the Creative Commons Attribution 4.0 License.
the Creative Commons Attribution 4.0 License.
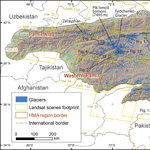
A consistent glacier inventory for Karakoram and Pamir derived from Landsat data: distribution of debris cover and mapping challenges
Tobias Bolch
Philipp Rastner
Tazio Strozzi
Frank Paul
Knowledge about the coverage and characteristics of glaciers in High Mountain Asia (HMA) is still incomplete and heterogeneous. However, several applications, such as modelling of past or future glacier development, run-off, or glacier volume, rely on the existence and accessibility of complete datasets. In particular, precise outlines of glacier extent are required to spatially constrain glacier-specific calculations such as length, area, and volume changes or flow velocities. As a contribution to the Randolph Glacier Inventory (RGI) and the Global Land Ice Measurements from Space (GLIMS) glacier database, we have produced a homogeneous inventory of the Pamir and the Karakoram mountain ranges using 28 Landsat TM and ETM+ scenes acquired around the year 2000. We applied a standardized method of automated digital glacier mapping and manual correction using coherence images from the Advanced Land Observing Satellite 1 (ALOS-1) Phased Array type L-band Synthetic Aperture Radar 1 (PALSAR-1) as an additional source of information; we then (i) separated the glacier complexes into individual glaciers using drainage divides derived by watershed analysis from the ASTER global digital elevation model version 2 (GDEM2) and (ii) separately delineated all debris-covered areas. Assessment of uncertainties was performed for debris-covered and clean-ice glacier parts using the buffer method and independent multiple digitizing of three glaciers representing key challenges such as shadows and debris cover. Indeed, along with seasonal snow at high elevations, shadow and debris cover represent the largest uncertainties in our final dataset. In total, we mapped more than 27 800 glaciers >0.02 km2 covering an area of 35 520±1948 km2 and an elevation range from 2260 to 8600 m. Regional median glacier elevations vary from 4150 m (Pamir Alai) to almost 5400 m (Karakoram), which is largely due to differences in temperature and precipitation. Supraglacial debris covers an area of 3587±662 km2, i.e. 10 % of the total glacierized area. Larger glaciers have a higher share in debris-covered area (up to >20 %), making it an important factor to be considered in subsequent applications (https://doi.org/10.1594/PANGAEA.894707).
- Article
(17883 KB) - Full-text XML
-
Supplement
(676 KB) - BibTeX
- EndNote
Glacier outlines and their accompanying attributes as recorded in glacier inventories provide the baseline for climate change impact assessments (Vaughan et al., 2013), numerous hydrology-related calculations that consider water resources and their changes (e.g. drinking water, irrigation, hydropower production, run-off, sea-level rise) (e.g. Kraaijenbrink et al., 2017; Bliss et al., 2014), climatic characteristics (Sakai et al., 2015), or modelling of past and future glacier changes (e.g. Huss and Hock, 2015). All of this applies to most catchments of High Mountain Asia (HMA), although some of their glacier meltwater does not directly contribute to sea-level rise as related rivers end in endorheic basins (e.g. Tarim basin, Aral Sea basin).
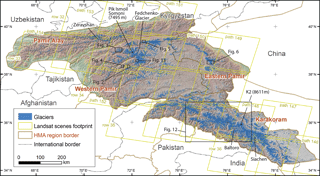
Figure 1The study region in High Mountain Asia (HMA) covers four mountain ranges. Annotations denote locations of figures in the paper and points of orientation. International borders are tentative only as they are disputed in several regions.
When glacier outlines are of poor quality, related hydrologic calculations at the catchment scale (e.g. Immerzeel et al., 2010) have higher uncertainties. This situation has improved significantly during recent years, during which several large-scale glacier inventories for HMA have been published – among others the Glacier Area Mapping for Discharge from the Asian Mountains (GAMDAM) inventory (Nuimura et al., 2015) and the second Chinese Glacier Inventory (CGI, Guo et al., 2015). Because the currently available version of GAMDAM only partially considered ice cover on steep slopes and the CGI only covered Chinese territory, a homogeneous basis for precise calculations covering all relevant catchments at the large scale is still missing. As both inventories have been combined for version 5.0 and transferred unchanged to version 6.0 of the Randolph Glacier Inventory (RGI) (Arendt et al., 2015; RGI Consortium, 2017), the related regional-scale calculations using this version (e.g. Brun et al., 2017; Kraaijenbrink et al., 2017; Dehecq et al., 2015; Kääb et al., 2015) still suffer from uncertainties which stem from outlines of varying quality.
To overcome this situation, several regional-scale studies digitized glacier outlines themselves (e.g. Rankl and Braun, 2016; Minora et al., 2016) to have better control on data quality. But these again applied different criteria to delineate glacier extents and are thus not comparable to the existing datasets, making change assessment difficult. On the other hand, the Karakoram and Pamir regions are characterized by a high number of surge-type glaciers (Bhambri et al., 2017; Copland et al., 2011; Kotlyakov et al., 2008) with often strong geometric changes over a short period of time (Paul, 2015; Quincey et al., 2015). A precise inventory is key to determine and maybe better understand such changes. Moreover, the large number of debris-covered glaciers in the region (Herreid et al., 2015; Minora et al., 2016) results in interpretation differences and is a large source of uncertainty.
The correct delineation of debris is also important for detecting very subtle past glacier changes (Scherler et al., 2011b) and to correctly model future glacier development (e.g. Kraaijenbrink et al., 2017; Shea et al., 2015), as surface mass balance of ice under a supraglacial debris layer is different from that of clean ice (Ragettli et al., 2015, 2016; Brock et al., 2010; Nicholson and Benn, 2006). The information on debris extent should thus be included in large-scale glacier inventories (Kraaijenbrink et al., 2017).
The main objective of this study is to present a consistent dataset of glacier coverage for the higher and more extensively glacierized mountain ranges – Pamir Alai, the western and eastern Pamir, and the Karakoram of HMA (Fig. 1) – along with the spatial distribution of debris cover for the years around 2000. In addition, we present a structured overview of the difficulties related to glacier mapping in this region as well as an estimate of the respective uncertainties. Key challenges are the entity assignment, as many glaciers in this region are of a surge type, and tributary glaciers can be either connected to or disconnected from a larger main glacier; the already mentioned mapping of debris-covered glacier ice; and the differentiation of debris-covered glaciers from rock glaciers that are increasingly abundant towards the north and the drier east of the study region.
2.1 Location and glacier characteristics
The study area comprises a major share of the western part of HMA (Fig. 1). It stretches over ∼300 000 km2 and fully covers the mountain ranges of (i) Pamir and its northern neighbour Pamir Alai in Uzbekistan, Turkmenistan, Kyrgyzstan, Tajikistan, Afghanistan and China and (ii) the Karakoram in Pakistan, India, and China.
The mountain ranges reach their highest elevations between 5500 m (Pamir Alai) and more than 8600 m (Karakoram, Hindu Kush), with K2 being the highest peak with 8611 m. Glaciers are found from ∼2300 m a.s.l. up to the highest peaks. The central Karakoram and inner Pamir are two of the most heavily glacierized mountain regions worldwide and include some extremely large glaciers such as Baltoro, Siachen, and Fedchenko with sizes of about 810, 1094, and 573 km2, respectively. High peaks and deeply incised valleys create an extreme topographic relief that is also reflected in the geometry of the glaciers, the majority of which are valley glaciers. In the Pamir, numerous cirques are also present, and hanging glaciers can be found at high elevations in all regions. Larger, flat, high-altitude accumulation areas are rare and can only be found for some of the largest glaciers. Due to the steep terrain, most glaciers are partly fed by avalanches from the surrounding steep valley walls (Dobreva et al., 2017; Iturrizaga, 2011; Hewitt, 2011; Scherler et al., 2011a). This also causes an abundance of glaciers with partly or completely debris-covered tongues. Whereas debris cover makes glacier mapping difficult, the strong geometric changes in surging glaciers create additional challenges for glacier inventory compilation. Rock glaciers are present in all periglacial environments and are abundant also in our study region.
2.2 Climate
The climate of the study region can be subdivided into two major and independent regimes that define both the thermal and the moisture conditions. The Pamir and the major part of the Karakoram are predominantly influenced by westerly air flows throughout the year (e.g. Bookhagen and Burbank, 2010; Singh et al., 1995); towards the south-east the influence of the Indian summer monsoon (approx. June–September) becomes continuously stronger (e.g. Archer and Fowler, 2004). In general, outer western areas of the mountain ranges (Pamir Alai, western Pamir, Hindu Kush, south-eastern Karakoram) receive more precipitation, whereas further inland and to the east (eastern Pamir, central Karakoram) the climate becomes drier and more continental (Lutz et al., 2014).
For most of the study region, the main share of precipitation falls in winter and spring (Archer and Fowler, 2004; Bookhagen and Burbank, 2010). In regions dominated by westerlies, winter precipitation is mostly advective, whereas convective precipitation plays an important role in drier regions and occurs predominantly in spring and summer (Böhner, 2006). In the eastern Pamir and also the central–eastern Karakoram, the precipitation peak is shifted towards spring, with important annual precipitation shares even in summer (Zech et al., 2005; Aizen et al., 2001, 1997). In monsoon-dominated regions (the border is roughly at 77∘ E, Bookhagen and Burbank, 2010) a mixture of western disturbances and monsoon dominates in summer (Maussion et al., 2014; Böhner, 2006; Bookhagen and Burbank, 2010; Archer and Fowler, 2004). Little is known about temperature and precipitation at the elevation of glaciers; stations in valley floors yield amounts between 70 and 300 mm yr−1 (Seong et al., 2009; Archer and Fowler, 2004). Precipitation amounts along the edge of mountain ranges and in high altitudes are largely unknown, but can be substantially higher (“by a factor of ten”: Wake, 1989; Immerzeel et al., 2015), which is also suggested by snow station measurements showing snow accumulations of >1000 mm w.e. (millimetre water equivalent) around 4000 m in the Hunza basin (Winiger et al., 2005).
2.3 Glacier changes
Glaciers in the Karakoram have gained considerable attention during the last decade. The “Karakoram anomaly” that was first identified by Hewitt (2005), based on the observed unusual behaviour of glacier termini, is now a major research topic, and numerous studies have investigated the recent and longer-term evolution of climate, changes in glacier extent and volume, and glacier dynamics. These studies suggest that since the 1970s the extent and mass of glaciers in the central Karakoram have on average hardly changed (Bolch et al., 2017; Bajracharya et al., 2015; Bhambri et al., 2013), which also applies to the beginning of the 21st century (Lin et al., 2017; Brun et al., 2017; Gardelle et al., 2013; Gardner et al., 2013; Kääb et al., 2012), while glaciers in the mountain ranges of the Hindu Kush and Hindu Raj are mostly retreating (Sarıkaya et al., 2013; Haritashya et al., 2009). However, the patterns of climate-induced glacier change are not to be confounded with the strong geometric changes observed for the abundant surge-type glaciers in the region that might occur independent of climatic forcing (Bhambri et al., 2017; Paul, 2015; Quincey et al., 2015; Rankl et al., 2014; Copland et al., 2011). Glaciers in the eastern Pamir were on average almost in balance, as in the Karakoram (Brun et al., 2017; Holzer et al., 2016). In the western Pamir, glacier volume evolution seems to be more negative, but is for the first decade of the 2000s still relatively modest (Brun et al., 2017); moreover, satellite images of the past two decades reveal that many glaciers in this region have surged (e.g. Wendt et al., 2017). However, many of the non-surge-type glaciers in the Pamir have been continuously retreating and losing area and mass since the Little Ice Age (Holzer et al., 2016; Khromova et al., 2006; Shangguan et al., 2006).
As a mapping basis we have used six Landsat 5 TM and 22 Landsat 7 ETM+ Level 1T scenes, with the latter offering a 15 m panchromatic band for improved mapping quality (Table 1). Additionally, we have also used coherence images derived from the Advanced Land Observing Satellite 1 (ALOS-1) Phased Array type L-band Synthetic Aperture Radar 1 (PALSAR-1) scenes acquired around 2007 to aid in mapping the debris-covered glacier parts (Atwood et al., 2010; Frey et al., 2012) and the global digital elevation model (GDEM) version 2 from ASTER (hereafter referred to as GDEM2; United States Geological Survey, 2018a). The TM and ETM+ scenes served as a basis for glacier mapping while the coherence images were used for corrections of debris-covered glacier areas. Moreover, satellite images available in Google Earth served as a visual control for outline detection, with data originating mainly from very high-resolution optical sensors such as QuickBird, Worldview, Pléiades 1A and 1B, and SPOT 6 and SPOT 7 (GoogleEarth 2017); unfortunately these were not available for all regions.
Coherence images have been produced from ALOS-1 PALSAR-1 scenes usually separated by 46 days and acquired over summer (Table 2). The processing of the images takes into account a number of effects (e.g. sensor geometry, radiometric calibration, frequency interference) that influence the noise of the radar interferogram. The remaining decorrelation can be ascribed to changes in landscape surface properties, e.g. due to movement of landforms. More details on the processing line can be found in Frey et al. (2012).
A DEM is needed to retrieve drainage divides and topographic information for a glacier inventory. The freely available SRTM DEM (United States Geological Survey, 2018b) and the GDEM2 (both with 30 m cell size) could have been used for this purpose. The optical GDEM2 has a potentially reduced quality in low-contrast regions such as shadow and snow-covered accumulation regions, but it has been averaged from scenes acquired over a 12-year period strongly reducing these factors. On the other hand, the SRTM DEM has a precise acquisition date (February 2000) but suffers from data voids in steep terrain due to radar shadow and layover, which affect the final quality over glacierized areas, in particular when using void-filled versions. A direct comparison (subtraction) of both DEMs as recommended by Frey and Paul (2012) confirmed these differences. We finally decided to work only with the GDEM2 as it had fewer data voids along mountain crests (important to derive correct drainage divides) and because it is spatially consistent; i.e. data voids over glaciers in the SRTM DEM did not have to be filled with some other DEM data (which is beneficial for deriving consistent topographic information and increases traceability). The vertical accuracy was found to be around 9 m (probably higher in steep terrain) and similar for both DEMs (Satgé et al., 2015). For consistency, the glacier separation and all subsequent topographic analysis of glacier elevation, slope, and aspect are thus based on the GDEM2.
4.1 Glacier mapping
We applied the well-established semi-automatic band ratio method (Paul et al., 2002) to classify glaciers (the clean-ice and snow part), taking advantage of the reflection contrast between snow–ice and other land surfaces in the red and short-wave infrared (SWIR) parts of the electromagnetic spectrum, corresponding to Landsat TM or ETM+ bands 3 (red) and 5 (SWIR). An individual, scene-adjusted band ratio threshold between 1.5 and 3.5 is applied to separate glaciers and snow from other terrain and to compute a binary raster image, which is smoothed using a 3 by 3 majority filter and is then converted to a vector file for further editing.
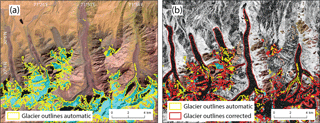
Figure 2Mapping of heavily debris-covered tongues. (a) False-colour image overlaid by raw outlines. (b) PALSAR-1 coherence images overlaid by raw (yellow) and corrected (red) outlines. The decorrelated image areas are shown in black.
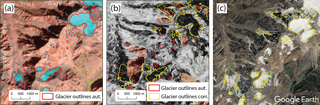
Figure 3Elongated rock glaciers that are (almost) connected to the active glacier tongue are hard to distinguish. (a) False-colour Landsat image overlaid by raw outlines. (b) PALSAR-1 coherence images are fuzzy and potentially misleading in this case. (c) High-resolution imagery is decisive for a correct decision, because rock glaciers can be visually distinguished from the glacier tongue.
Due to the spectral similarity of debris on and off glaciers, there is so far no method available to automatically map debris cover over a large set of glaciers using optical satellite imagery alone. Hence, several studies have tested combined approaches that generally include topographic information derived from a DEM and other data (Robson et al., 2016; Racoviteanu and Williams, 2012; Rastner et al., 2014; Bolch et al., 2007; Paul et al., 2004). However, all methods require time-consuming manual post-processing, and the quality of the results depends to some extent on the experience of the analyst.
As debris-covered glacier tongues can be difficult to identify visually, even when using high-resolution images (Paul et al., 2013), we have utilized ALOS-1 PALSAR-1 coherence images. Such images have also been used for glacier mapping in Alaska (Atwood et al., 2010) or as supportive means for correcting automatically derived glacier outlines in the western Himalaya by Frey et al. (2012). The coherence images were primarily used to detect the existence of debris-covered glacier tongues, while the exact positions of the glacier margin and terminus were detected using the optical Landsat image. The data are combined to account for the time difference of up to 9 years between coherence and Landsat images. Non-surging debris-covered glaciers commonly change little during such periods (Scherler et al., 2011b), especially in this region (e.g. Baltoro glacier has been stable for at least 25 years) (Paul, 2015).
The elevation of a glacier can be described by different elevation parameters. One that is well suited for a comparison between different glacier types and sizes as well as an indication for climatic differences is the median elevation, which is indicative of the equilibrium line altitude (ELA) at a balanced mass budget (Braithwaite and Raper, 2009) and similar to the midpoint elevation (Raper and Braithwaite, 2009), that has been used in several studies to characterize glaciers (e.g. Haeberli and Hoelzle, 1995) and climatic conditions, primarily precipitation amounts (e.g. Sakai et al., 2015; Bolch et al., 2013).
4.2 Mapping challenges and solutions
The main challenges for mapping glaciers in this region are the correct delineation of debris-covered glacier parts (including their separation from rock glaciers), seasonal snow, cast shadow, and orographic clouds. In the following, we shortly describe these challenges and present the techniques applied to overcome them.
Debris cover. The main reason for extensive debris cover on glaciers is steep/high topography with ice-free rock walls leading to rock falls and avalanches onto the glacier surface (e.g. Herreid et al., 2015; Scherler et al., 2011a; Paul et al., 2004). Apart from the central Karakoram, most regions exhibit glacier recession, which is another factor for increasing debris coverage on the glacier surface (Rowan et al., 2015; Kirkbride and Deline, 2013).
The debris-covered glacier area in this study was mapped manually by editing the automatically derived clean-ice outlines. Key difficulties in identifying these regions are the small solar incidence angle at these latitudes (reducing topographic contrast in the terminus region), unclear boundaries between supra-glacial debris and moraines or rock glaciers, and debris in shadow (e.g. Bishop et al., 2014). Heavily debris-covered glacier tongues are often in contact with lateral or frontal moraines (Figs. 2, 3, and 4) and their composition is very similar, leading to similar spectral properties and the need of applying other measures for identification. Whereas human recognition has the ability to trace very subtle features for identification of debris on glaciers, the 30 m spatial resolution of Landsat images is often too coarse for a clear assignment.
In this study we mostly relied on the ALOS-1 PALSAR-1 coherence images for identifying the margins of debris-covered glaciers (Fig. 2). Their usability decreases with decreasing glacier size as well as when the images become “fuzzy” and glacier margins less clear; in high-alpine terrain this could happen, as also glacier-free terrain can change: permafrost landforms such as rock glaciers, talus ramparts, and moraines complicate the proper identification of glaciers (Figs. 2, 3, and 4). In such cases we also used the very high-resolution imagery available in Google Earth and similar tools for identification. Furthermore, multi-temporal data aided in terminus identification by either providing better contrast or by using them in animations (Paul, 2015). In some cases it was also possible to consider glaciological relations for a first approximation of glacier extent. For example, a tiny accumulation area would likely not support a large glacier tongue (and vice versa).
In contrast to glaciers – massive bodies of ice originating from continuous snow accumulation – rock glaciers probably have a different genesis: they develop in a permafrost environment either from ice-cored moraines or on talus slopes that provide constant debris input, and they commonly have a higher debris content than glaciers (Berthling, 2011; Haeberli et al., 2006; Barsch, 1996). Especially towards the cold–dry regions of central Asia, rock glaciers of both types are increasingly abundant (Bolch and Gorbunov, 2014; Gorbunov and Titkov, 1989). In particular, moraine-derived rock glaciers challenge the analyst as there is often a continuous transition between the glacier and the rock glacier, making it hard to define a divide (cf. Monnier and Kinnard, 2015). A well-developed rock glacier can in principle be distinguished from a debris-covered glacier by characteristic surface patterns such as the arc-shaped transverse ridge and furrow structure instead of the longitudinal debris striations and supra-glacial ponds found on most debris-covered glaciers (Bishop et al., 2014; Bodin et al., 2010). However, identifying such differences using remotely sensed imagery requires a spatial resolution better than 15 m (Paul et al., 2003) and might not work at all when rock glaciers are not well developed. We separated debris-covered glaciers from rock glaciers based on interpreting the above data sources (Google Earth, coherence images) and their known morphological characteristics. In the cases where no clear boundary could be found, we followed a more conservative interpretation that might have resulted in a potential underestimation of the debris-covered glacier area.
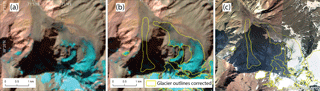
Figure 5Glacier detection in shadow with the supporting input of high-resolution Google Earth images.
Seasonal snow. Seasonal snow can obscure the underlying glacier ice and is included in the automatic classification result due to the similar reflection properties of snow and ice. Seasonal snow and clouds also required consideration of scenes from years other than 2000. For larger glaciers with a low-lying terminus, it would have been possible to adjust the (snow-free) terminus to the year 2000 scene; we have not applied this in favour of temporarily consistent glacier outlines. Interestingly, for some regions it was much harder to find satellite scenes with satisfying snow conditions than for others. It was particularly difficult for the eastern Pamir and some parts of the northern–central Karakoram, potentially resulting in related higher area uncertainties for the accumulation areas of the glaciers. Our strategy to reduce the impact of wrongly mapped seasonal snow was threefold: we applied a size filter of 0.02 km2 to remove the smallest snow patches; snow attached to glaciers was manually removed after visual inspection, and in some regions a different scene (with less snow but possibly more clouds) was chosen to improve results (see Table 1). Despite these measures, we assume that glacier area in this inventory is likely overestimated due to the inclusion of seasonal snow.
Shadow. Cast shadows from mountains decrease reflection values, partly down to near-zero. This results in considerable noise in this region for a band ratio using a red (or near infrared) band. As TM band 1 (blue) is strongly influenced by atmospheric scattering, ice and snow in shadow are much more visible and can be distinguished using an additional threshold (e.g. Paul and Kääb, 2005). Although the shadow problem is less pronounced in lower latitudes due to the higher solar elevation angle, it is still a problem in the study region due to the high and steep terrain. We have used the additional blue band to map glaciers in shadow automatically or applied manual corrections on contrast-enhanced true-colour composites in case the automated refinement was not successful. We also analysed scenes from a different date or another sensor (including very high-resolution imagery as available in Google Earth and similar tools) to reveal if glaciers are possibly present (Fig. 5). However, this is time-consuming and in some regions images are not available or do not meet the criteria for glacier identification (e.g. due to snow cover). In the cases where glaciers in shadow could not be identified, a related underestimation of glacier area results.
Cloud coverage. Cloud-free scenes were available for most of the study region. In the few cases when cloud cover prevented glacier mapping, the problem was solved multi-temporally by using additional scenes from years close to the year 2000 (Paul et al., 2017). In some regions, scenes with high cloud coverage and possible precipitation events were followed by scenes with extensive snow coverage, so that we had to use scenes from other years. The entire study region is thus a mosaic of many individual scenes (see Table 1).
4.3 Calculating the debris-covered area share of glaciers
For calculating the area share of debris cover, we decided to consider only the ablation areas of glaciers (i.e. the region below their median elevation), because debris deposited in the accumulation area should emerge on the glacier surface only below the ELA (Braithwaite and Raper, 2009; Braithwaite and Müller, 1980). We distinguished the debris cover from snow and ice surfaces by applying a constant threshold of 2.0 to all band ratio images from Landsat TM bands 3 and 5 (red and SWIR) and subtracted the resulting clean-ice glacier map from the corrected glacier map. The threshold was found empirically with satisfying results for all scenes from TM and ETM+ sensors. Changing the threshold by ±0.2 changed the result by less than the mapping uncertainties (∼5 % for debris-covered areas, see Sect. 6).
4.4 Glacier definition and separation using drainage divides
We based the mapping and division of glaciers on the Global Land Ice Measurements from Space (GLIMS) definition of a glacier (Raup and Khalsa, 2007), stating that a glacier includes “all tributaries and connected feeders that contribute ice to the main glacier, plus all debris-covered parts of it”. For the sake of consistency with earlier datasets and the GLIMS definition, surge-type tributaries were not separated from the main glacier tongue, even if they contribute only during an active surge phase. The preparation of a dataset where these short-term tributaries are properly separated is worthwhile but a considerable extra effort. Stagnant ice masses (e.g. from a former surge) that were still connected to the glacier tongue were mapped as part of the glacier. In cases where the active glacier has clearly receded away from the stagnant “dead” ice (e.g. after a surge phase), only the active glacier was mapped. In contrast to this definition, the surging Bivachny glacier tongue was separated from Fedchenko glacier in the confluence region although one can argue that Bivachny is a connected feeder (see Fig. 6 in Wendt et al., 2017). A size filter of 0.02 km2 was applied to remove small seasonal snowfields and remaining noise. Automatically classified polygons larger than this are considered as glaciers in this inventory, but this does not mean that all seasonal or perennial snowfields have been excluded, nor that some of the mapped glaciers are in fact perennial snow.
Glacier complexes – at least two glaciers connected in their accumulation areas – can be split into single glaciers using drainage divides derived from a DEM. This is performed in two steps. Firstly, raw drainage basins are calculated by watershed analysis using a flow direction grid derived from a sink-filled DEM. Afterwards, overlying raw basins are merged to one basin polygon per glacier considering pour points and a buffer (Falaschi et al., 2017; Kienholz et al., 2013; Bolch et al., 2010). This approach proved to be robust even for the large regions of the Karakoram and Pamir as in general glaciers are divided by very steep mountain crests. Secondly, manual corrections were performed, which took about 90 % of the total processing time. Gross errors were improved using a colour-coded flow direction grid in the background, a hillshade, the original Landsat scenes, and sometimes oblique views in Google Earth. We manually assigned the same ID to separated glacier polygons that were obviously linked by mass transport (e.g. regenerated glaciers).
4.5 Uncertainty estimation
Since there is no ground truth or reference data for any larger set of glaciers in the study region, we calculated uncertainties for the relevant input data rather than accuracy (Paul et al., 2017).
Glacier mapping uncertainties originate from the coverage of glaciers by seasonal snow and/or debris, shadow, and clouds. These need to be corrected manually (on-screen digitizing) by a well-trained analyst. According to the literature, the uncertainty of automatically and manually digitized glacier outlines (clean ice only) ranges between 2 % and 5 % and is dependent on glacier size (Paul et al., 2013, 2011; Andreassen et al., 2008; Bolch and Kamp, 2006). Paul et al. (2013) estimated uncertainties using a sample of manually and automatically digitized glaciers from a number of experts and found a mean standard deviation of ∼5 %. Other studies (Bolch et al., 2010; Granshaw and Fountain, 2006) have used a buffer-based estimate, where the final uncertainty depends on the pixel size of the input image. The study by Paul et al. (2017) suggested a tiered system of uncertainty assessment related to workload. We used three of the methods: (1) fixed uncertainty values applied to all glaciers, (2) the buffer method with different buffer sizes for clean and debris-covered glacier parts, and (3) independent multiple digitization of outlines by all analysts for three difficult debris-covered glaciers.
For (1), we applied an uncertainty of ±2 % for the clean ice and ±5 % for the debris-covered ice. This is an upper boundary estimate, because it does not account for the overlapping area of the two surface types. For the buffer method (2) we applied an uncertainty of pixel for clean-ice parts and ±1 pixel for debris-covered parts. This also provides an upper-bound estimate and we use the standard deviation of the uncertainty distribution for the estimate, as a normal distribution can be assumed for this type of mapping error. It is applied to glacier complexes excluding overlapping areas as well as the border of clean and debris-covered ice of the same glacier. Due to the abundant debris-covered glaciers in the study region, we also performed method (3) to obtain a more realistic uncertainty estimate for the analysts participating in the outline correction. They manually corrected the outlines of three example glaciers from different regions three times (Glacier 1: 38∘34.4′ N, 72∘12.8′ E; Glacier 2: 39∘38.8′ N, 69∘41.9′ E; Glacier 3: 36∘0.3′ N, 75∘14.6′ E), with differing additional information being considered (e.g. coherence images and Google Earth imagery). The glaciers are of different size and contain a substantial debris-covered part; they also feature difficulties of moraines, glacier confluences, and cast shadow.
As not all satellite scenes used to compile the inventory are from the same year, there is a certain temporal uncertainty introduced. However, glacier changes within the ±2-year difference to the target year 2000 are likely within the uncertainty of the glacier outlines and should thus not matter. The actual date information is given for each glacier in the attribute table.
5.1 Basic statistics
We identified 27 877 glaciers (larger than 0.02 km2) in the four HMA regions covering 35 519.7±1958 km2; western Pamir and Karakoram each host over 10 000 glaciers, whereas the other regions contain 2000–4000. As in other larger regions where detailed glacier inventories have been compiled (e.g. Kienholz et al., 2015; Guo et al., 2015; Pfeffer et al., 2014; Le Bris et al., 2011; Bolch et al., 2010), the histogram is strongly skewed towards small glaciers (Fig. 7).
Only 3.5 % (985) of all glaciers are larger than 5 km2, and most of them are located in the Karakoram. In total, they cover over 60 % of the glacierized area. On the other hand, 83 % (23 048) of all glaciers are smaller than 1 km2 but cover only ∼15 % of the total area. The mean glacier size is 1.29 km2, with large differences between the regions: from 0.57 km2 in the Pamir Alai to 2.07 km2 in the Karakoram (Table 3). The average median elevation is 4978 and 5169 m for all glaciers and glaciers larger than 5 km2, respectively, and differs by only a few metres from the mean elevation.
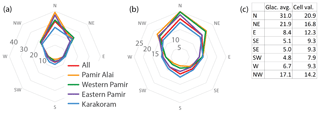
Figure 8Glacier orientation of the different HMA regions. (a) shows the values based on average glacier aspect; (b) is based on the 30 m raster cells. Lower elevations tend to have a higher share of the north-facing glacier area. The respective numbers of “All” are given in the table (c).
5.2 Extremes
In the Pamir Alai, the largest glacier is Zeravshan glacier, with an area of 106.3±6.7 km2, which is 3 times larger than the second largest. Zeravshan glacier stretches over 2600 m from 2800 to 5400 m, close to the highest elevations in the Pamir Alai range. The largest elevation range is covered by Tandykul glacier (39∘27′ N, 71∘8′ E) 50 km further east, with almost 3000 m (2450–5400 m). Its heavily debris-covered tongue lies in a deep valley that is well shielded from the south. Overall, only a few larger valley glaciers (19 larger than 10 km2) have several large tributaries.
In the western Pamir, Fedchenko glacier is by far the largest with 573±19.5 km2 (not including Bivachny glacier with 170±8.5 km2 since it is in contact but not contributing). Bivachny glacier starts right below the summit of Ismoil Somoni Peak (formerly known as Pik Kommunízma, 7495 m) and terminates at about 3420 m, whereas Fedchenko glacier stretches from Independence Peak, with an elevation of 6940 m, down to below 2900 m; hence, both glaciers are spanning an elevation range of over 4000 m. The region hosts several large glacier systems (13 larger than 50 km2, 108 larger than 10 km2) that are arranged in two clusters: one is around Fedchenko glacier in the Yazgulem Range and one around Lenin Peak in the Trans-Alai Range. Also this region has steep topography and several glaciers reach an elevation range of around 4000 m. However, these numbers are a snapshot in time and have to be treated with care, since there are many surge-type glaciers whose current phase state can significantly influence minimum elevation and area (Kotlyakov et al., 2008). We found the lowest-lying terminus at a very small, north-facing and likely avalanche-fed glacier (70.65∘ E/38.99∘ N) in the Petra Pervogo Range, reaching down to below 2400 m.
The eastern Pamir region has 38 glaciers larger than 10 km2, evenly distributed over the individual mountain ranges. The largest glacier (109.4±6.9 km2) is Karayaylak glacier, draining the northern basin of the Kongur Shan. It starts at the top of Kongur Tagh, with the highest mountain in the Pamir at an elevation of 7680 m, and reaches down to 2819 m, spanning an elevation range of over 4800 m, which is by far the largest value in this region. One of its tributaries has reportedly surged in 2015 (Shangguan et al., 2016). The neighbouring Qimgan glacier starts at the same peak facing south-east and reaches down to 3160 m (almost 4500 m elevation range). A smaller, east-facing glacier in the Oytagh glacier park reaches the same low elevation as Karayaylak glacier (2824 m).
Siachen glacier is the largest of its kind in the Karakoram. With an area of 1094.2±31.2 km2 (including all of its major tributaries) it is by far the largest glacier in the study area, and with over 70 km in length Siachen and Fedchenko are the longest glaciers in the mid-latitudes. Two more glaciers have an area over 500 km2: Baltoro with 810±36.1 km2 and Biafo with 560±23.8 km2. Both glaciers reach their lowest elevations in the central part of the Hunza valley (around Gilgit), with terminus elevations of around 2500 m and below (Hopar glacier: 2260 m). Two large glaciers reach elevation ranges of 5200 m (Batura and Baltoro), but also smaller glaciers like Shishper (45±4.1 km2), Passu (62.2±1.7 km2), and Rakaposhi (14.4±0.9 km2) stretch over an elevation range of 5000 m. Once again, many of these glaciers are of a surge type (e.g. Bhambri et al., 2017), and their minimum elevations and area values after a surge might strongly differ from those at the end of a quiescent phase. The highest glacierized regions in the Karakoram are found around K2 (8611 m; Baltoro glacier) and Distaghil Sar (7885 m; Yazghil glacier, Hispar glacier).
5.3 Glacier aspect analysis
On average, most glaciers are oriented towards the north sector (mean: 71.5 %±5.4 %, Fig. 8a). The relative distribution is similar among the regions, and the largest variations occur in the aspects with a small glacier share (SE, S, SW; normalized SD: 0.21, 0.32, 0.31). The distribution of single cells (instead of one mean aspect value per glacier) shows a similar pattern although with less significance of north aspect. Nevertheless, the north sector has the highest share in all regions (mean: 56.5 %±5.7 %, Fig. 8b), while the south and south-west host the smallest share of glacierized area.
In contrast to other regions, we found no correlation between median elevation and aspect.
5.4 Glacier slope analysis
The mean slope of all glaciers is 26.4∘. It decreases to 22.6∘ for glaciers larger than 5 km2; hence, mean slope is size dependent. The decrease in mean slope between the sample of all glaciers and glaciers larger than 5 km2 is relatively large for Pamir Alai (−5.6∘) and very small for the eastern Pamir (−1.4∘). Mean slope varies between different parts of the glacier, with the accumulation area being the steepest section and the debris-covered areas being far flatter in all regions (Fig. 9).
To determine whether glaciers constantly get steeper from the terminus to the upper reaches of the accumulation area, we normalized the elevation distribution of all glaciers such that each glacier covered the value range from 0 to 1 from the terminus to the upper end, divided into sections of 0.1. The result clearly reveals a mean slope of about 12∘ in the lower parts and a constant increase to over 30∘ at the highest elevations of each glacier (Fig. 10). The uppermost band is again somewhat flatter, possibly due to the transition of slope direction at crests. The pattern is similar in all regions, but the slope increase along the glacier is higher than average in the eastern Pamir and lower than average in the Pamir Alai.
5.5 Glacier elevation analysis
The median elevation of glaciers larger than 2 km2 ranges from 2800 to over 6500 m. There is a statistically significant correlation (p<0.001) between median elevation and latitude (r2=0.48) and longitude (r2=0.66), which appears as a rise of median elevation from the north-west towards the south-east across the study region (Fig. 11).
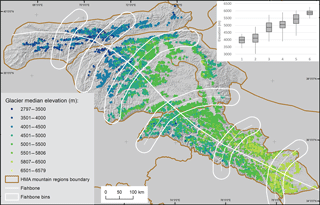
Figure 11Glacier median elevation over the study area of glaciers larger than 0.5 km2. The inset shows median elevation, standard deviations, and minimum and maximum elevations per bin.
This rise becomes even clearer when looking at separated areas along a “fishbone” transverse profile of our study region (inset). The average values of each segment reveal a rise in median elevation from 3980 m (bin 1) to 5860 m (bin 6), with an average trend of 1.9 m km−1 along the profile.
5.6 Hypsography
Plotting the glacier hypsography of the different HMA regions (Fig. 12a) reveals a number of further differences among the regions. Most apparent is the difference in elevation: the median elevation extends from 4141 m (Pamir Alai) to 5419 m (Karakoram), with the western Pamir (4941 m) and eastern Pamir (5119 m) in between the two. Most of the glacierized area is located in the Karakoram (60 %) where the ice is distributed over a large elevation range (Fig. 12b). In contrast, in the Pamir Alai most of the glacier area is situated closely around the median elevation. The large glaciers in the Karakoram reach far down and occupy large areas in lower elevations, further away from the median elevation than in other regions. The eastern Pamir shows a similar drop in the area share of higher elevations, but the curve flattens in elevations over 1000 m above the median elevation. This is related to the shape of topography that is dominated by distinct mountain ranges with large areas above 6500 m (Kongur, Muztag Ata, Kingata Shan). When analysing the hypsography of glaciers with over 10 % debris-covered area compared to the rest of the sample, the insulation effect becomes visible, with debris-covered glaciers occupying considerably more area at lower elevations (Fig. 12c).
5.7 Debris cover
The mapping quality of the debris-covered areas is defined by the corrected outlines as well as by the clean-ice threshold used to differentiate between debris cover and clean-ice surfaces. It contains the same uncertainties and is homogeneous throughout the different Landsat scenes (Fig. 13). The total amount of debris-covered glacier area is 3580 km2, i.e. 10 % of the total glacierized area with small differences among the four HMA regions. The uncertainty considering a buffer of ±1 pixel along the (manually mapped) debris-covered glacier boundary yields ±662 km2, including a buffer of pixel along the (automatically mapped) boundary between clean and debris-covered ice, and the uncertainty increases to ±1131 km2; the latter number is high due to the great number of small debris polygons. The lowest and highest debris-covered area shares are found in the western (8 %) and eastern Pamir (12 %). There is no significant relation between glacier size and debris-covered area share. The distribution in aspect is somewhat skewed towards the north and north-east (12 % and 11 % vs. 8 %–9 % in E, SE, S, SW, W, NW), but this is less of a systematic pattern than for the total glacierized area. The highest values are found in the eastern Pamir where north-facing glaciers are debris covered by over 17 %, whereas Pamir Alai exhibits the largest range (N = 15 % vs. SW = 6 %).
Generally, there is no relation between the mean slope of a glacier and the area share of its debris cover. However, the mean slope of the debris-covered part of the glaciers is 16.6∘ (±5.5), whereas the mean slope of these glaciers is 26.1∘ (±3.2). This was expected since the debris cover is usually situated at the flatter glacier tongues (Paul et al., 2004). Looking at the ablation area of all glaciers, the mean slope is 25.0∘ (±4.2). The ablation areas of more strongly debris-covered glaciers are somewhat flatter: glaciers with a debris-covered area of ≥10 % on average have a steepness of 22.7∘ (±4.0); in contrast, glaciers with less than 5 % debris cover have a mean slope of 25.7∘ (±4.1).
By applying previously assumed area uncertainties (±2.5 % for clean ice, ±5 % for debris-covered ice) to the mapped glacier area, the derived total glacier area is 35 520±1955 km2 . With the buffer method (clean ice , debris-covered ice ±1 pixel) we obtain a similar uncertainty of ±1948 km2. Both methods are applied to glacier complexes to avoid double counting of overlapping areas of adjacent glaciers. Finally, the multiple digitization experiment resulted in a ±13 % standard deviation (averaged over all experiments). This value appears high, but it reflects the mapping reality in challenging situations with debris-covered glacier tongues. For two of the three test glaciers, the difference between the largest and the smallest area mapped was less than 5 % of the mean glacier area. The third example is a small (∼2.9 km2) and steep glacier with a high share of its area hidden in shadow, a large and barely visible debris-covered part and adjacent rock glaciers (see Fig. S2 in the Supplement). Here, the respective uncertainty is ±33 %. Taking this as a worst-case scenario, only few such cases exist in a larger inventory and the high uncertainty has little impact on the overall uncertainty.
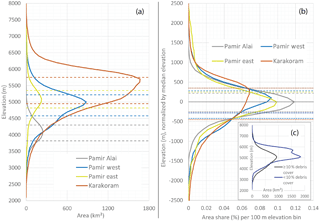
Figure 12Glacier hypsography of the different regions (a), normalized by the respective median elevation (b). Dashed lines represent the 25 % and 75 % area elevations. (c) Hypsography comparison of more and less debris-covered glaciers.
Paul et al. (2013, 2015) showed that analyst interpretations for debris-covered glaciers and glacier parts in shadow can differ by up to 50 %. Our experiment showed that, if the glacier is affected by both shadow and debris cover and is additionally small, the differences can be even higher with up to 70 %. The experiment also confirmed that area differences mainly depend on the interpretation of the debris-covered parts. Thereby, using coherence images improved the analyst's interpretation. Although the overall effect was small (on average ∼1 %), it reduced the dispersion of the analyst's interpretations considerably (see Fig. 14). The different timing of Landsat (2000) and ALOS-1 PALSAR-1 (2007–2009) imagery had only a small impact, as geometric changes during these 7 years were small. The use of Google Earth imagery did not lead to notable outline modifications as they either had low quality (resolution, snow cover) or provided a mere confirmation of the existing interpretation from Landsat and coherence images. We conclude that the area uncertainty of the debris-covered parts of a glacier is of the order of 10 % to 20 %. However, at least one third of this uncertainty can be disregarded due to direct contact to clean-ice glacier parts (see Fig. 13).
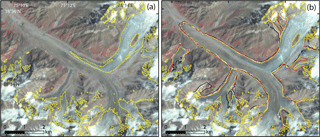
Figure 14Results of the expert round robin, example Glacier 2. (a) shows mapping results solely based on the satellite image, whereas (b) shows mapping results after manual corrections using the additional source of coherence images and Google Earth high-resolution imagery.
The mapping uncertainty for the clean-ice glacier parts was found to be low, notwithstanding the simple method applied (constant threshold for all scenes). Using different thresholds of 2.0±0.3 yielded results in the range of 5 % of the debris-covered area, which is smaller than the uncertainty from the manual correction of the debris-covered glacier parts.
All uncertainty values have to be seen from the perspective of methodological uncertainties, e.g. the inclusion of possible snowfields at high elevations, which can easily increase the area of a small glacier by 50 % or more. With this in mind, the uncertainties presented above are in general much smaller and are more of an academic nature. As the uncertainties from the expert round robin are close to those from the buffer method, we use the uncertainty derived by the buffer method as the uncertainties assigned to our results, knowing that they are on the conservative side.
We also performed a comparison in regions where the CGI (Guo et al., 2015), the GAMDAM (Nuimura et al., 2015), and our inventory have mapped the glaciers, to determine major differences among them. Compared to the CGI, our total glacier area is ∼15 % larger (despite a similar glacier definition) and the CGI overlaps with 82 % of our inventory. Our debris-covered areas are somewhat larger along the margins of the tongues and more of the smaller glaciers at higher elevations are included (Fig. 15). Regions where the CGI area is larger (7 % in total) are related to the inclusion of areas enclosed by different branches of the same glacier, as well as dead ice and rock glaciers in front of a terminus. The GAMDAM inventory covers 13 % less area than ours and also overlaps with 82 % of the area. Here the difference is clearly linked to a diverging glacier mapping definition that mostly excludes headwalls steeper than 40∘ (Nuimura et al., 2015). Moreover, many debris-covered glacier areas and in some cases entire glaciers have not been mapped. On the other hand, almost all of the areas covered by GAMDAM but not by our inventory are mapped as debris-covered glaciers. We think that excluding steep headwalls leads to an incomplete inventory and that the inclusion of rock outcrops in the CGI constitutes a commission error that needs to be corrected for some applications. Overall, the differing interpretation of debris-covered glacier parts and seasonal snow is seemingly the main source of differences in glacier extents for the same region when mapped by different analysts.
When compiling a large-scale glacier inventory, it is essential to have homogeneous quality of the input data used, at best also in a temporal sense, to ensure high credibility of the resulting outlines and topographic parameters as well as low uncertainties of the glacierized area. This can be achieved by using globally consistent datasets such as the Landsat images and the GDEM2. However, the latter does not fulfil the criterion of temporal consistency and future work might overcome this issue. There are strategies like DEM fusion to improve DEM quality in regions of very steep terrain or low-contrast glacier surfaces (e.g. Shean et al., 2016; Lee et al., 2015; Tran et al., 2014 and references therein), but the impact of such quality issues is difficult to assess without accurately geo-referenced high-resolution reference data (Kääb et al., 2016; Frey and Paul, 2012). With the semi-automated processing line applied here and the few experts involved in the manual corrections, we assume a homogeneous quality of the glacier outlines throughout the study area has been achieved. However, our glacier extents are likely on the conservative side for debris-covered ablation areas, leading to an underestimation of glacier area, whereas the included perennial ice and snowfields in steep terrain at high elevations are more generous than in other interpretations.
The extraction of debris-covered ice was performed automatically by applying a single threshold value to all scenes and removing the resulting clean-ice areas from the corrected glacier polygons. This is likely the easiest method that still provided good results. Adapting the clean-ice threshold changed the resulting debris-covered area only by ±2.5 %, indicating that the transition from clean ice to continuous debris cover is relatively sharp. Herreid et al. (2015) used a function applied to Landsat band combinations that was fit to manually derived reference data of a single glacier and adapted it to the various mapping dates (using different Landsat sensors). This method might be superior, but it is more labour-intensive and visual comparison with the figures in Herreid et al. (2015) shows very high agreement. Another approach was applied by Kraaijenbrink et al. (2017), who used the normalized difference snow index (NDSI) together with a composite image of Landsat 8 band 10 (thermal infrared) scenes to detect debris cover based on the RGI 5.0 outlines. A major prerequisite for all methods is the use of glacier outlines that are well adjusted for debris cover. Glacier retreat was found to correlate with an increase in supraglacial debris cover (e.g. Stokes et al., 2007) and, hence, multi-temporal mapping of debris extent should be applied. As extensive debris cover affects glacier melt and geometry (e.g. Anderson and Anderson, 2016), we recommend including it in the published glacier inventories (GLIMS, RGI), by (a) adding the debris mask as a polygon and (b) including debris cover share in the attribute table. Our results show a total of ∼10 % debris-covered area, with many of the larger glaciers reaching 20 % or more. These numbers complement and confirm existing estimates in HMA that are based on smaller samples. Values reported from the central Karakoram are 20 % (Minora et al., 2016) and ∼21 % (Herreid et al., 2015), Frey et al. (2012) calculated 16 % for the western Himalaya, and for the entire Himalaya a ∼10 % coverage was calculated (Kraaijenbrink et al., 2017; Bolch et al., 2012).
Comparing the freely available results by Kraaijenbrink et al. (2017) to our results, the total difference is <1.5 % of the total debris-covered area (3365 km2 vs. 3409 km2), a value well below the uncertainty of these areas. However, the differences vary from one region to another, from −18.4 % in the Pamir west to 5.7 % in the Karakoram.
The pattern of glacier median elevations found in our study reflects combinations of climatic and topographic aspects. A similar west–east and north–south gradient was also found in the study by Sakai et al. (2015), who determined median elevations from a glacier inventory (GAMDAM, Nuimura et al., 2015) for all of HMA. Whereas the latitudinal extent of 7∘ decreases air temperatures and thus median elevations towards the north, the precipitation decrease from west to east due to leeward rain shadow effects increases median elevations in the eastern Pamir and Karakoram. Approximating the balanced-budget ELA (ELA0) with the median elevation has been successfully applied in many mountain ranges and works well for different glacier types (Braithwaite and Raper, 2009). However, this concept likely does not apply to surge-type glaciers and glaciers that are mainly nourished by snow avalanches (Hewitt, 2011). For the latter as well as debris-covered glaciers, ELA0 values are expected to be higher than those calculated here due to the additional accumulation and reduced ablation. This is supported by the fact that we find debris-covered areas also above the median elevation and by a finding from Braithwaite and Raper (2009), who mention possible accumulation-area ratio values below 0.5 for Himalayan glaciers.
We also performed a detailed analysis of uncertainties and analysed the most important sources contributing to uncertainty. It is, however, impossible to perform a rigorous calculation, as this would require a comparison with appropriate reference data. The uncertainties presented here are based on different methods and some of the values are higher than reported previously. This is mainly because of the high debris coverage and the large number of (very) small glaciers. Under such challenging conditions, area differences among the analysts were as high as uncertainties due to the possible wrong consideration of seasonal snow. Hence, the total area of our inventory will likely be somewhat larger than other inventories for this region as these might have excluded the maybe just snow-covered steep regions at highest elevations. Once scenes without seasonal snow in these regions become available, glacier extents should be revisited and corrected as required.
The dataset is downloadable at https://doi.org/10.1594/PANGAEA.894707 (Mölg et al., 2018).
We derived a new glacier inventory for a substantial part of western High Mountain Asia (Karakoram and Pamir) and have presented in detail the derived characteristics of the glaciers in this region. Special emphasis was given to the description of mapping challenges for debris-covered glaciers (and distinguishing them from rock glaciers), seasonal snow, and shadow, along with the selected solutions. In the absence of appropriate reference datasets, we instead applied various methods for uncertainty assessment and compared our outlines to other existing inventories covering the same region. As an extension to already existing datasets we included outlines and percentages of the debris-covered area for each glacier.
We mapped 27 437 glaciers covering 35 287±1209 km2, with about 10 % of the area being debris covered. The ASTER GDEM2 was found to be superior to the SRTM DEM (1 arcsec) in deriving drainage divides and topographic information for each glacier as the latter suffered from too many (wrongly interpolated) data voids in this region. The application of a constant band ratio threshold to derive clean-ice areas for all scenes to create the debris cover maps was found to be of sufficient quality. Uncertainties derived from three different methods were all in good agreement (3.4 %) but the multiple-digitizing experiment also revealed larger deviations among the analysts under challenging conditions (debris, shadow, small glacier). However, the availability of coherence images improved the quality and consistency of the manual corrections for debris-covered glaciers considerably.
The analysis of the topographic information revealed several interesting dependencies among the glaciers and also across the regions. Despite the fact that in the Karakoram the largest glaciers are facing south-east (Siachen, Biafo), east (Batura, Skamri), or west (Baltoro, Hispar), most glacier area (47 %) is still exposed to the three northern sectors. Glacier median elevation has little dependence on aspect but a strong one on longitude and latitude (higher towards the drier north and east), indicating a close relation to precipitation amounts. Glacier hypsometry reveals a peak distribution that is highest (∼5700 m) in the Karakoram, similar but 700 m lower in the eastern and western Pamir, and lowest in Pamir Alai (∼4200 m). Glaciers in the Karakoram have a comparably higher area share at the lowest elevations and glaciers larger than 5 km2 or debris-covered glaciers are flatter (22.6 and 16.6∘, respectively) than on average (26.4∘). By location, glaciers are especially flat (<15∘) in their lowest third and progressively steeper (>30∘) in the uppermost third, indicating the dominance of large valley glaciers with very flat tongues and steep head walls. Both glacier outlines and the separate outlines of the debris-covered parts will be freely available from the GLIMS database to facilitate applications such as distributed mass balance modelling and albedo calculation; debris-thickness calculation; determination of run-off (with a melt reduction under debris-covered areas); and future geometric evolution, sediment transport, and mountain erosion rates, to name a few.
The supplement related to this article is available online at: https://doi.org/10.5194/essd-10-1807-2018-supplement.
NM, FP, TB, and PR designed the study. NM and FP wrote the manuscript. NM, PR, TB, and FP generated and edited glacier outlines and basins. TS produced the coherence images. NM extracted debris cover and performed all analysis. All authors contributed to the final form of the manuscript.
The authors declare that they have no conflict of interest.
We thank J. Graham Cogley and an anonymous reviewer as well as the journal
editor for their thorough reviews and constructive comments that helped
improve the clarity of the paper. We acknowledge funding from the ESA
projects Glaciers-cci (4000109873/14/I-NB) and Dragon 4 (4000121469/17/I-NB) as well as the
Copernicus Climate Change Service (C3S) that is implemented by ECMWF on behalf of the European Commission.
The manual digitizing experiment was performed by the authors with additional
contributions by Holger Frey and Raymond Le Bris.
Edited by: Reinhard Drews
Reviewed by: J. Graham Cogley and one anonymous referee
Aizen, E. M., Aizen, V. B., Melack, J. M., Nakamura, T., and Ohta, T.: Precipitation and atmospheric circulation patterns at mid-latitudes of Asia, Int. J. Climatol., 21, 535–556, https://doi.org/10.1002/joc.626, 2001.
Aizen, V. B., Aizen, E. M., Melack, J. M., and Dozier, J.: Climatic and Hydrologic Changes in the Tien Shan, Central Asia, J. Climate, 10, 1393–1404, 1997.
Anderson, L. S. and Anderson, R. S.: Modeling debris-covered glaciers: response to steady debris deposition, The Cryosphere, 10, 1105–1124, https://doi.org/10.5194/tc-10-1105-2016, 2016.
Andreassen, L. M., Paul, F., Kääb, A., and Hausberg, J. E.: Landsat-derived glacier inventory for Jotunheimen, Norway, and deduced glacier changes since the 1930s, The Cryosphere, 2, 131–145, https://doi.org/10.5194/tc-2-131-2008, 2008.
Archer, D. R. and Fowler, H. J.: Spatial and temporal variations in precipitation in the Upper Indus Basin, global teleconnections and hydrological implications, Hydrol. Earth Syst. Sci., 8, 47–61, https://doi.org/10.5194/hess-8-47-2004, 2004.
Arendt, A., Bliss, A., Bolch, T., Cogley, J. G., Gardner, A. S., Hagen, J.-O., Hock, R., Huss, M., Kaser, G., Kienholz, C., Pfeffer, W. T., Moholdt, G., Paul, F., Radić, V., Andreassen, L. M., Bajracharya, S., Barrand, N. E., Beedle, M., Berthier, E., Bhambri, R., Brown, I., Burgess, E. W., Burgess, D., Cawkwell, F., Chinn, T., Copland, L., Davies, B., Angelis, H. de, Dolgova, E., Earl, L., Filbert, K., Forester, R., Fountain, A. G., Frey, H., Giffen, B., Glasser, N. F., Guo, W., Gurney, S. D., Hagg, W., Hall, D., Haritashya, U. K., Hartmann, G., Helm, C., Herreid, S., Howat, I., Kapustin, G., Khromova, T. E., König, M., Kohler, J., Kriegel, D., Kutuzov, S., Lavrentiev, I., Le Bris, R., Liu, S., Lund, J., Manley, W., Marti, R., Mayer, C., Miles, E. S., Li, X., Menounos, B., Mercer, A., Mölg, N., Mool, P., Nosenko, G., Negrete, A., Nuimura, T., Nuth, C., Pettersson, R., Racoviteanu, A., Ranzi, R., Rastner, P., Rau, F., Raup, B., Rich, J., Rott, H., Sakai, A., Schneider, C., Seliverstov, Y., Sharp, M. J., Sigurðsson, O., Stokes, C. R., Way, R. G., Wheate, R., Winsvold, S., Wolken, G., Wyatt, F., and Zheltihyna, N.: Randolph Glacier Inventory – A Dataset of Global Glacier Outlines: Version 5.0: GLIMS Technical Report, Global Land Ice Measurement from Space, Colorado, USA, Digital Media, https://doi.org/10.7265/N5-RGI-50 (last access: 13 March 2018), 2015.
Atwood, D. K., Meyer, F., and Arendt, A.: Using L-band SAR coherence to delineate glacier extent, Can. J. Remote Sens., 36, S186–S195, https://doi.org/10.5589/m10-014, 2010.
Bajracharya, S. R., Maharjan, S. B., Shrestha, F., Guo, W., Liu, S., Immerzeel, W., and Shrestha, B.: The glaciers of the Hindu Kush Himalayas: Current status and observed changes from the 1980s to 2010, Int. J. Water Resour. D., 31, 161–173, https://doi.org/10.1080/07900627.2015.1005731, 2015.
Barsch, D.: Rockglaciers: Indicators for the Present and Former Geoecology in High Mountain Environments, in: Springer Series in Physical Environment, Springer Berlin Heidelberg, Berlin, Heidelberg, 16, 331 pp., 1996.
Berthling, I.: Beyond confusion: Rock glaciers as cryo-conditioned landforms, Geomorphology, 131, 98–106, https://doi.org/10.1016/j.geomorph.2011.05.002, 2011.
Bhambri, R., Bolch, T., Kawishwar, P., Dobhal, D. P., Srivastava, D., and Pratap, B.: Heterogeneity in glacier response in the upper Shyok valley, northeast Karakoram, The Cryosphere, 7, 1385–1398, https://doi.org/10.5194/tc-7-1385-2013, 2013.
Bhambri, R., Hewitt, K., Kawishwar, P., and Pratap, B.: Surge-type and surge-modified glaciers in the Karakoram, Scientific Reports, 7, 15391, https://doi.org/10.1038/s41598-017-15473-8, 2017.
Bishop, M. P., Shroder, J. F., Ali, G., Bush, A. B. G., Haritashya, U. K., Roohi, R., Sarikaya, M. A., and Weihs, B. J.: Remote Sensing of Glaciers in Afghanistan and Pakistan, in: Global Land Ice Measurements from Space, edited by: Kargel, J. S., Leonard, G. J., Bishop, M. P., Kääb, A., and Raup, B. H., Springer Berlin Heidelberg, Berlin, Heidelberg, 509–548, 2014.
Bliss, A., Hock, R., and Radić, V.: Global response of glacier runoff to twenty-first century climate change, J. Geophys. Res. Earth, 119, 717–730, https://doi.org/10.1002/2013JF002931, 2014.
Bodin, X., Rojas, F., and Brenning, A.: Status and evolution of the cryosphere in the Andes of Santiago (Chile, 33.5∘S.), Geomorphology, 118, 453–464, https://doi.org/10.1016/j.geomorph.2010.02.016, 2010.
Böhner, J.: General climatic controls and topoclimatic variations in Central and High Asia, Boreas, 35, 279–295, https://doi.org/10.1111/j.1502-3885.2006.tb01158.x, 2006.
Bolch, T. and Gorbunov, A. P.: Characteristics and Origin of Rock Glaciers in Northern Tien Shan (Kazakhstan/Kyrgyzstan), Permafrost Periglac., 25, 320–332, https://doi.org/10.1002/ppp.1825, 2014.
Bolch, T. and Kamp, U.: Glacier mapping in high mountains using DEMs, Landsat and ASTER data, in: Proceedings of the 8th International Symposium on High Mountain Remote Sensing Cartography, La Paz, Bolivia, 21–27 March 2005, edited by: Kaufmann, V. and Sulzer, W., 2006.
Bolch, T., Buchroithner, M., Kunert, A., and Kamp, U.: Automated delineation of debris-covered glaciers based on ASTER data, in: GeoInformation in Europe, edited by: Gomarasca, A., Millpress, Rotterdam, 403–410, 2007.
Bolch, T., Menounos, B., and Wheate, R.: Landsat-based inventory of glaciers in western Canada, 1985-2005, Remote Sens. Environ., 114, 127–137, https://doi.org/10.1016/j.rse.2009.08.015, 2010.
Bolch, T., Kulkarni, A., Kaab, A., Huggel, C., Paul, F., Cogley, J. G., Frey, H., Kargel, J. S., Fujita, K., Scheel, M., Bajracharya, S., and Stoffel, M.: The state and fate of Himalayan glaciers, Science, 336, 310–314, https://doi.org/10.1126/science.1215828, 2012.
Bolch, T., Sandberg Sørensen, L., Simonsen, S. B., Mölg, N., Machguth, H., Rastner, P., and Paul, F.: Mass loss of Greenland's glaciers and ice caps 2003–2008 revealed from ICESat laser altimetry data, Geophys. Res. Lett., 40, 875–881, https://doi.org/10.1002/grl.50270, 2013.
Bolch, T., Pieczonka, T., Mukherjee, K., and Shea, J.: Brief communication: Glaciers in the Hunza catchment (Karakoram) have been nearly in balance since the 1970s, The Cryosphere, 11, 531–539, https://doi.org/10.5194/tc-11-531-2017, 2017.
Bookhagen, B. and Burbank, D. W.: Toward a complete Himalayan hydrological budget: Spatiotemporal distribution of snowmelt and rainfall and their impact on river discharge, J. Geophys. Res., 115, F03019, https://doi.org/10.1029/2009JF001426, 2010.
Braithwaite, R. J. and Müller, F.: On the parameterization of glacier equilibrium line altitude, in: Proceedings of the Workshop at Riederalp, Switzerland, 17–22 September 1978, IAHS-AISH Publ. No. 126, 263–271, 1980.
Braithwaite, R. J. and Raper, S.C.B.: Estimating equilibrium-line altitude (ELA) from glacier inventory data, Ann. Glaciol., 50, 127–132, https://doi.org/10.3189/172756410790595930, 2009.
Brock, B. W., Mihalcea, C., Kirkbride, M. P., Diolaiuti, G., Cutler, M. E. J., and Smiraglia, C.: Meteorology and surface energy fluxes in the 2005–2007 ablation seasons at the Miage debris-covered glacier, Mont Blanc Massif, Italian Alps, J. Geophys. Res., 115, D09106, https://doi.org/10.1029/2009JD013224, 2010.
Brun, F., Berthier, E., Wagnon, P., Kääb, A., and Treichler, D.: A spatially resolved estimate of High Mountain Asia glacier mass balances, 2000–2016, Nat. Geosci., 10, 668–673, https://doi.org/10.1038/NGEO2999, 2017.
Copland, L., Sylvestre, T., Bishop, M. P., Shroder, J. F., Seong, Y. B., Owen, L. A., Bush, A., and Kamp, U.: Expanded and Recently Increased Glacier Surging in the Karakoram, Arct. Antarct. Alp. Res., 43, 503–516, https://doi.org/10.1657/1938-4246-43.4.503, 2011.
Dehecq, A., Gourmelen, N., and Trouve, E.: Deriving large-scale glacier velocities from a complete satellite archive: Application to the Pamir–Karakoram–Himalaya, Remote Sens. Environ., 162, 55–66, https://doi.org/10.1016/j.rse.2015.01.031, 2015.
Dobreva, I., Bishop, M., and Bush, A.: Climate–Glacier Dynamics and Topographic Forcing in the Karakoram Himalaya: Concepts, Issues and Research Directions, Water, 9, 405, https://doi.org/10.3390/w9060405, 2017.
Falaschi, D., Bolch, T., Rastner, P., Lenzano, M. G., Lenzano, L., Lo Veccio, A., and Moragues, S.: Mass changes of alpine glaciers at the eastern margin of the Northern and Southern Patagonian Icefields between 2000 and 2012, J. Glaciol., 63, 258–272, https://doi.org/10.1017/jog.2016.136, 2017.
Frey, H. and Paul, F.: On the suitability of the SRTM DEM and ASTER GDEM for the compilation of topographic parameters in glacier inventories, Int. J. Appl. Earth Obs., 18, 480–490, https://doi.org/10.1016/j.jag.2011.09.020, 2012.
Frey, H., Paul, F., and Strozzi, T.: Compilation of a glacier inventory for the western Himalayas from satellite data: Methods, challenges, and results, Remote Sens. Environ., 124, 832–843, https://doi.org/10.1016/j.rse.2012.06.020, 2012.
Gardelle, J., Berthier, E., Arnaud, Y., and Kääb, A.: Region-wide glacier mass balances over the Pamir-Karakoram-Himalaya during 1999–2011, The Cryosphere, 7, 1263–1286, https://doi.org/10.5194/tc-7-1263-2013, 2013.
Gardner, A. S., Moholdt, G., Cogley, J. G., Wouters, B., Arendt, A. A., Wahr, J., Berthier, E., Hock, R., Pfeffer, W. T., Kaser, G., Ligtenberg, S. R. M., Bolch, T., Sharp, M. J., Hagen, J. O., van den Broeke, M. R., and Paul, F.: A reconciled estimate of glacier contributions to sea level rise: 2003 to 2009, Science, 340, 852–857, https://doi.org/10.1126/science.1234532, 2013.
Gorbunov, A. P. and Titkov, S. N.: Kamennye Gletchery Gor Srednej Azii (Rock glaciers of the Central Asian Mountains), Akademia Nauk SSSR, Irkutsk, 1989.
Granshaw, F. D. and Fountain, A. G.: Glacier change (1958–1998) in the North Cascades National Park Complex, Washington, USA, J. Glaciol., 52, 251–256, https://doi.org/10.3189/172756506781828782, 2006.
Guo, W., Liu, S., Xu, J., Wu, L., Shangguan, D., Yao, X., Wei, J., Bao, W., Yu, P., Liu, Q., and Jiang, Z.: The second Chinese glacier inventory: Data, methods and results, J. Glaciol., 61, 357–372, https://doi.org/10.3189/2015JoG14J209, 2015.
Haeberli, W. and Hoelzle, M.: Application of inventory data for estimating characteristics of and regional climate-change effects on mountain glaciers: A pilot study with the European Alps, Ann. Glaciol., 21, 206–212, https://doi.org/10.3189/S0260305500015834, 1995.
Haeberli, W., Hallet, B., Arenson, L., Elconin, R., Humlum, O., Kääb, A., Kaufmann, V., Ladanyi, B., Matsuoka, N., Springman, S., and Mühll, D. V.: Permafrost creep and rock glacier dynamics, Permafrost Periglac., 17, 189–214, https://doi.org/10.1002/ppp.561, 2006.
Haritashya, U. K., Bishop, M. P., Shroder, J. F., Bush, A. B. G., and Bulley, H. N. N.: Space-based assessment of glacier fluctuations in the Wakhan Pamir, Afghanistan, Climatic Change, 94, 5–18, https://doi.org/10.1007/s10584-009-9555-9, 2009.
Herreid, S., Pellicciotti, F., Ayala, A., Chesnokova, A., Kienholz, C., Shea, J., and Shrestha, A.: Satellite observations show no net change in the percentage of supraglacial debris-covered area in northern Pakistan from 1977 to 2014, J. Glaciol., 61, 524–536, https://doi.org/10.3189/2015JoG14J227, 2015.
Hewitt, K.: The Karakoram Anomaly? Glacier Expansion and the 'Elevation Effect' Karakoram Himalaya, Mt. Res. Dev., 25, 332–340, 2005.
Hewitt, K.: Glacier Change, Concentration, and Elevation Effects in the Karakoram Himalaya, Upper Indus Basin, Mt. Res. Dev., 31, 188–200, https://doi.org/10.1659/MRD-JOURNAL-D-11-00020.1, 2011.
Holzer, N., Golletz, T., Buchroithner, M., and Bolch, T.: Glacier Variations in the Trans Alai Massif and the Lake Karakul Catchment (Northeastern Pamir) Measured from Space, in: Climate Change, Glacier Response, and Vegetation Dynamics in the Himalaya, edited by: Singh, R. B., Schickhoff, U., and Mal, S., Springer International Publishing, Cham, 139–153, 2016.
Huss, M. and Hock, R.: A new model for global glacier change and sea-level rise, Front. Earth Sci., 3, 382, https://doi.org/10.3389/feart.2015.00054, 2015.
Immerzeel, W. W., van Beek, L. P. H., and Bierkens, M. F. P.: Climate change will affect the Asian water towers, Science, 328, 1382–1385, https://doi.org/10.1126/science.1183188, 2010.
Immerzeel, W. W., Wanders, N., Lutz, A. F., Shea, J. M., and Bierkens, M. F. P.: Reconciling high-altitude precipitation in the upper Indus basin with glacier mass balances and runoff, Hydrol. Earth Syst. Sci., 19, 4673–4687, https://doi.org/10.5194/hess-19-4673-2015, 2015.
Iturrizaga, L.: Trends in 20th century and recent glacier fluctuations in the Karakoram Mountains, Z. Geomorphol. Supp., 55, 205–231, https://doi.org/10.1127/0372-8854/2011/0055S3-0059, 2011.
Kääb, A., Berthier, E., Nuth, C., Gardelle, J., and Arnaud, Y.: Contrasting patterns of early twenty-first-century glacier mass change in the Himalayas, Nature, 488, 495–498, https://doi.org/10.1038/nature11324, 2012.
Kääb, A., Treichler, D., Nuth, C., and Berthier, E.: Brief Communication: Contending estimates of 2003–2008 glacier mass balance over the Pamir–Karakoram–Himalaya, The Cryosphere, 9, 557–564, https://doi.org/10.5194/tc-9-557-2015, 2015.
Kääb, A., Winsvold, S., Altena, B., Nuth, C., Nagler, T., and Wuite, J.: Glacier Remote Sensing Using Sentinel-2. Part I: Radiometric and Geometric Performance, and Application to Ice Velocity, Remote Sensing, 8, 598, https://doi.org/10.3390/rs8070598, 2016.
Khromova, T. E., Osipova, G. B., Tsvetkov, D. G., Dyurgerov, M. B., and Barry, R. G.: Changes in glacier extent in the eastern Pamir, Central Asia, determined from historical data and ASTER imagery, Remote Sens. Environ., 102, 24–32, https://doi.org/10.1016/j.rse.2006.01.019, 2006.
Kienholz, C., Hock, R., and Arendt, A. A.: A new semi-automatic approach for dividing glacier complexes into individual glaciers, J. Glaciol., 59, 925–937, https://doi.org/10.3189/2013JoG12J138, 2013.
Kienholz, C., Herreid, S., Rich, J. L., Arendt, A. A., Hock, R., and Burgess, E. W.: Derivation and analysis of a complete modern-date glacier inventory for Alaska and northwest Canada, J. Glaciol., 61, 403–420, https://doi.org/10.3189/2015JoG14J230, 2015.
Kirkbride, M. P. and Deline, P.: The formation of supraglacial debris covers by primary dispersal from transverse englacial debris bands, Earth Surf. Proc. Land., 38, 1779–1792, https://doi.org/10.1002/esp.3416, 2013.
Kotlyakov, V. M., Osipova, G. B., and Tsvetkov, D. G.: Monitoring surging glaciers of the Pamirs, central Asia, from space, Ann. Glaciol., 48, 125–134, https://doi.org/10.3189/172756408784700608, 2008.
Kraaijenbrink, P. D. A., Bierkens, M. F. P., Lutz, A. F., and Immerzeel, W. W.: Impact of a global temperature rise of 1.5 degrees Celsius on Asia's glaciers, Nature, 549, 257–260, https://doi.org/10.1038/nature23878, 2017.
Le Bris, R., Paul, F., Frey, H., and Bolch, T.: A new satellite-derived glacier inventory for western Alaska, Ann. Glaciol., 52, 135–143, https://doi.org/10.3189/172756411799096303, 2011.
Lee, C., Oh, J., Hong, C., and Youn, J.: Automated Generation of a Digital Elevation Model Over Steep Terrain in Antarctica From High-Resolution Satellite Imagery, IEEE T. Geosci. Remote, 53, 1186–1194, https://doi.org/10.1109/TGRS.2014.2335773, 2015.
Lin, H., Li, G., Cuo, L., Hooper, A., and Ye, Q.: A decreasing glacier mass balance gradient from the edge of the Upper Tarim Basin to the Karakoram during 2000–2014, Scientific Reports, 7, 6712, https://doi.org/10.1038/s41598-017-07133-8, 2017.
Lutz, A. F., Immerzeel, W. W., Shrestha, A. B., and Bierkens, M. F. P.: Consistent increase in High Asia's runoff due to increasing glacier melt and precipitation, Nat. Clim. Change, 4, 587–592, https://doi.org/10.1038/nclimate2237, 2014.
Maussion, F., Scherer, D., Mölg, T., Collier, E., Curio, J., and Finkelnburg, R.: Precipitation Seasonality and Variability over the Tibetan Plateau as Resolved by the High Asia Reanalysis*, J. Climate, 27, 1910–1927, https://doi.org/10.1175/JCLI-D-13-00282.1, 2014.
Minora, U., Bocchiola, D., D'Agata, C., Maragno, D., Mayer, C., Lambrecht, A., Vuillermoz, E., Senese, A., Compostella, C., Smiraglia, C., and Diolaiuti, G. A.: Glacier area stability in the Central Karakoram National Park (Pakistan) in 2001–2010, Prog. Phys. Geog., 40, 629–660, https://doi.org/10.1177/0309133316643926, 2016.
Mölg, N., Bolch, T., Rastner, P., Strozzi, T., and Paul, F.: Glacier inventory of Pamir and Karakoram, https://doi.org/10.1594/PANGAEA.894707, in review, 2018.
Monnier, S. and Kinnard, C.: Reconsidering the glacier to rock glacier transformation problem: New insights from the central Andes of Chile, Geomorphology, 238, 47–55, https://doi.org/10.1016/j.geomorph.2015.02.025, 2015.
Nicholson, L. and Benn, D. I.: Calculating ice melt beneath a debris layer using meteorological data, J. Glaciol., 52, 463–470, https://doi.org/10.3189/172756506781828584, 2006.
Nuimura, T., Sakai, A., Taniguchi, K., Nagai, H., Lamsal, D., Tsutaki, S., Kozawa, A., Hoshina, Y., Takenaka, S., Omiya, S., Tsunematsu, K., Tshering, P., and Fujita, K.: The GAMDAM glacier inventory: a quality-controlled inventory of Asian glaciers, The Cryosphere, 9, 849–864, https://doi.org/10.5194/tc-9-849-2015, 2015.
Paul, F.: Revealing glacier flow and surge dynamics from animated satellite image sequences: examples from the Karakoram, The Cryosphere, 9, 2201–2214, https://doi.org/10.5194/tc-9-2201-2015, 2015.
Paul, F. and Kääb, A.: Perspectives on the production of a glacier inventory from multispectral satellite data in Arctic Canada: Cumberland Peninsula, Baffin Island, Ann. Glaciol., 42, 59–66, https://doi.org/10.3189/172756405781813087, 2005.
Paul, F., Kääb, A., Maisch, M., Kellenberger, T., and Haeberli, W.: The new remote-sensing-derived Swiss glacier inventory: I. Methods, Ann. Glaciol., 34, 355–361, https://doi.org/10.3189/172756402781817941, 2002.
Paul, F., Kääb, A., and Haeberli, W.: Mapping of rock glaciers with optical satellite imagery, in: Permafrost: Extended Abstracts Reporting Current Research and new Information, edited by: Haeberli, W. and Brandová, D., International Conference on Permafrost, Zurich, Switzerland, 20.-25. July, Glaciology and Geomorphodynamics Group, Department of Geography, University of Zurich, 125–126, 2003.
Paul, F., Huggel, C., and Kääb, A.: Combining satellite multispectral image data and a digital elevation model for mapping debris-covered glaciers, Remote Sens. Environ., 89, 510–518, https://doi.org/10.1016/j.rse.2003.11.007, 2004.
Paul, F., Frey, H., and Le Bris, R.: A new glacier inventory for the European Alps from Landsat TM scenes of 2003: Challenges and results, Ann. Glaciol., 52, 144–152, https://doi.org/10.3189/172756411799096295, 2011.
Paul, F., Barrand, N. E., Baumann, S., Berthier, E., Bolch, T., Casey, K., Frey, H., Joshi, S. P., Konovalov, V., Le Bris, R., Mölg, N., Nosenko, G., Nuth, C., Pope, A., Racoviteanu, A., Rastner, P., Raup, B., Scharrer, K., Steffen, S., and Winsvold, S.: On the accuracy of glacier outlines derived from remote-sensing data, Ann. Glaciol., 54, 171–182, https://doi.org/:10.3189/2013AoG63A296, 2013.
Paul, F., Bolch, T., Briggs, K., Kääb, A., McMillan, M., McNabb, R., Nagler, T., Nuth, C., Rastner, P., Strozzi, T., and Wuite, J.: Error sources and guidelines for quality assessment of glacier area, elevation change, and velocity products derived from satellite data in the Glaciers_cci project, Remote Sens. Environ., 203, 256–275, https://doi.org/10.1016/j.rse.2017.08.038, 2017.
Pfeffer, W. T., Arendt, A. A., Bliss, A., Bolch, T., Cogley, J. G., Gardner, A. S., Hagen, J.-O., Hock, R., Kaser, G., Kienholz, C., Miles, E. S., Moholdt, G., Mölg, N., Paul, F., Radić, V., Rastner, P., Raup, B. H., Rich, J., and Sharp, M. J.: The Randolph Glacier Inventory: A globally complete inventory of glaciers, J. Glaciol., 60, 537–552, https://doi.org/10.3189/2014JoG13J176, 2014.
Quincey, D. J., Glasser, N. F., Cook, S. J., and Luckman, A.: Heterogeneity in Karakoram glacier surges, J. Geophys. Res.-Earth, 120, 1288–1300, https://doi.org/10.1002/2015JF003515, 2015.
Racoviteanu, A. and Williams, M. W.: Decision Tree and Texture Analysis for Mapping Debris-Covered Glaciers in the Kangchenjunga Area, Eastern Himalaya, Remote Sensing, 4, 3078–3109, https://doi.org/10.3390/rs4103078, 2012.
Ragettli, S., Pellicciotti, F., Immerzeel, W. W., Miles, E. S., Petersen, L., Heynen, M., Shea, J. M., Stumm, D., Joshi, S., and Shrestha, A.: Unraveling the hydrology of a Himalayan catchment through integration of high resolution in situ data and remote sensing with an advanced simulation model, Adv. Water Resour., 78, 94–111, https://doi.org/10.1016/j.advwatres.2015.01.013, 2015.
Ragettli, S., Bolch, T., and Pellicciotti, F.: Heterogeneous glacier thinning patterns over the last 40 years in Langtang Himal, Nepal, The Cryosphere, 10, 2075–2097, https://doi.org/10.5194/tc-10-2075-2016, 2016.
Rankl, M. and Braun, M.: Glacier elevation and mass changes over the central Karakoram region estimated from TanDEM-X and SRTM/X-SAR digital elevation models, Ann. Glaciol., 57, 273–281, https://doi.org/10.3189/2016AoG71A024, 2016.
Rankl, M., Kienholz, C., and Braun, M.: Glacier changes in the Karakoram region mapped by multimission satellite imagery, The Cryosphere, 8, 977–989, https://doi.org/10.5194/tc-8-977-2014, 2014.
Raper, S. C. B. and Braithwaite, R. J.: Glacier volume response time and its links to climate and topography based on a conceptual model of glacier hypsometry, The Cryosphere, 3, 183–194, https://doi.org/10.5194/tc-3-183-2009, 2009.
Rastner, P., Bolch, T., Notarnicola, C., and Paul, F.: A Comparison of Pixel- and Object-Based Glacier Classification With Optical Satellite Images, IEEE J. Sel. Top. Appl., 7, 853–862, https://doi.org/10.1109/JSTARS.2013.2274668, 2014.
Raup, B. and Khalsa, S. J. S.: GLIMS Analysis Tutorial, Global Land Ice Measurement from Space, available at: https://www.glims.org/MapsAndDocs/guides.html (last access: 5 October 2018), 2007.
RGI Consortium: Randolph Glacier Inventory – A Dataset of Global Glacier Outlines, Version 6.0, Technical Report, Global Land Ice Measurements from Space, Colorado, USA, Digital Media, https://doi.org/10.7265/N5-RGI-60 (last access: 31 March 2018), 2017.
Robson, B., Hölbling, D., Nuth, C., Stozzi, T., and Dahl, S.: Decadal Scale Changes in Glacier Area in the Hohe Tauern National Park (Austria) Determined by Object-Based Image Analysis, Remote Sensing, 8, 67, https://doi.org/10.3390/rs8010067, 2016.
Rowan, A. V., Egholm, D. L., Quincey, D. J., and Glasser, N. F.: Modelling the feedbacks between mass balance, ice flow and debris transport to predict the response to climate change of debris-covered glaciers in the Himalaya, Earth Planet. Sc. Lett., 430, 427–438, https://doi.org/10.1016/j.epsl.2015.09.004, 2015.
Sakai, A., Nuimura, T., Fujita, K., Takenaka, S., Nagai, H., and Lamsal, D.: Climate regime of Asian glaciers revealed by GAMDAM glacier inventory, The Cryosphere, 9, 865–880, https://doi.org/10.5194/tc-9-865-2015, 2015.
Sarıkaya, M. A., Bishop, M. P., Shroder, J. F., and Ali, G.: Remote-sensing assessment of glacier fluctuations in the Hindu Raj, Pakistan, Int. J. Remote Sens., 34, 3968–3985, https://doi.org/10.1080/01431161.2013.770580, 2013.
Satgé, F., Bonnet, M. P., Timouk, F., Calmant, S., Pillco, R., Molina, J., Lavado-Casimiro, W., Arsen, A., Crétaux, J. F., and Garnier, J.: Accuracy assessment of SRTM v4 and ASTER GDEM v2 over the Altiplano watershed using ICESat/GLAS data, Int. J. Remote Sens., 36, 465–488, https://doi.org/10.1080/01431161.2014.999166, 2015.
Scherler, D., Bookhagen, B., and Strecker, M. R.: Hillslope-glacier coupling: The interplay of topography and glacial dynamics in High Asia, J. Geophys. Res., 116, F02019, https://doi.org/10.1029/2010JF001751, 2011a.
Scherler, D., Bookhagen, B., and Strecker, M. R.: Spatially variable response of Himalayan glaciers to climate change affected by debris cover, Nat. Geosci., 4, 156–159, https://doi.org/10.1038/NGEO1068, 2011b.
Seong, Y. B., Owen, L. A., Yi, C., and Finkel, R. C.: Quaternary glaciation of Muztag Ata and Kongur Shan: Evidence for glacier response to rapid climate changes throughout the Late Glacial and Holocene in westernmost Tibet, Geol. Soc. Am. Bull., 121, 348–365, https://doi.org/10.1130/B26339.1, 2009.
Shangguan, D., Liu, S., Ding, Y., Ding, L., Xiong, L., Cai, D., Li, G., Lu, A., Zhang, S., and Zhang, Y.: Monitoring the glacier changes in the Muztag Ata and Konggur mountains, east Pamirs, based on Chinese Glacier Inventory and recent satellite imagery, Ann. Glaciol., 43, 79–85, https://doi.org/10.3189/172756406781812393, 2006.
Shangguan, D., Liu, S., Ding, Y., Guo, W., XU, B., Xu, J., and Jiang, Z.: Characterizing the May 2015 Karayaylak Glacier surge in the eastern Pamir Plateau using remote sensing, J. Glaciol., 62, 944–953, https://doi.org/10.1017/jog.2016.81, 2016.
Shea, J. M., Immerzeel, W. W., Wagnon, P., Vincent, C., and Bajracharya, S.: Modelling glacier change in the Everest region, Nepal Himalaya, The Cryosphere, 9, 1105–1128, https://doi.org/10.5194/tc-9-1105-2015, 2015.
Shean, D. E., Alexandrov, O., Moratto, Z. M., Smith, B. E., Joughin, I. R., Porter, C., and Morin, P.: An automated, open-source pipeline for mass production of digital elevation models (DEMs) from very-high-resolution commercial stereo satellite imagery, ISPRS J. Photogramm., 116, 101–117, https://doi.org/10.1016/j.isprsjprs.2016.03.012, 2016.
Singh, P., Ramasastri, K. S., and Kumar, N.: Topographical Influence on Precipitation Distribution in Different Ranges of Western Himalayas, Nord. Hydrol., 26, 259–284, 1995.
Stokes, C. R., Popovnin, V., Aleynikov, A., Gurney, S. D., and Shahgedanova, M.: Recent glacier retreat in the Caucasus Mountains, Russia, and associated increase in supraglacial debris cover and supra-/proglacial lake development, Ann. Glaciol., 46, 195–203, https://doi.org/10.3189/172756407782871468, 2007.
Tran, T. A., Raghavan, V., Masumoto, S., Vinayaraj, P., and Yonezawa, G.: A geomorphology-based approach for digital elevation model fusion – case study in Danang city, Vietnam, Earth Surf. Dynam., 2, 403–417, https://doi.org/10.5194/esurf-2-403-2014, 2014.
United States Geological Survey: ASTER GDEM Version 2, available at: https://gdex.cr.usgs.gov/gdex/, last access: 1 January 2018a.
United States Geological Survey: SRTMGL30, available at: https://gdex.cr.usgs.gov/gdex/, last access: 1 January 2018b.
Vaughan, D. G., Comiso, J. C., Allison, I., Carrasco, J., Kaser, G., Kwok, R., Mote, P., Murray, T., Paul, F., Ren, J., Rignot, E., Solomina, O., Steffen, K., and Zhang, T.: Observations: Cryosphere, in: Climate Change 2013: Physical Science Basis. Contribution of Working Group I to the Fifth Assessment Report of the Intergovernmental Panel on Climate Change, edited by: Stocker, T. F., Qin, D., Plattner, G.-K., Tignor, M., Allen, S. K., Boschung, J., Nauels, A., Xia, Y., Bex, V., and Midgley, P. M., Cambridge University Press, Cambridge, United Kingdom and New York, NY, USA, 2013.
Wake, C. P.: Glaciochemical investigations as a tool for determining the spatial and seasonal variation of snow accumulation in the central Karakoram, northern Pakistan, Ann. Glaciol., 13, 279–284, 1989.
Wendt, A., Mayer, C., Lambrecht, A., and Floricioiu, D.: A Glacier Surge of Bivachny Glacier, Pamir Mountains, Observed by a Time Series of High-Resolution Digital Elevation Models and Glacier Velocities, Remote Sensing, 9, 388, https://doi.org/10.3390/rs9040388, 2017.
Winiger, M., Gumpert, M., and Yamout, H.: Karakorum-Hindukush-western Himalaya: Assessing high-altitude water resources, Hydrol. Process., 19, 2329–2338, https://doi.org/10.1002/hyp.5887, 2005.
Zech, R., Abramowski, U., Glaser, B., Sosin, P., Kubik, P. W., and Zech, W.: Late Quaternary glacial and climate history of the Pamir Mountains derived from cosmogenic 10Be exposure ages, Quaternary Res., 64, 212–220, https://doi.org/10.1016/j.yqres.2005.06.002, 2005.