production data are only available for primary goods and for a few processed goods, while trade includes primary goods and a larger set of processed goods. For example, the flour or the bread of wheat are only available as trade data because production data only include the primary commodity (wheat). Conversely, yams or sugar cane are only available as production data because their trade is not recorded in the FAO statistics, possibly because they are not internationally exchanged as raw product. Commodities have been subdivided into nine categories whose numbers of produced and traded commodities are specified in Fig. 1. The FAOSTAT database provides the amounts of goods produced (or traded) in any given country (or pair of countries) for each commodity and year expressed in tons or heads, depending on the type of product (see the details in Table 1).

Figure 1Commodities considered in the analysis, split into nine categories: number of commodities in the trade and production dataset. Icons designed by Freepik from Flaticon (https://www.flaticon.com/, last access: 5 May 2021).
2.2 Countries
The database considers all geographical, political, and economical entities reporting (or reported for) at least one product and 1 year, in either the trade or the production data. From 1961 to 2016, agricultural goods were produced and traded among 255 entities with a temporary or permanent activity (the full list is reported in the Appendix, in Table A2). Not all 255 countries were active along the whole considered period, as they underwent political and/or administrative changes. Examples include the collapse of the USSR, the separation of Eritrea from Ethiopia, or the split of Belgium and Luxembourg, which were considered a single entity until the year 2000. Despite being inactive, a country may be reported by partners as importing or exporting goods. Values reported for a country outside its range of active years are associated with the corresponding active country or the largest of them (e.g., a trade reported for USSR in 1992 is associated with the Russian Federation). The following non-overlapping FAO entries, “China, Mainland”, “China, Hong Kong SAR”, “China, Macao SAR”, and “China, Taiwan Province of”, have been considered in place of the aggregate entry “China”. Two entries of unclear location (Neutral Zone, Unspecified) are listed but values are not considered, in order to avoid the erroneous accounting of trade fluxes. Discontinuities in the active periods in each country are listed in the Appendix, in Table A2.
2.3 Trade matrices
The detailed trade data provided by FAO (2019b) include the international trade records reported by each country. Reporting countries across the years are 186, whereas the remaining ones (up to 255) are only reported by others. There is a total of 9 million records (i.e., trade flows per country pairs, per commodity, and per year, for the commodities included in the CWASI dataset), and the number of records reported by each country is detailed in Fig. 2. These records are used to reconstruct the trade matrix M for each commodity and year, having dimensions 255×255 and showing the exporting countries in the rows and the importing countries in the columns. The matrix element M(i,j) thus identifies the trade flow from country i to country j, which is clearly different than the flow from country j to country i, i.e., . Sub-national trade is not considered in these matrices, and the terms on the diagonals are zeros.
In the construction of trade matrices, one should consider that the same trade flow can be reported twice in the FAOSTAT database, once by the exporting country and once by the importing country. When a trade flow is reported by only one of the two countries, the reported flow is used to construct the matrix (single record); this is the case for 40 % of records in the database. All other records are “double” (reported twice) and require a comparison between the declarations of the exporting and the importing countries, which are usually different, with a mean (absolute) relative difference, across all goods, countries, and years, of 61 %. The choice of a value from two double records is called “reconciliation”, and the method adopted here is based on the identification of the most reliable reporting country among the two involved in each flow, and the use of the flow being reported by it. The reliability of countries is measured per commodity and per year with a data-based approach detailed below and adapted from Gehlhar (1996).
Country reliability
For each product, p, and year, t, two trade matrices are built, one matrix collecting all “Importer-Reported” flows and the other matrix collecting the “Exporter-Reported” flows. The matrices have the same structure and dimensions, with the exporter countries in the rows and the importing countries in the columns. Then a reliability index is calculated for each country, c, differentiating between import and export. First, an accuracy measure (A) is defined for every flux, from country i to country j, as
with IR(i,j) being the importer-reported trade flux and ER(i,j) being the exporter-reported flux. The measure is modified from Gehlhar (1996) to maintain the conceptual symmetry between import and export. The smaller the measure, the more similar the information reported by the importing and exporting country. Then, the reliability of each country is measured, separately for import and export, based on the comparison between the flows reported by the country and by its trade partners. For every country, c, the reliability index for imports, RIimp(c), and for exports, RIexp(c), is defined as follows:
where IR(j,c) is the flux from country j to c, as reported by c (importer-reported), and ER(c,i) is the flux from c to any country i, as reported by c (exporter-reported), respectively. Σall is the sum of all import or export fluxes reported by c, and Σacc is the sum of acceptable fluxes only, defined as the fluxes whose accuracy A (Eq. 1) is smaller than an acceptance threshold, set to 20 % as in Gehlhar (1996). IR(w,c) and ER(c,w) in Eq. (2) are, respectively, the import from and the export to the worse partner w defined as the ones having the maximum (worse) flow-weighted accuracy measure (WA) defined, for import and export fluxes, as
A(j,c) is the accuracy level of flux IR(j,c), and A(c,i) is the accuracy level of flux ER(c,i); the denominators are, respectively, the sum of all imports and all exports reported by country c.
Reliability indexes are calculated by country, commodity, year, and flow direction (import and export). This is because the reliability of a country in reporting import and export may be different; the attitude of a country to over-report or under-report may differ by products, e.g., depending on taxation; and the reliability of a country may change in time, e.g., according to socio-political factors. The direction- and commodity-averaged RI of reporting countries are shown in Fig. 2 with the darker (lighter) line corresponding to the newest (oldest) values. Countries more involved in trade and reporting more information (to the left) are characterized, on average, by a larger reliability, while countries less involved in trade have lower average reliability, which used to be very low in the past. Current RI values, instead, are more uniform across countries. Having computed all reliability indexes, the “reconciled” trade matrix for each good and year is built, combining importer-reported and exporter-reported data. Each matrix element M(i,j) is taken from the IR or ER matrix if the importing country j or the exporting i has a larger reliability index. Where the reliability indexes are equal, the country with larger acceptable fluxes is chosen.
The unit water footprint measures the amount of water required to produce a unit amount of product and it can be expressed as m3 t−1 or, equivalently, as L kg−1. The present work considers the sum of green water (originating from rainfall) and blue water (originating from surface water and groundwater bodies). Depending on the type of commodity, different approaches are applied for the computation of the unit water footprint. In the present work we propose a differentiation between the uWF of production (uWFp) and the uWF of supply (uWFs). The uWFp refers to locally produced crops whose water footprint depends on the actual crop evapotranspiration and crop yield, with annual estimates starting in 1961. The uWFp is a suitable indicator to assess the WF of agricultural production. The uWF, instead, refers to the domestic supply, which relies both on local production and on international trade. Country-scale domestic supply is available for human consumption, food manufacturing, feed for livestock, and export towards other countries. The impossibility to track local production and imports into consumption and exports, within each country, makes the uWFs the best indicator to be used in conjunction with consumption and export data. The uWF is computed averaging local production and imports, after having identified the countries of origin of the goods with an appropriate procedure applicable from the year 1986.
For primary crops, it has been possible to estimate both the uWFp and the uWFs. Processed crops are produced from a root product which may or may not originate from local production. The absence of systematic FAO data about the production of processed crops prevents the differentiation between the unit water footprint of production and of supply. Therefore, processed crops considered in this study will have a single unit water footprint, depending on country and year, computed from the uWFs of the root product. Finally, animal-based products are considered here only with the WaterStat values, without temporal variability.
3.1 Unit water footprint of locally produced primary crops in time
When considering the production of primary crops, the unit water footprint of production, uWFp, is a function of the actual evapotranspiration along the growing period of the crop and the actual crop yield. Due to precipitation, evapotranspiration, and yield fluctuations, the uWFp exhibits significant spatiotemporal variability. We computed the uWFp in a given year by means of the fast-track (FT) method, introduced and substantiated in Tuninetti et al. (2017). This method is based on the use of the WaterStat database (Mekonnen and Hoekstra, 2010a, 2010b) for expressing the spatial variations in evapotranspiration and on a ratio of agricultural yields for expressing the temporal variability of the unit water footprint, not detailed in WaterStat.
According to the fast-track method, the unit water footprint of an agricultural product p produced in country c in year t, i.e., , reads
where is the reference unit water footprint provided by WaterStat (Mekonnen and Hoekstra, 2010a, b) corresponding to an average in the period T=1996–2005, is the average crop yield over the same period T, and is the annual crop yield in a generic year t in the range 1961–2016. The average crop yield is obtained as an average of the annual yields in the years 1996–2005, weighted by the harvested areas across the years in country c, based on FAOSTAT data (FAO, 2019a).
The fast-track method keeps the actual evapotranspiration of crops implicitly constant, equal to the long-term average used in the WaterStat statistics, but this hypothesis should come at no surprise. On the one hand, yield implicitly expresses many factors, including climatic conditions, water availability, soil fertility, and agricultural practices among others, and temporal yield variations dominate the variability of the water volumes used (evapotranspired) by crops. On the other hand, the uWF is less sensitive to hydro-climatic conditions than actual evapotranspiration because it is defined as the ratio between evapotranspiration and yield, both reacting with equal signs to hydro-climatic fluctuations (see, e.g., Doorenbos et al., 1979). Additional indications about the uncertainty associated with the fast-track method are provided in Sect. 5.1.
3.2 Primary-equivalent trade matrix
For the correct identification of countries of origin of the crops traded internationally, the reconstruction of a primary-equivalent trade matrix, Meq, is necessary (Kastner et al., 2011). This is defined as
where Mp is the trade matrix of any root product, Mdp is the trade matrix of the derived products (dp), and fp and fv are the product fraction and value fraction which convert the derived products into a root-product equivalent quantity. The summation is extended to all derived products which originate from the same root product and, in the case of a multi-step supply chain, Eq. (5) is applied iteratively until reaching a root product that is also a primary crop. The product fraction, fp, is defined as the weight of a derived product obtained from a weight unit of input product. For example, a weight unit of nuts with shells leads to fp (<1) tons of shelled nuts. The value fraction, fv, is the market value of the derived product divided by the aggregated market value of all derived products resulting from a ton of input product. For example, in a production process of wheat flour there are other economically valuable by-products (e.g., wheat germs to feed animals); hence, the value of wheat flour constitutes only a portion (i.e., the value fraction) of the total value generated by the process. Product fractions and value fractions used in the CWASI database are time- and space-invariant and are taken from Mekonnen and Hoekstra (2010a, b), as well as the root products and the full supply chains of the considered commodities.
3.3 Supply-side unit water footprint of primary crops
The country supply of a primary crop results from the sum of local production and imports, where imports may occur from producing or non-producing countries, the latter case testifying a re-export of goods produced elsewhere. Therefore, the unit water footprint of supply, uWFs, is proportionally contributed by local production and by trade, specifying the relative contribution of every country from which the goods originated from, considering re-exports and the processing of goods, if necessary. For each primary-equivalent crop and each year, we can define a column vector, S, containing the supply of all countries as rows. This vector is calculated as the sum of the production vector, P, and of the imports obtained from the bilateral trade matrix Meq, where Meq(i,j) identifies the trade flow from i to j as
where I is a column vector of ones (i.e., a summation vector) and is the trade matrix transposed. Hence, the uWFs of a country depend both on the domestic uWFp (through P) and on the uWFp of the origin countries, where the product is produced.
In order to trace the actual origin of the country's supply, namely tracing its origin back to the country where it was produced, we adopt the approach proposed by Kastner et al. (2011). First, we define a matrix R, where each element R(i,j) is the quantity of supply in country i that is produced in country j. A first approximation of R can be based on reported flows only and is equal to the sum of a diagonal matrix with elements of the P vector on the diagonal, i.e., diag(P), and the transposed trade matrix, . However, this approximation misses the fact that exporting countries may obtain the exported products not only from local production, but also from import. To account for this fact, a matrix of export shares, X, can be defined as
where X(i,j) is the share of country j's supply that is exported to country i. The term diag(S−1) denotes a diagonal matrix made up by the reciprocal elements of S. In turn, the imported and re-exported products may partly originate from local production and import, and so on, recursively. It has been shown by Miller and Blair (2009) that such a procedure converges to
where the R matrix identifies where the supply of each country originates from and I is the identity matrix. For further details and exemplification, see Kastner et al. (2011).
By knowing the uWFp of the primary crop in such countries, we can now define the unit water footprint of supply in country c and year t of the primary product p, i.e., , as
The evaluation of uWFs corresponds to a weighted average of the uWFp values, where the weights are the actual fractions of supply, S, traced back to their origins. Equation (9) is valid for every primary crop p and year t, considering that trade matrices, production vectors, and uWFp values change from year to year. It is worth noticing that because the trade matrices are available from 1986 only, the uWFs can be built from that year only.
3.4 Unit water footprint of processed crops in time
Processed crops are based on the processing of root products, which are available as a country's supply. The time-varying unit water footprint thus depends on that of the root product and on the conversion factors (i.e., Hoekstra et al., 2011),
where is the unit water footprint of the processed crop (or derived product, dp); is the unit water footprint of supply of the root product from which it derives (p); c and t are country and year, respectively; fp is the product fraction; and fv is the value fraction of the processed crop (see Sect. 3.2). The method takes into account the temporal variability associated with both the crop production, through the fast-track method applied to the primary crop, and the evolution of trade, through the Kastner's method applied to the crop supply; the method does not include water inputs for processing of goods. When supply chains are formed by multiple steps, for example in the case of bread, made with flour, made in turn with wheat, Eq. (10) is applied routinely at each step. Within the CWASI dataset, the longest supply chain is made of four steps, leading to final products such as refined sugar or chocolate.
Equation (10) describes the unit water footprint without differentiating between production and supply. This is because the absence of FAOSTAT data of production of most processed crops (FAO, 2020a) hinders the application of the Kastner's method (Sect. 3.3) and thus an explicit accounting of countries of origin of trade, as in the case of primary crops. However, the trade of processed crops is implicitly taken into account in the procedure, thanks to the use of the primary-equivalent trade matrix (Eq. 5), which serves to compute the uWFs of the primary crop. For the very few derived products without indication of the root product (Mekonnen and Hoekstra, 2010a), an association is made which is based on logical considerations (such as “Figs dried” deriving from “Figs”) or on similitudes of products. The “Sugar” products (raw sugar, refined sugar, etc.) were also missing the root product, likely due to a lack of information. For these products, we have traced back the root product to the product most largely available as country supply (either “Sugar beet” or “Sugar cane”).
3.5 Unit water footprint of animal-based commodities
Animal-based commodities considered in FAOSTAT belong to three groups: “Live animals”, “Livestock primary”, and “Livestock processed” (FAO, 2020b, c, d). Products of the first group are given in heads, which have been converted to tons according to FAO conversion factors (FAO, 2013). The missing conversion values for some countries have been assigned with an average value of the same or similar animals. Products of the second group are here considered to be primary products, while live animals are only included in trade data. Due to the lack of reliable data about country-specific animal diets and their temporal variability as well as the lack of detailed trade matrices of feed crops, we do not currently provide a time-dependent unit water footprint for the animal-based commodities. Similarly, we do not provide a supply-side uWF. Nevertheless, we include these products in the present database adopting the country-specific values of unit water footprint provided by WaterStat (Mekonnen and Hoekstra, 2010b). These values take into account the feed–animal–commodity global supply chain, considering locally produced and imported feed (Mekonnen and Hoekstra, 2012), but are only available as time-averaged values over the period 1996–2005. Here data are generically referenced to the year 2000 and are arranged consistently with the rest of the CWASI database.
4.1 Water footprint and VWT data
The water footprint of agricultural production in a country and year is obtained by multiplying the production data (FAO, 2019a, 2020a, b, c, d), expressed in metric tons, by the corresponding (commodity, country, year) unit water footprint, considering the unit water footprint of production, , in the case of primary crops. A problem arises when a country was not a producer in the 1996–2005 decade; thus it does not have an associated value in the WaterStat database. In such a case, the uWF in the closest producing country within a 10∘ distance is taken; if no producing countries are found (e.g., in the case of remote islands or small producing areas), then the global average weighted by production is used. In the case of countries having experienced political discontinuity, for example belonging to a larger country before the years 1996–2005 considered in the WaterStat database (e.g., USSR), the reference value of uWF required in Eq. (4) is computed as a production-weighted average of the values of countries belonging to the union and available in WaterStat. This average value is then used to reconstruct the annual uWF from 1961 up to the year of the disaggregation. After converting the agricultural production into water volumes, the overall water footprint of production is obtained by summing across all commodities. Care must be used to avoid double accounting of water footprints of primary and derived goods. For this reason, only primary products must be considered in aggregated production data. In particular, when dealing with animal-based commodities, one should avoid the inclusion of both livestock and the corresponding products as well as the crops used to feed the livestock. Primary, or single-accounting, products to be included in the sum are indicated in the Appendix, in Table A1.
Computation of the supply-side unit water footprint of goods enables the fast computation of the water footprint associated with the consumption of commodities, under the hypothesis that consumption (and export) shares the same mix of local and imported goods with the country's supply. The water footprint of consumption in a country and year can thus be obtained by multiplying the consumed quantity of each good by the unit water footprint of supply, uWFs (per commodity, country, and year), and then summing across all commodities. In this case, there are no double-accounting issues. For commodities occasionally missing their uWF values, the last available value or the uWFp can be used instead.
The virtual water trade is obtained by multiplying trade data (FAO, 2019b), expressed in metric tons, by the unit water footprint of supply, , of the exporting country. Thanks to the new definition of supply-side unit water footprint, this computation allows one to take into account the origin of goods, which are traced back to their origin countries along the supply chain. In the few cases of goods exported from countries not having an associated uWF (less than 1 % of all existing links, mainly from minor countries or remote islands), the global average uWF of supply is used, weighted by all countries' exports. Virtual water trade associated with animal-based commodities is given for the year 2000 only, consistently with their unit water footprint, with the uWFp used for the conversion, since a uWF is not available for these commodities yet.
4.2 The uWF index
In the Results section, the (volumetric) water footprint and the virtual water trade are summed across different commodities, and the overall trends are assessed in time. However, the unit water footprint of different commodities cannot be summed across commodities but only for one commodity at a time. To overcome such a problem, an appropriate index is constructed analogously to economic indices aggregating prices of different commodities, such as the agriculture producer price index (in FAOSTAT) calculated with the Laspeyres approach. The index is built as the inverse ratio between the WF of production (m3) of all commodities (i) in all countries (c) in the year 2000 and the WF obtained with the same quantities (year 2000) but with uWF in year t, i.e.,
In such a way, I(t) expresses the variation in uWF across all agricultural commodities, weighted by the productions in the year 2000, . A similar index as in Eq. (11) can also be built referring to categories of goods by aggregating only the commodities belonging to one single category.
The importance of considering a time-dependent unit water footprint is highlighted in Fig. 3, which shows the temporal trends of the global average uWF of production of some commodities (other major crops are shown in Tuninetti et al., 2017). The global average is computed by weighting the uWFp of each country by the country production of the crop. The relevance of the temporal change is evident for example for wheat, with values ranging from 4000 to 1200 m3 t−1 in the considered period. The values considered in WaterStat refer to the period T=1996–2005, highlighted by a grey shade in Fig. 3: it is clear that the average value in such a reference period is scarcely representative of the whole period considered in the present dataset. It is thus very important to consider the temporal variability of unit water footprint, especially in analyses spanning long periods or periods different than the years 1996–2005.
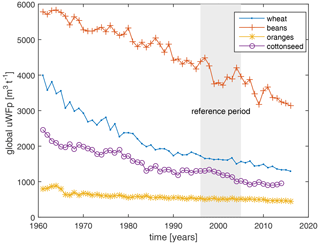
Figure 3Production-weighted global uWFp along the period 1961–2016 for wheat, beans, oranges, and cotton.
The temporal variation in the uWF of production of crops is marked all over the world. If compared to the values averaged over the period 1996–2005 (as in the WaterStat database), the uWFp values at the beginning and at the end of the considered period are very different. Figure 4 shows the relative change of the uWFp of wheat in 1961 and 2016 with respect to the 1996–2005 average. The variation is quite uniform worldwide with improvements (decreases in uWF) in both periods which are consistent with the period lengths. Extreme variations have occurred in China (largest improvement from 1961) and in African countries, showing large improvements in time, but also occasional worsening due to unstable socio-economic conditions. It should be noticed that a few countries worldwide do not produce wheat or miss FAOSTAT or WaterStat data: in such cases, countries are left in white.
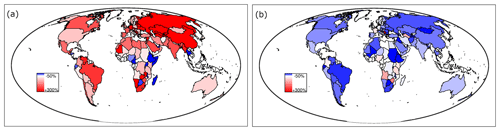
Figure 4Relative change in the uWFp of wheat in 1961 (a) and 2016 (b) with respect to the average in 1996–2005, using identical color ranges: red (blue) colors identify higher (lower) values and color intensity scales with change values. (Maps are created with MATLAB®R14 software, Mapping Toolbox v.2.0.3.)
A comparison between the uWF of production and supply of primary crops is very informative. Figure 5 highlights the absolute difference for wheat and soybean with red indicating countries where the uWFs is smaller than uWFp and green for the opposite. The more intense the red color, the more efficient the crop import in saving global water resources because the imported crops are produced with lower uWF than the local uWF of production. This is the case for several African countries, some South American ones, and Thailand for wheat and several South Asian countries for soybeans. Conversely, the more intense the green, the more efficient the global production, compared to imports. The extreme case of non-producing (but importing) countries is highlighted by bold contours. This is observed in several Far East countries for wheat and by most African countries for soybeans.
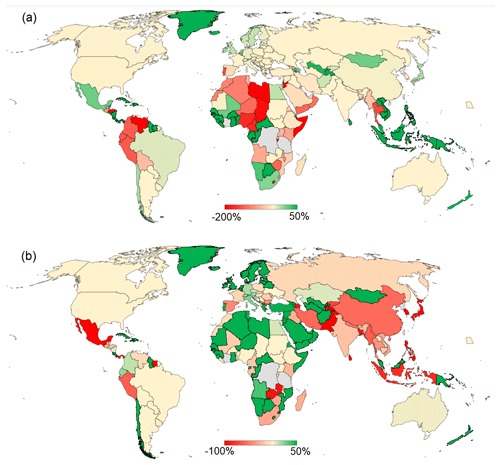
Figure 5Percentage difference between the uWF of production and supply of wheat (a) and soybean (b) in the year 2016, calculated as the difference between uWFs and uWFp, normalized by uWFs. Bold green countries do not produce the crop; hence they only have a supply-side uWF. (Maps are created with Microsoft Power Map for Excel, ©Microsoft.)
Considering all commodities together, the analysis of temporal evolution requires the use of a uWF index, which is applied to the uWF of production and uses the agricultural production of the year 2000 as weight (Eq. 11). The index is shown in Fig. 6 (left) and decreases monotonically in time, being at +60 % in 1961 and −10 % in 2016. The trend is less marked than in Fig. 3 because all commodities, and not only wheat, are being considered in the index, including those not having a uWF varying in time (e.g., animal-based products, as made explicit in Fig. 6, right). If one should include the temporal evolution of animal-based commodities, the temporal variation in the index would be more marked. The uWF index built by category of commodities, shown in Fig. 6 (right), allows one to find similarities and differences. In time, the uWF of production of cereals has improved constantly up to the 1990s. Then, after a period of stagnation, it has improved constantly again in the last 15 years. A similar dynamic, even if less regular, is observed in the seeds/oil category. Fruits and vegetables show a lower range of variability without stagnation in the period 1990–2000. Luxury foods show the only increasing dynamic observed in the last decade, dictated by coffee and cocoa beans, while non-edible goods show a more recent improvement, with the decrease in uWFp starting only in the mid-1970s and concluding the period with a small increase, mostly associated with natural rubber.
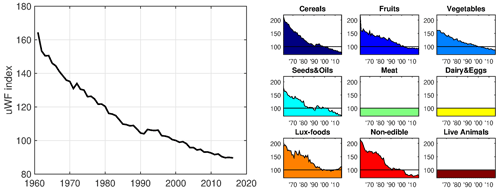
Figure 6Temporal variability of uWF indexes weighted with agricultural production (solid) and export (with dots) in the year 2000, aggregated across all goods (left) and (right) split into the nine categories of goods.
The time-varying uWF in the CWASI database is used to assess the temporal evolution of virtual water trade across the years, considering the contribution of different categories of goods. Figure 7 updates previous versions published in the literature (e.g., Konar el at., 2011; Carr el at., 2013; Tuninetti et al., 2017) by introducing the temporal variability of the uWF of crop-based goods and expanding the number of considered crops. Total VWT has increased from about 900 to almost 2400 km3 yr−1 in the considered period. Major categories are cereals, luxury food, and seeds/oils, followed by vegetables and meat. All categories show an increase in associated VWT, with non-edible goods showing the minimum increase (32 %) and seeds/oils showing the largest increase (more than 3-fold). The relative contribution of each category has changed in time, with the most relevant change shown by cereals, having decreased their contribution from 32 % to 21 % of total virtual water trade. The growth of animal-based products is remarkable, but it should be specified that it only reflects the increased trade quantity without considering the temporal variability of uWF.
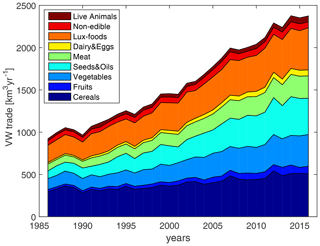
Figure 7Global virtual water trade (as derived from export data) from 1961 to 2016 considering the nine categories of goods from Fig. 2.
5.1 Uncertainties and limitations
Despite the large amount of information and the many improvements provided with the CWASI database, the data uncertainty and a few cautions are worth being mentioned. The time-varying unit water footprints of crops and crop-based commodities are estimated with a simplified method (the fast-track method) that has been thoroughly assessed before applying it widely. For example, the fast-track estimates of unit water footprint were compared to the results of a complete model based on a daily soil water balance fed by year-specific hydro-climatic variables, and the errors were found to be within a 10 % range (Tuninetti et al., 2017). The uncertainty introduced in the unit water footprint estimates with the fast-track method is also lower or comparable to the model uncertainty associated with the water footprint assessment, verified by a comparison with the WaterStat values (see Tuninetti et al., 2017).
The fast-track method, initially applied to four crops (wheat, maize, rice, and soybeans), has been extended in the CWASI database to a large set of primary products, including cereals, fruits, vegetables, seeds, luxury food, and non-edibles. The extension is justified by the fact that similar error ranges are expected in all crops, because water stress affects the evapotranspiration of different crops in a similar way, the only difference being the phases of the growing periods affected by water stress and the crop coefficients describing the plant water requirements. Water stress is assumed not to affect irrigated crops, implying that actual evapotranspiration matches the crop maximum evapotranspiration in irrigated conditions. Uncertainty associated with the fast-track method has been sparsely checked on other crops than the first four, and the range of errors found in Tuninetti et al. (2017) has been confirmed. Considering the hypothesis of a long-term average actual evapotranspiration of crops, we suggest using single-year data of uWF with care, as well as WF and VWT. It is precautionary to consider single-year data in a temporal perspective, such as a trend analysis, or use a multi-year average to minimize the error and avoid misinterpretations of year-specific results.
A minor point of caution is related to the supply-side uWF, which averages a country's local production and import. This variable is the best estimate to be used in association with countries' export and consumption, unless more detailed information is available about the origin of the country's export or consumption. If local production or import should prevail, compared to the average country's supply, a more precise weighted average of unit water footprint will be enabled by such information.
Concerning the uWF of animal-based commodities, as well as their WF and the VWT, they are here reported for the year 2000 only, referring to the average over the years 1996–2005 in the WaterStat database. Where necessary, these values have been applied to production and trade occurring in different years (see Figs. 6 and 7), although caution with such applications should be exercised. This limitation can be overcome when reliable data on the country-specific feed composition and diet of animals will become available along the considered time period.
Data are available on the Zenodo repository at https://doi.org/10.5281/zenodo.4606794 (Tamea et al., 2020).
The globalization of water resources through the international trade of food and agricultural goods is a remarkable global environmental change of our times, and the scientific community is devoting great effort to study it. The quantification of the volumes of water involved in the production and trade of agricultural goods is a key tool to investigate the water–food–trade nexus issues. This study presents an open-source database specifically developed for this purpose. The main outcome of this study is the time-varying unit water footprint for the years 1961–2016 and the virtual water trade matrices for the years 1986–2016 of hundreds of commodities from the food and agricultural sector. The water footprint of production per commodity is also available annually in the period 1961–2016. The current database includes a total of almost 30 million data, half of them being elements of the trade matrices. The introduction of a supply-side estimate of the unit water footprint brings much more detail in the water footprint accounting. This is a new concept and a key tool in the expedited and accurate accounting of the virtual water trade and of the water footprint of consumption. The supply-side unit water footprint overcomes previous problems related to the non-consideration of re-export, and it also enables a more accurate assessment of virtual water trade, with the correct identification of countries of origin of traded goods.
The open-source database presented in this work aims to help the scientific community and policy makers to quantify and investigate the complex linkages between the global food system and water resource issues. Potential applications of the CWASI dataset range from supporting national-scale policies of water management as well as agricultural policies oriented to the optimization of water use or, ultimately, to provide indications for price formation or for trade agreements based on the efficient and sustainable use of water resources worldwide. The CWASI database is shared through the Zenodo online open-access repository (Tamea et al., 2020), and it is planned to be improved upon and updated in the future, capitalizing contributions from the overall scientific community.
Commodities included in the CWASI database are listed in Table A1, which shows the commodity name, the FAO code, the presence of data in different database variables (1: yes, 0: no, 1*: without temporal variability), the presence of trade data (1: yes, 0: no), the indication of primary items and the associated category. Countries considered in the CWASI database are listed in Table A2, which include the country name, the FAO code, the position in the CWASI vectors/matrices, the indication of reporting (1) or non-reporting (0) countries, and the presence of discontinuities in the considered period.
ST, MT, and IS analyzed the data, developed the codes, and organized the dataset. All authors designed the methods, and FL supervised the work, ST prepared the manuscript with contributions from all co-authors.
The authors declare that there is no competing interest.
The authors acknowledge the ERC-2014-CoG funding of the project “Coping with water scarcity in a globalized world” (CWASI). Information about the project, including data and results, can be found at the website https://www.watertofood.org/ (last access: 5 May 2021). Giuseppe Zaccaria is also acknowledged for early contributions to the data collection and organization. This research is dedicated to the memory of Tony Allan, who developed the virtual water concept and enabled a global approach to water resources.
This research has been supported by the European Research Council under the European Union's Horizon 2020 program (grant agreement no. 647473).
This paper was edited by David Carlson and reviewed by two anonymous referees.
Allan, J.: Virtual water: A strategic resource – Global solutions to regional deficits, Ground Water, 36, 545–546, 1998.
Carr, J. A., D'Odorico, P., Laio, F., and Ridolfi, L.: Recent history and geography of virtual water trade, PLoS ONE, 8, e55825, https://doi.org/10.1371/journal.pone.0055825, 2013.
Dalin, C., Konar, M., Hanasaki, N., Rinaldo, A., and Rodriguez-Iturbe, I.: Evolution of the global virtual water trade network, Proc. Natl. Acad. Sci. USA, 109, 5989–5994, https://doi.org/10.1073/pnas.1203176109, 2012.
D'Odorico, P., Carr, J., Dalin, C., Dell'Angelo, J., Konar, M., Laio, F., Ridolfi, L., Rosa, L., Suweis, S., Tamea, S., and Tuninetti, M.: Global virtual water trade and the hydrological cycle: patterns, drivers, and socio-environmental impacts, Environ. Res. Lett., 14, 053001, https://doi.org/10.1088/1748-9326/ab05f4, 2019.
Doorenbos, J., Kassam, A., and Bentvelsen, C.: Yield response to water, FAO Irrigation and Drainage Paper, Food and Agriculture Organization, 1979.
Duarte, R. and Yang, H.: Input–Output and Water: Introduction to the Special Issue, Econ. Syst. Res., 23, 341–351. https://doi.org/10.1080/09535314.2011.638277, 2011.
FAO: Technical conversion factors for agricultural commodities, Food and Agriculture Organization, available online at: http://www.fao.org/fileadmin/templates/ess/documents/methodology/tcf.pdf (last access: May 2021), 2013.
FAO: FAOSTAT database of crop production, yield and harvested areas, Food and Agriculture Organization, available online at: http://www.fao.org/faostat/en/#data/QC, last access: October 2019a.
FAO: FAOSTAT database of detailed trade matrices, Food and Agriculture Organization, available online at: http://www.fao.org/faostat/en/#data/TM, last access: October 2019b.
FAO: FAOSTAT database of processed crops production, Food and Agriculture Organization, available online at: http://www.fao.org/faostat/en/#data/QD, last access: January 2020a.
FAO: FAOSTAT database of livestock primary production, Food and Agriculture Organization, available online at: http://www.fao.org/faostat/en/#data/QL, last access: March 2020b.
FAO: FAOSTAT database of livestock processed production, Food and Agriculture Organization, available online at: http://www.fao.org/faostat/en/#data/QP, last access: March 2020c.
FAO: FAOSTAT database of live animals, Food and Agriculture Organization, available online at: http://www.fao.org/faostat/en/#data/QA, last access: March 2020d.
Feng, K., Chapagain, A., Suh, S., Pfister, S., and Hubacek, K: comparison of bottom-up and top-down approaches to calculating the water footprint of nations, Econ. Syst. Res., 23, 371–385, https://doi.org/10.1080/09535314.2011.638276, 2011.
Gehlhar, M.: Reconciling bilateral trade data for use in GTAP, GTAP Technical paper, 10, available at: https://docs.lib.purdue.edu/gtaptp/11/ (last access: May 2021), 1996.
Geschke, A. and Hadjikakou, M.: Virtual laboratories and MRIO analysis – an introduction, Econ. Syst. Res., 29, 143–157, https://doi.org/10.1080/09535314.2017.1318828, 2017.
Hoekstra, A.: Water footprint assessment: evolvement of a new research field, Water Resour. Manag., 31, 3061–3081, https://doi.org/10.1007/s11269-017-1618-5, 2017.
Hoekstra, A. and Chapagain, A.: Water footprint of nations: Water use by people as a function of their consumption pattern, Water Resour. Manag., 21, 35–48, https://doi.org/10.1007/s11269-006-9039-x, 2007.
Hoekstra, A. and Chapagain, A. K.: Globalization of water: Sharing the planet's freshwater resources, Blackwell Publishing, Oxford, UK, 2008.
Hoekstra, A., Chapagain, A., Aldaya, M., and Mekonnen, M.: The Water Footprint Assessment Manual: Setting the Global Standard, Earthscan, London, 2011.
Hubacek, K., Feng, K.: Comparing apples and oranges: Some confusion about using and interpreting physical trade matrices versus multi-regional input–output analysis, Land Use Policy, 50, 194–201, https://doi.org/10.1016/j.landusepol.2015.09.022, 2016.
ISO: Environmental management – Water footprint (ISO Standard no. 14046), International Organization for Standardization, Geneva, Switzerland, 2014.
Kastner, T., Kastner, M., and Nonhebel, S.: Tracing distant environmental impacts of agricultural products from a consumer perspective, Ecol. Econ., 70, 1032–1040, https://doi.org/10.1016/j.ecolecon.2011.01.012, 2011.
Konar, M., Dalin, C., Suweis, S., Hanasaki, N., Rinaldo, A., and Rodriguez-Iturbe, I.: Water for food: The global virtual water trade network, Water Resour. Res., 47, W05520, https://doi.org/10.1029/2010WR010307, 2011.
Lenzen, M., Moran, D., Kanemoto, K., Geschke, A.: Building EORA: a multi-region Input–Output database at high country and sector resolution, Econ. Syst. Res., 25, 20–49, https://doi.org/10.1080/09535314.2013.769938, 2013.
Mekonnen, M. and Hoekstra, A.: The green, blue and grey water footprint of crops and derived crop products, Value of Water Research Report Series, no. 47, UNESCO-IHE Delft, the Netherlands, 2010a.
Mekonnen, M. and Hoekstra, A.: The green, blue and grey water footprint of farm animals and animal products, Value of Water Research Report Series, no. 48, UNESCO-IHE Delft, the Netherlands, 2010b.
Mekonnen, M. and Hoekstra, A.: A global assessment of the water footprint of farm animal products, Ecosystems, 15, 401–415, https://doi.org/10.1007/s10021-011-9517-8, 2012.
Miller, R. E. and Blair, P. D.: Input–Output Analysis: Foundations and Extensions, Cambridge University Press, 2009.
Pfister, S., Bayer, P., Koehler, A., and Hellweg, S.: Environmental impacts of water use in global crop production: hotspots and trade-offs with land use, Environ. Sci. Technol., 45, 5761–5768, https://doi.org/10.1021/es1041755, 2011.
Pfister, S. and Bayer, P.: Monthly water stress: spatially and temporally explicit consumptive water footprint of global crop production, J. Clean. Prod., 73, 52–62, https://doi.org/10.1016/j.jclepro.2013.11.031, 2014.
Pfister, S., Vionnet, S., Levova, T., and Humbert, S.: Ecoinvent 3: assessing water use in LCA and facilitating water footprinting, Int. J. Life Cycle Ass., 21, 1349–1360, https://doi.org/10.1007/s11367-015-0937-0, 2016.
SEI: A vision for Trase: 2016–2020, A report on TRASE (Transparency for Sustainable Economies), Stockholm Environment Institute and Global Canopy Programme, available online at: https://trase.earth/ (last access: May 2021), 2019.
Stadler, K., Wood, R., Bulavskaya, T., Södersten, C. J., Simas, M., Schmidt, S., Usubiaga, A., Acosta-Fernández, J., Kuenen, J., Bruckner, M., Giljum, S., Lutter, S., Merciai, S., Schmidt, J. H., Theurl, M. C., Plutzar, C., Kastner, T., Eisenmenger, N., Erb, K. H., Arjan de Koning, A., and Tukker, A., and Giljum, S.: EXIOBASE 3: Developing a time series of detailed environmentally extended multi-regional input–output tables, J. Ind. Ecol., 22, 502–515, https://doi.org/10.1111/jiec.12715, 2018.
Tamea, S., Tuninetti, M., Soligno, I., and Laio, F.: CWASI database: virtual water trade and water footprint of agricultural products (1961–2016), Zenodo repository, Dataset, last access: 6 May 2021, https://doi.org/10.5281/zenodo.4606794, 2020.
Tukker, A. and Dietzenbacher, E.: Global Multiregional Input-Output frameworks: an introduction and outlook, Econ. Syst. Res., 25, 1–19, https://doi.org/10.1080/09535314.2012.761179, 2013.
Tuninetti, M., Tamea, S., D'Odorico, P., Laio, F., and Ridolfi, L.: Global sensitivity of high-resolution estimates of crop water footprint, Water Resour. Res., 51, 8257–8272, https://doi.org/10.1002/2015WR017148, 2015.
Tuninetti, M., Tamea, S., Laio, F., and Ridolfi, L.: A Fast Track approach to deal with the temporal dimension of crop water footprint, Environ. Res. Lett., 12, 074010, https://doi.org/10.1088/1748-9326/aa6b09, 2017.
- Abstract
- Introduction
- Data and preliminary arrangements
- Unit water footprint
- Virtual water trade and water footprint indicators
- Results
- Data availability
- Conclusions
- Appendix A: Commodities and countries in the CWASI database
- Author contributions
- Competing interests
- Acknowledgements
- Financial support
- Review statement
- References
- Abstract
- Introduction
- Data and preliminary arrangements
- Unit water footprint
- Virtual water trade and water footprint indicators
- Results
- Data availability
- Conclusions
- Appendix A: Commodities and countries in the CWASI database
- Author contributions
- Competing interests
- Acknowledgements
- Financial support
- Review statement
- References