the Creative Commons Attribution 4.0 License.
the Creative Commons Attribution 4.0 License.
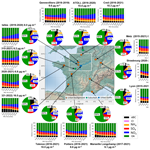
Multiyear high-temporal-resolution measurements of submicron aerosols at 13 French urban sites: data processing and chemical composition
Hasna Chebaicheb
Joel F. de Brito
Tanguy Amodeo
Florian Couvidat
Jean-Eudes Petit
Emmanuel Tison
Gregory Abbou
Alexia Baudic
Mélodie Chatain
Benjamin Chazeau
Nicolas Marchand
Raphaële Falhun
Florie Francony
Cyril Ratier
Didier Grenier
Romain Vidaud
Shouwen Zhang
Gregory Gille
Laurent Meunier
Caroline Marchand
Véronique Riffault
Olivier Favez
This paper presents a first comprehensive analysis of long-term measurements of atmospheric aerosol components from aerosol chemical speciation monitor (ACSM) and multiwavelength Aethalometer (AE33) instruments collected between 2015 and 2021 at 13 (sub)urban sites as part of the French CARA (Chemical Characterization of Particles) program. The datasets contain the mass concentrations of major chemical species within submicron aerosols (PM1), namely organic aerosols (OAs), nitrate (), ammonium (), sulfate (), non-sea-salt chloride (Cl−), and equivalent black carbon (eBC). Rigorous quality control, technical validation, and environmental evaluation processes were applied, adhering to both guidance from the French Reference Laboratory for Air Quality Monitoring (LCSQA) and the Aerosol, Clouds, and Trace Gases Research Infrastructure (ACTRIS) standard operating procedures. Key findings include geographical differences in the aerosol chemical composition, seasonal variations, and diel patterns, which are influenced by meteorological conditions, anthropogenic activities, and proximity to emission sources. Overall, OA dominates PM1 at each site (43 %–60 % of total mass), showing distinct seasonality with higher concentrations (i) in winter, due to enhanced residential heating emissions, and (ii) in summer, due to increased photochemistry favoring secondary aerosol formation. NO3 is the second most important contributor to PM1 (15 %–30 %), peaking in late winter and early spring, especially in northern France, and playing a significant role during pollution episodes. SO4 (8 %–14 %) and eBC (5 %–11 %) complement the major fine-aerosol species, with their relative contributions strongly influenced by the origin of air masses and the stability of meteorological conditions, respectively.
A comparison with the 3D chemical transport model (CTM) CHIMERE shows high correlations between simulations and measurements, albeit with an OA concentration underestimation of 46 %–76 %. Regional discrepancies in NO3 concentration levels emphasize the importance of these datasets with respect to validating air quality models and tailoring air pollution mitigation strategies. The datasets can be found at https://doi.org/10.5281/zenodo.13318298 (Chebaicheb et al., 2024).
- Article
(3426 KB) - Full-text XML
-
Supplement
(5390 KB) - BibTeX
- EndNote
The investigation of atmospheric aerosols holds significant importance in both the scientific and policy spheres due to their substantial impacts on climate (IPCC, 2023) and human health (WHO, 2021). For instance, in Europe in the year 2021, it is estimated that 97 % of the urban population experienced levels surpassing the annual concentration of 5 µg m−3 recommended by the World Health Organization (WHO) for particulate matter with an aerodynamic diameter smaller than 2.5 µm (PM2.5), and exposure to these fine particles was associated with more than 253 000 premature deaths in the aforementioned period (EEA, 2023). WHO guidelines as well as regulatory thresholds set at the national level (according to the Directive 2008/50/EC for European Member States) are mainly linked with the total mass concentration of suspended particles in a given size range. However, the elaboration and evaluation of specific action plans to improve air quality require a sound knowledge of their formation, which also allows the investigation of their emission sources and chemical processes in ambient air (Viana et al., 2008; Fuzzi et al., 2015). Moreover, forecasting systems, such as those using chemical transport models (CTMs), usually use chemically speciated emission inventories as inputs, and their validation benefits from comparisons with measurements of the PM chemical composition at representative sites (e.g., Ciarelli et al., 2016; EMEP, 2023).
Historically, PM chemical speciation has mainly been based on offline laboratory analyses of aerosol samples collected on filters (e.g., Putaud et al., 2004). Nowadays, such methods are well standardized and provide the opportunity for comprehensive characterization of major species as well as trace compounds (EMEP, 2022). However, they are known to be subject to various sampling artifacts (Schaap et al., 2004; Wittmaack and Keck, 2004) and are collected at relatively low temporal resolution (typically 24 h). They are also quite laborious and costly when used for long-term monitoring purposes. To overcome these limitations, significant efforts have been made to develop online chemical analyzers for in situ measurements in near-real time. In particular, there has been a growing interest in the continuous quantification of black carbon in ambient air, especially using filter-based absorption photometers (Savadkoohi et al., 2023), given the significant influence of this aerosol component on climate (Forster et al., 2023). In parallel, the development and worldwide deployment of the aerosol mass spectrometer (AMS; Canagaratna et al., 2007) over the last 2 decades has allowed the study of non-refractory compounds (i.e., organic aerosol, OA; nitrate, ; sulfate, ; chloride, Cl−; and ammonium, ) within the fine-aerosol mode (mainly submicron aerosols, PM1) (Crenn et al., 2017; Lanz et al., 2010; Roig Rodelas et al., 2019a; Sun et al., 2010). In addition to these sophisticated high-resolution instruments, which are well suited for intensive but short-term campaigns, the aerosol chemical speciation monitor (ACSM) has been designed for continuous, multiyear measurements of the same major chemical species in the PM1 or PM2.5 fractions (Bressi et al., 2021; Chebaicheb et al., 2023; Heikkinen et al., 2021; Ng et al., 2011a; Zhang et al., 2019). Both measurement methods (i.e., absorption photometers and ACSM) have become widely used in research monitoring, such as the Aerosol, Clouds, and Trace Gases Research Infrastructure (ACTRIS; https://www.actris.eu, last access: 28 October 2024) in Europe (Laj et al., 2024), and within the Atmospheric Science and mEasurement NeTwork (ASCENT; https://research.gatech.edu/, last access: 28 October 2024) in the United States. Their robustness and relatively low operating costs also make them good candidates for deployment at air quality monitoring stations operated by environmental agencies (Petit et al., 2015).
In this context, since 2015, multiwavelength Aethalometer (model AE33; Drinovec et al., 2015) and ACSM instruments have been operated at an increasing number of urban sites in France as part of the CARA (Chemical Characterization of Particles) program (set up by the French Reference Laboratory for Air Quality Monitoring in 2008) within the national air quality monitoring network (Favez et al., 2021), with the following main objectives: (i) documentation of the chemical composition (and possibly the dominant sources) of PM pollution episodes in near-real time; (ii) provision of multiyear datasets of the chemical composition of the fine-PM fraction, for inclusion in future trend analyses and/or epidemiological studies; (iii) provision of a comprehensive overview of the temporal and spatial variability in the chemical composition of fine PM over France, which can contribute, in particular, to evaluating and improving the accuracy of air quality models.
The main objective of this paper is to report on the chemically speciated multiyear datasets and major findings obtained so far from these observations. After describing the quality control procedures applied to the corresponding measurements, we investigate the geographical specificities exhibited by the main chemical species within the fine PM and then provide typical seasonal and diel variations displayed by these compounds in France over the period from 2015 to 2021. The datasets presented here are made fully available for complementary research activities, including trend analyses and epidemiological investigations. They are also vital for evaluating and validating regional air quality models through comparison exercises, examples of which are also discussed in this article using CHIMERE CTM simulations. Indeed, the CHIMERE model is routinely validated against observations, and the online data from the CARA program play a crucial role in France with respect to the continuous enhancement of CHIMERE, resulting in more accurate forecasts.
2.1 Sites and measurement periods
The current study presents the chemical composition of fine particles at 13 sites in France – including 11 stations from regional air quality monitoring networks (AASQAs) and 2 research platforms, i.e., SIRTA (Greater Paris area) and ATOLL (Lille metropolis), both of which are also part of the ACTRIS European research infrastructure – within the CARA program during the period from 2015 to 2021. These stations have gradually been equipped with AE33 and ACSM instruments since 2015. A 1-year (2016–2017) dataset of ACSM measurements for ATOLL (Lille), SIRTA (Paris), and Marseille Longchamp was previously integrated into a multisite European study (Chen et al., 2022). A detailed description of the instruments is given in the Sect. 2.2, and the temporal coverage of the measurements considered here for each site is presented in Fig. 1. A summary of each sampling site, including coordinates and related networks, can also be found in Table S1 in the Supplement. The majority of these sites are urban background sites, with the exception of two suburban sites (ATOLL and SIRTA) and one urban traffic site (Boulevard Périphérique Est, BPEst, in Paris). Geographically distributed throughout France, these sampling sites provide a global view of the chemical composition of fine particles at the national scale.
2.2 Non-refractory submicron aerosol measurements
2.2.1 ACSM measurement principles
The ACSM, developed by Aerodyne Research Inc., is based on mass spectrometry. As previously mentioned, it measures the chemical composition of non-refractory submicron aerosol (NR-PM1) in real time, allowing long-term measurements with less monitoring and technical intervention compared with AMS, at a relatively high temporal resolution of about 30 min (Watson, 2017). All stations presented in this study are equipped with quadrupole ACSM (Q-ACSM) instruments (Ng et al., 2011b), except for the Marseille Longchamp site, where a time-of-flight ACSM (ToF-ACSM) instrument (Fröhlich et al., 2013) is deployed. The Q-ACSM is the most commonly used analyzer, as it meets the operational monitoring needs of the French monitoring agencies and is less complex than the ToF-ACSM, although the latter has lower detection limits and slightly better time resolution (about 10 min). More information about these instruments is presented in Table S2 in the Supplement.
The operating principle of the ACSM is briefly described below. Ambient air first enters the vacuum system through a 100 µm diameter critical orifice. It then passes through an aerodynamic lens that focuses the aerosol into a concentrated beam, which is further directed onto a vaporizer heated at a temperature of about 600 °C, causing the particles to transition to the gas phase. The gas-phase molecules are then subjected to ionization at 70 eV, resulting in molecular fragmentation. The fragmented ions are guided by ion lenses to a quadrupole or time-of-flight mass filter, depending on the ACSM model.
In the ACSM, the atmospheric sample is analyzed alternatively by passing it or not passing it through a particulate filter. The air signal can thus be subtracted from the unfiltered measurements to quantify the particulate chemical species. A measurement time base of approximately 29 min (corresponding to 28 cycles of filtered or unfiltered atmospheric samples) was used for each Q-ACSM dataset, while data were acquired with a 10 min time base for the ToF-ACSM. All ACSM instruments that operated within the framework of the CARA program were equipped with a PM1 aerodynamic lens and a standard vaporizer.
In the measured mass spectra, each fragment is linked to one or more species based on a fragmentation table originally developed by Allan et al. (2004) and subsequently refined by Canagaratna et al. (2007). The concentration of each chemical species is then obtained as the sum of its contribution in every corresponding fragment. Moreover, the instrument-specific response factor (RF) of NO3 and the relative ionization efficiencies (RIEs) of NH4 and SO4 are determined by sampling 300 nm ammonium nitrate (NH4NO3) and ammonium sulfate ((NH4)2SO4) aerosols (Freney et al., 2019). For OA and Cl, the default RIE values of 1.4 and 1.3 are used here. Finally, to obtain quantitative mass concentrations for each measured chemical species, a collection efficiency (CE) correction factor is applied, following the procedure proposed by Middlebrook et al. (2011) discussed below.
2.2.2 ACSM quality checks and data handling
The data collected here from the ACSM instrument undergo strict quality control and technical validation, including an environmental evaluation involving comparison with complementary data. These validation procedures have been performed following the guidance provided by the French Reference Laboratory for Air Quality Monitoring (Amodeo, 2018) and in full agreement with the ACTRIS standard operating procedures, which are available online (https://www.actris-ecac.eu/pmc-non-refractory-organics-and-inorganics.html, last access: 28 October 2024).
On-site calibrations for air quality monitoring sites have been performed yearly by LCSQA personnel as well as after each sensitive maintenance by the instrument distributor in Europe (ADDAIR). A detailed description of the applied calibration procedures is available in a specific document edited at the national level (LCSQA, 2022). Moreover, each ACSM employed in the CARA program has routinely participated in intercomparison exercises organized by the Aerosol Chemical Monitor Calibration Center (ACMCC) at SIRTA, to ensure proper calibration and functioning of the instruments (e.g., LCSQA, 2023).
Given that the majority of instruments used here are Q-ACSM, data processing will be detailed with a focus on this model. ToF-ACSM data processing (deployed at Marseille Longchamp) is described more specifically in Chazeau et al. (2021). The Q-ACSM data handling was carried out using the manufacturer's software in Igor Pro (version 6.37). The first step involved checking the stability and continuity of technical parameters, including inlet pressure (maintained at approximately 1.3 ± 0.2 Torr), vaporizer temperature (regulated from the voltage calibration curve initially defined by the manufacturer), secondary electron multiplier (SEM) and heater bias voltages, filament emission, air beam value (set around 107 ± 30 % ions s−1), and relative humidity (ensuring it remains below 40 % using a Nafion dryer upstream of the inlet). Data points exhibiting inconsistencies were systematically flagged and invalidated. In the second step, the calibration results, notably the RF and RIE, were carefully analyzed for consistency. This approach ensured that the data-cleaning process was attuned to changes in RF and RIE, thereby improving the accuracy of the resulting dataset. If the RIE and RF values from two subsequent calibrations were deemed comparable, their average was used; otherwise, time-dependent RIE and RF were used, notably following instrument modification. During this data-cleaning phase, the CE was maintained at a constant value of 1. In the third step, data points with air ( 28, 32, and 40) and water ( 18) signal spikes were removed via a systematic cleaning procedure executed within the Igor Pro software, which allows the removal of signals that appear anomalous. This step also entails a comprehensive analysis of other ions to capture additional insights from the data. In particular, the examination of ions associated with chloride ( 35 and 36) allows for checking any possible measurement artifact that may be caused by sea salts (Tobler et al., 2020), while specific organic compounds ( 43, 44, and 55), including the fragment related to levoglucosan ( 60), serve as a crucial checkpoint for assessing the impact of distinct sources, such as biomass combustion, traffic emissions, and/or secondary formation processes.
As a next step, the implementation of the TIS (time series) and RIT (relative ion transmission) corrections was performed. The TIS correction encompasses the correction of crucial time-dependent signals that exert a significant influence on the measured concentrations captured by the instrument. These include the adjustment of variables such as the inflow rate directed into the Q-ACSM “reference P” (inlet pressure), the “reference N2” signal for air beam, and the “reference RF” for ionization efficiency. Subsequently, the RIT correction is applied to account for the mass spectrometer transmission efficiency within the Q-ACSM, based on the naphthalene peaks used as the internal standard and represented by 51, 62, 76, 102, and 128 (normalized to 1 below 51 and set at 0.05 for 154 and beyond with an exponential fit for the interval in between). We also closely examined the RIT time series linked to these ions, particularly in cases where the RIT standard deviation was high. We found several instances in which the mean RIT value may appear satisfactory, yet the time series could have periods of anomalous behavior. Thus, it is essential to carefully examine each time series of individual naphthalene masses, beyond the evaluation of average RIT values alone. After these corrections, the Middlebrook algorithm (Middlebrook et al., 2011), with a minimum CE of 0.5, was applied to correct the mass concentrations for the so-called composition-dependent collection efficiency (CDCE) correction.
The following verification step involves examining the ion balance, which implies assessing the correlation between the measured and predicted NH4 concentrations, with a target slope theoretically falling within the range of 1 ± 10 %, at sites and under atmospheric conditions where most aerosols should contain enough ammonium to be neutral as ammonium nitrate (NH4NO3), ammonium sulfate ((NH4)2SO4), and ammonium chloride (NH4Cl). To compute the measured and predicted NH4 concentrations, the following calculations were employed:
Finally, the analysis carefully accounted for the specific detection limits (DLs) corresponding to various chemical species. Following Ng et al. (2011b), DL values for the Q-ACSM are 0.284, 0.148, 0.024, 0.012, and 0.011 µg m−3 for NH4, OA, SO4, NO3, and Cl, respectively. The same DL has been considered here for the ToF-ACSM instrument deployed at Marseille Longchamp. Data levels above the DL were validated, whereas those between and the DL were replaced by DL2. Conversely, data below were invalidated (see Table S3 in the Supplement).
2.3 Equivalent black carbon measurements
2.3.1 Brief description of the AE33 device
Complementary to ACSM measurements, equivalent black carbon (eBC) was monitored at all sites over the same periods using a multiwavelength model AE33 Aethalometer (Magee Scientific). As with other filter-based absorption photometers, the AE33 primarily determines aerosol absorption coefficients (babs) at selected wavelengths, based on the rate of change in the attenuation of light transmitted through the particle-laden filter. A full description of the AE33 operating principles is given by Drinovec et al. (2015). Briefly, the instrument continuously captures aerosol particles by directing the airflow onto a specific spot on the filter tape. It assesses the aerosol by gauging the amount of light transmission that passes through a part of the filter tape containing the sample, compared with the light passing through a reference zone. In the AE33, the reference zone also samples aerosols, albeit with a reduced airflow and, thus, at different aerosol accumulation rates, allowing for more accurate eBC and particle light absorption estimates (termed “dual spot”). The analysis is carried out at seven optical wavelengths ranging from near-ultraviolet (UV) to near-infrared (IR) (370, 470, 525, 590, 660, 880, and 950 nm).
It should be noted that AE33 measurements used in the present paper have been performed in the PM1 fraction at both ACTRIS national facilities (ATOLL and SIRTA) but in the PM2.5 fraction at other stations. It is, however, considered that black carbon aerosols are overwhelmingly present in submicron particle matter (Bond et al., 2013) so that eBC concentrations discussed herewith can be (i) compared with one another (i.e., from one site to another) and (ii) combined with ACSM NR-PM1 measurements to describe the main chemical components of fine PM at the studied sites.
2.3.2 AE33 quality checks and data handling
Similarly to ACSM measurements, the AE33 devices were operated following LCSQA guidelines (LCSQA, 2020). The absorption coefficients used herewith were then calculated at each wavelength according to current ACTRIS guidelines (https://actris-ecac.eu/particle-light-absorption.html, last access: 28 October 2024), following Eq. (3):
where MAE represents the specific mass absorption efficiency corresponding to each wavelength (empirically determined by the manufacturer), and H is the appropriate harmonization factor to account for multiple scattering effects of the filter, which is set at 1.76 for AE33 devices using the M8060 filter tape. The eBC concentrations were then derived by normalization with a constant mass absorption cross-section (MACACTRIS) recently investigated in the frame of the EU H2020 RI-URBANS research program (Alastuey et al., 2022; Savadkoohi et al., 2024), following Eq. (4):
eBC concentrations are obtained at a wavelength of 880 nm, as this region is less prone to artifacts caused by other light-absorbing compounds, such as dust (notably iron oxides) and some organic compounds (termed brown carbon, BrC, which absorb light at shorter wavelengths in the UV spectrum). In ambient air, the MAC value varies from site to site and from season to season, which affects the quantification of eBC mass concentrations. The harmonization factor was introduced by ACTRIS to standardize the calculation of absorption coefficients, depending on the filter tape used. At 880 nm, the MACACTRIS factor used here is equivalent to 7.5 m2 g−1, also in good agreement with results previously obtained by Zanatta et al. (2016). It should be noted, nonetheless, that the application of the harmonization factor and the subsequent recalculation of eBC using a default and constant MAC value result in a reduction of about 40 % for eBC levels compared with the instrument raw outputs widely used in previous pan-European studies (such as Chen et al., 2022).
AE33 data qualification procedures include checking the absorption Ångström exponent (AAE) value obtained from the seven wavelengths for each data point, aggregated to a 15 min time base. Lower and upper acceptable AAE values of 0.7 and 3.0 are arbitrarily considered here, and the determination coefficient (r2) of the exponential fit used to calculate this AAE value must be greater than 0.9. Data points that did not meet these criteria were discarded. The validated data also underwent an assessment against the instrumental DL, which was set at approximately 100 ng m−3. Data falling within the range of to the DL were replaced by DL2, and data below were invalidated (Table S3).
The source apportionment of ambient eBC concentrations is based on the model of Sandradewi et al. (2008). Briefly, the two-component model calculates the aerosol optical absorption coefficient by combining fractions associated with wood burning (wb) and fossil fuel (ff) combustion. It exploits the variations in absorption characteristics at different wavelengths. This method is based on the assumption that wood combustion has a marked absorption in the UV (high AAE) compared with fossil fuels (low AAE). For this study, and the different sites, the separation between eBCff and eBCwb was performed using the values provided by the AE33 manufacturer: AAEff=1 and AAEwb=2 (Drinovec et al., 2015).
2.4 Chemical mass closure and related uncertainties
PM1 is a significant fraction of PM2.5, especially in Europe (Putaud et al., 2004); therefore, understanding the composition and concentration of PM1 is essential for assessing the health risks and wider environmental impacts associated with PM2.5 exposure. PM1 mass was reconstructed by combining chemical species from ACSM (non-refractory ) and eBC from AE33 (). For each station over the study period, PM1 mass concentrations were compared with continuous PM2.5 measurements conducted using a tapered-element oscillating microbalance equipped with a filter dynamics measurement system (TEOM-FDMS; Thermo Fisher Scientific) and/or a Fidas 200 optical particle counter (Palas GmbH) and/or a β gauge monitor (BAM 1020; Met One Instruments), according to the European standard for PM regulatory measurements (EN 16450). Linear regressions of hourly data reveal fairly good agreement between the reconstructed PM1 and the PM2.5 mass concentrations measured at each site (Fig. S1 in the Supplement), with determination coefficients (r2) ranging from 0.72 to 0.88 (except for Marseille Longchamp, which yielded an r2 value of 0.58) and slopes varying from 0.71 to 0.99 (except for Lyon, Strasbourg, and Metz, which showed distinct lower slopes of 0.57, 0.58, and 0.61, respectively). These results confirm that PM2.5 is predominantly made up of submicron particles and underscore the ACSM efficacy with respect to capturing a significant proportion of that fraction at most sites. Hereafter, PM1 (mass concentration) will be used to refer to submicron aerosol loadings estimated as the sum of eBC and NR-PM1 species measured by the AE33 and ACSM, respectively.
Reconstructed PM1 may overestimate measured PM2.5 loadings, mainly due to the respective measurement uncertainties of each technique used here. For PM2.5, the Fidas instrument has been demonstrated to be equivalent to the EN12341 standard method, with a maximum overall uncertainty of 25 % compared with this reference method according to EN16450 (Amodeo, 2024). It should also be stated that this instrument is sensitive to particles above 180 nm optical diameter only, which may result in even higher uncertainties for the estimation of the PM1 mass fraction. For eBC, a recent intercomparison between 23 AE33 devices (Cuesta-Mosquera et al., 2021) within the framework of the ACTRIS research infrastructure showed that the total mean deviation of the eBC concentrations at 880 nm for the 23 instruments was −2 % (range: −16 % to 7 %) before maintenance and −1 % (range: −14 % to 8 %) after maintenance, for soot measurements, emphasizing that the unit-to-unit variability was not significant. In our case, the post-processing of the datasets is the same for every site, thereby ensuring the comparability of the obtained concentration values. However, the main uncertainty in eBC concentrations lies in the various correction factors applied, not in the raw measurement itself. Considering the various approaches commonly used to transform absorption coefficients into eBC mass concentrations, as well as the related propagation of errors, an overall uncertainty of up to ±50 % can be associated with eBC estimates (Savadkoohi et al., 2024). Eventually, the Q-ACSM was shown to display reproducibility uncertainties of 9 % on NR-PM1 measurement, with uncertainties of 15 %, 19 %, 28 %, and 36 % for NO3, OA, SO4, and NH4, respectively (Crenn et al., 2015). The high uncertainties of SO4 may be related to the RIE of SO4, especially as it was considered constant in the early years. Additional uncertainties are related to possible measurement artifacts associated with interferences due to the nitrate (and sulfate) signal (e.g., the Pieber effect on the signal at 44; Pieber et al., 2016). This artifact is explained by NO3 (or SO4)-induced reactions on the vaporizer and ionizer surfaces, producing CO2 and, therefore, increasing the 44 signal that is otherwise attributed to organic aerosol. It can be quantified and evaluated over time by tracking the 44NO3 ( 30SO4) ratios during the different calibrations performed with pure ammonium nitrate (ammonium sulfate) solutions. During the ACSM intercomparison at the ACMCC in 2016 (Freney et al., 2019), the 44NO3 ratio was determined to vary between 0.01 and 0.26 for 15 instruments, while the 30SO4 ratio was determined to vary between 0.01 and 0.173. These were checked for each instrument in this study using calibration data, and the results obtained fell within these ranges; thus, no correction was applied. The overestimation of PM1 could also be linked to a change in the chemical composition of organic aerosols when this fraction dominates (e.g., Nault et al., 2023; Xu et al., 2018), as the RIE for organics is considered constant (1.4 by default) and these species are not considered in the Middlebrook correction (Middlebrook et al., 2011). Finally, other uncertainties can be related to size selection. It should be noted that the ACSM aerodynamic lens system is considered to be fully efficient for particles from 40 up to 600 nm (Liu et al., 2007); however, recent studies are suggesting collection size ranges that might be considered instrument-specific (Poulain et al., 2020).
3.1 Geographical specificities in the chemical composition
Figure 2 summarizes the PM1 average values, as well as their relative contributions as pie charts and bar plots, calculated according to the PM1 percentiles at various sites in France.
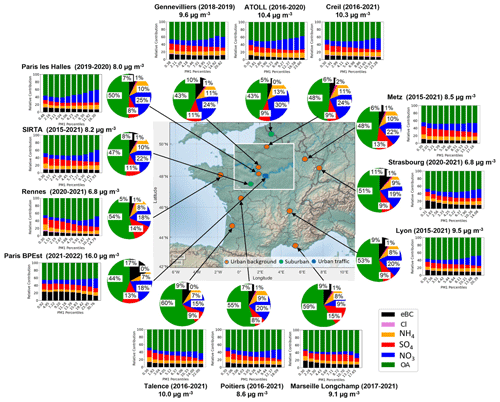
Figure 2The multiyear averaged PM1 mass concentration and pie charts of average relative contributions of non-refractory species and eBC at different sites in France; the bar charts represent the relative contribution as a function of PM1 deciles.
The mean PM1 concentrations at the 13 sites range from 6.8 to 16.0 µg m−3, reflecting the specificities of each urban site. These levels are comparable with the annual average NR-PM1 levels reported by Bressi et al. (2021) across 21 sampling sites in Europe (from 2.8 to 14 µg m−3, including remote mountain sites), with the highest NR-PM1 concentrations observed in midlatitude Europe. In addition, Chen et al. (2022) reported an average PM1 concentration of 12.2 ± 9.3 µg m−3 for 13 urban sites in Europe. In the present study, PM1 averaged 9.4 ± 8.3 µg m−3, while PM2.5 averaged 11.5 ± 9.2 µg m−3. It is important to note that this multiyear PM2.5 level exceeds the annual WHO guideline value of 5 µg m−3 (WHO, 2021), as is the case at most sites in Europe (EEA, 2023).
Figure 3 further displays some key statistics for the various chemical species as well as for PM1 and PM2.5 mass concentrations, as a function of mean levels measured at each site. The only site with a “road traffic” typology (BPEst), located on the east side of the Paris ring road, exhibits the highest mean PM1 concentration (16.0 µg m−3), standing out notably with respect to the eBC, SO4, and OA levels (Fig. 3). On the other hand, Rennes and Strasbourg display the lowest mass concentrations of PM1 (6.8 µg m−3), both having the lowest levels of OA (around 3.5 µg m−3). In addition, the site in Rennes shows a significantly lower mean eBC level (0.4 µg m−3), compared with the general average (0.8 µg m−3), thus depicting a lower influence of combustion aerosols at this site. The remaining sites generally exhibit a fairly homogeneous PM1 mass concentration, ranging from about 8 to 10 µg m−3. The ATOLL, Creil, and Talence sites have higher PM1 concentrations (between 10 and 10.4 µg m−3): the first two (located in the northern Hauts-de-France region) are influenced by higher NO3 concentration levels of 3.1 and 2.4 µg m−3, respectively, whereas Talence (near Bordeaux in the southern Nouvelle-Aquitaine region) has a strong contribution of OA (6.0 µg m−3).
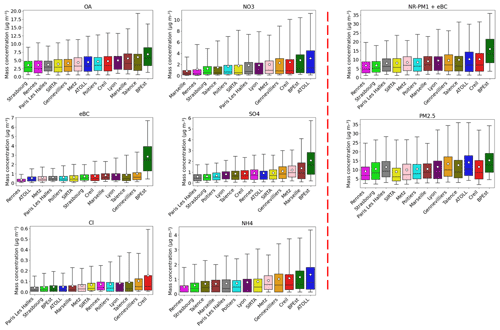
Figure 3Box plots of the statistical distribution (5th, 25th, 50th, 75th, and 95th percentiles) of each NR-PM1 species and eBC as well as of PM1 and PM2.5 mass concentrations; means are indicated by circles.
The high NO3 levels at the two sites in northern France are attributed to road traffic and combustion emissions (rich in nitrogen oxides; NOx), which combine with ammonia (NH3), typically associated with agricultural activities, forming ammonium nitrate (NH4NO3; AN) under favorable meteorological conditions (Roig Rodelas et al., 2019b), as well as to transboundary pollution from eastern Europe (Chebaicheb et al., 2023). Conversely, Talence has the highest 95th percentile of OA (higher than 19.0 µg m−3; Fig. 3), associated with strong biomass combustion in the Bordeaux area during the cold season (Favez et al., 2021).
For the Greater Paris region, the SIRTA facility is located 22 and 25 km away from the sites representing central areas of Paris, i.e., Paris Les Halles and Gennevilliers, respectively. Logically, due to the closer proximity to intense emission sources, Gennevilliers exhibits higher PM1 concentrations (9.6 µg m−3 on average over the 2018–2019 period) compared with SIRTA levels of 8.2 µg m−3. The comparable PM1 loading presented here between Paris Les Halles (8.0 µg m−3) and SIRTA is probably linked to the specific measurement periods analyzed for each site. Indeed, the data from Paris Les Halles presented here include the COVID-19 lockdown periods of 2020–2021, whereas SIRTA data are averaged over 2015–2021. When averaged over the same period as Paris Les Halles, the PM1 level at SIRTA decreases to 6.2 µg m−3. Moreover, an increased mixing-layer height over the Paris city center, due to the urban heat island effect which may dilute the aerosol content in a wider volume during daytime, should also be considered when comparing concentrations from inner and suburban sites within such a megapolis (e.g., Dupont et al., 2016).
The analysis of individual contributions shows that organic compounds make up about half of the PM1 total mass across all sites, ranging from 43 % to 60 %, which is comparable with the average of OA at urban sites in Europe (around 50 % of PM1), as reported by Chen et al. (2022). It is also consistent with the OA relative contribution observed by Bressi et al. (2021) in Europe (36 %–64 % of NR-PM1). The stations located in central and southern France, including Marseille Longchamp, Poitiers, Talence, and Lyon, show higher OA mass concentrations than sites in the north, which can be partly due to more intense secondary formation. Conversely, NO3 contributions are more pronounced at northern sites (22 %–30 %, vs. 9 %–20 % at southern sites), due to more favorable conditions for particulate AN formation (e.g., Favez et al., 2007). Consequently, NO3 mass concentrations in France decreased from north to south and from east to west, consistent with the findings by Favez et al. (2021). Furthermore, NO3 constitutes the second most significant contributor, accounting for 15 %–30 % of PM1 mass, except for at Marseille Longchamp, where it is less than 10 % (0.8 µg m−3). Other studies have also reported the predominance of NO3 over SO4 at many European sites (Bressi et al., 2021; Chen et al., 2022). As Marseille is characterized by high emissions from industry and shipping activities, the Marseille Longchamp site exhibits a higher contribution of SO4 (15 %), making it the second major contributor to PM1 at that site (Chazeau et al., 2021).
Overall, SO4 is the third largest contributor in France, with contributions ranging from 8 % to 14 %. Besides Marseille Longchamp and the BPEst traffic site, significant SO4 concentrations are also obtained for Metz and Gennevilliers (around 1 µg m−3 on average), probably reflecting transport from SO2-rich regions, given that local emissions are considered low or negligible. Furthermore, SO4 is considered to be influenced by long-range transport from central Europe, which is the case for many sites in northern and eastern France, including SIRTA, ATOLL, Creil, Paris Les Halles, Strasbourg, and Poitiers.
For the remaining compounds, mean NH4 levels range from 0.5 to 1.3 µg m−3, with a contribution fluctuating between 7 % and 13 %, showing a strong correlation with NO3 and SO4 levels, linked to the neutralization of sulfuric and nitric acids by NH3. Meanwhile, the contribution of eBC varies from 5 % to 11 % at the urban background sites investigated here. Previous studies, including Chen et al. (2022), reported higher contributions of BC at different European urban sites (12 %), which can be explained by recent changes in data processing, as discussed in Sect. 2.3.2. Finally, Cl makes a minor contribution of around 1 % at all sites, with averaged mass concentrations generally very low, remaining below 0.1 µg m−3, except for Gennevilliers (0.1 µg m−3) and Creil (0.15 µg m−3), with a slightly higher contribution of 2 %. Ammonium chloride (AC; NH4Cl) is formed in the atmosphere from the chemical reaction of hydrochloric acid (HCl) and NH3. The main sources of HCl in the atmosphere are biomass combustion (Andreae et al., 1996), coal burning (Tobler et al., 2020, 2021), and waste combustion (McCulloch et al., 1999). In Creil, there is a large waste treatment plant 2 km northeast of the monitoring station, which could explain the higher concentration of Cl observed at this site (Fig. S3 in the Supplement). Similarly, in Gennevilliers, industrial emissions could explain occasional spikes measured during easterly winds.
Figure 2 also illustrates the variations in PM1 chemical composition as a function of PM1 mass concentrations, divided into 10 concentration levels (corresponding to deciles) for each site. OA exhibits even higher contributions at high PM1 mass concentrations at Talence, Marseille Longchamp, and Poitiers, especially during the coldest and warmest months of the year (Fig. S4 in the Supplement). This can generally be explained by the influence of biomass burning during winter pollution episodes, as also previously described for the Paris area (Petit et al., 2015; Foret et al., 2022), and by the impact of secondary formation of organic compounds and emissions from forest fires in summer (Chen et al., 2022). However, OA decreases from the 30th percentile (around 4 to 5 µg m−3) of PM1 levels with an increase in NO3 at sites in northern France and Lyon. NO3 plays an important role during pollution events, particularly in spring, as reported previously in France (Dupont et al., 2016; Petit et al., 2017; Zhang et al., 2021) and at other midlatitude European sites (Bressi et al., 2021).
The contributions of SO4 and eBC are generally stable or show a slight decrease with increasing PM1. Nevertheless, eBC exhibits significant contributions at lower PM1 levels at BPEst and, to a lesser extent, Marseille Longchamp, Strasbourg, and Rennes, indicating significant local combustion sources at those sites. Furthermore, Marseille Longchamp exhibits fairly consistent OA, NO3, and SO4 contributions to PM1 levels, although it also show a significant increase in the first two during pollution events. Globally, SO4 is a relevant contributor for Metz, Rennes, Gennevilliers, SIRTA, Talence, and Marseille Longchamp, while OA retains significance at all sites throughout the PM1 percentiles.
3.2 Seasonal and diel cycles of fine-aerosol chemical species
The averaged seasonal and diel cycles were investigated for the different chemical species at all sites. Figure 4 shows the median and interquartile range (IQR) monthly variability for each species considered here, over the averaged cycles for the (sub)urban sites over France. The averaged monthly variabilities in the PM1 species for each site are shown in Fig. S5 in the Supplement.
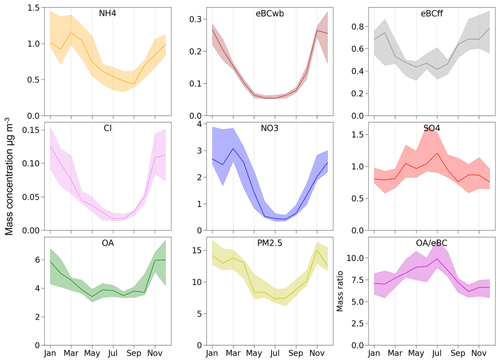
Figure 4Monthly variability in the mass concentrations of PM1 species, PM2.5, and the OAeBC ratio across all sites. The figure shows the median and IQR (25th and 75th percentiles) calculated from the averaged monthly concentrations for each site. Months were only considered if the data coverage was at least 75 %.
All chemical species exhibit significant variability in mass concentration over the months. In particular, eBCwb shows a clear seasonality, with higher concentrations during winter (around an average of 0.3 µg m−3) compared with summer (0.05 µg m−3), as expected due to the high level of wood combustion for residential heating in wintertime. Furthermore, there is substantial variability between sites in winter (represented by a larger IQR), probably as a result of different meteorological conditions as well as the fraction of wood combustion for residential heating in the surroundings. Conversely, eBCff shows seasonal variations comparable to eBCwb, although with smaller winter or summer difference spans ranging from around 0.4 to 0.7 µg m−3 in May and October, respectively. This variability is associated with seasonal meteorological conditions favoring (or disfavoring) the accumulation of atmospheric pollutants, compounded to a lesser extent to changes in road traffic intensity, leading to a maximum commonly observed in autumn (Petit et al., 2015). Similarly, OA displays higher levels during cold seasons (5.5 µg m−3), with reasons comparable to those for eBC, and lower levels during warm periods (3.5 µg m−3). Nevertheless, OA peaks (with a higher OAeBC mass ratio) in summer, reflecting the formation of secondary organic aerosol (SOA) from biogenic and anthropogenic sources (Favez et al., 2007). Notably, SOAs are formed mainly from biogenic volatile organic compounds in summer, when temperatures and sunlight are high (Canonaco et al., 2015; Cao et al., 2022), but also during nighttime, likely associated with nitrate chemistry (Kiendler-Scharr et al., 2016). Furthermore, OA yields lower site-to-site variability (i.e., IQR) (Fig. S6 in the Supplement), as most of the OA, even in wintertime, is associated with regional processes and secondary formation (Chen et al., 2022; Chebaicheb et al., 2023).
NO3 and NH4 concentrations display a marked seasonal pattern, peaking in late winter and early spring, and average around 3.0 and 1.2 µg m−3, respectively. As discussed in the previous section, AN concentrations depend on site-specific factors, contributing to a greater variability between sites. In contrast, SO4 shows a relatively stable monthly variation, with higher levels observed between April and August. Elevated summertime SO4 concentrations could be attributed to favorable meteorological conditions. In addition, SO4 can either be formed “locally” from the oxidation of SO2 or transported from emission hotspots, such as eastern European regions (Roig Rodelas et al., 2019b). Cl exhibits a strong seasonality, ranging from 0.02 (summer) to 0.14 µg m−3 (winter). The higher concentrations of HCl during the cold seasons can be partly attributed to its semi-volatile nature (similarly to AN, its formation should be favored by low temperatures and high humidity), as well as transport from emission hotspots areas, notably of intense coal combustion, further enhanced during wintertime (Tobler et al., 2021).
The mean diel profiles obtained for each chemical species across all (sub)urban background sites and for each season are shown in Fig. 5. All species exhibit higher concentrations at night, which could be, at least partially, associated with a lower boundary layer height. Some species show variability associated with local emission sources, including road traffic (morning and evening peaks), notably for OA and eBCff, with consistent behavior throughout the year. OA shows a stronger nighttime peak, notably during the colder months, mimicking eBCwb associated with wood heating. OA enhancement during nighttime in wintertime is linked with residential heating under a lower boundary layer (Favez et al., 2021). Furthermore, at Paris Les Halles, in the heart of the city center, OA further exhibits a small peak at noon (Fig. S7 in the Supplement), pointing to a possible influence of cooking emissions at this site. Overall, the PM2.5 profile aligns with OA diel cycles, with higher loadings during the morning and evening hours, due to the predominance of the organic species in the fine-aerosol fraction.
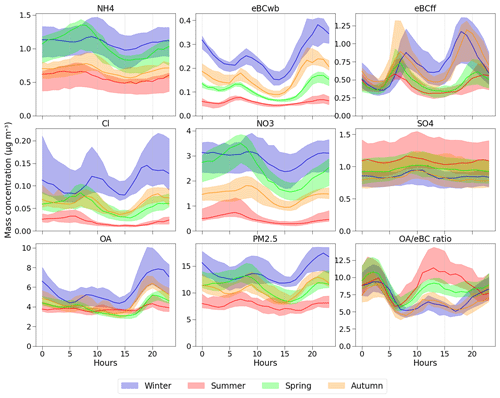
Figure 5Seasonal median and IQR of daily profiles for all sites for each PM1 component, PM2.5, and the OAeBC ratio.
Both NO3 and NH4 display a comparable diel cycle, featuring higher mass concentrations during the morning hours in all seasons, albeit at different levels. Lower temperatures and higher relative humidity in the morning favor the formation of AN. During the day, as temperatures rise, AN concentrations decrease due to the evaporation into the gas phase of NH3 and HNO3. Consequently, AN mass concentrations are lowest in summer, due to unfavorable weather conditions and, to some extent, reduced NOx levels associated with the school holidays (Roig Rodelas et al., 2019b). As discussed previously, AN levels are highest in spring, due to favorable meteorological conditions and intensive agricultural activities. On the other hand, the diel cycle of SO4 shows relatively constant values during the day, with higher levels observed in summer, as discussed previously. Notably, the diel cycle of SO4 at some sites features morning or afternoon peaks, especially for the Lyon and Marseille Longchamp sites, which may be explained by the presence of local (Chazeau et al., 2021) or regional sources (Figs. S7–S9 in the Supplement).
Finally, the OAeBC ratio shows an interesting diel cycle, exhibiting greater values at night in all seasons, ranging from 8 to 12, possibly associated with nighttime SOA formation or OA-rich sources such as wood combustion. This ratio also increases during the day, which could be explained by photochemistry and SOA formation, particularly of biogenic origin during summertime (Chebaicheb et al., 2023). As expected, the ratio decreases during the morning and evening rush hours, associated with more BC-rich traffic emissions.
Measurements of PM chemical composition are a valuable tool for validating atmospheric CTMs, specifically with respect to assessing their accuracy and reliability. In particular, observations and model outputs are complementary information used to track complex atmospheric sources and processes, including chemical transformations leading to secondary PM formation. Comparing chemically speciated observations with CTM results enables discrepancies to be identified and could provide clues on model improvement. In addition, near-real-time observations allow one to gauge a model's ability to represent the temporal and spatial distributions of atmospheric pollutants, which is essential for forecasting air quality and assessing environmental policies and scenarios. The continuous observations provided by the CARA program are of great importance for the continuous improvement of 3D air quality models, notably CHIMERE, leading to more accurate forecasts and a better understanding of atmospheric processes.
4.1 Model description
In order to exemplify the comparison of our database with CTM output, 3D simulations were performed with the CHIMERE version of Wang et al. (2024), which is based on a coupling between CHIMERE (Menut et al., 2021) and the SSH-aerosol v1.3 aerosol model (Sartelet et al., 2020). The secondary organic aerosol (SOA) mechanism of Wang et al. (2024) was used. This mechanism was obtained by using the GENOA (GENerator of reduced Organic Aerosol) v2.0 algorithm (Wang et al., 2022, 2023) to reduce the SOA mechanisms for monoterpenes and sesquiterpenes from the Master Chemical Mechanism (Saunders et al., 2003) coupled with the PRAM (Peroxy Radical Autoxidation Mechanism) (accounting for SOA formation from monoterpenes by autoxidation) (Roldin et al., 2019). Following Wang et al. (2023), the hydrophilic/hydrophobic organics (Chrit et al., 2017) mechanism was used for other precursors. Primary organic aerosols are treated as semivolatile organic compounds that partition as a function of environmental conditions and can undergo aging (Couvidat and Bessagnet 2021).
One important feature of SSH-aerosol is the computation of gas–particle partitioning with the thermodynamic module ISORROPIA (Nenes et al., 1998) and SOAP (Secondary Organic Aerosol Processor; Couvidat and Sartelet, 2015) models for inorganic and organic aerosols, respectively. The latter accounts for the condensation of semivolatile organic compounds onto the organic and aqueous phases of particles as well as the effect on the partitioning of interactions between organic and inorganic compounds based on their molecular structure. Thermodynamic equilibrium was assumed for gas–particle partitioning.
Meteorological data were obtained from the operational analysis of the Integrated Forecasting System (IFS) model of the European Centre for Medium-Range Weather Forecasts (ECMWF) (Flentje et al., 2021). Boundary conditions were taken from CAMS CIFS (IFS coupled to a tropospheric chemistry scheme) global model simulations (Flentje et al., 2021) for chemical species. Anthropogenic emissions of gases and particles were taken from the CAMS-REG-AP inventory at a 0.05°×0.1° grid resolution (version v5.1_REF2.1) (Kuenen et al., 2022).
4.2 Comparison results
CHIMERE model results for the year 2018, with a spatial resolution of 7 km over France, were used for a comparison with PM1 observations at nine of the sites where data were available (excluding BPEst, Paris Les Halles, Rennes, and Strasbourg). The time series of observed and modeled concentrations are shown in the Supplement (Fig. S10). Figure 6 summarizes results from the comparison between observations and simulations, typically showing good agreement. Loadings for inorganics (NO3, SO4, NH4, and Cl) and eBC are fairly well captured by the model across all sites, with some exceptions. In particular, at the Marseille Longchamp site, SO4, NO3, NH4, and eBC are consistently underestimated by the model (33 %, 41 %, 45 %, and 65 %, respectively). This discrepancy could be due to the low resolution of the model grid (0.0625°×0.125°), which may not be sufficient to capture local meteorology or sources, or more broadly a potential underestimation of emissions in the southeastern region of France. Several sites also present an underestimation of SO4 (Metz, SIRTA, and Talence) of around 35 %–39 %. In contrast, NO3 is strongly overestimated by the model (57 %) in the north of France (ATOLL). Organics, on the other hand, are consistently underestimated by the model at all sites by a factor of 2–3. As eBC is well represented, as discussed above, this leads to low modeled OAeBC ratios (2.7–5.2, vs. 3.9–8.8 for observed OAeBC ratios), suggesting an underestimation of secondary organic aerosols in the model. Other recent studies have also reported underestimations of OA at 11 European sites, focusing on winter 2009 (Ciarelli et al., 2016). In the present study, OA yields a strong underestimation particularly in the warmer months (60 %, vs. 41 % for the colder months).
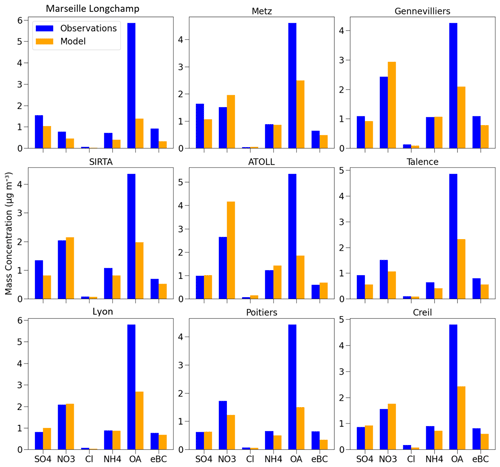
Figure 6Mean mass concentration (in µg m−3) of different chemical species for observations (in blue) and simulations (in orange) at nine French sites over the year 2018.
Figure 7 displays the diel profiles of each species, comparable with Fig. 5, for the winter and summer of 2018 (spring and autumn profiles can be found in Fig. S11 in the Supplement). In general, the species exhibit relatively consistent model performance between winter and summer, although there is a model underestimation for the latter. For NO3, the concentrations observed during wintertime are relatively stable throughout the day, whereas the model shows a strong daytime decrease due to the modeled volatilization of ammonium nitrate. During summertime, an enhancement of NO3 in the early morning is captured by both observations and the model, although as a smooth nighttime increase or decrease for the former and as a sharp peak for the latter. A similar pattern is observed for NH4. For SO4, the diel profile is quite constant for both observations and simulations in summer. In winter, the slight increase in SO4 during the day is not captured by the model, which instead shows a low peak at night. For eBC, both observations and model simulations show two peaks during rush hours. In winter, the night peak is more pronounced in the model; nonetheless, observations and model simulations display comparable levels, in contrast to summertime, when the model tends to underestimate the concentrations. These differences in daily eBC profiles may be attributed to meteorological conditions or issues in the seasonal temporality of emissions. Finally for OA, as discussed above, the model largely underestimates observations in summer. Generally, the behavior is fairly well represented, but the wintertime nighttime enhancement is larger than observations, similar to eBC.
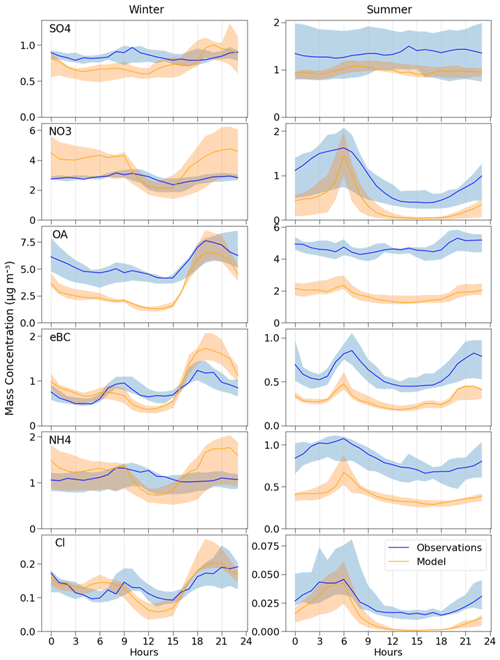
Figure 7Observed and modeled diel profiles during the winter and summer of 2018 across nine French sites.
Figure 8 presents some statistical parameters (mean bias; normalized root-mean-square error, RMSE; and correlation coefficient, r) calculated from the daily means for each chemical species across the nine urban sites in France. Overall, the correlations between observations and model results show good agreement, with correlation coefficients (r) ranging between 0.6 and 0.8, which is consistent with the literature (Couvidat et al., 2018; Cholakian et al., 2018). The mean bias and normalized RMSE confirm the model robustness. The mean bias is nearly negligible for SO4, NO3, NH4, Cl, and eBC, whereas it is approximately −2 µg m−3 for OA, reaching up to −4 µg m−3 for the Marseille Longchamp site. The RMSE exhibits a slightly more scattered distribution, generally ranging between 0.5 and 2 µg m−3.
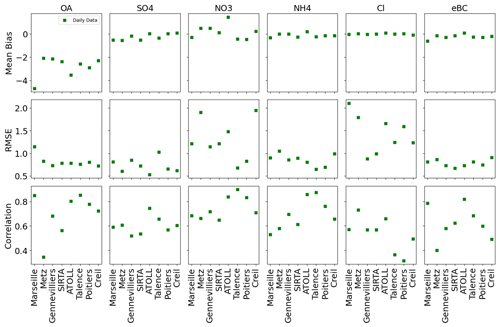
Figure 8Statistical parameters (mean bias; normalized RMSE; and the correlation coefficient, r) for different species at each site, using daily averages.
These comparisons between PM1 observations and model simulations reveal model underestimations or overestimations for each species. However, it remains challenging to pinpoint the exact reasons for these discrepancies, although hypotheses can be made. Generally, there is good agreement for SO4. On the other hand, significant peaks of modeled NO3 and NH4 are observed, particularly in November and December at northern France stations, which may be explained by an overestimation of NH3 emissions during this period in the model (Couvidat et al., 2018). For eBC, the results vary from one station to another, which may be linked to issues with the spatial distribution of emissions, which are not sufficiently accurate. OA is consistently underestimated across all stations. Further speciation of OA could provide more insights in this regard, which will be discussed in a forthcoming article on OA sources. Ultimately, conducting further simulations over other periods could help improve the model.
Furthermore, we could compare the model results with offline chemical information from filter samples collected in the submicron aerosol fraction at four sites in 2018 within the framework of the CARA program. These filter samples were collected daily from 15 March to 29 April 2018 in Talence; daily from 16 February to 1 April in Poitiers; daily from 1 January to 23 January, from 13 May to 27 May, and from 19 September to 22 September in Lyon; and every 4 h from 5 July to 27 July in Marseille Longchamp. They were analyzed in the laboratory with respect to their organic carbon (OC), elemental carbon (EC), SO4, NO3, and NH4 loadings. Figure S12 in the Supplement illustrates the comparison between model simulations and either online or offline observations for these four sites with respect to OA, NO3, NH4, SO4, and eBC.
A higher correlation is observed between simulations and ACSM observations for OA, NO3, and NH4 compared with filters (with r2 values of 0.5, 0.7, and 0.6 with ACSM, as opposed to 0.24, 0.54, and 0.36 with filters, respectively). SO4 and eBC show relatively similar correlations (with r2 values of 0.44 and 0.42 with ACSM and AE33, respectively, and 0.18 and 0.11 with filters, respectively), but they exhibit different slopes (the model vs. ACSM-AE33 PM1 demonstrates higher slopes of 0.45 and 0.5 compared with 0.36 and 0.33 with filters). Overall, the comparison of model results with observations from ACSM and AE33 instruments shows higher correlations than with filter analyses, emphasizing the importance of online measurements for validating air quality models.
The ACSM and AE33 datasets for SIRTA and ATOLL (Villeneuve-d'Ascq) are available from the EBAS database (https://ebas.nilu.no/, last access: 28 October 2024). Other measurements are freely available from https://doi.org/10.5281/zenodo.13318298 (Chebaicheb et al., 2024).
This study presents multiyear measurements from ACSM and AE33 instruments collected at 13 (sub)urban sites that are part of the French CARA program. The datasets ranged from 1 to 6 years in length and were collected between 2015 and 2021. Two of the sites are integrated into the ACTRIS European infrastructure, namely ATOLL (near Lille) and SIRTA (near Paris). The dataset contains submicron aerosol species, OA, NO3, NH4, SO4, Cl, and eBC, deconvolved into eBCff and eBCwb. A meticulous process of quality control, technical validation, and environmental assessment was employed to homogeneously and rigorously validate the datasets. This process followed guidelines provided by the French Reference Laboratory for Air Quality Monitoring and strictly adhered to the ACTRIS standard operating procedures. This article presents a comprehensive overview of these long-term datasets, offering an analysis of the geographical disparities in PM1 chemical composition, as well as the main seasonal and diel variations in fine-particle content.
Across all sites, OA is the predominant compound, with a mean concentration of 4.7 µg m−3 (43 %–60 %), in PM1, followed by NO3 (15 %–30 %), SO4 (8 %–14 %), NH4 (7 %–13 %), and eBC (5 %–11 %). Stations in central and southern France exhibit higher OA mass concentrations (5.3 µg m−3), likely attributed to more pronounced photochemical formation processes. Such secondary processes may also explain the fact that OA is the predominant compound with the highest concentration levels in summertime at all sites (Fig. S4). Additionally, in other seasons, OA exhibits greater contributions (>55 %) during periods of elevated PM1 levels in the southern half of France, while NO3 contributions (>40 %) are more notable during pollution episodes at northern sites, illustrating the competing influences of biomass burning emissions and favorable meteorological conditions on the aerosol chemical composition, leading to the formation of ammonium nitrate, depending on the site location.
Temporal variations reveal distinct seasonality in PM1 chemical species. eBCwb and OA peak during wintertime, with values of around 0.3 and 5.5 µg m−3, respectively, typically associated with increased residential heating emissions. Those values peak particularly at night, combining stronger emissions and a potentially shallower boundary layer height, facilitating pollutant accumulation. OA also peaks in summer (3.5 µg m−3), typically associated with enhanced SOA formation. NO3 peaks in late winter and early spring, correlated with a typical increase in NH3 and favorable meteorological conditions during cold periods. Diel variations also exhibit unique characteristics at certain sites, such as the Paris Les Halles site, where an organic peak at noon suggests a significant contribution from cooking activities; similarly, a more pronounced rush hour enhancement at BPEst suggests a strong effect of local traffic on OA levels.
Furthermore, the datasets presented here serve as essential tools for evaluating and validating regional and global air quality models. An illustrative comparison with CHIMERE is presented in this paper for 2018, encompassing nine French sites. Generally, the model successfully simulates inorganics (NO3, SO4, and NH4) and eBC but underestimates OA by 46 %–76 %, although with a high correlation between simulations and measurements (r value of between 0.6 and 0.8). Notably, NO3 seems to be overestimated at the ATOLL site in northern France (57 %), whereas it is substantially underestimated (by 29 %–42 %) at southern sites. Overall, these multiyear datasets from French urban background sites hold significant value for the scientific community, enabling future research endeavors, including source apportionment studies, trend analyses, and epidemiological and health-related investigations.
The supplement related to this article is available online at: https://doi.org/10.5194/essd-16-5089-2024-supplement.
MC, BC, HC, JEP, and SZ: data curation and formal analysis. OF and VR: funding acquisition. HC: investigation. HC: methodology. GA, AB, MC, BC, FC, RF, FF, GG, DG, NM, JEP, CR, VR, RV, and SZ: resources. JFdB, OF, CM, and VR: supervision. JFdB, OF, CM, and VR: validation. HC: visualization. HC and OF: writing – original draft preparation. All co-authors: writing – review and editing.
The contact author has declared that none of the authors has any competing interests.
Publisher's note: Copernicus Publications remains neutral with regard to jurisdictional claims made in the text, published maps, institutional affiliations, or any other geographical representation in this paper. While Copernicus Publications makes every effort to include appropriate place names, the final responsibility lies with the authors.
The authors are deeply grateful to the many technicians, engineers, and scientists working within the AASQA network and at Ineris, IMT Nord Europe, LSCE, and LCE for their past and current involvement in the long-term operation of the monitors and data handling at the sites investigated in the present study. We cannot cite each of them exhaustively but strongly hope that they will all recognize themselves here.
This work was notably supported by the French Ministry of Environment, through direct funding of activities achieved by the AASQA network and LCSQA within the framework of the CARA program. SIRTA observations have been partly funded by the EU H2020 ACTRIS-2 project (under grant agreement no. 654109) as well as within the framework of the CNRS-INSU long-term monitoring aerosol program SNO CLAP as a component of the ACTRIS French research infrastructure. Measurements conducted at ATOLL are also part of the Labex CaPPA project (grant no. ANR-11-LABX-0005-01) and the CLIMIBIO and ECRIN projects, the latter two of which are also funded by the regional council of Hauts-de-France and the European Regional Development Fund (ERDF). Observations at the Marseille Longchamp supersite benefited from complementary financial support from the PACA region (grant no. 2017_08809; PRISM project).
This paper was edited by Jing Wei and reviewed by two anonymous referees.
Alastuey, A., Querol, X., García, M., Trechera, P., Savadkoohi, M., Karanasiou, A., Minguillón, M. C., Fiebig, M., Dallënbach, K. R., Salameh, T., Sauvage, S., and Petäjä, T.: Deliverable D1 (D1.1): Guidelines, datasets of non-regulated pollutants incl. metadata, methods, https://riurbans.eu/wp-content/uploads/2022/10/RI-URBANS_D1_D1_1.pdf (last access: 28 October 2024), 2022.
Allan, J. D., Delia, A. E., Coe, H., Bower, K. N., Alfarra, M. R., Jimenez, J. L., Middlebrook, A. M., Drewnick, F., Onasch, T. B., Canagaratna, M. R., Jayne, J. T., and Worsnop, D. R.: A generalised method for the extraction of chemically resolved mass spectra from Aerodyne aerosol mass spectrometer data, J. Aerosol Sci., 35, 909–922, https://doi.org/10.1016/j.jaerosci.2004.02.007, 2004.
Amodeo, T.: Guide méthodologique : mesure de la composition chimique des particules submicroniques non réfractaires par Aerosol Chemical Speciation Monitor (ACSM), LCSQA, https://www.lcsqa.org/fr/rapport/2016/ienris/ (last access: 26 March 2024), 2018.
Amodeo, T.: Guide méthodologique pour la surveillance des PM10 et PM2.5 dans l'air ambiant par méthode optique FIDAS (révision 2023) | LCSQA, https://www.lcsqa.org/fr/rapport/, last access: 13 August 2024.
Andreae, M. O., Atlas, E., Harris, G. W., Helas, G., de Kock, A., Koppmann, R., Maenhaut, W., Manø, S., Pollock, W. H., Rudolph, J., Scharffe, D., Schebeske, G., and Welling, M.: Methyl halide emissions from savanna fires in southern Africa, J. Geophys. Res.-Atmos., 101, 23603–23613, https://doi.org/10.1029/95JD01733, 1996.
Bond, T. C., Doherty, S. J., Fahey, D. W., Forster, P. M., Berntsen, T., DeAngelo, B. J., Flanner, M. G., Ghan, S., Kärcher, B., Koch, D., Kinne, S., Kondo, Y., Quinn, P. K., Sarofim, M. C., Schultz, M. G., Schulz, M., Venkataraman, C., Zhang, H., Zhang, S., Bellouin, N., Guttikunda, S. K., Hopke, P. K., Jacobson, M. Z., Kaiser, J. W., Klimont, Z., Lohmann, U., Schwarz, J. P., Shindell, D., Storelvmo, T., Warren, S. G., and Zender, C. S.: Bounding the role of black carbon in the climate system: A scientific assessment, J. Geophys. Res.-Atmos., 118, 5380–5552, https://doi.org/10.1002/jgrd.50171, 2013.
Bressi, M., Cavalli, F., Putaud, J. P., Fröhlich, R., Petit, J.-E., Aas, W., Äijälä, M., Alastuey, A., Allan, J. D., Aurela, M., Berico, M., Bougiatioti, A., Bukowiecki, N., Canonaco, F., Crenn, V., Dusanter, S., Ehn, M., Elsasser, M., Flentje, H., Graf, P., Green, D. C., Heikkinen, L., Hermann, H., Holzinger, R., Hueglin, C., Keernik, H., Kiendler-Scharr, A., Kubelová, L., Lunder, C., Maasikmets, M., Makeš, O., Malaguti, A., Mihalopoulos, N., Nicolas, J. B., O'Dowd, C., Ovadnevaite, J., Petralia, E., Poulain, L., Priestman, M., Riffault, V., Ripoll, A., Schlag, P., Schwarz, J., Sciare, J., Slowik, J., Sosedova, Y., Stavroulas, I., Teinemaa, E., Via, M., Vodička, P., Williams, P. I., Wiedensohler, A., Young, D. E., Zhang, S., Favez, O., Minguillón, M. C., and Prevot, A. S. H.: A European aerosol phenomenology – 7: High-time resolution chemical characteristics of submicron particulate matter across Europe, Atmospheric Environ. X, 10, 100108, https://doi.org/10.1016/j.aeaoa.2021.100108, 2021.
Campagne 2021 d'étalonnage et de comparaison inter-laboratoire (CIL) des Q-ACSM | LCSQA: https://www.lcsqa.org/fr/rapport/ campagne-2021-detalonnage-et-de-comparaison-inter-laboratoire-cil-des-q-acsm, last access: 24 October 2023.
Canagaratna, M. R., Jayne, J. T., Jimenez, J. L., Allan, J. D., Alfarra, M. R., Zhang, Q., Onasch, T. B., Drewnick, F., Coe, H., Middlebrook, A., Delia, A., Williams, L. R., Trimborn, A. M., Northway, M. J., Decarlo, P. F., Kolb, C. E., Davidovits, P., and Worsnop, D. R.: Chemical and microphysical characterization of ambient aerosols with the aerodyne aerosol mass spectrometer, Mass Spectrom. Rev., 26, 185–222, https://doi.org/10.1002/mas.20115, 2007.
Canonaco, F., Slowik, J. G., Baltensperger, U., and Prévôt, A. S. H.: Seasonal differences in oxygenated organic aerosol composition: implications for emissions sources and factor analysis, Atmos. Chem. Phys., 15, 6993–7002, https://doi.org/10.5194/acp-15-6993-2015, 2015.
Cao, J., Situ, S., Hao, Y., Xie, S., and Li, L.: Enhanced summertime ozone and SOA from biogenic volatile organic compound (BVOC) emissions due to vegetation biomass variability during 1981–2018 in China, Atmos. Chem. Phys., 22, 2351–2364, https://doi.org/10.5194/acp-22-2351-2022, 2022.
Chazeau, B., Temime-Roussel, B., Gille, G., Mesbah, B., D'Anna, B., Wortham, H., and Marchand, N.: Measurement report: Fourteen months of real-time characterisation of the submicronic aerosol and its atmospheric dynamics at the Marseille–Longchamp supersite, Atmos. Chem. Phys., 21, 7293–7319, https://doi.org/10.5194/acp-21-7293-2021, 2021.
Chebaicheb, H., de Brito, J. F., Chen, G., Tison, E., Marchand, C., Prévôt, A. S. H., Favez, O., and Riffault, V.: Investigation of four-year chemical composition and organic aerosol sources of submicron particles at the ATOLL site in northern France, Environ. Pollut., 330, 121805, https://doi.org/10.1016/j.envpol.2023.121805, 2023.
Chebaicheb, H., Ferreira de Brito, J., Amodeo, T., Couvidat, F., Petit, J.-E., Tison, E., Abbou, G., Alexia, B., Chatain, M., Chazeau, B., Marchand, N., Falhun, R., Francony, F., Ratier, C., Grenier, D., Vidaud, R., Zhang, S., Gille, G., Meunier, L., Marchand, C., Riffault, V., and Favez, O.: Multi-year high time resolution measurements of fine PM at 13 sites of the French Operational Network (CARA program), In Earth System Science Data, Zenodo [data set], https://doi.org/10.5281/zenodo.13318298, 2024.
Chen, G., Canonaco, F., Tobler, A., Aas, W., Alastuey, A., Allan, J., Atabakhsh, S., Aurela, M., Baltensperger, U., Bougiatioti, A., De Brito, J. F., Ceburnis, D., Chazeau, B., Chebaicheb, H., Daellenbach, K. R., Ehn, M., El Haddad, I., Eleftheriadis, K., Favez, O., Flentje, H., Font, A., Fossum, K., Freney, E., Gini, M., Green, D. C., Heikkinen, L., Herrmann, H., Kalogridis, A.-C., Keernik, H., Lhotka, R., Lin, C., Lunder, C., Maasikmets, M., Manousakas, M. I., Marchand, N., Marin, C., Marmureanu, L., Mihalopoulos, N., Močnik, G., Nęcki, J., O'Dowd, C., Ovadnevaite, J., Peter, T., Petit, J.-E., Pikridas, M., Matthew Platt, S., Pokorná, P., Poulain, L., Priestman, M., Riffault, V., Rinaldi, M., Różański, K., Schwarz, J., Sciare, J., Simon, L., Skiba, A., Slowik, J. G., Sosedova, Y., Stavroulas, I., Styszko, K., Teinemaa, E., Timonen, H., Tremper, A., Vasilescu, J., Via, M., Vodička, P., Wiedensohler, A., Zografou, O., Cruz Minguillón, M., and Prévôt, A. S. H.: European aerosol phenomenology – 8: Harmonised source apportionment of organic aerosol using 22 Year-long ACSM/AMS datasets, Environ. Int., 166, 107325, https://doi.org/10.1016/j.envint.2022.107325, 2022.
Cholakian, A., Beekmann, M., Colette, A., Coll, I., Siour, G., Sciare, J., Marchand, N., Couvidat, F., Pey, J., Gros, V., Sauvage, S., Michoud, V., Sellegri, K., Colomb, A., Sartelet, K., Langley DeWitt, H., Elser, M., Prévot, A. S. H., Szidat, S., and Dulac, F.: Simulation of fine organic aerosols in the western Mediterranean area during the ChArMEx 2013 summer campaign, Atmos. Chem. Phys., 18, 7287–7312, https://doi.org/10.5194/acp-18-7287-2018, 2018.
Chrit, M., Sartelet, K., Sciare, J., Pey, J., Marchand, N., Couvidat, F., Sellegri, K., and Beekmann, M.: Modelling organic aerosol concentrations and properties during ChArMEx summer campaigns of 2012 and 2013 in the western Mediterranean region, Atmos. Chem. Phys., 17, 12509–12531, https://doi.org/10.5194/acp-17-12509-2017, 2017.
Ciarelli, G., Aksoyoglu, S., Crippa, M., Jimenez, J.-L., Nemitz, E., Sellegri, K., Äijälä, M., Carbone, S., Mohr, C., O'Dowd, C., Poulain, L., Baltensperger, U., and Prévôt, A. S. H.: Evaluation of European air quality modelled by CAMx including the volatility basis set scheme, Atmos. Chem. Phys., 16, 10313–10332, https://doi.org/10.5194/acp-16-10313-2016, 2016.
Couvidat, F. and Bessagnet, B.: Role of ecosystem-atmosphere exchanges of semi-volatile organic compounds in organic aerosol formation, Atmos. Environ., 263, 118541, https://doi.org/10.1016/j.atmosenv.2021.118541, 2021.
Couvidat, F. and Sartelet, K.: The Secondary Organic Aerosol Processor (SOAP v1.0) model: a unified model with different ranges of complexity based on the molecular surrogate approach, Geosci. Model Dev., 8, 1111–1138, https://doi.org/10.5194/gmd-8-1111-2015, 2015.
Couvidat, F., Bessagnet, B., Garcia-Vivanco, M., Real, E., Menut, L., and Colette, A.: Development of an inorganic and organic aerosol model (CHIMERE 2017β v1.0): seasonal and spatial evaluation over Europe, Geosci. Model Dev., 11, 165–194, https://doi.org/10.5194/gmd-11-165-2018, 2018.
Crenn, V., Sciare, J., Croteau, P. L., Verlhac, S., Fröhlich, R., Belis, C. A., Aas, W., Äijälä, M., Alastuey, A., Artiñano, B., Baisnée, D., Bonnaire, N., Bressi, M., Canagaratna, M., Canonaco, F., Carbone, C., Cavalli, F., Coz, E., Cubison, M. J., Esser-Gietl, J. K., Green, D. C., Gros, V., Heikkinen, L., Herrmann, H., Lunder, C., Minguillón, M. C., Močnik, G., O'Dowd, C. D., Ovadnevaite, J., Petit, J.-E., Petralia, E., Poulain, L., Priestman, M., Riffault, V., Ripoll, A., Sarda-Estève, R., Slowik, J. G., Setyan, A., Wiedensohler, A., Baltensperger, U., Prévôt, A. S. H., Jayne, J. T., and Favez, O.: ACTRIS ACSM intercomparison – Part 1: Reproducibility of concentration and fragment results from 13 individual Quadrupole Aerosol Chemical Speciation Monitors (Q-ACSM) and consistency with co-located instruments, Atmos. Meas. Tech., 8, 5063–5087, https://doi.org/10.5194/amt-8-5063-2015, 2015.
Crenn, V., Fronval, I., Petitprez, D., and Riffault, V.: Fine particles sampled at an urban background site and an industrialized coastal site in Northern France – Part 1: Seasonal variations and chemical characterization, Sci. Total Environ., 578, 203–218, https://doi.org/10.1016/j.scitotenv.2015.11.165, 2017.
Cuesta-Mosquera, A., Močnik, G., Drinovec, L., Müller, T., Pfeifer, S., Minguillón, M. C., Briel, B., Buckley, P., Dudoitis, V., Fernández-García, J., Fernández-Amado, M., Ferreira De Brito, J., Riffault, V., Flentje, H., Heffernan, E., Kalivitis, N., Kalogridis, A.-C., Keernik, H., Marmureanu, L., Luoma, K., Marinoni, A., Pikridas, M., Schauer, G., Serfozo, N., Servomaa, H., Titos, G., Yus-Díez, J., Zioła, N., and Wiedensohler, A.: Intercomparison and characterization of 23 Aethalometers under laboratory and ambient air conditions: procedures and unit-to-unit variabilities, Atmos. Meas. Tech., 14, 3195–3216, https://doi.org/10.5194/amt-14-3195-2021, 2021.
Drinovec, L., Močnik, G., Zotter, P., Prévôt, A. S. H., Ruckstuhl, C., Coz, E., Rupakheti, M., Sciare, J., Müller, T., Wiedensohler, A., and Hansen, A. D. A.: The “dual-spot” Aethalometer: an improved measurement of aerosol black carbon with real-time loading compensation, Atmos. Meas. Tech., 8, 1965–1979, https://doi.org/10.5194/amt-8-1965-2015, 2015.
Dupont, J.-C., Haeffelin, M., Badosa, J., Elias, T., Favez, O., Petit, J. E., Meleux, F., Sciare, J., Crenn, V., and Bonne, J. L.: Role of the boundary layer dynamics effects on an extreme air pollution event in Paris, Atmos. Environ., 141, 571–579, https://doi.org/10.1016/j.atmosenv.2016.06.061, 2016.
EMEP: EMEP/EEA air pollutant emission inventory guidebook 2023 [WWW Document], European Environment Agency, https://www.eea.europa.eu/publications/emep-eea-guidebook-2023 (last access: 28 October 2024), 2023.
European Environment Agency (EEA): Harm to human health from air pollution in Europe: burden of disease 2023, https://www.eea.europa.eu/publications/harm-to-human-health-from-air-pollution (last access: 4 March 2024), 2023.
Favez, O., Cachier, H., Sciare, J., and Le Moullec, Y.: Characterization and contribution to PM2.5 of semi-volatile aerosols in Paris (France), Atmos. Environ., 41, 7969–7976, https://doi.org/10.1016/j.atmosenv.2007.09.031, 2007.
Favez, O., Weber, S., Petit, J.-E., Alleman, L. Y., Albinet, A., Riffault, V., Chazeau, B., Amodeo, T., Salameh, D., Zhang, Y., Srivastava, D., Samaké, A., Aujay-Plouzeau, R., Papin, A., Bonnaire, N., Boullanger, C., Chatain, M., Chevrier, F., Detournay, A., Dominik-Sègue, M., Falhun, R., Garbin, C., Ghersi, V., Grignion, G., Levigoureux, G., Pontet, S., Rangognio, J., Zhang, S., Besombes, J.-L., Conil, S., Uzu, G., Savarino, J., Marchand, N., Gros, V., Marchand, C., Jaffrezo, J.-L., and Leoz-Garziandia, E.: Overview of the French Operational Network for In Situ Observation of PM Chemical Composition and Sources in Urban Environments (CARA Program), Atmosphere-Basel, 12, 207, https://doi.org/10.3390/atmos12020207, 2021.
Flentje, H., Mattis, I., Kipling, Z., Rémy, S., and Thomas, W.: Evaluation of ECMWF IFS-AER (CAMS) operational forecasts during cycle 41r1–46r1 with calibrated ceilometer profiles over Germany, Geosci. Model Dev., 14, 1721–1751, https://doi.org/10.5194/gmd-14-1721-2021, 2021.
Foret, G., Michoud, V., Kotthaus, S., Petit, J.-E., Baudic, A., Siour, G., Kim, Y., Doussin, J.-F., Dupont, J.-C., Formenti, P., Gaimoz, C., Ghersi, V., Gratien, A., Gros, V., Jaffrezo, J.-L., Haeffelin, M., Kreitz, M., Ravetta, F., Sartelet, K., Simon, L., Té, Y., Uzu, G., Zhang, S., Favez, O., and Beekmann, M.: The December 2016 extreme weather and particulate matter pollution episode in the Paris region (France), Atmos. Environ., 291, 119386, https://doi.org/10.1016/j.atmosenv.2022.119386, 2022.
Forster, P. M., Smith, C. J., Walsh, T., Lamb, W. F., Lamboll, R., Hauser, M., Ribes, A., Rosen, D., Gillett, N., Palmer, M. D., Rogelj, J., von Schuckmann, K., Seneviratne, S. I., Trewin, B., Zhang, X., Allen, M., Andrew, R., Birt, A., Borger, A., Boyer, T., Broersma, J. A., Cheng, L., Dentener, F., Friedlingstein, P., Gutiérrez, J. M., Gütschow, J., Hall, B., Ishii, M., Jenkins, S., Lan, X., Lee, J.-Y., Morice, C., Kadow, C., Kennedy, J., Killick, R., Minx, J. C., Naik, V., Peters, G. P., Pirani, A., Pongratz, J., Schleussner, C.-F., Szopa, S., Thorne, P., Rohde, R., Rojas Corradi, M., Schumacher, D., Vose, R., Zickfeld, K., Masson-Delmotte, V., and Zhai, P.: Indicators of Global Climate Change 2022: annual update of large-scale indicators of the state of the climate system and human influence, Earth Syst. Sci. Data, 15, 2295–2327, https://doi.org/10.5194/essd-15-2295-2023, 2023.
Freney, E., Zhang, Y., Croteau, P., Amodeo, T., Williams, L., Truong, F., Petit, J.-E., Sciare, J., Sarda-Esteve, R., Bonnaire, N., Arumae, T., Aurela, M., Bougiatioti, A., Mihalopoulos, N., Coz, E., Artinano, B., Crenn, V., Elste, T., Heikkinen, L., Poulain, L., Wiedensohler, A., Herrmann, H., Priestman, M., Alastuey, A., Stavroulas, I., Tobler, A., Vasilescu, J., Zanca, N., Canagaratna, M., Carbone, C., Flentje, H., Green, D., Maasikmets, M., Marmureanu, L., Minguillon, M. C., Prevot, A. S. H., Gros, V., Jayne, J., and Favez, O.: The second ACTRIS inter-comparison (2016) for Aerosol Chemical Speciation Monitors (ACSM): Calibration protocols and instrument performance evaluations, Aerosol Sci. Tech., 53, 830–842, https://doi.org/10.1080/02786826.2019.1608901, 2019.
Fröhlich, R., Cubison, M. J., Slowik, J. G., Bukowiecki, N., Prévôt, A. S. H., Baltensperger, U., Schneider, J., Kimmel, J. R., Gonin, M., Rohner, U., Worsnop, D. R., and Jayne, J. T.: The ToF-ACSM: a portable aerosol chemical speciation monitor with TOFMS detection, Atmos. Meas. Tech., 6, 3225–3241, https://doi.org/10.5194/amt-6-3225-2013, 2013.
Fuzzi, S., Baltensperger, U., Carslaw, K., Decesari, S., Denier van der Gon, H., Facchini, M. C., Fowler, D., Koren, I., Langford, B., Lohmann, U., Nemitz, E., Pandis, S., Riipinen, I., Rudich, Y., Schaap, M., Slowik, J. G., Spracklen, D. V., Vignati, E., Wild, M., Williams, M., and Gilardoni, S.: Particulate matter, air quality and climate: lessons learned and future needs, Atmos. Chem. Phys., 15, 8217–8299, https://doi.org/10.5194/acp-15-8217-2015, 2015.
Heikkinen, L., Äijälä, M., Daellenbach, K. R., Chen, G., Garmash, O., Aliaga, D., Graeffe, F., Räty, M., Luoma, K., Aalto, P., Kulmala, M., Petäjä, T., Worsnop, D., and Ehn, M.: Eight years of sub-micrometre organic aerosol composition data from the boreal forest characterized using a machine-learning approach, Atmos. Chem. Phys., 21, 10081–10109, https://doi.org/10.5194/acp-21-10081-2021, 2021.
IPCC: Summary for Policymakers, in: Climate Change 2023: Synthesis Report, Contribution of Working Groups I, II and III to the Sixth Assessment Report of the Intergovernmental Panel on Climate Change, edited by: Core Writing Team, Lee, H., and Romero, J., IPCC, Geneva, Switzerland, 1–34, https://doi.org/10.59327/IPCC/AR6-9789291691647.001, 2003.
Kiendler-Scharr, A., Mensah, A. A., Friese, E., Topping, D., Nemitz, E., Prevot, A. S. H., Äijälä, M., Allan, J., Canonaco, F., Canagaratna, M., Carbone, S., Crippa, M., Dall Osto, M., Day, D. A., De Carlo, P., Di Marco, C. F., Elbern, H., Eriksson, A., Freney, E., Hao, L., Herrmann, H., Hildebrandt, L., Hillamo, R., Jimenez, J. L., Laaksonen, A., McFiggans, G., Mohr, C., O'Dowd, C., Otjes, R., Ovadnevaite, J., Pandis, S. N., Poulain, L., Schlag, P., Sellegri, K., Swietlicki, E., Tiitta, P., Vermeulen, A., Wahner, A., Worsnop, D., and Wu, H.-C.: Ubiquity of organic nitrates from nighttime chemistry in the European submicron aerosol, Geophys. Res. Lett., 43, 7735–7744, https://doi.org/10.1002/2016GL069239, 2016.
Kuenen, J., Dellaert, S., Visschedijk, A., Jalkanen, J.-P., Super, I., and Denier van der Gon, H.: CAMS-REG-v4: a state-of-the-art high-resolution European emission inventory for air quality modelling, Earth Syst. Sci. Data, 14, 491–515, https://doi.org/10.5194/essd-14-491-2022, 2022.
Laj, P., Myhre, C. L., Riffault, V., Amiridis, V., Fuchs, H., Eleftheriadis, K., Petäjä, T., Kivekäs, N., Juurola, E., Saponaro, G., Philippin, S., Cornacchia, C., Alados Arboledas, L., Baars, H., Claude, A., De Mazière, M., Dils, B., Eder Murberg, L., Fiebig, M., Haeffelin, M., Herrmann, H., Höhler, K., Illmann, N., Kreuter, A., Ludewig, E., Marinou, E., Möhler, O., Mona, L., Nicolae, D., O'connor, E., Petracca Altieri, R. M., Picquet-Varrault, B., Popsichal, B., Putaud, J.-P., Reimann, S., Salameh, T., Siomos, N., Stachlewska, I., Van Pinxteren, D., Voudouri, K. A., Wandiger, U., Wiedensohler, A., Apituley, A., Comeron, A., Gysel-Beer, M., Mihalopoulos, N., Nikolova, N., Pietruczuk, A., Sauvage, S., Sciare, J., Skov, H., Svendby, T., Swietlicki, E., Tonev, D., Vaughan, G., Zdimal, V., Baltensperger, U., Doussin, J.-F., Kulmala, M., Pappalardo, G., Sorvari Sundet, S., and Vana, M.: Aerosol, Clouds and Trace Gases Research Infrastructure (ACTRIS): The European Research Infrastructure Supporting Atmospheric Science, Bull. Am. Meteorol. Soc., 105, E1098–E1136, https://doi.org/10.1175/BAMS-D-23-0064.1, 2024.
Lanz, V. A., Prévôt, A. S. H., Alfarra, M. R., Weimer, S., Mohr, C., DeCarlo, P. F., Gianini, M. F. D., Hueglin, C., Schneider, J., Favez, O., D'Anna, B., George, C., and Baltensperger, U.: Characterization of aerosol chemical composition with aerosol mass spectrometry in Central Europe: an overview, Atmos. Chem. Phys., 10, 10453–10471, https://doi.org/10.5194/acp-10-10453-2010, 2010.
LCSQA: Guide méthodologique pour la mesure du ≪ Black Carbon ≫ par Aethalomètre multi longueur d’onde AE33 dans l’air ambiant (version2020), LCSQA, https://www.lcsqa.org/fr/rapport/guide-methodologique-pour-la-mesure-du-black-carbon-par-aethalometre-multi-longueur-donde (last access: 28 October 2024), 2020.
LCSQA: Cahier des charges pour l’étalonnage des ACSM, LCSQA, https://www.lcsqa.org/fr/rapport/cahier-des-charges-pour-letalonnage-des-acsm (last access: 28 October 2024), 2022.
LCSQA: Campagne 2021 d’étalonnage et de comparaison inter-laboratoire (CIL) des Q-ACSM j LCSQA, https://www.lcsqa.org/fr/rapport/ (last access: 24 October 2023), 2023.
Liu, P. S. K., Deng, R., Smith, K. A., Williams, L. R., Jayne, J. T., Canagaratna, M. R., Moore, K., Onasch, T. B., Worsnop, D. R., and Deshler, T.: Transmission Efficiency of an Aerodynamic Focusing Lens System: Comparison of Model Calculations and Laboratory Measurements for the Aerodyne Aerosol Mass Spectrometer, Aerosol Sci. Tech., 41, 721–733, https://doi.org/10.1080/02786820701422278, 2007.
McCulloch, A., Aucott, M. L., Benkovitz, C. M., Graedel, T. E., Kleiman, G., Midgley, P. M., and Li, Y.-F.: Global emissions of hydrogen chloride and chloromethane from coal combustion, incineration and industrial activities: Reactive Chlorine Emissions Inventory, J. Geophys. Res.-Atmos., 104, 8391–8403, https://doi.org/10.1029/1999JD900025, 1999.
Menut, L., Bessagnet, B., Briant, R., Cholakian, A., Couvidat, F., Mailler, S., Pennel, R., Siour, G., Tuccella, P., Turquety, S., and Valari, M.: The CHIMERE v2020r1 online chemistry-transport model, Geosci. Model Dev., 14, 6781–6811, https://doi.org/10.5194/gmd-14-6781-2021, 2021.
Middlebrook, A. M., Bahreini, R., Jimenez, J. L., and Cana-Garatna, M. R.: Evaluation of Composition-Dependent Collection Efficiencies for the Aerodyne Aerosol Mass Spectrometer using Field Data, Aerosol Sci. Tech., 46, 258–271, 2011.
Nault, B. A., Croteau, P., Jayne, J., Williams, A., Williams, L., Worsnop, D., Katz, E. F., DeCarlo, P. F., and Canagaratna, M.: Laboratory evaluation of organic aerosol relative ionization efficiencies in the aerodyne aerosol mass spectrometer and aerosol chemical speciation monitor, Aerosol Sci. Tech., 57, 981–997, https://doi.org/10.1080/02786826.2023.2223249, 2023.
Nenes, A., Pandis, S. N., and Pilinis, C.: ISORROPIA: A New Thermodynamic Equilibrium Model for Multiphase Multicomponent Inorganic Aerosols, Aquat. Geochem., 4, 123–152, https://doi.org/10.1023/A:1009604003981, 1998.
Ng, N. L., Canagaratna, M. R., Jimenez, J. L., Zhang, Q., Ulbrich, I. M., and Worsnop, D. R.: Real-Time Methods for Estimating Organic Component Mass Concentrations from Aerosol Mass Spectrometer Data, Environ. Sci. Technol., 45, 910–916, https://doi.org/10.1021/es102951k, 2011a.
Ng, N. L., Herndon, S. C., Trimborn, A., Canagaratna, M. R., Croteau, P. L., Onasch, T. B., Sueper, D., Worsnop, D. R., Zhang, Q., Sun, Y. L., and Jayne, J. T.: An Aerosol Chemical Speciation Monitor (ACSM) for Routine Monitoring of the Composition and Mass Concentrations of Ambient Aerosol, Aerosol Sci. Technol., 45, 780–794, https://doi.org/10.1080/02786826.2011.560211, 2011b.
Petit, J.-E., Favez, O., Sciare, J., Crenn, V., Sarda-Estève, R., Bonnaire, N., Močnik, G., Dupont, J.-C., Haeffelin, M., and Leoz-Garziandia, E.: Two years of near real-time chemical composition of submicron aerosols in the region of Paris using an Aerosol Chemical Speciation Monitor (ACSM) and a multi-wavelength Aethalometer, Atmos. Chem. Phys., 15, 2985–3005, https://doi.org/10.5194/acp-15-2985-2015, 2015.
Petit, J.-E., Amodeo, T., Meleux, F., Bessagnet, B., Menut, L., Grenier, D., Pellan, Y., Ockler, A., Rocq, B., Gros, V., Sciare, J., and Favez, O.: Characterising an intense PM pollution episode in March 2015 in France from multi-site approach and near real time data: Climatology, variabilities, geographical origins and model evaluation, Atmos. Environ., 155, 68–84, https://doi.org/10.1016/j.atmosenv.2017.02.012, 2017.
Pieber, S. M., El Haddad, I., Slowik, J. G., Canagaratna, M. R., Jayne, J. T., Platt, S. M., Bozzetti, C., Daellenbach, K. R., Fröhlich, R., Vlachou, A., Klein, F., Dommen, J., Miljevic, B., Jiménez, J. L., Worsnop, D. R., Baltensperger, U., and Prévôt, A. S. H.: Inorganic Salt Interference on in Aerodyne AMS and ACSM Organic Aerosol Composition Studies, Environ. Sci. Technol., 50, 10494–10503, https://doi.org/10.1021/acs.est.6b01035, 2016.
Poulain, L., Spindler, G., Grüner, A., Tuch, T., Stieger, B., van Pinxteren, D., Petit, J.-E., Favez, O., Herrmann, H., and Wiedensohler, A.: Multi-year ACSM measurements at the central European research station Melpitz (Germany) – Part 1: Instrument robustness, quality assurance, and impact of upper size cutoff diameter, Atmos. Meas. Tech., 13, 4973–4994, https://doi.org/10.5194/amt-13-4973-2020, 2020.
Putaud, J.-P., Raes, F., Van Dingenen, R., Brüggemann, E., Facchini, M.-C., Decesari, S., Fuzzi, S., Gehrig, R., Hüglin, C., Laj, P., Lorbeer, G., Maenhaut, W., Mihalopoulos, N., Müller, K., Querol, X., Rodriguez, S., Schneider, J., Spindler, G., ten Brink, H., Tørseth, K., and Wiedensohler, A.: A European aerosol phenomenology – 2: chemical characteristics of particulate matter at kerbside, urban, rural and background sites in Europe, Atmos. Environ., 38, 2579–2595, https://doi.org/10.1016/j.atmosenv.2004.01.041, 2004.
Roig Rodelas, R., Chakraborty, A., Perdrix, E., Tison, E., and Riffault, V.: Real-time assessment of wintertime organic aerosol characteristics and sources at a suburban site in northern France, Atmos. Environ., 203, 48–61, https://doi.org/10.1016/j.atmosenv.2019.01.035, 2019a.
Roig Rodelas, R., Perdrix, E., Herbin, B., and Riffault, V.: Characterization and variability of inorganic aerosols and their gaseous precursors at a suburban site in northern France over one year (2015–2016), Atmos. Environ., 200, 142–157, https://doi.org/10.1016/j.atmosenv.2018.11.041, 2019b.
Roldin, P., Ehn, M., Kurtén, T., Olenius, T., Rissanen, M. P., Sarnela, N., Elm, J., Rantala, P., Hao, L., Hyttinen, N., Heikkinen, L., Worsnop, D. R., Pichelstorfer, L., Xavier, C., Clusius, P., Öström, E., Petäjä, T., Kulmala, M., Vehkamäki, H., Virtanen, A., Riipinen, I., and Boy, M.: The role of highly oxygenated organic molecules in the Boreal aerosol-cloud-climate system, Nat. Commun., 10, 4370, https://doi.org/10.1038/s41467-019-12338-8, 2019.
Sandradewi, J., Prévôt, A. S. H., Szidat, S., Perron, N., Alfarra, M. R., Lanz, V. A., Weingartner, E., and Baltensperger, U.: Using Aerosol Light Absorption Measurements for the Quantitative Determination of Wood Burning and Traffic Emission Contributions to Particulate Matter, Environ. Sci. Technol., 42, 3316–3323, https://doi.org/10.1021/es702253m, 2008.
Sartelet, K., Couvidat, F., Wang, Z., Flageul, C., and Kim, Y.: SSH-Aerosol v1.1: A Modular Box Model to Simulate the Evolution of Primary and Secondary Aerosols, Atmosphere-Basel, 11, 525, https://doi.org/10.3390/atmos11050525, 2020.
Saunders, S. M., Jenkin, M. E., Derwent, R. G., and Pilling, M. J.: Protocol for the development of the Master Chemical Mechanism, MCM v3 (Part A): tropospheric degradation of non-aromatic volatile organic compounds, Atmos. Chem. Phys., 3, 161–180, https://doi.org/10.5194/acp-3-161-2003, 2003.
Savadkoohi, M., Pandolfi, M., Reche, C., Niemi, J. V., Mooibroek, D., Titos, G., Green, D. C., Tremper, A. H., Hueglin, C., Liakakou, E., Mihalopoulos, N., Stavroulas, I., Artiñano, B., Coz, E., Alados-Arboledas, L., Beddows, D., Riffault, V., De Brito, J. F., Bastian, S., Baudic, A., Colombi, C., Costabile, F., Chazeau, B., Marchand, N., Gómez-Amo, J. L., Estellés, V., Matos, V., van der Gaag, E., Gille, G., Luoma, K., Manninen, H. E., Norman, M., Silvergren, S., Petit, J.-E., Putaud, J.-P., Rattigan, O. V., Timonen, H., Tuch, T., Merkel, M., Weinhold, K., Vratolis, S., Vasilescu, J., Favez, O., Harrison, R. M., Laj, P., Wiedensohler, A., Hopke, P. K., Petäjä, T., Alastuey, A., and Querol, X.: The variability of mass concentrations and source apportionment analysis of equivalent black carbon across urban Europe, Environ. Int., 178, 108081, https://doi.org/10.1016/j.envint.2023.108081, 2023.
Savadkoohi, M., Pandolfi, M., Favez, O., Putaud, J.-P., Eleftheriadis, K., Fiebig, M., Hopke, P. K., Laj, P., Wiedensohler, A., Alados-Arboledas, L., Bastian, S., Chazeau, B., María, Á. C., Colombi, C., Costabile, F., Green, D. C., Hueglin, C., Liakakou, E., Luoma, K., Listrani, S., Mihalopoulos, N., Marchand, N., Močnik, G., Niemi, J. V., Ondráček, J., Petit, J.-E., Rattigan, O. V., Reche, C., Timonen, H., Titos, G., Tremper, A. H., Vratolis, S., Vodička, P., Funes, E. Y., Zíková, N., Harrison, R. M., Petäjä, T., Alastuey, A., and Querol, X.: Recommendations for reporting equivalent black carbon (eBC) mass concentrations based on long-term pan-European in-situ observations, Environ. Int., 185, 108553, https://doi.org/10.1016/j.envint.2024.108553, 2024.
Schaap, M., Spindler, G., Schulz, M., Acker, K., Maenhaut, W., Berner, A., Wieprecht, W., Streit, N., Müller, K., Brüggemann, E., Chi, X., Putaud, J.-P., Hitzenberger, R., Puxbaum, H., Baltensperger, U., and ten Brink, H.: Artefacts in the sampling of nitrate studied in the “INTERCOMP” campaigns of EUROTRAC-AEROSOL, Atmos. Environ., 38, 6487–6496, https://doi.org/10.1016/j.atmosenv.2004.08.026, 2004.
Sun, J., Zhang, Q., Canagaratna, M. R., Zhang, Y., Ng, N. L., Sun, Y., Jayne, J. T., Zhang, X., Zhang, X., and Worsnop, D. R.: Highly time- and size-resolved characterization of submicron aerosol particles in Beijing using an Aerodyne Aerosol Mass Spectrometer, Atmos. Environ., 44, 131–140, https://doi.org/10.1016/j.atmosenv.2009.03.020, 2010.
Tobler, A. K., Skiba, A., Wang, D. S., Croteau, P., Styszko, K., Nȩcki, J., Baltensperger, U., Slowik, J. G., and Prévôt, A. S. H.: Improved chloride quantification in quadrupole aerosol chemical speciation monitors (Q-ACSMs), Atmos. Meas. Tech., 13, 5293–5301, https://doi.org/10.5194/amt-13-5293-2020, 2020.
Tobler, A. K., Skiba, A., Canonaco, F., Močnik, G., Rai, P., Chen, G., Bartyzel, J., Zimnoch, M., Styszko, K., Nȩcki, J., Furger, M., Różański, K., Baltensperger, U., Slowik, J. G., and Prevot, A. S. H.: Characterization of non-refractory (NR) PM1 and source apportionment of organic aerosol in Kraków, Poland, Atmos. Chem. Phys., 21, 14893–14906, https://doi.org/10.5194/acp-21-14893-2021, 2021.
Viana, M., Kuhlbusch, T. A. J., Querol, X., Alastuey, A., Harrison, R. M., Hopke, P. K., Winiwarter, W., Vallius, M., Szidat, S., Prévôt, A. S. H., Hueglin, C., Bloemen, H., Wåhlin, P., Vecchi, R., Miranda, A. I., Kasper-Giebl, A., Maenhaut, W., and Hitzenberger, R.: Source apportionment of particulate matter in Europe: A review of methods and results, J. Aerosol Sci., 39, 827–849, https://doi.org/10.1016/j.jaerosci.2008.05.007, 2008.
Wang, Z., Couvidat, F., and Sartelet, K.: GENerator of reduced Organic Aerosol mechanism (GENOA v1.0): an automatic generation tool of semi-explicit mechanisms, Geosci. Model Dev., 15, 8957–8982, https://doi.org/10.5194/gmd-15-8957-2022, 2022.
Wang, Z., Couvidat, F., and Sartelet, K.: Implementation of a parallel reduction algorithm in the GENerator of reduced Organic Aerosol mechanisms (GENOA v2.0): Application to multiple monoterpene aerosol precursors, J. Aerosol Sci., 174, 106248, https://doi.org/10.1016/j.jaerosci.2023.106248, 2023.
Wang, Z., Couvidat, F., and Sartelet, K.: Response of biogenic secondary organic aerosol formation to anthropogenic NOx emission mitigation, Sci. Total Environ., 927, 172142, https://doi.org/10.1016/j.scitotenv.2024.172142, 2024.
Watson, T. B.: Aerosol Chemical Speciation Monitor (ACSM) Instrument Handbook, OSTI.GOV, https://doi.org/10.2172/1375336, 2017.
WHO: Air Quality Guidelines, https://www.c40knowledgehub.org/s/article/WHO-Air-Quality-Guidelines?language=en_US (last access: 23 January 2023), 2021.
WHO Air Quality Guidelines: https://www.c40knowledgehub.org/s/article/WHO-Air-Quality-Guidelines?language=en_US, last access: 23 January 2023.
Wittmaack, K. and Keck, L.: Thermodesorption of aerosol matter on multiple filters of different materials for a more detailed evaluation of sampling artifacts, Atmos. Environ., 38, 5205–5215, https://doi.org/10.1016/j.atmosenv.2004.05.047, 2004.
Xu, W., Lambe, A., Silva, P., Hu, W., Onasch, T., Williams, L., Croteau, P., Zhang, X., Renbaum-Wolff, L., Fortner, E., Jimenez, J. L., Jayne, J., Worsnop, D., and Canagaratna, M.: Laboratory evaluation of species-dependent relative ionization efficiencies in the Aerodyne Aerosol Mass Spectrometer, Aerosol Sci. Tech., 52, 626–641, https://doi.org/10.1080/02786826.2018.1439570, 2018.
Zanatta, M., Gysel, M., Bukowiecki, N., Müller, T., Weingartner, E., Areskoug, H., Fiebig, M., Yttri, K. E., Mihalopoulos, N., Kouvarakis, G., Beddows, D., Harrison, R. M., Cavalli, F., Putaud, J. P., Spindler, G., Wiedensohler, A., Alastuey, A., Pandolfi, M., Sellegri, K., Swietlicki, E., Jaffrezo, J. L., Baltensperger, U., and Laj, P.: A European aerosol phenomenology-5: Climatology of black carbon optical properties at 9 regional background sites across Europe, Atmos. Environ., 145, 346–364, https://doi.org/10.1016/j.atmosenv.2016.09.035, 2016.
Zhang, S., Tison, E., Dusanter, S., Beaugard, C., Gengembre, C., Augustin, P., Fourmentin, M., Delbarre, H., and Riffault, V.: Near real-time PM1 chemical composition measurements at a French urban background and coastal site under industrial influence over more than a year: Temporal variability and assessment of sulfur-containing emissions, Atmos. Environ., 244, 117960, https://doi.org/10.1016/j.atmosenv.2020.117960, 2021.
Zhang, Y., Favez, O., Petit, J.-E., Canonaco, F., Truong, F., Bonnaire, N., Crenn, V., Amodeo, T., Prévôt, A. S. H., Sciare, J., Gros, V., and Albinet, A.: Six-year source apportionment of submicron organic aerosols from near-continuous highly time-resolved measurements at SIRTA (Paris area, France), Atmos. Chem. Phys., 19, 14755–14776, https://doi.org/10.5194/acp-19-14755-2019, 2019.
- Abstract
- Introduction
- Methodology
- Phenomenology of fine-aerosol chemistry in French urban environments
- Comparison between observations and the CHIMERE CTM
- Data availability
- Conclusions
- Author contributions
- Competing interests
- Disclaimer
- Acknowledgements
- Financial support
- Review statement
- References
- Supplement
- Abstract
- Introduction
- Methodology
- Phenomenology of fine-aerosol chemistry in French urban environments
- Comparison between observations and the CHIMERE CTM
- Data availability
- Conclusions
- Author contributions
- Competing interests
- Disclaimer
- Acknowledgements
- Financial support
- Review statement
- References
- Supplement