the Creative Commons Attribution 4.0 License.
the Creative Commons Attribution 4.0 License.
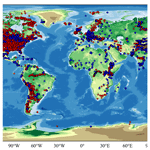
Global Stable Isotope Dataset for Surface Water
Rui Li
Longhu Chen
Xiaoyu Qi
Siyu Lu
Gaojia Meng
Yuhao Wang
Wenmin Li
Zhijie Zheng
Jiangwei Yang
Yani Gun
Hydrogen and oxygen stable isotopes are widely used as tracers of the water cycle, and surface water is an integral part of the water cycle. Compared with other waterbodies, surface water is more susceptible to different natural and anthropogenic factors, and an accurate understanding of surface water changes is of great significance in ensuring regional water security, maintaining ecological balance, and promoting sustainable economic and social development. The spatial and temporal distributions of global surface water stable isotope data are extremely uneven on a global scale due to factors such as observation conditions and instrumental analysis. For this reason, we have compiled and analysed the stable hydrogen and oxygen isotope data in surface water from 22 389 sampling stations worldwide from 1956 to 2023, with 102 511 data records. The results indicate the following: (1) global surface water stable isotopes are gradually depleted from the Equator to the poles and from the coast to the interior. However, there are significant differences in the spatial and temporal distributions of surface water isotopes in different regions. (2) The variation in stable isotopes in surface water is controlled by geographic location, topographic conditions and meteorological factors (especially temperature), and its heterogeneity is considerable. The global stable isotope dataset of surface water provides vital information for an in-depth understanding of the water cycle and climate change. It can provide essential data references for global water resource management and research. The Global Stable Isotope Dataset for Surface Water is available at https://doi.org/10.17632/fs7rwp7fpr.2 (Zhu, 2024).
- Article
(6329 KB) - Full-text XML
-
Supplement
(711 KB) - BibTeX
- EndNote
Water resources are an essential material basis for human survival and are indispensable for maintaining sustainable local socio-economic development, preserving ecological health and maintaining ecosystem stability (Immerzeel et al., 2020; Mehta et al., 2024). Due to human activity and climate change, global hydrological systems have changed in recent decades, increasing ecological vulnerability and sensitivity to climate change (Chahine, 1992; Liu et al., 2021; Satoh et al., 2022). Hydrogen and oxygen isotopes, as kinds of stable isotopes widely present in the water column (Reckerth et al., 2017; Sprenger et al., 2016), are important elements for conducting water cycle studies and have an essential indicative role in the study of the water cycle (Aggarwal et al., 2007; Joussaume et al., 1984; Vystavna et al., 2021). However, due to restrictions imposed by their conditions in various regions of the world, there are a number of difficulties and constraints in the gathering, integration and analysis of current stable isotope data for surface water (Chen et al., 2020; Penna et al., 2018).
In 1960, the Global Network of Isotopes in Precipitation (GNIP) was created by the International Atomic Energy Agency (IAEA) and the World Meteorological Organization (WMO), with the aim of constructing a worldwide monitoring network focusing on the in-depth study of hydrogen and oxygen isotopes in precipitation (Aggarwal et al., 2012). Compared to the monitoring of global stable isotopes in precipitation, global surface water monitoring is lagging behind. In 2002, the IAEA started building the Global Network of Isotopes in Rivers (GNIR), which aims to study the interactions between surface water and groundwater using stable isotopes in runoff and to identify the effects of climate change on river runoff and the effects of human activity on riverine variability (Halder et al., 2015). Many academics worldwide have studied the stable isotope composition of surface water. Around the world, scholars engaging in surface water stable isotope research have achieved many results; for example, a researcher using the US river water stable isotope data mapped the isotope distribution of US river water and used the model to analyse the US river water isotope changes (Bowen et al., 2011; Dutton et al., 2005). In addition, the stable isotope composition of surface water is affected by a variety of hydrological processes such as precipitation, evaporation and surface runoff and can therefore provide valuable information on water cycle processes, water resource management and the impacts of climate change (Bowen et al., 2019; Darling, 2004; Schulte et al., 2011). The source, flow, accumulation and change rule of surface water can be thoroughly understood by analysing and interpreting stable isotope data, which can offer a scientific foundation for water resource management, water resource assessment, and ecological and environmental protection (Dudley et al., 2022). In addition, surface water, as a “link” between groundwater and precipitation (Cooley et al., 2021), offers fresh scientific perspectives on a variety of hydrogeological phenomena, including the hydrogeologic evolution of the basin (Bershaw et al., 2016), groundwater–surface water interactions(Autio et al., 2023), groundwater recharge (Jameel et al., 2023) and precipitation processes (Reckerth et al., 2017).
The establishment of a global stable isotope dataset for surface water is of great significance in the current context of global climate change and water scarcity. The dataset will help integrate and utilise surface water stable isotope data from various regions, improve the accessibility and usability of stable isotope data, and provide more abundant and reliable data support for researchers to carry out global-scale hydrological and environmental studies. In addition, based on the global surface water stable isotope dataset, the analysis of the driving force of meteorological factors in the global surface water stable isotope changes can provide a data basis for research on water resources assessment, climate change adaptation and optimisation of agricultural irrigation. In this work, we present the first global surface water stable isotope dataset, comprising measured, website and reference data. Our goals are as follows: (1) to compile and gather surface water stable isotope data globally and (2) to construct a global surface water stable isotope dataset and to promote the application of this global surface water stable isotope dataset in hydrological, meteorological, ecological and other fields.
2.1 Composition of the dataset
The dataset consists of three main elements: website data (GNIR, http://nucleus.iaea.org/wiser/explore, login required, last access: July 2024; water isotope website, http://wateriso.utah.edu/waterisotopes, last access: July 2024), measured data and reference data. The dataset encompasses 22 432 surface water sampling sites across seven continents (Fig. 1). Since 2015, an ecohydrological observation system has been in operation in the Shiyang River basin in the arid zone of northwestern China, systematically gathering surface water stable isotope data (Fig. S1 in the Supplement) and serving as the primary source of measured data.
With regard to the measured data, surface water sampling sites are chosen, whenever feasible, from places where the water moves quickly because stagnant water is frequently impacted by pollution and evaporation. After the sampling bottle was rinsed three times prior to sampling using water from the sampling site, the bottle was placed below the surface of the water with the mouth facing up and was filled to approximately three-quarters of the bottle's volume. Following the completion of the water sample collection process, the bottles were promptly sealed tightly; their mouths were taped with waterproof tape; and labels bearing the name of the sampling location, the sampling date and additional information were affixed to the bottles. Every collected water sample was kept in a refrigerator to be frozen in order to avoid data errors caused by evaporation.
With regard to the reference data, we added more information to the database by searching for the terms “isotope”, “surface water” and “river” in published papers on the Web of Science. We chose scholarly articles containing isotope data in textual, tabular and graphical formats as the primary source of data to enhance the precision of our data. The aforementioned papers explicitly identified the waterbody type as “surface water”. Alongside isotope data, we gathered spatial and temporal information, including the latitude and longitude of the sampling sites and the exact time of sampling.
Moreover, the meteorological data utilised in this study were sourced from the NCEP-NCAR Reanalysis dataset (https://psl.noaa.gov/data/gridded/data.ncep.reanalysis.html, last access: July 2024) and the CRUTS v.4.07 dataset (https://crudata.uea.ac.uk/cru/data, last access: July 2024). The data utilised for the global climate division are derived from Köppen's global climate classification (Peel et al., 2007) (Fig. S2).
2.2 Data processing
2.2.1 Experiment
Prior to the commencement of the experiment, the samples to be analysed were removed from the refrigerator and transferred to standard 1.5 mL glass sample bottles once they had melted in the room. A filter with a pore size of 0.45 µm and a diameter of 13 mm was then applied to eliminate any contaminants, such as silt and dust, that may have been carried in with the samples during the transfer. All water samples were analysed for stable isotope values using a liquid-water isotope analyser (DLT-100, Los Gatos Research, USA). During the determination process, each water sample was measured six consecutive times. To prevent residual contamination from affecting the results, the first two measurements were discarded, and the stable isotope value was calculated as the average of the last four measurements. The test results obtained are expressed as thousandths deviation from the Vienna Standard Mean Ocean Water (VSMOW):
Here, Rs represents the ratio of 18O 16O or 2H 1H in the collected sample, and Rv is the ratio of 18O 16O or 2H 1H in the Vienna standard sample. The analytical accuracies for δ2H and δ18O are ±0.6 ‰ and ±0.2 ‰, respectively.
2.2.2 Data quality
The collected data included various problems such as missing values, outliers and obvious duplicate entries, as well as sampling date gaps and missing or incorrect latitude and longitude information. Therefore, the collected raw data were pre-processed and screened to eliminate erroneous data.
In addition, we use the liquid-water isotope analyser (LWIA) post-analysis software to examine the measured raw isotope data. Los Gatos Research (LGR) recommends the use of our customised post-processing software to analyse the data. This software uploads the data files, performs all required normalisation and processing, and saves the processed data as readable TXT files. In addition, the LWIA automatically checks for instrumental fault indications, provides a selection of data filters, displays a variety of graphical displays and can be configured by the user. With the LWIA, we can know which raw data values of the sample are wrong and need to be tested again, and we can see the reasons for the data errors. Additionally, all isotope data were thoroughly examined to ensure each entry included clear “longitude”, “latitude”, “sampling time” and “isotope” data. Outliers and duplicates were removed (Fig. 2).
2.3 Methods
Based on previous studies, a one-way analysis of variance (ANOVA) was used to determine the significance (p < 0.05 at a 95 % confidence level) of the slopes and intercepts of the linear regression fits for surface water stable isotope δ2H and δ18O across different climatic regions (Vystavna et al., 2021). Furthermore, the random forest (RF) model can assess the importance of variables. In this study, we employed the RF model to evaluate the impact of various meteorological factors on the stable isotopes of surface water globally. The RF algorithm integrates multiple decision trees to generate a cumulative effect. It predicts regression outcomes based on the average results of these randomised decision trees, employing bootstrapping to minimise the risk of overfitting (Breiman, 2001; Hu et al., 2017). Both root mean square error (RMSE) and mean absolute error (MAE) were utilised to estimate the model's error (Kartal, 2024). The detailed calculation process for RMSE and MAE is described in Sect. S1 in the Supplement.
3.1 Volume, geographic distribution and temporal coverage of datasets
As shown in Fig. 3, a total of 102 561 measurements of stable isotopes of hydrogen and oxygen in surface water were collected for this dataset. This includes 79 525 website data, 1040 measured data and 21 946 reference data. Most of GNIR data are primarily concentrated in a few regions, such as the United States and eastern Europe, and are sparsely distributed globally. To expand our dataset, we incorporated data from published literature. This expanded dataset now covers nearly the entire world, with a relatively even distribution, including regions with data that are traditionally difficult to access, such as Greenland, Antarctica, western Australia and high-altitude mountainous areas (Fig. 1). In terms of time range, the dataset covers the period from 1956 to 2023, and most of the data are distributed from 1990 onwards, which also suggests that the dataset can better characterise the global distribution of stable isotopes in surface water over the past decades.
3.2 Spatial and temporal variations in stable isotopes in global surface waters
The variation in δ2H ranged from −252.48 ‰ to 79.01 ‰, and the variation in δ18O ranged from −26.30 ‰ to 15.41 ‰ over the whole dataset. On a seasonal scale, global surface water stable isotopes typically exhibit pronounced variations, characterised by higher values in summer and lower values in winter (Fig. 4). To better observe these variations across different regions, we classified the globe into five climatic zones – tropical, temperate, arid, continental and polar – based on the Köppen climate zones classification. Across the six climatic zones, stable isotopes of surface water exhibit seasonal variations, with higher values in summer and lower values in winter, except in polar climatic zones. The most pronounced variations occur in arid zones, underscoring the influence of meteorological factors on stable isotopes of surface water.
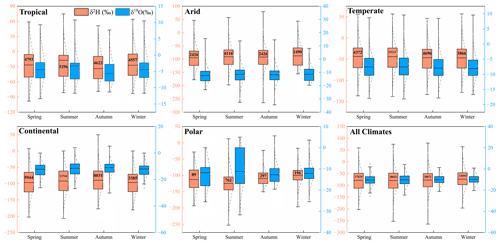
Figure 4Seasonal variation in δ2H and δ18O in surface water in different climatic zones (numbers indicates amount of stable isotope data).
Meanwhile, to better describe the spatial distribution of stable isotopes in global surface water, we conducted an interpolation to map their spatial distribution globally (Fig. 5). Generally, δ2H and δ18O exhibit a consistent trend of gradually decreasing values from equatorial regions to high latitudes and from coastal regions to the inland areas of regions such as Eurasia and North America. This trend is especially pronounced in high-latitude and high-altitude regions, where the values are significantly lower. However, some areas do not exhibit a clear pattern in the distribution of δ2H and δ18O values. This irregularity primarily results from the complex factors influencing runoff generation and water flow concentration processes in various regions. Additionally, the presence of open waterbodies, such as lakes and reservoirs, exacerbates this irregular-distribution phenomenon.
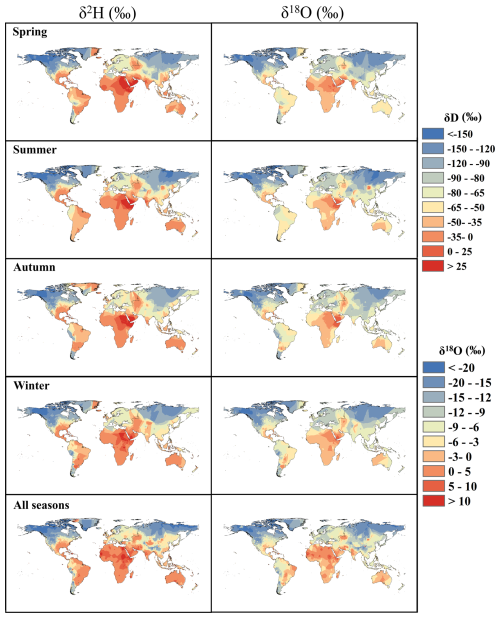
Figure 5Spatial distribution of global surface water δ2H and δ18O in different seasons (unweighted data, using Kriging grid methods).
To better understand the relationship between surface water and precipitation, we compared the spatial interpolation results of surface water isotopes with those of global precipitation isotopes. We found that the isotope distribution in surface water is largely consistent with the isotope distribution in precipitation across most areas (Fig. S3). This consistency primarily arises because surface water is predominantly recharged by precipitation. Moreover, the spatial variation in the isotopic composition of surface water serves as a valuable indicator of its recharge relationship with groundwater and precipitation (Kendall and Coplen, 2001). This is particularly evident in the tropics and at high altitudes, where precipitation serves as the primary source of surface water recharge. In these regions, the spatial distributions of surface water isotopes and precipitation isotopes exhibit a high degree of similarity (Fig. S3).
3.3 Global surface water δ2H and δ18O correlations
Here, we fit δ2H and δ18O to surface waters in six climatic zones, and the results indicated a strong correlation between δ2H and δ18O across the six climate zones (Fig. 6). The relationship between δ2H and δ18O for global surface water is δ2H = 7.92δ18O + 7.80 (R2 = 0.98), which is closer to the intercept and slope of the global meteoric water line (GMWL: δ2H = 8δ18O + 10), and this confirms once again that the source of recharge of global surface water is precipitation. However, the fitted lines of δ2H and δ18O for surface water were significantly different in different climatic zones (Fig. 6), and the fitted lines of δ2H and δ18O exhibited the lowest intercept and slope under an arid climate (δ2H = 7.50 δ18O + 3.30, R2 = 0.98), which also suggests that, under an arid climate, the surface water experienced significant evapotranspiration, which led to the isotopic enrichment of surface water and to δ2H and δ18O values being higher compared to other climatic zones. In the coldest polar climate zone, the fitted line of δ2H and δ18O is δ2H = 5.57δ18O + 17.18 (R2 = 0.95), and the higher slope and intercept indicate that, under the influence of the cold climate, the surface water undergoes little evaporation, and the presence of surface water may be in the form of snow and ice, resulting in significantly lower values of δ2H and δ18O compared to the other climate zones.
3.4 Controlling factors for stable isotopes in surface water
For precipitation stable isotopes, there is a significant “latitude effect” and “continent effect” (Dansgaard, 1964); this pattern of variation is also observed in the stable isotopes of surface water, characterised by a gradual decrease in stable isotope values from low to high latitudes and from coastal to arid inland areas. However, in low-latitude regions near the Equator, where surface water is primarily recharged by precipitation and where climatic factors do not vary significantly with latitude, there is no significant spatial variation in the stable isotopes of surface water.
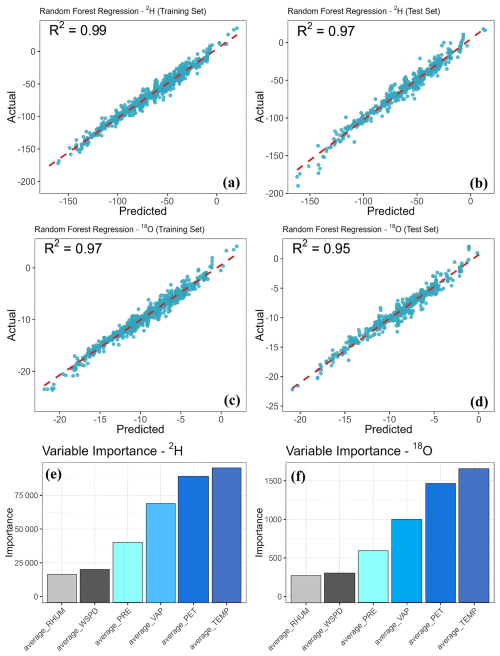
Figure 7The relationship between δ2H and δ18O and meteorological factors was analysed using the RF model. (a) δ2H regression results of the training set. (b) δ2H regression results of the test set. (c) δ18O regression results of the training set. (d) δ18O regression results of the test set. (e) Effect of meteorological factors on δ2H. (f) Effect of meteorological factors on δ18O.
Additionally, numerous studies have demonstrated that the stable isotope composition of surface water is predominantly influenced by climatic factors (Araguás-Araguás et al., 1998; Dansgaard, 1964; Wang et al., 2017). To assess the importance of various meteorological variables in relation to the stable isotopes of surface water globally, we employed an RF model. The RF regression analysis fitted to the stable isotopes of surface water indicated a strong model fit for both the training and test sets. This suggests that variables such as temperature, precipitation, potential evapotranspiration, vapour pressure, wind speed and relative humidity possess significant explanatory power for the stable isotopes of surface water (Fig. 7). The validation results of the RF model demonstrate excellent prediction performance for both δ18O and δ2H, with δ18O showing better prediction accuracy than δ2H, as indicated by the smaller RMSE and MAE values (Table S1). Among the six meteorological factors considered, temperature exerts the strongest influence on surface water stable isotopes. Potential evapotranspiration also exhibits a strong controlling effect, suggesting that temperature and evapotranspiration are the primary factors governing changes in global surface water stable isotopes. Additionally, relative humidity and wind speed demonstrate high explanatory power for variations in surface water stable isotopes. Previous studies have indicated that wind speed and relative humidity significantly influence evaporation from waterbodies (Gallart et al., 2024; Skrzypek et al., 2015), which can subsequently impact surface water stable isotopes. While vapour pressure and precipitation offer weaker explanations for variations in surface water stable isotopes, these factors can largely be attributed to the residence time of surface water and the local hydrological cycle. The residence time of surface water and the characteristics of the local hydrological cycle vary significantly across different regions. Large open waterbodies typically have longer residence times and slower hydrological cycles, resulting in a more enriched isotopic composition of surface water (Feng et al., 2016). In contrast, waterbodies with faster hydrological cycles, such as rivers, may exhibit different isotopic compositions (Ala-aho et al., 2018). However, interpreting these patterns on a large scale requires further investigation and validation.
Simultaneously, for lakes, reservoirs and other large open waterbodies, the controls on surface water stable isotopes can be more complex. Studies have demonstrated that global stable isotope variations in lakes result from the combined effects of solar radiation, evapotranspiration, catchment area size and other factors (Vystavna et al., 2021). These controls vary across different regions, contributing to diverse stable isotopic compositions in surface waters worldwide. For instance, in arid zones, solar radiation primarily controls stable isotopic variations in lakes, whereas, in temperate climatic zones, evaporation and transpiration play a dominant role. Consequently, the controlling factors for surface water stable isotopes vary significantly across different regions. However, overarching patterns suggest that geographic and meteorological factors collectively govern the stable isotopic changes in surface water within a region.
3.5 Challenges and limitations in the construction of surface water isotope datasets and future research directions
At present, due to the limitations of sampling techniques and methods, there may be significant differences in sampling methods and frequencies across various geological environments and hydrogeological conditions. These differences can affect the comparison and analysis of the data. Constructing a comprehensive isotope dataset for surface water requires careful consideration of spatial and temporal coverage to ensure data accuracy and comparability (Ankor et al., 2019). However, due to cost, labour and equipment constraints, as well as the harsh natural conditions in sampling areas, it is challenging to achieve continuous observation of different watersheds over long time frames. This limitation results in some incompleteness of the data in terms of spatial and temporal scales (Penna et al., 2014). In addition, the accuracy of the current stable isotope data has yet to be harmonised due to issues such as sample preservation, analytical techniques and instrumental accuracy. These challenges may lead to problems in the comparability and overall reliability of the data.
In the future, establishing harmonised standards for data collection, storage and sharing will be essential for creating a global isotope database for surface water. Additionally, integrating data from different sources, times and locations will be necessary to develop a more comprehensive global isotope database for surface water (Chen et al., 2024; Zhu et al., 2022). With advances in artificial intelligence, there is a growing trend towards integrating isotope data with hydrologic modelling (Gierz et al., 2017; Nelson et al., 2021). This integration promises to enhance our understanding of hydrologic processes and to improve water resource management practices. Furthermore, it facilitates improvements in the spatial and temporal coverage of data, offering more robust insights into water dynamics and interactions within ecosystems. Meanwhile, within the context of global change, the study of climate change and of isotopes is becoming increasingly integrated and interdisciplinary. In the longer term, there is potential to develop a comprehensive understanding and application of isotope datasets for surface water. This development will rely on integrating expertise from disciplines such as geology, hydrology, meteorology and others, fostering a holistic approach to studying and managing water resources in a changing climate.
The Global Stable Isotope Dataset for Surface Water is now publicly available, and the data can be found at https://doi.org/10.17632/fs7rwp7fpr.2 (Zhu, 2024).
The Global Stable Isotope Dataset for Surface Water provides crucial information for advancing our understanding of the water cycle, climate change and environmental monitoring. In this study, we established a global surface water stable isotope dataset by combining measured data and reference data from existing station data. This approach enriched the dataset and enabled comprehensive analysis across different regions and climatic zones. The results reveal pronounced spatial and temporal variations in the stable isotope composition of global surface water, with significant differences being observed in the isotopic composition of surface water across different climates. The variations in global surface water isotopes are influenced by a combination of geographic and meteorological factors, with temperature and evapotranspiration being among the climatic factors exhibiting strong explanatory power for the isotopic composition of surface water. Observations of stable isotopes in global surface water play a crucial role in enhancing our understanding of the global water cycle, climate change and water resource management. They provide essential data support for interdisciplinary research, helping to uncover connections between hydrological processes, climate variability and environmental changes worldwide. Although we have enriched this dataset as much as possible, there are still regions with sparse data, such as Siberia and eastern Europe. In the future, efforts should focus on strengthening observations in these challenging areas where data availability is limited. Improving the resolution of global surface water stable isotope data can be achieved by integrating interdisciplinary approaches and leveraging artificial intelligence methods. This approach will help fill data gaps, enhance accuracy and provide more comprehensive insights into global water dynamics and environmental changes.
The supplement related to this article is available online at https://doi.org/10.5194/essd-17-2135-2025-supplement.
GZ and RL: writing (original draft preparation). SL and LC: data curation. XQ: writing (reviewing and editing). GM and YW: methodology. WL: investigation. ZZ, JY and YG: software.
The contact author has declared that none of the authors has any competing interests.
Publisher's note: Copernicus Publications remains neutral with regard to jurisdictional claims made in the text, published maps, institutional affiliations, or any other geographical representation in this paper. While Copernicus Publications makes every effort to include appropriate place names, the final responsibility lies with the authors.
We thank the editor, Yuqiang Zhang, and the two anonymous reviewers for providing a list of critical and very valuable comments that helped to improve the paper. The authors would like to thank Annie L. Putman and Gabriel J. Bowen for their contributions to the wiDB database and the International Atomic Energy Agency for their outstanding contributions with respect to the GNIR dataset. Finally, the authors thank all of the researchers and institutions that provided data.
This study was supported by the National Natural Science Foundation of China (grant nos. 42371040 and 41971036), the Key Natural Science Foundation of Gansu Province (grant no. 23JRRA698), the Key Research and Development Program of Gansu Province (grant no. 22YF7NA122), the Gansu Provincial Basic Research Innovation Group Project (grant no. 22JR5RA129), the Western Light Young Scholars Program of the Chinese Academy of Sciences, the Northwest Normal University Major Key Project Cultivation Program (grant no. NWNU-LKZD-202302), and the Northwest Normal University Oasis Scientific Research Achievement Breakthrough Action Plan Project (grant no. NWNU-LZKX-202303).
This paper was edited by Yuqiang Zhang and reviewed by two anonymous referees.
Aggarwal, P. K., Alduchov, O., Araguás Araguás, L., Dogramaci, S., Katzlberger, G., Kriz, K., Kulkarni, K. M., Kurttas, T., Newman, B. D., and Purcher, A.: New capabilities for studies using isotopes in the water cycle, EoS Transactions, 88, 537–538, https://doi.org/10.1029/2007EO490002, 2007.
Aggarwal, P. K., Alduchov, O. A., Froehlich, K. O., Araguas-Araguas, L. J., Sturchio, N. C., and Kurita, N.: Stable isotopes in global precipitation: A unified interpretation based on atmospheric moisture residence time, Geophys. Res. Lett., 39, 2012GL051937, https://doi.org/10.1029/2012GL051937, 2012.
Ala-aho, P., Soulsby, C., Pokrovsky, O. S., Kirpotin, S. N., Karlsson, J., Serikova, S., Manasypov, R., Lim, A., Krickov, I., Kolesnichenko, L. G., Laudon, H., and Tetzlaff, D.: Permafrost and lakes control river isotope composition across a boreal Arctic transect in the Western Siberian lowlands, Environ. Res. Lett., 13, 034028, https://doi.org/10.1088/1748-9326/aaa4fe, 2018.
Ankor, M. J., Tyler, J. J., and Hughes, C. E.: Development of an autonomous, monthly and daily, rainfall sampler for isotope research, J. Hydrol., 575, 31–41, https://doi.org/10.1016/j.jhydrol.2019.04.074, 2019.
Araguás-Araguás, L., Froehlich, K., and Rozanski, K.: Stable isotope composition of precipitation over southeast Asia, J. Geophys. Res., 103, 28721–28742, https://doi.org/10.1029/98JD02582, 1998.
Autio, A., Ala-Aho, P., Rossi, P. M., Ronkanen, A.-K., Aurela, M., Lohila, A., Korpelainen, P., Kumpula, T., Klöve, B., and Marttila, H.: Groundwater exfiltration pattern determination in the sub-arctic catchment using thermal imaging, stable water isotopes and fully-integrated groundwater-surface water modelling, J. Hydrol., 626, 130342, https://doi.org/10.1016/j.jhydrol.2023.130342, 2023.
Bershaw, J., Saylor, J. E., Garzione, C. N., Leier, A., and Sundell, K. E.: Stable isotope variations (δ18O and δD) in modern waters across the Andean Plateau, Geochim. Cosmochim. Ac., 194, 310–324, https://doi.org/10.1016/j.gca.2016.08.011, 2016.
Bowen, G. J., Kennedy, C. D., Liu, Z., and Stalker, J.: Water balance model for mean annual hydrogen and oxygen isotope distributions in surface waters of the contiguous United States, J. Geophys. Res., 116, G04011, https://doi.org/10.1029/2010JG001581, 2011.
Bowen, G. J., Cai, Z., Fiorella, R. P., and Putman, A. L.: Isotopes in the Water Cycle: Regional- to Global-Scale Patterns and Applications, Annu. Rev. Earth Planet. Sci., 47, 453–479, https://doi.org/10.1146/annurev-earth-053018-060220, 2019.
Breiman, L.: Random Forests, Mach. Learn., 45, 5–32, https://doi.org/10.1023/A:1010933404324, 2001.
Chahine, M. T.: The hydrological cycle and its influence on climate, Nature, 359, 373–380, https://doi.org/10.1038/359373a0, 1992.
Chen, L., Wang, Q., Zhu, G., Lin, X., Qiu, D., Jiao, Y., Lu, S., Li, R., Meng, G., and Wang, Y.: Dataset of stable isotopes of precipitation in the Eurasian continent, Earth Syst. Sci. Data, 16, 1543–1557, https://doi.org/10.5194/essd-16-1543-2024, 2024.
Chen, Y., Helliker, B. R., Tang, X., Li, F., Zhou, Y., and Song, X.: Stem water cryogenic extraction biases estimation in deuterium isotope composition of plant source water, P. Natl. Acad. Sci. USA, 117, 33345–33350, https://doi.org/10.1073/pnas.2014422117, 2020.
Cooley, S. W., Ryan, J. C., and Smith, L. C.: Human alteration of global surface water storage variability, Nature, 591, 78–81, https://doi.org/10.1038/s41586-021-03262-3, 2021.
Dansgaard, W.: Stable isotopes in precipitation, Tellus, 16, 436–468, https://doi.org/10.1111/j.2153-3490.1964.tb00181.x, 1964.
Darling, W. G.: Hydrological factors in the interpretation of stable isotopic proxy data present and past: a European perspective, Quaternary Sci. Rev., 23, 743–770, https://doi.org/10.1016/j.quascirev.2003.06.016, 2004.
Dudley, B. D., Yang, J., Shankar, U., and Graham, S. L.: A method for predicting hydrogen and oxygen isotope distributions across a region's river network using reach-scale environmental attributes, Hydrol. Earth Syst. Sci., 26, 4933–4951, https://doi.org/10.5194/hess-26-4933-2022, 2022.
Dutton, A., Wilkinson, B. H., Welker, J. M., Bowen, G. J., and Lohmann, K. C.: Spatial distribution and seasonal variation in 18O 16O of modern precipitation and river water across the conterminous USA, Hydrol. Process., 19, 4121–4146, https://doi.org/10.1002/hyp.5876, 2005.
Feng, X., Lauder, A. M., Posmentier, E. S., Kopec, B. G., and Virginia, R. A.: Evaporation and transport of water isotopologues from Greenland lakes: The lake size effect, Quaternary Sci. Rev., 131, 302–315, https://doi.org/10.1016/j.quascirev.2015.07.029, 2016.
Gallart, F., González-Fuentes, S., and Llorens, P.: Technical note: Isotopic fractionation of evaporating waters: effect of sub-daily atmospheric variations and eventual depletion of heavy isotopes, Hydrol. Earth Syst. Sci., 28, 229–239, https://doi.org/10.5194/hess-28-229-2024, 2024.
Gierz, P., Werner, M., and Lohmann, G.: Simulating climate and stable water isotopes during the Last Interglacial using a coupled climate-isotope model, J. Adv. Model. Earth Syst., 9, 2027–2045, https://doi.org/10.1002/2017MS001056, 2017.
Halder, J., Terzer, S., Wassenaar, L. I., Araguás-Araguás, L. J., and Aggarwal, P. K.: The Global Network of Isotopes in Rivers (GNIR): integration of water isotopes in watershed observation and riverine research, Hydrol. Earth Syst. Sci., 19, 3419–3431, https://doi.org/10.5194/hess-19-3419-2015, 2015.
Hu, X., Belle, J. H., Meng, X., Wildani, A., Waller, L. A., Strickland, M. J., and Liu, Y.: Estimating PM 2.5 Concentrations in the Conterminous United States Using the Random Forest Approach, Environ. Sci. Technol., 51, 6936–6944, https://doi.org/10.1021/acs.est.7b01210, 2017.
Immerzeel, W. W., Lutz, A. F., Andrade, M., Bahl, A., Biemans, H., Bolch, T., Hyde, S., Brumby, S., Davies, B. J., Elmore, A. C., Emmer, A., Feng, M., Fernández, A., Haritashya, U., Kargel, J. S., Koppes, M., Kraaijenbrink, P. D. A., Kulkarni, A. V., Mayewski, P. A., Nepal, S., Pacheco, P., Painter, T. H., Pellicciotti, F., Rajaram, H., Rupper, S., Sinisalo, A., Shrestha, A. B., Viviroli, D., Wada, Y., Xiao, C., Yao, T., and Baillie, J. E. M.: Importance and vulnerability of the world's water towers, Nature, 577, 364–369, https://doi.org/10.1038/s41586-019-1822-y, 2020.
Jameel, Y., Stahl, M., Michael, H., Bostick, B. C., Steckler, M. S., Schlosser, P., Van Geen, A., and Harvey, C.: Shift in groundwater recharge of the Bengal Basin from rainfall to surface water, Commun. Earth Environ., 4, 14, https://doi.org/10.1038/s43247-022-00650-z, 2023.
Joussaume, S., Sadourny, R., and Jouzel, J.: A general circulation model of water isotope cycles in the atmosphere, Nature, 311, 24–29, https://doi.org/10.1038/311024a0, 1984.
Kartal, V.: Machine learning-based streamflow forecasting using CMIP6 scenarios: Assessing performance and improving hydrological projections and climate change, Hydrol. Process., 38, e15204, https://doi.org/10.1002/hyp.15204, 2024.
Kendall, C. and Coplen, T. B.: Distribution of oxygen-18 and deuterium in river waters across the United States, Hydrol. Process., 15, 1363–1393, https://doi.org/10.1002/hyp.217, 2001.
Liu, M., Vecchi, G., Soden, B., Yang, W., and Zhang, B.: Enhanced hydrological cycle increases ocean heat uptake and moderates transient climate change, Nat. Clim. Chang., 11, 848–853, https://doi.org/10.1038/s41558-021-01152-0, 2021.
Mehta, P., Siebert, S., Kummu, M., Deng, Q., Ali, T., Marston, L., Xie, W., and Davis, K. F.: Half of twenty-first century global irrigation expansion has been in water-stressed regions, Nat. Water, 2, 254–261, https://doi.org/10.1038/s44221-024-00206-9, 2024.
Nelson, D. B., Basler, D., and Kahmen, A.: Precipitation isotope time series predictions from machine learning applied in Europe, P. Natl. Acad. Sci. USA, 118, e2024107118, https://doi.org/10.1073/pnas.2024107118, 2021.
Peel, M. C., Finlayson, B. L., and McMahon, T. A.: Updated world map of the Köppen-Geiger climate classification, Hydrol. Earth Syst. Sci., 11, 1633–1644, https://doi.org/10.5194/hess-11-1633-2007, 2007.
Penna, D., Ahmad, M., Birks, S. J., Bouchaou, L., Brenčič, M., Butt, S., Holko, L., Jeelani, G., Martínez, D. E., Melikadze, G., Shanley, J. B., Sokratov, S. A., Stadnyk, T., Sugimoto, A., and Vreča, P.: A new method of snowmelt sampling for water stable isotopes, Hydrol. Process., 28, 5637–5644, https://doi.org/10.1002/hyp.10273, 2014.
Penna, D., Hopp, L., Scandellari, F., Allen, S. T., Benettin, P., Beyer, M., Geris, J., Klaus, J., Marshall, J. D., Schwendenmann, L., Volkmann, T. H. M., von Freyberg, J., Amin, A., Ceperley, N., Engel, M., Frentress, J., Giambastiani, Y., McDonnell, J. J., Zuecco, G., Llorens, P., Siegwolf, R. T. W., Dawson, T. E., and Kirchner, J. W.: Ideas and perspectives: Tracing terrestrial ecosystem water fluxes using hydrogen and oxygen stable isotopes – challenges and opportunities from an interdisciplinary perspective, Biogeosciences, 15, 6399–6415, https://doi.org/10.5194/bg-15-6399-2018, 2018.
Reckerth, A., Stichler, W., Schmidt, A., and Stumpp, C.: Long-term data set analysis of stable isotopic composition in German rivers, J. Hydrol., 552, 718–731, https://doi.org/10.1016/j.jhydrol.2017.07.022, 2017.
Satoh, Y., Yoshimura, K., Pokhrel, Y., Kim, H., Shiogama, H., Yokohata, T., Hanasaki, N., Wada, Y., Burek, P., Byers, E., Schmied, H. M., Gerten, D., Ostberg, S., Gosling, S. N., Boulange, J. E. S., and Oki, T.: The timing of unprecedented hydrological drought under climate change, Nat. Commun., 13, 3287, https://doi.org/10.1038/s41467-022-30729-2, 2022.
Schulte, P., Van Geldern, R., Freitag, H., Karim, A., Négrel, P., Petelet-Giraud, E., Probst, A., Probst, J.-L., Telmer, K., Veizer, J., and Barth, J. A. C.: Applications of stable water and carbon isotopes in watershed research: Weathering, carbon cycling, and water balances, Earth-Sci. Rev., 109, 20–31, https://doi.org/10.1016/j.earscirev.2011.07.003, 2011.
Skrzypek, G., Mydłowski, A., Dogramaci, S., Hedley, P., Gibson, J. J., and Grierson, P. F.: Estimation of evaporative loss based on the stable isotope composition of water using Hydrocalculator, J. Hydrol., 523, 781–789, https://doi.org/10.1016/j.jhydrol.2015.02.010, 2015.
Sprenger, M., Leistert, H., Gimbel, K., and Weiler, M.: Illuminating hydrological processes at the soil-vegetation-atmosphere interface with water stable isotopes, Rev. Geophys., 54, 674–704, https://doi.org/10.1002/2015RG000515, 2016.
Vystavna, Y., Harjung, A., Monteiro, L. R., Matiatos, I., and Wassenaar, L. I.: Stable isotopes in global lakes integrate catchment and climatic controls on evaporation, Nat. Commun., 12, 7224, https://doi.org/10.1038/s41467-021-27569-x, 2021.
Wang, S., Zhang, M., Crawford, J., Hughes, C. E., Du, M., and Liu, X.: The effect of moisture source and synoptic conditions on precipitation isotopes in arid central Asia, J. Geophys. Res.-Atmos., 122, 2667–2682, https://doi.org/10.1002/2015JD024626, 2017.
Zhu, G.: Global Stable Isotope Dataset for Surface Water, V2, Mendeley Data [data set], https://doi.org/10.17632/fs7rwp7fpr.2, 2024.
Zhu, G., Liu, Y., Shi, P., Jia, W., Zhou, J., Liu, Y., Ma, X., Pan, H., Zhang, Y., Zhang, Z., Sun, Z., Yong, L., and Zhao, K.: Stable water isotope monitoring network of different water bodies in Shiyang River basin, a typical arid river in China, Earth Syst. Sci. Data, 14, 3773–3789, https://doi.org/10.5194/essd-14-3773-2022, 2022.