the Creative Commons Attribution 4.0 License.
the Creative Commons Attribution 4.0 License.
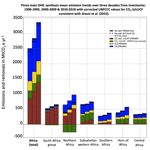
Greenhouse gas emissions and their trends over the last 3 decades across Africa
Mounia Mostefaoui
Philippe Ciais
Matthew J. McGrath
Philippe Peylin
Prabir K. Patra
Yolandi Ernst
A key goal of the Paris Agreement (PA) is to reach net-zero greenhouse gas (GHG) emissions by 2050 globally, which requires mitigation efforts from all countries. Africa's rapidly growing population and gross domestic product (GDP) make this continent important for GHG emission trends. In this paper, we study the emissions of carbon dioxide (CO2), methane (CH4) and nitrous oxide (N2O) in Africa over 3 decades (1990–2018). We compare bottom-up (BU) approaches, including United Nations Convention Framework on Climate Change (UNFCCC) national inventories, FAO, PRIMAP-hist, process-based ecosystem models for CO2 fluxes in the land use, land use change and forestry (LULUCF) sector and global atmospheric inversions. For inversions, we applied different methods to separate anthropogenic CH4 emissions. The BU inventories show that, over the decade 2010–2018, fewer than 10 countries represented more than 75 % of African fossil CO2 emissions. With a mean of 1373 Mt CO2 yr−1, total African fossil CO2 emissions over 2010–2018 represent only 4 % of global fossil emissions. However, these emissions grew by +34 % from 1990–1999 to 2000–2009 and by +31 % from 2000–2009 to 2010–2018, which represents more than a doubling in 30 years. This growth rate is more than 2 times faster than the global growth rate of fossil CO2 emissions. The anthropogenic emissions of CH4 grew by 5 % from 1990–1999 to 2000–2009 and by 14.8 % from 2000–2009 to 2010–2018. The N2O emissions grew by 19.5 % from 1990–1999 to 2000–2009 and by 20.8 % from 2000–2009 to 2010–2018. When using the mean of the estimates from UNFCCC reports (including the land use sector) with corrections from outliers, Africa was a mean source of greenhouse gases of Mt CO2 eq. yr−1 from all BU estimates (the subscript and superscript indicate min–max range uncertainties) and of Mt CO2 eq. yr−1 from top-down (TD) methods during their overlap period from 2001 to 2017. Although the mean values are consistent, the range of TD estimates is larger than the one of the BU estimates, indicating that sparse atmospheric observations and transport model errors do not allow us to use inversions to reduce the uncertainty in BU estimates. The main source of uncertainty comes from CO2 fluxes in the LULUCF sector, for which the spread across inversions is larger than 50 %, especially in central Africa. Moreover, estimates from national UNFCCC communications differ widely depending on whether the large sinks in a few countries are corrected to more plausible values using more recent national sources following the methodology of Grassi et al. (2022). The medians of CH4 emissions from inversions based on satellite retrievals and surface station networks are consistent with each other within 2 % at the continental scale. The inversion ensemble also provides consistent estimates of anthropogenic CH4 emissions with BU inventories such as PRIMAP-hist. For N2O, inversions systematically show higher emissions than inventories, on average about 4.5 times more than PRIMAP-hist, either because natural N2O sources cannot be separated accurately from anthropogenic ones in inversions or because BU estimates ignore indirect emissions and underestimate emission factors. Future improvements can be expected thanks to a denser network of monitoring atmospheric concentrations. This study helps to introduce methods to enhance the scope of use of various published datasets and allows us to compute budgets thanks to recombinations of those data products. Our results allow us to understand uncertainty and trends in emissions and removals in a region of the world where few observations exist and where most inventories are based on default IPCC guideline values. The results can therefore serve as a support tool for the Global Stocktake (GST) of the Paris Agreement. The referenced datasets related to the figures are available at https://doi.org/10.5281/zenodo.7347077 (Mostefaoui et al., 2022).
- Article
(17582 KB) - Full-text XML
-
Supplement
(21363 KB) - BibTeX
- EndNote
Large global reductions in greenhouse gas (GHG) emissions are needed to avoid “dangerous anthropogenic interference with the climate system” (IPCC, 2021). The Paris Agreement (PA) aims at limiting global warming below 2 ∘C and reaching net-zero GHG emissions by 2050. To improve the monitoring of emission trends, the PA has an Enhanced Transparency Framework (ETF) by which countries will have to report their GHG emissions and removals under a standardized format starting in 2024 (Perugini et al., 2021; UNFCCC, 2021) through Biennial Transparency Reports (BTRs), with the aim of using up-to-date data and best-available science to improve national inventories. This represents a challenge for many developing countries, where emission inventories have been irregular.
Recent analyses predict a fast increase in African emissions correlated with demographic growth. The African population is expected to double from 1.2 billion in 2019 to 2.5 billion at the 2050 horizon (UN, 2019). Using the TIAM-ECN Integrated Assessment Model (IAM) developed with data from the International Energy Agency (IEA), van der Zwaan et al. (2018) concluded that GHG emissions from Africa will become substantial at the global scale by 2050. In shared socioeconomic pathway (SSP) projection scenarios, Africa and the Middle East are grouped together despite having very different geographies, per capita emissions and gross domestic products (GDPs) (IIASA, 2017). According to IAM projections, the minimum projected share of Africa in global emissions would be close to 10 % by 2050 for a business-as-usual pathway. An “explosive growth in African combustion emissions”' (Liousse et al., 2014) could not be excluded from 2030 to 2050 if no drastic mitigation policies are implemented (IPCC, 2021). If a stringent emission reduction pathway limiting global warming to +2 ∘C is adopted, Africa could contribute around 20 % of global emissions by 2050, becoming the second largest worldwide emitting region. Further, under stringent climate policy scenarios, CH4 and N2O emissions in Africa were projected to contribute 80 % of the total emissions of these two gases in 2050 (van der Zwaan et al., 2018). Therefore, Africa will become an important global emission contributor under any mitigation pathway with a demographic and industrial development increase.
There are 56 African countries represented in the United Nations. National emission reports to the United Nations Convention Framework on Climate Change (UNFCCC) are available for 53 countries, including all major African emitters. Africa as a whole ranks fifth worldwide in terms of territorial fossil fuel use with a total of 1449 Mt CO2 eq., in between the Russian Federation and Japan (Friedlingstein et al., 2020). The global share of Africa is ∼4 % of fossil CO2 (FCO2) emissions, ∼16 % of CH4 emissions (Saunois et al., 2020) and ∼25 % of N2O emissions (Tian et al., 2020). South Africa is the biggest FCO2 emitter on the continent and ranks twelfth on the global scale, just after Brazil.
Despite projections of strong growth of emissions and population in Africa, the continent is understudied and lacks up-to-date comprehensive assessments of GHG emissions and removals, given sporadic and often outdated reports by individual countries. The literature tends to be scarce on African countries, and their emissions have rarely been analyzed comprehensively using the results from both statistical inventories that are also referred to as bottom-up (BU) methods and top-down (TD) atmospheric inversions. Country reports estimate GHG emissions through statistical inventories using estimates of national sectoral activity data multiplied by emission factors, with three levels of refinements depending on countries, named Tier 1 for default emission factors, Tier 2 for country-specific emission factors or activity data and Tier 3 for more emission factors or activity with tailored representation at the scale of the process. Other BU inventories for assessing national emissions also exist: they are based on the same approach as country-reported inventories but use their own parameters for activity data and emission factors coming from research groups, international statistical agencies, etc. Process-based ecosystem models developed by the research community are not used by countries. They are based on the representations of complex ecosystem processes and can also be viewed as a BU method. In addition, another approach is named “top-down” and refers to atmospheric inversions. Inversions consist in estimating causes (emissions and sinks) based on consequences (concentrations). The inverse modeling approach consists in adjusting a priori fluxes to the atmospheric transport in order to be as adjusted as possible with observation data by minimizing a cost function. This is a mathematically complex problem under-constrained because every point of the globe is an unknown emission, and there are only a limited number of observations: “regularization” techniques are used to find a unique solution. The African ground-based atmospheric network used by inversions is very sparse. There are only three currently active surface flasks over this whole continent, located in Namibia (Gobabeb), in the Seychelles (Mahé) and in South Africa (Cape Point). The one in Algeria (Assekrem) was terminated on 26 August 2020, and the one in Kenya has been inactive since 21 June 2011. The characteristics of the surface flasks in Africa available on the NOAA website are summarized in Table S1 in the Supplement. Inversion results are therefore uncertain due to this small number of atmospheric stations over the continent (Nickless et al., 2020).
A previous analysis of African emissions was solely focused on FCO2 emissions during the decade 2000–2009 (Canadell et al., 2009). A first budget for the period 1990–2009 was provided at the continental scale with the RECCAP1 project (Valentini et al., 2014). Ayompe et al. (2020) studied recent FCO2 emission trends using IEA data. Other studies are region-specific or sector-specific, focusing exclusively on agriculture (Bombelli et al., 2009), on natural ecosystems in sub-Saharan Africa (Kim et al., 2016) or on individual countries such as Kenya (Zhu et al., 2018).
Paying attention not only to commonly identified big emitters like South Africa but also to medium emitters and emerging emitters is important, not only in terms of scientific assessment but also for financial and climate policy purposes under the PA. The monitoring, reporting and verification (MRV) provisions of the PA indeed require scientific and policy tools to verify the pledges made by all the signatory countries. Instruments for financial transfers for mitigation and adaptation like the Green Fund on Climate Change (GCF) and the REDD+ initiatives cover the African scope and will require scientific assessment of trends for impact evaluation and credibility purposes and as an incentive for continued investments. As part of the Global Stocktake (GST) under article 14 of the PA aiming at assessing “collective progress”, all the signatory parties will have to show their contributions to global mitigation efforts. These efforts will be evaluated within an MRV system which includes the requirement for developing countries to submit their Biennial Update Reports (BURs) on a biennial basis starting in 2024. As no standard global reporting framework has been required to date, we anticipate that the data available for the first stocktake in 2023 will be very heterogeneous. As a continent that includes non-Annex I countries exclusively, the African case is characterized by the scarcity of national official inventories, which have been provided to date on a voluntary basis through the National Communication (NC) and BURs. BU estimates of emissions established by independent scientific methods are also discussed in the present study. In this context, different and complementary observation-based methods assessing national GHG emissions and sinks are needed.
The aim of this paper is to evaluate the relative merits of different existing types of datasets for the assessment of African emissions and removals and their trends for CO2, CH4 and N2O during the last 3 decades. In this paper, we standardize the metrics and the scope of application for different categories of GHG emissions to discuss budgets. We also validate and benchmark different independent datasets to evaluate the possibility of using them as a verifying tool for official country-reported data. In order to cover all the GHG sectors, we also describe recombinations of different historical datasets for the last 30 years that are necessary for filling the gap for some missing past sectoral emissions. This study offers a comparison of data products originally combined to compute a budget and an evaluation of their relative merits. The different data products discussed here include different BU approaches, including official country communications to the UNFCCC and estimations from the Food and Agriculture Organization (FAO), the Carbon Dioxide Information Analysis Center (CDIAC), global inventories for anthropogenic emissions (PRIMAP-hist, which integrates combinations of various datasets including FAO and the Global Carbon Project GCP) and process-based models for land CO2 fluxes with 14 dynamic general vegetation models (DGVMs) from the TRENDY version 9 ensemble (Table 1). We also analyze and combine TD products to discuss individual gases and to compute budgets: 3 atmospheric global inversions for CO2 land fluxes, 22 inversions for CH4 emissions (11 inversion models using surface station data and 11 satellite inversion models) and CH4 wildfire emissions from the Global Fires Emission Dataset (GFED) version 4. We used three inversion models for N2O fluxes (the PyVAR model, TOMCAT-INVICAT model and MIROC4-ACTM model; see Table 1). Inversions only solve for total fluxes or at best for groups of sectors, whereas BU estimates have a larger number of sectors. In Table 2, we present the correspondence between “sectors” defined by the TD and BU methods. For all the datasets, we chose an atmospheric convention with negative values representing removals from the atmosphere (i.e., a land sink). We deliver an original comparison of BU estimates from national inventories, global inventories and process-based models, with TD estimates from atmospheric inversions over Africa. The work is carried out for large countries or groups of small countries, as inversions do not have the capability to constrain fluxes over small areas given their coarse grid and sparse atmospheric data. Based on the benchmarking and relative merit evaluation of the various data products presented above, the scientific questions addressed in this study are the following. (1) How consistent are the mean values and trends of GHG emissions across BU estimates in Africa? (2) How consistent are the different inversion model results? (3) How do inversions compare with BU estimates? (4) What is the net GHG balance of the African continent from different observation-based methods, including CO2 sinks and sources in the land use sector? (5) What are the main sources of uncertainties?
The paper is organized into two main sections. First, a material and methods section describes the regional breakdown and input data (Sect. 1). We present our results for the whole of Africa and for six groups of aggregated countries (Sect. 2) with a specific analysis of CO2 emissions and sinks, divided between FCO2 (Sect. 2.1), fluxes in the land use, land use change and forestry (LULUCF) sector (Sect. 2.2) and emissions of non-CO2 greenhouse gases (Sect. 2.3 and 2.4). Conclusions are drawn about uncertainties in African GHG net emissions and removal assessment.
This study covers the period from 1990 to 2018 as well as emissions and sinks of CO2, CH4 and N2O. We used 1990 as a base year since reporting to the UNFCCC mostly started in that year and is often used as a reference comparison year in national pledges of the PA. The last year of analysis is 2018, reflecting the availability of inversion data and avoiding further uncertainty due to poorly understood emission changes before and after the COVID-19 crisis. This period allows the analysis of decadal features. It also has the advantage of being covered by several datasets listed in Table 1. We considered different BU approaches, including official country communications to the UNFCCC and estimations from the FAO, global inventories for anthropogenic emissions (PRIMAP-hist, which integrates combinations of various datasets, including FAO, GCP, EDGAR v4.3.2, Andrew 2018 cement data, BURs, Common Reporting Format (CRF), UNFCCC data, and BP) and process-based models for land CO2 fluxes with 14 DGVMs from the TRENDY version 9 ensemble (Table 1). We used three atmospheric global inversions for CO2 land fluxes, 22 inversions for CH4 emissions and three inversions for N2O fluxes (Table 1). For preliminary data quality control, we checked the consistency of prior fluxes by plotting them separately (Fig. S1 in the Supplement). Inversions only solve for total fluxes or at best for groups of sectors, whereas BU estimates have a larger number of sectors. In Table 2, we present the correspondence between the “sectors” defined by the TD and BU methods. For all the datasets, we chose an atmospheric convention with negative values representing removals from the atmosphere (i.e., the land sink). No specific standard guidelines currently exist to define uncertainties in the BU and TD data products. Given that some of our estimates are based on a small number of models and estimates, we cannot calculate the full distribution, e.g., with a 95 % confidence interval, but we rather reported ranges with minima and maxima. Assuming that the unknown distributions are Gaussian, like in Schulze et al. (2018), we could infer a 2σ (≈95 %) confidence interval if we assume that the minima and maxima are equivalent to 3σ, but in view of the small numbers of estimates, e.g., for N2O with only three inversions, we prefer to just give the min–max range. Moreover, for national inventories, as all African countries are non-Annex I, they do not deliver confidence intervals, but Grassi et al. (2022) estimated for CO2 LULUCF flux uncertainties of 50 % for the average of non-Annex-1 countries. Here, uncertainty estimates are understood as the spread among minimum and maximum values from one methodology. The main source of uncertainty in the comparison of country-reported data with other data products is the inclusion or not of natural fluxes in addition to anthropogenic emission sectors. For the comparability of the different data products presented in this study, we only discuss the mean value over the period of overlapping data availability. The referenced datasets are available at https://doi.org/10.5281/zenodo.7347077 (Mostefaoui et al., 2022).
Table 1List of the BU and TD methods used. For more details, see also Saunois et al. (2020) for CH4, Friedlingstein et al. (2020) for FCO2, UNFCCC country-reported data and Gütschow et al. (2021) for PRIMAP-hist.
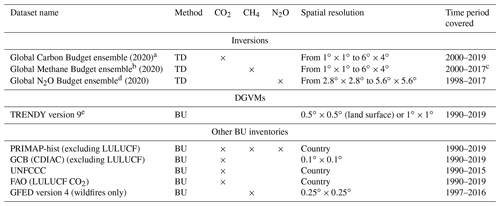
a See the three inversion details in Table S6. b See the 22 inversion details in Table S7. c Variations from 2003–2015, 2000–2015 and 2010–2017: see the detailed period coverage for each dataset in Table S7. d See the three inversion details in Table S8. e See Table S5 for the 14 products.
2.1 Regional breakdown
As some countries are small emitters and their area is too small to be resolved by inversions and in some cases even by DGVMs, we grouped African countries into six regions shown in Fig. S2 and listed in Table S2. The grouping followed national borders and biome similarity considering Köppen–Geiger climate zones (Beck et al., 2018), magnitudes of fossil fuel emissions and per capita emissions (Figs. S2, S3 and S8). We also grouped a maximum of about 10 countries per region.
2.2 Inventory datasets
2.2.1 PRIMAP-hist anthropogenic emission assessment for CO2, CH4 and N2O
The PRIMAP-hist version 2.2 BU dataset is derived from Gütschow et al. (2021) and combines UNFCCC reports with a gap-filling method to produce a time series of annual anthropogenic emissions for different IPCC sectors. PRIMAP-hist does not cover the LULUCF sector for CO2 due to the high uncertainties. PRIMAP-hist does not include emissions from shipping and international aviation but includes cement as part of the FCO2 emissions. We use data from the HISTCR scenario (data accessed from https://www.pik-potsdam.de/paris-reality-check/primap-hist/, last access: 24 April 2022) from the country-prioritized dataset, which mainly uses UNFCCC (BUR and NC) data unless such data are missing, in which case PRIMAP-hist uses extrapolated data from EDGAR, FAO and the BP Statistical Review of World Energy in their 2021 versions as described in Gütschow et al. (2021).
2.2.2 Global Carbon Project (GCP) fossil CO2 emissions
We used country-level FCO2 data published by the global CO2 budget by the GCP (Friedlingstein et al., 2020) separated per fuel type (gas, oil and coal) and including fossil fuel use in the combined industry, ground transportation and power sectors, natural gas flaring, cement production and process-related emissions (e.g., fertilizers and chemicals). Data for African countries come among others from the CDIAC compiled until 2018 (Gilfillan and Marland, 2021), the BP Statistical Review of World Energy (BP, 2020) and recent estimates of cement production and clinker-to-cement ratios (Andrew, 2020).
2.2.3 UNFCCC inventories for CO2 in the LULUCF sector
We used UNFCCC submissions for LULUCF CO2 fluxes from NC and BURs downloaded from the UNFCCC website (https://unfccc.int/, last access: 30 March 2022) in March 2021, and these were further processed into .csv tables by Deng et al. (2022). Those estimates are based on different accounting methods following IPCC guidelines (IPCC, 2019). Country-reported data quality control (QC), quality assurance (QA) and verification processes follow the 2019 IPCC guidelines detailed in the Chapter 6 QA and QC procedures of this document. African countries, being non-Annex I countries, do not report emissions every year. Figure 1 shows the number of BURs and NCs provided each year per African region. The years 1990, 1994, 1995, 2000 and 2005 are characterized by several updates, while most of the other years have few updates. About every 2 years, all the regions have at least one update. Note that flexibility for BURs is given to least developed countries (LDCs), which include 33 out of 56 African countries, and to small island developing states (SIDSs), which include 6 African countries (Table S4).
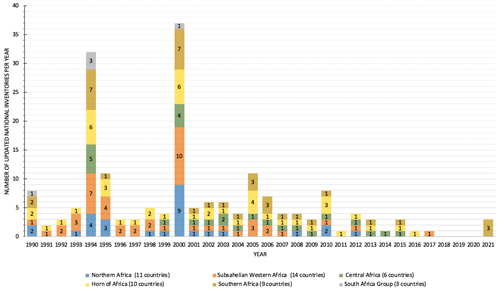
Figure 1Number of UNFCCC reports for LULUCF CO2 fluxes in the National Communications and Biennial Update Reports per group of countries defined in Table S2.
Non-Annex I African countries can use older versions of the IPCC guidelines (IPCC, 2006). This induces uncertainties from changes in accounting methods between versions, with recent guidelines having more detailed sectors and sources. There are no data for Libya, Equatorial Guinea, Malawi and Sierra Leone during the whole period. UNFCCC data are missing in some years for Rwanda, Sao Tome and Principe, Senegal, South Sudan and Angola. There are no data for 1990–1998 for Liberia.
We noticed that NCs and BURs lack details regarding the methods used and the sources of activity data and emission factors, and most of them are in the French language. BURs in .pdf format include a non-standardized table for emissions. The reader is sometimes referred to the “national coordinator for climate change service” with no link to any database or contact person.
Because the PA targets human-induced emissions, countries use the proxy of “managed lands” for the LULUCF sector as defined by the IPCC guidelines (IPCC, 2019). Managed lands are areas where LULUCF CO2 fluxes are assigned to some anthropogenic activities. Several African NCs and BURs do not contain information on their managed land areas. We thus looked at REDD+ national reports (https://redd.unfccc.int/submissions.html?topic=6; last access: 13 August 2022, REDD+, UNFCCC, 2022) to get this information (Fig. S3 and Table S9). LULUCF CO2 fluxes on managed lands result from either direct anthropogenic effects such as land use change and forestry or from indirect effects (such as change in CO2 and climate) on land remaining in the same land use, e.g., forest remaining forest (Grassi et al., 2022). The vast majority of African countries use a Tier 1 IPCC accounting method which does not distinguish between these different effects. Tier 1 methods use a classification with only three out of six possible types of land, “forest land”, “cropland” and “grassland”, and do not give spatially explicit land use data. Tier 2 methods include fluxes from six land use types: forest, cropland and grassland, wetlands and urban and other land use for the case of land remaining under the same land use type and for the case of conversions between land use types. In Africa, only South Africa and Zambia used Tier 2 methods for some LULUCF CO2 subsectors.
2.2.4 Processing of the UNFCCC LULUCF CO2 data and outlier corrections
We processed the UNFCCC LULUCF CO2 data for outlier corrections (Table S5). For Guinea-Bissau and Tanzania, we identified inconsistent values from successive communications with substantially differing numbers. For Guinea, Madagascar, Zimbabwe, Congo, Mali, the Central African Republic (CAF), Angola and Mauritius, we identified changes of more than 1 order of magnitude between two consecutive reports and likely implausibly large carbon sinks considering their national forest area. The computations of per area emissions and removals showed discrepancies, which points to the need for further examination and inspection of more recent reports in NDC and REDD+ reports (Table S5). Our corrections explained in the Supplement are consistent with those proposed by Grassi et al. (2022), who diagnosed “biophysically impossible” sequestration rates with a threshold value larger than 10 tCO2 ha−1 yr−1 over an area greater than 1 Mha. For Namibia, Nigeria and the Democratic Republic of the Congo (DRC), it was challenging to select a best estimate between recent and past reports. For those countries, corrections using more recent data than BURs or NCs have high uncertainties, as noted by Grassi et al. (2022). This includes the absence of any sink for the DRC for instance, in contrast to sinks consistently reported over time and large forested areas in this country's previous reports to the UNFCCC. We therefore systematically looked at corrected values for both case scenarios (with and without Namibia, Nigeria and DRC data corrections). In total, we corrected 13 outliers as shown in Table S5, consistent with Grassi et al. (2022).
2.2.5 FAO LULUCF CO2 fluxes
We used data from LULUCF CO2 fluxes over 1990–2019 from the FAO Global Forests Resource Assessments (FAOSTAT, 2021). According to the 2005 FAO categories and definitions, forest is land covering at least 0.5 ha and having vegetation taller than 5 m with a canopy cover higher than 10 %. Other wooded lands refer to land that is not classified as “forest” but is wider than 0.5 ha, that has a canopy cover of 5 %–10 % or that combines trees, shrubs and bushes with a cover higher than 10 %. The FAO data for forests comprise carbon stock changes from both aboveground and belowground living biomass pools. They are independent of country-reported UNFCCC emissions and removals. The FAO estimates are based on activity data, areas of forest land and CO2 emissions and removal factors. The FAO data report (1) net emissions and removals from “forest land remaining forest land” and from “land converted to forest” grouped together, together with (2) emissions from “net forest conversion”, i.e., deforestation. In contrast, the UNFCCC accounting uses a 20-year window for CO2 fluxes from land use change, while land use change fluxes from “land converted to forest” are reported separately from those of “forest remaining forest”.
2.3 DGVM datasets
We used the net biome productivity (NBP) from 14 DGVMs from the TRENDY version 9 ensemble covering the period 1990–2019. The different models described in Friedlingstein et al. (2019) are CABLE, CLASS, CLM5, DLEM, ISAM, JSBACH, JULES, LPJ, LPX, OCN, ORCHIDEE-CNP, ORCHIDEE-SDGVM and SURFEX (Table S6). The DGVMs are forced by historical reconstructions of land cover change, atmospheric CO2 concentration and climate since 1901. Detailed cropland management practices are generally ignored, except for the harvest of crop biomass. Forest harvest is prescribed from historical statistics in 11 models (Table A1 of Friedlingstein et al., 2020). The models simulate carbon stock changes in biomass, litter and soil pools. From the difference between simulations with and without historical land cover change, a flux called “land use emissions” can be obtained from the DGVMs. This flux includes the indirect effects of climate and CO2 on lands affected by land use change and a foregone sink called “loss or gain of atmospheric sink capacity”, which is absent from the methods used by UNFCCC and FAO. Pongratz et al. (2014) delivered the following definition of loss of sink capacity as “the CO2 fluxes in response to environmental changes on managed land as compared to potential natural vegetation. Historically, the potential natural vegetation would have provided a foregone sink as compared to human land use.” Thus, land use change fluxes from DGVMs were not compared with other estimates. Note that DGVMs do not explicitly separate managed and unmanaged land. Thus, we used all the forest lands to calculate their mean CO2 fluxes.
2.4 Atmospheric inversion datasets
2.4.1 CO2 inversions
We used the net land CO2 fluxes, excluding fossil fuel emissions (hereafter net ecosystem exchange) from three global inversions of the Global Carbon Project that cover a long period (see Table A4 of Friedlingstein et al., 2020), including CarbonTrackerEurope (CTRACKER-EU-v2019; van der Laan-Luijkx et al., 2017), the Copernicus Atmosphere Monitoring Service (CAMSV18-2-2019; Chevallier et al., 2005) and one variant of Jena CarboScope (JENA, sEXTocNEET_v2020; Rödenbeck, 2005). The GCP inversion protocol recommends using as a fixed prior the same gridded dataset of FCO2 emissions (GCP-GridFED). However, some modelers used different interpolations of this dataset, and one group used a different gridded dataset (Ciais et al., 2022). We applied a correction to the estimated total CO2 flux by subtracting a common FCO2 flux from each inversion (Fig. S1 and the Methodological Supplement S1). The resulting land–atmosphere CO2 fluxes, or net ecosystem exchange, cannot be directly compared with inventories aiming to assess C stock changes, given the existence of land–atmosphere CO2 fluxes caused by lateral processes. This issue was discussed by Ciais et al. (2022), and a practical correction of inversions was proposed by Deng et al. (2022) based on new datasets for CO2 fluxes induced by lateral processes involving river transport, crop and wood product trade. Here we applied the same correction to all the CO2 inversions.
2.4.2 CH4 inversions
We used the CH4 emissions from global inversions over 2000–2017 from the Global Methane Budget (Saunois et al., 2020) (Table 1). This ensemble includes 11 models using GOSAT satellite CH4 total-column observations covering 2010–2017 and 11 models assimilating surface station data (SURF) since 2000 (Table S5). Surface inversions are constrained by very few stations for Africa, while the GOSAT satellite data have a better coverage. One could thus expect GOSAT inversions to give more robust results. Inversions deliver an estimate of surface net CH4 emissions, although some of them solve for fluxes in groups of sectors called “super-sectors”. We have not used in situ dataset validation per se: only the GOSAT data were evaluated against the Total Carbon Column Observing Network (TCCON) independent ground-based total column-averaged abundance of CH4 (XCH4). In the inversion dataset, net CH4 surface emissions were interpolated to a resolution, regridded from coarser-resolution fluxes and separated into super-sectors using either prior emission maps or posterior estimates for those inversions solving fluxes per super-sector following Saunois et al. (2020). More specifically, these five super-sectors are (1) Fossil Fuel, (2) Agriculture and Waste, (3) Wetlands, (4) Biomass and Biofuel Burning (BBUR) and (5) Other natural emissions. We separated CH4 anthropogenic emissions from inversions using Method 1 and Method 2 proposed by Deng et al. (2022). Method 1 relies on the separation calculated by each inversion except for the BBUR super-sector from which wildfire emissions were subtracted based on GFED version 4 (van der Werf et al., 2017). Method 2 removes from total emissions the median of natural emissions from inversions (Deng et al., 2022). The two methods gave similar results, and only Method 1 was used in the Results section.
2.4.3 N2O inversions
We used three N2O atmospheric inversions from the global N2O budget synthesis (Tian et al., 2020) and from Deng et al. (2022) (Tables S1 and S7): PyVAR CAMS (Thomson et al., 2014), MATCM_JMASTEC (Rodgers, 2000; Patra et al., 2018) and TOMCAT (Wilson et al., 2014; Monks et al., 2017). We used the total N2O flux from inversions including natural emissions, given that natural emission estimates are highly uncertain for Africa. Inversion results are therefore not directly comparable to the PRIMAP-hist inventory, which only contains anthropogenic emissions.
2.5 Metrics to compare gases and ancillary data and data usage
We express emissions of non-CO2 gases in megatons of carbon dioxide equivalent (MtCO2e) using the global warming potential over 100-year time horizon (GWP100) values from the fourth IPCC Assessment Report (IPCC, 2007), consistent with PRIMAP-hist and historical country-reported data. We used AR4 GWP100 because many African countries have been following the 2006 IPCC guidelines referring to AR4 GWP100 2019 refinement in IPCC guidelines, which do not recommend any specific metrics, and therefore we are following the IPCC guidelines used by countries. The multiplicative coefficients to change AR4 to AR6 GWP100 values are 1.19 for fossil CH4, 1.09 for non-fossil CH4 and 0.92 for N2O. We used population data from the United Nations population (United Nations Department of Economic and Social Affairs, Population Division, 2019) to compute per capita FCO2 emissions and their disparities based on Gini indices (Dorfman et al., 1979) to measure statistical dispersions among a given population (Methodological Supplement S2). We also used African GDP data (World Bank, 2019).
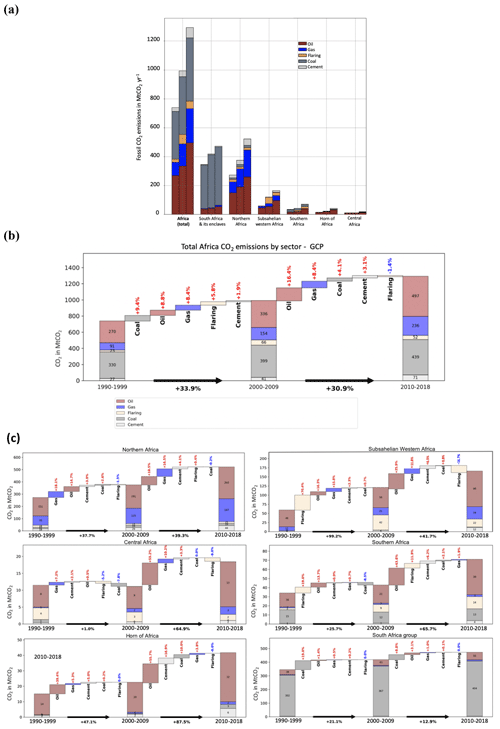
Figure 2(a) African fossil fuel CO2 emissions per fuel type and for cement per region over 1990–1999, 2000–2009 and 2010–2018. (b) The contribution of each fuel type to the change in African emissions. (c) The same for different regions, regrouping several countries. Data are from GCP (2019).
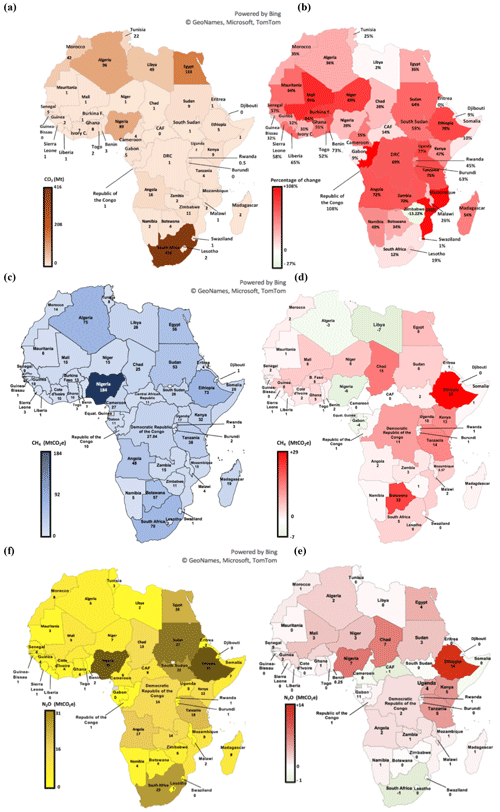
Figure 3(a) Maps of average fossil fuel CO2 emissions for African countries during 1999–2008 (Mt CO2 eq. yr−1) (GCP) and (b) the change from 1999–2008 to 2009–2018 using data from GCP (Friedlingstein et al., 2019) (Mt CO2 eq. yr−1) expressed as the ratio of the differences in percentage between the FCO2 for the 1999–2008 total in anthropogenic mean 2009–2018 emissions and mean 1999-2008 emissions (GCP) divided by the average value for both decades. (c–d) The same but with anthropogenic CH4 emissions from PRIMAP-hist and the differences between the CH4 mean emissions over 1999–2008 and over 2009–2018 (Mt CO2 eq. yr−1). (e–f) The same for anthropogenic N2O emissions from PRIMAP-hist (Mt CO2 eq. yr−1).
3.1 Fossil CO2 emissions
3.1.1 Continental, regional and country changes
PRIMAP-hist and GCP
First, we compared GCP and PRIMAP-hist fossil CO2 emissions. We found that most of the relative differences between these two datasets at country level considerably decreased with time, except for Mali. Those differences are less than 5 % for most of the main African emitters during the last decade, except for South Africa, where the difference is a bit larger than 10 % (see the maps in Fig. S8). The largest relative difference between the two datasets comes from Mali in the decade 2009–2018, with FCO2 emissions of 3 Mt CO2 yr−1 in GCP compared to 1 Mt CO2 yr−1 in PRIMAP-hist. Given the relatively small differences, we chose to use only GCP for trends between decades, but when computing net budgets for the three main GHGs, we show differences between the use of those two estimates.
The changes in African FCO2 emissions per fuel type and for cement using the GCP data are shown in Fig. 2a. In Fig. 2b, we show absolute values and relative contributions to the total change in each decade. During 2010–2018, total African FCO2 emissions from oil (497 Mt CO2 yr−1) and coal (439 Mt CO2 yr−1) were roughly similar. While global FCO2 emissions increased by +13 % over this period (Friedlingstein et al., 2019), African FCO2 almost doubled in 2018 compared to 1990 levels, a relative increase comparable to that of China over the same period. From 1990–1999 to 2000–2009, the mean emissions increased by 33.9 % from 741 to 996 Mt CO2 yr−1. All FCO2 sectors contributed to this decadal increase. The contribution from coal (+9.4 %) was slightly larger but comparable to that from oil (+9 %) and gas (+8 %). From 2000–2009 to 2010–2018, emissions further increased by 31 % from 996 to 1295 Mt CO2 yr−1. The oil and gas fuels contributed the most to this increase, with +16 % for oil and +8 % for gas. Coal emissions increased by only +4.1 %, and coal went from being the first source of African FCO2 emissions over 2000–2009 to the second one over 2010–2018.
As for the regional contributions to emission changes between 1990–1999 and 2000–2009 shown in Fig. 2b, the main contribution to the total increase came from the region of South Africa, where emissions increased from 302 to 367 Mt CO2 yr−1 (+21.1 %, coal being the largest contributor). The second largest contribution to the increase is from northern Africa, where oil was the largest contributor (emissions increased from 151 to 191 Mt CO2 yr−1; +15 %) together with gas (+18 %). The least increasing region was central Africa. Northern Africa experienced the largest increase from 1990–1999 to 2000–2009 and from 2000–2009 to 2010–2018 with successive increases of +38 % and +39 %, largely dominated by oil and gas (Fig. 4b). As a result, during the period 2010–2018, northern African countries were the dominant emitters with 545 Mt CO2 yr−1. The group of South Africa (including Lesotho and Botswana) was the second biggest emitter region over 2010–2018, mainly due to coal emissions from the Republic of South Africa. The two least contributing African regions were the Horn of Africa and central Africa.
At the country level, Fig. 3a–b show mean FCO2 emissions and relative changes over the last 2 decades. The main emitters do not have the biggest relative changes. The four main emitters over 2000–2009 were South Africa (416 Mt CO2 yr−1), Egypt (153 Mt CO2 yr−1), Algeria (96 Mt CO2 yr−1) and Nigeria (89 Mt CO2 yr−1). Those four countries altogether represented 67 % of the continental total emissions over 2000–2009 (987 Mt CO2 yr−1). The largest relative increases from 2000–2009 to 2010–2018 are from Congo (+108 %), Mozambique (+103 %) and Mali (91 %) compared to relative increases from the main emitters, the Republic of South Africa (+21 %), Egypt (+36 %) and Algeria (+36 %).
3.1.2 Variations of per capita and per GDP fossil fuel CO2 emissions
Per capita emissions
Using ancillary data on population (Figs. S3 and S4), we computed the mean African per capita emission of 1 tCO2 per capita per year for 2009–2018, which is 5 times larger than during 1990–1998 (0.2 tC per capita per year) and yet 5 times smaller than the global average (5 tCO2 per capita per year). From 1999–2008 to 2009–2018, African per capita emissions increased by 30 %. African per capita FCO2 emissions during 2009–2018 were 17 times less than in the USA (17 tCO2 per capita per year), 7 times less than in China (7 tCO2 per capita per year), 7 times less than in the EU27 and UK (7 tCO2 per capita per year) and 2 times less than in India (2 tCO2 per capita per year). At the country level, the biggest per capita emissions over 2009–2018 were from the Republic of South Africa with 9 tCO2 per capita per year, which ranks 14th worldwide, above China and just below Poland. The second biggest per capita emissions were from Libya (8 tCO2 per capita per year). The smallest ones were from the DRC (0.1 tCO2 per capita per year). For the first period, 1990–1998, per capita emissions of the African region ranked in this order: South African group (4 tCO2 per capita per year) > northern Africa (2 tCO2 per capita per year) > central African countries (1 tCO2 per capita per year) > southern countries (0.8 tCO2 per capita per year) > Horn of Africa (0.5 tCO2 per capita per year) > sub-Sahelian western Africa (0.3 tCO2 per capita per year). For the second period, 2009–2018, they ranked in this order: South African group (4 tCO2 per capita per year) > northern Africa (2 tCO2 per capita per year) > southern countries (1 tCO2 per capita per year) Horn of Africa (1 tCO2 per capita per year) > central African countries (1 tCO2 per capita per year) > sub-Sahelian western Africa (0.4 tCO2 per capita per year). At the country scale during the first period of 1990–1998, the four African largest per capita emissions ranked in this order: Libya (9 tCO2 per capita per year > Republic of South Africa (9 tCO2 per capita per year) > Gabon (5 tCO2 per capita per year) > Algeria (3 tCO2 per capita per year). The four African countries with the smallest per capita emissions ranked as follows: Burundi (0.04 tCO2 per capita per year) < Uganda, Ethiopia and Mali (0.1 tCO2 per capita per year).
We also computed the Gini index for African per capita FCO2 emissions for each of the last 3 decades, using data from Friedlingstein et al. (2020) (see Methodological Supplement S2). These Gini values were 0.7 for 1990–1998, 0.7 for 1999–2008 and 0.7 for 2009–2018 and thus were very stable over the last 30 years and close to 1, indicating high inequities among countries.
Emissions per GDP
Per exchange rate vs. per purchasing power parity (PPP) GDP
According to the International Monetary Fund (IMF), the GDP delivers an estimate “of the monetary value of goods and services produced in a country over a chosen period.” GDP data from the World Bank (2019) are available for 30 African countries only (Fig. S5). The four countries with the biggest GDP expressed in US Dollars (exchange rate GDP) (Fig. S6) are Nigeria (USD 490B) > South Africa (USD 350B) > Egypt (USD 330B) and Algeria (USD 330B) > Angola (USD 120B). The four countries with the smallest GDP in 2015 are Gambia (USD 1.4B) and Seychelles (USD 1.4B) > Guinea-Bissau (USD 1B) > Comoros (USD 970M). Emissions per USD GDP are shown in Fig. S6. The PPP calculated by the International Comparison Program (ICP) of the World Bank is a refined measure of what a given national currency can acquire in terms of goods or services in another country, removing the impact of currency exchange rates. Emissions per PPP USD GDP are shown in Fig. S7.
The mean of African emissions per unit PPP USD GDP in 2016 was 0.6 kgCO2 per PPP USD per year, which is more than 2 times the global value and 3 times the mean value of the USA (0.2 kgCO2 per PPP USD per year) and Europe (0.2 kgCO2 per PPP USD per yr−1). This points to more carbon-intensive economic growth in Africa than in developed countries, which may be an important barrier to future mitigation strategies as the GDP of Africa has grown by 112 % in the last 30 years and is projected to increase in the future by 3 % per year (World Bank, 2022). At the regional level, the largest values were from South Africa (0.4 kgCO2 per PPP USD per year) > northern Africa, southern countries and Sahelian western Africa (0.2 kgCO2 per PPP USD per year) > central Africa and the Horn of Africa (0.1 kgCO2/PPP USD of GDP). At the country scale, the largest emitters per unit of GDP were Libya (0.7 kgCO2 per PPP USD per year) and South Africa (0.7 kgCO2 per PPP USD per year) > Lesotho (0.4 kgCO2 per PPP USD per year) > Algeria (0.3 kgCO2 per PPP USD per year) (Fig. S7). The smallest emitters were the DRC (0.03 kgCO2 per PPP USD per yr−1) <(0.04 kgCO2 per PPP USD per year) < Burundi (0.06 kgCO2 per PPP USD per year) < Uganda (0.07 kgCO2 per PPP USD per year).
We also used GDP per unit exchange rate from the IMF (2020). The mean African emission per unit of GDPexch.rate was 0.5 kgCO2 per USD per year, larger than elsewhere, except in Asia (0.6 kgCO2 per GDPexch.rate per year. As shown in Fig. S6, over 2013–2017 the six biggest emitters were South Africa (0.7 kgCO2 per GDPexch.rate per year) > Libya (0.5 kgCO2 per GDPexch.rate per year) > South Sudan (0.4 kgCO2 per GDPexch.rate per year) > Zimbabwe, Benin and Algeria (0.3 kgCO2 per GDPexch.rate per year). The correlation coefficient between GDPexch.rate and FCO2 emissions per GDPexch.rate was 0.3, suggesting that countries with a high GDP do not always emit more CO2 per unit GDP. For instance, South Africa ranked first with 0.7 kgCO2 per GDPexch.rate per year and second for GDP (USD 350 billion), and Nigeria ranked first for GDP (USD 490 billion) but 21st for emissions per GDP (0.1 kgCO2 per GDPexch.rate per year). This may be related to the fact that countries with a high GDP are also more likely to create growth through sustainable activities.
3.2 LULUCF CO2 fluxes
3.2.1 Outlier corrections
In this section, we analyze CO2 fluxes from the LULUCF sector based on UNFCCC data (Sect. 1.1), which include forest lands, grasslands, croplands and all possible conversions between them (IPCC, 2003). As shown in Sect. 1.2 and Table S4, we found that some countries' reports are outliers with biophysically implausible CO2 sinks and/or sudden unexplained very large changes between successive reports. Due to scarce data over 1990–1998, we focus on the period 2001–2018. In the following paragraph, we discuss four approaches to including UNFCCC data: (a) uncorrected data, (b) corrections following Grassi et al. (2022) for all countries, (c) corrections following Grassi et al. (2022) except for the DRC, Namibia and Nigeria and (d) corrections following Grassi et al. (2022) except for the DRC.
Figure 4a shows UNFCCC data without correcting for outliers, based on BUR and NC data accessed in May 2022. The majority of the countries are sinks or small sources, except Tanzania and Nigeria, which are large sources. Very large (implausible) sinks are seen in Guinea and CAF. The continent is a CO2 sink of −3309 Mt CO2 yr−1 during the period 2001–2018.
Figure 4b shows the corrected fluxes according to Grassi et al. (2022), who excluded implausible large sink rates and used NDC and REDD+ reports instead of NC data for the DRC, Congo, CAF, Guinea and Madagascar and the most recent BUR, NC and inventory data for Namibia, Angola, Zimbabwe and Nigeria (see their Table 7). Africa as a whole is a CO2 source of 265 Mt CO2 yr−1. At a regional scale, the mean CO2 sources are distributed as follows in four regions: sub-Sahelian western Africa (235 Mt CO2 yr−1) > Horn of Africa (153 Mt CO2 yr−1) > central Africa (144 Mt CO2 yr−1) > southern Africa (14 Mt CO2 yr−1). The two sink regions are northern Africa (−259 Mt CO2 yr−1) and South Africa (−23 Mt CO2 yr−1). At the country scale, after the corrections of Grassi et al. (2022), the four countries with the larger sinks are CAF (−229 Mt CO2 yr−1) > Mali (−155 Mt CO2 yr−1) > Namibia (−106 Mt CO2 yr−1) > Cameroon (−77 Mt CO2 yr−1). The four countries with the largest sources are the DRC (529 Mt CO2 yr−1) > Nigeria (287 Mt CO2 yr−1) > Tanzania (77 Mt CO2 yr−1) > Ethiopia (56 Mt CO2 yr−1). The main issue with the correction from Grassi is that it reports no sink in the DRC, which has an important forest coverage representing 68 % of the country's area (FAO, 2020) and for which a sink was consistently reported in previous NCs.
Figure 4c shows LULUCF CO2 in African countries that is consistent with Grassi et al. (2022) except for three countries: Namibia (we used 2000 NC3 instead of NIR2019), Nigeria (we used 2014 NC2 instead of 2017 BUR2) and the DRC (we used 2015 NC3 instead of 2021 NDC). In that approach, Africa becomes a net CO2 sink of −589 Mt yr−1 over 2001–2018. At the regional scale, the region of central Africa (−620 Mt CO2) remains the main sink. However, the values and ranking of the top sources are Horn of Africa (153 Mt CO2) > southern Africa (141 Mt CO2) > sub-Sahelian western Africa (19 Mt CO2). At the country scale with this correction choice, the top sinks are the DRC (−235 Mt CO2) > CAF (−229 Mt CO2) > Mali (−155 Mt CO2), and the top three sources are Nigeria (98 Mt CO2) > Tanzania (77 Mt CO2) > Ethiopia (56 Mt CO2).
In the fourth approach, where we use the corrections of Grassi et al. (2022) except for the DRC and where we kept the latest national communication instead of the most recent NDC, the continent is a net sink of −504 Mt CO2 yr−1 over 2001–2018. At the regional scale, central Africa is a large CO2 sink, and the ranking of the sink regions is the central African group (−620 Mt CO2 yr−1) > northern Africa (−259 Mt CO2 yr−1) > South Africa (−23 Mt CO2 yr−1). The ranking of the source regions stays unchanged. At the country scale, the main sink is the DRC (−235 Mt CO2 yr−1). In this paper, we will mainly use data corrected following Grassi et al. (2022), but we want to raise a cautionary flag that adopting their correction for the DRC had an enormous effect on the CO2 budget of the continent, which becomes a source. Using the original latest national communication of the DRC instead of the NDC used by Grassi et al. (2022) and our own corrections for Namibia and Nigeria instead of those of Grassi et al. (2022) increased the continental CO2 uptake.
3.2.2 Comparison of UNFCCC-managed land area and FAO forest and other wooded land areas
Figure S10 shows a comparison of land areas in the NC, BUR and REDD+ reports (https://redd.unfccc.int/submissions.html?mode=browse-bycountry, last access: 24 June 2022) with FAO forest land areas (2015) and FAO forest land and other woodland areas for the year 2015 (see Table S9). Consistent with Grassi et al. (2022), all forest lands in Africa are considered to be managed. We found that FAO forest land areas are closer to UNFCCC estimates than the sum of FAO forest and other woodland areas, except for the DRC, Sudan, Senegal, Niger and Mauritania (Table S9). Forest areas in UNFCCC data using the IPCC default method do not exactly match FAO data estimates of forest areas.
3.2.3 LULUCF CO2 fluxes from UNFCCC vs. DGVMs and inversions
A comparison between LULUCF CO2 fluxes from UNFCCC, FAO, DGVMs and inversions is shown in Fig. 5 at the scale of the continent and for the six regions. The period of overlapping time series is 2001–2018. For the continent, DGVMs give a mean sink of −232 Mt CO2 yr−1 with a huge range from −1977 to 2095 Mt CO2 yr−1. The years with the biggest sinks for DGVMs (from the median of all the models) are 2006 and 2018, and the years with the smallest sinks are 2005 and 2016, which seem to be related to widespread drought years across Africa. A key result shown by this figure is that the DGVMs and inversions show a huge spread, making them of little value for “verifying” inventories for LULUCF CO2 fluxes in Africa. However, we observed that the medians of all the DGVMs point to a sink for Africa, unlike the UNFCCC data with the correction from Grassi et al. (2022).
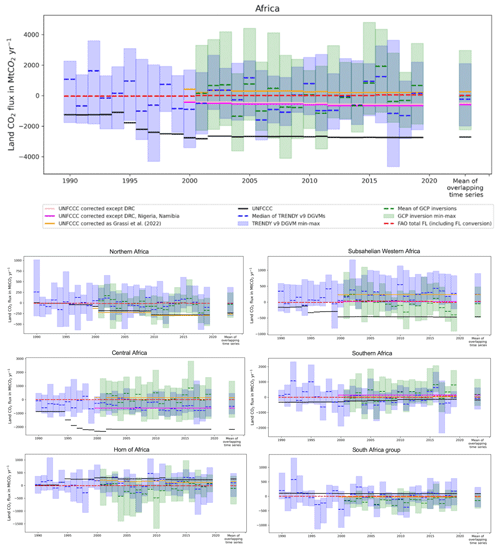
Figure 5LULUCF CO2 emissions and sinks: comparison between UNFCCC national greenhouse gas inventories, TRENDY version 9 DGVMs and inversions for the whole of Africa and for each of the six African subregions, and country details for the three main outliers (MtCO2 yr−1). Shaded green areas represent the minimum and maximum ranges from the inversions. Shaded blue represents the minimum and maximum ranges for TRENDY version 9 DGVMs. Green dashes denote the mean of the inversions, blue dashes denote the median of TRENDY version 9 DGVMs and green dashes denote the median of the inversions. Positive values represent a source, while negative values refer to a sink.
For three large countries, corrected UNFCCC values from Grassi et al. (2022) show a bigger discrepancy with other BU and TD methods than uncorrected ones (Fig. S9). In Namibia the corrected value gives a larger sink compared to other methods, while the uncorrected value is comparable. In the DRC, the corrected value, which was a source, seems a high overestimation compared to the other methods, while the uncorrected UNFCCC value is close to median values from inversions and to the FAO. In Nigeria, the corrected value seems to be a high overestimation of a net source compared to the other methods, pointing to either a smaller source (FAO, inversions) or even a sink (DGVMs).
The data in Fig. 5 show that most of the methods agree on a small net sink for African LULUCF CO2 fluxes, except for corrections following Grassi et al. (2022). However, disagreements exist among the different methods. Inversions give a smaller net sink () of Mt CO2 yr−1 than DGVMs ( Mt CO2 yr−1). The median value of the inversions is nevertheless within the range of the DGVMs. At the scale of Africa, the inversions' mean sink is ∼12 times smaller than the median from the DGVMs. The min–max range of the inversions (5216 Mt CO2 yr−1) is larger than the range of the DGVMs by 17 %. The DGVMs and inversions show a positive temporal correlation coefficient (r=0.7) for annual trends (linear fit to the time series).
UNFCCC values with the fourth approach point to a net sink (−503 Mt CO2 yr−1), similar to the third one. Corrected values as in Grassi et al. (2022) give a net source estimate of 265 Mt CO2 yr−1. FAO net emissions and removals represent a small net source (18 Mt CO2 yr−1). Differences between FAO and UNFCCC, as explained in Grassi et al. (2022), could be due to the fact that FAO estimates of CO2 fluxes for forest remaining forest can be set to zero in the absence of any national stock change inventory (Table 3).
Table 3Mean net LULUCF CO2 (emissions and removals) over the overlapping period of the different datasets (2001–2018) (MtCO2 yr−1).
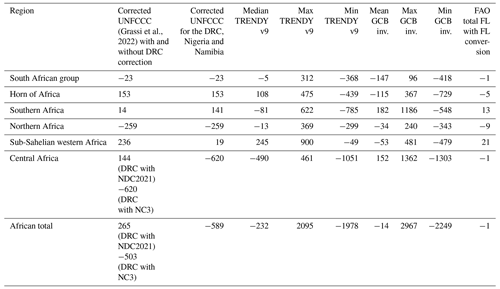
At the regional scale, we note some agreement between the different BU approaches. First, for the South African region, the mean of the DGVM medians during the overlapping period 2001–2018 (−5 Mt CO2 yr−1) and the FAO estimate (−1 Mt CO2 yr−1) are comparable and not too far from Grassi et al. (2022) (−23 Mt CO2 yr−1). Second, for northern Africa, the DGVM median (−13 Mt CO2 yr−1) and the FAO mean estimate over the same period (−9 Mt CO2 yr−1) are comparable. Finally, in sub-Sahelian western Africa, the DGVM (236 Mt CO2 yr−1) and UNFCCC corrected following Grassi et al. (2022) (245 Mt CO2 yr−1) are also close to each other.
Northern Africa is the group where DGVMs and inversions point to the closest values in terms of both signs (sinks) and magnitudes with small sinks of and Mt CO2 yr−1, respectively.
Looking at DGVMs and inversions in the region of South Africa, we found that both DGVMs and inversions point to a sink ( and Mt CO2 yr−1, respectively), with however a different magnitude. The region showing the highest discrepancies between inversions and DGVM values is central Africa with a source for inversions ( Mt CO2 yr−1) and a sink for DGVMs ( Mt CO2 yr−1). Sub-Sahelian western Africa also shows discrepancies in both sign and magnitude with Mt CO2 yr−1 for DGVMs and Mt CO2 yr−1 for inversions. The same is true for southern Africa with Mt CO2 yr−1 for DGMVs and Mt CO2 yr−1 for inversions as well as the Horn of Africa with Mt CO2 yr−1 for DGVMs and Mt CO2 yr−1 for inversions. At the regional scale, the inversions systematically give smaller sinks than DGVMs in the regions of central Africa, sub-Sahelian western Africa and northern Africa after 2010 (Fig. 5).
We also computed the correlation coefficient at the regional level between DGVM and inversion trends for each region. The highest correlation coefficients are in the South African region (r=0.7), followed by northern Africa (r=0.6) and southern Africa (r=0.5). The lowest correlation coefficients are for the group of central African countries (r=0.3), sub-Sahelian western African countries (r=0.2) and the Horn of Africa (r=0.1).
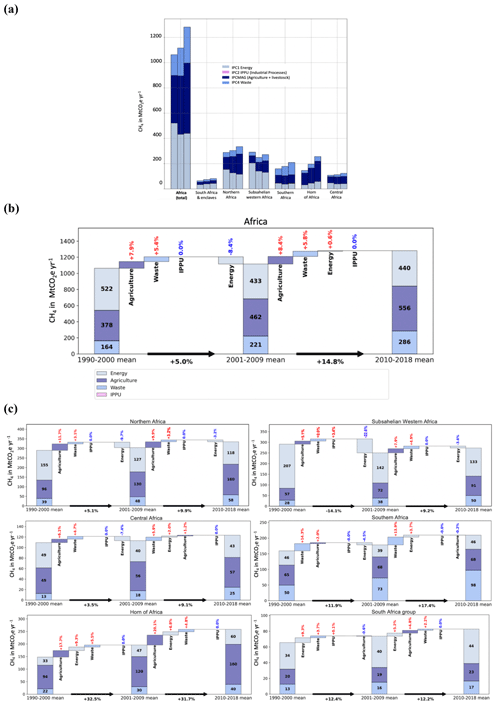
Figure 6(a) African mean anthropogenic CH4 emissions (MtCO2e yr−1) over 3 decades (1990–1998, 1999–2008 and 2010–2018). (b) The contributions of each sector to the change in African emissions in the last 3 decades. (c) The same for different regions, regrouping several countries. Data are from PRIMAP-hist (2021).
3.3 CH4 anthropogenic emissions
3.3.1 Total and sectoral bottom-up CH4 anthropogenic emissions and decadal changes
Figure 6 shows anthropogenic CH4 emissions from PRIMAP-hist grouped into four super-sectors (see Sect. 1). A map of CH4 emissions and their trends per country is given in Fig. 3c–d. LULUCF CH4 emissions are not considered in PRIMAP-hist. African anthropogenic CH4 emissions sum up to 1154 Mt CO2 eq. yr−1 over the last 3 decades. They increased from 1064 Mt CO2 eq. yr−1 in 1990–2000 to 1116 Mt CO2 eq. yr−1 in 2001–2009 and further to 1282 Mt CO2 eq. yr−1 over 2010–2018 (Fig. 6a). Over the last 3 decades, the main African CH4-emitting super-sectors shifted from Energy (49 % over 1990–2000) to Agriculture, mainly due to a northern African contribution. At the regional level, the main contributing region to the total emissions shifted over the last 30 years from sub-Sahelian western Africa (297 Mt CO2 eq. yr−1 for all sectors in 1990–2000) to northern Africa (333 Mt CO2 eq. yr−1 for all sectors in 2010–2018).
Northern African emissions increased from 290 Mt CO2 eq. yr−1 in 1990–2000 to 305 Mt CO2eq yr−1 in 2001–2009 and further to 333 Mt CO2 eq. yr−1 in 2010–2018. Sub-Sahelian emissions decreased from 297 Mt CO2 eq. yr−1 in 1990–2000 to 252 Mt CO2 eq. yr−1 in 2001–2009 and re-increased to 274 Mt CO2 eq. yr−1 in 2010–2018, a level lower than in the first decade (Fig. 6b). The Horn of Africa emissions increased from 149 Mt CO2 eq. yr−1 over 1990–2000 to 197 Mt CO2 eq. yr−1 over 2001–2009 and further to 260 Mt CO2 eq. yr−1 over 2010–2018. The emissions from southern Africa increased from 184 Mt CO2 eq. yr−1 in 1990–2000 to 180 Mt CO2 eq. yr−1 in 2001–2009 and further to 212 Mt CO2 eq. yr−1 in 2010–2018. Emissions from the central African region increased from 111 Mt CO2 eq. yr−1 in 1990–2000 to 114 Mt CO2 eq. yr−1 in 2001–2009 and further to 125 Mt CO2 eq. yr−1 in 2010–2018. We also computed the Gini indices of African countries' anthropogenic CH4 per capita emissions and obtained the following values: 0.6 in 1990–1998, 0.5 in 1999–2008 and 0.5 in 2009–2018, i.e., with a trend of increasing “inequality” between countries. As compared to per capita FCO2 emissions, more homogeneity is observed for CH4 per capita emissions. Similar to FCO2 emissions, the Gini values remained stable over the 3 decades, showing a similar level of inequality over time.
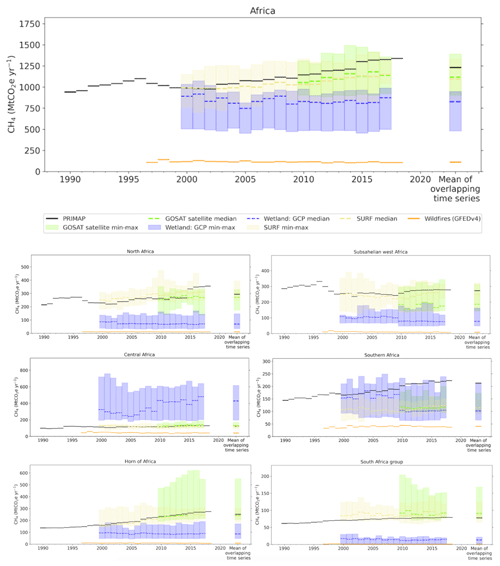
Figure 7Comparison of the total anthropogenic CH4 emissions (MtCO2e yr−1) from the PRIMAP-hist inventory (black) and global inversions. The shaded green and yellow areas represent the minimum and maximum range from GOSAT satellite and surface inversions, respectively. The shaded blue areas represent the minimum and maximum range of wetland natural emissions from inversions. The orange lines represent wildfire emissions from GFED version 4.
3.3.2 BU vs. inversions for total and anthropogenic CH4 emissions
Figure 7 compares BU anthropogenic emissions from PRIMAP-hist for the period 2000–2018 with the inversions' anthropogenic emissions (see Sect. 1). Wetland natural emissions are shown in the figure only for information from the median and range of the inversions. Over the overlapping time period, medians of both GOSAT and surface inversions are always smaller than PRIMAP-hist emissions at the continental and regional levels, except for the central African region. For the African continent, the mean, minimum and maximum of GOSAT inversions for anthropogenic CH4 emissions over 2000–2018 are Mt CO2 eq. yr−1, very close to the mean of surface inversions of Mt CO2 eq. yr−1. Good agreement between GOSAT and surface inversions was also found in other high-emitting countries (Deng et al., 2022). In contrast, PRIMAP-hist gives a mean of CH4 anthropogenic emissions of 1231 Mt CO2 eq. yr−1 over the period 2010–2017. The mean wetland flux from inversions over 2010–2017 is Mt CO2 eq. yr−1. Methane emissions from wildfires over Africa for the same period are less important, with a mean of 110 Mt CO2 eq. yr−1.
The regional emissions from PRIMAP-hist ranked in decreasing order are northern Africa (293 Mt CO2 eq. yr−1) > sub-Sahelian western Africa (272 Mt CO2 eq. yr−1) > Horn of Africa (252 Mt CO2 eq. yr−1) > southern Africa (212 Mt CO2 eq. yr−1) > central Africa (123 Mt CO2 eq. yr−1) > South Africa (78 Mt CO2 eq. yr−1). For both GOSAT and surface inversions, the ranking of the regions (Table S11) is almost the same for surface inversions and PRIMAP-hist, with the exception of central Africa and southern Africa.
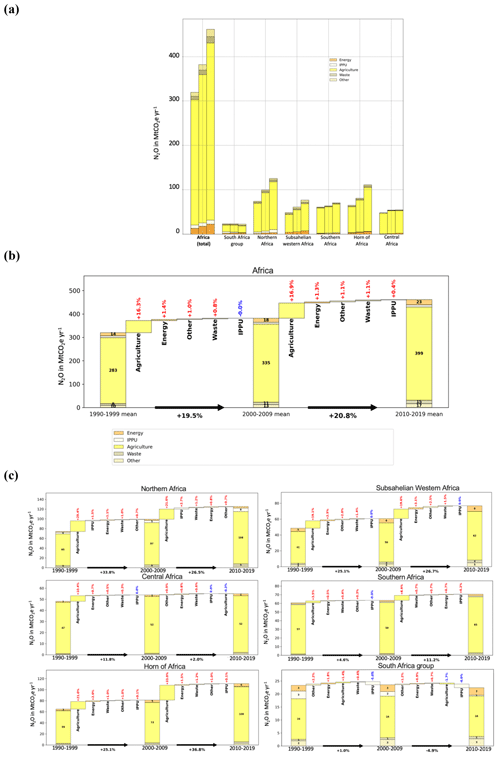
Figure 8(a) African anthropogenic N2O emissions (MtCO2e yr−1) over 3 decades: 1990–1998, 1999–2008 and 2009–2019. Data are from PRIMAP-hist (2021). (b) Contribution of each sector to the change in African N2O emissions between the last 3 decades. (c) The same for different regions, regrouping several countries. Data are from PRIMAP-hist (2021).
3.4 Results for N2O emissions
3.4.1 N2O PRIMAP-hist vs. atmospheric inversions (total flux)
Total and sectoral N2O anthropogenic emissions (PRIMAP-hist)
Figure 8 presents anthropogenic N2O emissions from PRIMAP-hist for five sectors (for the country values, see Fig. 4). Over the last 3 decades, the mean African emissions are 378 Mt CO2 eq. yr−1, 3 times less than CH4 emissions. The mean decadal N2O emissions increased from 319 Mt CO2 eq. yr−1 in 1990–1999 to 382 Mt CO2 eq. yr−1 in 2000–2009 (+20 %) and further to 431 Mt CO2 eq. yr−1 in 2010–2018. Over the last 3 decades, the main emitting sector remained Agriculture. The N2O emission increase also originates from Agriculture, with an increase from 283 to 335 Mt CO2 eq. yr−1 between 1990–1999 and 2000–2009, i.e., +16.3 % compared to the total emission increase of +19.5 %. The three other sectors show a smaller contribution to the emission increase: Energy (+1.4 %), Other (+1 %) and Waste (+0.8 %). The Industrial Processes and Product Use (IPPU) sector shows no change. Similarly, between 2000–2009 and 2010–2019, the N2O emission increase also came from the Agriculture sector, with an increase from 335 to 399 Mt CO2 eq. yr−1 between 1990–1999 and 2000–2009.
The main contributing regions to the continental emissions are northern Africa and the Horn of Africa (Fig. 8a). Between 2000–2009 and 2010–2019, the northern African contribution increased from 99 to 125 Mt CO2 eq. yr−1 (+27 %). The main sectoral contribution is always Agriculture, which increased in that region from 86 to 107 Mt CO2 eq. yr−1 (+21 %). Emissions from the second largest emitting region, the Horn of Africa, increased from 81.19 Mt CO2 eq. yr−1 in 2000–2009 to 111 Mt CO2 eq. yr−1 in 2010–2019 (+37 %), mainly from Agriculture. In the third most emitting region, sub-Sahelian Africa, emissions increased from 61 Mt CO2 eq. yr−1 in 2000–2009 to 77 Mt CO2 eq. yr−1 in 2010–2019 (+27 %), also from Agriculture. The least contributing region to the increase in the total N2O emissions from 2000–2009 to 2010–2019 is South Africa, which had a very small decrease, mainly from IPPU (−6 %), followed by Agriculture (−2 %). By contrast, there is a slight increase in N2O emissions for the group of South Africa for the Other (+1 %), Energy (+1 %) and Waste (+1 %) sectors.
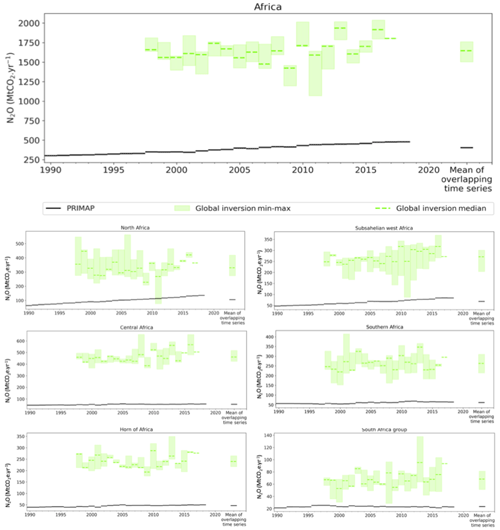
Figure 9Total N2O emissions from PRIMAP-hist (MtCO2e yr−1) (black line) from three GCP atmospheric inversions for the entire African continent and for six African subregions. The green line is the median of the three inversions, and the light green areas show the maximum–minimum range.
Figure 9 compares N2O emissions from PRIMAP-hist and the inversions. For all of Africa, the mean of inversion emissions over the overlapping time period 1998–2017 is Mt CO2 eq. yr−1, much larger than the PRIMAP-hist estimate of 360 Mt CO2 eq. yr−1. According to PRIMAP-hist, the total African emissions increased by 28 % between 1998 and 2017, while the trend of emissions from the inversions is 16±8 %. At the regional scale, the emissions from the inversions ranked in decreasing order are central Africa ( Mt CO2 eq. yr−1 Mt CO2 eq. yr−1) > northern Africa ( Mt CO2 eq. yr−1) > sub-Sahelian western Africa ( Mt CO2 eq. yr−1) > southern Africa ( Mt CO2 eq. yr−1 > Horn of Africa Mt CO2 eq. yr−1 > South Africa ( Mt CO2 eq. yr−1). According to PRIMAP-hist, the ranking is northern Africa (106 Mt CO2 eq. yr−1) > sub-Sahelian western Africa (68 Mt CO2 eq. yr−1) > southern Africa (62 Mt CO2 eq. yr−1) > central Africa (54 Mt CO2 eq. yr−1)> Horn of Africa (46 Mt CO2 eq. yr−1) > South Africa (24 Mt CO2 eq. yr−1) (see also Table S13). Emissions from PRIMAP-hist are smaller than the inversions by a factor of 16. This is likely due to the fact that we did not attempt to separate natural and anthropogenic emissions in the inversions. Other studies (Deng et al., 2022; Petrescu et al., 2021, in Europe) showed that, even after subtracting N2O natural estimates, the inversions always point to higher estimates than the BU methods.
4.1 Uncertainties specific to DGVMs and inversions for LULUCF CO2
In Fig. 5, we showed important disagreements among models regarding LULUCF CO2 on whether Africa has been a small source over the last 20 years (as shown by inversions) or a net sink (as shown by DGVMs and UNFCCC except with the Grassi correction). There is also more interannual variability in the DGVMs results, mainly from climate variability, which is absent from UNFCCC as inventories provide only decadal smoothed flux estimates. The larger sink in the DGVMs compared to the corrected UNFCCC estimates using the method of Grassi et al. (2022) may be due to the fact that non-Annex I UNFCCC estimates generally do not include dead biomass or harvested wood products. If forest biomass is estimated by a stock change approach, therefore, changes in living biomass due to transfer to dead biomass and harvested wood products will be considered emitted in that year, while in the DGVMs it will decay more slowly over time. Another difference is the treatment of land use change emissions based on historical global land use change maps for the DGVMs, which can significantly differ from national land use datasets. On the other hand, DGVMs do not represent forestry and may underestimate sinks in intensively managed young forests. DGVMs do not distinguish between unmanaged and managed lands, while UNFCCC inventories only account for managed land but include conservation areas and indigenous territories. Grassi et al. (2022) showed that the difference between the global UNFCCC sink (1100 Mt CO2 yr−1) and the global land carbon sink (4767 Mt CO2 eq. yr−1) must be explained by the contribution of non-managed lands. However, in the case of Africa, it was not possible to extract from UNFCCC reports the national areas of unmanaged land, and we also had to look at the UNFCCC Technical Assessment Reports (TARs) as well as REDD+ reports to extract information. Methods of assessment have not been fully standardized since 1990, and they differ depending on the countries analyzed and on the emission categories considered. In this context, when comparing UNFCCC estimates with data from DGVMs and inversion models, different layers of aggregated uncertainties affect the analysis (Deng et al., 2022; Petrescu et al., 2021; Grassi et al., 2018). The fact that LULUCF CO2 fluxes have the greatest uncertainties is true globally.
4.2 Differences and sources of uncertainties between BU and TD CH4 emissions
The methodology used to remove natural CH4 emissions from inversions is key to comparing them with BU estimates of anthropogenic emissions only. In this paper, we used a separation based on the natural emissions solved by each inversion (Sect. 2.3, Method 1). Using an alternative method from Deng et al. (2022) based on natural emissions from the median of all the inversions gives smaller anthropogenic emissions than PRIMAP-hist (Fig. S10).
4.3 Differences and sources of uncertainties between BU and TD N2O emissions
For N2O emissions, discrepancies between inventories and inversions are very high, especially for the group of central African countries where the vegetation covers an important land area with likely high natural N2O (Deng et al., 2022). We can suppose that, more broadly for all African groups, the lack of accounting for natural emissions is the main reason why PRIMAP-hist estimates are much smaller than those of inversions. All the African countries used Tier 1 emission factors and include only direct N2O emissions. The study by Deng et al. (2022) underlined that indirect anthropogenic emissions notably coming from “atmospheric nitrogen deposition and leaching from anthropogenic nitrogen additions to aquifers and inland water are usually not reported by non-Annex I countries” and that this underreported source of anthropogenic emissions tends to represent about 5 % to 10 % of anthropogenic N2O. According to Deng et al. (2022), the global situation from inversions for the main emitters is similarly affected by the potential contribution of natural sources as well, which is difficult to estimate and separate. Figure 11 from Deng et al. (2022) shows that, even when removing “intact/non-managed lands” from inversions, in many countries, especially tropical countries, the inversions give a systematically much higher anthropogenic level of N2O than the inventories, suggesting that there are either missing anthropogenic sources or some “natural” sources (e.g., conservation areas) in managed lands that are underestimated by inventories.
4.4 Synthesis of the steps for assessing net GHG trends over Africa
Here, we propose a first step towards the elaboration of what could become a more systematic method for a scientific benchmark of non-Annex I national inventories: (1) correct outliers, (2) check the plausibility of estimates, (3) have an independent evaluation of inventory data by experts, (4) compare UNFCCC data corrected thanks to expert judgment and other BU and TD methods, (5) compute the mean of all the BU and TD methods, (6) compute “best-fitted BU values” (meaning best-fitted BU values excluding uncorrected UNFCCC data) and “TD values” (meaning “best-fitted TD values” without considering N2O inversions replaced with PRIMAP-hist values), and (7) identify ranking anomalies.
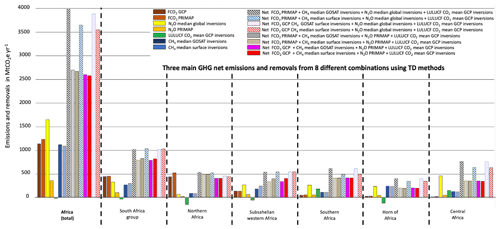
Figure 10Synthesis for the three main GHGs with net African budget computation by all the TD methods for Africa as a whole and for six subgroups of African countries across overlapping time series (2001–2017). Following the atmospheric convention, positive numbers represent an emission into the atmosphere and negative values represent a sink. The CO2 emissions and sinks from LULUCF are represented in green; they are taken from the GCP 2020 dataset (MtCO2e yr−1).
4.5 Net GHG budget from inversions
Figure 10 shows different combinations of inversion GHG budgets and individual gas contributions.
For the African total, the mean net GHG budget from the inversions where N2O inversions are replaced by PRIMAP-hist is Mt CO2 eq. yr−1. The regional GHG budgets in decreasing order are northern Africa ( Mt CO2 eq. yr−1) > South African group ( Mt CO2 eq. yr−1) > southern Africa Mt CO2 eq. yr−1) > sub-Sahelian western Africa ( Mt CO2 eq. yr−1) > central Africa ( Mt CO2 eq. yr−1) > Horn of Africa Mt CO2 eq. yr−1) (Table S17). The mean net of inversions including N2O inversions is substantially higher, Mt CO2 eq. yr−1. The regional GHG budgets in decreasing order are northern Africa Mt CO2 eq. yr−1) > central Africa ( Mt CO2 eq. yr−1) > southern Africa ( Mt CO2 eq. yr−1) > sub-Sahelian western Africa ( Mt CO2 eq. yr−1) > South African group Mt CO2 eq. yr−1) (Table S17).
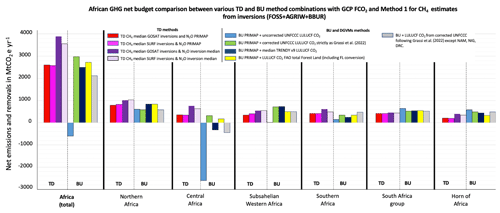
Figure 11Synthesis for the three main GHG net African budgets from the TD and BU methods, using Method 1 for separating anthropogenic CH4 emissions from inversions (FOSS + AGRIW + BBUR) during 2001–2017. FCO2 data are from GCP. The N2O is from the global inversions and from PRIMAP-hist. For the TD method, anthropogenic CH4 from both GOSAT and surface inversions is used, and LULUCF is from GCP inversions only. For the BU methods, anthropogenic CH4 and N2O from PRIMAP are used and with five different methods for assessing LULUCF CO2: from uncorrected UNFCCC data; from corrected UNFCCC data according to Grassi et al. (2022); from corrected UNFCCC except for Namibia, Nigeria and the DRC; from TRENDY version 9; and from the FAO FL, including FL conversions. Following the atmospheric convention, positive numbers represent an emission into the atmosphere and the negative values represent a sink (MtCO2e).
4.6 Comparison between the BU and TD methods
Figure 11 shows the GHG budgets from all the combinations of the BU and TD methods. The mean of all the methods after filtering outliers (Grassi et al. (2022) and UNFCCC corrections using PRIMAP instead of inversions for N2O) is Mt CO2 eq. yr−1, which represents only 7.3 % of global FCO2 emissions. The mean of all the estimates points to a source in the six African regions ranked in decreasing order as northern Africa Mt CO2 eq. yr Mt CO2 eq. yr−1) > Horn of Africa Mt CO2 eq. yr−1) > sub-Sahelian western Africa ( Mt CO2 eq. yr−1) > southern Africa Mt CO2 eq. yr−1) > central Africa Mt CO2 eq. yr−1).
We initially did not make any assumption regarding which approach is “better” between the TD and BU methods, as this actually depends on the considered gas, sector and spatial scales. Comparability between TD and BU results is not completely obvious either, as they do not represent the same processes (the example of LULUCF CO2 for DGVMs is explained in Sect. 3.1). For N2O specifically, we highlighted in Sect. 3.3 the large uncertainty in the TD estimates, underlining the importance of separating natural N2O emissions from total estimates in order to deliver appropriate anthropogenic assessments thanks to the inversions.
We showed in the results of this paper that inversions in general tend to have larger uncertainties than the inventories as well as large differences in terms of minima or maxima and at the annual scale, even among similar typologies of the methods. However, at the decadal scale, they show reliable overall trends (with good matches among the median values of various estimates in the overlapping time period), especially at the spatial scale of groups of countries and of a continent. Under such conditions, TD estimates help identify or confirm outliers or large uncertainties in inventories that may occur, especially for non-Annex I countries like in Africa.
Inversions therefore cannot be a substitute, but they are rather a complement to check trend consistencies in inventories and to help identify and correct the main outliers. That is why we chose BU estimates to deliver a final budget over Africa (with CO2 LULUCF corrections) as synthesis figures (see Figs. 12 and 13 in the next paragraph). The possibilities for reducing the gap BU and TD estimates are the following. (1) For inversions, have a coarser network of surface stations and coarser spatial resolutions. (2) For DGVMs, see Sect. 3.1. (3) For national UNFCCC inventories, have regularly updated activity data, use country-specific emission data and include indirect emissions, which is not the case to date for African countries, and use expert judgment to correct outliers as done by Grassi et al. (2022) and in this study for CO2 LULUCF emissions.
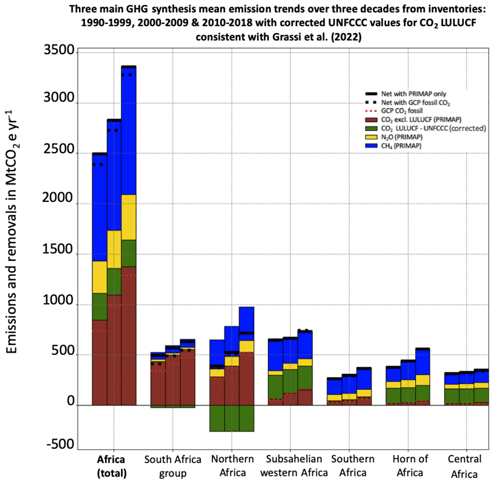
Figure 12Synthesis for the three main GHGs from the inventories (after UNFCCC LULUCF CO2 corrections consistent with Grassi et al., 2022) for the three main GHGs with net African budget computation by BU inventories for Africa as a whole and for six subgroups of African countries across 3 different decades (1990–1999, 2000–2010 and 2010–2018) using data and corrections from country inventories. Following the atmospheric convention, positive numbers represent an emission into the atmosphere and negative values represent a sink. Black horizontal lines represent a net flux resulting from the addition of the three main GHGs using PRIMAP-hist only, and dashed black horizontal lines also represent the net flux resulting from the addition of the three main GHGs but using the GCP dataset for FCO2. Dashed red lines represent the fluxes from GCP FCO2 available in the most recent GCP paper to compare them with PRIMAP-hist results, which are represented with the brown bar plots. The N2O and CH4 fluxes from PRIMAP-hist are represented with yellow and blue bars, respectively. CO2 emissions and sinks from LULUCF are represented in green; they are taken from NC or BUR UNFCCC datasets with corrections applied (MtCO2e yr−1).
4.7 Net GHG budget from BU estimates
Figure 12 shows the budget for the three GHGs from UNFCCC data with LULUCF data corrected using the second approach. There is a clear increase in African total GHG emissions during the last 3 decades. The differences between the BU datasets are mainly due to different sectoral allocations. However, the trends are consistent and comparable, and differences among inventories tend to be less for the most recent decade.
Table 4Mean net total African and regional group emissions and removals from BU methods using either GCP or PRIMAP-hist for FCO2 over 2001–2017 (MtCO2e yr−1).
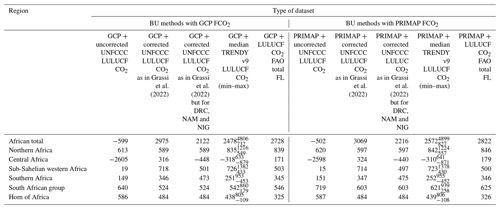
At the country level, a small number of countries showed an increasing difference between PRIMAP-hist and GCP estimates of fossil CO2 emissions over time, but they are small FCO2 emitters. The differences may also be partly explained by changes in accounting methods as mentioned in Gütschow et al. (2016). The biggest discrepancies are noticeable for Mali (64 %), Cameroon (−62 %) and the DRC (−38 %), but those three countries are not major FCO2 emitters (Fig. 4a–b).
Table 4 shows the differences in the net African budget from various BU methods using GCP or PRIMAP-hist for FCO2 over 2001–2017 that are also illustrated in Fig. 11.
4.7.1 BU LULUCF budget from UNFCCC corrected by Grassi et al. (2022)
Over 2001–2017, the net BU GHG budget is 2975 Mt CO2 eq. yr−1. Regionally, the ranking in decreasing order is sub-Sahelian western Africa (718 Mt CO2 eq. yr−1) > northern Africa (588 Mt CO2 eq. yr−1) > South African group (524 Mt CO2 eq. yr−1) > Horn of Africa (484 Mt CO2 eq. yr−1) > southern Africa (346 Mt CO2 eq. yr−1) > central Africa (316 Mt CO2 eq. yr−1).
4.7.2 BU LULUCF budget CO2 from the FAO
The BU budget from the FAO data is 2728 Mt CO2 eq. yr−1, 8 % less than above. The ranking of the regions in decreasing order is northern Africa (838 Mt CO2 eq. yr−1) > South African group (546 Mt CO2 eq. yr−1) > sub-Sahelian western Africa (503 Mt CO2 eq. yr−1) > southern Africa (345 Mt CO2 eq. yr−1) > Horn of Africa (325 Mt CO2 eq. yr−1) > central Africa (171 Mt CO2 eq. yr−1).
4.7.3 BU LULUCF budget from DGVMs
The net GHG budget for Africa is Mt CO2 eq. yr−1, 9 % less than with the FAO. The ranking of the regions in decreasing order is northern Africa ( Mt CO2 eq. yr−1) > sub-Sahelian western Africa ( Mt CO2 eq. yr−1) > South Africa ( Mt CO2 eq. yr−1) > Horn of Africa ( Mt CO2 eq. yr−1) > southern Africa ( Mt CO2 eq. yr−1 > central Africa ( Mt CO2 eq. yr−1).
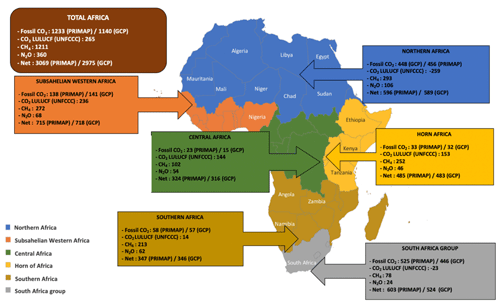
Figure 13The 2001–2018 emissions (MtCO2e yr−1) for fossil CO2 (GCP and PRIMAP-hist), LULUCF CO2 (corrected UNFCCC data consistent with Grassi et al., 2022), CH4 (PRIMAP-hist) and N2O (PRIMAP-hist) for Africa and for the six regions.
For information, in the Supplement, Figs. S13 and S14 illustrate the differences (MtCO2e and percentage) for CH4, N2O and the total net GHG budget that would result from the use of AR6 GWP100 compared to AR4 GWP100 currently in use by UNFCCC non-Annex I countries, for the six African regions considered in Fig. 13 as well as for the African total. The net difference in the total African budget for the use of GWP100 AR6 instead of AR4 is +4.6 %, which means a relatively small increasing impact on the net budget, with a prevailing effect of the slight increase in CH4 GWP100 in AR6 as compared to AR4 over the strong decrease in N2O GWP-100. The two African regions that are the most impacted in terms of net budget are the southern countries (+7.2 %) and the Horn of Africa (+6.3 %). The least impacted region in terms of overall net budget with an updated AR6 GWP-100 for CH4 and N2O is South Africa (+1.7 %).
The datasets from the three main greenhouse gases used in this paper (CO2, CH4 and N2O) from the various BU inventories, TD inversions and DGVMs over Africa will be made publicly available. This database is available from Zenodo at https://doi.org/10.5281/zenodo.7347077 (Mostefaoui et al., 2022).
This dataset contains 32 data files.
-
CO2 inversions (annual flux for LULUCF CO2)
-
African CO2 TD inversions GCB2020 1990–2019: annual CO2 flux from GCB inversion models
-
African CO2 lateral flux 2001–2019: annual CO2 lateral flux including river transport, crop and wood product trade
-
African CO2 TRENDY version 9 1990–2019: annual CO2 flux from 14 DGVMs
-
FAO 1990–2019: annual emissions and removals from the FAO dataset
-
Inventory IPCC 1990–2019: annual flux from inventory data collected from UNFCCC national inventories in the IPCC categories
-
CH4 inversions for 2000–2017 (annual flux)
-
African CH4 global inversion 2000–2017: CH4 flux over 2000–2017 from 11 surface inversions and 11 satellite inversion models from four sectors. “Fossil” refers to emissions from the fossil sector. “Agriculture” and “Waste” refer to emissions from both the agriculture and waste sectors. “Biomass burning” refers to emissions from biomass burning.
-
GFED version 4 1997–2016: wildfire emissions from GFED version 4
-
N2O inversions for 1998–2017 (annual flux)
-
N2O PYVAR 1998–2017: total N2O emissions from PyVAR inversions
-
N2O TOMCAT-INVICAT 1998–2015: total N2O emissions from the TOMCAT-INVICAT model
-
N2O MIROC4-ACTM 1998–2016: total N2O emissions from the MIROC4-ACTM model
Data used in this study are also included in the Supplement (for example, from FAO data) and on public websites (CDIAC, PRIMAP-hist, World Bank data). Any other data that support the findings of this study are available from the corresponding author upon request.
Africa is a large continent with 56 countries, and some of the countries are major GHG emitters. Because of its rapidly growing population and high industrial potential, Africa has a critical geography regarding climate change mitigation and adaptation policy. Depending on the emission pathways, Africa, which is already a big emitting region, is expected to represent at least a bit more than 10 % of the global share by 2050, which could become as high as 18 % of global emissions by 2050 (van der Zwaan et al., 2018).
This paper presents both a continental view and a detailed analysis of the three main GHG trends during the last 30 years across this continent as a whole and across relevant groups of countries given the inversions' resolutions and also considering the countries' details. Thanks to the comparison of different methods and datasets, the uncertainty about the net emissions and removals of GHGs decreases. The interest in studying Africa is high not only from a scientific point of view but also from a climate-policy perspective, as under the UNFCCC principle of “common but differentiated responsibility” about global warming, the credibility of the PA lies in the effective participation and inclusiveness of all the parties, including non-Annex I countries. Our effort in comparing BU datasets and inversions and analyzing differences for African GHG emissions and removal assessment by looking at trends since 1990 will also be useful for future updates on a regular basis within the 2023 GST perspective.
At the scale of Africa, there is a rapid increase in FCO2 emissions that has roughly doubled since 1990. This increase is dominated by coal emissions for the decade 1990–1998 compared to 1999–2008 (+9 %) and by oil for the decade 1999–2008 compared to the decade 2008–2017 (+16 %). As for CO2 LULUCF, we found that BU estimates are characterized by important annual fluctuations, as opposed to periodic national inventory assessments, and the reconciliation between the sectoral classification for anthropogenic estimates between TD and BU has to be done “manually” and is not uniform to date, which does not facilitate the comparability of those different approaches. There are also differences between GCP inversions for CO2 due to the fact that choices of model transport may differ among models, because prior fluxes can also differ between modeling teams and because the African GHG observation network is characterized by few stations and relatively scarce data. The lack of integration of CO2 lateral anthropogenic and river fluxes is also an issue to be taken into account when trying to compare BU and TD methods (Ciais et al., 2022), and in the present study we did integrate those lateral fluxes. Anthropogenic CH4 from PRIMAP-hist estimates indicates that, of the total African emission increase from 1064 to 1116 Mt CO2 eq. yr−1 between 1990–2000 and 2001–2009 (+5 %), only two sectors contributed: Agriculture, in a dominant way (+8 %), and Waste (+5 %). Energy contributes to emission decrease (−8 %), which is however too small to offset other sectors' CH4 emissions that represent a net increase. The main regional contributions come from northern Africa and from the Agriculture sector (+12 %). Over the same period, the least contributing emitter is the group of South Africa (+12 %), with only one decreasing emission sector: Agriculture (−1 %). The mean 2001–2009 emissions increased by +15 % over 2010–2018, with contributions from all the sectors except for IPPU. This increase is dominated by Agriculture (+8 %) and Waste (+6 %). For 2010–2018, the two main contributing regions for CH4 emissions are northern Africa and sub-Sahelian western Africa, Agriculture being the dominant emitting sector. From inversions, after withdrawing natural emissions and wildfires using the GFED dataset from the total CH4 emissions, median values are almost always below PRIMAP-hist estimates. CH4 natural emissions have an important impact in Africa, especially in the central African region as well as in the southern countries. N2O TD estimates are always higher than the ones from PRIMAP-hist, underlining the importance of separating natural N2O emissions from total estimates in order to deliver appropriate anthropogenic assessments thanks to the inversions.
To compute a net budget for the three main GHG emissions and removals, and for comparability, we used the Mt CO2 eq. yr−1 metric and the latest IPCC report-recommended GWP. The choice of a constructed GWP metric, however, creates additional associated uncertainties, notably due to the selected time horizon. By computing the mean of methods excluding uncorrected UNFCCC and N2O inversion data from 20 different ways of assessing GHG emissions and removals in Africa, we found that the most recent net budget from the three main GHGs in Africa is a source of Mt CO2 eq. yr−1.
Our assessment of African GHG emission trends over 30 years through different methods can enable comparisons of ex post and ex ante pledges of the PA, whose baseline year is often 1990. However, given the global geopolitics to date characterized by the prevailing principle of national sovereignty, a scientific assessment of GHGs can only work as a supporting tool (Janssens-Maenhout et al., 2020) and cannot be directly policy-prescriptive. We note a relatively good match among the various types of estimates in terms of overall trends, especially at a regional level and on a decadal basis, but large differences exist in terms of minima and maxima and at the annual scale, even among similar typologies of the methods (TD or BU). The large discrepancies are a scientific limit to the possibility of precise verification of the African country-reported emissions, but they are good enough to indicate trends. To compute a net from the three main GHGs, no purely TD method is available due to the necessity to replace N2O inversion data with BU data. An original result of this study is that we proposed at a small scale what may become a systematic formalized methodological protocol for independent verification of a net estimate using country-reported data to be possibly implemented at the UNFCCC secretariat scale in a centralized way. The African GHG increasing trend is not in line with the mitigation aims of the PA towards net-zero globally. Research teams focusing on inversion methods (Nickless et al., 2020) underline that uncertainties should not be above 15 % in order to deliver a reasonable verification support capacity. A major source of complexity for the evaluation of the respect of the Paris Agreement comes from the fact that national pledges generally fall below the discrepancies between different scientific independent estimates. This calls for investments not only in improvements of atmospheric measurement devices, but also in research efforts for standardizing verification methods. At the policy level, the extrapolation of this study to the climate policy field could also serve as a compelling argument for the creation of a global dedicated “climate inspection task force” of the UNFCCC.
The supplement related to this article is available online at: https://doi.org/10.5194/essd-16-245-2024-supplement.
MM, PC, PP and MJM designed research and led the discussions. MM wrote the initial draft of the paper and edited all the following versions. MM made all the figures. MJM and PP processed the original data from inversions and DGVMs. MM processed the UNFCCC data and corrections. PC, PP and YE made valuable suggestions for the paper's structure. PC, MJM and PPP read and gave comments and advice on previous versions of the manuscript. All the co-authors commented on specific parts related to their datasets. PC, MJM, PKP, FC, SS, CR, IL, MS and PP were data providers.
The contact author has declared that none of the authors has any competing interests.
Publisher’s note: Copernicus Publications remains neutral with regard to jurisdictional claims made in the text, published maps, institutional affiliations, or any other geographical representation in this paper. While Copernicus Publications makes every effort to include appropriate place names, the final responsibility lies with the authors.
MM acknowledges funding from Sorbonne University, Institute of Environmental Transition. Philippe Ciais, Philippe Peylin and Matthew J. McGrath were supported by the European Commission's Horizon 2020 Framework Program (VERIFY, grant no. 776810). PC and YE are also supported by the RECCAP2 project (grant no. ESRIN/4000123002/18/I-NB). We acknowledge Stephen Sitch and the TRENDY modelers for the use of their dataset. We also acknowledge Christian Rödenbeck for the use of CarboScope, Frédéric Chevallier for CAMS, Ingrid Luijkx for CTE and Marielle Saunois for CH4 inversions. The PyVAR-N2O modeling results were provided by Rona Thompson (NILU), were funded by the Copernicus Atmosphere Monitoring Service (https://atmosphere.copernicus.eu/, last access: 28 August 2022) implemented by the ECMWF on behalf of the European Commission, and were generated using computing from the Institut Pierre Simon Laplace: LSCE/CEA and LMD (École Normale Supérieure, PSL Research University, Sorbonne University, École Polytechnique, IP Paris).
This research has been mainly supported by Sorbonne University (Institute of the Environmental Transition). It was also supported by the RECCAP2 project (grant no. ESRIN/4000123002/18/I-NB), the European Commission, Horizon 2020 Framework Program (VERIFY, grant no. 776810), and the Copernicus Atmosphere Monitoring Service 1135 (https://atmosphere.copernicus.eu/, last access: 26 August 2022), implemented by ECMWF on behalf of the European Commission, and with computing resources from the Institut Pierre Simon Laplace: LSCE/CEA and LMD (École Normale Supérieure, PSL Research University, Sorbonne University, École Polytechnique, IP Paris).
This paper was edited by Francesco N. Tubiello and reviewed by Chris Jones and one anonymous referee.
Andrew, R. M.: A comparison of estimates of global carbon dioxide emissions from fossil carbon sources, Earth Syst. Sci. Data, 12, 1437–1465, https://doi.org/10.5194/essd-12-1437-2020, 2020.
Ayompe, L. M., Davis, S. J., and Egoh, B. N.: Trends and drivers of African fossil fuel CO2 emissions 1990–2017, Environ. Res. Lett., 15, 124039, https://doi.org/10.1088/1748-9326/abc64f, 2020.
BP: BP Statistical Review of World Energy, https://bp.com/statisticalreview (last access: 23 June 2022), 2020.
Beck, H. E., Zimmermann, N. E., McVicar, T. R., Vergopolan, N., Berg, A., and Wood, E. F.: Present and future Köppen-Geiger climate classification maps at 1-km resolution, Sci. Data, 5, 180214, https://doi.org/10.1038/sdata.2018.214, 2018.
Bombelli, A., Henry, M., Castaldi, S., Adu-Bredu, S., Arneth, A., de Grandcourt, A., Grieco, E., Kutsch, W. L., Lehsten, V., Rasile, A., Reichstein, M., Tansey, K., Weber, U., and Valentini, R.: An outlook on the Sub-Saharan Africa carbon balance, Biogeosciences, 6, 2193–2205, https://doi.org/10.5194/bg-6-2193-2009, 2009.
Canadell, J. G., Raupach, M. R., and Houghton, R. A.: Anthropogenic CO2 emissions in Africa, Biogeosciences, 6, 463–468, https://doi.org/10.5194/bg-6-463-2009, 2009.
Chevallier, F., Fisher, M., Peylin, P., Serrar, S., Bousquet, P., Bréon, F.-M., Chédin, A., and Ciais, P.: Inferring CO2 sources and sinks from satellite observations: Method and application to TOVS data, J. Geophys. Res.-Atmos., 110, D24309, https://doi.org/10.1029/2005JD006390, 2005.
Ciais, P., Bastos, A., Chevallier, F., Lauerwald, R., Poulter, B., Canadell, J. G., Hugelius, G., Jackson, R. B., Jain, A., Jones, M., Kondo, M., Luijkx, I. T., Patra, P. K., Peters, W., Pongratz, J., Petrescu, A. M. R., Piao, S., Qiu, C., Von Randow, C., Regnier, P., Saunois, M., Scholes, R., Shvidenko, A., Tian, H., Yang, H., Wang, X., and Zheng, B.: Definitions and methods to estimate regional land carbon fluxes for the second phase of the REgional Carbon Cycle Assessment and Processes Project (RECCAP-2), Geosci. Model Dev., 15, 1289–1316, https://doi.org/10.5194/gmd-15-1289-2022, 2022.
Deng, Z., Ciais, P., Tzompa-Sosa, Z. A., Saunois, M., Qiu, C., Tan, C., Sun, T., Ke, P., Cui, Y., Tanaka, K., Lin, X., Thompson, R. L., Tian, H., Yao, Y., Huang, Y., Lauerwald, R., Jain, A. K., Xu, X., Bastos, A., Sitch, S., Palmer, P. I., Lauvaux, T., d'Aspremont, A., Giron, C., Benoit, A., Poulter, B., Chang, J., Petrescu, A. M. R., Davis, S. J., Liu, Z., Grassi, G., Albergel, C., Tubiello, F. N., Perugini, L., Peters, W., and Chevallier, F.: Comparing national greenhouse gas budgets reported in UNFCCC inventories against atmospheric inversions, Earth Syst. Sci. Data, 14, 1639–1675, https://doi.org/10.5194/essd-14-1639-2022, 2022.
Dorfman, R.: A formula for the Gini Coefficient, The Review of Economics and Statistics, 61, 1, https://doi.org/10.2307/1924845,1979.
FAO: Global Forest Resources Assessment 2020: Main Report, FAO, Rome, http://www.fao.org/documents/card/en/c/ca9825en (last access: 25 May 2022), 2020.
FAOSTAT: Emissions from agriculture and forest land, Global, regional and country trends 1990–2019, FAO, Rome [data set], https://www.fao.org/faostat/en/#data/GT (last access: 25 May 2022), 2021.
Friedlingstein, P., Jones, M. W., O'Sullivan, M., Andrew, R. M., Hauck, J., Peters, G. P., Peters, W., Pongratz, J., Sitch, S., Le Quéré, C., Bakker, D. C. E., Canadell, J. G., Ciais, P., Jackson, R. B., Anthoni, P., Barbero, L., Bastos, A., Bastrikov, V., Becker, M., Bopp, L., Buitenhuis, E., Chandra, N., Chevallier, F., Chini, L. P., Currie, K. I., Feely, R. A., Gehlen, M., Gilfillan, D., Gkritzalis, T., Goll, D. S., Gruber, N., Gutekunst, S., Harris, I., Haverd, V., Houghton, R. A., Hurtt, G., Ilyina, T., Jain, A. K., Joetzjer, E., Kaplan, J. O., Kato, E., Klein Goldewijk, K., Korsbakken, J. I., Landschützer, P., Lauvset, S. K., Lefèvre, N., Lenton, A., Lienert, S., Lombardozzi, D., Marland, G., McGuire, P. C., Melton, J. R., Metzl, N., Munro, D. R., Nabel, J. E. M. S., Nakaoka, S.-I., Neill, C., Omar, A. M., Ono, T., Peregon, A., Pierrot, D., Poulter, B., Rehder, G., Resplandy, L., Robertson, E., Rödenbeck, C., Séférian, R., Schwinger, J., Smith, N., Tans, P. P., Tian, H., Tilbrook, B., Tubiello, F. N., van der Werf, G. R., Wiltshire, A. J., and Zaehle, S.: Global Carbon Budget 2019, Earth Syst. Sci. Data, 11, 1783–1838, https://doi.org/10.5194/essd-11-1783-2019, 2019.
Friedlingstein, P., O'Sullivan, M., Jones, M. W., Andrew, R. M., Hauck, J., Olsen, A., Peters, G. P., Peters, W., Pongratz, J., Sitch, S., Le Quéré, C., Canadell, J. G., Ciais, P., Jackson, R. B., Alin, S., Aragão, L. E. O. C., Arneth, A., Arora, V., Bates, N. R., Becker, M., Benoit-Cattin, A., Bittig, H. C., Bopp, L., Bultan, S., Chandra, N., Chevallier, F., Chini, L. P., Evans, W., Florentie, L., Forster, P. M., Gasser, T., Gehlen, M., Gilfillan, D., Gkritzalis, T., Gregor, L., Gruber, N., Harris, I., Hartung, K., Haverd, V., Houghton, R. A., Ilyina, T., Jain, A. K., Joetzjer, E., Kadono, K., Kato, E., Kitidis, V., Korsbakken, J. I., Landschützer, P., Lefèvre, N., Lenton, A., Lienert, S., Liu, Z., Lombardozzi, D., Marland, G., Metzl, N., Munro, D. R., Nabel, J. E. M. S., Nakaoka, S.-I., Niwa, Y., O'Brien, K., Ono, T., Palmer, P. I., Pierrot, D., Poulter, B., Resplandy, L., Robertson, E., Rödenbeck, C., Schwinger, J., Séférian, R., Skjelvan, I., Smith, A. J. P., Sutton, A. J., Tanhua, T., Tans, P. P., Tian, H., Tilbrook, B., van der Werf, G., Vuichard, N., Walker, A. P., Wanninkhof, R., Watson, A. J., Willis, D., Wiltshire, A. J., Yuan, W., Yue, X., and Zaehle, S.: Global Carbon Budget 2020, Earth Syst. Sci. Data, 12, 3269–3340, https://doi.org/10.5194/essd-12-3269-2020, 2020.
Gilfillan, D. and Marland, G.: CDIAC-FF: global and national CO2 emissions from fossil fuel combustion and cement manufacture: 1751–2017, Earth Syst. Sci. Data, 13, 1667–1680, https://doi.org/10.5194/essd-13-1667-2021, 2021.
Grassi, G., House, J., Kurz, W. A., Cescatti, A., Houghton, R. A., Peters, G. P., Sanz, M. J., Viñas, R. A., Alkama, R., Arneth, A., Bondeau, A., Dentener, F., Fader, M., Federici, S., Friedlingstein, P., Jain, A. K., Kato, E., Koven, C. D., Lee, D., Nabel, J. E. M. S., Nassikas, A. A., Perugini, L., Rossi, S., Sitch, S., Viovy, N., Wiltshire, A., and Zaehle, S.: Reconciling global-model estimates and country reporting of anthropogenic forest CO2 sinks, Nat. Clim. Change, 8, 914–920, https://doi.org/10.1038/s41558-018-0283-x, 2018.
Grassi, G., Conchedda, G., Federici, S., Abad Viñas, R., Korosuo, A., Melo, J., Rossi, S., Sandker, M., Somogyi, Z., Vizzarri, M., and Tubiello, F. N.: Carbon fluxes from land 2000–2020: bringing clarity to countries' reporting, Earth Syst. Sci. Data, 14, 4643–4666, https://doi.org/10.5194/essd-14-4643-2022, 2022.
Gütschow, J., Jeffery, M. L., Gieseke, R., Gebel, R., Stevens, D., Krapp, M., and Rocha, M.: The PRIMAP-hist national historical emissions time series, Earth Syst. Sci. Data, 8, 571–603, https://doi.org/10.5194/essd-8-571-2016, 2016.
Gütschow, J., Günther, A., Jeffery, M. L., and Gieseke, R.: The PRIMAP-hist national historical emissions time series (1850–2018) v2.2, Zenodo, https://doi.org/10.5281/zenodo.4479172, 2021.
IIASA: International Institute for Applied Systems Analysis 2017 Annual Report, https://ar17.iiasa.ac.at/wp-content/uploads/sites/3/AR17.pdf, (last access: 13 February 2022), 2017.
International Monetary Fund (IMF): World Economic Outlook, Washington, DC, https://www.imf.org/external/pubs/ft/weo/2020/02/weodata/index.aspx (last access: 23 February 2022), 2020.
IPCC: Good Practice Guidance for Land Use, Land Use Change and Forestry, https://www.ipcc.ch/publication/good-practice-guidance-for-land-use-land-use-change-andforestry/, (last access: 10 July 2022), 2003.
IPCC: IPCC Guidelines for National Greenhouse Gas Inventories, prepared by the National Greenhouse Gas Inventories Program, edited by: Eggleston, H. S., Buendia, L., Miwa, K., Ngara, T., and Tanabe, K., IGES, Japan, ISBN 4-88788-032-4, 2006.
IPCC: Climate Change 2007: The Physical Science Basis. Contribution of Working Group I to the Fourth Assessment Report of the Intergovernmental Panel on Climate Change, edited by: Solomon, S., Qin, D., Manning, M., Chen, Z., Marquis, M., Averyt, K. B., Tignor, M., and Miller, H. L., Cambridge University Press, Cambridge, United Kingdom and New York, NY, USA, 2007.
IPCC: 2019 Refinement to the 2006 IPCC Guidelines for National Greenhouse Gas Inventories, edited by: Buendia, E., Tanabe, K. , Kranjc, A., Baasansuren, J., Fukuda, M., Ngarize, S., Osako, A., Pyrozhenko, Y., Shermanau, P., and Federici, S., Intergovemmental Panel on Climate Change (IPCC), Switzerland, ISBN 978-4-88788-232-4, 2019.
IPCC: Climate Change 2021: The Physical Science Basis. Contribution of Working Group I to the Sixth Assessment Report of the Intergovernmental Panel on Climate Change, edited by: Masson-Delmotte, V., Zhai, P., Pirani, A., Connors, S. L., Péan, C., Berger, S., Caud, N., Chen, Y., Goldfarb, L., Gomis, M. I., Huang, M., Leitzell, K., Lonnoy, E., Matthews, J. B. R., Maycock, T. K., Waterfield, T., Yelekçi, O., Yu, R., and Zhou, B., Cambridge University Press, Cambridge, United Kingdom and New York, NY, USA, https://doi.org/10.1017/9781009157896, 2021.
IPCC: Climate Change and Land: an IPCC special report on climate change, desertification, land degradation, sustainable land management, food security, and greenhouse gas fluxes in terrestrial ecosystems, edited by: Shukla, P. R., Skea, J., CalvoBuendia, E., Masson-Delmotte, V., Pörtner, H.-O., Roberts, D. C., Zhai, P., Slade, R., Connors, S., van Diemen, R., Ferrat, M., Haughey, E., Luz, S., Neogi, S., Pathak, M., Petzold, J., Portugal Pereira, J., Vyas, P., Huntley, E., Kissick, K., Belkacemi, M., and Malley, J., https://doi.org/10.1017/9781009157988, 2022.
Janssens-Maenhout, G., Pinty, B., Dowell, M., Zunker, H., Andersson, E., Balsamo, G., Bézy, J.-L., Brunhes, T., Bösch, H., Bojkov, B., Brunner, D., Buchwitz, M., Crisp, D., Ciais, P., Counet, P., Dee, D., Gon, H. D. van der, Dolman, H., Drinkwater, M. R., Dubovik, O., Engelen, R., Fehr, T., Fernandez, V., Heimann, M., Holmlund, K., Houweling, S., Husband, R., Juvyns, O., Kentarchos, A., Landgraf, J., Lang, R., Löscher, A., Marshall, J., Meijer, Y., Nakajima, M., Palmer, P. I., Peylin, P., Rayner, P., Scholze, M., Sierk, B., Tamminen, J., and Veefkind, P.: Toward an Operational Anthropogenic CO2 Emissions Monitoring and Verification Support Capacity, B. Am. Meteor. Soc., 101, E1439–E1451, https://doi.org/10.1175/BAMS-D-19-0017.1, 2020.
Kim, D.-G., Thomas, A. D., Pelster, D., Rosenstock, T. S., and Sanz-Cobena, A.: Greenhouse gas emissions from natural ecosystems and agricultural lands in sub-Saharan Africa: synthesis of available data and suggestions for further research, Biogeosciences, 13, 4789–4809, https://doi.org/10.5194/bg-13-4789-2016, 2016.
Liousse, C., Assamoi, E., Criqui, P., Granier, C., and Rosset, R.: Explosive growth in African combustion emissions from 2005 to 2030, Environ. Res. Lett., 9, 035003, https://doi.org/10.1088/1748-9326/9/3/035003, 2014.
Mostefaoui, M., Ciais, P., McGrath, M. J., Peylin, P., Prabir, P. K., Saunois, M., Chevallier, F., Sitch, S., Rodenbeck, C., Luijkx, I., and Thompson, R.: Datasets for greenhouse gasses emissions and removals from inventories and global models over Africa v0.1, Zenodo [data set], https://doi.org/10.5281/zenodo.7347077, 2022.
Monks, S. A., Arnold, S. R., Hollaway, M. J., Pope, R. J., Wilson, C., Feng, W., Emmerson, K. M., Kerridge, B. J., Latter, B. L., Miles, G. M., Siddans, R., and Chipperfield, M. P.: The TOMCAT global chemical transport model v1.6: description of chemical mechanism and model evaluation, Geosci. Model Dev., 10, 3025–3057, https://doi.org/10.5194/gmd-10-3025-2017, 2017.
Nickless, A., Scholes, R. J., Vermeulen, A., Beck, J., López-Ballesteros, A., Ardö, J., Karstens, U., Rigby, M., Kasurinen, V., Pantazatou, K., Jorch, V., and Kutsch, W.: Greenhouse gas observation network design for Africa, Tellus B, 72, 1–30, https://doi.org/10.1080/16000889.2020.1824486, 2020.
Patra, P. K., Takigawa, M., Watanabe, S., Chandra, N., Ishijima, K., and Yamashita, Y.: Improved Chemical Tracer Simulation by MIROC4.0-based Atmospheric Chemistry-Transport Model (MIROC4-ACTM), Sola, 14, 91–96, https://doi.org/10.2151/sola.2018-016, 2018.
Perugini, L., Pellis, G., Grassi, G., Ciais, P., Dolman, H., House, J. I., Peters, G. P., Smith, P., Günther, D., and Peylin, P.: Emerging reporting and verification needs under the Paris Agreement: How can the research community effectively contribute?, Environ. Sci. Policy, 122, 116–126, https://doi.org/10.1016/j.envsci.2021.04.012, 2021.
Petrescu, A. M. R., Qiu, C., Ciais, P., Thompson, R. L., Peylin, P., McGrath, M. J., Solazzo, E., Janssens-Maenhout, G., Tubiello, F. N., Bergamaschi, P., Brunner, D., Peters, G. P., Höglund-Isaksson, L., Regnier, P., Lauerwald, R., Bastviken, D., Tsuruta, A., Winiwarter, W., Patra, P. K., Kuhnert, M., Oreggioni, G. D., Crippa, M., Saunois, M., Perugini, L., Markkanen, T., Aalto, T., Groot Zwaaftink, C. D., Tian, H., Yao, Y., Wilson, C., Conchedda, G., Günther, D., Leip, A., Smith, P., Haussaire, J.-M., Leppänen, A., Manning, A. J., McNorton, J., Brockmann, P., and Dolman, A. J.: The consolidated European synthesis of CH4 and N2O emissions for the European Union and United Kingdom: 1990–2017, Earth Syst. Sci. Data, 13, 2307–2362, https://doi.org/10.5194/essd-13-2307-2021, 2021.
Pongratz, J., Reick, C. H., Houghton, R. A., and House, J. I.: Terminology as a key uncertainty in net land use and land cover change carbon flux estimates, Earth Syst. Dynam., 5, 177–195, https://doi.org/10.5194/esd-5-177-2014, 2014.
PRIMAP-hist: PRIMAP-hist dataset, https://www.pik-potsdam.de/paris-reality-check/primap-hist (last access: 23 April 2022), 2021.
REDD+, UNFCCC: REDD+ National Reports, REDD+, UNFCCC [data set], https://redd.unfccc.int/submissions.html?topic=6, last access: 13 August 2022.
Rödenbeck, C.: Estimating CO2 sources and sinks from atmospheric mixing ratio measurements using a global inversion of atmospheric transport, Technical Reports – Max-Planck-Institut für Biogeochemie 6, https://www.bgc-jena.mpg.de/~christian.roedenbeck/download/2005-Roedenbeck-TechReport6.pdf (last access: 24 April 2022), 2005.
Rodgers, C. D.: Inverse Methods For Atmospheric Sounding: Theory And Practice, World Scientific, 256 pp., ISBN 981022740X, 2000.
Saunois, M., Stavert, A. R., Poulter, B., Bousquet, P., Canadell, J. G., Jackson, R. B., Raymond, P. A., Dlugokencky, E. J., Houweling, S., Patra, P. K., Ciais, P., Arora, V. K., Bastviken, D., Bergamaschi, P., Blake, D. R., Brailsford, G., Bruhwiler, L., Carlson, K. M., Carrol, M., Castaldi, S., Chandra, N., Crevoisier, C., Crill, P. M., Covey, K., Curry, C. L., Etiope, G., Frankenberg, C., Gedney, N., Hegglin, M. I., Höglund-Isaksson, L., Hugelius, G., Ishizawa, M., Ito, A., Janssens-Maenhout, G., Jensen, K. M., Joos, F., Kleinen, T., Krummel, P. B., Langenfelds, R. L., Laruelle, G. G., Liu, L., Machida, T., Maksyutov, S., McDonald, K. C., McNorton, J., Miller, P. A., Melton, J. R., Morino, I., Müller, J., Murguia-Flores, F., Naik, V., Niwa, Y., Noce, S., O'Doherty, S., Parker, R. J., Peng, C., Peng, S., Peters, G. P., Prigent, C., Prinn, R., Ramonet, M., Regnier, P., Riley, W. J., Rosentreter, J. A., Segers, A., Simpson, I. J., Shi, H., Smith, S. J., Steele, L. P., Thornton, B. F., Tian, H., Tohjima, Y., Tubiello, F. N., Tsuruta, A., Viovy, N., Voulgarakis, A., Weber, T. S., van Weele, M., van der Werf, G. R., Weiss, R. F., Worthy, D., Wunch, D., Yin, Y., Yoshida, Y., Zhang, W., Zhang, Z., Zhao, Y., Zheng, B., Zhu, Q., Zhu, Q., and Zhuang, Q.: The Global Methane Budget 2000–2017, Earth Syst. Sci. Data, 12, 1561–1623, https://doi.org/10.5194/essd-12-1561-2020, 2020.
Schulz, E., Speekenbrink, M., and Krause, A.: A tutorial on Gaussian process regression: Modelling, exploring, and exploiting functions, J. Math. Psychol., 85, 1–16, https://doi.org/10.1016/j.jmp.2018.03.001, 2018.
Thompson, R. L., Chevallier, F., Crotwell, A. M., Dutton, G., Langenfelds, R. L., Prinn, R. G., Weiss, R. F., Tohjima, Y., Nakazawa, T., Krummel, P. B., Steele, L. P., Fraser, P., O'Doherty, S., Ishijima, K., and Aoki, S.: Nitrous oxide emissions 1999 to 2009 from a global atmospheric inversion, Atmos. Chem. Phys., 14, 1801–1817, https://doi.org/10.5194/acp-14-1801-2014, 2014.
Tian, H., Xu, R., Canadell, J. G., Thompson, R. L., Winiwarter, W., Suntharalingam, P., Davidson, E. A., Ciais, P., Jackson, R. B., Janssens-Maenhout, G., Prather, M. J., Regnier, P., Pan, N., Pan, S., Peters, G. P., Shi, H., Tubiello, F. N., Zaehle, S., Zhou, F., Arneth, A., Battaglia, G., Berthet, S., Bopp, L., Bouwman, A. F., Buitenhuis, E. T., Chang, J., Chipperfield, M. P., Dangal, S. R. S., Dlugokencky, E., Elkins, J. W., Eyre, B. D., Fu, B., Hall, B., Ito, A., Joos, F., Krummel, P. B., Landolfi, A., Laruelle, G. G., Lauerwald, R., Li, W., Lienert, S., Maavara, T., MacLeod, M., Millet, D. B., Olin, S., Patra, P. K., Prinn, R. G., Raymond, P. A., Ruiz, D. J., van der Werf, G. R., Vuichard, N., Wang, J., Weiss, R. F., Wells, K. C., Wilson, C., Yang, J., and Yao, Y.: A comprehensive quantification of global nitrous oxide sources and sinks, Nature, 586, 248–256, https://doi.org/10.1038/s41586-020-2780-0, 2020.
UNFCCC: UNFCCC: National Inventory Submissions, https://unfccc.int/, last access: 28 March 2021.
United Nations Department of Economic and Social Affairs, Population Division: World Population Prospects 2019: Summary of Results, 2019.
Valentini, R., Arneth, A., Bombelli, A., Castaldi, S., Cazzolla Gatti, R., Chevallier, F., Ciais, P., Grieco, E., Hartmann, J., Henry, M., Houghton, R. A., Jung, M., Kutsch, W. L., Malhi, Y., Mayorga, E., Merbold, L., Murray-Tortarolo, G., Papale, D., Peylin, P., Poulter, B., Raymond, P. A., Santini, M., Sitch, S., Vaglio Laurin, G., van der Werf, G. R., Williams, C. A., and Scholes, R. J.: A full greenhouse gases budget of Africa: synthesis, uncertainties, and vulnerabilities, Biogeosciences, 11, 381–407, https://doi.org/10.5194/bg-11-381-2014, 2014.
van der Laan-Luijkx, I. T., van der Velde, I. R., van der Veen, E., Tsuruta, A., Stanislawska, K., Babenhauserheide, A., Zhang, H. F., Liu, Y., He, W., Chen, H., Masarie, K. A., Krol, M. C., and Peters, W.: The CarbonTracker Data Assimilation Shell (CTDAS) v1.0: implementation and global carbon balance 2001–2015, Geosci. Model Dev., 10, 2785–2800, https://doi.org/10.5194/gmd-10-2785-2017, 2017.
van der Werf, G. R., Randerson, J. T., Giglio, L., van Leeuwen, T. T., Chen, Y., Rogers, B. M., Mu, M., van Marle, M. J. E., Morton, D. C., Collatz, G. J., Yokelson, R. J., and Kasibhatla, P. S.: Global fire emissions estimates during 1997–2016, Earth Syst. Sci. Data, 9, 697–720, https://doi.org/10.5194/essd-9-697-2017, 2017.
van der Zwaan, B., Kober, T., Longa, F. D., van der Laan, A., and Jan Kramer, G.: An integrated assessment of pathways for low-carbon development in Africa, Energ. Pol., 117, 387–395, https://doi.org/10.1016/j.enpol.2018.03.017, 2018.
Wilson, C., Chipperfield, M. P., Gloor, M., and Chevallier, F.: Development of a variational flux inversion system (INVICAT v1.0) using the TOMCAT chemical transport model, Geosci. Model Dev., 7, 2485–2500, https://doi.org/10.5194/gmd-7-2485-2014, 2014.
World Bank: GDP exchange rate estimates, https://data.worldbank.org/indicator/NY.GDP.MKTP.CD (last access: 15 August 2022), 2019.
World Bank: World Bank economic data, https://www.worldbank.org/ (last access: 22 May 2022), 2022.
Zhu, Y., Merbold, L., Pelster, D., Diaz-Pines, E., Wanyama, G. N., and Butterbach-Bahl, K.: Effect of Dung Quantity and Quality on Greenhouse Gas Fluxes From Tropical Pastures in Kenya, Glob. Biogeochem. Cy., 32, 1589–1604, https://doi.org/10.1029/2018GB005949, 2018.
- Abstract
- Introduction
- Methods, datasets and dataset usage
- Results and discussion
- Discussion: uncertainties, comparison between BU and TD methods as well as synthesis for the three main GHGs
- Data availability
- Summary, concluding remarks and perspectives
- Author contributions
- Competing interests
- Disclaimer
- Acknowledgements
- Financial support
- Review statement
- References
- Supplement
- Abstract
- Introduction
- Methods, datasets and dataset usage
- Results and discussion
- Discussion: uncertainties, comparison between BU and TD methods as well as synthesis for the three main GHGs
- Data availability
- Summary, concluding remarks and perspectives
- Author contributions
- Competing interests
- Disclaimer
- Acknowledgements
- Financial support
- Review statement
- References
- Supplement