the Creative Commons Attribution 4.0 License.
the Creative Commons Attribution 4.0 License.
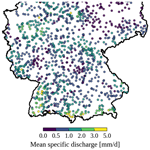
CAMELS-DE: hydro-meteorological time series and attributes for 1582 catchments in Germany
Alexander Dolich
Eduardo Acuña Espinoza
Pia Ebeling
Björn Guse
Jonas Götte
Sibylle K. Hassler
Corina Hauffe
Ingo Heidbüchel
Jens Kiesel
Mirko Mälicke
Hannes Müller-Thomy
Michael Stölzle
Larisa Tarasova
Comprehensive large-sample hydrological datasets, particularly the CAMELS datasets (Catchment Attributes and MEteorology for Large-sample Studies), have advanced hydrological research and education in recent years. These datasets integrate extensive hydro-meteorological observations with landscape features, such as geology and land use, across numerous catchments within a national framework. They provide harmonised large-sample data for various purposes, such as assessing the impacts of climate change or testing hydrological models on a large number of catchments. Furthermore, these datasets are essential for the rapid progress of data-driven models in hydrology in recent years. Despite Germany's extensive hydro-meteorological measurement infrastructure, it has lacked a consistent, nationwide hydrological dataset, largely due to its decentralised management across different federal states. This fragmentation has hindered cross-state studies and made the preparation of hydrological data labour-intensive. The introduction of CAMELS-DE represents a step forward in bridging this gap. CAMELS-DE includes 1582 streamflow gauges with hydro-meteorological time series data covering up to 70 years (median length of 46 years and a minimum length of 10 years), from January 1951 to December 2020. It includes consistent catchment boundaries with areas ranging from 5 to 15 000 km2 along with detailed catchment attributes covering soil, land cover, hydrogeologic properties, and data on human influences. Furthermore, it includes a regionally trained long short-term memory (LSTM) network and a locally trained HBV (Hydrologiska Byråns Vattenbalansavdelning) model that were used as quality control and that can be used to fill gaps in discharge data or act as baseline models for the development and testing of new hydrological models. Given the large number of catchments, including numerous relatively small ones (636 catchments < 100 km2), and the time series length of up to 70 years (166 catchments with 70 years of discharge data), CAMELS-DE is one of the most comprehensive national CAMELS datasets available and offers new opportunities for research, particularly in studying long-term trends and runoff formation in small catchments and in analysing catchments with strong human influences. This article describes CAMELS-DE version 1.0, which is available at https://doi.org/10.5281/zenodo.13837553 (Dolich et al., 2024).
- Article
(5918 KB) - Full-text XML
- BibTeX
- EndNote
The CAMELS (Catchment Attributes and MEteorology for Large-sample Studies) datasets have become a cornerstone within the hydrological community for their comprehensive and consistent integration of hydrological and meteorological data across entire countries, including the USA, UK, Australia, Brazil, Chile, and others (e.g. Addor et al., 2017; Coxon et al., 2020). These datasets combine catchment attributes (e.g. land use, geology, and soil properties), hydrological time series (e.g. water level and discharge), and meteorological time series (e.g. precipitation and temperature) for a multitude of catchments typically within a single country. A distinctive feature of CAMELS datasets is their role as a benchmark for hydrological modelling and large-sample analysis, enabling the comparison of hydrological models and the validation of water resources management strategies across diverse landscapes and climates (Brunner et al., 2021). Particularly the CAMELS-US dataset has thereby formed the basis for the ongoing rise of machine learning methods in hydrology (e.g. Kratzert et al., 2019).
Despite the widespread adoption and utility of CAMELS datasets in research, teaching, and practical applications globally, Germany with its extensive hydro-meteorological measurement network has no comprehensive and harmonised dataset yet. While there are large-sample hydrological datasets that either cover parts of Germany (Klingler et al., 2021), i.e. only a fraction of the available national hydrological data (Färber et al., 2023), or focus on catchment water quality and thus cover a lower sampling frequency (Ebeling et al., 2022), the absence of a full CAMELS dataset that includes harmonised, daily, high-quality national hydrological and meteorological data together with catchment attributes and catchment boundaries derived from national and international products limits the potential for comprehensive analyses and advancements in hydrological research and practice. The CAMELS-DE dataset addresses this gap (Dolich et al., 2024). CAMELS-DE compiles discharge, water levels, catchment attributes, and catchment boundaries together with a suite of meteorological time series and catchment attributes for 1582 catchments across Germany. Furthermore, the dataset includes discharge simulations from two sources: a regionally trained long short-term memory (LSTM) network (Hochreiter and Schmidhuber, 1997; Hochreiter, 1998) and a locally trained conceptual HBV model (Hydrologiska Byråns Vattenbalansavdelning; Bergström and Forsman, 1973; Seibert, 2005; Feng et al., 2022). These simulations can serve as a benchmark for future hydrological modelling studies in Germany or help fill data gaps in hydrological time series. Each component of the CAMELS-DE processing pipeline is fully containerised (see Sect. 7), which solves code dependency issues and generally contributes to the traceability, comprehensiveness, and reproducibility of the generation of CAMELS-DE. This study introduces not only a comprehensive dataset but also a suite of tools designed to generate reproducible hydrological datasets from the provided raw data. In the following sections, we provide a comprehensive description of all data contained within CAMELS-DE, including (1) its source data, (2) how the time series and attributes were produced, and (3) a discussion of the associated limitations and uncertainties. The structure of this paper (and also the corresponding dataset) closely mirrors that of the CAMELS-UK (Coxon et al., 2020) and CAMELS-CH (Höge et al., 2023) studies, ensuring comparability of the datasets while maintaining distinct elements that are not identical but closely related.
CAMELS-DE brings together hydrological data, consisting of daily measurements of discharge (m3 s−1) and water levels (m) from 13 German federal state agencies, namely the Landesanstalt für Umwelt Baden-Württemberg (LUBW, “Nomenclature of territorial units for statistics” (NUTS) level 1: DE1); Bayerisches Landesamt für Umwelt (LfU-Bayern, DE2); Landesamt für Umwelt Brandenburg (LfU-Brandenburg, DE4); Hessisches Landesamt für Naturschutz, Umwelt und Geologie (HLNUG, DE7); Landesamt für Umwelt, Naturschutz und Geologie Mecklenburg-Vorpommern (LUNG MV, DE8); Niedersächsischer Landesbetrieb für Wasserwirtschaft, Küsten- und Naturschutz, Landesamt für Natur (NLWKN, DE9); Umwelt und Verbraucherschutz Nordrhein-Westfalen (LANUV NRW, DEA); Landesamt für Umwelt Rheinland-Pfalz (LUA-Rheinland Pfalz, DEB); Landesamt für Umwelt- und Arbeitsschutz Saarland (LUA, DEC); Landesamt für Umwelt, Landwirtschaft und Geologie Sachsen (LfULG, DED); Landesamt für Umweltschutz Sachsen-Anhalt (LAU, DEE); Landesamt für Landwirtschaft, Umwelt und ländliche Räume Schleswig-Holstein (LLUR, DEF); and Thüringer Landesamt für Umwelt, Bergbau und Naturschutz (TLUBN, DEG). The only federal states not included are the city states of Bremen, Hamburg, and Berlin, which together account for less than 0.6 % of Germany's area, ensuring that the CAMELS-DE dataset remains representative for Germany.
Meteorological data, specifically precipitation, temperature, relative humidity, and radiation, were obtained from the Deutscher Wetterdienst (DWD, German weather service), from the HYRAS dataset (DWD-HYRAS, 2024). Spatially aggregated catchment attributes were obtained from various sources. From the European Union, we incorporated open-access datasets from Copernicus, the EU's earth observation programme, in particular the Copernicus GLO-30 DEM (global 30 m digital elevation model; EU-DEM, 2022) for information about topography and the CORINE Land Cover 2018 dataset (CLC, 2018) for information about land cover. Soil attributes were derived from the global SoilGrids250m dataset (Poggio et al., 2021). Hydrogeological catchment attributes were derived from the “Hydrogeologische Übersichtskarte von Deutschland 1:250.000” (HGM250, 2019), provided by the Bundesanstalt für Geowissenschaften und Rohstoffe (BGR), while information about human influences, e.g. dams or weirs, was sourced from Speckhann et al. (2021).
For CAMELS-DE, we sourced discharge (m3 s−1), water level data (m), and metadata for 2964 gauges and water level stations from the different federal state agencies (see Sect. 2). We created a subset of the data by only selecting measurement stations that contained all required information, such as gauge name, location, and catchment area in their metadata (n=2700 stations); have at least a total of 10 years of discharge data, which must not necessarily be continuous (n=2227 stations); have a catchment area larger than 5 km2 and smaller than 15 000 km2 (n=2586 stations); have a catchment area located entirely within the borders of Germany (n=2298 stations); and meet the condition where the derived catchment area does not differ by more than 20 % from the reported value by the federal states (n=2164 stations; see Sect. 3.1). These requirements were established based on the following rationale: a minimum of 10 years of discharge data are necessary to ensure an adequate time series length for hydrological modelling and for calculating hydrological signatures. The minimum catchment area of 5 km2 was chosen to match the 1×1 km resolution of the precipitation raster product, ensuring that multiple raster cells intersect with the catchment boundary. The upper limit was set because catchments larger than 15 000 km2 are predominantly influenced by human activities and often extend beyond Germany's borders, necessitating their exclusion. The 20 % discrepancy between derived and reported catchment areas was arbitrarily chosen as an acceptable threshold for mass balance errors. This threshold prevents the inclusion of catchments with significantly inaccurate delineations while avoiding the exclusion of too much data (see Fig. 2b). Catchments partially located outside Germany's borders were excluded to avoid complications with cross-border data, especially given the absence of open, high-quality meteorological data from the DWD beyond Germany's national borders from 1951 to 2020. These criteria resulted in a subset of 1582 gauges for the CAMELS-DE dataset, which provides a reliable representation of hydrological processes in Germany (Fig. 1c, d).
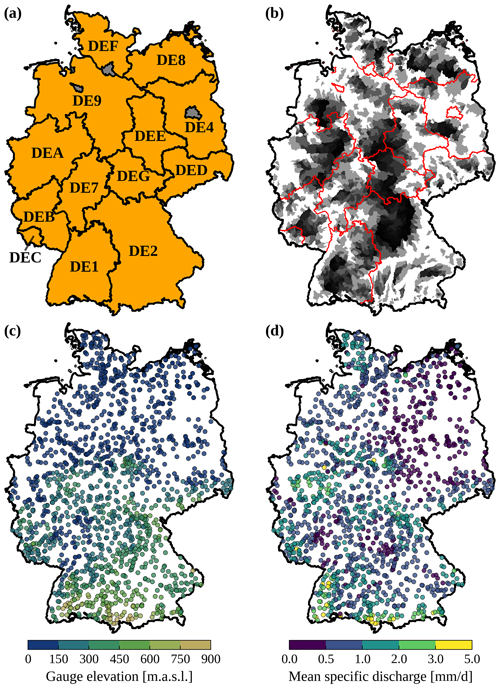
Figure 1Panel (a) shows the German federal states labelled with their NUTS level 1 ID as used for the CAMELS-DE gauge IDs. Panel (b) shows all 1582 catchments provided in CAMELS-DE; the geometries of the catchments are shown transparently, so a darker colour means that the geometries of the catchments in that area overlap; the darker the colour, the higher the density of catchments in that area. Panel (c) and panel (d) show the location of all 1582 gauging stations in CAMELS-DE; in panel (c), the locations are coloured according to the elevation of the gauging station, while in panel (d) the locations are coloured according to their mean specific discharge value. Borders of Germany: © GeoBasis-DE/BKG (VG250, 2023).
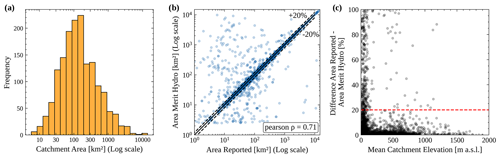
Figure 2Panel (a) shows the distribution of CAMELS-DE catchment areas on a logarithmic scale. Panel (b) shows the accuracy of catchment areas derived using MERIT Hydro compared to the area reported by the federal agencies; the dashed lines indicate the ±20 % error tolerance that was set for catchment selection. Panel (c) shows the absolute relative difference between the reported area by the federal states and the MERIT Hydro area against the mean catchment elevation. The red line marks the threshold of 20 % allowed difference for the inclusion of a catchment in the CAMELS-DE dataset.
3.1 Catchment boundaries
Not all state authorities provided official catchment boundaries for their gauging stations, and the methods used by the federal states to derive these boundaries are not uniform and remain unclear. Therefore, we tested two different global catchment datasets, HydroSHEDS (Lehner et al., 2021) and MERIT Hydro (Yamazaki et al., 2019), to derive a consistent set of catchment boundaries across Germany for the CAMELS-DE dataset. For that, we compared the catchment areas determined with HydroSHEDS and MERIT Hydro to the catchment areas reported by the state authorities. This comparison was possible because all federal states shared the area of the catchments while not always sharing the actual catchment boundaries. Overall, the comparison revealed that MERIT Hydro has lower errors between the reported and derived catchment areas compared to HydroSHEDS. Among other reasons, this is because MERIT Hydro derives the catchment boundaries directly at the gauge locations provided by the federal states (see Sect. 3.2). The comparison between MERIT Hydro and HydroSHEDS was further supported by extensive manual assessments, involving the visual inspection of numerous catchments to evaluate their shapes and alignments when the federal state provided the data. Consequently, MERIT Hydro was used for the derivation of catchment boundaries for CAMELS-DE. Note that the derivation of the catchment boundaries is a major source of uncertainty as the meteorological time series and the catchment attributes are dependent on the catchment boundaries. To minimise the uncertainty of the catchment delineation, we only included catchments with a deviation of up to 20 % from the catchment area reported by the federal agencies (Fig. 2b). We report the original catchment area as (area_metadata) and the MERIT-Hydro-based area (area) in the table of topographic attributes (Table 2).
3.2 Catchment boundaries derived from MERIT Hydro
MERIT (Multi-Error-Removed Improved-Terrain) Hydro was released by Yamazaki et al. (2019), providing a global hydrography dataset based on the MERIT DEM and various maps of water bodies (e.g. global 3 arcsec water body map by Yamazaki et al., 2017). It includes information such as flow direction, flow accumulation, adjusted elevations for hydrological purposes, and the width of river channels. The delineator.py package (Heberger, 2023) was used to delineate catchment boundaries. The method automatically derives catchment boundaries from the MERIT Hydro dataset based on the longitude and latitude of a gauging station and snaps the catchment pour point to the closest stream. Figure 1b shows all derived CAMELS-DE catchments using MERIT Hydro within the German borders. The median catchment area within CAMELS-DE is 129.1 km2 (Fig. 2a). Compared to other CAMELS datasets, CAMELS-DE includes a large number of relatively small catchments with an area of less than 100 km2 (i.e. 636 catchments, CAMELS-GB: 242 catchments, CAMELS-US: 142). Uncertainties in catchment delineation arise when comparing areas reported by federal states with those derived from MERIT Hydro, as shown in Fig. 2b, and these discrepancies are not uniformly distributed across Germany. They tend to be higher in the flat lowland regions with minimal topography (Fig. 2c), particularly in the federal states in the north and east of Germany. Consequently, a large number of catchments are excluded from the CAMELS-DE dataset in the northern parts of Germany due to mismatches between reported and estimated areas. In the federal states of Brandenburg (DE4) and Mecklenburg–Western Pomerania (DE8), for example, we received 447 gauging stations, but given the uncertainty of the delineation in flat areas, only 277 of them showed a deviation of less than 20 % from the reported area. In contrast, in the more mountainous state of Baden-Württemberg (DE1), 225 of 241 catchments met this criterion. As we report both the catchment areas provided by the federal states and those estimated by MERIT Hydro, the differences between these two measurements can be used to select or exclude catchments where there are significant uncertainties in the catchment shape and correspondingly in the derived static and dynamic attributes.
CAMELS-DE includes three sets of hydro-meteorological daily time series, as detailed in Table 1, covering the period from 1 January 1951 to 31 December 2020. These datasets are observed hydrologic time series (e.g. station discharge and water levels), observed meteorologic time series (e.g. precipitation, temperature, humidity, and radiation), and simulated hydro-meteorologic time series (e.g. discharge simulated by a LSTM and a HBV model, including estimated evapotranspiration). Note that we do not include any information on evaporation in the non-simulated time series data, as we only include observation-based data here. However, a time series of potential evaporation based on the temperature-based Hargreaves methodology is included in the simulated data (see Sect. 6.2 for more details). However, due to the simplicity of the chosen approach, the potential evapotranspiration time series are highly uncertain, and one should exercise caution when using them.
Table 1Catchment-specific hydro-meteorological variables available as daily time series in CAMELS-DE.
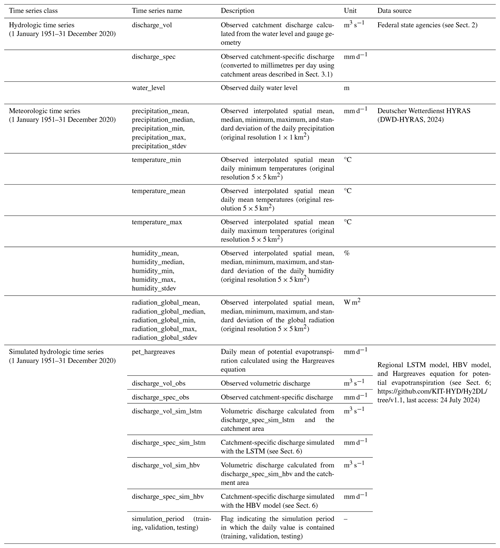
All meteorological forcing data within CAMELS-DE are sourced from the HYRAS datasets, which are based on the interpolation of meteorological station data (DWD-HYRAS, 2024). This interpolation was conducted by the DWD (see Sect. 4.1, 4.2, and 4.3). The reliability of these datasets can be compromised by the individual interpolation methods employed (see Sect. 4.1 to 4.3). In addition, inaccuracies in meteorological measurements can introduce uncertainties in the generated grid fields, especially given the extended timescale of 70 years, which may include changes in location and sensor types. Another source of uncertainty is the fact that the number of stations used in the interpolation process varies over time, mirroring changes in the measurement network. For example, the number of stations used for interpolating precipitation data fluctuates, starting at around 4500 in 1951, peaking at approximately 7500 in 2000, and then decreasing to approximately 5000 by 2020. In contrast, the number of stations used for radiation interpolation shows a consistent increase over the years, though the total number remains significantly lower, reaching about 900 stations by 2020. This uncertainty is crucial to consider when comparing data across different years, particularly if the focus is on a single or a few catchments in a certain area. Finally, we use the “exact extract” method, which ensures that raster cells that are only partially covered are treated properly as they are weighted by the proportion of the cell that is covered; that is, a raster cell that is only 20 % covered by the catchment is only weighted by 20 % when we aggregate to the spatial catchment mean (Fig. 3a illustrates partially covered cells at the catchment boundary). This is particularly important when deriving meteorological data for very small catchment areas. Although this approach also aids in comparing products with different resolutions, it is important to consider that the spatial resolution of the precipitation data, at 1×1 km, offers finer detail compared to the 5×5 km resolution used for temperature, humidity, and radiation data. This difference is crucial when comparing these datasets within smaller catchments.
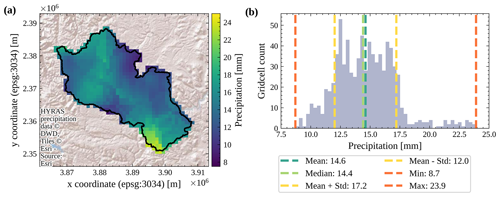
Figure 3Panel (a) shows the catchment boundaries (black line) of the Kirchen-Hausen catchment in Baden-Württemberg overlaid by a clipped daily precipitation field from the HYRAS dataset on the date of 20 February 1951. Panel (b) shows the spatial distribution of rainfall during the same high precipitation event as (a) over the catchment on 20 February 1951 and the statistical moments (mean, median, standard deviation, minimum, and maximum) derived from the spatial distribution.
4.1 Precipitation
CAMELS-DE utilises precipitation data (mm d−1) with daily resolution, sourced from the HYRAS-DE-PRE dataset v5.0 (HYRAS-DE-PRE, 2022). We have calculated daily spatial minimum, mean, median, maximum, and standard deviation of the rainfall field over the catchment for each day. We estimated these statistical measures, rather than just the mean, because this allows us to capture spatial variations and patterns that can be crucial for event characterisation or rainfall–runoff modelling, as illustrated in Fig. 3. The HYRAS-DE-PRE v5.0 dataset is produced using the REGNIE interpolation method (Rauthe et al., 2013), which employs daily measured values from meteorological stations to generate an interpolated product on a 1×1 km grid. A detailed description of the interpolation method and the related uncertainties can be found in the official data description (HYRAS-DE-PRE, 2022).
4.2 Temperature and relative humidity
CAMELS-DE employs daily temperature (°C) and relative humidity (%), derived from the HYRAS-DE-TAS (daily mean temperature; HYRAS-DE-TAS, 2022), TASMIN (daily minimum temperature; HYRAS-DE-TASMIN, 2022), TASMAX (daily maximum temperature; HYRAS-DE-TASMAX, 2022), and HURS (daily average relative humidity; HYRAS-DE-HURS, 2022) datasets v5.0, which cover the period from 1951 to 2020 on a 5 km × 5 km grid. This includes the spatial mean, median, and standard deviation of temperature from HYRAS-DE-TAS, alongside the spatial minimum and maximum temperatures from TASMIN and TASMAX, respectively. Additionally, for humidity, we integrate daily minimum, mean, median, maximum, and standard deviation values across the catchment area. The temperature and humidity data are based on interpolated station values (Razafimaharo et al., 2020). This interpolation method involves a nonlinear regression at each time step, aiming to estimate regional vertical temperature profiles across 13 subregions. These subregions are delineated based on criteria such as weather divides, proximity to the coast, and the extent of north–south variation. A detailed description of the interpolation method and the related uncertainties can be found in the corresponding data descriptions (HYRAS-DE-TAS, 2022; HYRAS-DE-TASMIN, 2022; HYRAS-DE-TASMAX, 2022; HYRAS-DE-HURS, 2022).
4.3 Radiation
The CAMELS-DE dataset utilises daily mean global radiation data (in W m−2), derived from the HYRAS-DE-RSDS dataset v3.0 (HYRAS-DE-RSDS, 2023), that cover a period from 1951 to 2020 with a 5 km × 5 km grid. We have derived daily, spatial minimum, mean, median, maximum, and standard deviation of the radiation field over the catchment for each day. The global radiation dataset (RSDS) integrates station measurement data (including sunshine duration and global radiation), satellite data, and ERA5 data (Muñoz-Sabater et al., 2021). A detailed description of the interpolation method and the related uncertainties can be found in the official data description (HYRAS-DE-RSDS, 2023).
4.4 Discharge and water levels
Observed discharge and water level data were requested from 13 state agencies (see Sect. 2) as time series recorded at the gauging stations (Table 1). The number of stations with daily discharge data available per year increases in time from 187 on 1 January 1951 to a maximum of 1486 between November 2010 and February 2011 (Fig. 4a). The number of stations with water level data is generally lower, starting at 110 stations on 1 January 1951 and reaching a maximum of 1471 stations between March 2015 and December 2015. The time series span a maximum of 70 years, with each measuring station providing at least 10 years of data between January 1951 and December 2020 (Fig. 4b). These 10 years do not need to be consecutive but typically are. The median time series length of discharge is 46 years, while the median time series length of water level is 40 years. There is a sharp drop-off in Fig. 4a of 137 stations without data from 2017 to 2018 as the provided data from NLWKN (Lower Saxony, DE9) only range until the end of 2017. Another anomaly in Fig. 4a is the drop immediately followed by a rise in the year 2020, which is due to the fact that all measuring stations in Rhineland-Palatinate (DEB) show a gap in the discharge data from 10 to 15 February 2020 and in the water level data from 13 to 15 February 2020. No explanation could be found for this gap. The remaining data after the gap were manually quality controlled by visual inspection of the observed and simulated time series, and we found no reason to exclude these data. In total, CAMELS-DE includes 156 stations for which the entire temporal range of 70 years of discharge data are available and for which a maximum of 2 % of the data are missing in this period. There are 85 stations where this is the case for water level data.
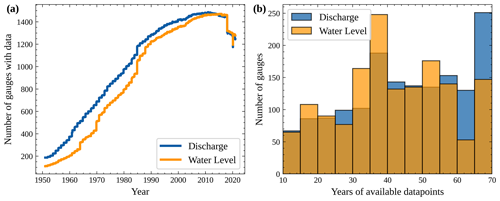
Figure 4Panel (a) shows the number of gauging stations with available discharge (blue) and water level data (orange) in the period from 1951 to 2020, taking into account data gaps, i.e. the data must actually be available at the respective time. Panel (b) shows a histogram of the years of available data points for all measuring stations, i.e. the length of the time series minus eventual gaps in the time series.
4.5 Discharge and water levels – quality control
The quality control of all discharge and water level data was conducted by the respective federal states (quality controlled data were requested). However, the specific methods employed in this quality control are not the same across the states, and they are not documented in some cases. Typically, quality control means that a technical clerk has visually inspected the hydrological time series data. To account for this uncertainty, we conducted an additional review of all time series data for high negative values and unrealistically high outliers, and we replaced such data points with “NaN” (not a number). We were conservative in these cases, and we only deleted values that were clear data errors to avoid removing potential extreme flood events from the time series. This adjustment was necessary in eight catchments and is documented in the processing pipeline to assure reproducibility. Please note that negative discharge values are still possible in the CAMELS-DE dataset due to the influence of the tide in the northern part of Germany or due to human influences related to water resources management. Moreover, we assessed the hydro-meteorological time series using both a hydrological model and a data-driven model. This analysis helped us identify catchments with weak correlations between meteorological conditions and hydrological responses, as well as catchments in which the mass balance is far from being closed. All catchments that exhibited a low model performance of the HBV model were subjected to manual visual inspection, resulting in the removal of 14 catchments (for more details we refer the reader to Sect. 6).
In addition to the daily time series of hydro-meteorological variables available in CAMELS-DE, the dataset also includes a series of static catchment attributes which are considered time invariant and include information about topography (Sect. 5.1); hydroclimatic signatures (Sect. 5.2); and catchment attributes covering land cover (Sect. 5.3), soil (Sect. 5.4), hydrogeology (Sect. 5.5), and human influences (Sect. 5.6).
5.1 Location and topography
For CAMELS-DE, we developed a system of catchment IDs, since the official IDs used by the federal states are inconsistent beyond federal state boundaries. However, the official IDs are contained in the topographic attributes of the dataset (Table 2). The gauge IDs in CAMELS-DE are based on the NUTS classification, which divides the EU territory hierarchically according to administrative boundaries. In Germany, the first hierarchical level, NUTS 1, provides a code for each federal state (e.g. DE7 for Hessen, DED for Saxony; Fig. 1b). We assign an ID code to each gauge as follows. The ID of each gauge starts with the NUTS 1 code of the corresponding federal state. For each federal state, the gauges are coded in arbitrary order starting from 10000 for the first gauge and adding a step of 10 for each following gauge (DE710000 for the first station in Hessen, DE710010 for the second station, DE710020 for the third station, etc.). This system ensures consistency of the gauge IDs in Germany and additionally provides the information about the federal state of each gauge. Topographic attributes such as the location (coordinate systems WGS84 and ETRS89), gauge elevation (m), and catchment area (km2) were provided by the federal agencies; the area of the MERIT Hydro catchment is also provided. Additionally, we derived the gauge point elevation (m) and basic statistical variables (min, mean, median, 5th and 95th percentiles, max) of the catchment elevation (m) from the GLO-30 DEM. CAMELS-DE additionally provides the location of all gauging stations and catchment boundaries as a shapefile and a GeoPackage file.
5.2 Climate and hydrology
For the CAMELS-DE dataset, we calculated long-term climatic and hydrological signatures in line with the attributes found in CAMELS-CH (covering the period 1981–2020) and CAMELS-UK (covering the period 1970–2015), with the difference that we cover the period 1951–2021 (see Table 2). Both types of attributes are calculated based solely on complete hydrological years with respect to the discharge (1 October to 30 September of the following year; again in line with the definition of a hydrological year chosen in CAMELS-UK and CAMELS-CH), with a maximum tolerance of 5 % missing values per hydrological year, ensuring robustness in the data used for analysis. If a specific catchment has discharge data for only a limited number of hydrologic years, we calculate the climatic and hydrological indices for those same years to maintain consistency across all CAMELS datasets and across the climatic and hydrological attributes.
For each catchment, the hydrologic attributes include values for the mean specific discharge (mm d−1); the runoff ratio; the start and end dates of available discharge data; the percentage of days on which discharge data are available (%); the slope of the flow duration curve between the log-transformed 33rd and 66th percentiles; the number of days after which the cumulative discharge since 1 October reaches half of the annual discharge (day of year); the 5th and 95th percentiles of specific discharge (mm d−1); and the frequency of high-flow, low-flow, and zero-flow days (d yr−1) together with the average duration of high-flow and low-flow events (days of year). The climatic attributes are calculated on the basis of the HYRAS meteorological data for each catchment and include mean daily precipitation (mm d−1), the seasonality of precipitation, the fraction of precipitation falling as snow, the frequency of high- and low-precipitation days (d yr−1), the average duration of high-precipitation events and dry periods (days of year) as well as the season during which most high- and low-precipitation days occur. The code to estimate the signatures in CAMELS-DE is based on the codes used to derive the signatures for CAMELS-US (https://github.com/naddor/camels, last access: 19 July 2024), CAMELS-UK, and CAMELS-CH to assure compatibility.
5.3 Land cover
Land cover in CAMELS-DE is derived from the Corine Land Cover dataset (CLC, 2018), which provides consistent and thematically detailed information on land cover across Europe. The dataset was produced within the frame of the Copernicus Land Monitoring Service, referring to land cover/land use status of the year 2018, and is based on the classification of satellite images (other major releases have been published in the years 1990, 2000, 2006, and 2012). The CLC dataset from 2018 has a spatial resolution of 100 m for raster data. This ensures detailed and consistent land cover information across Europe. CAMELS-DE includes land cover percentages per catchment of the first hierarchical land cover level: artificial surfaces, agricultural areas, forests and semi-natural areas, wetlands, and water bodies. The decision to not mix the hierarchical land cover levels ensures that uncertainties in classification due to varying levels of detail are minimised. Catchment shapes and codes to derive land cover classes of lower order or from different releases of CLC in a consistent manner with CAMELS-DE are delivered with the dataset (Dolich, 2024).
5.4 Soil
Soil attributes for CAMELS-DE are derived from the SoilGrids250m dataset (Poggio et al., 2021), which maps the spatial distribution of soil properties globally at six standard depths. The SoilGrids dataset is generated by training a machine learning model on approximately 240 000 locations worldwide, using over 400 global environmental covariates that describe vegetation, terrain morphology, climate, geology, and hydrology. For CAMELS-DE, we derived the mean values of the soil bulk density; soil organic carbon; volumetric percentage of coarse fragments; and proportions of clay, silt, and sand for each catchment. The resulting variables are aggregated from the six SoilGrids depths to the depths 0–30, 30–100, and 100–200 cm by calculating a weighted mean. The accuracy of soil property models, as described by Poggio et al. (2021), is limited by the availability and quality of input data as well as the assumptions in the modelling process. For instance, discrepancies in how soil data are collected, analysed, and reported by different entities challenge efforts toward data standardisation and harmonisation. However, the relatively high number of observations in Germany reduces this uncertainty to a certain extent. Furthermore, the defined catchment boundaries allow for an assessment of the reported uncertainties within each catchment. If needed, the catchment boundaries delivered with CAMELS-DE can be used to calculate the reported uncertainties of SoilGrids within each catchment.
5.5 Hydrogeology
The hydrogeological attributes for CAMELS-DE are derived from the hydrogeological overview map of Germany on the scale of 1:250 000; HÜK250 (HGM250, 2019), which describes the hydrogeological characteristics of the upper, large-scale contiguous aquifers in Germany. For CAMELS-DE, the areal percentage of the various HÜK250 classes (see Table 2) was calculated for each catchment, whereby the variables of the classes' permeability, aquifer media type, cavity type, consolidation, rock type, and geochemical rock type sum to 100 %. Uncertainties in these data may arise from the generalisation required to scale point measurements to a gridded product, which can oversimplify complex hydrogeological features, potentially leading to inaccuracies in the representation of local variations and the spatial distribution of aquifer properties.
5.6 Human influence
CAMELS-DE includes information on human influences within catchments, primarily focusing on existing dams and reservoirs in Germany. This information is sourced from the inventory of dams in Germany (Speckhann et al., 2021), which offers detailed data including dam names, locations, associated rivers, years of construction and operation start, crest lengths, dam heights, lake areas, lake volumes, purposes (such as flood control or water supply), dam structure types, and specific building characteristics for 530 dams across Germany. For catchments containing multiple dams, these data are aggregated to provide a comprehensive overview. Specifically, CAMELS-DE includes key information about the dams within each catchment, such as the number of dams, the names of the dams, the rivers where these dams are located, the operational years of the oldest and newest dams, the total area and volume of all dam lakes at full capacity, and the overall purposes of these dams. It is important to note that the “Inventory of Dams in Germany” does not claim to be exhaustive. The absence of recorded dams in this inventory does not necessarily indicate a lack of human influence within a catchment. Nearly all catchments in Germany experience substantial anthropogenic influences, and it is likely that some dams, weirs, or reservoirs (particularly smaller ones) are not documented in the dataset. Another relevant indicator of human influence included in CAMELS-DE is hence the proportion of artificial and agricultural surfaces derived from land cover attributes (see Sect. 5.3).
CAMELS-DE, in addition to hydro-meteorological observations and catchment attributes, includes results from data-driven and conceptual lumped rainfall–runoff simulations for each catchment. More specifically, these results are derived from a regionally trained LSTM network (trained on all catchments at the same time) and a locally trained lumped HBV model (trained at each individual catchment; Bergström and Forsman, 1973; Seibert, 2005; Feng et al., 2022). These models serve three main purposes: (a) they are used to identify catchments where the relationship between meteorological forcing and streamflow is difficult to capture (low model performance), indicating possible strong human influences, such as dams or reservoirs, or potential issues with the catchment delineation or the streamflow or meteorological time series; (b) they can serve as a benchmark for future modelling studies based on CAMELS-DE in a sense that the reported performance values and time series can be used as a baseline model; and (c) in the case of a good model performance, they can be used to fill missing values of the observed discharge time series. Both models were trained over the period from 1 October 1970 to 31 December 1999, validated from 1 October 1965 to 30 September 1970, and tested from 1 January 2000 to 31 December 2020. CAMELS-DE includes the simulated discharges for both models for the entire 70 years (Table 1), and a flag was added to indicate if the corresponding time step was used in training, validation, or testing. In the following, we explain the model setups and analyse the simulation results in detail. The code of the LSTM model and the HBV model were carefully tested and benchmarked (Acuña Espinoza et al., 2024). The codes have been designed to allow for easy access, and a permalink to the code version used for CAMELS-DE can be found here (https://github.com/KIT-HYD/Hy2DL/tree/v1.1, last access: 24 July 2024).
6.1 Setup of the LSTM model
The LSTM uses mean precipitation, standard deviation of precipitation, mean radiation, mean minimum temperature, and mean maximum temperature as dynamic (time varying) input features and specific discharge as a target variable. Static features and hyperparameters were set according to the study by Acuña Espinoza et al. (2024) with modifications made to (1) an increased hidden size from 64 to 128 and (2) a reduced number of epochs from 30 to 20. The remaining hyperparameters were set as follows: number of hidden layers = 1; learning rate = 0.001; dropout rate = 0.4; batch size = 256; sequence length = 365 d; iterative optimisation algorithm = Adam. We use the basin-averaged Nash–Sutcliffe efficiency (NSE∗) loss function proposed by Kratzert et al. (2019) to avoid an imbalance during training due to the higher influence of catchments with a higher runoff generation. In addition to the model results (see Table 2), we provide the model training epochs of the regional LSTM as part of the CAMELS-DE dataset.
6.2 Setup of the HBV model
The lumped HBV model used in CAMELS-DE is a variant of the well-known HBV (Hydrologiska Byråns Vattenbalansavdelning; Bergström and Forsman, 1973) model. A detailed description of the model architecture and setup can be found in the studies by Seibert (2005) and Feng et al. (2022). HBV uses mean precipitation and potential evapotranspiration (Epot; mm d−1) as inputs. The Epot is calculated using the temperature-based Hargreaves formula, detailed by Adam et al. (2006) and based on earlier work by Droogers and Allen (2002), as explained and cited in Clerc-Schwarzenbach et al. (2024). This variant of the Hargreaves formula resulted in the lowest mass balance error in most catchments with respect to other methods (e.g. Penman, Priestly–Taylor) to estimate evapotranspiration and was additionally chosen due to its low data requirements, enabling the utilisation of HYRAS precipitation and temperature data to generate the Epot time series with a limited number of assumptions. The Epot time series are included in CAMELS-DE (Table 2) for the entire time period of 70 years. In terms of model calibration, the HBV was trained individually for each basin using the NSE as a loss function, employing the DiffeRential Evolution Adaptive Metropolis (DREAM; Vrugt, 2016) algorithm as implemented in the SPOTPY (a statistical parameter optimization tool for Python; Houska et al., 2015) library. In contrast to the LSTM, the HBV model is mass conserving and hence more sensitive to errors in the catchment delineation that can lead to mass balance errors (see Sect. 3). The difference between the HBV and the LSTM performances can be seen as an indicator for either a strong human influence or an imprecise catchment delineation as the LSTM can create mass. In addition to the model results (see Table 2), we provide the HBV model parameters for each catchment as part of the CAMELS-DE dataset.
6.3 Results LSTM and HBV models
In this section, we focus our analysis on the LSTM and HBV models in catchments where at least 20 % of the daily data are available during the 30-year training period and 10 % during the testing period, covering a total of 1411 catchments. The median performance of the LSTM, as quantified by the NSE during the testing period, is 0.84 across 1411 catchments. Of these, 94 catchments have a NSE lower than 0.5 (6.66 % of all catchments), out of which 28 have a negative NSE (1.98 % of all catchments). For the 94 catchments with NSE below 0.5, most streamflow time series exhibit a low Pearson correlation with daily precipitation (<0.1), and these catchments are often considerably affected by the construction and/or operation of dams or flood control structures (human-influence attributes). Therefore, model performance of the LSTM network can be used to identify catchments that are subject to considerable uncertainties, due to either measurement inaccuracies or significant human influences.
Figure 5a illustrates the performance of the LSTM model across various federal states, with relatively consistent results across the board except for the federal states of Brandenburg (DE4) and Saxony-Anhalt (DEE). In Brandenburg, lowland catchments characterised by sandy soils; considerable groundwater impacts; abundance of natural lakes; and human-constructed weirs, canals, and cross-connections between streams most likely yield a distinctly lower model performance compared to the rest of the German federal states. Besides the federal states of Brandenburg and Saxony-Anhalt, the analysis of the LSTM simulations reveals no clear correlation between the model performance and the topographic attributes (e.g. area), climatic attributes (e.g. long-term mean precipitation), or hydrological attributes (e.g. long-term mean flow).
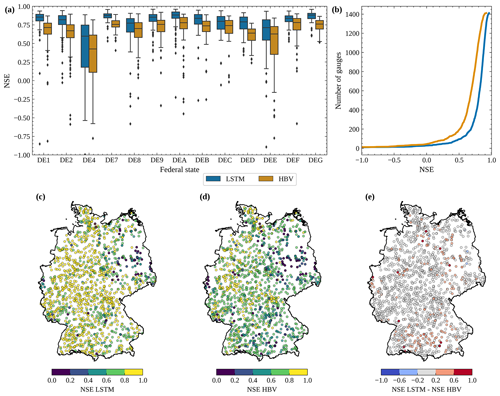
Figure 5Panel (a) shows boxplots visualising the distribution of the NSE of the LSTM network (blue) and the HBV model (orange) for each federal state in Germany for the testing period. Panel (b) shows a cumulative plot of the NSE for the general comparison of the LSTM model and the HBV model. Panel (c) shows the NSE values of the LSTM for 1411 gauging stations in Germany, while panel (d) shows the same for the NSE values of the HBV model. Panel (e) shows the difference between the NSE values of the LSTM and the HBV model for all gauging stations in Germany. Borders of Germany: © GeoBasis-DE/BKG (VG250, 2023).
The performance of HBV is with a median NSE of 0.72 lower than that of the LSTM (Fig. 5b). In 192 catchments (13.61 %), the HBV shows a performance below a NSE of 0.5, and in 44 catchments (3.12 %) a performance below a NSE of 0 is shown. The spatial patterns of performance measured by the NSE are consistent between the LSTM and HBV. In other words, catchments where the LSTM performs well are typically also accurately represented by HBV and vice versa, as illustrated in Fig. 5e. Catchments in which HBV significantly underperforms compared to the LSTM are almost invariably strongly influenced by human-made structures such as dams or weirs, or they are located in areas with uncertain catchment delineation. We propose that the HBV model, which conserves mass and uses time-invariant parameters, struggles to adapt to dynamic changes in catchment function caused by human activities that result in inaccuracies in water flow and storage due to structures like dams or weirs or due to irrigation or pumping. This is a hypothesis that requires further testing in the few catchments where this is the case.
The processing of CAMELS-DE is structured in a modular manner to enhance the clarity and reproducibility of the processing pipeline. The CAMELS-DE processing pipeline was published separately with more details and permalinks to the released repository versions that represent the code state that was used to process and compile CAMELS-DE (Dolich, 2024). For each component of CAMELS-DE, a distinct GitHub repository was established. Within each repository, a dedicated Docker container was developed to process specific input datasets (e.g. HYRAS, GLO-30 DEM). Containerisation is particularly well suited for this project as it ensures that each component of the data-processing pipeline runs consistently across different computing environments. This containerisation simplifies dependency management, enhances reproducibility, and facilitates the deployment and version control of each processing module. Figure 6 illustrates the architecture of the processing pipeline, where each blue block represents an individual GitHub repository equipped with a Docker container that processes the yellow input data to produce the green output data. All repositories are uniformly structured, and the accompanying documentation provides detailed descriptions of each repository, guidelines for building and running the Docker containers (including the necessary folder mounts), and instructions for accessing the required input data. In the initial phase of the CAMELS-DE data-processing pipeline, raw discharge and water level data, along with station metadata provided by the federal states, are processed and harmonised. Subsequently, MERIT Hydro catchment boundaries are delineated for each station, a pivotal step since all further datasets depend extensively on these catchment boundaries. Meteorological time series data for these catchments are then processed to compute statistics such as areal mean and median. Following this, attributes such as soil properties, hydrogeology, land cover, topography, and human influences are derived for each catchment (see Table 2). In the final stage, all derived data are integrated and formatted according to the established structure of the CAMELS-DE dataset, mirroring the organisational schema of CAMELS-GB or CAMELS-CH.
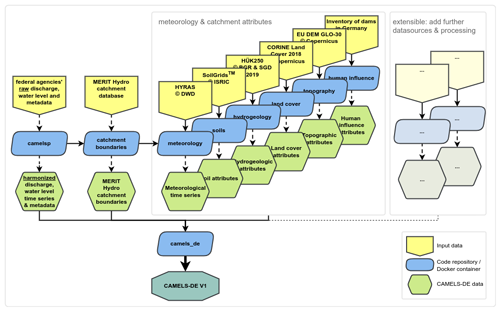
Figure 6Diagram of the CAMELS-DE data-processing pipeline. Starting with raw discharge and metadata harmonisation, it proceeds to derive MERIT Hydro catchment boundaries. Subsequent processing includes meteorological data extraction and aggregation followed by the extraction of various catchment attributes. In the final step, all extracted data sources are integrated in the structured CAMELS-DE dataset, consistent with CAMELS-GB or CAMELS-CH (Dolich, 2024).
The modular design of the CAMELS-DE processing pipeline enhances its traceability, comprehensibility, and reproducibility, differing significantly from a monolithic code approach that compiles the entire dataset into a single repository. This structure not only facilitates the extension of the pipeline to incorporate additional data sources (especially further catchment attributes) without the need to rerun or rewrite the entire system but also allows for the adaptation of processing or aggregation methods and for the seamless release of updated versions of the CAMELS-DE dataset. The publicly available Docker containers and the code within them serve not only as a comprehensive guide to understanding the data-processing methods used in CAMELS-DE but also as a foundation for further data processing using the catchment geometries included in the dataset. We encourage researchers to enrich CAMELS-DE with additional data sources and explore ways to enhance the baseline model results. Such contributions are invaluable for continuous improvements and expansions of the CAMELS-DE dataset, reflecting our commitment to advancing hydrological research and applications through reproducible science.
This article describes the state of version 1.0 of CAMELS-DE, which is freely available at https://doi.org/10.5281/zenodo.13837553 (Dolich et al., 2024), accompanied by a comprehensive data description. The CAMELS-DE processing pipeline with all codes can be found at https://doi.org/10.5281/zenodo.13842287 (Dolich, 2024).
CAMELS-DE is a significant step forward in hydrological research for Germany and beyond, offering a comprehensive dataset that spans 1582 catchments with hydro-meteorological daily time series from 1951 to 2020. CAMELS-DE includes detailed catchment delineations and properties, such as reservoir data, land use, soils, and hydrogeology, which are all vital to analyse and describe the local and regional hydrology of Germany. Furthermore, CAMELS-DE includes simulations from a regionally trained LSTM and locally trained HBV model that can be used either to fill gaps in discharge data in case of good model performance or to act as baseline models for the development and testing of new hydrological models. Due to the length of the provided time series of up to 70 years, CAMELS-DE opens up new opportunities for investigating long-term hydrological trends or conducting large-sample studies across diverse catchments, including a large number of catchments smaller than 100 km2. The dataset's modular design, achieved through the containerisation of each processing component, ensures that the data processing is traceable, comprehensible, and reproducible. This approach makes it easier to extend the dataset by incorporating new data sources, adapting processing methods, and releasing updated versions without the need to rerun the entire pipeline. While CAMELS-DE serves as a useful benchmark for large-sample hydrology, we invite the scientific community to enrich it with additional data sources and improved methods. In conclusion, CAMELS-DE aims to support a broad range of hydrological research and applications, to foster better understanding and management of water resources in Germany and beyond, and to contribute to future global hydrological studies.
RL and MS initiated the CAMELS-DE project. AD prepared and processed data, created most figures, and wrote (together with RL) most of the article. All other authors suggested improvements and made additions to the article, as well as provided data and expertise for specific topics. The order of the co-authors is sorted alphabetically.
At least one of the (co-)authors is a member of the editorial board of Earth System Science Data. The peer-review process was guided by an independent editor, and the authors also have no other competing interests to declare.
Publisher’s note: Copernicus Publications remains neutral with regard to jurisdictional claims made in the text, published maps, institutional affiliations, or any other geographical representation in this paper. While Copernicus Publications makes every effort to include appropriate place names, the final responsibility lies with the authors.
We thank the various German institutions for providing observation-based data and sharing their expertise. We are grateful to the Volkswagen Foundation for funding the “CAMELS-DE” project within the framework of the project “Invigorating Hydrological Science and Teaching: Merging Key Legacies with New Concepts and Paradigms” (ViTamins). We also extend our thanks to NFDI4Earth, particularly Jörg Seegert, for their support and suggestions.
The article processing charges for this open-access publication were covered by the Karlsruhe Institute of Technology (KIT).
This paper was edited by Conrad Jackisch and reviewed by Juliane Mai and one anonymous referee.
Acuña Espinoza, E., Loritz, R., Álvarez Chaves, M., Bäuerle, N., and Ehret, U.: To bucket or not to bucket? Analyzing the performance and interpretability of hybrid hydrological models with dynamic parameterization, Hydrol. Earth Syst. Sci., 28, 2705–2719, https://doi.org/10.5194/hess-28-2705-2024, 2024.
Adam, J. C., Clark, E. A., Lettenmaier, D. P., and Wood, E. F.: Correction of global precipitation products for orographic effects, J. Climate, 19, 15–38, https://doi.org/10.1175/JCLI3604.1, 2006.
Addor, N., Newman, A. J., Mizukami, N., and Clark, M. P.: The CAMELS data set: catchment attributes and meteorology for large-sample studies, Hydrol. Earth Syst. Sci., 21, 5293–5313, https://doi.org/10.5194/hess-21-5293-2017, 2017.
Bergström, S. and Forsman, A.: Development of a Conceptual Deterministic Rainfall-runoff Model, Hydrol. Res., 4, 147–170, https://doi.org/10.2166/nh.1973.0012, 1973.
Brunner, M. I., Slater, L., Tallaksen, L. M., and Clark, M.: Challenges in modeling and predicting floods and droughts: A review, WIREs Water, 8, e1520, https://doi.org/10.1002/wat2.1520, 2021.
CLC: Corine Land Cover, CLC [data set], https://doi.org/10.2909/960998c1-1870-4e82-8051-6485205ebbac, 2018.
Clerc-Schwarzenbach, F., Selleri, G., Neri, M., Toth, E., van Meerveld, I., and Seibert, J.: Large-sample hydrology – a few camels or a whole caravan?, Hydrol. Earth Syst. Sci., 28, 4219–4237, https://doi.org/10.5194/hess-28-4219-2024, 2024.
Coxon, G., Addor, N., Bloomfield, J. P., Freer, J., Fry, M., Hannaford, J., Howden, N. J. K., Lane, R., Lewis, M., Robinson, E. L., Wagener, T., and Woods, R.: CAMELS-GB: hydrometeorological time series and landscape attributes for 671 catchments in Great Britain, Earth Syst. Sci. Data, 12, 2459–2483, https://doi.org/10.5194/essd-12-2459-2020, 2020.
Dolich, A.: CAMELS-DE Processing Pipeline (1.0.0), Zenodo [code], https://doi.org/10.5281/zenodo.13842287, 2024.
Dolich, A., Espinoza, E. A., Ebeling, P., Guse, B., Götte, J., Hassler, S., Hauffe, C., Kiesel, J., Heidbüchel, I., Mälicke, M., Müller-Thomy, H., Stölzle, M., Tarasova, L., and Loritz, R.: CAMELS-DE: hydrometeorological time series and attributes for 1582 catchments in Germany (1.0.0), Zenodo [data set], https://doi.org/10.5281/zenodo.13837553, 2024.
Droogers, P. and Allen, R. G.: Estimating reference evapotranspiration under inaccurate data conditions, Irrig. Drain. Syst., 16, 33–45, https://doi.org/10.1023/A:1015508322413, 2002.
DWD-HYRAS: HYRAS – Hydrometeorologische Rasterdaten, https://www.dwd.de/DE/leistungen/hyras/hyras.html, last access: 25 March 2024.
Ebeling, P., Kumar, R., Lutz, S. R., Nguyen, T., Sarrazin, F., Weber, M., Büttner, O., Attinger, S., and Musolff, A.: QUADICA: water QUAlity, DIscharge and Catchment Attributes for large-sample studies in Germany, Earth Syst. Sci. Data, 14, 3715–3741, https://doi.org/10.5194/essd-14-3715-2022, 2022.
EU-DEM: Copernicus GLO-30 DEM, Copernicus [data set], https://doi.org/10.5270/esa-c5d3d65, 2022.
Färber, C., Plessow, H., Kratzert, F., Addor, N., Shalev, G., and Looser, U.: GRDC-Caravan: extending the original dataset with data from the Global Runoff Data Centre (0.2), Zenodo [data set], https://doi.org/10.5281/zenodo.10074416, 2023.
Feng, D., Liu, J., Lawson, K., and Shen, C.: Differentiable, learnable, regionalized process-based models with multiphysical outputs can approach state-of-the-art hydrologic prediction accuracy, Water Resour. Res., 58, e2022WR032404, https://doi.org/10.1029/2022WR032404, 2022.
Heberger, M.: delineator.py: Fast, accurate watershed delineation using hybrid vector- and raster-based methods and data from MERIT-Hydro (v1.3), Zenodo [code], https://doi.org/10.5281/zenodo.10143149, 2023.
HGM250: Hydrogeological Map of Germany (), Geodatenkatalog [data set], https://gdk.gdi-de.org/geonetwork/srv/api/records/61ac4628-6b62-48c6-89b8-46270819f0d6 (last access: 24 July 2024), 2019.
Hochreiter, S.: The vanishing gradient problem during learning recurrent neural nets and problem solutions. International Journal of Uncertainty, Fuzziness and Knowledge-Based Systems, 06, 107–116, https://doi.org/10.1142/s0218488598000094, 1998.
Hochreiter, S. and Schmidhuber, J.: Long short-term memory, Neural Comput., 9, 1735–1780, https://doi.org/10.1162/neco.1997.9.8.1735, 1997.
Houska, T., Kraft, P., Chamorro-Chavez, A., and Breuer, L.: SPOTting Model Parameters Using a Ready-Made Python Package, PLOS ONE, 10, e0145180, https://doi.org/10.1371/journal.pone.0145180, 2015.
Höge, M., Kauzlaric, M., Siber, R., Schönenberger, U., Horton, P., Schwanbeck, J., Floriancic, M. G., Viviroli, D., Wilhelm, S., Sikorska-Senoner, A. E., Addor, N., Brunner, M., Pool, S., Zappa, M., and Fenicia, F.: CAMELS-CH: hydro-meteorological time series and landscape attributes for 331 catchments in hydrologic Switzerland, Earth Syst. Sci. Data, 15, 5755–5784, https://doi.org/10.5194/essd-15-5755-2023, 2023.
HYRAS-DE-HURS: Raster data set of daily mean relative humidity in % for Germany – HYRAS-DE-HURS, Version v5.0, DWD [data set], https://opendata.dwd.de/climate_environment/CDC/grids_germany/multi_annual/hyras_de/humidity/DESCRIPTION_GRD_DEU_P30Y_RH_HYRAS_DE_en.pdf (last access: 24 July 2024), 2022.
HYRAS-DE-PRE: Raster data set of daily sums of precipitation in mm for Germany – HYRAS-DE-PRE, Version v5.0, DWD [data set], https://opendata.dwd.de/climate_environment/CDC/grids_germany/daily/hyras_de/precipitation/DESCRIPTION_GRD_DEU_P1D_RR_HYRAS-DE_en.pdf (last access: 24 July 2024), 2022.
HYRAS-DE-RSDS: Raster data set of daily mean global radiation in W/m2 for Germany – HYRAS-DE-RSDS, DWD [data set], https://opendata.dwd.de/climate_environment/CDC/grids_germany/daily/hyras_de/radiation_global/DESCRIPTION_GRD_DEU_P1D_RAD_G_HYRAS_DE_en.pdf (last access: 24 July 2024), Version v3.0, 2023.
HYRAS-DE-TAS: Raster data set of daily mean temperature in °C for Germany – HYRAS-DE-TAS, Version v5.0, DWD [data set], https://opendata.dwd.de/climate_environment/CDC/grids_germany/daily/hyras_de/air_temperature_mean/DESCRIPTION_GRD_DEU_P1D_T2M_HYRAS_DE_en.pdf (last access: 24 July 2024), 2022.
HYRAS-DE-TASMAX: Raster data set of daily maximum temperature in °C for Germany – HYRAS-DE-TASMAX, Version v5.0, DWD [data set], https://opendata.dwd.de/climate_environment/CDC/grids_germany/monthly/hyras_de/air_temperature_max/DESCRIPTION_GRD_DEU_P1M_T2M_X_HYRAS_DE_en.pdf (last access: 24 July 2024), 2022.
HYRAS-DE-TASMIN: Raster data set of daily minimum temperature in °C for Germany – HYRAS-DE-TASMIN, Version v5.0, DWD [data set], https://opendata.dwd.de/climate_environment/CDC/grids_germany/daily/hyras_de/air_temperature_min/DESCRIPTION_GRD_DEU_P1D_T2M_N_HYRAS_DE_en.pdf (last access: 24 July 2024), 2022.
Klingler, C., Schulz, K., and Herrnegger, M.: LamaH-CE: LArge-SaMple DAta for Hydrology and Environmental Sciences for Central Europe, Earth Syst. Sci. Data, 13, 4529–4565, https://doi.org/10.5194/essd-13-4529-2021, 2021.
Kratzert, F., Klotz, D., Shalev, G., Klambauer, G., Hochreiter, S., and Nearing, G.: Towards learning universal, regional, and local hydrological behaviors via machine learning applied to large-sample datasets, Hydrol. Earth Syst. Sci., 23, 5089–5110, https://doi.org/10.5194/hess-23-5089-2019, 2019.
Lehner, B., Roth, A., Huber, M., Anand, M., Grill, G., Osterkamp, N., Tubbesing, R., Warmedinger, L., and Thieme, M.: HydroSHEDS v2.0 – Refined global river network and catchment delineations from TanDEM-X elevation data, EGU General Assembly 2021, online, 19–30 Apr 2021, EGU21-9277, https://doi.org/10.5194/egusphere-egu21-9277, 2021.
Muñoz-Sabater, J., Dutra, E., Agustí-Panareda, A., Albergel, C., Arduini, G., Balsamo, G., Boussetta, S., Choulga, M., Harrigan, S., Hersbach, H., Martens, B., Miralles, D. G., Piles, M., Rodríguez-Fernández, N. J., Zsoter, E., Buontempo, C., and Thépaut, J.-N.: ERA5-Land: a state-of-the-art global reanalysis dataset for land applications, Earth Syst. Sci. Data, 13, 4349–4383, https://doi.org/10.5194/essd-13-4349-2021, 2021.
Poggio, L., de Sousa, L. M., Batjes, N. H., Heuvelink, G. B. M., Kempen, B., Ribeiro, E., and Rossiter, D.: SoilGrids 2.0: producing soil information for the globe with quantified spatial uncertainty, SOIL, 7, 217–240, https://doi.org/10.5194/soil-7-217-2021, 2021.
Rauthe, M., Steiner, H., Riediger, U., Mazurkiewicz, A., and Gratzki, A.: A Central European precipitation climatology Part I: Generation and validation of a high-resolution gridded daily data set (HYRAS), Meteorol. Z., 22, 235–256, https://doi.org/10.1127/0941-2948/2013/0436, 2013.
Razafimaharo, C., Krähenmann, S., Höpp, S., Rauthe, M., and Deutschländer, T.: New high-resolution gridded dataset of daily mean, minimum, and maximum temperature and relative humidity for Central Europe (HYRAS), Theor. Appl. Climatol., 142, 1531–1553, https://doi.org/10.1007/s00704-020-03388-w, 2020.
Seibert, J.: HBV Light Version 2, User's Manual, Department of Physical Geography and Quaternary Geology, Stockholm University, Stockholm, https://www.geo.uzh.ch/dam/jcr:c8afa73c-ac90-478e-a8c7-929eed7b1b62/HBV_manual_2005.pdf (last access: 19 September 2024), 2005.
Speckhann, G. A., Kreibich, H., and Merz, B.: Inventory of dams in Germany, Earth Syst. Sci. Data, 13, 731–740, https://doi.org/10.5194/essd-13-731-2021, 2021.
VG250: Verwaltungsgebiete 1:250000 – Stand 01.01., https://gdk.gdi-de.org/geonetwork/srv/api/records/93a98c5c-cf03-4a95-bf0a-54001fbf3949 (last access: 24 July 2024), 2023.
Vrugt, J. A.: Markov chain Monte Carlo simulation using the DREAM software package: Theory, concepts, and MATLAB implementation, Environ. Modell. Softw., 75, 273–316, https://doi.org/10.1016/j.envsoft.2015.08.013, 2016.
Yamazaki, D., Ikeshima, D., Tawatari, R., Yamaguchi, T., O'Loughlin, F., Neal, J. C., Sampson, C. C., Kanae, S., and Bates, P. D.: A high-accuracy map of global terrain elevations, Geophys. Res. Lett., 44, 5844–5853, https://doi.org/10.1002/2017gl072874, 2017.
Yamazaki, D., Ikeshima, D., Sosa, J., Bates, P. D., Allen, G. H., and Pavelsky, T. M.: MERIT Hydro: A High-Resolution Global Hydrography Map Based on Latest Topography Dataset, Water Resour. Res., 55, 5053–5073, https://doi.org/10.1029/2019wr024873, 2019.
- Abstract
- Introduction
- Data sources and providers
- Catchments
- Time series
- Catchment attributes
- Benchmark LSTM model and HBV model
- Code availability, reproducibility, and extensions
- Data availability
- Conclusions
- Author contributions
- Competing interests
- Disclaimer
- Acknowledgements
- Financial support
- Review statement
- References
- Abstract
- Introduction
- Data sources and providers
- Catchments
- Time series
- Catchment attributes
- Benchmark LSTM model and HBV model
- Code availability, reproducibility, and extensions
- Data availability
- Conclusions
- Author contributions
- Competing interests
- Disclaimer
- Acknowledgements
- Financial support
- Review statement
- References