the Creative Commons Attribution 4.0 License.
the Creative Commons Attribution 4.0 License.
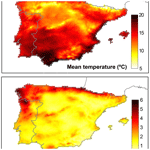
Iberia01: a new gridded dataset of daily precipitation and temperatures over Iberia
Sixto Herrera
Rita Margarida Cardoso
Pedro Matos Soares
Fátima Espírito-Santo
Pedro Viterbo
José Manuel Gutiérrez
The present work presents a new observational gridded dataset (referred to as Iberia01) for daily precipitation and temperatures produced using a dense network (thousands) of stations over the Iberian Peninsula for the period 1971–2015 at 0.1∘ regular (and 0.11∘ CORDEX-compliant rotated) resolutions. We analyze mean and extreme indices and compare the results with the E-OBS v17 dataset (using both the standard and ensemble versions, at 0.25 and 0.1∘ resolutions, respectively), in order to assess observational uncertainty in this region. We show that Iberia01 produces more realistic precipitation patterns than E-OBS for the mean and extreme indices considered, although both are comparable for temperatures. To assess the differences between these datasets, a new probabilistic intercomparison analysis was performed, using the E-OBS ensemble (v17e) to characterize observational uncertainty and testing whether Iberia01 falls within the observational uncertainty range provided by E-OBS. In general, uncertainty values are large in the whole territory, with the exception of a number of kernels where the uncertainty is small, corresponding to the stations used to build the E-OBS grid. For precipitation, significant differences – at the 10 % level – between both datasets were found for fewer than 25 % of days over the Iberian Peninsula. For temperature, a very inhomogeneous spatial pattern was obtained, with either a small (in most of the regions) or large fraction of significantly different days, thus indicating sensible regions for observational uncertainty.
Iberia01 is publicly available (Herrera et al., 2019a, https://doi.org/10.20350/digitalCSIC/8641).
- Article
(2444 KB) - Full-text XML
-
Supplement
(960 KB) - BibTeX
- EndNote
The Iberia01 gridded dataset is made available under the Open Database License. Any rights in individual contents of the database are licensed under the Database Contents License.
The availability of high-resolution climate data together with an estimate of their uncertainty (observational uncertainty) is of paramount importance for climate studies, from global (Sun et al., 2018) to regional and local scales (Kidd et al., 2011). The first comprehensive gridded temperature dataset was obtained by Jones et al. (1982). This dataset only covered the Northern Hemisphere and produced monthly means on a 5∘ latitude by 10∘ longitude grid. Later, this grid was extended to cover the entire globe with a higher spatial resolution (at 0.5∘ resolution; Jones et al., 1986a, b) and currently includes several variables covering Earth's land areas for 1901–2015 (CRU TS4.0; Harris et al., 2014; Trenberth et al., 2014). However, this kind of resolution is too coarse for regional analysis, which typically requires datasets with spatial resolution of tens of kilometers and daily to sub-daily temporal data in order to differentiate climatic subregions and extreme events. In Europe, the first high-resolution continental observational gridded dataset was produced within the framework of the ENSEMBLES project (E-OBS dataset), for daily precipitation and maximum, minimum, and mean temperatures (Klein Tank et al., 2002; Haylock et al., 2008; Klok and Klein Tank, 2009) and sea level pressure (van den Besselaar et al., 2011). With more than 1490 citations in Scopus (as of August 2019), this is the most used climate reference for European climate studies. Yet, in some regions, E-OBS relies on a sparse observational network which limits its ability to correctly represent not only mean values, but also the variance and extremes, particularly over complex topography (Klok and Klein Tank, 2009). The influence of stations density in the quality of gridded products has been analyzed in the last decades by several authors: Rudolf et al. (1994) were able to significantly reduce the precipitation error, from a maximum of 40 % to 20 %, by doubling the number of stations within a 2.5∘ grid box; Prein and Gobiet (2017) found that in regions with sparse data the uncertainties associated with mean seasonal precipitation could reach 60 %; Beguería et al. (2016) found that, in a high-resolution observationally based gridded dataset, the density of the underlying observations determines its spatial variance and thus strongly influences climate variability; Hofstra et al. (2010) concluded that, by randomly changing the number of stations in each grid box, a reduction in the density of stations decreases the variability of both precipitation and temperature with large implications for the representation of extremes. Moreover, large temporal differences in the number of stations within each grid box also add another source of uncertainty since they can change trends of the time series (Hofstra et al., 2009; Frei, 2014; Beguería et al., 2016). Finally, in an analysis of the sources of uncertainty in observationally based gridded datasets, Herrera et al. (2019b) highlight that the station density represents the major variability factor, irrespective of the interpolation method. The authors analyzed several grids for Spain (complex topography) and Poland (smooth topography) and concluded that the influence of station density is more pronounced in Spain than in Poland due to the large spatial variability and complex orography of the former.
The quality of the station observations is an additional source of observational uncertainty for gridded products. These uncertainties may be reduced by applying quality control procedures and homogenizing the time series (Herrera et al., 2012). Precipitation time series also commonly suffer from undercatch associated with windy conditions, which usually results in underestimation of the correct precipitation rate (Frei et al., 2003). Yet in complex topography an increased uncertainty may be associated with the use of these types of corrections (Adam et al., 2006). The areal representativeness of a particular station also poses a challenge. Again, in regions with high terrain gradients, like mountains or coastal areas, surface temperatures are affected by local circulations like sea breeze, up- or downslope breeze associated with nighttime radiative cooling in the valleys and with differentiated warming–cooling at sunrise–sunset of the slopes (Whiteman, 1982; Whiteman and McKee, 1982; Whiteman, 1990). Frei (2014) proposed a new interpolation method to tackle the latter, in which the thermal vertical profile of the station surrounding area is considered. Yet, Frei (2014) also acknowledges that the best way to reduce this type of uncertainty is through high station density.
Recently, several national high-resolution grids have been compiled for individual European countries from dense observation networks: SAFRAN analysis at 8 km grid spacing covering France at an hourly time step (Durand et al., 1993; Quintana-Seguí et al., 2008; Vidal et al., 2010) and its recently published extension for continental Spain and the Balearic Islands (Quintana-Seguí et al., 2017); PTHBV, a 4 km daily dataset for Sweden (Johansson, 2000; Johansson and Chen, 2003); the 5 km resolution HYRAS for Germany (Rauthe et al., 2013; Frick et al., 2014); seNorge2, a daily dataset with a 1 km resolution for Norway (Uboldi et al., 2008; Lussana et al., 2018); TabsD (MeteoSwiss, 2013a) and RhiresD (MeteoSwiss, 2013b) at 2 km for Switzerland; CARPATCLIM, a 0.1∘ grid covering parts of nine countries along the Carpathian Mountains (Lakatos et al., 2013); and a 0.11∘ grid for Poland (Herrera et al., 2019b).
On the Iberian Peninsula, Herrera (2011) and Herrera et al. (2012) built a precipitation regular grid for continental Spain and the Balearic Islands based on 2756 stations (Spain02) following the methodology used in E-OBS. The same methodology was also applied by Belo-Pereira et al. (2011) for continental Portugal using more than 400 stations (PT02). Both datasets had consistent grids (with 0.2∘ resolution) and time periods (1950–2003) and were combined to build a gridded precipitation dataset of opportunity for the Iberian Peninsula (IB02). However, this is not a homogeneous product for the Iberian Peninsula due to the discrepancies existing between the two datasets near the borders, particularly in the northern mountains. Recently, Herrera et al. (2015) updated the Spanish grid including precipitation and temperatures (daily maximum, mean, and minimum) and enhancing the spatial resolution to 0.1∘ (regular); moreover, they also provided results on a 0.11∘ rotated grid (CORDEX compliant) for the purpose of regional climate model (RCM) evaluation. The lack of temperature for PT02 (and IB02) hinders a comprehensive analysis of the large inter-annual and spatial variability, characteristic of the Iberian climate (Esteban-Parra et al., 1998; Muñoz-Díaz and Rodrigo, 2004; Cardoso et al., 2013). These problems could be solved building an Iberian grid, using observational station data from both countries.
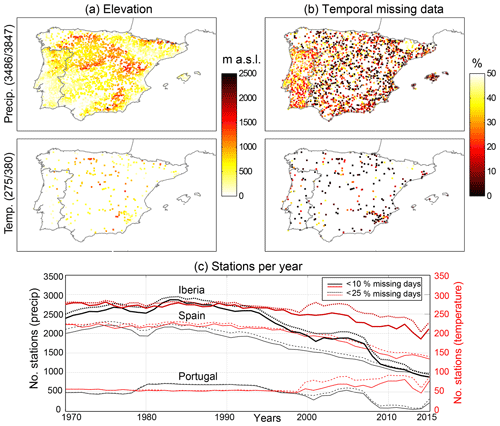
Figure 1(a) Elevation and (b) data availability (percentage of missing days) in the period 1971–2015 for precipitation (top) and temperature (bottom) for the observational networks considered for the interpolation. The numbers on the left of the figure reflect the initial and final number of stations used, once the quality control is applied. (c) Number of stations – considering the Iberian Peninsula, Spain, or Portugal – per year for different thresholds of annual missing data for precipitation (black) and temperature (red).
In this paper, we develop an Iberian-wide daily regular grid at 0.1∘ resolution, for precipitation and temperatures (maximum, mean, and minimum), as well as a 0.11∘ rotated grid (EURO-CORDEX compliant) suitable for model evaluation purposes. This grid is based on a high-density network of stations across continental Portugal and Spain and the Balearic Islands, with a reasonably stable number of stations for the period 1971–2015. This represents the first gridded dataset of daily precipitation and temperatures for Iberia as a whole and can be considered an update of the PT02 and IB02 datasets. Here, we also introduce the elevation as covariate in the interpolation process, which was missing in the initial PT02 and Spain02. The resulting dataset is compared against the most recent version of E-OBS (v17.0, referred to as v17), which includes a new ensemble version (v17e) to assess observational uncertainty and allows for a new probabilistic intercomparison of these datasets.
The paper is structured as follows. In Sect. 2 a description of the data and methods considered in this work is presented. The main results are described in Sect. 3. Finally, the main conclusions from the analysis are detailed in Sect. 5.
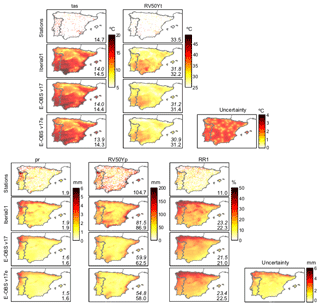
Figure 2Climatology of the different temperature (top) and precipitation (bottom) indices defined in Table 1, from the stations (local values), Iberia01 (0.1∘ resolution), E-OBS v17 (0.2∘), and E-OBS v17e (0.1∘), in rows. The climatologies have been obtained by averaging the annual values of the indices (tas, pr, and RR1); in the case of the 50-year return value the index is representative of the whole period. For the ensemble E-OBS version (v17e), the climatology of daily standard deviations of the ensemble values is also shown (characterizing E-OBS observational uncertainty). The numbers shown in each panel correspond to the spatial mean values, calculated for gridded datasets averaging over all grid boxes (top, in italics) or over the grid boxes closest to the stations (bottom), to provide a fair comparison with the station mean values in the top panels (in particular these numbers are used to assess the biases of the different gridded datasets).
2.1 Observation network and quality control
The present work is based on a dense network of thousands of stations from the Spanish Meteorological Agency (AEMET), the Portuguese Institute for Sea and Atmosphere (IPMA), and the Portuguese Environmental Agency (APA). To keep consistency with previous datasets, the final network was obtained by applying the same quality control used to build Spain02 (see Herrera, 2011; Herrera et al., 2012, for a detailed description), which requires stations with at least 15 (40) years in the period 1951–2015 with less than 10 % yearly missing precipitation (temperature) data. The resulting observational network includes 3486 and 275 stations for precipitation and temperature, respectively, as shown in Fig. 1a, b. Note that detailed metadata for each station, including geographical and data availability information, are provided as part of the dataset in the same repository. Figure 1c shows the data availability on a yearly basis, exhibiting a clear decline in the number of stations in the last 2 decades, mainly for precipitation. Therefore, the resulting gridded product is not suitable for historical trend analysis since biased results could be obtained as a result of the changing number of stations. Moreover, during the period 2009–2014 there are very few precipitation stations in Portugal and therefore results should be interpreted with caution in this period. Therefore, we recommend using the reference climate period 1971–2000 for this dataset. Overall, the spatial distribution of the stations is quite homogeneous over the Iberian Peninsula with a good representation of the orographical gradients, especially for the case of precipitation (see the first column of Fig. 1a). Therefore, the elevation was included as a covariate in the interpolation process (at a monthly scale) to model and reflect these gradients (see Sect. 2.4).
2.2 E-OBS gridded datasets (v17 and v17e)
E-OBS (Haylock et al., 2008) is the reference gridded dataset of daily precipitation and temperatures in Europe and has been previously used to analyze the observational uncertainty in the context of the evaluation of regional climate models (see, e.g., Kotlarski et al., 2019). In this study, we use both the standard (v17, 0.2∘ resolution) and the ensemble (v17e, 0.1∘ resolution; Cornes et al., 2018) versions of E-OBS v17 as a benchmark for comparison purposes. In addition to the estimated daily value for each grid box, the ensemble grid also provides a measure of daily uncertainty, characterized by the standard deviation of the ensemble. E-OBS v17 is used for the sake of comparison with Iberia01 (see Fig. 2), and E-OBS v17e (the ensemble) is used to assess the observational uncertainty provided by this dataset and test whether Iberia01 does not differ significantly from the ensemble (i.e., it falls within the observational uncertainty range) with a certain confidence (90 %) day by day. For this purpose, the E-OBS ensemble mean (μ) and spread (σ) are used to define a normal distribution N(μ,σ) characterizing observational uncertainty for each grid box and day, and the corresponding Iberia01 values are classified as either inside or outside (values outside the P5–P95 percentile range) the uncertainty range for each grid box and day. Note that outsider values indicate significant differences between both datasets (as characterized by the E-OBS ensemble).
2.3 Weather indices
To analyze the mean and extreme regimes of precipitation and temperature we use the indicators shown in Table 1. In particular, the 50-year return value for each grid box was used to characterize the extreme regimes (for the period 1971–2015) obtained by adjusting a generalized extreme value (GEV) distribution to the series of annual maximum of daily values (see Herrera et al., 2015, for a detailed description). In the case of precipitation, both wet-day frequency and rainfall intensity have been considered to properly characterize the mean regime.
2.4 Gridding method
The Iberia01 daily gridded dataset for precipitation and temperature was built using the previously described observational network and applying the area-averaged three-dimensional (AA-3D) interpolation method described in previous studies (Herrera, 2011; Herrera et al., 2012, 2015). This interpolation method is an area-averaged method based on ordinary kriging (OK; Krige, 1951; Matheron, 1962) and three-dimensional thin plate splines (3D-TPSs; Craven and Wahba, 1979; Wahba, 1990; Hutchinson, 1998a, b) in a two-step process:
-
first, the 3D-TPS is applied to the monthly value considering the elevation given by the Global Digital Elevation Model (GTOPO30; see Sect. 4) as covariate;
-
second, the daily anomaly is interpolated by applying OK;
-
and as a result both the daily anomaly and monthly value are combined to obtain the interpolated daily values
To ensure the area-averaged representativity of the final values, the initial interpolation is performed over an auxiliary 0.01∘ resolution grid, and then the interpolated results are upscaled (averaged) to the target resolutions, in our case a regular version of 0.10∘ spatial resolution (approximately 10 km) and a rotated version matching the grids considered in the EURO-CORDEX project (0.11 and 0.44∘). In this work we only describe for simplicity the regular version of 0.10∘ spatial resolution, although the other datasets are also provided (these datasets will be used in a future paper to evaluate the performance of EURO-CORDEX models over Iberia).
Taking into account the two-step interpolation procedure followed to develop the area-average representative gridded dataset, a natural doubt surges about the possible application of the auxiliary very-high-resolution grid (1 km) to build a grid at a resolution higher than 10 km. For instance, the new RCM convection-permitting simulations performed in the framework of the CORDEX Flagship Pilot Studies (FPS) reach a resolution of 2–3 km, and thus high-resolution grids are needed for the evaluation of these projects (Giorgi et al., 2009; Jacob et al., 2014). Some previous works warn against the development of high-resolution grids with few (or none) stations per grid box (Herrera et al., 2019b). Therefore, we limited the resolution of Iberia01 to 0.10∘ and do not provide higher-resolution intermediate products. As an illustrative example to illustrate the effective resolution of the datasets, we consider a convective high-resolution extreme precipitation event that occurred on 4–5 November 1997, characterized by heavy precipitation over most of the Iberian Peninsula. This event had great socioeconomic impacts in Portugal (Ramos and Reis, 2002) and Spain (Lorente et al., 2008), and was ranked as the second greatest extreme precipitation event of the Iberian Peninsula (Ramos et al., 2015). We use this event as an illustrative case study of the effective resolution of a potential 3 km gridded version of Iberia01, compared with the standard 10 km resolution.
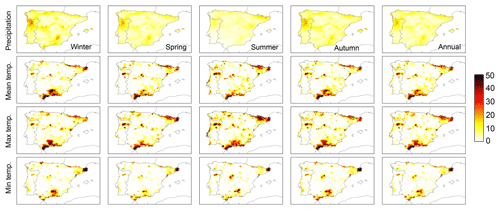
Figure 3Percentage of significantly different days between Iberia01 and E-OBS v17e for each grid box, variable, and season, defined as the Iberia01 daily values outside the P5–P95 percentile interval of the normal distribution given by the E-OBS ensemble, in the period 1970–2015 for wet days (first row), and mean (second row), maximum (third row), and (fourth row) minimum temperatures.
Figure 2 shows the climatologies (mean annual values) of the indices shown in Table 1 for Iberia01 (0.1∘) and E-OBS (v17, 0.2∘, and v17e, 0.1∘). The three datasets provide similar results in the case of temperature, with the main differences being located in the south, around the Guadalquivir and Guadiana basins, where the maximum values are attained. In addition, the climatology of observational uncertainty (calculated as the temporal mean of the daily standard deviations of the ensemble values) is also provided for the ensemble version of E-OBS, showing a value around 2.5∘ in all the territory, with the exception of a number of kernels where the uncertainty is very small, corresponding to the stations used to build the E-OBS grid. Note that the observational uncertainty pattern is different in Spain and Portugal, with more clear kernels in the first case; this could be due to the different temporal coverage of both networks, with an increase in uncertainty due to days with no observations. Therefore, the uncertainty conveys relevant information on the station network used to build the grid.
In the case of precipitation, E-OBS cannot reproduce either the mean spatial pattern (pr) or the intensity of the 50-year return value (RV50Yp). E-OBS underestimates mean precipitation by 15 % (mean relative bias, for E-OBSv17 and v17e, as obtained from the spatial mean numbers in Fig. 2), particularly in the Central System range of the Iberian Peninsula, and 50-year return values by 40 %–44 % (mean relative bias, for E-OBSv17 – v17e, respectively), with some very high biases in some southern and Mediterranean regions. The case of wet-day frequency is different since all datasets show a clear overestimation, with Iberia01 showing a more orographic pattern than E-OBS. In this case, the higher resolution of E-OBS v17e provides further spatial detail compared to the standard v17 one, which is not evident for precipitation intensity. Moreover, the E-OBS observational uncertainty is of similar magnitude to the mean value (also with kernels of small uncertainty corresponding to stations), reflecting a large uncertainty for this variable.
Figure 3 shows the percentage of significantly different days for each grid box, variable, and season. For precipitation (first row), only Iberia01 wet days were used in order to minimize the effect of the different wet-day frequencies. The differences for this variable exhibit a homogeneous spatial pattern over the peninsula with values around 10 % in general; this is due to the large uncertainty of the daily E-OBS ensemble spread (see Fig. 2). Regarding the temperatures, most of the spatial pattern presents values close to zero, reflecting the similarity between both datasets for these variables. However, some local differences are found, particularly for the mean (second row) and maximum (third row) temperatures, with the greatest values reached in the Pyrenean and Central System ranges and the south coast of the Iberian Peninsula, in agreement with the differences shown in Fig. 2. In this case, the ensemble uncertainty is in agreement with the differences between these two datasets found in Fig. 2.
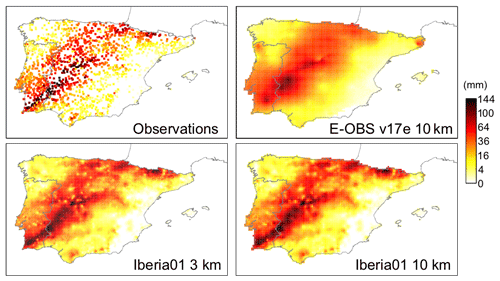
Figure 4Daily precipitation of 4–5 November 1997 observed and represented by E-OBS v17e and Iberia01 (10 km) and the higher-resolution 3 km version of Iberia01.
Finally, we consider an illustrative extreme event that occurred on 4–5 November 1997 to illustrate the effective resolution of the different datasets (including potentially new higher-resolution products). To this aim, compare the resulting values of the 0.1∘ grid with a higher-resolution 0.03∘ one developed using the auxiliary 0.01∘ grid generated in the interpolation process. Figure 4 shows the results obtained for the extreme event indicating that an increment of the Iberia01 resolution beyond 10 km has no clear impact in the effective resolution of the precipitation pattern. In particular, in spite of the apparent improvement of both versions of Iberia01 with respect to E-OBS v17e, there are only slight differences between both versions of Iberia01 when compared with observations.
All the datasets used in this work are publicly available. The Iberia01 dataset is publicly available through the DIGITAL.CSIC open science service (Herrera et al., 2019a, https://doi.org/10.20350/digitalCSIC/8641). Moreover, THREDDS remote access to this dataset is available from the Santander Climate Data Service, via the User Data Gateway (instructions at http://meteo.unican.es/udg-wiki, last access: 22 September 2019). The E-OBS v17 dataset is remotely available through the KNMI's THREDDS server (http://opendap.knmi.nl/knmi/thredds/e-obs/e-obs-catalog.html, last access: 22 September 2019), and a frozen version of the ensemble version E-OBS v17e is available through the Santander Climate Data Service (see above). Elevation data are taken from the Global 30 Arc-Second Elevation (USGS EROS Archive – GTOPO30; https://doi.org/10.5066/F7DF6PQS).
The R code needed to partially reproduce the results of this paper (for the remotely accessible datasets Iberia01 and E-OBS v17) is publicly available at https://github.com/SantanderMetGroup/notebooks (last access: 22 September 2019), building on the remote data services described above and in the climate4R R framework (Iturbide et al., 2019).
In this work a new gridded dataset for the Iberian Peninsula and the Balearic Islands based on a quality-controlled and dense station network has been described and compared with E-OBS v17, considering both the standard and the ensemble version of this product, to reflect and analyze the observational uncertainty related with both datasets.
It is shown that Iberia01 is able to reproduce the spatial pattern and intensity of both the mean and extreme regimes of precipitation and temperature, in terms of the weather indices defined in Table 1, including extreme events such as the illustrative case study that occurred on 4–5 November 1997 shown in Fig. 4. However, E-OBS v17 tends to underestimate the extremes and soften the spatial pattern of precipitation, in agreement with previous studies (Herrera et al., 2012). In the case of temperature, both datasets exhibit similar spatial patterns with the main differences appearing in the Guadalquivir and Guadiana basins and the Pyrenean range.
We have also used the ensemble version of E-OBS, E-OBS v17e, to define observational uncertainty and analyzed whether Iberia01 does differ significantly from the ensemble (i.e., it falls outside the observational uncertainty range). In this case, we conclude that both datasets are significantly different. In general, uncertainty values are large in all of Iberia, with the exception of a number of kernels where the uncertainty is small, corresponding to the stations used to build the E-OBS grid. For precipitation, significant differences – at a 10 % level – between both datasets were found for fewer than 25 % of days over the Iberian Peninsula. For temperature, a very inhomogeneous spatial pattern was obtained, with either a small (in most of the regions) or large fraction of significantly different days, thus indicating sensible regions for observational uncertainty.
The complex orography and the influence of both the Atlantic Ocean and the Mediterranean Sea modulate the precipitation over the Iberian Peninsula. This leads to particular regimes, such as the cold drop in the east coast, that a continental adjustment of the interpolation model is not able to reproduce, particularly when a low-dense observational network is considered. In this sense, the large increase in rain gauges considered in Iberia01, when compared with E-OBS, gives rise to a much improved precipitation rendering. In the case of temperature, although the observational network considered is similar in both cases, the pattern tends to be more orographic in E-OBS v17 due to the continental adjustment of the interpolation method that overrates this component, avoiding regional behaviors. In addition, the contribution of the observational network considered in France also has a clear effect on the interpolated value over the Pyrenees and the northeast of the Iberian Peninsula.
Note that the interpolation method, independent of the target resolution, is calibrated to reproduce the spatial dependence of the mean field of the target variable, which is usually greater than the grid resolution (approximately 1∘ in this case). Therefore, the effective resolution of purely interpolated gridded products is limited by this spatial value, which defines the size of the kernels used for the interpolation process. As a result, in order to properly evaluate the convection-permitting CORDEX simulations, other approaches like regional reanalysis (e.g., Häggmark et al., 2000) or methods combining interpolation and analysis such as the proposed by Quintana-Seguí et al. (2017) and Peral et al. (2017), among others, should be used.
The supplement related to this article is available online at: https://doi.org/10.5194/essd-11-1947-2019-supplement.
SH, JMG, and PMS conceived of the study; JMG, PMS, RMC, FES, and PV obtained and processed the Spanish and Portuguese observational datasets; SH implemented the code to perform the interpolation and the analysis and created the dataset and figures of the paper; SH, PMS, JMG, and RMC wrote the paper and all the authors revised the results.
The authors declare that there are not any competing interests.
The authors are grateful to the Portuguese Institute for Sea and Atmosphere (IPMA), the Portuguese Environmental Agency, and the Spanish Meteorological Agency (AEMET) for providing the needed observational data. We acknowledge the E-OBS dataset from the EU-FP6 ENSEMBLES and UERRA projects and the Copernicus Climate Change Service, as well as the data providers in the ECA&D project (https://www.ecad.eu, last access: 22 September 2019, Cornes et al., 2018).
This research has been partially funded by the Spanish R&D program MINECO/FEDER (grant no. CGL2015-66583-R, project MULTI-SDM), the Portuguese Foundation for Science and Technology – FCT (grant no. PTDC/GEOMET/7078/2014, SOLAR project; grant no. UID/GEO/50019/2019, Instituto Dom Luiz).
This paper was edited by Scott Stevens and reviewed by Shawn Marshall and one anonymous referee.
Adam, J. C., Clark, E. A., Lettenmaier, D. P., and Wood, E. F.: Correction of Global Precipitation Products for Orographic Effects, J. Climate, 19, 15–38, https://doi.org/10.1175/JCLI3604.1, 2006. a
Beguería, S., Vicente-Serrano, S. M., Tomás-Burguera, M., and Maneta, M.: Bias in the variance of gridded data sets leads to misleading conclusions about changes in climate variability, Int. J. Climatol., 36, 3413–3422, https://doi.org/10.1002/joc.4561, 2016. a, b
Belo-Pereira, M., Dutra, E., and Viterbo, P.: Evaluation of global precipitation data sets over the Iberian Peninsula, J. Geophys. Res.-Atmos., 116, D20101, https://doi.org/10.1029/2010JD015481, 2011. a
Cardoso, R., Soares, P., Miranda, P., and Belo-Pereira, M.: WRF high resolution simulation of Iberian mean and extreme precipitation climate, Int. J. Climatol., 33, 2591–2608, https://doi.org/10.1002/joc.3616, 2013. a
Cornes, R. C., van der Schrier, G., van den Besselaar, E. J. M., and Jones, P. D.: An Ensemble Version of the E-OBS Temperature and Precipitation Data Sets, J. Geophys. Res.-Atmos., 123, 9391–9409, https://doi.org/10.1029/2017JD028200, 2018. a, b
Craven, P. and Wahba, G.: Smoothing noisy data with spline functions, Numer. Math., 31, 377–403, 1979. a
Durand, Y., Brun, E., Merindol, L., Guyomarc'h, G., Lesaffre, B., and Martin, E.: A meteorological estimation of relevant parameters for snow models, Ann. Glaciol., 18, 65–71, https://doi.org/10.3189/S0260305500011277, 1993. a
Esteban-Parra, M., Rodrigo, F., and Castro-Diez, Y.: Spatial and temporal patterns of precipitation in Spain for the period 1880–1992, Int. J. Climatol., 18, 1557–1574, https://doi.org/10.1002/(SICI)1097-0088(19981130)18:14<1557::AID-JOC328>3.0.CO;2-J, 1998. a
Frei, C.: Interpolation of temperature in a mountainous region using nonlinear profiles and non-Euclidean distances, Int. J. Climatol., 34, 1585–1605, https://doi.org/10.1002/joc.3786, 2014. a, b, c
Frei, C., Christensen, J. H., Déqué, M., Jacob, D., Jones, R. G., and Vidale, P. L.: Daily precipitation statistics in regional climate models: Evaluation and intercomparison for the European Alps, J. Geophys. Res.-Atmos., 108, https://doi.org/10.1029/2002JD002287, 2003. a
Frick, C., Steiner, H., Mazurkiewicz, A., Riediger, U., Rauthe, M., Reich, T., and Gratzki, A.: Central European high-resolution gridded daily data sets (HYRAS): Mean temperature and relative humidity, Meteorol. Z., 23, 15–32, https://doi.org/10.1127/0941-2948/2014/0560, 2014. a
Giorgi, F., Jones, C., and Asrar, G. R.: Addressing climate information needs at the regional level: the CORDEX framework, World Meteorological Organization (WMO) Bulletin, 58, 175–183, 2009. a
Häggmark, L., Ivarsson, K.-I., Gollvik, S., and Olofsson, P.-O.: Mesan, an operational mesoscale analysis system, Tellus A, 52, 2–20, https://doi.org/10.3402/tellusa.v52i1.12250, 2000. a
Harris, I., Jones, P., Osborn, T., and Lister, D.: Updated high-resolution grids of monthly climatic observations – the CRU TS3.10 Dataset, Int. J. Climatol., 34, 623–642, https://doi.org/10.1002/joc.3711, 2014. a
Haylock, M., Hofstra, N., Klein-Tank, A., Klok, E. J., Jones, P., and New, M.: A European daily high-resolution gridded data set of surface temperature and precipitation for 1950–2006, J. Geophys. Res., 113, D20119, https://doi.org/10.1029/2008JD010201, 2008. a, b
Herrera, S.: Desarrollo, Validación Y Aplicaciones de Spain02: Una Rejilla de Alta Resolución de Observaciones Interpoladas Para Precipitación Y Temperatura en España, PhD thesis, Universidad de Cantabria, available at: http://www.meteo.unican.es/tesis/herrera (last access: 22 September 2019), 2011 (in Spanish). a, b, c
Herrera, S., Gutiérrez, J. M., Ancell, R., Pons, M. R., Frías, M., and Fernandez, J.: Development and analysis of a 50–year high–resolution daily gridded precipitation dataset over Spain (Spain02), Int. J. Climatol., 36, 74–85, https://doi.org/10.1002/joc.2256, 2012. a, b, c, d, e
Herrera, S., Fernández, J., and Gutierrez, J. M.: Update of the Spain02 gridded observational dataset for EURO-CORDEX evaluation: assessing the effect of the interpolation methodology, Int. J. Climatol., 36, 900–908, https://doi.org/10.1002/joc.4391, 2015. a, b, c
Herrera, S., Cardoso, R. M., Soares, P. M. M., Espírito-Santo, F., Viterbo, P., and Gutiérrez, J. M.: Iberia01: Daily gridded (0.1∘ resolution) dataset of precipitation and temperatures over the Iberian Peninsula, DIGITAL.CSIC, https://doi.org/10.20350/digitalCSIC/8641, 2019a. a, b
Herrera, S., Kotlarski, S., Soares, P. M. M., Cardoso, R. M., Jaczewski, A., Gutiérrez, J. M., and Maraun, D.: Uncertainty in gridded precipitation products: Influence of station density, interpolation method and grid resolution, Int. J. Climatol., 39, 3717–3729, https://doi.org/10.1002/joc.5878, 2019b. a, b, c
Hofstra, N., Haylock, M., New, M., and Jones, P.: Testing E-OBS European high-resolution gridded data set of daily precipitation and surface temperature, J. Geophys. Res., 114, D21101, https://doi.org/10.1029/2009JD011799, 2009. a
Hofstra, N., New, M., and McSweeney, C.: The influence of interpolation and station network density on the distributions and trends of climate variables in gridded daily data, Clim. Dynam., 35, 841–858, https://doi.org/10.1007/s00382-009-0698-1, 2010. a
Hutchinson, M.: Interpolation of rainfall data with thin plate smoothing splines – Part I: two dimensional smoothing of data with short range correlation, Journal of Geographic Information and Decision Analysis, 2, 139–151, 1998a. a
Hutchinson, M.: Interpolation of rainfall data with thin plate smoothing splines – Part II: analysis of topographic dependence, Journal of Geographic Information and Decision Analysis, 2, 152–167, 1998b. a
Iturbide, M., Bedia, J., Herrera, S., Baño-Medina, J., Fernández, J., Frías, M. D., Manzanas, R., San-Martín, D., Cimadevilla, E., Cofiño, A. S., and Gutiérrez, J. M.: The R-based climate4R open framework for reproducible climate data access and post-processing, Environ. Model. Softw., 111, 42–54, https://doi.org/10.1016/j.envsoft.2018.09.009, 2019. a
Jacob, D., Petersen, J., Eggert, B., Alias, A., Christensen, O., Bouwer, L., Braun, A., Colette, A., Déqué, M., Georgievski, G., Georgopoulou, E., Gobiet, A., Menut, L., Nikulin, G., Haensler, A., Hempelmann, N., Jones, C., Keuler, K., Kovats, S., Kröner, N., Kotlarski, S., Kriegsmann, A., Martin, E., van Meijgaard, E., Moseley, C., Pfeifer, S., Preuschmann, S., Radermacher, C., Radtke, K., Rechid, D., Rounsevell, M., Samuelsson, P., Somot, S., Soussana, J.-F., Teichmann, C., Valentini, R., Vautard, R., Weber, B., and Yiou, P.: EURO-CORDEX: new high-resolution climate change projections for European impact research, Reg. Environ. Change, 14, 563–578, https://doi.org/10.1007/s10113-013-0499-2, 2014. a
Johansson, B.: Areal Precipitation and Temperature in the Swedish Mountains: An Evaluation from a Hydrological Perspective, Hydrol. Res., 31, 207, https://doi.org/10.2166/nh.2000.0013, 2000. a
Johansson, B. and Chen, D.: The influence of wind and topography on precipitation distribution in Sweden: statistical analysis and modelling, Int. J. Climatol., 23, 1523–1535, https://doi.org/10.1002/joc.951, 2003. a
Jones, P. D., Wigley, T. M. L., and Kelly, P. M.: Variations in Surface Air Temperatures: Part 1. Northern Hemisphere, 1881–1980, Mon. Weather Rev., 110, 59–70, https://doi.org/10.1175/1520-0493(1982)110<0059:VISATP>2.0.CO;2, 1982. a
Jones, P. D., Raper, S. C. B., Bradley, R. S., Diaz, H. F., Kellyo, P. M., and Wigley, T. M. L.: Northern Hemisphere Surface Air Temperature Variations: 1851–1984, J. Climate Appl. Meteorol., 25, 161–179, https://doi.org/10.1175/1520-0450(1986)025<0161:NHSATV>2.0.CO;2, 1986a. a
Jones, P. D., Raper, S. C. B., and Wigley, T. M. L.: Southern Hemisphere Surface Air Temperature Variations: 1851–1984, J. Climate Appl. Meteorol., 25, 1213–1230, https://doi.org/10.1175/1520-0450(1986)025<1213:SHSATV>2.0.CO;2, 1986b. a
Kidd, C., Bauer, P., Turk, J., Huffman, G. J., Joyce, R., Hsu, K.-L., and Braithwaite, D.: Intercomparison of High-Resolution Precipitation Products over Northwest Europe, J. Hydrometeor., 13, 67–83, https://doi.org/10.1175/JHM-D-11-042.1, 2011. a
Klein Tank, A. M. G., Wijngaard, J. B., Können, G. P., Böhm, R., Demarée, G., Gocheva, A., Mileta, M., Pashiardis, S., Hejkrlik, L., Kern-Hansen, C., Heino, R., Bessemoulin, P., Müller-Westermeier, G., Tzanakou, M., Szalai, S., Pálsdóttir, T., Fitzgerald, D., Rubin, S., Capaldo, M., Maugeri, M., Leitass, A., Bukantis, A., Aberfeld, R., van Engelen, A. F. V., Forland, E., Mietus, M., Coelho, F., Mares, C., Razuvaev, V., Nieplova, E., Cegnar, T., Antonio López, J., Dahlström, B., Moberg, A., Kirchhofer, W., Ceylan, A., Pachaliuk, O., Alexander, L. V., and Petrovic, P.: Daily dataset of 20th-century surface air temperature and precipitation series for the European Climate Assessment, Int. J. Climatol., 22, 1441–1453, https://doi.org/10.1002/joc.773, 2002. a
Klok, E. J. and Klein Tank, A. M. G.: Updated and extended European dataset of daily climate observations, Int. J. Climatol., 29, 1182–1191, https://doi.org/10.1002/joc.1779, 2009. a, b
Kotlarski, S., Szabó, P., Herrera, S., Räty, O., Keuler, K., Soares, P. M., Cardoso, R. M., Bosshard, T., Pagé, C., Boberg, F., Gutiérrez, J. M., Isotta, F. A., Jaczewski, A., Kreienkamp, F., Liniger, M. A., Lussana, C., and Pianko-Kluczynska, K.: Observational uncertainty and regional climate model evaluation: A pan-European perspective, Int. J. Climatol., 39, 3730–3749, https://doi.org/10.1002/joc.5249, 2019. a
Krige, D. G.: A statistical approach to some basic mine valuation problems on the Witwatersrand, Journal of the Chemical, Metallurgical and Mining Society of South Africa, 52, 119–139, 1951. a
Lakatos, M., Szentimrey, T., Bihari, Z., and Szalai, S.: Creation of a homogenized climate database for the Carpathian region by applying the MASH procedure and the preliminary analysis of the data, Q. J. Hungarian Meteorol. Serv., 117, 143–158, 2013. a
Lorente, P., Hernández, E., Queralt, S., and Ribera, P.: The flood event that affected Badajoz in November 1997, Adv. Geosci., 16, 73–80, 2008. a
Lussana, C., Saloranta, T., Skaugen, T., Magnusson, J., Tveito, O. E., and Andersen, J.: seNorge2 daily precipitation, an observational gridded dataset over Norway from 1957 to the present day, Earth Syst. Sci. Data, 10, 235–249, https://doi.org/10.5194/essd-10-235-2018, 2018. a
Matheron, G.: Traité de Géostatistique appliquée, Tome 1–2, Editions Technip, Paris, France, 1962. a
MeteoSwiss: Documentation of MeteoSwiss Grid-Data Products: Daily Mean, Minimum and Maximum Temperature: TabsD, TminD, TmaxD, Tech. rep., Federal Office of Meteorology and Climatology MeteoSwiss, Federal Department of Home Affairs FDHA, Switzerland, available at: https://www.meteoswiss.admin.ch/content/dam/meteoswiss/de/service-und-publikationen/produkt/raeumliche-daten-temperatur/doc/ProdDoc_TabsD.pdf (last access: 22 September 2019), 2013a. a
MeteoSwiss: Documentation of MeteoSwiss Grid-Data Products: Daily Precipitation (final analysis): RhiresD, Tech. rep., Federal Office of Meteorology and Climatology MeteoSwiss, Federal Department of Home Affairs FDHA, Switzerland, available at: https://www.meteoswiss.admin.ch/content/dam/meteoswiss/de/service-und-publikationen/produkt/raeumliche-daten-niederschlag/doc/ProdDoc_RhiresD.pdf (last access: 22 September 2019), 2013b. a
Muñoz-Díaz, D. and Rodrigo, F. S.: Spatio-temporal patterns of seasonal rainfall in Spain (1912-2000) using cluster and principal component analysis: comparison, Ann. Geophys., 22, 1435–1448, https://doi.org/10.5194/angeo-22-1435-2004, 2004. a
Peral, C., Navascués, B., and Ramos, P.: Serie de precipitación diaria en rejilla con fines climáticos., Nota técnica de AEMET 24/2017, Ministerio de Agricultura y Pesca, Alimentación y Medio Ambiente – Agencia Estatal de Meteorología, C/ Leonardo Prieto Castro, 8, 28040 Madrid, available at: http://www.aemet.es/documentos/es/conocermas/recursos_en_linea/publicaciones_y_estudios/publicaciones/NT_24_AEMET/NT_24_AEMET.pdf (last access: 22 September 2019), 2017 (in Spanish). a
Prein, A. F. and Gobiet, A.: Impacts of uncertainties in European gridded precipitation observations on regional climate analysis, Int. J. Climatol., 37, 305–327, https://doi.org/10.1002/joc.4706, 2017. a
Quintana-Seguí, P., Le Moigne, P., Durand, Y., Martin, E., Habets, F., Baillon, M., Canellas, C., Franchisteguy, L., and Morel, S.: Analysis of Near-Surface Atmospheric Variables: Validation of the SAFRAN Analysis over France, J. Appl. Meteorol. Climatol., 47, 92–107, https://doi.org/10.1175/2007JAMC1636.1, 2008. a
Quintana-Seguí, P., Turco, M., Herrera, S., and Miguez-Macho, G.: Validation of a new SAFRAN-based gridded precipitation product for Spain and comparisons to Spain02 and ERA-Interim, Hydrol. Earth Syst. Sci., 21, 2187–2201, https://doi.org/10.5194/hess-21-2187-2017, 2017. a, b
Ramos, A. M., Trigo, R., Liberato, M. L., and Tomé, R.: Daily Precipitation Extreme Events in the Iberian Peninsula and Its Association with Atmospheric Rivers, J. Hydrometeorol., 16, 579–597, https://doi.org/10.1175/JHM-D-14-0103.1, 2015. a
Ramos, C. and Reis, E.: Floods in southern Portugal: their physical and human causes, impacts and human response, in: Mitigation and Adaptation Strategies for Global Change, 7, 267–284, https://doi.org/10.1023/A:1024475529524, 2002. a
Rauthe, M., Steiner, H., Riediger, U., Mazurkiewicz, A., and Gratzki, A.: A Central European precipitation climatology – Part I: Generation and validation of a high-resolution gridded daily data set (HYRAS), Meteorol. Z., 22, 235–256, https://doi.org/10.1127/0941-2948/2013/0436, 2013. a
Rudolf, B., Hauschild, H., Rueth, W., and Schneider, U.: Terrestrial Precipitation Analysis: Operational Method and Required Density of Point Measurements, in: Global Precipitations and Climate Change, edited by: Desbois, M. and Désalmand, F., Springer Berlin Heidelberg, Berlin, Heidelberg, 173–186, 1994. a
Sun, Q., Miao, C., Duan, Q., Ashouri, H., Sorooshian, S., and Hsu, K.-L.: A Review of Global Precipitation Data Sets: Data Sources, Estimation, and Intercomparisons, Rev. Geophys., 56, 79–107, https://doi.org/10.1002/2017RG000574, 2018. a
Trenberth, K. E., Dai, A., van der Schrier, G., Jones, P. D., Barichivich, J., Briffa, K. R., and Sheffield, J.: Global warming and changes in drought, Nat. Clim. Change, 4, 17–22, https://doi.org/10.1038/nclimate2067, 2014. a
Uboldi, F., Lussana, C., and Salvati, M.: Three-dimensional spatial interpolation of surface meteorological observations from high-resolution local networks, Meteorol. Appl., 15, 331–345, https://doi.org/10.1002/met.76, 2008. a
van den Besselaar, E. J. M., Haylock, M. R., van der Schrier, G., and Klein Tank, A. M. G.: A European daily high-resolution observational gridded data set of sea level pressure, J. Geophys. Res.-Atmos., 116, D11110, https://doi.org/10.1029/2010JD015468, 2011. a
Vidal, J.-P., Martin, E., Franchisteguy, L., Baillon, M., and Soubeyroux, J.-M.: A 50-year high-resolution atmospheric reanalysis over France with the Safran system, Int. J. Climatol., 30, 1627–1644, https://doi.org/10.1002/joc.2003, 2010. a
Wahba, G.: Spline models for Observational Data, in: CBMS-NSF Regional Conference Series in Applied Mathematics, Society for Industrial and Applied Mathematics, Philadelphia, https://doi.org/10.1137/1.9781611970128, 1990. a
Whiteman, C. D.: Breakup of Temperature Inversions in Deep Mountain Valleys: Part I. Observations, J. Appl. Meteorol., 21, 270–289, https://doi.org/10.1175/1520-0450(1982)021<0270:BOTIID>2.0.CO;2, 1982. a
Whiteman, C. D.: Observations of Thermally Developed Wind Systems in Mountainous Terrain, American Meteorological Society, Boston, MA, 5–42, https://doi.org/10.1007/978-1-935704-25-6_2, 1990. a
Whiteman, C. D. and McKee, T. B.: Breakup of Temperature Inversions in Deep Mountain Valleys: Part II. Thermodynamic Model, J. Appl. Meteorol., 21, 290–302, https://doi.org/10.1175/1520-0450(1982)021<0290:BOTIID>2.0.CO;2, 1982. a