the Creative Commons Attribution 4.0 License.
the Creative Commons Attribution 4.0 License.
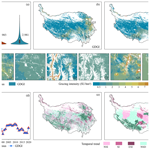
Annual high-resolution grazing-intensity maps on the Qinghai–Tibet Plateau from 1990 to 2020
Jia Zhou
Jin Niu
Ning Wu
Grazing activities constitute the paramount challenge to grassland conservation over the Qinghai–Tibet Plateau (QTP), underscoring the urgency of obtaining detailed information regarding the extent, patterns, and trends of grazing to enable efficient grassland management and sustainable development. Here, to inform stakeholders about these issues, we provided the first annual Gridded Dataset of Grazing Intensity (GDGI), with a resolution of 100 m, from 1990 to 2020 for the QTP. The five most commonly used machine learning algorithms were leveraged to develop a livestock spatialization model, which spatially disaggregates the livestock census data at the county level into a detailed 100 m × 100 m grid based on seven key predictors from terrain, climate, vegetation, and socio-economic factors. Among these algorithms, the extreme-tree (ET) model performed the best in representing the complex nonlinear relationship between various environmental factors and livestock intensity, with an average absolute error of just 0.081 SU ha−2 (where SU denotes sheep units), a rate outperforming the other models by 21.58 %–414.60 %. By using the ET model, we further generated the GDGI for the QTP to reveal the spatio-temporal heterogeneity and variations in grazing intensities. The GDGI indicates that grazing intensity remained high and largely stable from 1990 to 1997, followed by a sharp decline from 1997 to 2001 and fluctuations thereafter. Encouragingly, compared to other open-access datasets for grazing distribution on the QTP, the GDGI has the highest accuracy, with the determinant coefficient (R2) exceeding 0.8. Given its high resolution, recentness, and robustness, we believe that the GDGI dataset can significantly enhance our understanding of the substantial threats to grasslands emanating from overgrazing activities. Furthermore, the GDGI product holds considerable potential as a foundational source for other research, facilitating the rational utilization of grasslands, refined environmental impact assessments, and the sustainable development of animal husbandry. The GDGI product developed in this study is available at https://doi.org/10.5281/zenodo.10851119 (Zhou et al., 2024).
- Article
(10683 KB) - Full-text XML
-
Supplement
(3282 KB) - BibTeX
- EndNote
Livestock is a crucial contributor to global food systems through the provision of essential animal proteins and fats and plays a significant role in supporting human survival and socio-economic development (Gilbert et al., 2018; Godfray et al., 2018; Humpenöder et al., 2022; Kumar et al., 2022). However, the escalating increase in human demand for meat and dairy products over recent decades has triggered a livestock boom, which, in turn, has increasingly threatened grassland ecosystems and placed a heavy burden on the environment through overgrazing and land use change (Tabassum et al., 2016; Wei et al., 2022; Minoofar et al., 2023). It is estimated that up to 300 ×106 ha of land is used globally for grazing and cultivating fodder crops (Tabassum et al., 2016). Grazing activities could alter vegetation phenology and community structure (Dong et al., 2020) and could trigger deforestation (García Ruiz et al., 2020), grassland degradation (Sun et al., 2020), soil erosion (Shakoor et al., 2021), and associated direct releases of greenhouse gases that lead to climate change feedback (Godfray et al., 2018; Chang et al., 2021). Additionally, livestock are responsible for large-scale dispersion of pathogens, organic matter, and residual medications into soil and groundwater, thereby contaminating the environment (Venglovsky et al., 2009; Tabassum et al., 2016; Hu et al., 2017; Muloi et al., 2022). Consequently, more and more scholars have called for attention to provide reliable contemporary datasets to illustrate the spatio-temporal heterogeneity and variations of livestock (Petz et al., 2014; Fetzel et al., 2017; Zhang et al., 2018; Li et al., 2021).
One of the major challenges in monitoring grazing activity at regional or even larger scales is the determination of the livestock distribution pattern. Despite the importance of geographical grazing information, high spatio-temporal grazing datasets remain unavailable, posing the most critical challenge to grassland management, particularly for vulnerable grassland ecosystems in fragile regions grappling with economic- and sustainable-development contradictions (Meng et al., 2023; Pozo et al., 2021; Miao et al., 2020; He et al., 2022). In the early 2000s, the Food and Agriculture Organization of the United Nations (FAO) launched the Gridded Livestock of the World (GLW) project to facilitate a detailed evaluation of livestock production, aiming to provide pixel-scale livestock densities instead of traditional administrative unit benchmarks (Nicolas et al., 2016). Consequently, the world's inaugural dataset of livestock spatialization (GLW1) was released in 2007, providing the first globally standardized livestock density distribution map at a spatial resolution of 0.05 decimal degrees (≈ 5 km at the Equator) for 2002. It was not until 2014 that an updated GLW2 map with a 1 km resolution for 2006 was released; this updated map used a stratified regression approach, superior spatial resolution predictor variables, and more detailed livestock census data (Robinson et al., 2014). Furthermore, an evolutionary step in machine learning technology saw Gilbert et al. (2018) using random forest algorithms to forge a global livestock distribution map with a 10 km resolution for 2010 (GLW3), succeeding traditional multivariate regression methods and surpassing the precision of previous GLW1 and GLW2 maps. Beyond these global mappings, several maps with different scales have also been published, including ones on intercontinental, national, state or provincial, and local scales (Neumann et al., 2009; Prosser et al., 2011; Van Boeckel et al., 2011; Nicolas et al., 2016). However, these maps are fundamentally coarse due to constraints regarding the availability of fine-scale and contemporary census data, the grazing-spatialization method, and the identification of appropriate indicators, thereby limiting their application to local- or regional-scale studies (Nicolas et al., 2016; Gilbert et al., 2018; Robinson et al., 2014). Hence, there is an emergent demand for more refined grazing map products (Mulligan et al., 2020; Martinuzzi et al., 2021).
An example of this need can be observed in the Qinghai–Tibet Plateau (QTP), the world's most elevated pastoral region and an important grazing area in China (Zhan et al., 2023). It is made up of abundant grassland that spans 1.5 ×106 km2, accounting for 50.43 % of China's total grassland area, with Yak and Tibetan sheep as the primary grazing livestock (Feng et al., 2009; Cai et al., 2014; Zhan et al., 2023). Over recent decades, the QTP has undergone escalating grassland degradation, leading to many ecological and socio-economic problems, which represent the urgent need for detailed livestock distribution datasets (M. Q. Li et al., 2022). Unfortunately, despite researchers' efforts at mapping the QTP's grazing intensity, current livestock datasets still suffer from coarse spatio-temporal resolutions and modeling accuracies. Apart from the aforementioned global grazing dataset, several other maps also cover the QTP. For instance, Liu (2021) generated annual 250 m gridded carrying-capacity maps for 2000–2019 by employing multiple linear regressions of livestock numbers, population density, net primary productivity (NPP), and topographic features. Li et al. (2021) used machine learning algorithms to produce gridded livestock distribution data at 1 km resolution for 2000–2015 in western China at a 5-year interval based on county-level livestock census data and 13 factors from land use practice, topography, climate, and socio-economic aspects, including grassland coverage, arable land coverage, forest land coverage, desert coverage, normalized difference vegetation index (NDVI), elevation, slope, daytime surface temperature, precipitation, distance to river, travel time to major cities, population density, and gross domestic product (Li et al., 2021). A contribution by Meng et al. (2023) brought forth longer annual time series grazing maps by using random forest models, integrating climate, soil, NDVI, water distance, and settlement density to decompose county-level livestock census data to a 0.083° (≈ 10 km at the Equator) grid for 1982–2015 (Meng et al., 2023). Similarly, Zhan et al. (2023) also used random forest algorithms to combine 11 influence factors to provide a winter- and summer-grazing density map at 500 m resolution for 2020 (Zhan et al., 2023).
However, although these maps have provided good help in understanding grazing conditions on the QTP, there are currently still no maps that can satisfy the need for fine-scale grassland management with a long time span. In addition, the available livestock distribution maps of the QTP still need improvement in terms of modeling techniques and factor selection to obtain high-precision livestock spatialization data. For example, traditional methods like multiple linear regression, while having been proven to be fundamental and widely applicable for livestock spatialization (Robinson et al., 2014; Ma et al., 2022), are being challenged by the development of computational science in recent years. Among them, machine learning technology is providing new opportunities for more accurate predictions of livestock distribution (García et al., 2020). Random forest regression, for instance, is currently widely used to construct global, national, and regional livestock spatialization datasets and has been proved to have much better accuracy than traditional mapping techniques (Rokach, 2016; Nicolas et al., 2016; Gilbert et al., 2018; Dara et al., 2020; Chen et al., 2019; Li et al., 2021). Nevertheless, other more advanced machine learning methods with superior feature learning and more robust generalization capabilities remain largely untapped for modeling geographic data (Ahmad et al., 2018; Heddam et al., 2020; Long et al., 2022). Thus, exploring the potential application of new advanced machine learning technologies in livestock spatialization remains a critical task. Furthermore, selecting suitable factors that influence livestock grazing preferences is also the other critical challenge for enhancing the precision of grazing-distribution datasets (Meng et al., 2023). Livestock grazing activities are often affected by abiotic and biotic resources, including climatic and environmental factors (Waha et al., 2018), herd foraging and grazing behaviors (Garrett et al., 2018; Miao et al., 2020), and conservation-oriented policies (Li et al., 2021). For instance, regions exceeding elevations of 5600 m or with slopes greater than 40 % are customarily unsuitable for grazing (Luo et al., 2013; Mack et al., 2013; Robinson et al., 2014; Chen et al., 2019). Moreover, the livestock generally prefer areas abundant in water and pasture resources for foraging (Li et al., 2021). Besides, ecological conservation policies also exert a substantial influence, significantly affecting grazing distribution relative to the level of conservation priority. In addition, the health status of the grassland is an important factor influencing whether or not livestock choose to feed (Li et al., 2021). Consequently, indicators related to the above aspects are often employed to gauge the spatial heterogeneity of livestock distribution (Allred et al., 2013; Sun et al., 2021; Meng et al., 2023). Nonetheless, some of the most commonly used indicators like NPP or NDVI can result in misconceptions as they may not fully characterize the grazing intensity. For example, grasslands with high NPP or NDVI are often preferred by livestock, but this does not necessarily correlate with grazing intensity in nature reserves due to strict policy restrictions (Veldhuis et al., 2019; O'Neill and Abson, 2009; Y. Zhang et al., 2021). Conversely, areas with sparse grassland cover may support considerable livestock numbers despite evidence of degradation (B. H. Zhang et al., 2021; Guo et al., 2015). Accordingly, further investigation into novel indicators is imperative to enhance the correlation between grassland and grazing intensity, thereby optimizing the integration of such influencing factors into grazing-spatialization models.
In summary, the QTP is in pressing need of a high-spatio-temporal-resolution grazing dataset to address urgent and realistic challenges. However, the existing livestock datasets specific to the QTP are fraught with several insufficient and predominantly concerning rough resolutions, relatively backward census data, and conventional methods in livestock spatialization. Moreover, the discrepancies in terms of predictive indicators and modeling approaches within these datasets discourage their application in time series analysis. Consequently, the generation of high-resolution and high-quality grazing map products has emerged as the most pressing challenge for the QTP. Here, we aim to (1) establish a methodological framework by using more rational models and indicators than traditional studies to achieve fine-scale livestock spatialization; (2) select the grazing spatialization model with good performance by incorporating multi-source data with advanced machine learning techniques; and (3) ultimately, provide an annual grazing-intensity dataset with 100 m resolution spanning from 1990 to 2020. These maps can not only provide fundamental datasets with finer spatio-temporal resolutions to address the limitations of existing grazing-intensity maps but also enable a better understanding of sustainable management practices, as well as of other grassland-related issues, across the QTP.
2.1 Study area
Known as Asia's water tower and the world's third pole, the QTP is geographically situated between 26°00′–39°47′ N and 73°19′–104°47′ E, with a total area of about 2.61 ×106 km2 (Fig. 1). Its jurisdiction encompasses 182 counties within six provincial regions of China, including the Tibet Autonomous Region, Qinghai Province, Xinjiang Uygur Autonomous Region, Gansu Province, Sichuan Province, and Yunnan Province (Meng et al., 2023). The elevation of the QTP predominantly ranges between 3000 and 5000 m, with an average altitude exceeding 4000 m. With grasslands constituting over half of its land cover, the QTP emerges as one of the most important pastoral areas in China. Alpine steppe, alpine meadow, and temperate steppe characterize the main grassland types on the QTP (Han et al., 2019; Zhai et al., 2022; Y. Y. Zhu et al., 2023). The complex geographical and climatic conditions of the QTP contribute to the markedly heterogeneous grassland distribution, which correspondingly leads to the high heterogeneity in livestock distribution. Moreover, social and economic developments, coupled with policy initiatives directed towards grassland restoration, have noticeably impacted the livestock numbers on the QTP over recent decades (Li et al., 2021; Li et al., 2016).
2.2 Data source
2.2.1 Census livestock data
The county-level census livestock data for the period between 1990 and 2020 were obtained from the Bureau of Statistics of each county across the QTP (Table 1). The data include the number of cattle, sheep, horse, and mule, with the exception of counties in Yunnan Province, which lack data for the years from 1990 to 2007; counties in Ganzi Prefecture in Sichuan Province, which lacks data for the years from 1990 to 1999; and Muli County in Sichuan Province, which lacks data for the years from 1990 to 2007. For these counties belonging to the same prefecture, including counties in Ganzi and Aba prefectures in Sichuan Province, we used the livestock census data at the prefecture level to carry out spatialization. For these counties in Yunnan Province, since they belong to different municipalities, it is not reasonable to replace them with municipal-level data. For these counties without livestock census data for some years, we supplemented the missing data by linear interpolation with grazing-density data in available years. In total, livestock data were available for 182 counties, and 4998 independent records were finally generated. Furthermore, the respective quantities of different livestock types are converted to standard sheep units (SU), in compliance with the Chinese national regulations (Meng et al., 2023).
Due to the difficulty of collecting township-level census livestock data, the validation data at the township scale collected in this study only involved the townships of Baching County (2010–2018) and Gaize County (2018–2020) in Tibet and Hongyuan County in Sichuan Province (2008). The township-level census livestock data cumulatively involve 18 townships with a total of 112 records and were only used for auxiliary validation of the simulation results.
The validation data at the pixel scale also encompass a total of 112 records from 68 sites, which were collected from the literature, questionnaires, and field surveys. Specifically, 93 records at 49 sites spanning the 1990–2021 period were obtained from 17 literature sources, and 19 records from 19 sites were obtained from the questionnaires and the field survey in 2021. The detailed information for these records can be found in Fig. S3 and Table S3 in the Supplement.
2.2.2 Factors affecting grazing activities
Livestock grazing activities are often affected by abiotic and biotic resources, including climatic and environmental factors (Waha et al., 2018), as well as herd foraging and grazing behaviors (Garrett et al., 2018; Miao et al., 2020). For instance, high-altitude and steep hillsides are unsuitable for grazing due to terrain constraints, and the distribution of herders directly affects the grazing areas (Luo et al., 2013; Mack et al., 2013; Robinson et al., 2014; Chen et al., 2019). Moreover, the livestock generally prefer areas abundant in water and pasture resources for foraging (Li et al., 2021). Therefore, in this study, topography, climatic, environmental, and socio-economic impacts were considered to be influential factors in grazing activities (Li et al., 2021; Meng et al., 2023).
We utilized correlation analysis and the random forest importance-ranking tool to eliminate redundant environmental factors and to determine the contribution of each factor. Ultimately, altitude, slope, distance to water source, population density, air temperature, precipitation, and human-induced impacts on NPP (HNPP) were selected as indicators (Table 2). Specifically, elevation is derived from the digital elevation model (DEM) dataset accessible via the Resource and Environmental Data Cloud Platform of the Chinese Academy of Sciences (https://www.gscloud.cn, last access: 12 July 2022), which also facilitated slope calculation. Data on rivers and lakes were obtained from the National Tibetan Plateau Data Center (https://data.tpdc.ac.cn, last access: 21 October 2022), and the nearest Euclidean distance from each pixel to rivers or lakes is calculated accordingly. Meteorological elements such as daily air temperature and precipitation were downloaded from the China Meteorological Data Service Center (http://data.cma.cn, last access: 18 April 2022). For the grid dataset that is not conditionally available, including population density, temperature, precipitation, and HNPP, we detailed the creation process in the Supplement. All datasets utilized in this study were harmonized in relation to consistent coordinate systems and resolutions (WGS 1984 Albers, 100 m).
2.3 Methodological framework
We adopted a comprehensive methodological framework for mapping high-resolution grazing intensity on the QTP. This study applied FAO's assumption that the relationship between environmental factors and livestock intensity is identical at both the administrative and pixel level. Three major steps are included to predict the distribution pattern of grazing intensity: (1) identifying factors affecting grazing activities and extracting theoretically suitable areas for livestock grazing, (2) building a grazing-spatialization model, and (3) filtering the model and correcting the grazing map. An exhaustive explanation of each step is provided in Fig. 2.
2.3.1 Identifying factors and theoretically suitable areas for grazing
In this study, we assumed that grazing activities are confined solely to grassland. Consequently, the potential grazing areas for each year were identified on the basis of grassland boundaries, which were extracted from the 30 m annual land cover dataset (CLCD) (Yang and Huang, 2021). Furthermore, grasslands with slopes over 40 % and elevations higher than 5600 m were considered to be unsuitable for grazing and were therefore excluded from the potential grazing area in the subsequent simulations (Robinson et al., 2014). In addition, the grasslands with a population density greater than 50 inhabitants per square kilometer were also excluded (Li et al., 2018). The remaining isolated grasslands were thus categorized as theoretically feasible grazing regions.
The spatial patterns of abiotic and biotic resources, incorporating food availability, environmental stress, and herder preference, critically affect grazing activities (Meng et al., 2023). In light of this, seven influencing factors covered by four categories were selected for grazing-intensity mapping (Fig. 2.1).
2.3.2 Building a grazing-spatialization model
By assessing regional statistics, the annual average values for each grazing influence factor were extracted from the theoretically suitable grazing areas at the county scale and were further used as independent variables in the model construction. The dependent variable for the model was acquired by determining the livestock density within each county, followed by a logarithmic transformation of the values to normalize the distribution of the dependent variable. Consequently, a total of 4998 samples were derived from the aforementioned independent and dependent variables. Of these samples, 70 % were allocated to model training, while the remaining 30 % comprised the test sets, serving to validate the model's performance. Subsequently, we built grazing-spatialization models using five machine learning algorithms at the county scale, including support vector (SV) regression (Cortes and Vapnik, 1995; Lin et al., 2022), K-nearest neighbors (KNNs) (Cover and Hart, 1967), gradient-boosting (GB) regression (Friedman, 2001; Pan et al., 2019), random forests (RFs) (Breiman, 2001), and extra-tree (ET) regression (Geurts et al., 2006; Ahmad et al., 2018) (see the Supplement for details). Lastly, to assess the accuracy of the spatialized livestock map, the predicted livestock intensity values were juxtaposed with the livestock statistical data from each respective county.
2.3.3 Correcting the grazing map
We further used the optimal model to predict the geographical distribution of grazing density across the QTP. To maintain better consistency between the predicted livestock number and the census data, the estimated results were adjusted using the census livestock numbers at the county scale as a control according to Eq. (1). Consequently, the corrected and refined map is presented as the final grazing-intensity map in this study.
In the above, Lcorrection is the predicted pixel-scale livestock number after adjustment, LCgrid represents the estimated livestock number for each county, LCCensus is the census livestock number for each county, and Lgrid refers to the predicted livestock number at the pixel scale.
2.4 Accuracy evaluation
We used three accuracy validation indices, namely coefficients of determination (R2), mean absolute error (MAE), and root mean square error (RMSE), to evaluate the performance of five machine learning algorithms through a comparison of the predicted value with the census data. The definitions of three metrics are presented in Eqs. (2) to (4).
In the above, Ci and Pi are the census livestock data and the predicted value for county i, respectively; represents the mean census value for all counties; and n gives the total number of counties.
2.5 Uncertainty evaluation
Uncertainty in our grazing intensity maps can stem from multiple sources, such as the constraints of cross-scale modeling and the intrinsic inaccuracies of the input data. To quantify these uncertainties, we utilized the Monte Carlo (MC) method, conducting 100 iterations of simulation. Subsequently, we evaluated uncertainty through the mean relative error (MRE) and assessed the model's robustness using the standard deviation (SD) following established methodologies (Yang et al., 2020; Alexander et al., 2017; McMillan et al., 2018). The definitions for these metrics are delineated in Eqs. (5) to (7).
In the above, xi are random samples, f(xi) is the function evaluated at xi, and n is the number of simulations. represents the mean value for all simulation maps.
3.1 Performances of models
Table 3 summarizes the efficiency of the five machine learning models used, with a consideration of all three accuracy evaluators of R2, MAE, and RMSE. It can be seen that the ET model performs the best, with its R2 exceeding 0.955 and with its MAE (0.081 SU ha−2) and RMSE (0.164 SU ha−2) being significantly lower than the values of RF, GB, KNN, and support vector machine (SVM) models. Figure 3 illustrates the correlation between the census livestock data and the livestock numbers predicted by the model for each county from 1990 to 2020. It is demonstrated that the ET-predicted data displayed a distribution pattern consistent with that of other models, but the scatter points of the ET model were more convergent in relation to the 1:1 diagonal line, indicating a superior fit compared to the other models. These comparisons suggest that the ET model possesses superior robustness and can, therefore, provide stable estimations of livestock intensity on the QTP.
Table 3Comparison of mapping accuracy for five machine learning models based on the same validation datasets.
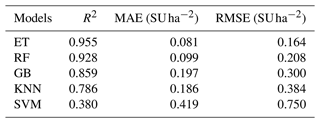
Note that the MAE and RMSE have been calculated using inverse logarithmic transformation, representing the actual values.
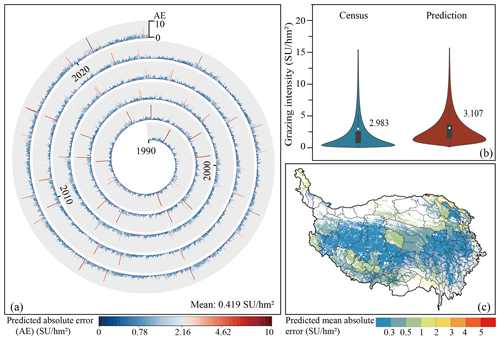
Figure 4Accuracy of the ET-predicted grazing-intensity results at a spatial resolution of 100 m from 1990 to 2020. (a) Absolute error (AE) between the predicted and the census data at the county scale from 1990 to 2020; (b) comparison of the predicted and census data of the whole QTP from 1990 to 2020; (c) spatial distribution of the mean absolute error (MAE) during 1990 to 2020 for each county.
Using the ET model, we projected the spatio-temporal distribution of grazing intensity across the QTP from 1990 to 2020 at a 100 m × 100 m resolution. To validate the accuracy of these predictive maps, we upscaled the pixel-level predictions to the county level and compared them against livestock census data (Fig. 4a and b). The results clearly show a high degree of consistency between the predicted livestock intensity and the county-level census data, especially in areas with lower grazing intensity (Fig. 4a and b). Specifically, while the mean census data indicated 2.983 SU ha−2 for livestock intensity, our county-level predictions yielded an average of 3.106 SU ha−2, with an MAE of 0.123 SU ha−2, an RMSE of 0.580 SU ha−2, and an R2 value of 0.669. Additionally, 76.31 % of the counties (n = 3814) exhibited data discrepancies of no more than 0.6 SU ha−2, and 91.74 % (n = 4585) had discrepancies under 1.0 SU ha−2. Regarding the spatial distribution, areas with data discrepancies of less than 0.3 SU ha−2 were predominantly located in the northwestern and southeastern regions of the QTP. In certain counties of the northeast and southwest, the variations were even below 1.0 SU ha−2 (Fig. 4c).
3.2 Evaluation of uncertainties
We have chosen the mean relative error (MRE) as a key metric for evaluating the simulation accuracy of grazing intensity within the QTP. Employing Monte Carlo simulations spanning the period from 1990 to 2020, our research findings demonstrate that the average MRE for grazing intensity across the QTP ranged between 6.84 % and 9.08 % (Fig. 5a). The spatial distribution of MRE indicates that the majority of the plateau exhibits low error margins. For example, in 2020, areas with an MRE of less than 5 % accounted for 35.86 % of the total grassland area, while those with an MRE below 10 % constituted 75.84 %. Only 3.38 % of the grasslands had an MRE exceeding 20 %, with these regions being primarily located in the southwestern portion of the QTP (Fig. 5b). Moreover, the robustness analysis suggests that the majority of regions within the QTP display relatively stable grazing-intensity trends. For instance, the overall standard deviation (SD) in 2020 was 0.059 SU ha−2, with the northwestern region demonstrating remarkable stability, reflected in an SD of less than 0.005 SU ha−2. Although some areas within the Yarlung Zangbo River basin and in the eastern part of Qinghai Province experienced higher variability, their SD was still maintained below 0.3 SU ha−2 (Fig. 5c).
3.3 Validation of the GDGI dataset
After employing the county-level livestock census as a benchmark for quality control, we obtained the annual Gridded Dataset of Grazing Intensity (GDGI) across the QTP, spanning 31 years from 1990 to 2020. We firstly confirmed the accuracy of the GDGI dataset based on 112 field grazing-intensity records at 68 sites (see Table S3 in the Supplement for details), which ranged from 0 to 5.61 sheep units per hectare (SU ha−2) and covered three main grasslands on the QTP: the alpine steppe (N = 62), alpine meadow (N = 46), and alpine desert steppe (N = 4). The GDGI dataset was assessed by undertaking a comparative accuracy assessment with the field grazing-intensity data (Fig. 6a). It can be seen that, in general, our dataset was highly consistent with the reference ground-truth validation data, with R2 = 0.804, MAE = 0.572 SU ha−2, and RMSE = 0.953 SU ha−2. Moreover, the absolute errors between the GDGI data and the field grazing-intensity data were relatively small, with more than half of the records having an error below 0.3 SU ha−2, 78.57 % having an error below 1.0 SU ha−2, and 89.29 % having an error below 1.5 SU ha−2 (Fig. 6b).
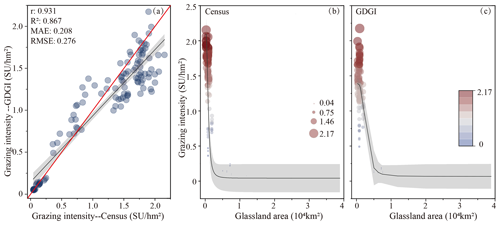
Figure 7Validation of the GDGI dataset using census livestock data at the township level: (a) linear fit of predicted number and census data, (b, c) logistic fit of grazing-intensity data and grassland area.
We further validated the precision of the GDGI dataset using the township-level livestock census data. Encouragingly, the evaluation results showed that the GDGI dataset demonstrates excellent performance at the township scale (Fig. 7a), with R2 of 0.867, MAE of 0.208 SU ha−2, and RMSE of 0.276 SU ha−2. In addition, similarly to the census data, the GDGI dataset indicated that some townships with few grasslands areas are still under high grazing pressure (Fig. 7b and c).
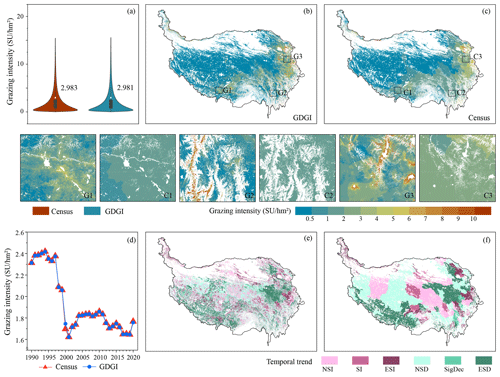
Figure 8Validation of the GDGI maps using the census grazing data from 1990 to 2020: (a) violin plot of the census data and the predicted value, (b, c) spatial distribution (in SU per pixel), (d) temporal change (in SU per year) (only including 124 counties with livestock census data), (e–f) spatial distribution of SU changes tested by Sen's slope and Mann–Kendall tests. Note that ESI stands for extremely significant increase (slope > 0 and p < 0.01), SI stands for significant increase (slope > 0 and p < 0.05), NSI stands for non-significant increase (slope > 0 and p > 0.05), ESD stands for extremely significant decrease (slope < 0 and p < 0.01), SigDec stands for significant decrease (slope < 0 and p < 0.05), and NSD stands for non-significant decrease (slope < 0 and p > 0.05).
3.4 Spatio-temporal variations in grazing intensity
In terms of the temporal trends of grazing intensity, overall, the GDGI dataset exhibited consistent trends with the livestock census data (Fig. 8d–f). Specifically, the census data indicated that the livestock numbers remained high and largely stable from 1990 to 1997, followed by a sharp decline from 1997 to 2001 and then a period of fluctuation post-2001, which was successfully captured by the GDGI dataset. Moreover, the spatial heterogeneity of grazing intensity within the counties over the QTP was also effectively reflected by the GDGI dataset, a characteristic not illustrated by the census dataset. For example, areas of high grazing intensity were concentrated in the northeastern and south-central regions of the plateau, mainly including the eastern part of Qinghai Province, the southwestern part of Gansu Province, the northwestern part of Sichuan Province, and the eastern region of the Tibet Autonomous Region (Fig. 8e and f).
Over the past 31 years, 63.95 % of the plateau's grassland showed a decreasing trend in grazing intensity, with 49.80 % showing significant decreases, primarily located in the eastern Sanjiangyuan area and the southwestern region of the QTP (Fig. 8e and f). Meanwhile, grazing intensity saw an increase in 36.05 % of the grassland, but most of these areas (60.16 %) did not reach the level of significance and were mainly distributed in the northeastern plateau (Fig. 8e and f).
4.1 Comparison with other grazing-intensity maps
To further assess the effectiveness and reliability of the developed GDGI dataset, the mapping results were juxtaposed with seven publicly available grazing-intensity maps covering the QTP (Table 4). It can be seen that, despite their public availability, these maps were lacking in both spatial and temporal resolutions when juxtaposed with the GDGI maps. Our analysis was extended to four openly accessible gridded livestock datasets, including GI-Sun (Sun et al., 2021), ALCC (Liu, 2021), GI-Meng (Meng et al., 2023) and GLW (Gilbert et al., 2018). A commonality among all five maps was the consistency of the spatial patterns of grazing intensity, with prevalent high and low intensities in the northeastern and northwestern regions, respectively (Fig. 9). However, these maps differed significantly in terms of accuracy. As the grazing intensity maps of GLW and ALCC were produced based on the livestock census data in 2001 and 2015, an accuracy comparison for the corresponding years was conducted among the five datasets at both the county and township scale. Observations at the county scale indicate that all four datasets, with the exception of GI-Sun, are largely in alignment with the county census data (Fig. 9b). When examined at the township scale, GI-Sun and GLW demonstrate the most significant discrepancies, with MRE values surpassing 68 %. ALCC and GI-Meng follow, recording MREs of 30.69 % and 38.80 %, respectively. Additionally, the GDGI shows the highest degree of accuracy in relation to the township census data, as indicated by the lowest MAE and RMSE values (Fig. 9c). Moreover, the GDGI dataset spanning 31 years (1990–2020) was earmarked as a more suitable choice for long-term studies in comparison to the other four datasets. Regarding spatial distribution, the overall patterns of these grazing maps are largely consistent, exhibiting higher density patterns in the southeast and lower in the northwest. However, notable discrepancies are still apparent in the finer details. In general, in terms of visually representing the spatial distribution of livestock, the GDGI maps exhibit the best performance.
Several potential factors may contribute to the improved accuracy of the GDGI. First, the livestock census data used in GDGI are more detailed, aiding in enhancing the accuracy of the estimation results. Specifically, GI-sun, ALCC, GI-Meng, and GDGI all use county-level livestock statistics to map grazing intensity, whereas GLW3 and GLW4 are based on provincial-level census data, which results in their accuracy lagging significantly behind the other four datasets (Nicolas et al., 2016; Sun et al., 2021). Second, after ruling out areas unsuitable for grazing, we estimate grazing intensity by dividing the livestock numbers from statistical data by the area of suitable grazing regions. However, these maps differ in terms of their definitions of suitable grazing areas. In this study, as with the GI-sun and GI-Meng maps, we considered grazing to occur only on grasslands and further excluded unsuitable areas such as high elevations and steep slopes. This kind of definition is clearly more reasonable than the GLW series, which removed only waterbodies, urban core areas, and protected areas with relatively tight regulations regarding human activity (McSherry and Ritchie, 2013; He et al., 2022). However, the GI-Meng dataset considers the core areas of protected areas to be grazing-free regions; this does not match the actual situation on the QTP (Jiang et al., 2023; T. Li et al., 2022; Zhao et al., 2020). Those different thresholds for the definition of suitable grazing areas account for the fact that each map has different theoretical grazing regions. Third, the selection of models and environmental factors may also be a significant contributing factor, leading to variations in predictive accuracy. For instance, different algorithms were employed, including linear regression and machine learning methods (Nicolas et al., 2016; Li et al., 2021). Additionally, the environmental factors considered varied; specifically, the GDGI utilized the human-induced net primary productivity (HNPP) to represent grasslands, whereas other maps relied on net primary productivity (NPP) and the normalized difference vegetation index (NDVI) (Allred et al., 2013; Sun et al., 2021; Meng et al., 2023).
4.2 Spatial heterogeneity of grazing intensities
In general, the multiyear average grazing intensity on the QTP increased from west to east during 1990 to 2020, with broad spatial heterogeneity (Fig. 8). Highest grazing intensity was found mainly in the northeastern and south-central regions of the Plateau (mostly higher than 5.0 SU ha−2), while they were lowest in the northwest (mostly less than 1.0 SU ha−2). Over the past 31 years, the average grazing intensity decreased across most of the Plateau, but 36.05 % of the entire QTP grassland still encountered a continuous grazing-intensity increase, especially in the northeastern regions (Fig. 8).
The spatial heterogeneity of grazing intensities on the QTP may be attributed to the following reasons. First, complex geographic and climatic conditions on the QTP determine the heterogeneity of grasslands, which in turn affects livestock distribution (Wang et al., 2018; Wei et al., 2022). In general, the grazing-intensity patterns shown in the GDGI maps are basically consistent with the stocking-rate threshold patterns in the QTP grasslands, which both decreased from east to west (Q. Zhu et al., 2023). This phenomenon partially reflects the heterogeneity of the grasslands as the alpine meadows and the steppes are mainly distributed in the east and the west, respectively. Second, the dynamics of socio-economic development are obviously another important factor determining grazing intensity. In areas falling behind in terms of the socio-economic indicators, herders prefer to increase livestock in an effort to improve household incomes, leading to greater pressure on grasslands in these regions (Fang and Wu, 2022). In addition, the perceived increases in human population also resulted in the considerably increased need for more livestock (Wei et al., 2022).
The grazing-intensity dynamics across the QTP are partly reflective of the impacts of various management policies that have been implemented over distinct periods. For example, a significant increase in grazing intensity on the QTP was observed in the early 1990s, potentially a direct result of the introduction of the household contract responsibility system. Moreover, the grazing intensity experienced a pronounced decline from 1997 to 2001, as illustrated in Fig. 8d, indicative of the adverse effects of natural disasters. Notably, the severe snowstorms that struck Naqu in the central QTP during 1997–1998 are documented to have caused the mortality of over 820 000 livestock (Ye et al., 2020). Figure 8d further delineates a considerable upsurge in grazing intensity on the QTP between 2000 and 2010, aligning with the trends reported by Sun et al. (2021) and Li et al. (2021). This observed increase may be attributed to a rebound in grazing activity following the aforementioned natural disasters. In addition, Fig. 8d indicates a sustained decrease in grazing intensity post-2010 across the plateau, which can be predominantly ascribed to the implementation of extensive ecological conservation projects.
4.3 Implications for grazing management
Nearly half of the grasslands on the QTP have been reported to be degraded over the past 4 decades (Wang et al., 2018; Dong et al., 2020), with some reports even indicating that the degraded grassland has reached 90 % (Wang et al., 2021). It is widely recognized that overgrazing is the predominant and most pervasive unsustainable human activity continuing to drive grassland degradation on the QTP (Wang et al., 2018; Chen et al., 2019). Generally, these degraded grasslands on the QTP can be effectively restored by adaptive management (Wang et al., 2022). However, better management of grasslands requires a deeper understanding of the anthropogenic activities, which still remains an important challenge and can be effectively addressed by the GDGI dataset.
According to the GDGI maps generated in this study, high-intensity grazing activities are mainly concentrated in the northeastern and south-central parts of the QTP, with the grazing intensity in some areas even being nearly more than 10 times the average value of the entire plateau (Fig. 6b), and have exceeded the stocking-rate threshold of these grasslands (Q. Zhu et al., 2023). Population growth and the related increasing livelihood demands constitute one of the main reasons for this increase. To meet daily needs and to enhance household incomes, the herders have endeavored to increase livestock, thereby intensifying grazing pressures on the grasslands over the QTP (Fang and Wu, 2022; Abu Hammad and Tumeizi, 2012). Although the current average grazing intensity in the northwestern QTP (around 1.0 SU ha−2) is below the average stocking-rate threshold (around 1.5 SU ha−2) (Q. Zhu et al., 2023), the grassland management should still be given adequate attention. The northwestern QTP, the driest area on the plateau with low stocking-rate thresholds, has seen an increase in grazing intensity in recent years. Nevertheless, it must be noted that the stocking-rate threshold may exceed the carrying capacity because it is predicted to lead to extreme grassland degradation (Q. Zhu et al., 2023). The GDGI dataset also showed a similar pattern between the grazing-intensity data and the WorldPop data near the built-up areas, indicating higher grazing intensity around settlements compared to other regions on the QTP. In addition, the GDGI dataset also indicates that, from 1990 to 2020, although the grazing intensity of the Plateau has generally decreased, the hotspot areas for grazing activities have remained almost unchanged. This implies that these regions should be the focus of adaptive grassland management to effectively prevent grassland degradation, mainly based on the grass–livestock balance which varies with time and space.
Encouragingly, the GDGI dataset shows that the grazing intensity for two-thirds of the entire QTP grassland has decreased over the past 31 years, which is also consistent with the findings of other studies (Sun et al., 2021; Li et al., 2021). Recent decades of biodiversity protection; active restoration projects; and management measures, such as nature reserves, grazing exclusion, partial grazing bans, and fencing enclosure, are believed to have driven these decreases (Deng et al., 2017; Li and Bennett, 2019). In addition, most grassland in eastern Sanjiangyuan, mid-eastern Changtang, and the northern foothills of the Himalayas showed a significant decrease in grazing intensity (Fig. 6e), indicating the importance of protected areas in preventing overstocking and grassland degradation. Meanwhile, the GDGI maps also show that the grazing density varies greatly among protected areas, possibly owing to the difference in policy implementation. For instance, it can be seen from the GDGI maps that grazing intensity is increasing in some protected areas, especially in several wetland nature reserves on the Zoigê Plateau (Fig. 6e). Moreover, the average grazing intensity in all nature reserves on the QTP has increased overall from 1990 to 2020, although their increase rate is much lower than that of the non-protected areas (0.0125 SU ha−2 ⋅ 10a vs. 0.0304 SU ha−2 ⋅ 10a), which implies that grassland management in protected areas still needs to be strengthened on the QTP.
The grazing initiatives in alignment with the Sustainable Development Goals (SDGs) on the QTP can benefit from the GDGI dataset. Firstly, determining a reasonable stocking rate is vital to prevent overstocking of the pastures, which will possibly induce extreme grassland degradation (Q. Zhu et al., 2023). Stocking-rate determination can be optimized by using our grazing-intensity maps and the stocking-rate threshold maps of the QTP. Secondly, the GDGI maps can contribute to strategic placement of fence, which is a common practice adopted to prevent grassland degradation on the QTP. Building fences in areas with high grazing intensity and exceeding the carrying capacity can improve the effectiveness of fence construction (Zhou et al., 2023; Zhang et al., 2023). Thirdly, the GDGI dataset can provide a solid support for promoting effective nature reserve management, with nature reserves covering, in total, nearly one-third of the entire QTP. For example, the GDGI maps showed that grazing activities still exist in most nature reserves on the Plateau, although most of them have significantly lower grazing intensities compared with their adjacent non-protected areas. By using the GDGI maps, the conflict between ecological protection and grazing activities in nature reserves can be alleviated. Finally, our grazing-intensity maps can act as a basic dataset to support other grassland-related policies. Currently, these policies on the QTP often adopt a one-size-fits-all approach to determine the carrying capacity and to carry out ecological compensation, which may lead to overstocking or an unfair financial distribution (Wang et al., 2022). The grassland management strategies balancing carrying capacity and stocking rates are more likely to result in optimal management choices for policymakers and stakeholders, and our GDGI maps can contribute to this decision-making processes.
4.4 Uncertainties and limitations
Although this study has collected datasets that are as reliable as possible, users of the GDGI products should be cognizant of the inherent uncertainties and limitations within these datasets. Notably, the mean relative error of the GDGI dataset spanning 1990 to 2020 was recorded at 4.2 % (Fig. 4a), calculated from the average errors across 182 counties within the QTP that had accessible livestock census data. Furthermore, approximately 8.26 % of grassland areas exhibited a relative error exceeding 1.0 SU ha−2 (Fig. 4b). Such discrepancies arise from several limitations that were subsequently propagated to the final grazing-intensity maps, thereby contributing to the dataset's overall uncertainties.
Firstly, the estimations of grazing intensities were fundamentally conservative, primarily due to the lack of comprehensive input data. Livestock numbers, derived from year-end data at the county level, inadvertently led to underestimations of grazing intensity by not accounting for livestock off-take rates. Likewise, the evaluation focused solely on livestock grazing intensity, excluding wild herbivores and forage-dependent livestock, which potentially results in an underestimation of actual grazing pressures on the QTP. Additionally, despite identifying seven main factors influencing livestock distribution, the study did not encompass all potential factors, such as fencing, forage availability, road proximity, and seasonal transformation in grazing practices. Moreover, to align with county-scale livestock census data, we averaged the environmental factors at the county scale. Although this approach has been widely used based on the hypothesis that a consistent causal relationship between livestock intensity and environmental factors persists across various scales (Robinson et al., 2014; Nicolas et al., 2016; Li et al., 2021; Meng et al., 2023), this might oversimplify the intricate dynamics between grazing intensity and lead to a certain degree of estimation inaccuracies. In addition, the reliance on linear extrapolation to supplementary missing gridded 100 m population density data from 1990–1999 introduced further uncertainties due to the limited resolution (1 km) and interval (5 year) of the ChinaPop dataset.
Secondly, the modeling process for mapping grazing intensity also suffered from several challenges. Specifically, this study adopted the FAO's assumption that the relationship between environmental factors and livestock intensity is uniform across both administrative and pixel levels. However, it is unlikely that these relationships are entirely consistent across scales, and the county-level model's approach inevitably smooths spatial details, potentially reducing the precision of the data. Furthermore, the ET model was trained with a limited sample size of 4998 and was applied to a vast area consisting of 150 million pixels, which could compromise the model's accuracy. In addition, despite the ET model's design being intended to reduce overfitting risks by using randomly selected features and partitioning decisions, the potential for overfitting effects still remains, particularly when faced with a high number of output classes or insufficient sample sizes (Geurts et al., 2006; Galelli and Castelletti, 2013). In fact, this limitation was evident in this study as the generalization capability of the ET model was restricted by the disparity between the number of training samples and the total number of pixels, leading to predictions that often exceed actual livestock census (Fig. 4a).
Thirdly, our methodological framework for high-resolution gridded grazing dataset mapping was developed based on the assumption that all grasslands were accessible to livestock. However, in reality, the amount of available grassland was less due to fencing and grazing bans on the QTP (Zhan et al., 2023). Moreover, transhumant herders generally follow a seasonal calendar for summer pastures and winter pastures on the QTP. However, we did not consider these seasonal movements due to data limitations, which further restrict the analysis of seasonal livestock distribution patterns (Kolluru et al., 2023). Additionally, the model's reliance on the human population as a proxy for livestock locations overlooked the possibility of high grazing intensity in areas with low human populations on the QTP, particularly in regions designated for summer pastures.
Finally, it is important to note that gathering livestock census data in the Qinghai–Tibet Plateau presents significant challenges, leading to a scarcity of livestock validation data in this study, particularly at the township and pixel scales. This limitation may, to some extent, impact the reliability of the grazing-intensity data we have presented.
In summary, all these limitations associated with input data, the modeling process, and the methodological framework collectively contribute to the uncertainties and reduce the accuracy of the GDGI maps. We henceforth recommend that future research should aim to incorporate more detailed data, consider additional influential factors, enhance key datasets' time series consistency, and refine the methodological framework to improve the accuracy of grazing-intensity mapping.
The annual gridded grazing-intensity maps of the QTP spanning from 1990 to 2020 are accessible at the following link: https://doi.org/10.5281/zenodo.10851119 (Zhou et al., 2024). Each map is catalogued by year and recorded in GeoTIFF format, with values represented in . These datasets, with a spatial resolution of 100 m and annual temporal resolution, utilize the WGS 1984 Albers geographic coordinate system. To streamline data transfer and download processes, the comprehensive 31-year dataset has been compressed into a ZIP file, readily available for download and compatible with geographic information system (GIS) software for viewing.
In this study, we introduce a framework utilizing ET machine learning algorithms to achieve fine-scale livestock spatialization, subsequently generating the GDGI dataset across the QTP. The GDGI has a spatial resolution of 100 m and spans 31 years, from 1990 to 2020. It is consistent with county livestock census data of the QTP, and the accuracy evaluations at both the pixel level and township level underscore the outstanding reliability and applicability of the GDGI dataset, which can successfully capture the spatial heterogeneity and variations in grazing intensities in greater detail. Moreover, comparisons between the GDGI dataset and other existing grazing map products further proved the robustness and efficiency of our dataset and demonstrated the validity of the proposed framework in the research of livestock spatialization. Nonetheless, it is imperative for data users to recognize that the GDGI may still contain inherent uncertainties. Our Monte Carlo simulations have estimated the average MRE for grazing intensity across the QTP to vary between 6.84 % and 9.08 %. The GDGI dataset, as presented in this study, can enhance the understanding of grazing activities on the QTP. This, in turn, can aid in the rational utilization of grasslands and facilitate the implementation of informed and sustainable management practices.
For gridded datasets that are not directly available or that do not meet the spatio-temporal resolution requirements – such as those pertaining to population density, temperature, precipitation, and HNPP – we have delineated the processing or creation procedures in the Supplement. The supplement related to this article is available online at: https://doi.org/10.5194/essd-16-5171-2024-supplement.
TL conceived the research; JZ and JN performed the analyses and wrote the first draft of the paper; NW and TL reviewed and edited the paper before submission. All the authors made substantial contributions to the discussion of content.
The contact author has declared that none of the authors has any competing interests.
Publisher's note: Copernicus Publications remains neutral with regard to jurisdictional claims made in the text, published maps, institutional affiliations, or any other geographical representation in this paper. While Copernicus Publications makes every effort to include appropriate place names, the final responsibility lies with the authors.
. We would like to thank the Bureau of Statistics of each county across the QTP for providing the census livestock data.
This research was supported by the Second Tibetan Plateau Scientific Expedition and Research Program (STEP), the Ministry of Science and Technology of the People's Republic of China (grant no. 2019QZKK0402), and the National Natural Science Foundation of China (grant no. 42071238).
This paper was edited by Peng Zhu and reviewed by five anonymous referees.
Abu Hammad, A. and Tumeizi, A.: Land degradation: socioeconomic and environmental causes and consequences in the eastern Mediterranean, Land. Degrad. Dev., 23, 216–226, https://doi.org/10.1002/ldr.1069, 2012.
Ahmad, M. W., Reynolds, J., and Rezgui, Y.: Predictive modelling for solar thermal energy systems: A comparison of support vector regression, random forest, extra trees and regression trees, J. Clean. Prod., 203, 810–821, https://doi.org/10.1016/j.jclepro.2018.08.207, 2018.
Alexander, P., Prestele, R., Verburg, P. H., Arneth, A., Baranzelli, C., Batista e Silva, F., Brown, C., Butler, A., Calvin, K., and Dendoncker, N.: Assessing uncertainties in land cover projections, Glob. Change Biol., 23, 767–781, 2017.
Allred, B. W., Fuhlendorf, S. D., Hovick, T. J., Dwayne Elmore, R., Engle, D. M., and Joern, A.: Conservation implications of native and introduced ungulates in a changing climate, Glob. Change Biol., 19, 1875–1883, https://doi.org/10.1111/gcb.12183, 2013.
Breiman, L.: Random Forests, Mach. Learn., 45, 5–32, https://doi.org/10.1023/A:1010933404324, 2001.
Cai, Y. J., Wang, X. D., Tian, L. L., Zhao, H., Lu, X. Y., and Yan, Y.: The impact of excretal returns from yak and Tibetan sheep dung on nitrous oxide emissions in an alpine steppe on the Qinghai-Tibetan Plateau, Soil Biol. Biochem., 76, 90–99, https://doi.org/10.1016/j.soilbio.2014.05.008, 2014.
Chang, J. F., Ciais, P., Gasser, T., Smith, P., Herrero, M., Havlík, P., Obersteiner, M., Guenet, B., Goll, D. S., Li, W., Naipal, V., Peng, S. S., Qiu, C. J., Tian, H. Q., Viovy, N., Yue, C., and Zhu, D.: Climate warming from managed grasslands cancels the cooling effect of carbon sinks in sparsely grazed and natural grasslands, Nat. Commun., 12, 118, https://doi.org/10.1038/s41467-020-20406-7, 2021.
Chen, Y. Z., Ju, W. M., Mu, S. J., Fei, X. R., Cheng, Y., Propastin, P., Zhou, W., Liao, C. J., Chen, L. X., Tang, R. J., Qi, J. G., Li, J. L., and Ruan, H. H.: Explicit Representation of Grazing Activity in a Diagnostic Terrestrial Model: A Data-Process Combined Scheme, J. Adv. Model. Earth Sy., 11, 957–978, https://doi.org/10.1029/2018ms001352, 2019.
Cortes, C. and Vapnik, V.: Support-vector networks, Mach. Learn., 20, 273–297, https://doi.org/10.1007/BF00994018, 1995.
Cover, T. and Hart, P.: Nearest neighbor pattern classification, IEEE T. Inform. Theory, 13, 21–27, https://doi.org/10.1109/TIT.1967.1053964, 1967.
Dara, A., Baumann, M., Freitag, M., Hölzel, N., Hostert, P., Kamp, J., Müller, D., Prishchepov, A. V., and Kuemmerle, T.: Annual Landsat time series reveal post-Soviet changes in grazing pressure, Remote Sens. Environ., 239, 111667, https://doi.org/10.1016/j.rse.2020.111667, 2020.
Deng, L., Zhou, S. G., Wu, P., Gao, L., and Chang, X.: Effects of grazing exclusion on carbon sequestration in China's grassland, Earth-Sci. Rev., 173, 84–95, https://doi.org/10.1016/j.earscirev.2017.08.008, 2017.
Dong, S. K., Shang, Z. H., Gao, J. X., and Boone, R. B.: Enhancing sustainability of grassland ecosystems through ecological restoration and grazing management in an era of climate change on Qinghai-Tibetan Plateau, Agr. Ecosyst. Environ., 287, 106684, https://doi.org/10.1016/j.agee.2019.106684, 2020.
Fang, X. N. and Wu, J. G.: Causes of overgrazing in Inner Mongolian grasslands: Searching for deep leverage points of intervention, Ecol. Soc., 27, 8, https://doi.org/10.5751/es-12878-270108, 2022.
Feng, R. Z., Long, R. J., Shang, Z. H., Ma, Y. S., Dong, S. K., and Wang, Y. L.: Establishment of Elymus natans improves soil quality of a heavily degraded alpine meadow in Qinghai-Tibetan Plateau, China, Plant Soil, 327, 403–411, https://doi.org/10.1007/s11104-009-0065-3, 2009.
Fetzel, T., Havlik, P., Herrero, M., Kaplan, J. O., Kastner, T., Kroisleitner, C., Rolinski, S., Searchinger, T., Van Bodegom, P. M., Wirsenius, S., and Erb, K. H.: Quantification of uncertainties in global grazing systems assessment, Global Biogeochem. Cy., 31, 1089–1102, https://doi.org/10.1002/2016gb005601, 2017.
Friedman, J. H.: Greedy function approximation: a gradient boosting machine, Ann. Stat., 29, 1189–1232, https://doi.org/10.1214/aos/1013203451, 2001.
Galelli, S. and Castelletti, A.: Assessing the predictive capability of randomized tree-based ensembles in streamflow modelling, Hydrol. Earth Syst. Sci., 17, 2669–2684, https://doi.org/10.5194/hess-17-2669-2013, 2013.
García, R., Aguilar, J., Toro, M., Pinto, A., and Rodríguez, P.: A systematic literature review on the use of machine learning in precision livestock farming, Comput. Electron. Agr., 179, 105826, https://doi.org/10.1016/j.compag.2020.105826, 2020.
García Ruiz, J. M., Tomás Faci, G., Diarte Blasco, P., Montes, L., Domingo, R., Sebastián, M., Lasanta, T., González Sampériz, P., López Moreno, J. I., Arnáez, J., and Beguería, S.: Transhumance and long-term deforestation in the subalpine belt of the central Spanish Pyrenees: An interdisciplinary approach, Catena, 195, 104744, https://doi.org/10.1016/j.catena.2020.104744, 2020.
Garrett, R. D., Koh, I., Lambin, E. F., le Polain de Waroux, Y., Kastens, J. H., and Brown, J. C.: Intensification in agriculture-forest frontiers: Land use responses to development and conservation policies in Brazil, Global Environ. Chang., 53, 233–243, https://doi.org/10.1016/j.gloenvcha.2018.09.011, 2018.
Geurts, P., Ernst, D., and Wehenkel, L.: Extremely randomized trees, Mach. Learn., 63, 3–42, https://doi.org/10.1007/s10994-006-6226-1, 2006.
Gilbert, M., Nicolas, G., Cinardi, G., Van Boeckel, T. P., Vanwambeke, S. O., Wint, G. R. W., and Robinson, T. P.: Global distribution data for cattle, buffaloes, horses, sheep, goats, pigs, chickens and ducks in 2010, Sci. Data, 5, 180227, https://doi.org/10.1038/sdata.2018.227, 2018.
Godfray, H. C. J., Aveyard, P., Garnett, T., Hall, J. W., Key, T. J., Lorimer, J., Pierrehumbert, R. T., Scarborough, P., Springmann, M., and Jebb, S. A.: Meat consumption, health, and the environment, Science, 361, 243, https://doi.org/10.1126/science.aam5324, 2018.
Guo, Z. L., Li, Z., and Cui, G. F.: Effectiveness of national nature reserve network in representing natural vegetation in mainland China, Biodivers. Conserv., 24, 2735–2750, https://doi.org/10.1007/s10531-015-0959-8, 2015.
Han, Y. H., Dong, S. K., Zhao, Z. Z., Sha, W., Li, S., Shen, H., Xiao, J. N., Zhang, J., Wu, X. Y., Jiang, X. M., Zhao, J. B., Liu, S. L., Dong, Q. M., Zhou, H. K., and Yeomans, J. C.: Response of soil nutrients and stoichiometry to elevated nitrogen deposition in alpine grassland on the Qinghai-Tibetan Plateau, Geoderma, 343, 263–268, https://doi.org/10.1016/j.geoderma.2018.12.050, 2019.
He, M., Pan, Y. H., Zhou, G. Y., Barry, K. E., Fu, Y. L., and Zhou, X. H.: Grazing and global change factors differentially affect biodiversity–ecosystem functioning relationships in grassland ecosystems, Glob. Change Biol., 28, 5492–5504, https://doi.org/10.1111/gcb.16305, 2022.
Heddam, S., Ptak, M., and Zhu, S. L.: Modelling of daily lake surface water temperature from air temperature: Extremely randomized trees (ERT) versus Air2Water, MARS, M5Tree, RF and MLPNN, J. Hydrol., 588, 125130, https://doi.org/10.1016/j.jhydrol.2020.125130, 2020.
Hu, Y., Cheng, H., and Tao, S.: Environmental and human health challenges of industrial livestock and poultry farming in China and their mitigation, Environ. Int., 107, 111–130, https://doi.org/10.1016/j.envint.2017.07.003, 2017.
Humpenöder, F., Bodirsky, B. L., Weindl, I., Lotze Campen, H., Linder, T., and Popp, A.: Projected environmental benefits of replacing beef with microbial protein, Nature, 605, 90–96, https://doi.org/10.1038/s41586-022-04629-w, 2022.
Jiang, M. J., Zhao, X. F., Wang, R., Yin, L., and Zhang, B. L.: Assessment of Conservation Effectiveness of the Qinghai–Tibet Plateau Nature Reserves from a Human Footprint Perspective with Global Lessons, Land, 12, 869, https://doi.org/10.3390/land12040869, 2023.
Kolluru, V., John, R., Saraf, S., Chen, J. Q., Hankerson, B., Robinson, S., Kussainova, M., and Jain, K.: Gridded livestock density database and spatial trends for Kazakhstan, Sci. Data, 10, 839, https://doi.org/10.1038/s41597-023-02736-5, 2023.
Kumar, P., Abubakar, A. A., Verma, A. K., Umaraw, P., Adewale Ahmed, M., Mehta, N., Nizam Hayat, M., Kaka, U., and Sazili, A. Q.: New insights in improving sustainability in meat production: opportunities and challenges, Crit. Rev. Food Sci., 63, 11830–11858, https://doi.org/10.1080/10408398.2022.2096562, 2022.
Li, M. Q., Liu, S. L., Wang, F. F., Liu, H., Liu, Y. X., and Wang, Q. B.: Cost-benefit analysis of ecological restoration based on land use scenario simulation and ecosystem service on the Qinghai-Tibet Plateau, Glob. Ecol. Conserv., 34, e02006, https://doi.org/10.1016/j.gecco.2022.e02006, 2022.
Li, P. and Bennett, J.: Sci. Total. Environ., Understanding herders' stocking rate decisions in response to policy initiatives, Sci. Total Environ., 672, 141–149, https://doi.org/10.1016/j.scitotenv.2019.03.407, 2019.
Li, Q., Zhang, C. L., Shen, Y. P., Jia, W. R., and Li, J.: Quantitative assessment of the relative roles of climate change and human activities in desertification processes on the Qinghai-Tibet Plateau based on net primary productivity, Catena, 147, 789–796, https://doi.org/10.1016/j.catena.2016.09.005, 2016.
Li, S., Wu, J., Gong, J., and Li, S.: Human footprint in Tibet: Assessing the spatial layout and effectiveness of nature reserves, Sci. Total Environ., 621, 18–29, https://doi.org/10.1016/j.scitotenv.2017.11.216, 2018.
Li, T., Cai, S. H., Singh, R. K., Cui, L. Z., Fava, F., Tang, L., Xu, Z. H., Li, C. J., Cui, X. Y., Du, J. Q., Hao, Y. B., Liu, Y. X., and Wang, Y. F.: Livelihood resilience in pastoral communities: Methodological and field insights from Qinghai-Tibetan Plateau, Sci. Total Environ., 838, 155960, https://doi.org/10.1016/j.scitotenv.2022.155960, 2022.
Li, X. H., Hou, J. L., and Huang, C. L.: High-Resolution Gridded Livestock Projection for Western China Based on Machine Learning, Remote Sens.-Basel, 13, 5038, https://doi.org/10.3390/rs13245038, 2021.
Lin, G. C., Lin, A. J., and Gu, D. L.: Using support vector regression and K-nearest neighbors for short-term traffic flow prediction based on maximal information coefficient, Inform. Sciences, 608, 517–531, https://doi.org/10.1016/j.ins.2022.06.090, 2022.
Liu, B. T.: Actual livestock carrying capacity estimation product in Qinghai-Tibet Plateau (2000-2019), National Tibetan Plateau Data Center, [dataset], https://doi.org/10.11888/Ecolo.tpdc.271513, 2021.
Long, S. J., Wei, X. L., Zhang, F., Zhang, R. H., Xu, J., Wu, K., Li, Q. Q., and Li, W. W.: Estimating daily ground-level NO2 concentrations over China based on TROPOMI observations and machine learning approach, Atmos. Environ., 289, 119310, https://doi.org/10.1016/j.atmosenv.2022.119310, 2022.
Luo, J. F., Hoogendoorn, C., van der Weerden, T., Saggar, S., de Klein, C., Giltrap, D., Rollo, M., and Rys, G.: Nitrous oxide emissions from grazed hill land in New Zealand, Agr. Ecosyst. Environ., 181, 58–68, https://doi.org/10.1016/j.agee.2013.09.020, 2013.
Ma, C., Xie, Y., Duan, H., Wang, X., Bie, Q., Guo, Z., He, L., and Qin, W.: Spatial quantification method of grassland utilization intensity on the Qinghai-Tibetan Plateau: A case study on the Selinco basin, J. Environ. Manage., 302, 114073, https://doi.org/10.1016/j.jenvman.2021.114073, 2022.
Mack, G., Walter, T., and Flury, C.: Seasonal alpine grazing trends in Switzerland: Economic importance and impact on biotic communities, Environ. Sci. Policy, 32, 48–57, https://doi.org/10.1016/j.envsci.2013.01.019, 2013.
Martinuzzi, S., Radeloff, V. C., Pastur, G. M., Rosas, Y. M., Lizarraga, L., Politi, N., Rivera, L., Herrera, A. H., Silveira, E. M. O., Olah, A., and Pidgeon, A. M.: Informing forest conservation planning with detailed human footprint data for Argentina, Glob. Ecol. Conserv., 31, e01787, https://doi.org/10.1016/j.gecco.2021.e01787, 2021.
McMillan, H. K., Westerberg, I. K., and Krueger, T.: Hydrological data uncertainty and its implications, WIREs Water, 5, e1319, https://doi.org/10.1002/wat2.1319, 2018.
McSherry, M. E. and Ritchie, M. E.: Effects of grazing on grassland soil carbon: a global review, Glob. Change Biol., 19, 1347–1357, https://doi.org/10.1111/gcb.12144, 2013.
Meng, N., Wang, L. J., Qi, W. C., Dai, X. H., Li, Z. Z., Yang, Y. Z., Li, R. N., Ma, J. F., and Zheng, H.: A high-resolution gridded grazing dataset of grassland ecosystem on the Qinghai-Tibet Plateau in 1982–2015, Sci. Data, 10, 68, https://doi.org/10.1038/s41597-023-01970-1, 2023.
Miao, L. J., Sun, Z. L., Ren, Y. J., Schierhorn, F., and Müller, D.: Grassland greening on the Mongolian Plateau despite higher grazing intensity, Land. Degrad. Dev., 32, 792–802, https://doi.org/10.1002/ldr.3767, 2020.
Minoofar, A., Gholami, A., Eslami, S., Hajizadeh, A., Gholami, A., Zandi, M., Ameri, M., and Kazem, H. A.: Renewable energy system opportunities: A sustainable solution toward cleaner production and reducing carbon footprint of large-scale dairy farms, Energ. Convers. Manage., 293, 117554, https://doi.org/10.1016/j.enconman.2023.117554, 2023.
Mulligan, M., van Soesbergen, A., Hole, D. G., Brooks, T. M., Burke, S., and Hutton, J.: Mapping nature's contribution to SDG 6 and implications for other SDGs at policy relevant scales, Remote Sens. Environ., 239, 111671, https://doi.org/10.1016/j.rse.2020.111671, 2020.
Muloi, D. M., Wee, B. A., McClean, D. M. H., Ward, M. J., Pankhurst, L., Phan, H., Ivens, A. C., Kivali, V., Kiyong'a, A., Ndinda, C., Gitahi, N., Ouko, T., Hassell, J. M., Imboma, T., Akoko, J., Murungi, M. K., Njoroge, S. M., Muinde, P., Nakamura, Y., Alumasa, L., Furmaga, E., Kaitho, T., Öhgren, E. M., Amanya, F., Ogendo, A., Wilson, D. J., Bettridge, J. M., Kiiru, J., Kyobutungi, C., Tacoli, C., Kang'ethe, E. K., Davila, J. D., Kariuki, S., Robinson, T. P., Rushton, J., Woolhouse, M. E. J., and Fèvre, E. M.: Population genomics of Escherichia coli in livestock-keeping households across a rapidly developing urban landscape, Nat. Microbiol., 7, 581–589, https://doi.org/10.1038/s41564-022-01079-y, 2022.
Neumann, K., Elbersen, B. S., Verburg, P. H., Staritsky, I., Pérez-Soba, M., de Vries, W., and Rienks, W. A.: Modelling the spatial distribution of livestock in Europe, Landscape Ecol., 24, 1207–1222, https://doi.org/10.1007/s10980-009-9357-5, 2009.
Nicolas, G., Robinson, T. P., Wint, G. R., Conchedda, G., Cinardi, G., and Gilbert, M.: Using Random Forest to Improve the Downscaling of Global Livestock Census Data, PLOS ONE, 11, e0150424, https://doi.org/10.1371/journal.pone.0150424, 2016.
O'Neill, D. W. and Abson, D. J.: To settle or protect? A global analysis of net primary production in parks and urban areas, Ecol. Econ., 69, 319–327, https://doi.org/10.1016/j.ecolecon.2009.08.028, 2009.
Pan, Y. J., Chen, S. Y., Qiao, F. X., Ukkusuri, S. V., and Tang, K.: Estimation of real-driving emissions for buses fueled with liquefied natural gas based on gradient boosted regression trees, Sci. Total Environ., 660, 741–750, https://doi.org/10.1016/j.scitotenv.2019.01.054, 2019.
Petz, K., Alkemade, R., Bakkenes, M., Schulp, C. J. E., van der Velde, M., and Leemans, R.: Mapping and modelling trade-offs and synergies between grazing intensity and ecosystem services in rangelands using global-scale datasets and models, Global Environ. Chang., 29, 223–234, https://doi.org/10.1016/j.gloenvcha.2014.08.007, 2014.
Pozo, R. A., Cusack, J. J., Acebes, P., Malo, J. E., Traba, J., Iranzo, E. C., Morris-Trainor, Z., Minderman, J., Bunnefeld, N., Radic-Schilling, S., Moraga, C. A., Arriagada, R., and Corti, P.: Reconciling livestock production and wild herbivore conservation: challenges and opportunities, Trends Ecol. Evol., 36, 750–761, https://doi.org/10.1016/j.tree.2021.05.002, 2021.
Prosser, D. J., Wu, J., Ellis, E. C., Gale, F., Van Boeckel, T. P., Wint, W., Robinson, T., Xiao, X., and Gilbert, M.: Modelling the distribution of chickens, ducks, and geese in China, Agr. Ecosyst. Environ., 141, 381–389, https://doi.org/10.1016/j.agee.2011.04.002, 2011.
Robinson, T. P., Wint, G. R., Conchedda, G., Van Boeckel, T. P., Ercoli, V., Palamara, E., Cinardi, G., D'Aietti, L., Hay, S. I., and Gilbert, M.: Mapping the global distribution of livestock, PLOS ONE, 9, e96084, https://doi.org/10.1371/journal.pone.0096084, 2014.
Rokach, L.: Decision forest: Twenty years of research, Inform. Fusion, 27, 111–125, https://doi.org/10.1016/j.inffus.2015.06.005, 2016.
Shakoor, A., Shakoor, S., Rehman, A., Ashraf, F., Abdullah, M., Shahzad, S. M., Farooq, T. H., Ashraf, M., Manzoor, M. A., Altaf, M. M., and Altaf, M. A.: Effect of animal manure, crop type, climate zone, and soil attributes on greenhouse gas emissions from agricultural soils-A global meta-analysis, J. Clean. Prod., 278, 124019, https://doi.org/10.1016/j.jclepro.2020.124019, 2021.
Sun, J., Liu, M., Fu, B. J., Kemp, D., Zhao, W. W., Liu, G. H., Han, G. D., Wilkes, A., Lu, X. Y., Chen, Y. C., Cheng, G. W., Zhou, T. C., Hou, G., Zhan, T. Y., Peng, F., Shang, H., Xu, M., Shi, P. L., He, Y. T., Li, M., Wang, J. N., Tsunekawa, A., Zhou, H. K., Liu, Y., Li, Y. R., and Liu, S. L.: Reconsidering the efficiency of grazing exclusion using fences on the Tibetan Plateau, Sci. Bull., 65, 1405–1414, https://doi.org/10.1016/j.scib.2020.04.035, 2020.
Sun, Y. X., Liu, S. L., Liu, Y. X., Dong, Y. H., Li, M. Q., An, Y., and Shi, F. N.: Grazing intensity and human activity intensity data sets on the Qinghai-Tibetan Plateau during 1990–2015, Geosci. Data J., 9, 140–153, https://doi.org/10.1002/gdj3.127, 2021.
Tabassum, A., Abbasi, T., and Abbasi, S. A.: Reducing the global environmental impact of livestock production: the minilivestock option, J. Clean. Prod., 112, 1754–1766, https://doi.org/10.1016/j.jclepro.2015.02.094, 2016.
Van Boeckel, T. P., Prosser, D., Franceschini, G., Biradar, C., Wint, W., Robinson, T., and Gilbert, M.: Modelling the distribution of domestic ducks in Monsoon Asia, Agr. Ecosyst. Environ., 141, 373–380, https://doi.org/10.1016/j.agee.2011.04.013, 2011.
Veldhuis, M. P., Ritchie, M. E., Ogutu, J. O., Morrison, T. A., Beale, C. M., Estes, A. B., Mwakilema, W., Ojwang, G. O., Parr, C. L., Probert, J., Wargute, P. W., Hopcraft, J. G. C., and Han, O.: Cross-boundary human impacts compromise the Serengeti-Mara ecosystem, Science, 363, 1424–1428, https://doi.org/10.1126/science.aav0564, 2019.
Venglovsky, J., Sasakova, N., and Placha, I.: Pathogens and antibiotic residues in animal manures and hygienic and ecological risks related to subsequent land application, Bioresource Technol., 100, 5386–5391, https://doi.org/10.1016/j.biortech.2009.03.068, 2009.
Waha, K., van Wijk, M. T., Fritz, S., See, L., Thornton, P. K., Wichern, J., and Herrero, M.: Agricultural diversification as an important strategy for achieving food security in Africa, Glob. Change Biol., 24, 3390–3400, https://doi.org/10.1111/gcb.14158, 2018.
Wang, R. J., Feng, Q. S., Jin, Z. R., and Liang, T. G.: The Restoration Potential of the Grasslands on the Tibetan Plateau, Remote Sens.-Basel, 14, 80, https://doi.org/10.3390/rs14010080, 2021.
Wang, Y. F., Lv, W. W., Xue, K., Wang, S. P., Zhang, L. R., Hu, R. H., Zeng, H., Xu, X. L., Li, Y. M., Jiang, L. L., Hao, Y. B., Du, J. Q., Sun, J. P., Dorji, T., Piao, S. L., Wang, C. H., Luo, C. Y., Zhang, Z. H., Chang, X. F., Zhang, M. M., Hu, Y. G., Wu, T. H., Wang, J. Z., Li, B. W., Liu, P. P., Zhou, Y., Wang, A., Dong, S. K., Zhang, X. Z., Gao, Q. Z., Zhou, H. K., Shen, M. G., Wilkes, A., Miehe, G., Zhao, X. Q., and Niu, H. S.: Grassland changes and adaptive management on the Qinghai–Tibetan Plateau, Nat. Rev. Earth. Env., 3, 668–683, https://doi.org/10.1038/s43017-022-00330-8, 2022.
Wang, Y. X., Sun, Y., Wang, Z. F., Chang, S. H., and Hou, F. J.: Grazing management options for restoration of alpine grasslands on the Qinghai-Tibet Plateau, Ecosphere, 9, e02515, https://doi.org/10.1002/ecs2.2515, 2018.
Wei, Y. Q., Lu, H. Y., Wang, J. N., Wang, X. F., and Sun, J.: Dual Influence of Climate Change and Anthropogenic Activities on the Spatiotemporal Vegetation Dynamics Over the Qinghai-Tibetan Plateau From 1981 to 2015, Earths Future, 10, 1–23, https://doi.org/10.1029/2021EF002566, 2022.
Yang, J. and Huang, X.: The 30 m annual land cover dataset and its dynamics in China from 1990 to 2019, Earth Syst. Sci. Data, 13, 3907–3925, https://doi.org/10.5194/essd-13-3907-2021, 2021.
Yang, Y. J., Song, G., and Lu, S.: Assessment of land ecosystem health with Monte Carlo simulation: A case study in Qiqihaer, China, J. Clean. Prod., 250, 119522, https://doi.org/10.1016/j.jclepro.2019.119522, 2020.
Ye, T., Liu, W. H., Mu, Q. Y., Zong, S., Li, Y. J., and Shi, P. J.: Quantifying livestock vulnerability to snow disasters in the Tibetan Plateau: Comparing different modeling techniques for prediction, Int. J. Disast. Risk Re., 48, 101578, https://doi.org/10.1016/j.ijdrr.2020.101578, 2020.
Zhai, D. C., Gao, X. Z., Li, B. L., Yuan, Y. C., Jiang, Y. H., Liu, Y., Li, Y., Li, R., Liu, W., and Xu, J.: Driving Climatic Factors at Critical Plant Developmental Stages for Qinghai–Tibet Plateau Alpine Grassland Productivity, Remote Sens.-Basel, 14, 1564, https://doi.org/10.3390/rs14071564, 2022.
Zhan, N., Liu, W. H., Ye, T., Li, H. D., Chen, S., and Ma, H.: High-resolution livestock seasonal distribution data on the Qinghai-Tibet Plateau in 2020, Sci. Data, 10, 142, https://doi.org/10.1038/s41597-023-02050-0, 2023.
Zhang, B. H., Zhang, Y. L., Wang, Z. F., Ding, M. J., Liu, L. S., Li, L. H., Li, S. C., Liu, Q. H., Paudel, B., and Zhang, H. M.: Factors Driving Changes in Vegetation in Mt. Qomolangma (Everest): Implications for the Management of Protected Areas, Remote Sens.-Basel, 13, 4725, https://doi.org/10.3390/rs13224725, 2021.
Zhang, R. Y., Wang, Z. W., Han, G. D., Schellenberg, M. P., Wu, Q., and Gu, C.: Grazing induced changes in plant diversity is a critical factor controlling grassland productivity in the Desert Steppe, Northern China, Agr. Ecosyst. Environ., 265, 73–83, https://doi.org/10.1016/j.agee.2018.05.014, 2018.
Zhang, W. B., Li, J., Struik, P. C., Jin, K., Ji, B. M., Jiang, S. Y., Zhang, Y., Li, Y. H., Yang, X. J., and Wang, Z.: Recovery through proper grazing exclusion promotes the carbon cycle and increases carbon sequestration in semiarid steppe, Sci. Total Environ., 892, 164423, https://doi.org/10.1016/j.scitotenv.2023.164423, 2023.
Zhang, Y., Hu, Q. W., and Zou, F. L.: Spatio-Temporal Changes of Vegetation Net Primary Productivity and Its Driving Factors on the Qinghai-Tibetan Plateau from 2001 to 2017, Remote Sens.-Basel, 13, 1566, https://doi.org/10.3390/rs13081566, 2021.
Zhao, X. Q., Xu, T. W., Ellis, J., He, F. Q., Hu, L. Y., and Li, Q.: Rewilding the wildlife in Sangjiangyuan National Park, Qinghai-Tibetan Plateau, Ecosyst. Health Sust., 6, 1776643, https://doi.org/10.1080/20964129.2020.1776643, 2020.
Zhou, W. X., Li, C. J., Wang, S., Ren, Z. B., and Stringer, L. C.: Effects of grazing and enclosure management on soil physical and chemical properties vary with aridity in China's drylands, Sci. Total Environ., 877, 162946, https://doi.org/10.1016/j.scitotenv.2023.162946, 2023.
Zhou, J., Niu, J., Wu, N., and Lu, T.: Annual high-resolution grazing intensity maps on the Qinghai-Tibet Plateau from 1990 to 2020 [Dataset], Zenodo, https://doi.org/10.5281/zenodo.10851119,2024.
Zhu, Q., Chen, H., Peng, C. H., Liu, J. X., Piao, S., He, J. S., Wang, S. P., Zhao, X. Q., Zhang, J., Fang, X. Q., Jin, J. X., Yang, Q. E., Ren, L. L., and Wang, Y. F.: An early warning signal for grassland degradation on the Qinghai-Tibetan Plateau, Nat. Commun., 14, 6406, https://doi.org/10.1038/s41467-023-42099-4, 2023.
Zhu, Y. Y., Zhang, H. M., Ding, M. J., Li, L. H., and Zhang, Y. L.: The Multiple Perspective Response of Vegetation to Drought on the Qinghai-Tibetan Plateau, Remote Sens.-Basel, 15, 902, https://doi.org/10.3390/rs15040902, 2023.