the Creative Commons Attribution 4.0 License.
the Creative Commons Attribution 4.0 License.
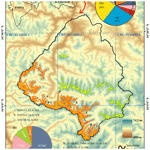
Temporal inventory of glaciers in the Suru sub-basin, western Himalaya: impacts of regional climate variability
Aparna Shukla
Siddhi Garg
Manish Mehta
Vinit Kumar
Uma Kant Shukla
The importance of updated knowledge about the glacier extent and characteristics in the Himalaya cannot be overemphasized. Availability of precise glacier inventories in the latitudinally diverse western Himalayan region is particularly crucial. In this study we have created an inventory of the Suru sub-basin in the western Himalaya for the year 2017 using Landsat Operational Land Imager (OLI) data. Changes in glacier parameters have also been monitored from 1971 to 2017 using temporal satellite remote-sensing data and limited field observations. Inventory data show that the sub-basin has 252 glaciers covering 11 % of the basin, having an average slope of 25±6∘ (standard deviations have been italicized throughout the text) and dominantly north orientation. The average snow line altitude (SLA) of the basin is 5011±54 m a.s.l. with smaller (47 %) and cleaner (43 %) glaciers occupying the bulk area. Long-term climate data (1901–2017) show an increase in the mean annual temperature (Tmax and Tmin) of 0.77 ∘C (0.25 and 1.3 ∘C) in the sub-basin, driving the overall glacier variability in the region. Temporal analysis reveals a glacier shrinkage of %, an average retreat rate of 4.3±1.02 m a−1, debris increase of 62 % and a 22±60 m SLA increase in the past 46 years. This confirms their transitional response between the Karakoram and the Greater Himalayan Range (GHR) glaciers. Besides, glaciers in the sub-basin occupy two major ranges, the GHR and Ladakh Range (LR), and experience local climate variability, with the GHR glaciers exhibiting a warmer and wetter climate as compared to the LR glaciers. This variability manifests itself in the varied response of GHR and LR glaciers. While the GHR glaciers exhibit an overall rise in SLA (GHR: 49±69 m; LR: decrease of 18±50 m), the LR glaciers have deglaciated more (LR: 7 %; GHR: 6 %) with an enhanced accumulation of debris cover (LR: 73 %; GHR: 59 %). Inferences from this study reveal prevalence of glacier disintegration and overall degeneration, transition of clean ice to partially debris-covered glaciers, local climate variability and non-climatic (topographic and morphometric)-factor-induced heterogeneity in glacier response as the major processes operating in this region. The Shukla et al. (2019) dataset is accessible at https://doi.org/10.1594/PANGAEA.904131.
- Article
(14585 KB) - Full-text XML
-
Supplement
(930 KB) - BibTeX
- EndNote
The state of the Himalayan cryosphere has a bearing on multiple aspects of hydrology, climatology, the environment and sustenance of living organisms at large (Immerzeel et al., 2010; Miller et al., 2012). Being sensitive to the ongoing climate fluctuations, glaciers keep adjusting themselves, and these adaptations record the changing patterns in the global climate (Bolch et al., 2012). Any alteration in the glacier parameters would ultimately affect the hydrology of the region, thereby influencing the downstream communities (Kaser et al., 2010; Pritchard, 2017). Owing to these reasons, quantifying the mass loss over different Himalayan regions in the past years as well as ascertaining the present status of the cryosphere and how these changes are likely to affect the freshwater accessibility in the region is at the forefront of contemporary cryospheric research (Brun et al., 2017; Sakai and Fujita, 2017). This aptly triggered several regional (Kääb et al., 2012; Gardelle et al., 2013; Brun et al., 2017; Zhou et al., 2018; Azam et al., 2018; Maurer et al., 2019), local (Bhushan et al., 2018; Vijay and Braun, 2018) and glacier-specific studies (Dobhal et al., 2013; Bhattacharya et al., 2016; Azam et al., 2016) in the region. These studies at varying scales contribute towards solving the jigsaw puzzle of the Himalayan cryosphere. The regional-scale studies operate on a small scale for bringing out more comprehensive, holistic and synoptic spatio-temporal patterns of glacier response; the local-scale studies monitor glaciers at the basin or group level and offer more details on heterogenous behaviour and plausible reasons thereof. However, the glacier-specific studies, whether based on field, satellite or integrative information, are magnified versions of the local-scale studies and hold the potential to provide valuable insights into various morphological, topographic and local climate-induced controls on glacier evolution. Despite these efforts, data on the glacier variability and response remain incomplete, knowledge of the governing processes is still preliminary and the future-viability pathways of the Himalayan cryospheric components are uncertain.
Though the literature suggests a generalized mass loss scenario (except for the Karakoram region) over the Himalayan glaciers, disparities in rates and pace of shrinkage remain. Maurer et al. (2019) report an average mass wastage of −0.32 m w.e. a−1 for the Himalayan glaciers between 1975 and 2016. They suggest that the glaciers in the eastern Himalaya (−0.46 m w.e. a−1) have experienced slightly higher mass loss as compared to the western Himalaya (−0.45 m w.e. a−1), followed by the central Himalaya (−0.38 m w.e. a−1). However, considerable variability in the glacier behaviour exists within the western Himalaya (Scherler et al., 2011; Kääb et al., 2012; Vijay and Braun, 2017; Bhushan et al., 2018; Mölg et al., 2018). Studies suggest that the glaciers in the Karakoram Himalaya have either remained stable or gained mass in the last few decades (Kääb et al., 2015; Cogley, 2016), while a contrasting behaviour is observed for the Greater Himalayan Range (GHR) glaciers experiencing large-scale degeneration, with more than 65 % of glaciers retreating between 2000 and 2008 (Scherler et al., 2011). However, there are two views pertaining to the glaciers in the Trans-Himalayan range, with one suggesting their intermediate response between the Karakoram Himalaya and GHR (Chudley et al., 2017) and the other emphasizing their affinity either towards the GHR or the Karakoram Himalayan glaciers (Schmidt and Nuesser, 2017). Therefore, in order to add more data and build a complete understanding of the glacier response, particularly in the western Himalaya, more local-scale studies are necessary.
Complete and precise glacier inventories form the basic prerequisites not only for comprehensive glacier assessment but also for various hydrological and climate-modelling-related applications (Vaughan et al., 2013). Information on spatial coverage of glaciers in any region is a much valued dataset and holds paramount importance in the future assessment of glaciers. Errors in the glacier outlines may propagate and introduce higher uncertainties in the modelled outputs (Paul et al., 2017). Besides, results from modelling studies conducted over the same region but using different sources of glacier boundaries are rendered incomparable, constraining the evaluation of models and thus their future development. On the other hand, quality, accuracy and precision associated with glacier-mapping and outline delineation require dedicated efforts. Several past studies discuss the methods for and challenges associated with achieving an accurate glacier inventory and resolutions for the same (Paul et al., 2013, 2015, 2017). Thorough knowledge of glaciology and committed manual endeavour are two vital requirements in this regard. Realization of the above facts has resulted in several devoted attempts to prepare detailed glacier inventories at a global scale, such as the Randolph Glacier Inventory (RGI), Global Land Ice Measurements from Space (GLIMS) and recently the Chinese Glacier Inventory (CGI) and Glacier Area Mapping for Discharge from the Asian Mountains (GAMDAM) (Raup et al., 2007; Pfeffer et al., 2014; Shiyin et al., 2014; Nuimura et al., 2015). However, several issues related to gap areas, differences in mapping methods and skills of the analysts involved act as limitations and need further attention.
Considering the above, present work studies the glaciers in the Suru sub-basin (SSB), western Himalaya, Jammu and Kashmir. Primary objectives of this study include (1) presenting the inventory of recent glacier data (area, length, debris cover, snow line altitude, min and max elevation, slope, and aspect) in the SSB; (2) assessing the temporal changes for four epochs in the past 46 years; and (3) analysing the observed glacier response in relation to the regional climate trends, local climate variability and other factors (regional hypsometry, topographic characteristics, debris cover and geomorphic features). Several remote-sensing and field-based studies of a regional (Vijay and Braun, 2018), local (Bhushan et al., 2018; Kamp et al., 2011; Pandey et al., 2011; Shukla and Qadir, 2016; Rashid et al., 2017; Murtaza and Romshoo, 2015) and glacier-specific nature (Garg et al., 2018; 2019; Shukla et al., 2017) have been conducted for monitoring the response of the glaciers to climate change. Glaciological studies carried out in or adjacent to the SSB suggest increased shrinkage, slowdown and downwasting of the studied glaciers at variable rates (Kamp et al., 2011; Pandey et al., 2011; Shukla and Qadir, 2016; Bhushan et al., 2018). These studies also hint towards the possible role of topographic and morphometric factors as well as debris cover in glacier evolution, though confined to their own specific regions. Previous studies have also estimated the glacier statistics of the SSB and reported the total number of glaciers and the glacierized area to be 284 and 718.86 km2 (Sangewar and Shukla, 2009) and 110 and 156.61 km2 (SAC, 2016), respectively, while the RGI reports varying results by two groups of analysts (number of glaciers: 514 and 304 covering an area of 550 and 606 km2, respectively) for the year 2000.
Previous findings suggesting progressive degeneration of glaciers, apparent variation and discrepancies in inventory estimates and also the fact that the currently available glacier details for the sub-basin are nearly 20 years old mandate the recent and accurate assessment of the glaciers in the SSB and drive the present study.
The present study focuses on the glaciers of the SSB situated in the region of Jammu and Kashmir in the western Himalaya (Fig. 1). The geographic extent of the study area lies within a latitude and longitude of to N and to E, respectively.
Geographically, the sub-basin covers part of two major ranges, i.e. the GHR and LR, and shows the presence of the highest peaks of Nun (7135 m a.s.l.) and Kun (7077 m a.s.l.) in the GHR (Vittoz, 1954). The glaciers in these ranges have distinct morphology, with the larger ones located in the GHR and the smaller ones towards the LR (Fig. 1).
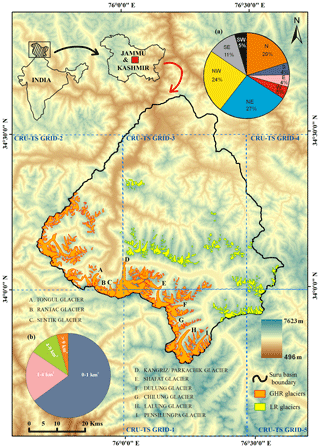
Figure 1Location map of the study area. The glaciers in the Suru sub-basin (SSB: black outline) are studied for their response towards the climatic conditions during the period 1971–2017. Blue rectangles with dashed outlines (GRID-1, 2, 3, 4 and 5) are the Climate Research Unit (CRU) time series (TS) 4.02 grids of dimension . (a) Pie chart inset showing orientation-wise percentage distribution of glaciers in the sub-basin. North (N), north-east (NE), north-west (NW), south (S), south-east (SE), south-west (SW), east (E) and west (W) represent the direction of the glaciers. (b) Pie chart inset showing size distribution of glaciers in the SSB. The glacier boundaries GHR (orange) and LR (yellow) are overlain on the Advanced Land Observing Satellite (ALOS) digital surface model (DSM).
The meltwater from these glaciers feeds the Suru River (tributary of the Indus River), which emerges from the Pensilungpa glacier (Fig. 2a) at an altitude of ∼4675 m a.s.l. The river flows further north for a distance of ∼24 km and takes a westward turn from Rangdum (∼4200 m a.s.l.). While flowing through this path, the Suru River is fed by some of the major glaciers of the GHR, namely Lalung, Dulung (Fig. 1), Chilung (Fig. 2b), Shafat (Fig. 2c, d), Kangriz/Parkachik (Fig. 2e), Sentik, Rantac (Fig. 2f), Tongul (Fig. 2g) and glacier no. 47 (Fig. 2h). Amongst these major glaciers, Kangriz forms the largest glacier in the SSB, covering an area of ∼53 km2 and descending down from the peaks of Nun and Kun (Garg et al., 2018). The Suru River continues to flow for a distance of nearly 54 km, and after crossing a mountain spur and the townships of Tongul, Panikhar and Sankoo, the river flows further north until it finally merges with the Indus River at Nurla (∼3028 m a.s.l.).
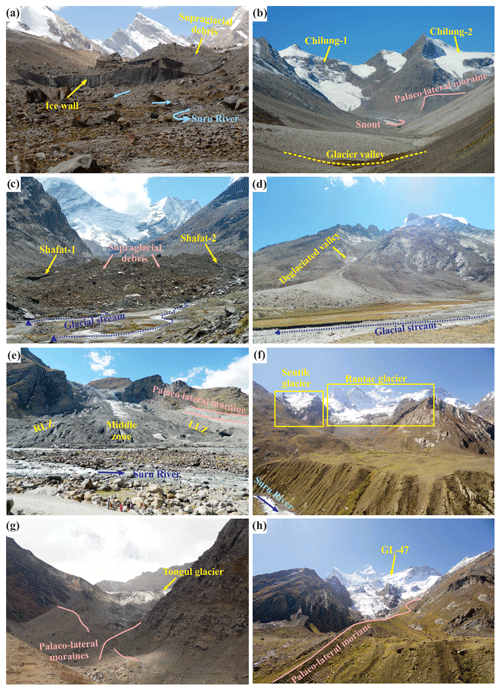
Figure 2Field photographs of some of the investigated glaciers in the study area captured during the field visits in September 2016 and 2017. Snouts of (a) Pensilungpa, (b) Chilung, (c) Shafat, (e) Kangriz, (f) Sentik and Rantac, (g) Tongul and (h) glacier no. 47. (d) Deglaciated valley near the Shafat glacier.
The westerlies are an important source of moisture in this region (Dimri, 2013) with a wide range of fluctuations in snowfall during winters. In the Padum valley, annual mean precipitation (snowfall) and temperature amount to nearly 2050 to 6840 mm and 4.3 ∘C, respectively (Raina and Kaul, 2011; http://en.climate-data.org, last access: 30 November 019). The long-term average annual temperature and precipitation have varied from 5.5 ∘C and 588.77 mm (Kargil) to −2.04 ∘C and 278.65 mm in Leh during the period 1901–2002 (IMD, 2015). However, in order to understand the long-term variability of climatic conditions in the SSB, we have utilized the Climate Research Unit (CRU) time series (TS) version 4.02 data during the period 1901–2017 (Fig. 3; Harris and Jones, 2018). Derived from this data, the annual mean temperature and precipitation of the SSB for the period 1901–2017 was 0.99±0.45 ∘C and 393±76 mm, respectively. (Standard deviations associated with the mean temperature and precipitation have been italicized throughout the text.)
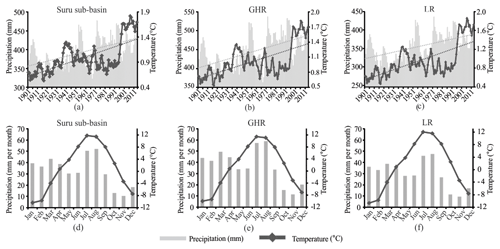
Figure 3Annual and seasonal variability in the climate data for the period 1901–2017. (a, b, c) Five-year moving average of the mean annual precipitation (mm) and temperature (∘C) recorded for five grids covering the glaciers in the entire SSB, GHR and LR (subregions), respectively, during the period 1901–2017. The dashed light and dark grey lines depict the respective trend lines for precipitation and temperature conditions during the period 1901–2017. (d, e, f) Monthly mean precipitation and temperature data for the entire SSB, GHR and LR (subregions), respectively, for the time period 1901–2017.
3.1 Datasets used
The study uses multi-sensor and multi-temporal satellite remote-sensing data for extracting the glacier parameters for four time periods: 1971/1977, 1994, 2000 and 2017. Details of these time periods are mentioned in Table 1. The study involves six Landsat level-1 terrain corrections (L1Ts), three strips of declassified Corona KH-4B and one Sentinel multispectral scene, downloaded from USGS Earth Explorer (https://earthexplorer.usgs.gov/, last access: 5 March 2020). Additionally, a global digital surface model (DSM) dataset utilizing the data acquired by the Panchromatic Remote-sensing Instrument for Stereo Mapping (PRISM) on board the Advanced Land Observing Satellite (ALOS) has also been incorporated (https://www.eorc.jaxa.jp/ALOS/en/aw3d30/, last access: 14 August 2019). ALOS World 3D comprises a fine-resolution DSM (approx. 5 m vertical accuracy). It is primarily used for delineating the basin boundary and extraction of the snow line altitude (SLA), elevation range, regional hypsometry and slope.
Table 1Detailed specifications of the satellite data utilized in the present study. GB: glacier boundaries; DC: debris cover.
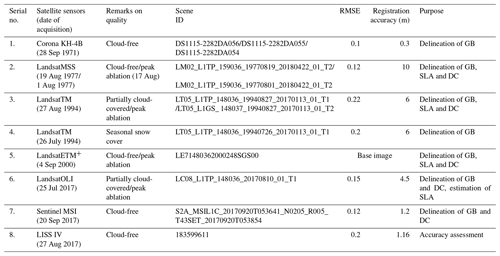
The aforementioned satellite images were acquired taking into consideration certain necessary prerequisites such as peak ablation months (July, August, and September), regional coverage and minimal snow and cloud cover for the accurate identification and demarcation of the glaciers. Only three Corona KH-4B strips were available for 1971, which covered the SSB partially, i.e. 40 % of the GHR and 57 % of the LR glaciers. Therefore, the rest of the glaciers were delineated using the Landsat Multispectral Scanner System (MSS) image of the year 1977 (Table 1). Similarly, some of the glaciers could not be mapped using the Landsat Thematic Mapper (TM) image of 27 August 1994 as the image was partially covered with clouds. Therefore, the 26 July 1994 image of the same sensor was used in order to delineate the boundaries of the cloud-covered glaciers.
Furthermore, long-term climate data have been obtained from CRU TS 4.02, which is a high-resolution gridded climate dataset obtained from the monthly meteorological observations collected at different weather stations of the world. In order to generate this long-term data, station anomalies from 1961 to 1990 are interpolated into 0.5∘ latitude and longitude grid cells (Harris and Jones, 2018). This dataset includes six independent climate variables (mean temperature, diurnal temperature range, precipitation, wet-day frequency, vapour pressure and cloud cover). However, in this study monthly mean, minimum and maximum temperature and precipitation data are taken into consideration.
3.2 Methodology adopted
The following section mentions the methods adopted for data extraction, analysis and uncertainty estimation.
3.2.1 Glacier-mapping and estimation of glacier parameters
Initially, the satellite images were co-registered by projective transformation with sub-pixel accuracy and a root mean square error (RMSE) of less than 1 m (Table 1), taking the Landsat Enhanced Thematic Mapper (ETM+) image and the ALOS DSM for reference. However, the Corona image was co-registered following a two-step approach: (1) projective transformation was performed using nearly 160–250 ground control points (GCPs) and (2) spline adjustment of the image strips (Bhambri et al., 2012). The glaciers were mapped using a hybrid approach, i.e. normalized-difference snow index (NDSI) for delineating snow–ice boundaries and manual digitization of the debris cover. Considering that not many changes would have occurred in the accumulation region, major modifications have been done in the boundaries below the equilibrium line altitude (ELA; Paul et al., 2017). The glacierets and tributary glaciers contributing to the main trunk are considered as a single glacier entity. The NDSI was applied to a reference image of Landsat ETM+ using an area threshold range of 0.55–0.6. A median filter of kernel size 3×3 was used to remove the noise and very small pixels. In this manner, glaciers covering a minimum area of 0.01 km2 have been mapped. However, some pixels of frozen water and shadowed regions were manually corrected. Thereafter, the debris-covered part of the glaciers was mapped manually by taking help from the slope and thermal characteristics of the glaciers. Furthermore, high-resolution imagery from Google Earth™ was also referred to for the accurate demarcation of the glaciers. Identification of the glacier terminus was done based on the presence of certain characteristic features at the snout such as ice wall, proglacial lakes and emergence of streams. The length of the glacier was measured along the central flow line (CFL) drawn from the bergschrund to the snout. Fluctuations in the snout position (i.e. retreat) of an individual glacier were estimated using the parallel line method, in which parallel strips of 50 m spacing are taken on both sides of the CFL. Thereafter, the average values of these strips intersecting the glacier boundaries were used to determine the frontal retreat of the glaciers (Shukla and Qadir, 2016; Garg et al., 2017a, b). The mean SLA estimated at the end of the ablation season can be effectively used as a reliable proxy for mass balance estimation for a hydrological year (Guo et al., 2014). The maximum spectral contrast between snow and ice in the SWIR and NIR bands helps in the delineation of the snow line separating the two facies. The same principle was used in this study to yield the snow line. Further, a 15 m buffer was created on both sides of the snow line to obtain the mean SLA. Other factors such as elevation (max and min), regional hypsometry and slope were extracted utilizing the ALOS DSM.
3.2.2 Analysis of climate variables
To ascertain the long-term climate trends in the sub-basin, mean annual temperature (min and max) and precipitation are derived by averaging the mean monthly data of the respective years. Furthermore, seasonal trends are also analysed for the winter (November–March) and summer (April–October) months. Moreover, the climate variables are assessed separately for the ∼46-year period (1971–2017), which is the study period of the present research. Further, the climate dataset was statistically analysed for five grids using the Mann–Kendall test to obtain the magnitude and significance of the trends (Table S2 in the Supplement). The magnitude of the trends in the time series data was determined using Sen's slope estimator (Sen, 1968). Quantitatively, the temperature and precipitation trends have been assessed here in absolute terms (determined from Sen's slope). The change in climate parameters (temperature and precipitation) was determined using the following formula:
where β is Sen's slope estimator, L is the length of the period, and M is the long-term mean.
These tests were performed with a confidence level S=0.1 (90 %), 0.05 (95 %) and 0.01 (99 %), which differed for both variables (Table S2). Spatial interpolation of climate data was achieved using the inverse distance weighted (IDW) algorithm. For this purpose, a total number of 15 CRU TS grids (in the vicinity of our study area) were taken so as to have an ample number of data points in order to achieve accurate results.
Further, in order to check data consistency, we have taken instrument data from the nearest stations of Kargil and Leh (due to the unavailability of meteorological stations in the SSB) and compared with the CRU-TS-derived data for the entire SSB during the 1901–2002 period (Fig. 4).
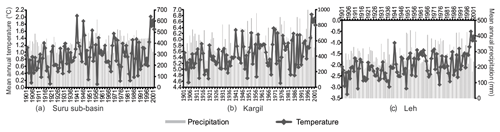
Figure 4Mean annual temperature and precipitation patterns of gridded data derived from the Climate Research Unit (CRU) time series (TS) in the (a) Suru sub-basin and data recorded by the Indian Meteorological Department (IMD) stations at (b) Kargil and (c) Leh.
The mean annual temperature pattern of the SSB shows a near-negative trend until 1937, with an increase thereafter. Similar trends have been observed for Kargil and Leh, despite their distant location from the SSB (areal distance of Kargil and Leh is ∼63 and 126 km, respectively from the centre of the SSB). However, it is worth mentioning that all the locations had attained a maximum mean annual temperature in 1999 (Suru: 2.02 ∘C; Kargil: 6.84 ∘C; Leh: −0.5 ∘C). We observe an almost similar trend in all the cases (Fig. 4), with an accelerated warming post-1995/1996. However, the magnitude varies, with a long-term mean annual temperature of 0.9, 5.5 and −2.04 ∘C observed in SSB, Kargil and Leh, respectively (Fig. 4). The possible reason for this difference in their magnitudes could possibly be attributed to their distinct geographical locations and difference in their nature, with the former being point data and the latter being the interpolated gridded data.
Furthermore, we have used the station data, obtained from the nearest available Indian Meteorological Department (IMD) sites, i.e. Kargil and Leh, and compared with their respective CRU TS data (mean annual temperature and precipitation).
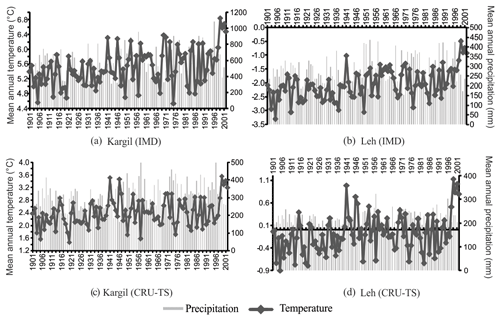
Figure 5Analysis of meteorological (mean annual temperature and precipitation) datasets derived from the Indian Meteorological Department (IMD) stations at (a) Kargil and (b) Leh and the respective (c) Kargil and (d) Leh gridded data obtained from the Climate Research Unit (CRU) time series (TS).
Though varying in magnitude, the climate data obtained from the IMD as well as CRU TS suggest almost similar trends of temperature and precipitation during the period 1901–2002 for both Kargil and Leh (Fig. 5). During this period, the annual mean temperature amounted to 5.5 ∘C (IMD) and 2.4 ∘C (CRU TS) in Kargil and −2.04 ∘C (IMD) and −0.09 ∘C (CRU TS) in Leh; the mean annual precipitation amounted to 589 mm (IMD) and 315 mm (CRU TS) in Kargil and 279 mm (IMD) and 216 mm (CRU TS) in Leh (Fig. 5). We observed that climatic variables show a lower magnitude in the case of the CRU TS as compared to the station data from IMD (except CRU-TS-derived temperature data recorded for Leh). The possible reason for this difference between CRU TS and station data can primarily be attributed to the difference in their nature, with former being point and the latter being gridded data (0.5∘ latitude and longitude grid cells). This analysis aptly brings out the bias in the CRU TS gridded data. Importantly, the comparison shows that the gridded data correctly bring out the temporal trends in meteorological data but differ with station data in magnitude (being lower than the station estimates). This helps us better appreciate the climate variations in the SSB as well since we learn that the reported temperature and precipitation changes are probably lower than the actual variations.
3.2.3 Uncertainty assessment
This study involves extraction of various glacial parameters utilizing satellite data with variable characteristics; hence, it is susceptible to uncertainties which may arise from various sources. These sources may be locational (LEs), interpretational (IEs), classification (CEs) or processing (PEs) errors (Racoviteanu et al., 2009; Shukla and Qadir, 2016). In our study, the LEs and PEs may have resulted on account of misregistration of the satellite images and inaccurate mapping, respectively. IEs and CEs would have been introduced due to the misinterpretation of glacier features during mapping. The former can be rectified by co-registration of the images and estimation of the sub-pixel co-registration RMSE (Table 1) as well as using standard statistical measures. However, the latter can be visually identified and corrected, but exact quantification can be difficult owing to a lack of reliable reference data (field data) in most cases. As a standard procedure for uncertainty estimation, glacier outlines are compared directly with the ground truth data as acquired using a differential global positioning system (DGPS) (Racoviteanuet al., 2008). In this study, a DGPS survey was conducted on the Pensilungpa and Kangriz glaciers at an error of less than 1 cm. Therefore, by comparing the snout position of Pensilungpa (2017) and Kangriz (2018) glaciers derived from the DGPS and Operational Land Imager (OLI) image, an accuracy of ±23 and ±1.4 m, respectively, was obtained. Furthermore, the frontal retreat estimated for the Kangriz glacier using the DGPS and OLI image is found to be 38.63±47.8 and 39.98±56.6 m, respectively, during the period 2017–2018. In this study, high-resolution Linear Imaging Self Scanner (LISS) IV imagery (spatial resolution of 5.8 m) is also used for validating the glacier-mapping results for the year 2017 (Table 1). Glaciers of varying dimensions and distribution of debris cover were selected for this purpose. The area- and length-mapping accuracy for these selected glacier boundaries (G-1, G-2, G-3, G-13, G-41, G-209, G-215, G-216, G-220, G-233) was found to be 3 % and 0.5 %, respectively.
The multi-temporal datasets were assessed for glacier length and area change uncertainty as per the methods given by Hall et al. (2003) and Granshaw and Fountain (2006). The following formulations (Hall et al., 2003) were used for estimation of the said parameters:
where a and b are the pixel resolution of image 1 and 2, respectively, and σ is the registration error. The terminus and areal uncertainty estimated are given in Table 2.
where x is the spatial resolution of the sensor.
Table 2Terminus and area change uncertainty associated with the satellite dataset as defined by Hall et al. (2003). UT: terminus uncertainty; UA: area change uncertainty; x: spatial resolution; σ: registration accuracy.
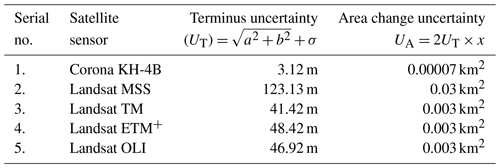
Area-mapping uncertainty was estimated using the buffer method, in which a buffer size equal to the registration error of the satellite image was taken into consideration (Bolch et al., 2012; Garg et al., 2017a, b). Using this method, the error was estimated to be 0.48, 27.2, 9.6 and 3.41 km2 for the 1971 (Corona), 1977 (MSS), 1994 (TM) and 2017 (OLI) images, respectively. Since the debris extents were delineated within the respective glacier boundaries, the proportionate errors are likely to have propagated in debris cover estimations, which were estimated accordingly (Garg et al., 2017b).
Uncertainty in SLA estimation needs to be reported in the X, Y and Z directions. In this context, error in the X and Y directions should be equal to the distance taken for creating the buffer on either side of the snow line demarcating the snow and ice facies. Since the buffer size taken in this study was 15 m, the error in the X and Y direction was considered to be ±15 m. However, uncertainty in the Z direction would be similar to the ALOS DSM, i.e. ±5 m.
The present study involved the creation of a glacier inventory for the year 2017 and the estimation of glacier (area, length, debris cover and SLA) parameters for four different time periods. For detailed insight, the variability of the glacier parameters has also been evaluated on a decadal scale, in which the total time period has been subdivided into three time frames: 1971–1994 (23 years), 1994–2000 (6 years) and 2000–2017 (17 years).
4.1 Basin statistics
The SSB covers an area of ∼4429 km2. In 1971, the sub-basin had around 240 glaciers, with 126 glaciers located in the GHR and 114 in the LR, which remained the same until 2000. However, a major disintegration of glaciers took place during the period 2000–2017, which resulted in the breakdown of about 12 glaciers into smaller glacierets. The recent (2017) distribution of the glaciers in the GHR and LR is 130 and 122, respectively (Table S1). The overall glacierized area is ∼11 %, with the size and length of the glaciers varying from 0.01 to 53.1 km2 and 0.15 to 16.34 km, respectively.
Within the sub-basin, the size range of glaciers in the GHR and LR varies from 0.01 (G-115) to 53.1 km2 (G-50) and 0.03 (G-155/165) to 6.73 km2 (G-209), respectively. Considering this, glaciers have been categorized as small (0–7 km2∕0–2 km), medium (7–15 km2∕2–7 km) and large (>15 km km). Based on the size distribution, small (comprising all the LR and some GHR glaciers), medium and large glaciers occupy 47 %, 15 % and 38 % of the glacierized sub-basin, respectively. Depending on the percentage of area occupied by the supraglacial debris out of the total glacier area, the glaciers have been categorized as clean (CGs: 0 %–25 %), partially debris-covered (PDGs: 25 %–50 %) and heavily debris-covered (HDGs: >50 %) glaciers. Categorization of the glaciers based on this criteria shows their proportion in the glacierized basin as 43 % (CGs), 40 % (PDGs) and 17 % (HDGs). A majority of the glaciers in the sub-basin are north-facing (N/NW/NE: 71 %), followed by south (S/SW/SE: 20 %), with very few oriented in other (E/W: 9 %) directions (Fig. 1a). The mean elevation of the glaciers in the SSB is 5134.8±225 m a.s.l., with an average elevation of 5020±146 and 5260±117 m a.s.l. in the GHR and LR, respectively. The mean slope of the glaciers is ∘ and varies from ∘ to ∘ in the GHR and LR, respectively, while the percentage distribution of the glaciers shows that nearly 80 % of the LR glaciers have a steeper slope (20–40∘) as compared to the GHR glaciers (57 %).
4.2 Area changes
The glaciated area reduced from 513±14 km2 (1971) to 481±3.4 km2 (2017), exhibiting an overall deglaciation of 32±9 km2 (6±0.02 %) during the period 1971–2017. The percentage of area loss of the individual glaciers ranges between 0.8 % (G-50; Parkachik glacier) and 45 % (G-81), with a majority of the glaciers undergoing an area loss in the range of 6 %–12 % during the period 1971–2017 (Fig. 6a).
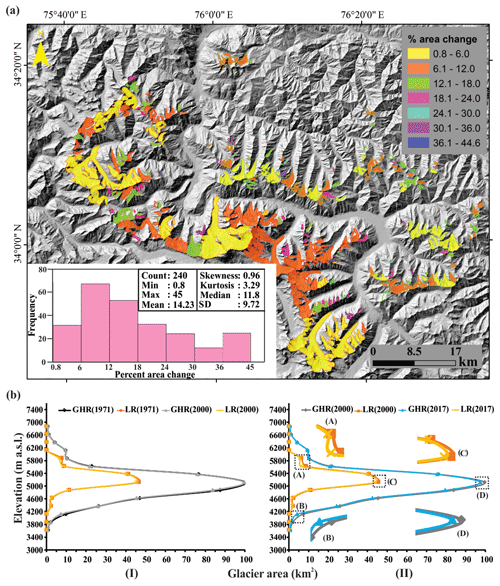
Figure 6(a) Percentage of area loss of the glaciers in the SSB during the period 1971–2017. Frequency distribution histogram depicting that the majority of the glaciers have undergone an area loss in the range of 6 %–12 %. (b) Hypsometric distribution of glacier area in the GHR and LR regions during the periods (I) 1971–2000 and (II) 2000–2017. (A), (B), (C) and (D) insets in (II) show the significant change in area at different elevation ranges of the GHR and LR glaciers.
The results show that the highest pace of deglaciation is observed in the periods 1994–2000 (0.95±0.005 km2 a−1) and 2000–2017 (0.86±0.0002 km2 a−1), followed by 1971–1994 (0.5±0.001 km2 a−1) (Fig. S1a in the Supplement). Within the SSB, glaciers in the LR exhibit higher deglaciation (7±7.2 %) as compared to GHR (6±2 %) during the period 1971–2017. Apart from deglaciation, G-50 also showed an increase in glacier area during the period 1994–2000; however, this increase was insignificant.
4.3 Length changes
Fluctuations in the glacier snout have been estimated during the period 1971–2017, and it is observed that nearly all the glaciers have retreated during the said period; however the retreat rates vary considerably. The overall average retreat rate of the glaciers is observed to be 4.3±1.02 m a−1 during the period 1971–2017. The percentage of length change of the glaciers ranges between 0.9 % and 47 %, with the majority of the glaciers retreating in the range of 6 %–14 % during the period 1971–2017 (Fig. 7).
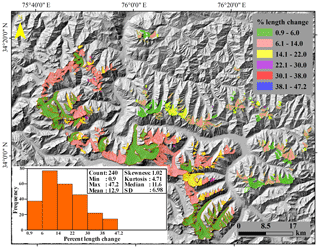
Figure 7Percentage of length change of the glaciers in the SSB during the period 1971–2017. Frequency distribution histogram showing that majority of the glaciers have undergone length change in the range of 6 %–14 %.
Decadal observations reveal the highest rate of retreat between 1994 and 2000 (7.37±8.6 m a−1) followed by 2000–2017 (4.66±1.04 m a−1), and the lowest between 1971 and 1994 (3.22±2.3 m a−1) (Fig. S1b). Moreover, the average retreat rate in the GHR and LR glaciers was observed to be 5.4±1.04 and 3.3±1.04 m a−1, respectively, during the period 1971–2017. The retreat rate of individual glaciers varied from 0.72±1.02 m a−1 (G-114) to 28.92±1.02 m a−1 (G-7, i.e. Dulung glacier) during the period 1971–2017. Furthermore, the Kangriz glacier (G-50) also showed advancement during the period 1994–2000 by 5.23±8.6 m a−1.
4.4 Debris cover changes
The results show an overall increase in debris cover extent of 62 % ( km2) in the SSB glaciers during the period 1971–2017. Decadal variations exhibit the maximum increase in the debris cover of approximately 19±0.00004 km2 (24 %) between 2000 and 2017 followed by an increase of 13±0.0001 km2 (20 %) and 5±0.0001 km2 (9 %) between 1994 and 2000 and between 1971 and 1994, respectively (Fig. S1c). However, GHR and LR glaciers show an overall increase of debris cover extent of 59 % and 73 %, respectively, during the entire study period, i.e. 1971–2017.
4.5 SLA variations
The mean SLA shows an average increase of 22±60 m during the period 1977–2017. On the decadal scale, SLA variations showed the highest increase (161±59 m) between 1994 and 2000 with a considerably lower increase (8±59 m) between 1977 and 1994 and a decrease (150±60 m) between 2000 and 2017. Amongst the four time periods (1977, 1994, 2000 and 2017) used for mean SLA estimation, the highest SLA is noted in 2000 (5158±65 m a.s.l.) and the lowest in 1977 (4988±65 m a.s.l.) (Fig. S1d).
During the period 1977–2017, the average SLA of the LR glaciers is observed to be relatively higher (5155±7 m a.s.l.) as compared to the GHR glaciers (4962±9 m a.s.l.). In contrast, an overall rise in mean SLA was noted in the GHR (49±69 m), while a decrease was observed in the LR glaciers (18±45 m) during the time frame of 1977–2017.
The present study reports detailed temporal inventory data of the glaciers in the SSB considering multiple glacier parameters, evaluates the ensuing changes for ascertaining the status of the glaciers and relates them to climate variability and other inherent terrain characteristics. The results suggest an overall degeneration of the glaciers with pronounced spatial and temporal heterogeneity in response.
5.1 Glacier variability in the Suru sub-basin: a comparative evaluation
Basin statistics reveal that in the year 2000, the SSB comprised 240 glaciers covering an area of approximately 496 km2. However, these figures differ considerably from the previously reported studies in this particular sub-basin, with the total number of glaciers and the glacierized area varying from 284 and 718.86 km2 (Sangewar and Shukla, 2009) to 110 and 156.61 km2 (SAC, 2016), respectively. In contrast, the glacierized area is found to be less than – yet comparable with – the RGI boundaries (550.88 km2). Furthermore, debris cover distribution of the glaciers in 2000 is observed to be ∼16 % in the present study, which is almost half of that reported in the RGI (30 %). Variability in these figures is possibly due to the differences in the mapping techniques, thereby increasing the risk of systematic error. Moreover, due to the involvement of different analysts in the latter, the results may more likely suffer from random errors.
Results from this study reveal an overall deglaciation of the glaciers in the SSB at an annual rate of % during the period 1971–2017. This quantum of area loss is comparatively less than the average annual rate of 0.4 % reported in the western Himalaya (Table S3). However, our results are comparable with Birajdar et al. (2014), Chand and Sharma (2015) and Patel et al. (2018) and differ considerably with other studies in the western Himalaya (Table S3). Period-wise deglaciation varied from 0.1±0.0007 to 0.2±0.005 % a−1 between 1971 and 2000 as well as between 2000 and 2017, respectively. This result is in line with the recent findings by Maurer et al. (2019), who suggest a higher average mass loss post-2000 (−0.43 m w.e. a−1), which is almost double the rate reported between 1975 and 2000 (−0.22 m w.e. a−1) for the entire Himalaya.
A comparison of the deglaciation rates of the glaciers within the western Himalayan region reveals considerable heterogeneity therein (Table S3). It is observed that the Karakoram Himalayan glaciers in particular had been losing area until 2000 at an average rate of 0.09 % a−1, with an increase in area thereafter of ∼0.05 % a−1 (Liu et al., 2006; Minora et al., 2013; Bhambri et al., 2013). However, glaciers in the GHR and Trans-Himalayan range have been deglaciating with higher average annual rates of 0.4 % a−1 and 0.6 % a−1, respectively, during the period 1962–2016 (Kulkarni et al., 2007, 2011; Rai et al., 2013; Chand and Sharma, 2015; Mir et al., 2017; Schmidt and Nuesser, 2017; Chudley et al., 2017; Patel et al., 2018; Das and Sharma, 2018). In contrast to these studies, deglaciation rates in the SSB, which comprises glaciers in the GHR as well as the LR, have varied from 0.1 % a−1 (GHR) to 0.2 % a−1 (LR) (present study). These results evidently depict that the response of the SSB glaciers is transitional between the Karakoram Himalayan and GHR glaciers. Period-wise area loss of the glaciers in the Himalayan region suggests maximum average deglaciation of eastern (0.49 % a−1) followed by central (0.36 % a−1) and western (0.35 % a−1) Himalayan glaciers before 2000. Contrarily, after 2000, the central Himalayan glaciers deglaciated at the maximum rate (0.52 % a−1) followed by western (0.46 % a−1) and eastern (0.44 % a−1) Himalayan glaciers (Fig. 8). Though these rates reflect the possible trend of deglaciation in the Himalayan terrain, any conclusion drawn would be biased due to insufficient data, particularly in the eastern and central Himalaya.
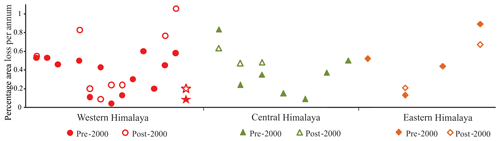
Figure 8Annual rate of area loss of glaciers (in per cent) in three major sections of the Himalaya before and after 2000. Details of the same have been mentioned in Table S3. Results from the present study have been star-marked in the western Himalaya.
In this study, we found an overall average retreat rate of 4.3±1.02 m a−1 during the period 1971–2017. However, the average retreat rates of seven glaciers in the SSB reported by Kamp et al. (2011) are found to be nearly twice (24 m a−1) of those found in this study (10 m a−1). The comparatively higher retreat rates in the former might be due to the consideration of different time frames. The average retreat rates in other basins of the western Himalaya are also found to be higher (7.8 m a−1) in the Doda valley (Shukla and Qadir, 2016), 8.4 m a−1 in the Liddar valley (Murtaza and Romshoo, 2015), 15.5 m a−1 in the Chandra–Bhaga basin (Pandey and Venkataraman, 2013) and 19 m a−1 in the Baspa basin (Mir et al., 2017). These results show lower average retreat rates of the glaciers in the SSB as compared to the other studies in the western Himalaya.
The observed average retreat rates between 2000 and 2017 (4.6±1.02 m a−1) are found to be nearly twice of those noted between 1971 and 2000 (2±1.7 m a−1). Similar higher retreat rates post-2000 have been reported in the Tista basin (Raina, 2009), Doda valley (Shukla and Qadir, 2016), Chandra–Bhaga basin (Pandey and Venkataraman, 2013) and Zanskar basin (Pandey et al., 2011). However, these studies may not sufficiently draw a generalized picture of glacier recession in the Himalayan region.
5.2 Spatio-temporal variability in the climate data
Climatic fluctuations play a crucial role in understanding glacier variability. In this regard, the CRU TS 4.02 dataset helped in delineating the long-term fluctuations in the temperature and precipitation records.
5.2.1 Basin-wide climate variability
During an entire duration of 116 years, i.e. from 1901 to 2017, the maximum mean annual temperature is observed in 2016 (3.23 ∘C) and minimum in 1957 (−0.51 ∘C). The mean annual temperature shows an almost uniform trend until 1996, with a pronounced rise thereafter until 2005/2006 (Fig. 3a, b, c). The globally averaged combined land and ocean surface temperature data of the 1983–2012 period are considered to be the warmest 30-year period in the last 1400 years (IPCC, 2013). This unprecedented rate of warming is primarily attributed to the rapid scale of industrialization, increase in regional population and anthropogenic activities prevalent during this time period (Bajracharya et al., 2008; IPCC, 2013). Thus, one of the probable reasons for this sudden increase in temperature pattern is possibly due to the greenhouse effect from enhanced emission of black carbon in this region (by 61 %) from 1991 to 2001. Evidence of incessant increase in temperature during the 1990s has also been observed (through chronology of the Himalayan pine) from the contemporaneous surge in tree growth rate (Singh and Yadav, 2000). In fact, 50 % of the years since 1970 have experienced considerably high solar irradiance and warm phases of El Niño–Southern Oscillation, which is possibly one of the reasons for the considerable rise in temperature throughout the Himalaya (Shekhar et al., 2017). The maximum mean annual precipitation is noted in 2015 (615 mm) and the minimum in 1946 (244 mm). However, the mean annual precipitation followed a similar trend until 1946, with an increase thereafter (Fig. 3a, b, c). Besides these general trends in temperature and precipitation, an overall absolute increase in the mean annual temperature (Tmax and Tmin) and precipitation data has been noted as 0.77 ∘C (0.25 and 1.3 ∘C) and 158 mm, respectively, during the period 1901–2017. These observations suggest an enhanced increase in Tmin by nearly 5 times as compared to the Tmax along with a simultaneous increase in the precipitation during the period 1901–2017.
Seasonal variations reveal monthly mean temperatures and precipitation of 6.7 ∘C and 1071 mm during summer (April–October) and −6.9 ∘C and 890 mm during winter (November–March) recorded during the 1901–2017 period. The maximum monthly mean temperature and precipitation have been observed in July (11.8 ∘C∕50.4 mm) and August (11.4 ∘C∕52 mm) during the period 1901–2017, suggesting that they were the warmest and wettest months, while January is noted to be the coldest (−10.4 ∘C) and November (10.3 mm) to be the driest month in the duration of 116 years (Fig. 3d, e, f). Summer and winter mean annual temperatures and precipitation have increased significantly by an average of 0.74∕1.28 ∘C and 85∕72 mm, respectively, during the period 1901–2017. These values reveal a relatively higher rise in winter average temperature in contrast to the summer. However, enhanced increase in Tmin (1.8 ∘C) during winter and Tmax (0.78 ∘C) during summer has also been observed during the 1901–2017 time period. The relatively higher rise in the winter temperature (particularly Tmin) and precipitation possibly suggests that the form of precipitation might have changed from solid to liquid during this particular time span. A similar increase in the winter temperature has also been reported from the NW Himalaya during the 20th century (Bhutiyani et al., 2007).
In contrast to the long-term climate trends, we have also analysed the climate data for the study period, i.e. 1971–2017. An overall increase in the average temperature (0.3 ∘C), Tmax (0.45 ∘C), Tmin (1.02 ∘C) and precipitation (213 mm) is observed. Meanwhile, an enhanced increase in winter Tmin (1.7 ∘C) and summer Tmax (0.45 ∘C) is observed. These findings aptly indicate the important role of winter Tmin and summer Tmax in the SSB.
5.2.2 Local climate variability
Apart from these generalized climatic variations, grid-wise analysis of the meteorological parameters reveals the existence of local climate variability within the sub-basin (Figs. 3, 9).
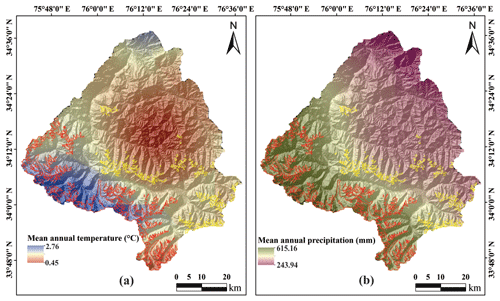
Figure 9Spatial variation in meteorological data recorded for 15 grids in the SSB during the period 1901–2017. Map showing the long-term mean annual (a) temperature (∘C) and (b) precipitation (mm) data within the sub-basin, suggesting the existence of significant local climate variability in the region. Glacier boundaries are shown as GHR (red) and LR (yellow).
Observations indicate that the glaciers covered in grid 4 have been experiencing warmer climatic regimes with a maximum annual mean temperature of 1.69 ∘C as compared to the other glaciers in the region (grid 2: 1.4 ∘C; grid 5: 0.74 ∘C; grid 1: 0.65 ∘C; grid 3: 0.45 ∘C). Spatial variability in annual mean precipitation data reveals that grid 2 (448 mm) and grid 1 (442 mm) experienced wetter climate as compared to grid 4 (383 mm), grid 3 (373 mm) and the minimum in grid 5 (318 mm). These observations suggest that GHR glaciers have been experiencing a warmer and wetter climate (1.03 ∘C∕445 mm) as compared to the LR glaciers (0.96 ∘C∕358 mm) (Fig. 3e, f). These observations clearly show that local climate variability does exist in the basin for the entire duration of 116 years (Fig. 9).
5.3 Glacier changes: impact of climatic and other plausible factors
The alterations in the climatic conditions discussed in Sect. 5.2 would in turn influence the glacier parameters, but these would vary with time. This section correlates the climatic and other factors (elevation range, regional hypsometry, slope, aspect and proglacial lakes) with the variations in the glacier parameters.
5.3.1 Impact of climatic factors
An overall degenerating pattern of the glaciers in the SSB is observed during the period 1971–2017, with deglaciation of 32±9 km2 (6±0.02 %). In the same duration, the glaciers have also retreated by an average 199±46.9 m (retreat rate: 4.3±1.02 m a−1) along with an increase in the debris cover of ∼62 %. The observed overall degeneration of the glaciers possibly resulted from the warming of climatic conditions during this particular time frame. The conspicuous degeneration of these glaciers might have led to an increased melting of the glacier surface, which in turn would have unveiled the englacial debris cover and increased its coverage in the ablation zone (Shukla et al., 2009; Scherler et al., 2011). A more enhanced degeneration of the glaciers has been noted between 2000 and 2017 (0.85±0.005 km2 a−1) than between 1971 and 2000 (0.59±0.005 km2 a−1). Moreover, nearly 12 glaciers have shown disintegration into glacierets after 2000. These observations may be attributed to the relatively higher annual mean temperature (1.68 ∘C) during the former as compared to the period 1971–2000 (0.89 ∘C). Concomitant to the maximum glacier degeneration during the period 2000–2017, debris cover extent has also increased more (24 %) as compared to 1971–2000 (16 %). The enhanced degeneration of the glaciers between 2000 and 2017 might have facilitated an increase in the distribution of supraglacial debris cover. A transition from CGs to PDGs has also been noticed, which resulted from an increase in the debris cover percentage over nearly 99 glaciers. A conversion from PDGs to HDGs (39) and from CGs to HDGs (2) has also occurred. Furthermore, most of these transitions occurred between 2000 and 2017, which confirms the maximum degeneration of the glaciers during this particular period.
It is observed in our study that smaller glaciers have deglaciated more (4.13 %) than the medium- (1.08 %) and larger-sized (1.03 %) glaciers during the period 1971–2017 (Fig. S2). This result depicts an enhanced sensitivity of the smaller glaciers to climate change (Bhambri et al., 2011; Basnett et al., 2013; Ali et al., 2017). A similar pattern of glacier degeneration is noted between 1971 and 2000, with smaller glaciers deglaciating more (5 %) as compared to the medium-sized (3 %) and larger (1 %) ones. However between 2000 and 2017, medium glaciers showed slightly greater degeneration (3.9 %) as compared to the smaller (3.7 %) and larger ones (1.5 %). We have also observed the maximum length change for smaller glaciers (8 %) in comparison to medium (5 %) and large glaciers (3 %). These results indicate that the snout retreats are commonly associated with small- and medium-sized glaciers (Mayewski et al., 1980).
Table 3Coefficients of determination (r) between respective meteorological (temperature and precipitation) data and observed glacier parameters in the Greater Himalayan Range (GHR) and Ladakh Range (LR) at 90 % confidence. Tavg, Tmin and Tmax are monthly mean, monthly mean minimum and monthly mean maximum temperatures, and Ppt is monthly mean precipitation at a different point in time (1971, 1994, 2000 and 2017).
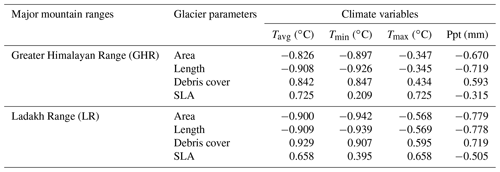
Temporal and spatial variations in SLAs are an indicator of ELAs, which in turn provide direct evidence related to the change in climatic conditions (Hanshaw and Bookhagen, 2014). SLAs are amongst the dynamic glacier parameters that alter seasonally and annually, indicating their direct dependency on climatic factors such as temperature and precipitation. In the present study, the mean SLA has gone up by an average 22±60 m during the period 1977–2017. This rise in SLA is synchronous with the increase in mean annual temperature of 0.43 ∘C. Moreover, the maximum rise in SLA between 1994 and 2000 is contemporaneous with the rise in temperature of 0.64 ∘C during this time period.
Further, in order to understand the regional heterogeneity in glacier response within the sub-basin, parameters of the GHR and LR glaciers are analysed separately for four different time periods and correlated with the climatic variables. The LR glaciers were found to have deglaciated more (7.2 %) as compared to the GHR glaciers (5.9 %). Similarly, more debris cover is found to have accumulated over the LR (73 %) glaciers as compared to the GHR (59 %) glaciers between 1971 and 2017. This result shows that the relatively cleaner (LR) glaciers tend to deglaciate more and accumulate more debris as compared to the debris-covered and partially debris-covered glaciers (GHR glaciers) (Bolch et al., 2008; Scherler et al., 2011). Moreover, the increase in mean annual temperature in the LR (0.3 ∘C) is slightly greater than in the GHR (0.25 ∘C) during the period 1971–2017, thus exhibiting a positive correlation with deglaciation and debris cover distribution in these regions. We also observed that the glacier area, length and debris cover extent of the LR glaciers show a good correlation with winter Tmin and average precipitation as compared to the GHR glaciers (Table 3). This shows that both temperature and precipitation influence the degeneration of the glaciers and in turn affect the supraglacial debris cover. It is believed that winter precipitation primarily influences the accumulation of snow on the glaciers and hence acts as an essential determinant of glacier health (Mir et al., 2017). Furthermore, the negative correlation between glacier area and precipitation in this study possibly indicates the major role of increased winter temperature and precipitation, which might have decreased the accumulation of snow, thereby decreasing the overall glacier area. The average SLA for LR glaciers is observed to be higher as compared to the GHR glaciers. However, a relatively higher rise in SLA is observed for the GHR in contrast to the LR glaciers. Moreover, the mean SLA of the GHR glaciers shows a good positive correlation with summer Tmax as compared to the LR glaciers and a negative correlation with precipitation in the respective year (Table 3). Considering these observations, it appears that a general rise in SLA can be attributed to regional climatic warming, while that of individual SLA variation in glaciers may be related to their unique topography (Shukla and Qadir, 2016).
From this analysis, it is quite evident that climatic factors directly influence the glacier response. Furthermore, summer Tmax has a stronger influence on SLA, while glacier area, length and debris cover are predominantly influenced by the winter Tmin in the sub-basin.
5.3.2 Impact of other factors
In addition to the climate variables, other factors such as hypsometry, maximum elevation, altitude range, slope, aspect and proglacial lakes also influence the response of an individual glacier.
Glacier hypsometry is a measure of mass distribution over varying altitudes. It is affected by the mean SLA of the glaciers to a greater extent because if a large portion of the glacier has an elevation equivalent to the SLA, then even a slight alteration in SLA might significantly change the ablation and accumulation zones (Rivera et al., 2011; Garg et al., 2017b).
In this study, we observed that GHR and LR glaciers have nearly 45 % and 10 % of their area, respectively, at an elevation similar to the SLA. This suggests that GHR glaciers are more susceptible to retreat as compared to the LR glaciers as a larger portion of the former is at the SLA. Moreover, the hypsometric distribution of glacier area in the GHR and LR of the SSB reveals maximum area change post-2000 (Fig. 6b). In this regard, while GHR glaciers have undergone relatively higher area loss (21 %) at lower elevation (3800–4200 m a.s.l.), the LR glaciers lost maximum area (30 %) at much higher elevation (5600–5900 m a.s.l.) ranges (Fig. 6b). Additionally, a significant area loss has also been observed for both GHR (6 %) and LR (7 %) glaciers at their mean elevations post-2000 (Fig. 6b).
Elevation plays an important role in understanding the accumulation pattern at higher altitudes and ablation at lower altitudes. The general perception is that the glaciers situated at relatively higher elevation are subjected to a greater amount of precipitation and hence are susceptible to less deglaciation or even mass gain (Pandey and Venkataraman, 2013). Similarly, we have also noticed that the glaciers extending to comparatively higher maximum elevation experience minimum retreat (10 %) and exhibit a higher percentage of deglaciation (33 %) as compared to the glaciers with a lower maximum elevation (retreat: 15 %; deglaciation: 20 %) (Fig. 10a).
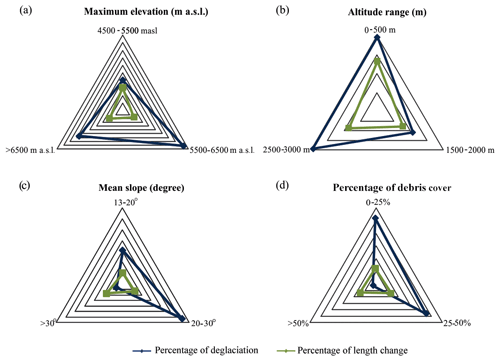
Figure 10Differential degeneration of the glaciers during the period 1971–2017 with variability in non-climatic factors. (a) Percentage of deglaciation and length change of the glaciers at different ranges of maximum elevation, (b) altitude range, (c) mean slope and (d) percentage of debris cover.
Moreover, our study shows that the glaciers with a lower altitude range have retreated and deglaciated more (13 % and 20 %, respectively) as compared to their counterparts (Fig. 10b). These observations indicate that glaciers with a higher maximum elevation and altitudinal range are subjected to less retreat and undergo greater deglaciation.
Slope is another important factor which has a major role in the sustenance of the glacier as accumulation of ice is facilitated by a gentler bedrock topography (DeBeer and Sharp, 2009; Patel et al., 2018). It is observed that glaciers with steep slopes (30–40∘) have retreated more (17 %), however with minimum deglaciation (7 %) during the period 1971–2017 (Fig. 10c). Similar results with steeper glaciers exhibiting minimum deglaciation have been reported in the Parbati, Chandra and Miyar basins (Venkatesh et al., 2012; Patel et al., 2018). However, these results differ from Pandey and Venkataraman (2013) and Garg et al. (2017b), likely due to the differing average size (25±33.78 and 17±33.2 km2; present study: 2±5.7 km2) and slope (5–20∘ and 12–26∘; present study: 13–41∘) of the glaciers used in these studies.
The presence of supraglacial debris cover influences glacier processes. Depending on thickness, debris cover may either enhance or retard the ablation process (Scherler et al., 2011). In this study, we observed that clean glaciers have undergone maximum deglaciation (52 %) as compared to the partially (46 %) and heavily debris-covered glaciers (2 %). However, they have all retreated almost similarly (12 % to 14 %), with a slightly higher retreat of partially debris-covered glaciers (Fig. 10d). The aspect and orientation of glaciers provide information regarding the duration for which they are exposed to incoming solar radiation. Since the south-facing glaciers are subjected to a longer duration of exposure to solar radiation as compared to the north-facing glaciers, they are prone to greater deglaciation and retreat (Deota et al., 2011). Here, it is observed that the glaciers with a northerly aspect (north, north-east, north-west) have undergone maximum deglaciation as compared to their counterparts. However, a majority (71 %) of the glaciers have northerly aspect so any inferences drawn in this respect would be biased. It is worth stating that most of the south-facing slopes in the basin are devoid of glaciers but show a presence of relict glacier valleys which would have been glaciated in the past. At present only 48 south-facing glaciers (south, south-east, south-west), with an average size of 1±1.9 km2, exist in the SSB.
Similarly, the glacier changes are also influenced by the presence of certain features such as glacial (proglacial or supraglacial) lakes or differential distribution of supraglacial debris cover. The presence of proglacial or supraglacial lakes significantly enhances the rate of glacier degeneration by increasing the melting processes (Sakai, 2012; Basnett et al., 2013). As per our results, the highest average retreat rate (∼31 m a−1) is observed for glacier G-4 (Dulung glacier). Although it is a debris-free glacier, it shows the highest retreat rates. Furthermore, a moraine-dammed lake is observed at the snout of this glacier and has continuously increased its size from 0.15 km2 in 1977 to 0.56 km2 in 2017. This significant increase in the size of the moraine-dammed lake has possibly influenced the enhanced retreat rate of the glacier.
Temporal inventory data for glaciers of the Suru sub-basin in the western Himalaya are available at https://doi.org/10.1594/PANGAEA.904131 (Shukla et al., 2019).
The major inferences drawn from the study include the following:
-
The sub-basin comprised 252 glaciers, covering an area of 481.32±3.41 km2 (11 % of the glacierized area) in 2017. Major disintegration of the glaciers occurred after 2000, with breakdown of 12 glaciers into glacierets. Small (47 %) and clean (43 %) glaciers cover the maximum glacierized area of the sub-basin. Topographic parameters reveal that the majority of the glaciers are north-facing, and the mean elevation and slope of the glaciers are 5134.8±225 m a.s.l. and ∘, respectively.
-
Variability in glacier parameters reveals an overall degeneration of the glaciers during the period 1971–2017, with deglaciation of approximately 0.13±0.0004 % a−1 along with an increase in the debris cover of 37±0.002 km2 (∼62 %). Meanwhile, the glaciers have shown an average retreat rate of nearly 4.3±1.02 m a−1, with SLA exhibiting an overall increase of an average 22±60 m.
-
Long-term meteorological records during the period 1901–2017 exhibit an overall increase in the temperature (Tmin: 1.3 ∘C; Tmax: 0.25 ∘C; Tavg: 0.77 ∘C) and precipitation (158 mm) trends. Both temperature and precipitation gradients influence the changes in glacier parameters; however, winter Tmin strongly influences the glacier area, length and debris cover, while summer Tmax influences the SLA. Spatial patterns in climate parameter changes reveal the existence of local climate variability in the sub-basin, with progressively warmer (1.03 ∘C) and wetter (445 mm) climatic regimes for glaciers hosted in the GHR as compared to the LR (0.96 ∘C and 358 mm).
-
The inherent local climate variability in the sub-basin has influenced the behaviour of the glaciers in the GHR and LR. It has been observed that LR glaciers have been shrinking faster (area loss: 7 %) and accumulating more debris cover (debris increase: 73 %) as compared to the GHR glaciers (6 % and 59 %) during the period 1971–2017. The GHR glaciers have, however, experienced a greater increase in SLA (220±121 m) in comparison to the LR ones (91±56 m) during the period 1977–2000, with a decrease thereafter.
The results presented here show the transitional response of the glaciers in the SSB between the Karakoram Himalayan and GHR glaciers. The study also confirms the possible influence of factors other than climate such as glacier size, regional hypsometry, elevation range, slope, aspect and presence of proglacial lakes in the observed heterogenous response of the glaciers. Therefore, these factors need to be accounted for in more detail in future for a complete understanding of the observed glacier changes and response.
The supplement related to this article is available online at: https://doi.org/10.5194/essd-12-1245-2020-supplement.
AS and SG conceived the idea and led the writing of the manuscript. AS structured the study. SG performed the temporal analysis of the data. MM and VK helped in the field investigation of the glaciers. UKS helped in the interpretation of the results and finalizing the structure of the article. All the authors helped in the interpretation of the results and contributed towards the final form of the paper.
The authors declare that they have no conflict of interest.
The authors are grateful to the director of the Wadia Institute of Himalayan Geology, Dehradun, for providing all the research facilities and support for the successful completion of this work. We wish to convey our sincere thanks to the anonymous reviewers for detailed reviews and constructive comments, which greatly helped to improve the previous version of the paper. We are thankful to Prasad Gogineni (handling topical editor) and Jens Klump (handling chief editor) for their thoughtful suggestions regarding the manuscript. We also appreciate the efforts of the entire editorial team of Earth System Science Data (ESSD) for the timely processing of the article. Aparna Shukla acknowledges the secretory of the Ministry of Earth Science (MoES), New Delhi, India, for providing requisite support.
This paper was edited by Prasad Gogineni and reviewed by two anonymous referees.
Ali, I., Shukla, A., and Romshoo, S. A.: Assessing linkages between spatial facies changes and dimensional variations of glaciers in the upper Indus Basin, western Himalaya, Geomorphology, 284, 115–129, https://doi.org/10.1016/j.geomorph.2017.01.005, 2017.
Azam, M. F., Ramanathan, A. L., Wagnon, P., Vincent, C., Linda, A., Berthier, E., Sharma, P., Mandal, A., Angchuk, T., Singh, V. B., and Pottakkal, J. G.: Meteorological conditions, seasonal and annual mass balances of Chhota Shigri Glacier, western Himalaya, India, Ann. Glaciol., 57, 328–338, https://doi.org/10.3189/2016AoG71A570, 2016.
Azam, M. F., Wagnon, P., Berthier, E., Vincent, C., Fujita, K., and Kargel, J. F.: Review of the status and mass changes of Himalayan-Karakoram glaciers, J. Glaciol., 64, 61–74, https://doi.org/10.1017/jog.2017.86, 2018.
Bajracharya, S. R., Mool, P. K., and Shrestha, B. R.: Global climate change and melting of Himalayan glaciers. Melting glaciers and rising sea levels: Impacts and implications, edited by: Prabha Shastri Ranade, The Icfai's University Press, India, 28–46, 2008.
Basnett, S., Kulkarni, A. V., and Bolch, T.: The influence of debris cover and glacial lakes on the recession of glaciers in Sikkim Himalaya, India, J. Glaciol., 59, 1035–1046, https://doi.org/10.3189/2013JoG12J184, 2013.
Bhambri, R., Bolch, T., Chaujar, R. K., and Kulshreshtha, S. C.: Glacier changes in the Garhwal Himalaya, India, from 1968 to 2006 based on remote sensing, J. Glaciol., 57, 543–556, https://doi.org/10.3189/002214311796905604, 2011.
Bhambri, R., Bolch, T., and Chaujar, R. K.: Frontal recession of Gangotri Glacier, Garhwal Himalayas, from 1965 to 2006, measured through high-resolution remote sensing data, Curr. Sci. India, 102, 489–494, 2012.
Bhambri, R., Bolch, T., Kawishwar, P., Dobhal, D. P., Srivastava, D., and Pratap, B.: Heterogeneity in glacier response in the upper Shyok valley, northeast Karakoram, The Cryosphere, 7, 1385–1398, https://doi.org/10.5194/tc-7-1385-2013, 2013.
Bhattacharya, A., Bolch, Mukherjee, K., Pieczonka, T., Kropacek, J., and Buchroithner, M.: Overall recession and mass budget of Gangotri Glacier, Garhwal Himalayas, from 1965 to 2015 using remote sensing data, J. Glaciol., 62, 1115–1133, https://doi.org/10.1017/jog.2016.96, 2016.
Bhushan, S., Syed, T. H., Arendt, A. A., Kulkarni, A. V., and Sinha, D.: Assessing controls on mass budget and surface velocity variations of glaciers in Western Himalaya, Sci. Rep., 8, 8885, https://doi.org/10.1038/s41598-018-27014-y, 2018.
Bhutiyani, M. R., Kale, V. S., and Pawar, N. J: Long term trends in maximum, minimum and mean annual air temperature across the Northwestern Himalaya during the twentieth century, Climate Change, 85, 159–177, https://doi.org/10.1007/s10584-006-9196-1, 2007.
Birajdar, F., Venkataraman, G., Bahuguna, I., and Samant, H.: A revised glacier inventory of Bhaga Basin Himachal Pradesh, India: current status and recent glacier variations, ISPRS Annals of Photogrammetry, Remote Sensing and Spatial Information Sciences, II-8, 37–43, https://doi.org/10.5194/isprsannals-ii-8-37-2014, 2014.
Bolch, T., Buchroithner, M., Pieczonka, T., and Kunert, A.: Planimetric and Volumetric Glacier Changes in the Khumbu Himal, Nepal, Since 1962 Using Corona, Landsat TM and ASTER Data, Journal of Glaciology, 54, 592–600, https://doi.org/10.3189/002214308786570782, 2008.
Bolch, T., Kulkarni, A., Kääb, A., Huggel, C., Paul, F., Cogley, J. G., Frey, H., Kargel, J. S., Fujita, K., Scheel, M., Bajracharya, S., and Stoffel, M.: The State and Fate of Himalayan Glaciers, Science, 336, 310–314, https://doi.org/10.1126/science.1215828, 2012.
Brun, F., Berthier, E., Wagnon, P., Kääb, A., and Treichler, D.: A spatially resolved estimate of High Mountain Asia glacier mass balances from 2000 to 2006, Nat. Geosci., 10, 668–673, https://doi.org/10.1038/NGEO2999, 2017.
Chand, P. and Sharma, M. C.: Glacier changes in Ravi basin, North-Western Himalaya (India) during the last four decades (1971–2010/13), Global Planet. Change, 135, 133–147, https://doi.org/10.1016/j.gloplacha.2015.10.013, 2015.
Chudley, T. R., Miles, E. S., and Willis, I. C.: Glacier characteristics and retreat between 1991 and 2014 in the Ladakh Range, Jammu and Kashmir, Remote Sens. Lett., 8, 518–527, https://doi.org/10.1080/2150704X.2017.1295480, 2017.
Cogley, J. G.: Glacier shrinkage across High Mountain Asia, Ann. Glaciol., 57, 41–49, https://doi.org/10.3189/2016AoG71A040, 2016.
Das, S. and Sharma, M. C.: Glacier changes between 1971 and 2016 in the Jankar Chhu Watershed, Lahaul Himalaya, India, J. Glaciol., 65, 13–28, https://doi.org/10.1017/jog.2018.77, 2018.
DeBeer, C. M. and Sharp, M. J.: Topographic influences on recent changes of very small glaciers in the Monashee mountains, British Columbia, Canada, J. Glaciol., 55, 691–700, https://doi.org/10.3189/002214309789470851, 2009.
Deota, B. S., Trivedi, Y. N., Kulkarni, A. V., Bahuguna, I. M., and Rathore, B. P.: RS and GIS in mapping of geomorphic records and understanding the local controls of glacial retreat from the Baspa Valley, Himachal Pradesh, India, Curr. Sci. India, 100, 1555–1563, 2011.
Dimri, A. P.: Interseasonal oscillation associated with the Indian winter monsoon, J. Geophys. Res.-Atmos., 118, 1189–1198, https://doi.org/10.1002/jgrd.50144, 2013.
Dobhal, D. P., Mehta, M., and Srivastava, D.: Influence of debris cover on terminus retreat and mass changes of Chorabari Glacier, Garhwal region, central Himalaya, India, J. Glaciol., 59, 961–971, https://doi.org/10.3189/2013jog12j180, 2013.
Gardelle, J., Berthier, E., Arnaud, Y., and Kääb, A.: Region-wide glacier mass balances over the Pamir-Karakoram-Himalaya during 1999–2011, The Cryosphere, 7, 1263–1286, https://doi.org/10.5194/tc-7-1263-2013, 2013.
Garg, P. K., Shukla, A., Tiwari, R. K., and Jasrotia, A. S.: Assessing the status of glaciers in parts of the Chandra basin, Himachal Himalaya: A multiparametric approach, Geomorphology, 284, 99–114, https://doi.org/10.1016/j.geomorph.2016.10.022, 2017a.
Garg, P. K., Shukla, A., and Jasrotia, A. S.: Influence of topography on glacier changes in the central Himalaya, India, Global Planet. Change, 155, 196–212, https://doi.org/10.1016/j.gloplacha.2017.07.007, 2017b.
Garg, S., Shukla, A., Mehta, M., Kumar, V., Samuel, S. A., Bartarya, S., and Shukla, U. K.: Field evidences showing rapid frontal degeneration of the Kangriz glacier, Suru basin, Jammu and Kashmir, J. Mountain Sci., 15, 1199–1208, https://doi.org/10.1007/s11629-017-4809-x, 2018.
Garg, S., Shukla, A., Mehta, M., Kumar, V., and Shukla, U. K.: On geomorphic manifestations and glaciation history of the Kangriz glacier, western Himalaya, Himal. Geol., 40, 115–127, 2019.
Guo, Z., Wanga, N., Kehrwald, N. M., Mao, R., Wua, H., Wu, Y., and Jiang, X.: Temporal and spatial changes in western Himalayan firn line altitudes from 1998 to 2009, Global Planet. Change, 118, 97–105, https://doi.org/10.1016/j.gloplacha.2014.03.012, 2014.
Hall, D. K., Bayr, K. J., Schöner, W., Bindschadlerd, R. A., and Chiene, J. Y. L.: Consideration of the Errors Inherent in Mapping Historical Glacier Positions in Austria from the Ground and Space (1893–2001), Remote Sens. Environ., 86, 566–577, https://doi.org/10.1016/S0034-4257(03)00134-2, 2003.
Hanshaw, M. N. and Bookhagen, B.: Glacial areas, lake areas, and snow lines from 1975 to 2012: status of the Cordillera Vilcanota, including the Quelccaya Ice Cap, northern central Andes, Peru, The Cryosphere, 8, 359–376, https://doi.org/10.5194/tc-8-359-2014, 2014.
Harris, I. C. and Jones, P. D.: CRU TS 4.02: Climatic Research Unit (CRU) year-by-year variation of selected climate variables by country (CY) version 4.02 (Jan. 1901–Dec. 2017), Centre for Environmental Data Analysis, https://doi.org/10.5285/d4e823f0172947c5ae6e6b265656c273, 2018.
India Meteorological Department (IMD): Climatological table, available online: http://www.imd.gov.in/pages/city_weather_show.php (last access: 15 December 2019), 2015.
Immerzeel, W. W., Beek, L. P. H., and Bierkens M. F. P.: Climate change will affect the Asian water towers, Science, 328, 1382–1385, https://doi.org/10.1126/science.1183188, 2010.
IPCC: Summary for policymakers, in: Climate Change 2013: The Physical Science Basis, Contribution of Working Group III to the Fifth Assessment Report of Intergovernmental Panel on Climate Change, edited by: Stocker, T. F., Qin, D., Plattner, G.-K., Tignor, M., Allen, S. K., Boschung, J., Nauels, A., Xia, Y., Bex, V., and Midgley, P. M., Cambridge University Press, Cambridge and New York, 2013.
Kääb, A., Berthier, E., Nuth, C., Gardelle, J., and Arnaud, Y.: Contrasting patterns of early twenty first century glacier mass change in the Himalayas, Nature, 488, 495–498, https://doi.org/10.1038/nature11324, 2012.
Kääb, A., Treichler, D., Nuth, C., and Berthier, E.: Brief Communication: Contending estimates of 2003–2008 glacier mass balance over the Pamir–Karakoram–Himalaya, The Cryosphere, 9, 557–564, https://doi.org/10.5194/tc-9-557-2015, 2015.
Kamp, U., Byrne, M., and Bolch, T.: Glacier Fluctuations between 1975 and 2008 in the Greater Himalaya Range of Zanskar, Southern Ladakh, J. Mountain Sci., 8, 374–389, https://doi.org/10.1007/s11629-011-2007-9, 2011.
Kaser, G., Großhauser, M., and Marzeion, B.: Contribution potential of glaciers to water availability in different climate regimes, P. Natl. Acad. Sci. USA, 107, 20223–20227, https://doi.org/10.1073/pnas.1008162107, 2010.
Kulkarni, A. V., Bahuguna, I. M., Rathore, B. P., Singh, S. K., Randhawa, S. S., Sood, R. K., and Dhar, S.: Glacial retreat in Himalaya using remote sensing satellite data, Curr. Sci. India, 92, 69–74, https://doi.org/10.1117/12.694004, 2007.
Kulkarni, A. V., Rathore, B. P., Singh, S. K., and Bahuguna, I. M.: Understanding changes in Himalayan Cryosphere using remote sensing technique, Int. J. Remote Sens., 32, 601–615, https://doi.org/10.1080/01431161.2010.517802, 2011.
Liu, S., Ding, Y., Shangguan, D., Zhang, Y., Li, J., Han, H., Wang, J., and Xie, C.: Glacier retreat as a result of climate warming and increased precipitation in the Tarim river basin, northwest China, An. Glaciol., 43, 91–96, 2006.
Maurer, J. M., Schaefer, J. M., Rupper, S., and Corley, A.: Acceleration of ice loss across the Himalayas over the past 40 years, Sci. Adv., 5, 1–12, https://doi.org/10.1126/sciadv.aav7266, 2019.
Mayewski, P. A. and Jeschke, P. A.: Himalayan and Trans-Himalayan Glacier Fluctuations Since A.D. 1812, Arctic Alpine Res., 11, 267–287, 1980.
Miller, J. D., Immerzeel, W. W., and Rees, G.: Climate change impacts on glacier hydrology and river discharge in the Hindu Kush-Himalaya, Mountain Research and Development, 32, 461–467, https://doi.org/10.1659/MRD-JOURNAL-D-12-00027.1, 2012.
Minora, U., Bocchiola, D., D'Agata, C., Maragno, D., Mayer, C., Lambrecht, A., Mosconi, B., Vuillermoz, E., Senese, A., Compostella, C., Smiraglia, C., and Diolaiuti, G.: 2001–2010 glacier changes in the Central Karakoram National Park: a contribution to evaluate the magnitude and rate of the “Karakoram anomaly”, The Cryosphere Discuss., 7, 2891–2941, https://doi.org/10.5194/tcd-7-2891-2013, 2013.
Mir, R. A., Jain, S. K., Jain, Thayyen, R. J., and Saraf, A. K.: Assessment of recent glacier changes and its controlling factors from 1976 to 2011 in Baspa Basin, western Himalaya, Arct. Antarct. Alp. Res., 49, 621–647, https://doi.org/10.1657/AAAR0015-070, 2017.
Mölg, N., Bolch, T., Rastner, P., Strozzi, T., and Paul, F.: A consistent glacier inventory for Karakoram and Pamir derived from Landsat data: distribution of debris cover and mapping challenges, Earth Syst. Sci. Data, 10, 1807–1827, https://doi.org/10.5194/essd-10-1807-2018, 2018.
Murtaza K. O. and Romshoo S. A.: Recent glacier changes in the Kashmir Alpine Himalayas, India, Geocarto Int., 32, 188–205, https://doi.org/10.1080/10106049.2015.1132482, 2015.
Nuimura, T., Sakai, A., Taniguchi, K., Nagai, H., Lamsal, D., Tsutaki, S., Kozawa, A., Hoshina, Y., Takenaka, S., Omiya, S., Tsunematsu, K., Tshering, P., and Fujita, K.: The GAMDAM glacier inventory: a quality-controlled inventory of Asian glaciers, The Cryosphere, 9, 849–864, https://doi.org/10.5194/tc-9-849-2015, 2015.
Pandey, A., Ghosh, S., and Nathawat, M. S.: Evaluating patterns of temporal glacier changes in Greater Himalayan Range, Jammu and Kashmir, India, Geocarto Int., 26, 321–338, https://doi.org/10.1080/10106049.2011.554611, 2011.
Pandey, P. and Venkataraman, G.: Changes in the glaciers of Chandra–Bhaga basin, Himachal Himalaya, India, between 1980 and 2010 measured using remote sensing, Int. J. Remote Sens., 34, 5584–5597, https://doi.org/10.1080/01431161.2013.793464, 2013.
Patel, L. K., Sharma, P., Fathima, T. N., and Thamban, M.: Geospatial observations of topographical control over the glacier retreat, Miyar basin, western Himalaya, India, Environ. Earth Sci., 77, 190, https://doi.org/10.1007/s12665-018-7379-5, 2018.
Paul, F., Barrand, N. E., Baumann, S., Berthier, E., Bolch, T., Casey, K., Frey, H., Joshi, S. P., Konovalov, V., Bris, R. L., and Mölg, N.: On the accuracy of glacier outlines derived from remote-sensing data, Ann. Glaciol., 54, 171–182, https://doi.org/10.3189/2013AoG63A296, 2013.
Paul, F., Bolch, T., Kääb, A., Nagler, T., Nuth, C., and Scharrer, K.: The glaciers climate change initiative: methods for creating glacier area, elevation change and velocity products, Remote Sens. Environ., 162, 408–426, https://doi.org/10.1016/j.rse.2013.07.043, 2015.
Paul, F., Bolch, T., Briggs, K., Kääb, A., McMillan, M., McNabb, R., Nagler, T., Nuth, C., Rastner, P., Strozzi, T., and Wuite, J.: Error sources and guidelines for quality assessment of glacier area, elevation change, and velocity products derived from satellite data in the Glaciers_cci project, Remote Sens. Environ., 203, 256–275, https://doi.org/10.1016/j.rse.2017.08.038, 2017.
Pfeffer, W. T., Arendt, A., Bliss, A., Bolch, T., Cogley, J. G., Gardner, A. S., Hagen, J. O., Hock, R., Kaser, G., Kienholz, C., Miles, E. S., Moholdt, G., Molg, N., Paul, F., Radic, V., Rastner, P., Raup, B. H., Rich, J., and Sharp, M.: The Randolph Glacier Inventory: A globally complete inventory of glaciers, J. Glaciol., 60, 537–552, https://doi.org/10.3189/2014JoG13J176, 2014.
Pritchard, H. D.: Asia's glaciers are a regionally important buffer against drought, Nature, 545, 169–187, https://doi.org/10.1038/nature22062, 2017.
Racoviteanu, A. E., Arnaud, Y., Williams, M. W., and Ordonez, J.: Decadal changes in glacier parameters in the Cordillera Blanca, Peru, derived from remote sensing, J. Glaciol., 54, 499–510, https://doi.org/10.3189/002214308785836922, 2008.
Racoviteanu, A., Paul, F., Raup, B., Khalsa, S. J. S., and Armstrong, R.: Challenges and recommendations in mapping of glacier parameters from space: results of the 2008 Global Land Ice Measurements from Space (GLIMS) workshop, Boulder, Colorado, USA, Ann. Glaciol., 50, 53–69, https://doi.org/10.3189/172756410790595804, 2009.
Rai, P. K., Nathawat, M. S., and Mohan, K.: Glacier retreat in Doda valley, Zanskar basin, Jammu and Kashmir, India, Universal Journal of Geoscience, 1, 139–149, https://doi.org/10.13189/ujg.2013.010304, 2013.
Raina, R. K. and Koul, M. N.: Impact of Climatic Change on Agro-Ecological Zones of the Suru-Zanskar Valley, Ladakh (Jammu and Kashmir), India, Journal of Ecology and the Natural Environment, 3, 424–440, 2011.
Raina, V. K.: Himalayan glaciers: a state-of-art review of glacial studies, glacial retreat and climate change, Himal. Glaciers State-Art Review, Glacial Stud. Glacial Retreat Climate Change,Ministry of Environment and Forests Discussion paper, 2009.
Rashid, I., Romshoo, S. A., and Abdullah, T.: The recent deglaciation of Kolahoi Valley in Kashmir Himalaya, India in response to the changing climate, J. Asian Earth Sci., 138, 38–50, https://doi.org/10.1016/j.jseaes.2017.02.002, 2017.
Raup, B., Racoviteanu, A., Khals, S. J. S., Helm, C., Armstrong, R., and Arnaud, Y.: The GLIMS geospatial glacier database: a new tool for studying glacier change, Global Planet. Change, 56, 101–110, https://doi.org/10.1016/j.gloplacha.2006.07.018, 2007.
Rivera, A., Cawkwell, F., Rada, C., and Bravo, C.: Hypsometry, in: Encyclopaedia of Snow, Ice and glaciers, Springer, Netherlands, 551–554, 2011.
Sakai, A.: Glacial lakes in the Himalayas: A review on formation and Expansion process, Global Environ. Res., 16, 23–30, 2012.
Sakai, A. and Fujita, K.: Contrasting glacier responses to recent climate change in high-mountain Asia, Sci. Rep., 7, 1–18, https://doi.org/10.1038/s41598-017-14256-5, 2017.
Sangewar, C. V. and Shukla, S. P.: Inventory of the Himalayan Glaciers: A Contribution to the International Hydrological Programme, An Updated Edition, Kolkata: Geological Survey of India, Special Publication 34, IISN: 1:0254–0436, 2009.
Scherler, D., Bookhagen, B., and Strecker, M. R.: Spatially variable response of Himalayan glaciers to climate change affected by debris cover, Nat. Geosci., 4, 156–159, https://doi.org/10.1038/ngeo1068, 2011.
Schmidt, S. and Nuesser, M.: Changes of High Altitude Glaciers in the Trans-Himalaya of Ladakh over the Past Five Decades (1969–2016), Geosciences, 7, 27, https://doi.org/10.3390/geosciences7020027, 2017.
Sen, P. K.: Estimates of the regression coefficient based on Kendall's Tau, Am. Stat. J., 63, 1379–1389, https://doi.org/10.2307/2285891, 1968.
Shekhar, M., Bhardwaj, A., Singh, S., Ranhotra1, P. S., Bhattacharyya, A., Pal, A. K., Roy, I., Martín- Torres, F. J., and Zorzano, M.P.: Himalayan glaciers experienced significant mass loss during later phases of little ice age, Sci. Rep., 7, 1–14, 2017.
Shiyin, L., Donghui, S., Junli, Xu., Xin, W., Xiaojun, Y., Zongli, J., Wanqin, G., Anxin, L., Shiqiang, Z., Baisheng, Ye., Zhen, Li., Junfeng, W., and Lizong, W.: Glaciers in China and Their Variations, in: Global Land Ice Measurements from Space, edited by: Kargel, J., Leonard, G., Bishop, M., Kääb, A., and Raup, B., Springer Praxis Books, Springer, Berlin, Heidelberg, 2014
Shukla, A., Gupta, R. P., and Arora, M. K.: Estimation of debris cover and its temporal variation using optical satellite sensor data: a case study in Chenab basin, Himalaya, J. Glaciol., 55, 444–452, https://doi.org/10.3189/002214309788816632, 2009.
Shukla, A. and Qadir, J.: Differential response of glaciers with varying debris cover extent: evidence from changing glacier parameters, Int. J. Remote Sens., 37, 2453–2479, https://doi.org/10.1080/01431161.2016.1176272, 2016.
Shukla, A., Garg, P. K., Mehta, M., and Kumar, V.: Changes in dynamics of Pensilungpa glacier, western Himalaya, over the past two decades, in: Proceedings of the 38th Asian Conference on Remote Sensing, Delhi, India, 23–27 October 2017.
Shukla, A., Garg, S., Mehta, M., Kumar, V., and Shukla, U. K.: Temporal inventory of glaciers in the Suru sub- basin, western Himalaya, PANGAEA, https://doi.org/10.1594/PANGAEA.904131, 2019.
Singh, J. and Yadav, R. R.: Tree-ring indications of recent glacier fluctuations in Gangotri, western Himalaya, India, Curr. Sci. India, 79, 1598–1601, 2000.
Space Application Centre (SAC): Report: Monitoring Snow and Glaciers of Himalayan Region, Space Application Centre, ISRO, Ahmedabad, India, 413 pp., 2016.
Vaughan, D. G., Comiso, J. C., Allison, I., Carrasco, J., Kaser, G., Kwok, R., Mote, P., Murray, T., Paul, F., Ren, J., Rignot, E., Solomina, O., Steffen, K., and Zhang, T.: Observations: Cryosphere, in: Climate change 2013: The physical science basis. Contribution of working group I to the fifth assessment report of the intergovernmental panel on climate change, edited by: Stocker, T. F., Qin, D., Plattner, G. K., Tignor, M., Allen, S. K., Boschung, J., Nauels, A., Xia, Y., Bex, V., and Midgley, P. M., Cambridge University Press, Cambridge, United Kingdom and New York, NY, USA, 2013.
Venkatesh, T. N., Kulkarni, A. V., and Srinivasan, J.: Relative effect of slope and equilibrium line altitude on the retreat of Himalayan glaciers, The Cryosphere, 6, 301–311, https://doi.org/10.5194/tc-6-301-2012, 2012.
Vijay, S. and Braun, M.: Early 21st century spatially detailed elevation changes of Jammu and Kashmir glaciers (Karakoram–Himalaya), Global Planet. Change, 165, 137–146, https://doi.org/10.1016/j.gloplacha.2018.03.014, 2018.
Vittoz, P.: Ascent of the Nun in the Mountain World: 1954, edited by: Kurz, M., George Allen and Unwin, Ltd., London, 1954.
Zhou, Y., Li, Z., Li, J., Zhao, R., and Ding, X.: Geodetic glacier mass balance (1975–1999) in the central Pamir using the SRTM DEM and KH-9 imagery, J. Glaciol., 65, 309–320, https://doi.org/10.1017/jog.2019.8, 2018.