the Creative Commons Attribution 4.0 License.
the Creative Commons Attribution 4.0 License.
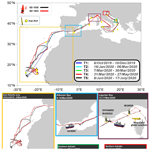
CO2 and hydrography acquired by autonomous surface vehicles from the Atlantic Ocean to the Mediterranean Sea: data correction and validation
Michele Giani
Elena Mauri
Laurent Coppola
Melf Paulsen
Marine Fourrier
Sara Pensieri
Vanessa Cardin
Carlotta Dentico
Roberto Bozzano
Carolina Cantoni
Anna Lucchetta
Alfredo Izquierdo
Miguel Bruno
Ingunn Skjelvan
The ATL2MED demonstration experiment involved two autonomous surface vehicles from Saildrone Inc. (SD) which travelled a route from the eastern tropical North Atlantic to the Adriatic Sea between October 2019 and July 2020 (see Table A6). This 9-month experiment in a transition zone between the temperate and tropical belts represents a major challenge for the SD's operations. The sensors on board were exposed to varying degrees of degradation and biofouling depending on the geographical area and season, which led to a deterioration in the measurements. As a result, some maintenance measures were required during the mission.
We address the difficulty of correcting the data during a period of COVID-19 restrictions, which significantly reduced the number of discrete samples planned for the SD salinity and dissolved oxygen validation. This article details alternative correction methods for salinity and dissolved oxygen. Due to the lack of in situ data, model products have been used to correct the salinity data acquired by the SD instruments, and then the resulting corrected salinity was validated with data from fixed ocean stations, gliders, and Argo floats. In addition, dissolved oxygen data acquired from the SD instruments after correction using air oxygen measurements were tested and found to be coherent with the variation in oxygen concentrations expected from changes in temperature and phytoplankton abundance (from chlorophyll a). The correction methods are relevant and useful in situations where validation capabilities are lacking, which was the case during the ATL2MED demonstration experiment. For future experiments, a more frequent sample collection would improve the data qualification and validation.
- Article
(7091 KB) - Full-text XML
- BibTeX
- EndNote
Automated observations contribute to the steadily increasing knowledge of the ocean and its role in the global climate system. For a long time, fixed ocean stations and research vessels formed the backbone of the monitoring network. In recent years, efforts have been made to improve the frequency of acquisition through technological developments (e.g. the EU infrastructures ICOS, https://www.icos-cp.eu/, last access: 16 September 2024; EMSO, https://emso.eu, last access: 16 September 2024; and Euro-Argo, https://www.euro-argo.eu, last access: 16 September 2024). Among other improvements, fixed ocean stations and ships of opportunity (Lüger et al., 2004) were equipped with autonomous and accurate sensors for measurements of the partial pressure of CO2 (pCO2) in addition to sensors for complementary measurements (e.g. water temperature, salinity, dissolved oxygen, pH, nutrients, fluorescence) needed to understand the dynamics and the effects of CO2 fluxes on the carbon budget. Despite efforts having been made, it remains difficult to obtain a comprehensive overview of CO2 fluxes at regional and larger scales because of very sparse coverage by fixed observatories, low measurement frequency, and limited systematic reference measurements.
One way to address such observational gaps (Tanhua et al., 2019) is to develop and deploy autonomous surface vehicles (ASVs) equipped with a suite of sensors and capable of measuring CO2 fluxes at the air–sea interface with gas reference, high sampling frequency, and real-time data transmission. ASV monitoring systems have the potential to collect data from large ocean areas and at a frequency that resolves processes at multiple timescales. Nevertheless, there are challenges with those surface monitoring systems, and one of the most important is biofouling, which can interfere with measurements of, e.g. conductivity; dissolved oxygen; and, especially, chlorophyll a (chl a) and could ultimately render the sensors inoperable (e.g. Delauney et al., 2010). Regular maintenance counteracts biofouling or at least reduces the impact on measurements, but this is not always possible due to the positioning of the ASVs at long distances from the shore or from the maintenance vessel. Therefore, the value of ASV data depends heavily on quality control and quality assurance.
During the 9-month-long demonstration experiment ATL2MED, two wind-driven Saildrone (SD) ASVs (Gentemann et al., 2020) manufactured by Saildrone Inc. (Alameda, CA, USA) were used to improve data coverage and to link CO2 surface observations at fixed ocean stations on a larger scale, from the eastern tropical North Atlantic (ETNA) to the central Mediterranean Sea. The SD instruments are prone to errors primarily due to sensor drift, which can be caused by either biofouling or malfunctioning sensor parts. During the ATL2MED demonstration experiment, problems were found with the data collected by several SD sensors, and severe biofouling occurred, as expected in such a long-duration experiment.
Still, the use of the SD instruments provided the opportunity to expand and link fixed CO2 observations at the surface on a larger scale, particularly during the COVID-19 pandemic when access to ocean platforms and ship visits were restricted or even prohibited. Furthermore, the demonstration experiment allowed us to focus the SD measurements on different marine environments, the Atlantic Ocean and the Mediterranean Sea, which made it possible to assess the quality of measurements across a wide range of values. The experiment additionally evaluated the ability of such ASVs to provide data with sufficient quality to be relevant for the scientific community.
The objective of the present work is to evaluate and correct the data collected by the SD instruments in order to provide a homogenised and comparable data set useful for the study of processes such as air–sea gas exchange in the Atlantic Ocean and Mediterranean Sea. While this paper focuses on the methods, a follow-up paper will focus on biogeochemical processes occurring in the area.
2.1 Data collection and experiment
The ATL2MED demonstration experiment took place between 18 October 2019 and 17 July 2020 as a joint effort among a number of European academic institutions and the SD piloting team. A detailed description of the ATL2MED demonstration experiment can be found in Skjelvan et al. (2021). During the experiment, the SD instruments crossed the ETNA region; the Strait of Gibraltar; and the northern part of the western and central Mediterranean Sea, including the Ligurian Sea, the Strait of Sicily, the Strait of Otranto, and the Adriatic Sea (Fig. 1).
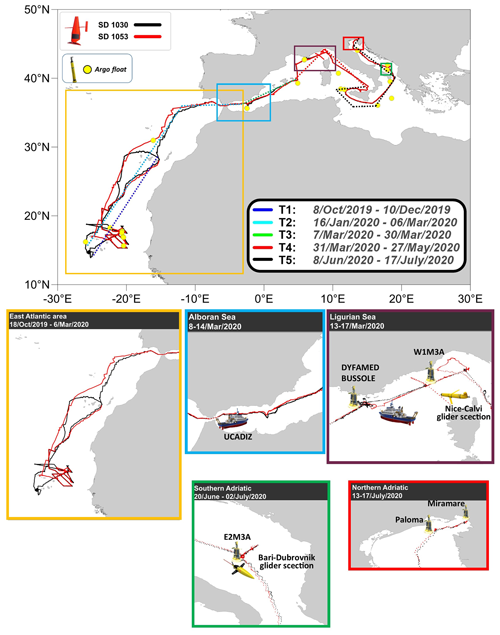
Figure 1Study area, with the upper map showing the route of the two SD instruments (SD 1030 as black lines and SD 1053 as red lines), the positions of the Argo floats (yellow dots), and the SD routes divided into transects (T). The lower maps zoom in on areas with in situ observations (buoys, ships, and glider sections).
The aim of the ATL2MED demonstration experiment was to (1) study eddies in the Canary Current upwelling system off western Africa jointly with a vessel-based research expedition (RV Meteor M160) and (2) to validate the CO2 measurements acquired at five fixed ocean stations (DYFAMED, W1M3A, E2M3A, PALOMA, and MIRAMARE). This monitoring experiment was achieved with sensors and instruments installed on the SD devices but also with equipment deployed at a number of facilities that were used to correct data from the SD instruments (see Sect. 3). Table 1 provides an overview of the various facilities and the times at which the SD visits were carried out. A detailed description of the instruments and sensors installed on the different platforms, as well as their characteristics, can be found in Tables A1–A3 of Appendix A.
Table 1Research vessels and fixed ocean stations from which temperature, salinity, and/or carbon measurements were compared with those of the SD instruments.
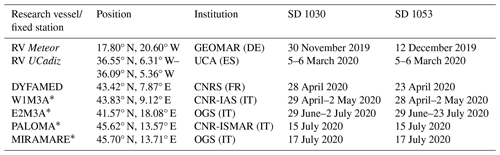
∗ These stations are part of the ICOS station network (Steinhoff et al., 2019).
Maintenance operations ensured the reliability and accuracy of the data collected by the SD instruments. Throughout the expedition, the data collected by the SD instruments were categorised into different transects, designated as T1, T2, T3, T4, and T5. These transects corresponded to specific sections of the expedition timeline in terms of maintenance events (see Table A1 in Appendix A), which facilitates data correction.
The SD instruments were equipped with a number of autonomous sensors (CTD: conductivity, temperature, and depth; dissolved oxygen; fluorescence; pH; pCO2; meteorological sensors). This study focuses primarily on sensors acquiring temperature, salinity, dissolved oxygen, and pCO2 data. This selection is based on the available options for correcting the SD data sets: some of the sensors (e.g. fluorescence) were so severely affected by biofouling that they could not be accounted for, while others only worked for a short period of time (e.g. the Durafet Honeywell pH sensor). One of the SD instruments (SD 1030) was equipped with an ASVCO2 system developed by PMEL (NOAA's Pacific Marine Environmental Laboratory). The ASVCO2 system is a compressed version of the more voluminous system described in detail in Sutton et al. (2014) and Sabine et al. (2020). Water from a depth of approximately 0.5 m is fed into a bubble equilibrator (Friederich et al., 1995), and the partially dried xCO2 is measured with an infrared detector (LI-COR 820 CO2 gas analyser). A two-point calibration was used, where the first is a reference gas from NOAA/ESRL, while the second is air purged for CO2. An air inlet was mounted approximately 1 m above sea level, and atmospheric xCO2 was measured between measurements of the sea surface. See Table A2 in Appendix A for the measurement frequency and initial accuracy of the SD sensors during the ATL2MED experiment.
2.2 Comparative data sets
2.2.1 Liguro–Provençal basin facilities
In the French exclusive economic zone (EEZ), the open-ocean station DYFAMED is located in the Ligurian Sea in the northwestern Mediterranean Sea. The CNRS (French National Centre for Scientific Research) is in charge of the station as part of the national MOOSE programme (Coppola et al., 2019). At the DYFAMED site, a CARIOCA pCO2 sensor ensures autonomous measurements, and a detailed description can be found in Merlivat et al. (2018). In addition, gliders are regularly operating in the Nice–Calvi section where the DYFAMED site is located (MOOSE programme; Coppola et al., 2019; Bosse et al., 2015; Testor et al., 2019). During the demonstration experiment, a deployment of the Slocum glider was used along the endurance line (MOOSE T00-43 mission) performed from 12 March to 20 June 2020. Table A2 includes information about which sensors the glider was equipped with. Discrete samples were collected from the DYFAMED site in February and March 2020 for comparison with the pCO2 sensor measurements (Table A4).
The open-ocean station W1M3A is located in the Italian EEZ of the Liguro–Provencal basin. Operated by CNR-IAS, the W1M3A consists of a large spar buoy and a subsurface mooring positioned in the immediate vicinity. A detailed description of the observatory can be found in Canepa et al. (2015), and some of this information is found in Table A2. Discrete samples were collected from W1M3A in October 2020 (Table A4).
2.2.2 Adriatic Sea facilities
The fixed station E2M3A is situated in the open sea of the southern Adriatic Sea and is operated by the Italian National Institute of Oceanography and Applied Geophysics – OGS. Information on this site can be found in Bozzano et al. (2013) and Ravaioli et al. (2016). In the southern Adriatic, OGS also regularly operates an ocean glider in the Bari–Dubrovnik section (Mauri et al., 2016; Pirro et al., 2022; Kokkini et al., 2019). During the ATL2MED demonstration experiment, the glider transect was extended to include the area of the E2M3A fixed station from 12 June to 2 July 2020. During the 20 d campaign, 250 dives between 20 to 950 m profiles separated by 3–5 km and 4–6 h were collected. Table A2 contains information about the specific sensors mounted on the glider.
The coastal stations PALOMA (operated by CNR-ISMAR) and MIRAMARE (operated by OGS) are situated in the Gulf of Trieste in the northern Adriatic. A description of the PALOMA station can be found in Ravaioli et al. (2016) and Cantoni et al. (2012), while the MIRAMARE site is described in Ravaioli et al. (2016). See Table A2 for information about which sensors are used at the sites. By means of comparing the pCO2 sensor measurements performed at the sites, discrete carbon samples were collected near PALOMA on 15 July 2020 and in the vicinity of MIRAMARE on 17 July 2020 (Table A4).
2.3 Shipboard data
Discrete samples for dissolved inorganic carbon (DIC) and TA were collected on board the RV Meteor (M160) during autumn 2019 and were analysed by GEOMAR. Discrete samples for DIC, total alkalinity (TA), pH, and dissolved oxygen are regularly collected next to the fixed ocean stations; however, this was not always possible during the ATL2MED demonstration experiment due to COVID-19 pandemic restrictions. Table A4 gives an overview of the discrete samples collected during the ATL2MED demonstration experiment, along with their sampling depth and analysis methods.
In addition, salinity was measured continuously on board the RV UCadiz at a depth of 2.3 m between 5 and 6 March 2020, when the SD crossed the Strait of Gibraltar. Table A2 contains information about the sensor used.
2.4 Argo float
Float data were retrieved from the Argo Coriolis Global Data Assembly Center in France (GDAC; ftp://ftp.ifremer.fr/argo, last access: 16 September 2024, Wong et al., 2020; GDAC, 2023). For each Argo float, the variable SALINITY ADJUSTED was extracted and then used for comparison with the SD salinity data. Every profile close in space and time (1 d and 30 km) was chosen, and then salinity was averaged in the upper 5 m of the water column.
2.5 Model output
The Copernicus Marine Service (CMEMS) model products, specifically the global ocean ° physics analysis and forecast (https://doi.org/10.48670/moi-00016, CMEMS, 2024a) and the Mediterranean Sea physics analysis and forecast (Escudier et al., 2020; Clementi et al., 2021), were used. Daily data were developed for the global ocean and the Mediterranean Sea.
2.6 Satellite product
To evaluate the ocean response, sea surface chl a (OCEANCOLOUR_MED_BGC_L3_NRT_009_141), sea surface temperature (Merchant et al., 2019; Buongiorno Nardelli er al., 2022), and the vertical structure of ocean temperature (MEDSEA_MULTIYEAR_PHY_006_004) were downloaded from the CMEMS data portal and analysed (Table A6 in Appendix A).
3.1 Salinity
Here, the salinity is measured using the PSS-78 scale. During the first transect, T1 (Fig. 2), the two salinity sensors on board the SD instruments showed high consistency (Fig. 2a and b). After the first maintenance in T2, the SD 1053 showed a reduction in salinity of about 1 compared to the salinity measured by the SD 1030. In T3, the difference in salinity decreased to, on average, 0.15. During this period, the SD instruments crossed the Alboran Sea characterised by high thermohaline variability due to the presence of Atlantic and Mediterranean waters (Poulain et al., 2021), and the high spatial and temporal variabilities in salinity distribution in the area (Capó et al., 2021) complicate the understanding of the observed differences (i.e. sensor error or natural variability). In T4 and T5, salinity shifts of 1 were observed until the end of the experiment.
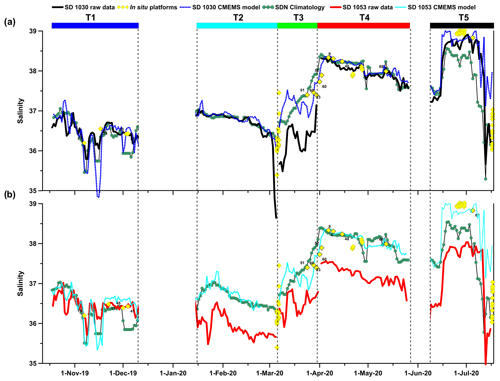
Figure 2Salinity time series of daily raw data, CMEMS model salinity products, and climatology and float data. (a) SD 1030 salinity raw data (black line), model data (dotted blue line), climatological data (green circle) from the nodes nearest to the SD trajectory, and in situ data (yellow diamonds). (b) SD 1053 salinity raw data (red line), CMEMS model 1053 trajectory (dotted cyan line), climatological data (green circle), and in situ platforms (yellow diamonds). Numbers close to diamonds indicate the distance (km) from the trajectory of the SD. In situ platforms include data from Argo buoys, fixed stations, gliders, and RV UCadiz.
Given the large variability found in the salinity data of the SD instruments, a comparison with in situ data along the trajectory of the experiment was necessary. We first identified the observing systems (fixed buoy, Argo float) that were temporally and spatially close to the positions of the SD instruments. Salinity data, with a temporal and spatial interval lower than 1 d and 30 km, respectively, were used for the comparison and/or correlation; however, these were extremely scarce.
To further evaluate the salinity data of the two SD instruments, a comparison was made with climatological data, considering the point in the climatology data set closest to the SD measurements (Fig. 2). The SD 1030 exhibited consistent salinity data in periods T1, T2, and T4 (ΔS<0.1), with deviations being observed in periods T3 and T5 (Fig. 2a). Conversely, the SD 1053 displayed consistent salinity data in period T1 only (ΔS<0.1), with higher deviations in periods T2, T3, T4, and T5. Subsequent evaluation of the data distribution characteristics revealed variances between the two SD instruments (Fig. 2b).
In T5, the climatology failed to represent salinity in the Ionian and Adriatic seas, characterised by a continuous increase in salinity since 2017 (Mauri et al., 2021; Mihanović et al., 2021; Menna et al., 2022; Neri et al., 2023; Pranić et al., 2023). This was due to the bipolar behaviour of the Ionian Sea, subject to an alternation between the highly saline waters of the Levantine basin and the less saline waters of Atlantic origin (Pinardi et al., 2019; Gačić et al., 2021; Menna et al., 2022; Civitarese et al., 2023).
To overcome the problem of a lack of data, we decided to compare the data acquired by the SD instruments with the reanalysis model products along the entire route (Fig. 2a and b). The model, while not deviating much from the in situ and climatological data (Fig. 2), can provide salinity products along the SD's trajectory, allowing for the correction of the salinity recorded by the SD. Moreover, comparative works between the physical model and experimental observations have shown a satisfactory correlation both in the open ocean (Escudier et al., 2021; Menna et al., 2023) and in the coastal environment (Martellucci et al., 2021). Despite all the limitations a model may have in such cases, the use of model products allows a minimum spatial and temporal distance in the comparison of the along-track SD measurements. The nodes nearest to the SD trajectory (in km) with respect to the model data grid were chosen. The salinity provided by the model along the two SD trajectories shows very similar values to that measured by the SD 1030 (Fig. 3). Salinity differences between the CMEMS model and the SD 1030 observations show a difference of less than 0.1 in T1, T2, T4, and T5. During the Alboran Sea crossing (T3), the observed salinity deviated strongly from the model (by about 0.6) over only 20 d. In contrast, the SD 1053 showed deviating values compared to the model and the SD 1030, which cannot be explained by space–time variability. With the exception of T1, the remaining transects (Fig. 3i and j) showed large deviations between the model and observed salinities (T2: 0.8, T3: 0.7, T4: 0.9, and T5: 1). This could be related to the long period between the SD 1053 maintenance (early January and early May 2020), but it is more likely that a sensor error occurred in mid-January which even maintenance could not correct.
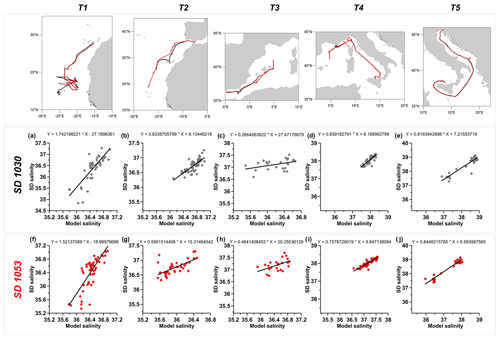
Figure 3Least-square regression between model and the SD raw salinity during each transect from T1 (left) to T5 (right) for the SD 1030 (a–e) and the SD 1053 (f–j). The solid line represents the linear regression fit.
The salinity correction was performed using a linear regression method in which the salinity data recorded by the autonomous vehicles averaged over the day were calibrated with the corresponding data from numerical models. A strict criterion, with a significance level of p<0.05 (Table 2), was applied to the correction process.
3.2 Dissolved oxygen
Due to the strong dependence of dissolved oxygen on temperature, we first analyse the temperature along the track of the SD instruments. During the demonstration experiment, sea temperature (Fig. 4a) showed a seasonal signal similar to those observed at these latitudes (Pastor et al., 2019). The high observed temperature variability also includes the wide geographical coverage of the SD instruments. The highest temperatures were measured in November 2019 and July 2020 in the tropical Atlantic and the southern Adriatic, respectively. The lowest temperatures were measured in the Gulf of Lion in April 2020. Along the SD tracks, the salinity (Fig. 2b) showed a gradual increase from the Atlantic Ocean to the eastern Mediterranean Sea. Given the correct temperature measurement, any dissolved oxygen drift can be assessed through comparison with dissolved oxygen saturation values. This procedure was also used to correct Argo float data with climatological observations (Takeshita et al., 2013). The dissolved oxygen saturation showed a gradual decrease from 100 % at the start of the demonstration experiment to 80 % at the end (Fig. 4c). This behaviour is also reflected in the dissolved oxygen concentration, which decreased by about 40 µmol kg−1 for the SD 1030 and 60 µmol kg−1 for the SD 1053 (Fig. 4b) over the course of 9 months, with standard deviations in terms of the uncorrected oxygen record of 16 and 72 µmol kg−1 for the SD 1030 and 1053, respectively.
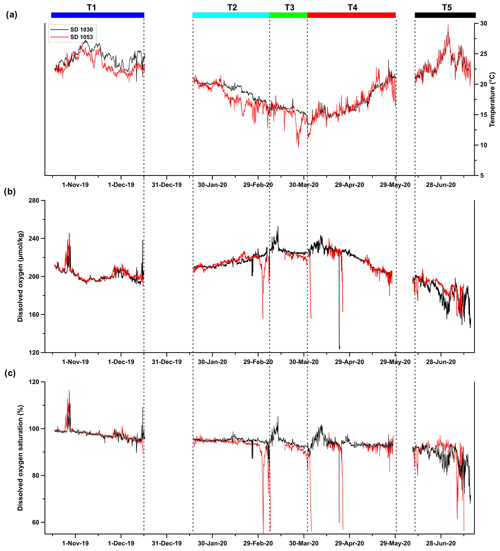
Figure 4(a) Temperature, (b) dissolved oxygen concentration, and (c) dissolved oxygen saturation for the SD raw data (SD 1030 – black line, SD 1053 – red line).
Prior to applying the correction, all the outliers were excluded. After the first analysis, we proceeded to correct the dissolved oxygen data using the same correction method as that used in the Argo programme (Bittig et al., 2018). The principle of this method is to compare the dissolved oxygen measurements performed while the Argo oxygen sensor is in the air with the oxygen partial pressure (pO2) in the air (Johnson et al., 2015). The latter variable is easily calculated from the air temperature, air pressure, and relative humidity acquired by the SD instruments. Considering the fact that the SD oxygen sensor is installed on the hull about 0.5 m below sea surface and that the SD sailing causes mixing of the water surface while sailing, we assume that the SD oxygen sensors were in equilibrium with the atmosphere above, and, furthermore, we can correct for the oxygen sensor drift using the in-air calibration method (Bittig et al., 2018; Johnson et al., 2015). Specifically, we computed vapour pressure (Vp, in hPa) from the empirical equation reported in the operating manual of the Aanderaa oxygen optode (model 4330) using the air temperature (Tsd) recorded from the SD instruments,
and the expected partial pressure (EPP, in hPa) from the volume fraction of oxygen (; Glueckauf, 1951), atmospheric pressure (APsd), vapour pressure (Vp), and relative humidity (RHsd) as follows:
The EPP was then compared to the pO2 from the SD instruments to compute the gain factor (G) for daily correction.
The corrected oxygen concentration (O2csd) from the SD instruments was calculated by adjusting the oxygen data from the SD instruments (O2sd) with the gain factor.
For each transect, the mean gain was calculated, and then the gain factor was multiplied by the hourly oxygen data, allowing us to correct the time series.
3.3 Correction and adjustment of pCO2 data
3.3.1 Fixed-site pCO2 data acquisition and qualification
The pCO2 measurements from the different fixed ocean stations were regularly compared to the pCO2 calculated from discrete water samples collected by the fixed stations and analysed for TA, pH, and DIC. During the last half of the ATL2MED demonstration experiment, this routine was hampered due to COVID-19 restrictions; thus, between March and July 2020, there were fewer discrete carbon samples for comparison with fixed-station pCO2. Furthermore, there was minor variability in sampling frequency with regards to the fixed-station pCO2 measurements and in the pair of measured variables used for pCO2 calculation (TA–pH or DIC–TA) between the different fixed ocean stations (see Tables A2 and A4). During the ATL2MED demonstration experiment, DIC, TA, and pH were analysed according to SOP 2, 3b, and 6b, respectively (Dickson et al., 2007), with some minor local variations (Table A4). Certified reference material (CRM) and TRIS buffer (buffers based on 2-amino-2-hydroxy-methyl-1,3-propanediol) provided by Andrew Dickson (Scripps, USDC, USA) were used to determine the accuracy. pCO2 was calculated using the speciation software CO2SYS (Pelletier et al., 2007), with the discrete carbon pairs TA–pH or DIC–TA as input variables. In the computation, the carbonate system constants from Lueker et al. (2000), the constant from Dickson (1990), the total borate–salinity relationship of Lee et al. (2010), and the hydrogen fluoride constant KF from Perez and Fraga (1987) were used. The uncertainties connected to this calculation ranged from 1.82 % when using TA–pH as input variables to 2.65 % when DIC–TA were the input variables (Orr et al., 2018). Based on this, no adjustments were performed for the fixed-station pCO2 data when the deviations from pCO2 calculated from discrete carbon data were less than 7.5 and 10 µatm for the discrete carbon pairs TA–pH and DIC–TA, respectively. Uncertainty thresholds were set based on measurement uncertainties at each facility, as well as temperature and pCO2 in the vicinity of the fixed stations.
3.3.2 Correction of the SD CO2 data
The general accuracy of the ASVCO2 system attached to the SD 1030 was checked by PMEL prior to deployment by comparing the results with ESRL CO2 standards traceable to WMO standards (Sutton et al., 2014). For this test, typically, six standard gases were used. On the return of the ASVCO2 system to PMEL, it was discovered that the span gas was adjusted too low to completely flush the detector and that this had been so during the whole ATL2MED demonstration experiment. Thus, the LI-COR had to be recalibrated at the PMEL lab, and this implied that the onboard gas spanning was bypassed, and new calibration coefficients were developed. Furthermore, the pre-mission test data from the PMEL lab were reprocessed using the new calibration coefficients. Based on the reported issues with the ASVCO2 instrument, the accuracy of the CO2 measurements is estimated to be <5 µatm. Laboratory tests of the ASVCO2 system on the SD platforms highlighted an uncertainty of less than 2 µatm (Table 3 in Sutton et al., 2014).
4.1 Salinity
The salinity correction was based on the significant linear correlation (Fig. 3) observed across the different periods (Table 2). The periods characterised by small differences in salinity (<0.1) were not corrected. In general, the corrected salinity for both SD instruments showed similar values (Fig. 5), and the major differences between the two SD instruments were mainly due to their temporal and spatial distance. Overall, the correction was largest for the SD 1053 (see RMSE values in Table 2). To validate the salinity corrected data, a comparison with different observing systems was done.
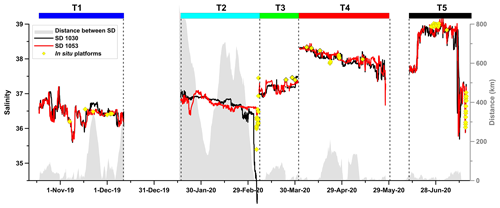
Figure 5Salinities corrected from the SD 1030 (black line) and the SD 1053 (red line) and in situ platforms (Argo floats, RV UCadiz, gliders, and fixed stations; yellow diamonds) over the SD trajectory. The grey-shaded area indicates the distance (km) between the SD trajectories. The coloured rectangles on top of the figure represent the different transects.
For the SD 1030, the corrected salinity data showed a slight overestimation of salinity, while the raw salinity data showed an underestimation. The SD 1030 salinity highlights good agreement in T1 with respect to the SD 1053 (Fig. 5); the average difference was less than 0.05, and the highest difference between Argo float data and corrected salinity data observed on 17 November 2019 was ∼0.15. In T2, the comparison can only be made for the SD 1030 with only one Argo float profile.
Between T2 and T3, a drop in salinity was observed when the SD instruments crossed the ETNA area, where the salinity exhibits a strong variability (Reverdin et al., 2007), triggered by freshwater flux and eddy transport (Gordon and Giulivi, 2014). This salinity drop was also observed in the climatological data (Fig. 2).
The salinity in T2 (SD 1030) differed only slightly (ΔS∼0.05) with respect to the model, and values were in agreement with the observations of the Argo floats during the crossing of the Strait of Gibraltar. In T3, a significant difference was observed between the model and observations (RMSE=0.906; Table 2), while T4 was in line with the climatology, as well as the fixed stations. In T5, the RMSE was 0.279 (Table 2); in the southern Adriatic, the SD instruments spent 4 d sampling the area, which allowed a robust comparison between data from the E2M3A fixed ocean station and the glider measurements. The comparison showed a very good agreement between the observations, which had almost the same salinity. In the northern Adriatic (T5), the comparison with in situ data showed the highest differences with respect to the other in situ platform comparison. However, the comparison with the fixed stations (MIRAMARE and PALOMA) showed the same temporal changes, with an average difference between the SD instruments and the MIRAMARE fixed ocean station of ∼0.3.
Regarding the SD 1053, the comparison with the different fixed ocean stations shows that the corrected salinities in T2, T3, T4, and T5 are consistent with the values measured at the stations (Argo float, glider, buoy, and RV UCadiz), with the differences mainly being due to the distance between the different observatories and to the natural variability of the areas. Also, the corrected data fit well with climatological values and in situ platforms. Considering the fact that, during T1, the SD raw data showed a smaller deviation from the Argo float data, the salinity correction was applied after this transect (i.e. from the start of T2).
4.2 Dissolved oxygen
For dissolved oxygen concentration, it would have been preferable to be able to compare the SD data to discrete data. However, over the period of the ATL2MED demonstration experiment, no discrete dissolved oxygen measurements were available due to COVID-19 restrictions. The corrected oxygen measurements (Fig. 6a) spanned from 170 to 270 µmol kg−1, highlighting the highest concentrations during spring 2020. Time series of the percentage dissolved oxygen saturation did not show any significant trend (Fig. 6b). Oversaturation was observed at the end of October 2019 (∼115 %) and at the beginning of March 2020 (∼105 %), while strong undersaturation was observed on 1–2 April 2020 (∼95 %) and 8–11 July 2020 (∼92 %).
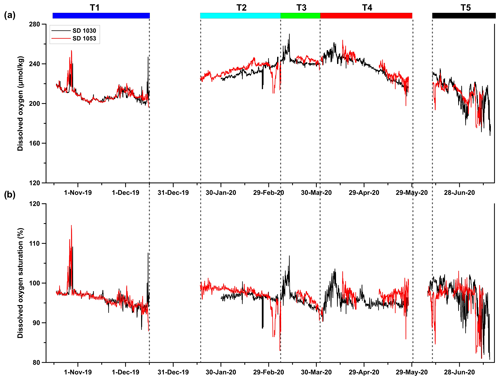
Figure 6(a) Time series of corrected dissolved oxygen concentration and (b) corrected dissolved oxygen saturation (SD 1030 – black line, SD – 1053 red line) in percent.
Furthermore, we evaluated the change in dissolved oxygen measured by the two SD instruments in two different geographical areas (the Canary Islands area and the Balearic basin), where dissolved oxygen showed oversaturation (Fig. 7) and undersaturation (Fig. 8). In the first region, we made use of chl a data and temperature, while, in the second region, temperature was used to evaluate the representativeness of the correction with respect to ecosystem dynamics. The optical sensors on the SD instruments and, thus, the chl a measurements were strongly affected by biofouling for most of the demonstration experiment, which is why we do not use these measurements in this work. However, during the first 10 d in October 2019, the chl a data acquired by the SD instruments seemed to produce reasonable values in accordance with Delory and Jay (2018), who found that, for new sensors, the increase in biofouling needs weeks to become significant. We refer to these chl a data, collected by the SD instruments in transect T1, when explaining the dissolved oxygen oversaturation episode off the Canary Islands.
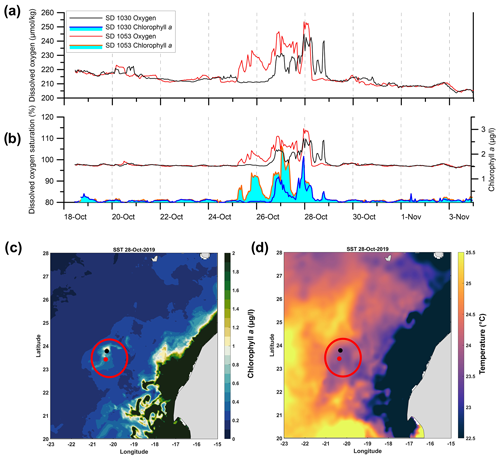
Figure 7Time series of (a) dissolved oxygen concentration and (b) dissolved oxygen saturation in the Canary Islands area. Sea surface chl a concentration (c) and (d) sea surface temperature on 28 October 2019. The red circle highlights the position of the SD instruments (the black dot indicates SD 1030, and the red dot indicates SD1053).
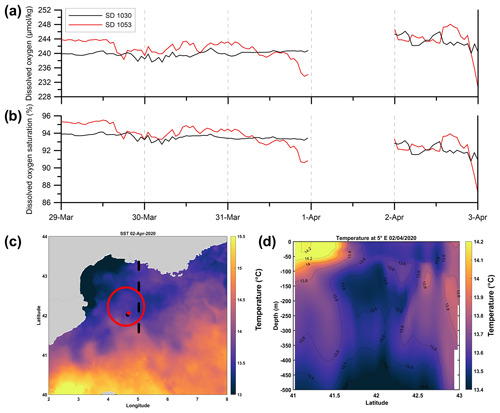
Figure 8Time series of (a) dissolved oxygen concentration and (b) percentage dissolved oxygen saturation in the Balearic basin. (c) Sea surface temperature evolution between 31 March and 2 April 2020. The dotted black line highlights the vertical section in (d). The red circle highlights the position of the SD instruments (the black dot indicates SD 1030, and the red dot indicates SD1053).
The oxygen saturation concentration can be expressed as a function of salinity and temperature in terms of solubility (Garcia and Gordon, 1992). The gas concentration in seawater depends on thermohaline characteristics and biological activity. The solubility of oxygen decreases with increasing temperature and salinity, showing a strong correlation. In the ocean, a dissolved oxygen saturation lower than 100 % can be observed during the cold seasons, while, in the warm season, the oxygen saturation is higher than 100 %, inversely to the dissolved oxygen concentrations (i.e. high concentrations during cold seasons and low concentrations in the warm season). This is because heating and cooling are generally faster than outgassing, except for during episodes of high wind speeds, which intensify the air–sea gas exchange (Ulses et al., 2021). Furthermore, dissolved oxygen concentration is affected by primary production and respiration.
Between 25 and 29 October, the dissolved oxygen concentration and saturation were high around the Canary Islands (>240 µmol kg−1 and >110 %; Fig. 7a and b). During the same period, high concentrations of chl a were measured by the SD instruments (∼2 µg L−1; Fig. 7b, blue and orange lines). The area with high chl a concentrations off the Canary Islands was visible in the satellite images of sea surface chl a concentration (Fig. 7c), and, at the same time, low sea surface temperature was observed (Fig. 7d). High chl a concentrations and low temperatures identify a mesoscale structure that has moved away from the African shelf. Considering the fact that the latter is a very productive area due to the permanent upwelling off the NW African coast (Cropper et al., 2014; Fischer et al., 2016), this justifies the high chl a concentration observed by the SD instruments at that time.
Between 29 March and 3 April 2020, the SD instruments crossed the Balearic basin, reaching the Gulf of Lion on 1 April 2020; the SD 1053 measured a decrease in dissolved oxygen concentrations of about 10 µmol kg−1 (Fig. 8a). This behaviour was also observed in the dissolved oxygen saturation (Fig. 8b), which reached values lower than 95 %. The northern part of the basin was characterised by lower surface temperatures (Fig. 8c) than the southern part. The vertical temperature section (Fig. 8d) highlighted the presence of upwelling of cold water to the surface, justifying the lower surface temperature observed in Fig. 8c. The presence of this upwelled water caused the decrease in dissolved oxygen saturation (Fig. 8b) observed by the SD instruments as the upwelled water is commonly characterised by low dissolved oxygen concentrations due to biological respiration (Chan et al., 2019).
4.3 pCO2
The pCO2 (in µatm) values from the ASVCO2 instrument attached to the SD 1030 were calculated according to Sutton et al. (2014) using temperature and salinity from the SBE37-SMP-ODO at the SD. Figure 9a shows the uncorrected and corrected pCO2 acquired from the SD 1030. In Fig. 9b, the difference between the corrected and uncorrected pCO2 is shown, and the offset increases from approximately 1 µatm at the start of the experiment to approximately 12 µatm at the end.
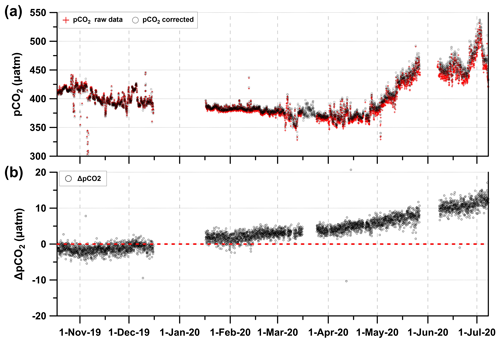
Figure 9(a) Raw (red crosses) and corrected (black circles) pCO2 data acquired by the SD 1030 as functions of time. (b) Difference between raw and corrected pCO2 ().
The pCO2 sensors at the different fixed stations were deployed at depths between 2 to 10 m, while the SD measured at 0.5 m depth. To be able to compare pCO2 measurements from the different depths, the station pCO2 data were normalised to surface temperature by using the relationship of Takahashi et al. (1993):
where T is temperature, and 1 and 2 refer to the measurements of the SD at 0.5 m depth and at the measurement depth of each local station, respectively. Furthermore, the pCO2 measurements acquired by the SD 1030 were compared to the corrected pCO2, surface temperature normalised, from the fixed ocean stations (Fig. 10 and Table 3). The difference varied between −0.5 and −16.9 µatm. The largest difference occurred in the eastern Atlantic, where calculated pCO2 values from discrete DIC and TA were compared to the SD 1030 pCO2 data. Part of this deviation is likely to be attributable to calculation errors, which are estimated to about 10 µatm when errors in both DIC, TA, and the carbon constants are included (Orr et al., 2018). The smallest difference between the SD 1030 pCO2 and the pCO2 acquired from the fixed stations and normalised to surface temperature is seen at DYFAMED toward the end of April 2020 (−2.9 µatm) and at MIRAMARE in mid-July 2020 (−0.5 µatm). The larger discrepancy at W1M3A and PALOMA might be attributable to processes which are not taken into account by temperature normalising, e.g. spatial gradients due to primary production or remineralisation, which would decrease or increase the pCO2. However, it is difficult to estimate the impact of these processes.
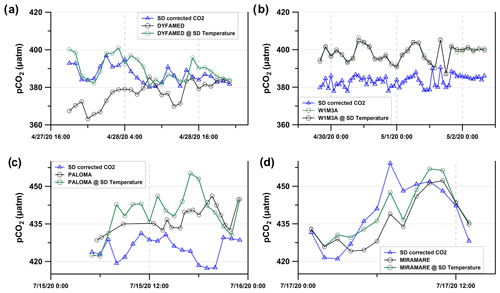
Figure 10Comparison between pCO2 measured by the SD 1030 (blue) and at the fixed ocean stations (black and green): the fixed-ocean-station pCO2 measured at in situ depth and temperature (black) and the fixed-ocean-station pCO2 normalised to surface temperature (green). (a) DYFAMED fixed station, (b) W1M3A fixed station, (c) PALOMA fixed station, and (d) MIRAMARE fixed station.
To assess the representativeness of the pCO2 correction in terms of ecosystem dynamics, a comparison was made between the corrected pCO2, temperature, and chl a concentrations from satellites. The pCO2 in seawater is influenced by primary production, respiration, air–sea gas exchange, formation and dissolution of calcium carbonates, water mixing, riverine discharges, and advection (Zeebe and Wolf-Gladrow, 2007; Bauer et al., 2013; Millero, 2007), which leads to significant variations in different regions. The temperature affects the pCO2 through the thermodynamic dissociation constants of the carbonic acids, which directly affect the CO2 equilibria (e.g. Millero, 2007) and also, to a lesser extent, the gas solubility.
Throughout the ATL2MED demonstration experiment, the pCO2 value (Fig. 11a) showed almost the same pattern as the surface temperature (Fig. 11b), and, furthermore, the pCO2 values in the ETNA were lower than those in the Mediterranean at the same sea surface temperature. The main reason for this difference is attributed to the lower DIC in the Atlantic waters with respect to the Mediterranean (Álvarez et al., 2014).
We observed the highest pCO2 variability in the Mediterranean Sea as the temperature increased by more than 15 °C from winter to summer, leading to an increase in pCO2. A reduction in pCO2 due to phytoplankton photosynthesis is present at the end of the mission in the northern Adriatic, where the fertilisation by nutrients carried by the Po River induced an increase in chl a concentrations (green line in Fig. 11b).
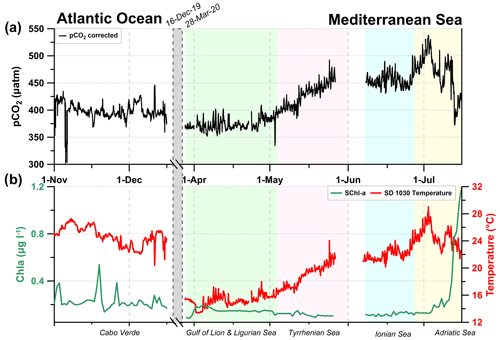
Figure 11Shown are the (a) pCO2 and (b) temperature measured by the SD 1030 (red) and the sea surface chl a concentration (from satellite in green) between 1 November 2019 and 8 July 2020. The x axis was interrupted between 16 December 2019 and 28 March 2020 to highlight the Cabo Verde area and the Mediterranean Sea; the coloured boxes evidence the different Mediterranean sub basins.
The ATL2MED demonstration experiment, which lasted for 273 d, represented the first monitoring experiments of the SD instruments covering both the ETNA region and the Mediterranean Sea, evaluating dynamics between fixed ocean stations within the same basin, as well as comparing characteristics between basins. The experiment covered all seasons with varying meteorological and oceanographic conditions, primary productivity, and maritime traffic. The ATL2MED lasted longer than planned primarily due to challenges with heavy biofouling of the two SD instruments, COVID-19 pandemic restrictions, low winds, and strong contrary winds.
A huge amount of data has been produced during the ATL2MED demonstration experiment, and the data required quality controlling and assurance to varying degrees, primarily depending on how sensitive the sensors were to biofouling. Due to the COVID-19 pandemic restrictions, there was a lack of validation samples collected from cruise transects, Argo floats, and fixed stations, and this has enforced a new way of thinking regarding drift correction. The SBE salinity data acquired by the SD instruments have been corrected, when necessary, using model products, and the method was validated by comparing the data corrected with available in situ measurements. This resulted in remarkable consistency in the corrected salinity values between both the SD instruments. Data from the Aanderaa dissolved oxygen sensors mounted on the SD instruments were corrected, making use of in-air oxygen measurements to correct for the erroneous trend in O2 saturation (%). The pCO2 data from the SD 1030 were corrected at PMEL and compared with pCO2 data acquired from fixed ocean stations. The corrected SD data sets fit well with the data from fixed stations and gliders, which means that the correction methods used are valid. The output is data sets that are available for process interpretations in future research.
Other SD sensors were affected by biofouling to such a degree that the data sets were unable to be corrected given the limited samples available for validation, such as the optical sensors for fluorescence measurements. Some recommendations related to this issue are presented in the next section.
The ATL2MED demonstration experiment is an example of how ASVs can be used to perform multi-variable and high-resolution sampling from areas which are not easily accessible, e.g. due to remote locations, limited ship time availability, or pandemic restrictions. The SD instruments are environmentally friendly platforms, and they, together with other ASVs, are useful as a complement in the validation of fixed ocean stations. However, the experiment clearly shows some of the challenges faced when this type of surface vehicle is part of long-term missions.
Data described in this work are available from different sources; see Table A6 in the Appendix A.
Our experiences and recommendations from the ATL2MED demonstration experiment can be summarised in the bullet points below, which are explained in more detail at the end of this paragraph.
We experienced that
-
the SD sensors were exposed to severe biofouling
-
a substantial amount of effort was required to correct the SD data sets
-
some of the SD sensors were mounted in an unfavourable way
-
the COVID-19 pandemic limited the access to ship time and thus impacted the collection of discrete validation samples.
We recommend
-
ensuring a frequency of the maintenance and cleaning of the SD sensors and the hull that is adapted to the local environment
-
the use of bio-limiting equipment on the SD instruments
-
implementing an automatic in-air calibration procedure for SD oxygen measurements
-
ensuring that the SD sensors are mounted in such a way that they are exposed to open water
-
ensuring that a sufficient amount of independent measurements (e.g. salinity, dissolved oxygen, carbonate system, chl a) are collected in the vicinity of the SD trajectories in order to validate the SD sensors.
In general, the use of SD instruments requires considerable effort to ensure that the data are of scientifically usable quality as these vehicles operate on the surface and are more exposed to biofouling. For future trials, a frequency of sensor cleaning and hull maintenance cleaning should be introduced depending on the monitoring area. In situations where this is not possible, bio-limiting devices should be used, such as UV systems and wipers powered by the solar panels that regularly clean the optical sensors. Experience from the ATL2MED demonstration experiment has shown that the SBE37 sensors appear to be reliable and robust with respect to biofouling. Regarding the dissolved oxygen correction, it is recommended that an in-air calibration, as used for Argo floats, be performed to be able to correct the drift of the oxygen sensor more easily.
The ATL2MED demonstration experiment suffered from a lack of discrete samples for validation. Therefore, future experiments should be organised to collect discrete samples for acquired variables at appropriate frequencies, which will greatly facilitate validation of the quality of the SD data set. Finally, the suitability of SD instruments as a tool to validate other types of measuring platforms (e.g. fixed ocean stations, mobile devices, or ships) strongly depends on various conditions, such as the distance to the platforms, the depth of measurements at fixed stations, and the environmental conditions. All these factors need to be carefully considered to ensure the best possible data set for such a validation.
Table A2Instruments, sensors, accuracy, and associated measurement frequencies for the different fixed ocean stations, gliders, and ships during the ATL2MED demonstration experiment.
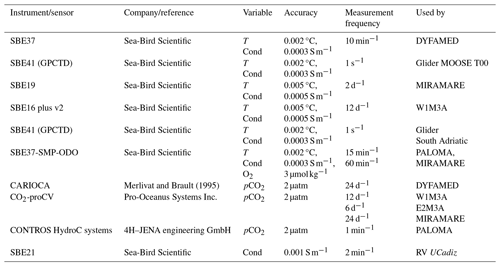
T refers ti temperature, Cond refers to conductivity, O2 refers to dissolved oxygen, and pCO2 refer to partial pressure of carbon dioxide.
Table A3Instruments and sensors of the SD devices from Saildrone Inc. during the ATL2MED demonstration experiment and used in this work.
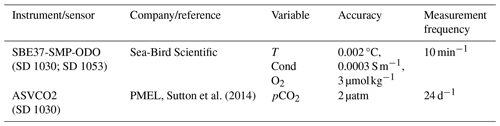
T refers to temperature, Cond refers to conductivity, O2 refers to dissolved oxygen, and pCO2 refers to partial pressure of carbon dioxide.
Table A4Instruments and methods used to analyse discrete samples collected by the RV Meteor and from different fixed stations during the ATL2MED demonstration experiment.
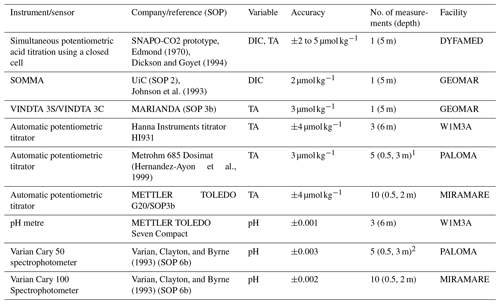
O2 refers to dissolved oxygen, DIC refers to dissolved inorganic carbon, and TA refers to total alkalinity.
1 For each measurement, two replicate samples were collected and analysed.
2 For each measurement, two replicate samples were collected, and two to three analyses were performed at each replicate.
SOP refers to the standard operating procedure according to Dickson et al. (2007).
Table A5Temperature offsets between SD sensor (SBE37-SMP-ODO) at 0.5 m depth and fixed stations during the ATL2MED demonstration experiment. More details are available in Skjelvan et al. (2021).
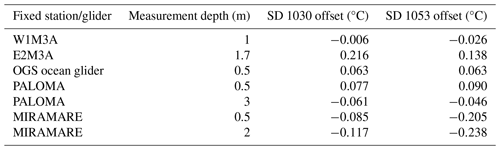
RM: data curation, investigation, validation, visualisation, writing (original draft), writing (review and editing), conceptualisation, formal analysis, methodology, software. MG: investigation, validation, methodology, writing (original draft), writing (review and editing), conceptualisation, formal analysis. EM: writing (original draft), writing (review and editing), funding acquisition, resources. LC: methodology, writing (review and editing), funding acquisition, investigation. MP: writing (review and editing), investigation, data curation. MF: writing (review and editing), investigation, data curation. SP: writing (review and editing), investigation, data curation. VC: writing (review and editing), conceptualisation, funding acquisition, resources. CD: writing (review and editing), investigation, data curation. RB: writing (review and editing), data curation. CC: writing (review and editing), data curation. AL: writing (review and editing), data curation. AI: writing (review and editing), data curation. MB: writing (review and editing), data curation. IS: data curation, investigation, validation, visualisation, writing (original draft), writing (review and editing), conceptualisation, methodology, funding acquisition, project administration, resources.
The contact author has declared that none of the authors has any competing interests.
Publisher's note: Copernicus Publications remains neutral with regard to jurisdictional claims made in the text, published maps, institutional affiliations, or any other geographical representation in this paper. While Copernicus Publications makes every effort to include appropriate place names, the final responsibility lies with the authors.
The ATL2MED experiment has received generous funding from the US company PEAK6 Invest and invaluable support regarding coordination, operation, and data deliverance from Saildrone Inc. Furthermore, funding has been provided by the GEOMAR Helmholtz Centre for Ocean Research (GEOMAR), the Integrated Carbon Observation System – Ocean Thematic Centre (ICOS-OTC), the French National Centre for Scientific Research (CNRS), the Oceanography Laboratory of Villefranche (LOV), the Oceanic Platform of the Canary Islands (PLOCAN), the Ocean Science Centre Mindelo (OSCM), the Hydrographic Institute of Portugal (IH), the Balearic Islands Coastal Observing and Forecasting System (SOCIB), the Italian National Institute of Oceanography and Applied Geophysics (OGS), the Helmholtz-Zentrum Hereon Geesthacht (HZG), the Centre Scientifique de Monaco (CSM), the National Research Council-Institute of Marine Sciences (CNR-ISMAR), and the National Research Council – Institute for the study of Anthropic Impact and Sustainability in the Marine Environment (CNR-IAS). We thank the OGS engineers Paolo Mansutti and Giuseppe Siena for their assistance during the final recovery of the SD instruments, and we thank Piero Zuppelli, Riccardo Gerin, Antonio Bussani, and Massimo Pacciaroni for piloting the OGS glider. Furthermore, we thank Björn Fiedeler and Benjamin Pfeil for initialising the demonstration experiment and for executing the first phase of the experiment. Finally, we thank Adrienne Sutton and Stacy Manner (PMEL) for the invaluable help with correcting the ASVCO2 pCO2 data.
This paper was edited by Xingchen (Tony) Wang and reviewed by three anonymous referees.
Álvarez, M., Sanleón-Bartolomé, H., Tanhua, T., Mintrop, L., Luchetta, A., Cantoni, C., Schroeder, K., and Civitarese, G.: The CO2 system in the Mediterranean Sea: a basin wide perspective, Ocean Sci., 10, 69–92, https://doi.org/10.5194/os-10-69-2014, 2014.
Bauer, J. E., Cai, W.-J., Raymond, P. A., Bianchi, T. S., Hopkinson, C. S., and Regnier, P. A. G.: The changing carbon cycle of the coastal ocean, Nature, 504, 61–70, 2013.
Bittig, H. C., Körtzinger, A., Neill, C., van Ooijen, E., Plant, J. N., Hahn, J., Johnson, K. S., Jang, B., and Emerson, S. R.: Oxygen optode sensors: principle, characterization, calibration, and application in the ocean, Front. Mar. Sci., 4, 429, https://doi.org/10.3389/fmars.2017.00429, 2018.
Bosse, A., Testor, P., Mortier, L., Prieur, L., Taillandier, V., D'Ortenzio, F., and Coppola, L.: Spreading of Levantine Intermediate Waters by submesoscale coherent vortices in the northwestern Mediterranean Sea as observed with gliders, J. Geophys. Res-Oceans, 120, 1599–1622, https://doi.org/10.1002/2014JC010263, 2015.
Bozzano, R. and Pensieri, S.: W1M3A fixed station data collected as part of the ATL2MED demonstration experiment 2019–2020, ICOS database [data set], https://hdl.handle.net/11676/Z9bGSnVObyglR0o8zcvmIXBz, last access: 16 September 2024.
Bozzano, R., Pensieri, S., Pensieri, L., Cardin, V., Brunetti, F., Bensi, M., Petihakis, G., Tsagaraki, T. M., Ntoumas, M., Podaras, D., and Perivoliotis, L.: The M3A network of open ocean observatories in the Mediterranean Sea, in: 2013 MTS/IEEE OCEANS-Bergen, IEEE, Bergen, Norway, 10–14 June 2013, 1–10, https://doi.org/10.1109/OCEANS-Bergen.2013.6607996, 2013.
Buongiorno Nardelli, B., Tronconi, C., Pisano, A., and Santoleri, R.: High and Ultra-High resolution processing of satellite Sea Surface Temperature data over Southern European Seas in the framework of MyOcean project, Copernicus Monitoring Environment Marine Service (CMEMS) [data set], https://doi.org/10.48670/moi-00172, 2022.
Canepa, E., Pensieri, S., Bozzano, R., Faimali, M., Traverso, P., and Cavaleri, L.: The ODAS Italia 1 buoy: More than forty years of activity in the Ligurian Sea, Prog. Oceanogr., 135, 48–63, https://doi.org/10.1016/j.pocean.2015.04.005, 2015.
Cantoni, C. and Luchetta, A.: PALOMA fixed station data collected as part of the ATL2MED demonstration experiment 2019–2020, ICOS database [data set], https://hdl.handle.net/11676/an-PJSKTiEVHj3H0gA8ak3lG, last access: 16 September 2024.
Cantoni, C., Luchetta, A., Celio, M., Cozzi, S., Raicich, F., and Catalano, G.: Carbonate system variability in the gulf of Trieste (north Adriatic Sea), Estuar. Coast. Shelf S., 115, 51–62, https://doi.org/10.1016/j.ecss.2012.07.006, 2012.
Capó, E., McWilliams, J. C., Mason, E., and Orfila, A.: Intermittent frontogenesis in the Alboran Sea, J. Phys. Oceanogr., 51, 1417–1439, 2021.
Cardin, V., Ursella, L., Siena, G., Brunetti, F., Kuchler, S., and Partescano, P.: E2M3A-2017-2019-CTD-time-series-South Adriatic, OGS [data set], https://doi.org/10.6092/D0D50095-BD30-4FF7-8D0A-A12121E72F78, 2020.
Chan, F., Barth, J. A., Kroeker, K. J., Lubchenco, J., and Menge, B. A.: The dynamics and impact of ocean acidification and hypoxia, Oceanography, 32, 62–71, 2019.
Civitarese, G., Gačić, M., Batistić, M., Bensi, M., Cardin, V., Dulčić, J., Garić, R., and Menna, M.: The BiOS mechanism: history, theory, implications, Prog. Oceanogr., 216, 103056, https://doi.org/10.1016/j.pocean.2023.103056, 2023.
Clayton, T. D. and Byrne, R. H.: Spectrophotometric seawater pH measurements: total hydrogen ion concentration scale calibration of m-creosol purple and at-sea results, Deep-Sea Res., 40, 2115–2129, 1993.
Clementi, E., Aydogdu, A., Goglio, A. C., Pistoia, J., Escudier, R., Drudi, M., Grandi, A., Mariani, A., Lyubartsev, V., Lecci, R., Cretí, S., Coppini, G., Masina, S., and Pinardi, N.: Mediterranean Sea Physical Analysis and Forecast (CMEMS MED-Currents, EAS6 system) (Version 1), Copernicus Monitoring Environment Marine Service (CMEMS) [data set], https://doi.org/10.25423/CMCC/MEDSEA_ANALYSISFORECAST_PHY_006_013_EAS7, 2021.
Coppola, L., Raimbault, P., Mortier, L., and Testor, P.: Monitoring the environment in the northwestern Mediterranean Sea, Eos T. Am. Geophys. Un., 100, https://doi.org/10.1029/2019EO125951, 2019.
Coppola, L., Diamond, R. E., Carval, T., Irisson, J. O., and Desnos, C.: Dyfamed observatory data, SEANOE [data set], https://doi.org/10.17882/43749, 2023.
Cropper, T. E., Hanna, E., and Bigg, G. R.: Spatial and temporal seasonal trends in coastal upwelling off Northwest Africa, 1981–2012, Deep-Sea Res. Pt. I, 86, 94–111, https://doi.org/10.1016/j.dsr.2014.01.007, 2014.
Delauney, L., Compère, C., and Lehaitre, M.: Biofouling protection for marine environmental sensors, Ocean Sci., 6, 503–511, https://doi.org/10.5194/os-6-503-2010, 2010.
Delory, E. and Jay, P. (Eds.): Challenges and Innovations in Ocean In Situ Sensors: Measuring Inner Ocean Processes and Health in the Digital Age, Elsevier, 408. pp, ISBN: 9780128098868, 2018.
Dickson, A. G.: Standard potential of the reaction: , and the standard acidity constant of the ion in synthetic sea water from 273.15 to 318.15 K, J. Chem. Thermodyn., 22, 113–127, https://doi.org/10.1016/0198-0149(90)90004-F, 1990.
Dickson, A. G. and Goyet, C.: Handbook of methods for the analysis of the various parameters of the carbon dioxide system in sea water, Version 2, Oak Ridge National Lab. (ORNL), https://doi.org/10.2172/10107773, 1994.
Dickson, A. G., Sabine, C. L., and Christian, J. R. (Eds.): Guide to best practices for ocean CO2 measurements, PICES Special Publication 3, North Pacific Marine Science Organization Sidney, British Columbia, 191, https://doi.org/10.25607/OBP-1342, 2007.
Edmond, J. M.: High precision determination of titration alkalinity and total carbon dioxide content of sea water by potentiometric titration, Deep-Sea Research and Oceanographic Abstracts, 17, 737–750, 1970.
Escudier, R., Clementi, E., Omar, M., Cipollone, A., Pistoia, J., Aydogdu, A., Drudi, M., Grandi, A., Lyubartsev, V., Lecci, R., Cretí, S., Masina, S., Coppini, G., and Pinardi, N.: Mediterranean Sea Physical Reanalysis (CMEMS MED-Currents) (Version 1), Copernicus Monitoring Environment Marine Service (CMEMS) [data set], https://doi.org/10.25423/CMCC/MEDSEA_MULTIYEAR_PHY_006_004_E3R1I, 2020.
Escudier, R., Clementi, E., Cipollone, A., Pistoia, J., Drudi, M., Grandi, A., Lyubartsev, V., Lecci, R., Aydogdu, A., Delrosso, D., Omar, M., Masina, S., Coppini, G., and Pinardi, N.: A High Resolution Reanalysis for the Mediterranean Sea, Front. Earth Sci., 9, https://doi.org/10.3389/feart.2021.702285, 2021.
E.U. Copernicus Marine Service Information (CMEMS): Global Ocean Physics Analysis and Forecast, Marine Data Store (MDS) [data set], https://doi.org/10.48670/moi-00016, last access: 2024a.
E.U. Copernicus Marine Service Information (CMEMS): Mediterranean Sea- In-Situ Near Real Time Observations, Marine Data Store (MDS) [data set], https://doi.org/10.48670/moi-00044, last access: 2024b.
E.U. Copernicus Marine Service Information (CMEMS): Mediterranean Sea, Bio-Geo-Chemical, L3, daily Satellite Observations (Near Real Time), Marine Data Store (MDS) [data set], https://doi.org/10.48670/moi-00297, 2024c.
Fischer, G., Romero, O., Merkel, U., Donner, B., Iversen, M., Nowald, N., Ratmeyer, V., Ruhland, G., Klann, M., and Wefer, G.: Deep ocean mass fluxes in the coastal upwelling off Mauritania from 1988 to 2012: variability on seasonal to decadal timescales, Biogeosciences, 13, 3071–3090, https://doi.org/10.5194/bg-13-3071-2016, 2016.
Friederich, G. E., Brewer, P. G., Herlien, R., and Chavez, F. P.: Measurement of sea surface partial pressure of CO2 from a moored buoy, Deep-Sea Res. Pt. I, 42, 1175–1186, https://doi.org/10.1016/0967-0637(95)00044-7, 1995.
Gačić, M., Ursella, L., Kovačević, V., Menna, M., Malačič, V., Bensi, M., Negretti, M.-E., Cardin, V., Orlić, M., Sommeria, J., Viana Barreto, R., Viboud, S., Valran, T., Petelin, B., Siena, G., and Rubino, A.: Impact of dense-water flow over a sloping bottom on open-sea circulation: laboratory experiments and an Ionian Sea (Mediterranean) example, Ocean Sci., 17, 975–996, https://doi.org/10.5194/os-17-975-2021, 2021.
Garcia, H. E. and Gordon, L. I.: Oxygen solubility in seawater: Better fitting equations, Limnol. Oceanogr., 37, 1307–1312, 1992.
GDAC: Global data centres, ftp://ftp.ifremer.fr/argo, last access: 17 October 2023.
Gentemann, C. L., Scott, J. P., Mazzini, P. L. F., Pianca, C., Akella, S., Minnett, P. J., Cornillon, P., Fox-Kemper, B., Cetinić, I., Chin, T. M., Gomez-Valdes, J., Vazquez-Cuervo, J., Tsontos, V., Yu, L., Jenkins, R., De Halleux, S., Peacock, D., and Cohen, N.: Salidrone – Adaptively sampling the marine environment, B. Am. Meteorol. Soc., 101, E744–E762, https://doi.org/10.1175/BAMS-D-19-0015.1, 2020.
Gerin, R., Bussani, A., Kuchler, S., Martellucci, R., Pacciaroni, M., Pirro, A., Zuppelli, P., and Mauri, E.: OGS GLIDER MISSION Convex20 Dataset, OGS [data set], https://doi.org/10.13120/e7277c6b-444a-4d61-8288-596af1bac3ff, 2021.
Giani, M.: MIRAMARE fixed station data collected as part of the ATL2MED demonstration experiment 2019–2020, ICOS database [data set], https://hdl.handle.net/11676/ngPlu-Q0dtDcDx2wMFTNOtnZ, last access: 16 September 2024.
Glueckauf, E.: The Composition of Atmospheric Air, in: Compendium of Meteorology, edited by: Malone, T. F., American Meteorological Society, Boston, MA, 3–10, https://doi.org/10.1007/978-1-940033-70-9_1, 1951.
Gonzalez, A. I. and Bruno, M.: Data from RV Ucadiz, 5–6 March 2020, ICOS database [data set], https://fileshare.icos-cp.eu/s/eyLp9m685QA8ME7, last access: 16 September 2024.
Gordon, A. L. and Giulivi, C. F.: Ocean eddy freshwater flux convergence into the North Atlantic subtropics, J. Geophys. Res.-Oceans, 119, 3327–3335, 2014.
Hernandez-Ayon, J. M., Belli, S. L., and Zirino, A.: pH, alkalinity and total CO2 in coastal seawater by potentiometric titration with a difference derivative readout, Anal. Chim. Acta, 394, 101–108, 1999.
Johnson, K. M., Wills, K. D., Butler, D. B., Johnson, W. K., and Wong, C. S.: Coulometric total carbon dioxide analysis for marine studies, Mar. Chem., 44, 167–187, 1993.
Johnson, K. S., Plant, J. N., Riser, S. C., and Gilbert, D.: Air oxygen calibration of oxygen optodes on a profiling float array, J. Atmos. Ocean. Tech., 32, 2160–2172, https://doi.org/10.1175/JTECH-D-15-0101.1, 2015.
Kokkini, Z., Mauri, E., Gerin, R., Poulain, P.-M., Simoncelli, S., and Notarstefano, G.: On the salinity structure in the South Adriatic as derived from float and glider observations in 2013–2016, Deep-Sea Res. Pt. II, 171, 104625, https://doi.org/10.1016/j.dsr2.2019.07.013, 2019.
Lee, K., Kim, T.-W., Byrne, R. H., Millero, F .J., Feely, R. A., and Liu, Y.-M.: The universal ratio of boron to chlorinity for the North Pacific and North Atlantic oceans, Geochim. Cosmochim. Ac., 74, 1801–1811, https://doi.org/10.1016/j.gca.2009.12.027, 2010.
Lueker, T. J., Dickson, A. G., and Keeling, C. D.: Ocean pCO2 calculated from dissolved inorganic carbon, alkalinity, and equations for K1 and K2: validation based on laboratory measurements of CO2 in gas and seawater at equilibrium, Mar. Chem., 70, 105–119, https://doi.org/10.1016/S0304-4203(00)00022-0, 2000.
Lüger, H., Wallace, D. W., Körtzinger, A., and Nojiri, Y.: The pCO2 variability in the midlatitude North Atlantic Ocean during a full annual cycle, Global Biogeochem. Cy., 18, GB3023, https://doi.org/10.1029/2003GB002200, 2004.
Martellucci, R., Salon, S., Cossarini, G., Piermattei, V., and Marcelli, M.: Coastal phytoplankton bloom dynamics in the Tyrrhenian Sea: Advantage of integrating in situ observations, large-scale analysis and forecast systems, J. Marine Syst., 218, 103528, https://doi.org/10.1016/j.jmarsys.2021.103528, 2021.
Mauri, E., Gerin, R., and Poulain, P.-M.: Measurements of water-mass properties with a glider in the South-western Adriatic Sea, J. Oper. Oceanogr., 9, S3–S9, https://doi.org/10.1080/1755876X.2015.1117766, 2016.
Mauri, E., Menna, M., Garić, R., Batistić, M., Libralato, S., Notarstefano, G., Martellucci, R., Gerin, R., Pirro, A., Hure, M., and Poulain, P.-M.: Recent changes of the salinity distribution and zooplankton community in the South Adriatic Pit, J. Oper. Oceanogr., 14, 1–185, https://doi.org/10.1080/1755876X.2021.1946240, 2021.
Menna, M., Gačić, M., Martellucci, R., Notarstefano, G., Fedele, G., Mauri, E., Gerin, R., and Poulain, P. M.: Climatic, decadal, and interannual variability in the upper layer of the Mediterranean Sea using remotely sensed and in-situ data, Remote Sens.-Basel, 14, 1322, https://doi.org/10.3390/rs14061322, 2022.
Menna, M., Martellucci, R., Reale, M., Cossarini, G., Salon, S., Notarstefano, G., Mauri, E., Poulain, P.-M., Gallo, A., and Solidoro, C.: Impacts of an extreme weather system on the oceanographic features of the Mediterranean Sea: the Medicane Apollo, Sci. Rep.-UK, 13, 3870, https://doi.org/10.1038/s41598-023-29942-w, 2023.
Merchant, C. J., Embury, O., Bulgin, C. E., Block, T., Corlett, G. K., Fiedler, E., Good, S. A., Mittaz, J., Rayner, N. A., Berry, D., Eastwood, S., Taylor, M., Tsushima, Y., Waterfall, A., Wilson, R., and Donlon, C.: Satellite-based time-series of sea-surface temperature since 1981 for climate applications, Scientific Data, 6, 223, https://doi.org/10.1038/s41597-019-0236-x, 2019.
Merlivat, L. and Brault, P.: CARIOCA Buoy: Carbon Dioxide Monitor, Sea Technol., 10, 23–30, 1995.
Merlivat, L., Boutin, J., Antoine, D., Beaumont, L., Golbol, M., and Vellucci, V.: Increase of dissolved inorganic carbon and decrease in pH in near-surface waters in the Mediterranean Sea during the past two decades, Biogeosciences, 15, 5653–5662, https://doi.org/10.5194/bg-15-5653-2018, 2018.
Mihanović, H., Vilibić, I., Šepić, J., Matić, F., Ljubešić, Z., Mauri, E., Gerin, R., Notarstefano, G., and Poulain, P.-M.: Observation, Preconditioning and Recurrence of Exceptionally High Salinities in the Adriatic Sea, Front. Mar. Sci., 8, 834, https://doi.org/10.3389/fmars.2021.672210, 2021.
Millero, F. J.: The Marine Inorganic Carbon Cycle, Chem Rev., 107, 308–341, https://doi.org/10.1021/cr0503557, 2007.
Neri, F., Romagnoli, T., Accoroni, S., Ubaldi, M., Garzia, A., Pizzuti, A., Campanelli, A., Grilli, F., Marini, M., and Totti, C.: Phytoplankton communities in a coastal and offshore stations of the northern Adriatic Sea approached by network analysis and different statistical descriptors, Estuar. Coast. Shelf S., 282, 108224, https://doi.org/10.1016/j.ecss.2023.108224, 2023.
Orr, J. C., Epitalon, J.-M., Dickson, A. G., and Gattuso, J.-P.: Routine uncertainty propagation for the marine carbon dioxide system, Mar. Chem., 207, 84–107, https://doi.org/10.1016/j.marchem.2018.10.006, 2018.
Pastor, F., Valiente, J. A., and Palau, J. L.: Sea surface temperature in the Mediterranean: Trends and spatial patterns (1982–2016), in: Meteorology and Climatology of the Mediterranean and Black Seas, 297–309, https://doi.org/10.1007/978-3-030-11958-4_18, 2019.
Paulsen M. and Fiedler, B.: Data from RV Meteor 30 November 2019, ICOS database [data set], https://fileshare.icos-cp.eu/s/eyLp9m685QA8ME7 (last access: 16 September 2024), 2023.
Pelletier, G., Lewis, E., and Wallace, D.: CO2SYS.XLS: A calculator for the CO2 system in seawater for Microsoft Excel/VBA, Wash. State Dept. of Ecology/Brookhaven Nat. Lab., Olympia, WA/Upton, NY, USA, http://www.ecy.wa.gov/programs/eap/models.html (last access: 16 Sept 2024), 2007.
Perez, F. F. and Fraga, F.: Association constant of fluoride and hydrogen ions in seawater, Mar. Chem., 21, 161–168, https://doi.org/10.1016/0304-4203(87)90036-3, 1987.
Pinardi, N., Cessi, P., Borile, F., and Wolfe, C.: The Mediterranean Sea Overturning Circulation, J. Phys. Oceanogr., 49, 1699–1721, 2019.
Pirro, A., Mauri, E., Gerin, R., Martellucci, R., Zuppelli, P., and Poulain, P.-M.: New insights on the formation and breaking mechanism of convective cyclonic cones in the South Adriatic Pit during winter 2018, J. Phys. Oceanogr., 52, 2049–2068, https://doi.org/10.1175/JPO-D-21-0108.1, 2022.
Poulain, P.-M., Centurioni, L., Özgökmen, T., Tarry, D., Pascual, A., Ruiz, S., Mauri, E., Menna, M., and Notarstefano, G.: On the Structure and Kinematics of an Algerian Eddy in the Southwestern Mediterranean Sea, Remote Sens.-Basel, 13, 3039, https://doi.org/10.3390/rs13153039, 2021.
Pranić, P., Denamiel, C., Janeković, I., and Vilibić, I.: Multi-model analysis of the Adriatic dense-water dynamics, Ocean Sci., 19, 649–670, https://doi.org/10.5194/os-19-649-2023, 2023.
Ravaioli, M., Bergami, C., Riminucci, F., Langone, L., Cardin, V., Di Sarra, A., Aracri, S., Bastianini, M., Bensi, M., Bergamasco, A., Bommarito, C., Borghini, M., Bortoluzzi, G., Bozzano, R., Cantoni, C., Chiggiato, J., Crisafi, E., D'Adamo, R., Durante, S., Fanara, C., Grilli, F., Lipizer, M., Marini, M., Miserocchi, S., Paschini, E., Penna, P., Pensieri, S., Pugnetti, A., Raicich, F., Schroeder, K., Siena, G., Specchiulli, A., Stanghellini, G., Vetrano, A., and Crise, A.: The RITMARE Italian Fixed-Point Observatory Network (IFON) for marine environmental monitoring: a case study, J. Oper. Oceanogr., 9, S202–S214, https://doi.org/10.1080/1755876X.2015.1114806, 2016.
Reverdin, G., Kestenare, E., Frankignoul, C., and Delcroix, T.: Surface salinity in the Atlantic Ocean (30 S–50 N), Prog. Oceanogr., 73, 311–340, 2007.
Sabine, C., Sutton, A., McCabe, K., Lawrence-Slavas, N., Alin, S., Feely, R., Jenkins, R., Maenner, S., Meinig, C., Thomas, J., van Ooijen, E., Passmore, A., and Tilbrook, B.: Evaluation of a new carbon dioxide system for autonomous surface vehicles, J. Atmos. Ocean. Tech., 37, 1305–1317, 2020.
Skjelvan, I., Coppola, L., Cardin, V., Juza, M., Bozzano, R., Pensieri, S., Giani, M., Siena, G., Urbini, L., Mauri, E., Martellucci, R., Cantoni, C., Luchetta, A., Izquierdo, A., Paulsen, M., and Fiedler, B.: The ATL2MED mission – experiences and lessons learnt, Technical report, ICOS-OTC, https://doi.org/10.18160/9HK5-807K, 2021.
Skjelvan, I., Fiedler, B., and Martellucci, R.: Data from Saildrone 1030 during the ATL2MED demonstration experiment 2019–2020, ICOS database [data set], https://hdl.handle.net/11676/QN7XZKcJ2f4kBCGxQEeDdU3P, last access: 16 September 2024a.
Skjelvan, I., Fiedler, B., and Martellucci, R.: Data from Saildrone 1053 during the ATL2MED demonstration experiment 2019–2020, ICOS database [data set], https://hdl.handle.net/11676/9G9rntDvhmu-4nI4w91O11_g, last access: 16 September 2024b.
Steinhoff, T., Gkritzalis, T., Lauvset, S. K, Jones, S., Schuster, U., Olsen, A., Becker, M., Bozzano, R., Brunetti, F., Cantoni, C., Cardin, V., Diverrès, D., Fiedler, B., Fransson, A., Giani, M., Hartman, S., Hoppema, M., Jeansson, E., Johannessen, T., Kitidis, V., Körtzinger, A., Landa, C., Lefèvre, N., Luchetta, A., Naudts, L., Nightingale, P. D., Omar, A. M., Pensieri, S., Pfeil, B., Castaño-Primo, R., Rehder, G., Rutgersson, A., Sanders, R., Schewe, I., Siena, G., Skjelvan, I., Soltwedel, T., van Heuven, S., and Watson, A.: Constraining the Oceanic Uptake and Fluxes of Greenhouse Gases by Building an Ocean Network of Certified Stations: The Ocean Component of the Integrated Carbon Observation System, ICOS-Oceans, Front. Mar. Sci., 6, 544, https://doi.org/10.3389/fmars.2019.00544, 2019.
Sutton, A. J., Sabine, C. L., Maenner-Jones, S., Lawrence-Slavas, N., Meinig, C., Feely, R. A., Mathis, J. T., Musielewicz, S., Bott, R., McLain, P. D., Fought, H. J., and Kozyr, A.: A high-frequency atmospheric and seawater pCO2 data set from 14 open-ocean sites using a moored autonomous system, Earth Syst. Sci. Data, 6, 353–366, https://doi.org/10.5194/essd-6-353-2014, 2014.
Takahashi, T., Olafsson, J., Goddard, J. G., Chipman, D. W., and Sutherland, S. C.: Seasonal variation of CO2 and nutrients in the high-latitude surface oceans: a comparative study, Global Biogeochem. Cy., 7, 843–878, https://doi.org/10.1029/93GB02263, 1993.
Takeshita, Y., Martz, T. R., Johnson, K. S., Plant, J. N., Gilbert, D., Riser, S. C., Craig, N., and Tilbrook, B.: A climatology-based quality control procedure for profiling float oxygen data, J. Geophys. Res.-Oceans, 118, 5640–5650, 2013.
Tanhua, T., McCurdy, A., Fischer, A., Appeltans, W., Bax, N., Currie, K., DeYoung, B., Dunn, D., Heslop, E., Glover, L. K., Gunn, J., Hill, K., Ishii, M., Legler, D., Lindstrom, E., Miloslavich, P., Moltmann, T., Nolan, G., Palacz, A., Simmons, S., Sloyan, B., Smith, L. M., Smith, N., Telszewski, M., Visbeck, M., and Wilkin, J.: What We Have Learned From the Framework for Ocean Observing: Evolution of the Global Ocean Observing System, Front. Mar. Sci., 6, 471, https://doi.org/10.3389/fmars.2019.00471, 2019.
Testor, P., Mortier, L., Coppola, L., Claustre, H., D'Ortenzio, F., Bourrin, F., Durrieu de Madron, X., and Raimbault, P.: Glider MOOSE sections, SEANOE [data set], https://www.seanoe.org/data/00409/52027/ (last access: 16 September 2024), 2017.
Testor, P., de Young, B., Rudnick, D. L., Glenn, S., Hayes, D., Lee, C. M., Pattiaratchi, C., Hill, K., Heslop, E., Turpin, V., Alenius, P., Barrera, C., Barth, J. A., Beaird, N., Bécu, G., Bosse, A., Bourrin, F., Brearley, J. A., Chao, Y., Chen, S., Chiggiato, J., Coppola, L., Crout, R., Cummings, J., Curry, B., Curry, R., Davis, R., Desai, K., DiMarco, S., Edwards, C., Fielding, S., Fer, I., Frajka-Williams, E., Gildor, H., Goni, G., Gutierrez, D., Haugan, P., Hebert, D., Heiderich, J., Henson, S., Heywood, K., Hogan, P., Houpert, L., Huh, S., Inall, E., Ishii, M., Ito, S.-i., Itoh, S., Jan, S., Kaiser, J., Karstensen, J., Kirkpatrick, B., Klymak, J., Kohut, J., Krahmann, G., Krug, M., McClatchie, S., Marin, F., Mauri, E., Mehra, A., Meredith, P., Meunier, T., Miles, T., Morell, J. M., Mortier, L., Nicholson, S., O'Callaghan, J., O'Conchubhair, D., Oke, P., Pallàs-Sanz, E., Palmer, M., Park, J., Perivoliotis, L., Poulain, P.-M., Perry, R., Queste, B., Rainville, L., Rehm, E., Roughan, M., Rome, N., Ross, T., Ruiz, S., Saba, G., Schaeffer, A., Schönau, M., Schroeder, K., Shimizu, Y., Sloyan, B. M., Smeed, D., Snowden, D., Song, Y., Swart, S., Tenreiro, M., Thompson, A., Tintore, J., Todd, R. E., Toro, C., Venables, H., Wagawa, T., Waterman, S., Watlington, R. A., and Wilson, D.: OceanGliders: A component of the integrated GOOS, Front. Mar. Sci., 6, https://doi.org/10.3389/fmars.2019.00422, 2019.
Ulses, C., Estournel, C., Fourrier, M., Coppola, L., Kessouri, F., Lefèvre, D., and Marsaleix, P.: Oxygen budget of the north-western Mediterranean deep- convection region, Biogeosciences, 18, 937–960, https://doi.org/10.5194/bg-18-937-2021, 2021.
Wong, A. P. S., Wijffels, S. E., Riser, S. C., Pouliquen, S., Hosoda, S., Roemmich, D., Gilson, J., Johnson, G. C., Martini, K., Murphy, D. J., Scanderbeg, M., Bhaskar, T. V. S. U., Buck, J. J. H., Merceur, F., Carval, T., Maze, G., Cabanes, C., André, X., Poffa, N., Yashayaev, I., Barker, P. M., Guinehut, S., Belbéoch, M., Ignaszewski, M., Baringer, M. O. N., Schmid, C., Lyman, J. M., McTaggart, K. E., Purkey, S. G., Zilberman, N., Alkire, M. B., Swift, D., Owens, W. B., Jayne, S. R., Hersh, C., Robbins, P., West-Mack, D., Bahr, F., Yoshida, S., Sutton, P. J. H., Cancouët, R., Coatanoan, C., Dobbler, D., Juan, A. G., Gourrion, J., Kolodziejczyk, N., Bernard, V., Bourlès, B., Claustre, H., D'Ortenzio, F., Le Reste, S., Le Traon, P. Y., Rannou, J. P., Saout-Grit, C., Speich, S., Thierry, V., Verbrugge, N., Angel-Benavides, I. M., Klein, B., Notarstefano, G., Poulain, P. M., Vélez-Belchí, P., Suga, T., Ando, K., Iwasaska, N., Kobayashi, T., Masuda, S., Oka, E., Sato, K., Nakamura, T., Sato, K., Takatsuki, Y., Yoshida, T., Cowley, R., Lovell, J. L., Oke, P. R., van Wijk, E. M., Carse, F., Donnelly, M., Gould, W. J., Gowers, K., King, B. A., Loch, S. G., Mowat, M., Turton, J., Rama Rao, E. P., Ravichandran, M., Freeland, H. J., Gaboury, I., Gilbert, D., Greenan, B. J. W., Ouellet, M., Ross, T., Tran, A., Dong, M., Liu, Z., Xu, J., Kang, K. R., Jo, H. J., Kim, S. D., and Park, H. M.: Argo Data 1999–2019: Two Million Temperature-Salinity Profiles and Subsurface Velocity Observations From a Global Array of Profiling Floats, Front. Mar. Sci., 7, 700, https://doi.org/10.3389/fmars.2020.00700, 2020.
Zeebe, R. E. and Wolf-Gladrow, D.: CO2 in seawater: equilibrium, kinetics, isotope, Elsevier, Matserdam, 346 pp., ISBN 9780444509468, 2007.