the Creative Commons Attribution 4.0 License.
the Creative Commons Attribution 4.0 License.
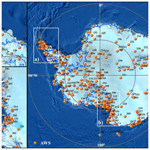
The AntAWS dataset: a compilation of Antarctic automatic weather station observations
Xueying Zhang
Wentao Ning
Matthew A. Lazzara
Minghu Ding
Carleen H. Reijmer
Paul C. J. P. Smeets
Paolo Grigioni
Petra Heil
Elizabeth R. Thomas
David Mikolajczyk
Lee J. Welhouse
Linda M. Keller
Zhaosheng Zhai
Yuqi Sun
A new meteorological dataset derived from records of Antarctic automatic weather stations (here called the AntAWS dataset) at 3 h, daily and monthly resolutions including quality control information is presented here. This dataset integrates the measurements of air temperature, air pressure, relative humidity, and wind speed and direction from 267 Antarctic AWSs obtained from 1980 to 2021. The AWS spatial distribution remains heterogeneous, with the majority of instruments located in near-coastal areas and only a few inland on the East Antarctic Plateau. Among these 267 AWSs, 63 have been operating for more than 20 years and 27 of them in excess of more than 30 years. Of the five meteorological parameters, the measurements of air temperature have the best continuity and the highest data integrity. The overarching aim of this comprehensive compilation of AWS observations is to make these data easily and widely accessible for efficient use in local, regional and continental studies; it may be accessed at https://doi.org/10.48567/key7-ch19 (Wang et al., 2022). This dataset is invaluable for improved characterization of the surface climatology across the Antarctic continent, to improve our understanding of Antarctic surface snow–atmosphere interactions including precipitation events associated with atmospheric rivers and to evaluate regional climate models or meteorological reanalysis products.
- Article
(11421 KB) - Full-text XML
-
Supplement
(1809 KB) - BibTeX
- EndNote
Against the background of global warming, Antarctica plays a crucial role in the global sea level rise, changes in the atmospheric circulation and heat balance, and its general climate evolution and thus has experienced intense scientific scrutiny (e.g., IPCC, 2019; Kennicutt et al., 2019; Rignot et al., 2019). In recent decades, much attention has been paid to changes in atmospheric variables, such as air temperature, snow deposition, and wind speed over the Antarctic continent (Huai et al., 2019; Dong et al., 2020; IPCC, 2021), because they have a profound impact on the surface energy balance, changes in the ice sheet mass, as well as the ecosystem in coastal and surrounding regions (e.g., Giovinetto et al., 1990; Gregory et al., 2006; Herbei et al., 2016; Convey et al., 2018). To quantify the underlying variability and trends, accurate and continuous atmospheric measurements like these are a vital prerequisite.
Extensive efforts have been made to obtain continuous atmospheric observations in Antarctica since the International Geophysical Year (IGY) in 1957/1958. For example, a total of approximately 50 staffed stations were established by the end of the IGY, of which 17 have continuous meteorological records to date (Summerhayes et al., 2008; Lazzara et al., 2013). Nevertheless, the majority of the staffed stations are concentrated along the coast, and only seven stations are located in the interior of the Antarctic continent (Allison et al., 1993), which is insufficient to resolve the atmospheric conditions of the interior of Antarctica. At the same time, harsh weather conditions as well as the unique geographical topography and remoteness of Antarctica make it extremely difficult to install and maintain staffed stations. Automatic weather stations (AWSs) have the advantage of collecting meteorological data in remote areas or severe weather conditions and help to fill the gaps of staffed weather observations (Stearns and Wendler, 1988; Allison et al., 1993; Stearns et al., 1993; Reijmer and Oerlemans, 2002; Renfrew and Anderson, 2002). A sustained AWS network is required to observe the weather and climate across the Antarctic continent (Lazzara et al., 2013).
Remote AWSs became practical with the advent of the Advanced Research and Global Observation Satellite network (ARGOS) data relay system on polar-orbiting satellites in 1978, and thus real-time or near-real-time meteorological data could be obtained from remote high-latitude locations. Based on this, numerous national programs independently developed AWSs to support atmospheric observations, glaciological studies, and monitoring projects in Antarctica. Since 1979, the United States Antarctic Program (USAP) has supported the University of Wisconsin-Madison (UW-Madison) in the deployment of AWSs in Antarctica, mainly located in the Ross Ice Shelf and the West Antarctic Ice Sheet, beyond an initial landmark research effort by Stanford University (Stearns et al., 1993; Lazzara et al., 2012). In 1982, the Australian Antarctic Division (AAD) deployed its first Antarctic AWS inland from Casey Station (Allison and Morrissy, 1983). As part of the International Antarctic Glaciology Program, an AWS network was deployed inland from Casey Station (Allison et al., 1993). Later, the Australian National Antarctic Research Expedition (ANARE) set up a regional AWS network with an updated AWS version around the Lambert Glacier (Allison, 1998). In 1985, the Italian National Program of Antarctic Research (PNRA) installed its first AWS, in Terra Nova Bay, named “Mario Zucchelli”. Currently its AWS network is mainly located in Victoria Land and on the Antarctic Plateau. Over the Antarctic Peninsula and Dronning Maud Land, the British Antarctic Survey (BAS, who did collaborate with UW-Madison initially) and the Institute for Marine and Atmospheric Research, Utrecht University (IMAU), installed their respective AWS networks. The Chinese National Antarctic Research Expedition (CHINARE) installed its PANDA AWS network, including 11 AWSs from the coast to the summit of the East Antarctic Plateau (Ding et al., 2022). There are other AWS networks in the Antarctic of several nations (e.g., Japan, France, New Zealand, South Korea). Despite the different designs of AWSs between nations, all stations obtain measurements of air temperature, air pressure, relative humidity as well as wind speed and direction.
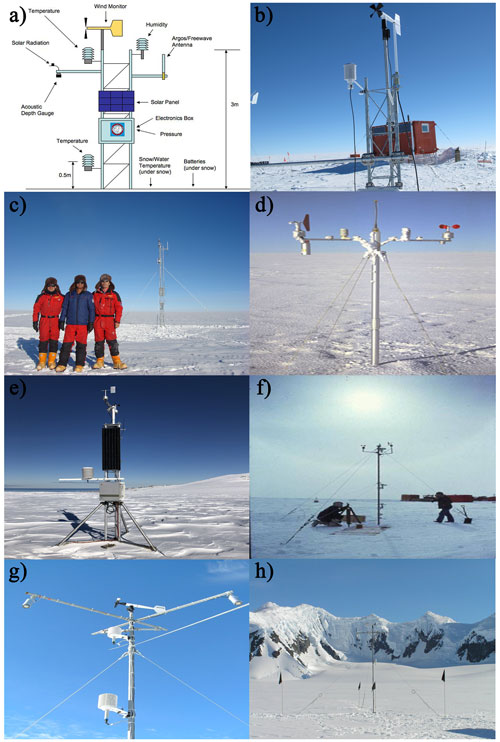
Figure 1Typical AWSs of the six research institutions, but the sensors at other sites vary slightly depending on the local environment. (a) AMRC-CR1000 device, (b) AMRC-AGO-4, (c) AMRC and CHINARE-Panda_South, (d) IMAU-AWS10, (e) PNRA-Maria, (f) AAD-LGB00, (g) BAS-the sensors used on Latady, and (h) BAS-Latady. (a) http://amrc.ssec.wisc.edu/news/2010-May-01.html (last access: 25 April 2022), (b) https://amrc.ssec.wisc.edu/aws/images/station_images/AGO_4.jpg (last access: 4 November 2022), (c) personal communication with Minghu Ding, 2022, (d) https://www.projects.science.uu.nl/iceclimate/aws/technical.php (last access: 25 April 2022), (e) https://www.climantartide.it/attivita/aws/index.php?lang=en (last access: 4 November 2022), (f) personal communication with Ian Allison, 2022, and (g) and (h) https://ramadda.data.bas.ac.uk/repository/entry/show?entryid=synth:44d1a477-0852-4620-a1f4-63f559b44e94:L0RvY3VtZW50cy9waG90b3NfYXdz (last access: 25 April 2022).
Given the funding constraints of different national Antarctic programs, AWSs provide the most economical way of obtaining weather data in support of ongoing scientific applications, numerical weather prediction, remote field activities and the planning of maintenance visits. Early scientific studies supported by Antarctic AWSs focused on the local meteorological processes and the climatology of basic atmospheric parameters, such as temperature, pressure and wind (Allison et al., 1993; Stearns et al., 1993; Aristidi et al., 2005; Seefeldt et al., 2007). Over the Antarctic Ice Sheet (AIS), there are still missing data points in the record of each AWS, which present a constraint on the climatological studies. Spatial and temporal interpolations are required, often used to fill any data gaps in the generation of continuous time series of meteorological variables (e.g., Shuman and Stearns, 2001; Bromwich et al., 2013, 2014; Reusch and Alley, 2004). In addition, the AWS observations have also been used to evaluate and validate atmospheric reanalysis products, regional climate models and remote sensing retrievals (e.g., Gallée and Gorodetskaya, 2010; Tastula et al., 2012; Wang et al., 2013; Huai et al., 2019). Antarctic AWS observations are also used in the glaciological studies, such as estimates of snow accumulation (e.g., Wang et al., 2021), calculation of the surface energy balance (e.g., van Wessem et al., 2014) and understanding of the AIS mass changes (e.g., Knuth et al., 2010).
To better characterize the regional or even continental weather and climate status over Antarctica, many attempts have been made to compile all available past and present AWS observations into the Antarctic climate database. Jacka et al. (1984) carried out the pioneering work to compile all annually and monthly averaged temperature observations of Antarctic and Southern Ocean island stations. Jones and Limbert (1987) assembled an integrated annual and monthly mean sea level pressure and temperature dataset from 29 weather stations located at 60–90∘ S. Stearns et al. (1993) provided a detailed description of the monthly mean climate data, including monthly mean and extreme values of temperature, pressure, wind speed and direction collected by the Antarctic AWSs and processed at UW-Madison. This dataset is continuously updated. Turner et al. (2004) described the Reference Antarctic Data for Environmental Research (READER) by the Scientific Committee on Antarctic Research (SCAR). Their dataset includes the monthly and annual mean near-surface air temperature, pressure and wind speed data from 43 staffed stations and 61 AWSs. Rodrigo et al. (2013) compiled Antarctic surface wind observations from 115 AWSs to assess the performance of regional climate models and ERA-40 and ERA-Interim reanalysis products. These AWS observation compilations generally suffer from a range of limitations, including the duration of datasets, collection of single meteorological parameters only, low temporal data resolution, limited spatial coverage, limited or no rigorous quality control, and in some cases limited public accessibility. Most recently, Kittel (2021) compiled a near-surface weather observation database at a high temporal resolution, which to a great extent remedied the deficiency of the previous database and has already been used in the studies of the ice sheet surface processes, climate model validation and atmospheric diagnoses (e.g., Donat-Magnin et al., 2020; Kittel, 2021; Kittel et al., 2021; Mottram et al., 2021; Wille et al., 2022). However, these data were only qualitatively compared with models to detect and remove any outliers, and they are still not widely available. Thus, better composition and quality control would allow for a more reliable dataset.
In this study, our main goal is to use all available Antarctic AWS records to construct a comprehensive quality-controlled database of Antarctic meteorological variables, including air temperature, air pressure, relative humidity as well as wind speed and direction. The database provides 3 h, daily and monthly records. We describe the methods used to generate this dataset, including criteria for record inclusion and data quality control. In addition, the main temporal and spatial features of the database are summarized.
AWSs are ground-based meteorological data collection devices, which after their deployment may be run without any on-site support and all year round. All Antarctic AWSs are similar in design. They are equipped with a set of standard atmospheric sensors based on the standards of the World Meteorological Organization (WMO, 2018). The UW-Madison AWS network at the Antarctic Meteorological Research Center (AMRC) initially consisted of dataloggers developed in-house at UW-Madison, with the AWS 2B series becoming their primary electronics system in the 1980s and early 1990s. Beginning in the late 1990s, UW-Madison switched to using commercial off-the-shelf dataloggers manufactured by Campbell Scientific. Currently, the primary AWS system used by the AMRC is composed of a Campbell Scientific CR1000 device datalogger, which is a commercial off-the-shelf system wired and programmed much like AMRC's original AWS 2B series. The CR1000 datalogger has the ability to keep track of additional weather observations on AWSs which the AWS 2B system cannot measure, such as snow accumulation and incoming/outgoing shortwave/longwave radiation. Initially, the British Antarctic Survey (BAS) employed its in-house AWS technology and then, in collaboration with UW-Madison, switched to using the CR1000 datalogger. The IMAU Antarctic AWS Project also used the CR1000 device and a custom system. Most of the AWSs of the PNRA are acquisition and control units provided by Vaisala series. The glaciology program of the AAD designed and built three of their own AWS types over the past 20 years, with the latest version being series 098 AWSs. The CHINARE AWSs consist of standard components provided by Campbell Scientific and within the Vaisala series, except for the XFY3-1 sensor, a domestic propeller anemometer (Ding et al., 2022). The supporting framework for AWS instruments varies between models, but in general, the AWS body is made up of a mast and instrument arms fitted with different sensors. The AWS datalogger, satellite transmitter, pressure sensor, power-regulating circuit and battery are generally installed in a box (or a series of boxes) at the base of the mast. In summer, the battery is charged by a small solar panel installed vertically near the top of the mast. However, the sensors of the AMRC AWS are mounted on Röhn tower sections, and similar towers have been used by others. Table 1 presents the different types of sensors used on the AWSs and the corresponding techniques in detail. Although the instrument manufacturers may vary across the different AWS networks, the measuring range, accuracy and resolution are identical or at least similar. Figure 1 shows the typical AWSs in the four Antarctic research projects, but other AWSs may have different sensors depending on the local environment.
Typically an AWS system stores meteorological observations locally on a datalogger, which is convenient for managing operations (e.g., DT50, CR1000). The datalogger transmits the observations through the ARGOS system, carried on board the National Oceanic and Atmospheric Administration (NOAA) (NOAA-19 and earlier) and Metop series of polar-orbiting satellites. Figure 2 provides the data acquisition diagram of the AWSs, taking the Wisconsin AMRC AWS relay network as an example. The default way how AMRC receives the AWS data via ARGOS (the archive data) is directly through file transfer protocol (FTP) services from the Service ARGOS complete worldwide collection system, including all data (e.g., repeated data transmissions). These data are regularly processed into meteorological values via the quality control and then provided to the community. AMRC also has a set of newer AWS units using the Iridium communications system.
Each AWS measures air temperature, pressure, relative humidity and other meteorological elements at a height range of ∼1 to 6 m above the surface of the Antarctic Ice Sheet, which are the initial heights when an AWS was installed prior to any local snow accumulation and site tilt, except for Zhongshan Station, which measures wind speed and wind direction at a height of 10 m. In fact, due to the accumulation of snow, the measurement height of each meteorological variable varies over time, which may result in the notable meteorological observation disparities such as temperature and wind speed caused by the instrument height differences. Some AWSs also measure air temperature, wind speed and other variables at multiple heights to provide near-ground vertical gradient data, which is convenient for checking the accuracy of data and the redundancy of certain sensors. Some AWSs have added sensors that measure snow temperature at different depths, solar radiation and snow depth as well as a series of internal management parameters, such as battery voltage and internal temperature (see Fig. 1).
Cost-effective AWSs provide timely research data and input to numerical weather prediction from remote areas on the Antarctic Ice Shelf throughout the year. Maintenance is still required, and generally one visit is performed per summer to ensure that electric power generation and battery capacity are sufficient for operation during the upcoming polar night. However, several AWSs have not been revisited after initial deployment. For example, since its first deployment in October 1984, AWS GC41 has been operating continuously in the interior of Antarctica with no maintenance access. The accuracy of the data from these sites can only be estimated by the internal consistency of the diverse sensors.
Table 1The sensor types used on Antarctic automatic weather stations and the technical specifications.
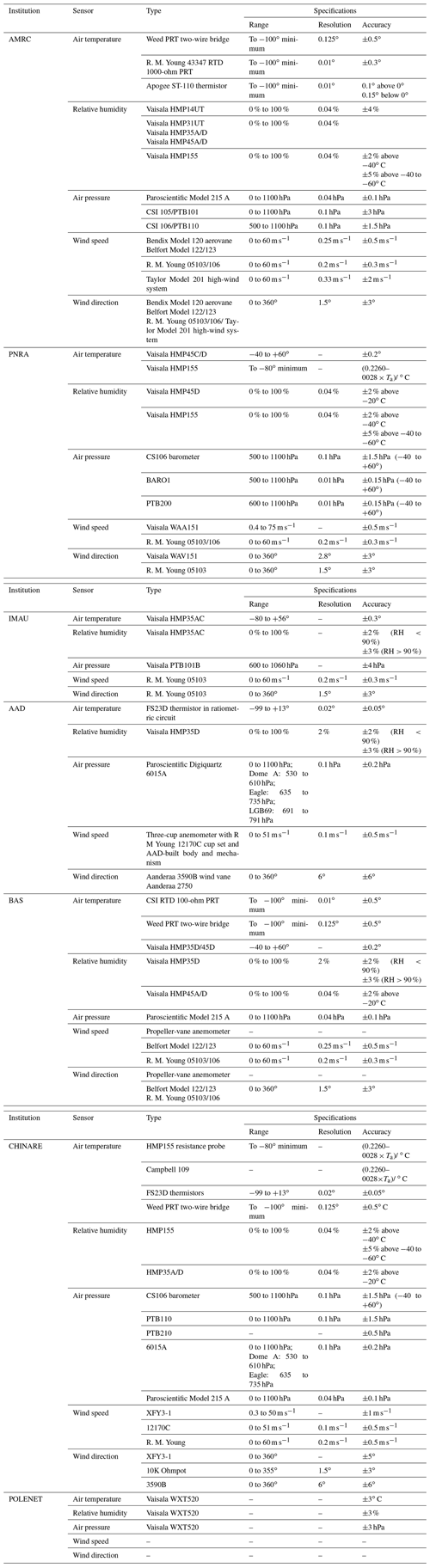
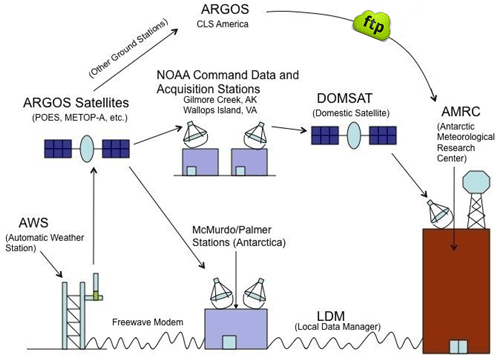
Figure 2Data acquisition diagram of AWS, using AMRC as an example. http://amrc.ssec.wisc.edu/aws/images/datastream_v2.jpg (last access: 8 November 2021).
3.1 Data collections and sources
Here the AWS meteorological observations were obtained from seven Antarctic AWS project databases, including the CHINARE (https://doi.org/10.11888/Atmos.tpdc.272721, Ding et al., 2022), the BAS (https://data.bas.ac.uk/datasets.php, last access: 19 October 2022), the PNRA (http://www.climantartide.it, last access: 17 October 2022), the IMAU Antarctic AWS Project (https://www.projects.science.uu.nl/iceclimate/aws/antarctica.php, last access: 24 October 2022) (data available at https://doi.org/10.1594/PANGAEA.910473, Jakobs et al., 2020), the AAD (http://aws.cdaso.cloud.edu.au/datapage.html, last access: 19 October 2022), the AMRC (http://amrc.ssec.wisc.edu/, last access: 15 October 2022) at the University of Wisconsin (Lazzara et al., 2012), and the Polar Earth Observing Network (POLENET) program (https://www.unavco.org/, last access: 29 October 2022). The AMRC includes not only its own AWS network, but also brings together data from several Antarctic research programs, such as the Japanese Antarctic Research Expedition (JARE), the French Antarctic Program (Institut Polaire Francais-Paul Emile Victor, IPEV), the AAD, the BAS and the CHINARE. The JARE installed and maintained JASE2007, Dome Fuji, Mizuho and Relay Station on the East Antarctic Plateau. The IPEV installed and took charge of the AWSs from the Adélie Coast to Dome C, including Port Martin, D-10, D-17, D-47, D-85, Dome C and Dome C II. Cape Denison AWS on the Adélie Coast is serviced by the AAD. The BAS installed and maintains the AWSs on the Antarctic Peninsula and the East Antarctic Plateau, including Butler Island, Larsen Ice Shelf, Limbert, Sky-Blu, Fossil Bluff, Dismal Island and Baldrick. The PANDA_South AWS, located on the East Antarctic Plateau, is a cooperation between CHINARE and AMRC, which was installed, maintained and operated by CHINARE.
Firstly, AWSs with data records of less than 1 year in length are excluded. Then, the records from all the remaining stations were collected. In total, measurements from 267 AWSs were compiled, including at least one of the five meteorological variables, i.e., near-surface air temperature, relative humidity, air pressure, wind speed and wind direction. Figure 3 shows the spatial distribution of the 267 AWSs, and the corresponding longitude and latitude coordinates, elevation and data sources of these AWSs are summarized in Table S1 in the Supplement.
3.2 Quality control
The quality check of observational data is aimed at detecting missing data and errors, including any due to transmission issues, to provide the highest possible standard of accuracy. Our compilation is based on the hourly and 3 h synoptic measurements from AWSs, which were subjected to quality checks by data providers, including a coarse error check using threshold values at the time of decoding, manually filtering errors or gaps due to the presence of instrument failures such as sensor freezing and screens covered by snow/frost, transmission issues through the datalogger, Global Telecommunications System (GTS) or ARGOS, and changes in units. Despite the quality checks, previous studies pointed out that caution should be taken when using these AWS data, at least wind speed data, which are the least reliable variable of the measurements (e.g., Stearns et al., 1993). To perform a more rigorous quality control, a set of interactive quality control programs using interactive data language (IDL) software was developed for the quality check of the AMRC data (Lazzara et al., 2012). In our compilation, we use the 3 h AMRC AWS data through preliminary quality control. Since our objective was to construct a dataset with high quality, restrictive quality control criteria were used to filter the compiled data from a variety of sources.
First, we removed the records from the dataset outside the measurement range of sensors installed over the AWSs (Table 2). Data with zero values for both wind speed and direction were also eliminated. Furthermore, if the wind speed and direction values remained unchanged for 6 consecutive hours, which is likely caused by a sensor seizing up due to very cold temperatures, the values were set to the null values (NA). Secondly, the mean and standard deviation were calculated for the 3 h data in each month. We also checked physically unrealistic rapid synoptic variability in the parameters using the 6 h change threshold values of 10 hPa for surface pressure, 5 ∘C for air temperature, and 74.08 km h−1 for wind speed (Turner et al., 2004). Following Lazzara et al. (2012), the observation values exceeding 3 standard deviations from the mean were considered to be possibly erroneous and thus were flagged. Thirdly, we flagged the air temperature records in the austral summer months (December–January–February) during the low wind speed conditions (less than 2 m s−1), which can result in a warm temperature bias during this period because of the lack of ventilation (Genthon et al., 2011; Lazzara et al., 2012; Jones et al., 2016). Lastly, after these physically based filters, we performed a visual cross-comparison of each time series of the filtered data with the corresponding outputs of ERA-5 (Hersbach et al., 2020) and MAR (Kittel, 2021) to further remove outliers and improve the reliability of the dataset.
3.3 Averaging procedure
For all meteorological variables, daily and monthly mean values are calculated from the 3 h data (eight values a day between 00:00 and 21:00 UTC). Unfortunately, at a number of instances data gaps occurred. For daily values to be included, at least two 3 h observed values (25 %) must be available on that day, since less than 25 % of the 3 h observations are not representative of the weather conditions of a day, and daily averages cannot be obtained. Then, if at least 25 % of the 3 h observations are available in a month, we calculate a monthly average. For monthly data, with less than 25 % of the 3 h observations available, this typically occurs when a weather station starts or ceases during a given month. This may lead to the deviation of the monthly average, especially in the period of rapid changes in meteorological conditions such as air temperature. All missing values are set to NA. To provide more reliable daily and monthly values, we also calculate the daily and monthly products using a 75 % threshold: that is, at least six 3 h observed values are available, based on Kittel (2021).
4.1 Air temperature
Air temperature is a sensitive indicator of the climate extremes experienced across the Antarctic continent. It is measured at heights of approximately 2 to 3 m above the ground, using a thermistor (such as the Apogee ST-110 thermistor and FS23D thermistor in ratiometric circuit) or resistive platinum probe (such as PRT series and Vaisala HMP series). The air temperature sensor is installed in the AWS's naturally ventilated radiation shields to protect the sensor from direct sunlight, and the measurement uncertainty is within . It should be emphasized that, over areas with strong temperature inversions, especially the Antarctic Plateau in winter, measurements of near-surface air temperature are influenced by changes in the height of sensors installed on an AWS (generally a relative “lowering”) caused by snow accumulation (Genthon et al., 2021).
Figure 5 and Tables S2–S4 show the mean, maximum and minimum values of 3 h, daily and monthly air temperature from each AWS. The overall statistical results highlight the effects of sea–land distribution and elevation, as the air temperature in coastal areas is generally higher than that in inland areas, showing a gradual decrease from coastal to inland areas. The near-surface temperature is clearly affected by elevation due to the adiabatic lapse rate (Martin and Peel, 1978), with a significant decrease in near-surface temperature with increasing elevation (Fig. 5). Figure 6 and Table S2 show that the mean temperature of 3 h data ranges from −59.94 to 2.13 ∘. The extreme maximum temperatures of the Antarctic Peninsula, most of the West AIS, the Ross Ice Shelf and Victoria Land are almost all over 0∘. The warmest AWSs are South Georgia 1, South Georgia 3 and King Edward Point, with elevations of 85, 53 and 346 m, respectively, and the maximum temperature can reach 15∘. The AWSs with extreme minimum temperatures below −70∘ C are mainly distributed in the East Antarctic Plateau. The minimum temperature value is lower than , occurring at aws12, aws13, Dome C and Dome F. Statistics of the daily air temperature indicate that the daily mean air temperature values range from −58.42 to 2.36∘ (Table S3). The maximum daily temperature occurs at King Edward Point Station on Berkner Island, reaching 13.95∘. The lowest daily temperature is , occurring at aws13. According to the statistical results of monthly data in Table S4, the mean temperature of monthly data ranges from −59.02 to 2.32∘. King Edward Point Station still has the highest monthly averaged air temperature of 5.9∘. Concordia, located on the East Antarctic Plateau, has the lowest monthly averaged temperature of .
4.2 Air pressure
All the AAD AWSs use Paroscientific digiquartz barometers, with an accuracy of ±0.2 hPa and a resolution of 0.1 hPa. AMRC AWSs also use Paroscientific digiquartz barometers (Paroscientific Model 215 A), which have a higher resolution of 0.04 hPa and an accuracy of ±0.1 hPa. Most AWSs at the other institutions use Vaisala's PTB series and Campbell's CS series. Both series of barometers use Vaisala's BAROCAP silicon capacitive absolute pressure sensor, which have excellent accuracy, repeatability, and long-term stability over a wide range of operating temperatures. The barometer kept in the electronics enclosure measures the station pressure and is not corrected to sea level. The accuracy of all air pressure measurements ranges from 0.15 to 4 hPa, depending on the sensor used.
Figure 6 and Table S2 show the mean, maximum and minimum pressure of the 267 AWSs at 3 h time resolution. The range of the mean air pressure values goes between 573.49 and 996.24 hPa. AWSs with a 3 h average pressure greater than 900 hPa are mainly located along the coast of the Ross Ice Shelf, the Antarctic Peninsula, Dronning Maud Land, the Lambert Glacier basin, and Victoria Land. The maximum 3 h air pressure is 1039.2 hPa at South Georgia 3, followed by the station on the Larsen Ice Shelf of the Antarctic Peninsula. The minimum (536 hPa) is present at Dome A Station, with an elevation of 4093 m. Mainly affected by elevation, the mean, maximum and minimum air pressure decreases with the increase in altitude and spatially decreases from the coast to the interior (Fig. 5). The major features of the spatial distribution of daily and monthly air pressure are almost the same as those of 3 h data.
4.3 Relative humidity
The height of the humidity sensor is often the same as that of the air temperature probe. Correct measurements of relative humidity are key to calculating sublimation. However, it is quite difficult to accurately measure, especially in Antarctica. The original network did not include such measurements, but humidity detectors (Vaisala HMP series) have been deployed since about 1990. Humidity measurements are based on a capacitive thin film polymer sensor. The resolution of the series of humidity sensors is approximately 1 %, and the annual drift in the field is approximately ±2 %–3 %. The Vaisala humicap, which itself takes the conversion of ice and water form into account, is factory calibrated to provide relative humidity with respect to liquid water even at below-freezing temperatures (Genthon et al., 2013; Amory, 2020). The relative humidity is computed with respect to liquid water. Data should be converted to get relative humidity with respect to ice using the method of Goff and Gratch (1945) (Amory, 2020), but these additional computed data are left for forthcoming papers. In Antarctica, even near the surface, the relative humidity with respect to ice often reaches well over 100 %, and this is especially frequent on the high Antarctic Plateau, where supersaturation often occurs (Genthon et al., 2017, 2022). The sensors used on the AWS cannot report supersaturation and measure humidity above 100 %, and as a consequence humidity data are biased low there.
Many AWSs lack relative humidity measurements in consecutive years or entirely, which culminates in great challenges to humidity research over the whole Antarctic continent. The relative humidity of the coastal AWSs is usually higher than that of the inland AWSs and shows similar spatial patterns to air temperature.
4.4 Wind speeds and directions
Wind speeds and directions are monitored at a height of approximately 3 m above the ice sheet surface (Lazzara et al., 2012). It is notable that, at Zhongshan Station, the 10 m wind directions are measured. Due to the influence of katabatic wind, the wind directions at this station are relatively stable and resemble the 3 m wind directions (Ma and Bian, 2014). Different sensors are used to measure wind speed and direction at different AWSs. The most widely used model is R. M. Young Company 05103/106, in which wind speeds are measured using an impeller anemometer that is a helical, four-blade impeller. The rotation of the impeller generates a signal proportional to wind speeds, and wind directions are measured using a potentiometer. In addition, some AWSs adopt the heated Vaisala WA15 series, which is based on precise sensors mounted on cross arms. Its WAA151 anemometer has the characteristics of fast response and low threshold. Similarly, the optoelectronic vane WAV151 has the advantages of counterbalance, sensitivity, accuracy and low threshold. It is more suitable for more demanding wind measurements. The measurement accuracy of wind speeds is approximately ±0.5 m s−1, and wind direction is . The wind direction listed is clockwise from 0 to 360∘ (so 90∘ is east, 180∘ is south, and 270∘ is west). The stations established by CHINARE use a domestic propeller anemometer (XFY3-1 sensor), which can measure the wind speed and direction of horizontal airflow at very low critical wind speed, with uncertainties of ±1 m s−1 and , respectively (Ding et al., 2022). It is important to recall that wind speed varies strongly with height in the first few meters above the surface, and the height of the sensors above the surface gradually decreases with snow accumulation, causing poorly known variations of the instrument height above the snow surface and affecting the data quality and consistency (Genthon et al., 2021). Still, information on the evolution of wind speed with time is important, but the modulus is not well known and is not consistent in the dataset. To improve the accuracy of air temperature and wind observations, the vertical temperature and wind profiles should be corrected by accounting for the sensor height variations, as done by Ma et al. (2008) and Smeets et al. (2018). However, these additional computed data will be left until we have sufficient snow height data.
The results of Fig. 6 and Tables S2, 3 and 4 show that wind speed is consistent whether parsed in 3 h values or in daily and monthly values, and so is wind direction. The mean near-surface wind speeds of the 267 AWSs vary from 2.17 to 23.66 m s−1. The average wind speed is higher along the East AIS coast, where the average wind speed exceeds 20 m s−1 (e.g., Cape Denison, Lucia, Virginia and Zoraida stations). The average wind speed at AGO-5, Dome C, Dome F and Dome A stations on the Antarctic inland plateau is less than 3 m s−1, mainly due to the gentler surface slopes of the inland plateau (Van den Broeke and Van Lipzig, 2003). The maximum wind speed (exceeding 60 m s−1) is observed at Alessandra, Eneide, Lanyon, Lola, Lucia, Minna Bluff, Rita, Silvia, Sofia, Sofiab, Virginia and Zoraida stations in North Victoria Land. Spatial patterns of wind speed are generally high along the coast and low on the inland ice sheet, which is mainly determined by the terrain and pressure gradient from coastal to inland. Southerly or easterly winds prevail over most of the AIS, influenced by circumpolar westerly winds, katabatic winds, large-scale pressure gradient forces and topography, which contributes to driving the movement of the AIS atmospheric boundary layer (Van den Broeke et al., 2002). The winds over the AIS are persistent throughout most of the year, which is reflected in a high mean value of daily mean constancy of the wind direction (defined as the ratio of the magnitude of the mean wind vector to the scalar average wind speed) (≥0.6) for the majority of the AWSs (Fig. 6).
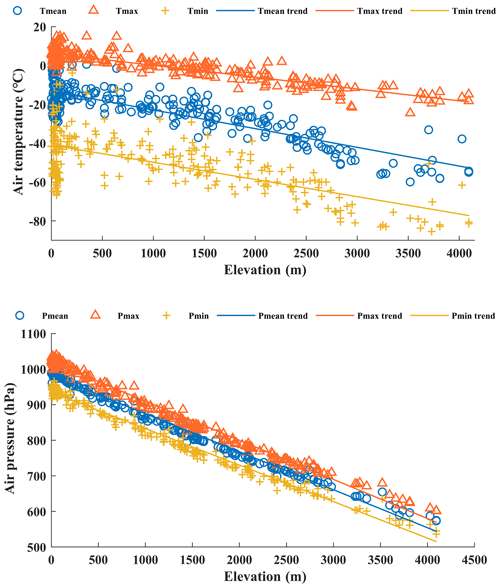
Figure 5Multiyear 3 h mean, maximum and minimum air temperature and pressure as a function of elevation.
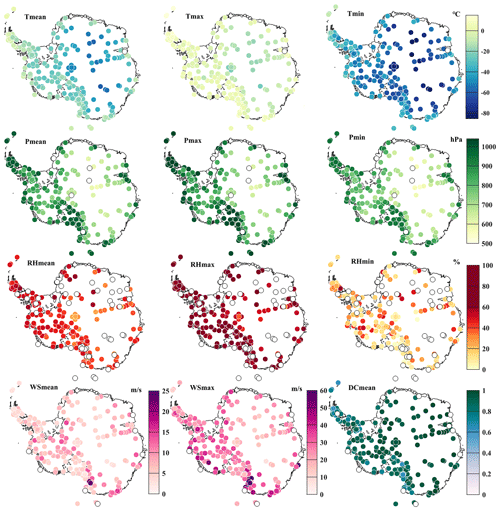
Figure 6Spatial distribution of AWS multiyear 3 h mean, maximum and minimum meteorological elements (temperature, pressure, relative humidity, wind speed) and daily mean constancy of the wind direction (DC) during 1980–2021. White circles represent the missing data. Tmean is mean temperature, Tmax is maximum temperature, Tmin is minimum temperature, Pmean is mean pressure, Pmax is maximum pressure, Pmin is minimum pressure, RHmean is mean relative humidity, RHmax is maximum relative humidity, RHmin is minimum relative humidity, WSmean is mean wind speed, WSmax is maximum wind speed, and DCmean is daily mean constancy of the wind direction.
5.1 Spatial coverage of AWS records
The spatial distribution of AWSs is heterogeneous over the AIS. On the whole, since 1980, the number and coverage of AWSs have been gradually increasing (see Figs. 7 and 8 and Table S5). In 1980, there were only nine AWSs, of which five were located in the vicinity of Ross Island, two along the coast of Adélie Land, and two in inland Antarctica (Byrd and Dome C). The number and spatial coverage of AWSs when their data are available peaked in 2014, with a total of 146 AWSs. Approximately 90 % of the AWSs were distributed in coastal areas and regions of lower elevation. Among them, the densest regions covered by AWSs are the Ross Ice Shelf and Victoria Land, accounting for approximately 50 % of AWSs in 2014. The gradually improved AWS network has helped fill the wide gaps in climate observations across the whole Antarctic continent.
Despite the significant improvement in the spatial coverage of AWSs, the data availability is still not evenly distributed but is clustered in specific areas of Antarctica (see Fig. 6 and Table S5). Air temperature and pressure are relatively easy to measure and have the highest data availability of any sensor, high integrity and wide spatial coverage. Additionally, the quality of air temperature data is the best, with only two stations missing air temperature records. Measuring wind speed and direction is a huge challenge in Antarctica, however, due to covering such a wide range of speeds from calm/breeze to sustained hurricane intensity. Another challenge is the freezing/breaking of wind sensors due to extreme environmental conditions (including due to snow/riming or high winds). The loss of wind speed and direction data mainly occurs in the coastal areas of the Lambert Glacier basin, Wilkes Land, Victoria Land, Mary Byrd Land and Ellsworth Land. The measurement accuracy of humidity sensors may be very unreliable under the very cold temperature conditions, and as a result, their data losses are the highest. In addition to the West AIS and near the South Pole, there are many AWSs that lack humidity measurements all year round in other parts of Antarctica.
5.2 Temporal variability in the AWS records
The five meteorological elements of each AWS cover different time spans, from 1 to 42 years. The time covered is closely related to sensor technology and weather conditions. Statistical results in Supplement Table S5 show that the time span of 63 AWSs exceeds 20 years, of which 27 stations exceed 30 years, but still approximately 24.3 % of the AWSs operated for less than 5 years. For various reasons, many time series in the AWS dataset have gaps for one or all of the meteorological variables (Fig. 9).
Figures 9 and S1–S4 provide details on the data availability of the daily air temperature, air pressure, wind speed and relative humidity, respectively, calculated by more than 25 % of the 3 h observations. Among the 267 AWSs, the air temperature measurement data have the best continuity and the highest data integrity. Approximately 30 % of the stations have more than 15 years of daily temperature measurement data. Furthermore, 237 stations have a daily data integrity exceeding 50 %. In recent years, the improvement in air pressure sensor technology has greatly enhanced the quality of air pressure measurement data. The integrity of daily pressure data of 225 meteorological stations exceeds 50 %, and approximately 28 % of stations have daily pressure data over a 15-year time span. The wind sensor is affected by temperature, and the resulting data have the poorest continuity. Only approximately 28 % of the stations have daily scalar wind speed and vector direction data for a duration of more than 15 years. There are 114 stations exhibiting a data integrity of more than 50 % for daily scalar wind speed and vector wind direction. For the 1980–2021 period, the lack of relative humidity data is the poorest-performing AWS record, with 46 stations having no relative humidity data all year round and only 167 stations having a daily data integrity of more than 50 %. Moreover, the data continuity is the lowest, with only 20 % of stations measuring daily relative humidity covering more than 15 years.
The entire dataset consists of four subdatasets, including three quality-controlled subdatasets and one flagged subdataset of suspicious data in raw data, which are all provided in spreadsheet form. In quality-controlled daily and monthly subdatasets, all “wt” columns are the proportion of observations entered into the average value of the day or month. Number “1” indicates integrated continuous data without missing data. In the flagged subdataset, “flag_*” marks the suspicious data of each variable detected in Sect. 3.2 quality control. Number “4” indicates that the observed value exceeds the 3 standard deviations from the mean. A multiple of 100 represents the physically unrealistic 6 h rapid synoptic variability in the parameters. The air temperature records in the austral summer months (December–January–February) during the low wind speed conditions (less than 2 m s−1) are flagged with number “10 000”. Time is in 3 h, daily and monthly formats, and UTC time is used in the 3 h data files (UTC + 8). At the same time, we also provide the data integrity of 3 h, daily and monthly data of each variable.
The raw data we collected from different Antarctic AWS projects include four different data storage formats: ASCII format (.dat), NetCDF format (.nc), TXT format (.txt) and Excel format (.xlsx). Five meteorological elements are extracted and saved in comma-separated value format (.csv). The .csv format is selected due to its simple file structure and storage mode, basic security, and extensive support in scientific applications, which is convenient for programming software (e.g., R) to process data in batches. The file names are composed using the station's name and data type. A file name such as AGO Site_3 h.csv can be read as the station AGO site, 3 h data, with the extension indicating .csv format data. The data are arranged in columns of year, month, day, 3-hourly observation time (UTC), temperature (∘), pressure (hPa), wind speed (m s−1), wind direction (∘) and relative humidity (%).
The comprehensive AWS dataset is freely available as 3 h, daily, and monthly data separated for each station at https://amrdcdata.ssec.wisc.edu/dataset/antaws-dataset (Wang et al., 2022). The DOI identifier is https://doi.org/10.48567/key7-ch19 (Wang et al., 2022). All codes for the AWS data quality control have been developed in the R environment and are available from the corresponding authors on request.
We provide a comprehensive compilation of long-term measurements of the Antarctic AWSs. The dataset includes the locations, specifications of used instrumentation, and measurements of five variables, i.e., air temperature, air pressure, relative humidity, wind speed and wind direction, of 267 AWSs at 3 h, daily and monthly resolutions, covering much of the Antarctic continent from 1980 to 2021. Relative to earlier studies, our compilation presents improved spatial coverage, although the spatial density is least over the East Antarctic Plateau.
We adopt a comprehensive quality control process to maximize the reliability of the data. This results in the reduction in the temporal data density for some of the AWSs. However, the statistical results of 267 AWSs from 1980 to 2021 show that the integrity of the 3 h air temperature and air pressure data records from 192 stations included here exceeds 50 %. Moreover, 159 stations have a 3 h relative humidity data integrity of more than 50 %, which is the variable with the lowest data integrity. There are 92 stations with an integrity of the 3 h wind measurement data of less than 50 %. This is easily understood as, among the five variables, wind speed and direction observations have the highest uncertainties, caused by excessive speed, snow buildup, and so on.
Our dataset may provide the currently most accurate and effective input and verification data for the validation of reanalyses, remote sensing products and regional climate models as well as crucial input to numerical weather prediction. At the same time, as demonstrated by Steig et al. (2009), by combining the dataset with reanalysis data or remote sensing products, gridded data products can be reconstructed which can better display the temporal and spatial variation in the AIS meteorological elements at different scales and provide basic data for the studies of Antarctic mass balance and climate changes. It is hoped that the dataset will facilitate glaciological, meteorological, hydrological, or other studies over Antarctica.
The AWS network in the Antarctic remains incomplete, and there is scope for improvement. In the near future, deployments of additional AWSs on the East Antarctic Plateau are a priority, especially in the summit region. However, it is highly challenging to install and maintain AWSs in the extreme environment of the East Antarctic Plateau. Moreover, ultrasonic sounders are systematically implemented to provide snow height data along with the meteorological data. Mechanically ventilated aspirated radiation shields should be considered to reduce radiation bias, especially in summer, when solar power is available. In addition, the relative humidity supersaturated observation systems under extreme cold conditions described by Genthon et al. (2017, 2022) can be widely applied. With the continuous improvement in the AWS network and updating of AWS data, we will further refine the dataset, adopt more rigorous quality control criteria, check the unrecognizable errors in the raw data, and even provide quality marks for the dataset.
The supplement related to this article is available online at: https://doi.org/10.5194/essd-15-411-2023-supplement.
YW conceived this work and constructed the AntAWS dataset. XZ prepared the figures and tables based on the compiled data analysis. WN wrote the codes of the data processing algorithm. MAL, MD, CHR, PCJPS, PG, PH and ERT provided parts of AWS observations for constructing the dataset. MAL and PG provided some necessary information on AWSs. ZZ and YS performed the primary data collections. SH supervised this work. XZ and YW wrote the original draft, with contributions by all the other authors.
The contact author has declared that none of the authors has any competing interests.
Publisher’s note: Copernicus Publications remains neutral with regard to jurisdictional claims in published maps and institutional affiliations.
The authors thank Christoph Kittel, Changqing Ke, Ian Allison, Christophe Genthon and David Carlson for their constructive comments and suggestions to improve the paper.
This work was provided by the National Natural Science Foundation of China (41971081, 41830644 and 42122047), the National Key Research and Development Program of China (2020YFA0608202), the Strategic Priority Research Program of the Chinese Academy of Sciences (XDA19070103), the Project for Outstanding Youth Innovation Team in the Universities of Shandong Province (2019KJH011) and the Basic Research Fund of the Chinese Academy of Meteorological Sciences (2021Y021 and 2021Z006). This work is also supported by funding to the University of Wisconsin-Madison and Madison Area Technical College from the US National Science Foundation Office of Polar Programs (1924730, 1951720, and 1951603) and by the Australian Antarctic Program under projects AAS187, 4007, 5032 and 4506. Petra Heil was supported by grant funding from the Australian government as part of the Antarctic Science Collaboration Initiative program (ASCI000002; Australian Antarctic Program Partnership) and the International Space Science Institute (Switzerland) Project 405.
This paper was edited by David Carlson and reviewed by Christoph Kittel and one anonymous referee.
Allison, I.: The surface climate of the interior of the Lambert Glacier basin: 5 years of automatic weather station data, Ann. Glaciol., 27, 515–520, https://https://doi.org/10.3189/1998AoG27-1-515-520, 1998.
Allison, I. and Morrissy, J. V.: Automatic weather stations in Antarctica, Aust. Meteorol. Mag., 31, 71–76, 1983.
Allison, I., Wendler, G., and Radok, U.: Climatology of the East Antarctic ice sheet (100∘ E to 140∘ E) derived from automatic weather stations, J. Geophys. Res.-Atmos., 98, 8815–8823, https://doi.org/10.1029/93JD00104, 1993.
Amory, C.: Drifting-snow statistics from multiple-year autonomous measurements in Adélie Land, East Antarctica, The Cryosphere, 14, 1713–1725, https://doi.org/10.5194/tc-14-1713-2020, 2020.
Aristidi, E., Agabi, K., Azouit, M., Azouit, M., Fossat, E., Vernin, J., Travouillon, T., Lawrence, J. S., Meyer, C., Storey, J. W. V., Halter, B., Roth W. L., and Walden, V.: An analysis of temperatures and wind speeds above Dome C, Antarctica, Astron. Astrophys., 430, 739–746, https://doi.org/10.1051/0004-6361:20041876, 2005.
Bromwich, D. H., Nicolas, J. P., Monaghan, A. J., Lazzara, M. A., Keller, L. M., Weidner, G. A., and Wilson, A. B.: Central West Antarctica among the most rapidly warming regions on Earth, Nat. Geosci., 6, 139–145, https://doi.org/10.1038/NGEO1671, 2013.
Bromwich, D. H., Nicolas, J. P., Monaghan, A. J., Lazzara, M. A., Keller, L. M., Weidner, G. A., and Wilson, A. B.: Correction: Corrigendum: Central West Antarctica among the most rapidly warming regions on Earth, Nat. Geosci., 7, 76–76, https://doi.org/10.1038/ngeo2016, 2014.
Convey, P., Coulson, S. J., Worland, M. R., and Sjöblom, A.: The importance of understanding annual and shorter-term temperature patterns and variation in the surface levels of polar soils for terrestrial biota, Polar Biol., 41, 1587–1605, https://doi.org/10.1007/s00300-018-2299-0, 2018.
Ding, M., Zou, X., Sun, Q., Yang, D., Zhang, W., Bian, L., Lu, C., Allison, I., Heil, P., and Xiao, C.: The PANDA automatic weather station network between the coast and Dome A, East Antarctica, Earth Syst. Sci. Data, 14, 5019–5035, https://doi.org/10.5194/essd-14-5019-2022, 2022.
Donat-Magnin, M., Jourdain, N. C., Gallée, H., Amory, C., Kittel, C., Fettweis, X., Wille, J. D., Favier, V., Drira, A., and Agosta, C.: Interannual variability of summer surface mass balance and surface melting in the Amundsen sector, West Antarctica, The Cryosphere, 14, 229–249, https://doi.org/10.5194/tc-14-229-2020, 2020.
Dong, X., Wang, Y., Hou, S., Ding, M., Yin, B., and Zhang, Y.: Robustness of the recent global atmospheric reanalyses for Antarctic near-surface wind speed climatology, J. Climate, 33, 4027–4043, https://doi.org/10.1175/JCLI-D-19-0648.1, 2020.
Gallée, H. and Gorodetskaya, I. V.: Validation of a limited area model over Dome C, Antarctic Plateau, during winter, Clim. Dynam., 34, 61–72, https://doi.org/10.1007/s00382-008-0499-y, 2010.
Genthon, C., Six, D., Favier, V., Lazzara, M., and Keller, L.: Atmospheric temperature measurement biases on the Antarctic plateau, J. Atmos. Ocean. Tech., 28, 1598–1605, https://doi.org/10.1175/JTECH-D-11-00095.1, 2011.
Genthon, C., Six, D., Gallée, H., Grigioni, P., and Pellegrini, A.: Two years of atmospheric boundary layer observations on a 45-m tower at Dome C on the Antarctic plateau, J. Geophys. Res.-Atmos., 118, 3218–3232, https://doi.org/10.1002/jgrd.50128, 2013.
Genthon, C., Piard, L., Vignon, E., Madeleine, J.-B., Casado, M., and Gallée, H.: Atmospheric moisture supersaturation in the near-surface atmosphere at Dome C, Antarctic Plateau, Atmos. Chem. Phys., 17, 691–704, https://doi.org/10.5194/acp-17-691-2017, 2017.
Genthon, C., Veron, D., Vignon, E., Six, D., Dufresne, J.-L., Madeleine, J.-B., Sultan, E., and Forget, F.: 10 years of temperature and wind observation on a 45 m tower at Dome C, East Antarctic plateau, Earth Syst. Sci. Data, 13, 5731–5746, https://doi.org/10.5194/essd-13-5731-2021, 2021.
Genthon, C., Veron, D. E., Vignon, E., Madeleine, J.-B., and Piard, L.: Water vapor in cold and clean atmosphere: a 3-year data set in the boundary layer of Dome C, East Antarctic Plateau, Earth Syst. Sci. Data, 14, 1571–1580, https://doi.org/10.5194/essd-14-1571-2022, 2022.
Goff, J. A. and Gratch, S.: Thermodynamic properties of moist air, Trans. ASHVE, 51, 179–199, 1945.
Gregory, J. M. and Huybrechts, P.: Ice-sheet contributions to future sea-level change, Philos. T. Roy. Soc. A, 364, 1709–1732, https://doi.org/10.1098/rsta.2006.1796, 2006.
Giovinetto, M. B., Waters, N. M., and Bentley, C. R.: Dependence of Antarctic surface mass balance on temperature, elevation, and distance to open ocean, J. Geophys. Res.-Atmos., 95, 3517–3531, https://doi.org/10.1029/JD095iD04p03517, 1990.
Hersbach, H., Bell, B., Berrisford, P., Hirahara, S., Horányi, A., Muñoz-Sabater, J., Nicolas, J., Peubey, C., Radu, R., Schepers, D., Simmons, A., Soci, C., Abdalla, S., Abellan, X., Balsamo, G., Bechtold, P., Biavati, G., Bidlot, J., Bonavita, M., Chiara, G. D., Dahlgren, P., Dee, D., Diamantakis, M., Dragani, R., Flemming, J., Forbes, R., Fuentes, M., Geer, A., Haimberger, L., Healy, S., Hogan, R. J., Hólm, E., Janisková, M., Keeley, S., Laloyaux, P., Lopez, P., Lupu, C., Radnoti, G., Rosnay, P. d., Rozum, I., Vamborg, F., Villaume, S., and Thépaut, J-N.: The ERA5 global reanalysis, Q. J. Roy. Meteor. Soc., 146, 1999–2049, https://doi.org/10.1002/qj.38, 2020.
Herbei, R., Rytel, A. L., Lyons, W. B., McKnight, D. M., Jaros, C., Gooseff, M. N., and Priscu, J. C.: Hydrological Controls on Ecosystem Dynamics in Lake Fryxell, Antarctica, PloS one, 11, e01590382016, https://doi.org/10.1371/journal.pone.0159038, 2016.
Huai, B., Wang, Y., Ding, M., Zhang, J., and Dong, X.: An assessment of recent global atmospheric reanalyses for Antarctic near surface air temperature, Atmos. Res., 226, 181–191, https://doi.org/10.1016/j.atmosres.2019.04.029, 2019.
IPCC: IPCC Special Report on the Ocean and Cryosphere in a Changing Climate, edited by: Pörtner, H.-O., Roberts, D. C., Masson-Delmotte, V., Zhai, P., Tignor, M., Poloczanska, E., Mintenbeck, K., Alegría, A.,, Nicolai, Okem, A., Petzold, J., Rama, B., Weyer, N. M., Cambridge University Press, Cambridge, UK and New York, NY, USA, 755 pp., https://doi.org/10.1017/9781009157964, 2019.
IPCC: Climate Change 2021: The Physical Science Basis. Contribution of Working Group I to the Sixth Assessment Report of the Intergovernmental Panel on Climate Change, edited by: Masson-Delmotte, V., Zhai, P., Pirani, A., Connors, S. L., Péan, C., Berger, S., Caud, N., Chen, Y., Goldfarb, L., Gomis, M. I., Huang, M., Leitzell, K., Lonnoy, E., Matthews, J. B. R., Maycock, T. K., Waterfield, T., Yelekçi, O., Yu, R., and Zhou, B., Cambridge University Press, Cambridge, United Kingdom and New York, NY, USA, in press, https://doi.org/10.1017/9781009157896, 2021.
Jacka, T. H., Christou, L., and Cook, B. J.: A data bank of mean monthly and annual surface temperatures for Antarctica, the Southern Ocean and South Pacific Ocean. Australian National Antarctic Research Expeditions Research, 22, 98 pp., ISSN 0729-6533, 1984.
Jakobs, C. L., Reijmer, C. H., Smeets, P. C. J. P., Trusel, L. D., van de Berg, W. J., van den Broeke, M. R., and van Wessem, J. M.: A benchmark dataset of in situ Antarctic surface melt rates and energy balance, J. Glaciol., 66, 291–302, https://doi.org/10.1017/jog.2020.6, 2020.
Jones, P. D., and Limbert, D W. S.: A data bank of Antarctic surface temperature and pressure data, East Anglia Univ. (UK), Climatic Research Unit; British Antarctic Survey, Cambridge, DOE/ER/60397-H2, 52 pp., 6413951, 1987.
Jones, R., Renfrew, I., Orr, A., Webber, B., Holland, D., and Lazzara, M.: Evaluation of four global reanalysis products using in situ observations in the Amundsen Sea Embayment, Antarctica, J. Geophys. Res.-Atmos., 121, 6240–6257, https://doi.org/10.1002/2015JD024680, 2016.
Kennicutt II, M. C., Bromwich, D., Liggett, D., Njåstad, B., Peck, L., Rintoul, S. R., Ritz, C., Siegert, M. J., Aitken, A., Brooks, C. M., Cassano, J., Chaturvedi, S., Chen, D., Dodds, K., Golledge, N. R., Bohec, C. L., Leppe, M., Murray, A., Nath, P. C., Raphael, M. N., Rogan-Finnemore, M., Schroeder, D. M., Talley, L., Travouillon, T., Vaughan, D. G., Wang, L., Weatherwax, A. T., Yang, H., and Chown, S. L.: Sustained Antarctic research: a 21st century imperative, One Earth, 1, 95–113, https://doi.org/10.1016/j.oneear.2019.08.014, 2019.
Kittel, C.: Present and future sensitivity of the Antarctic surface mass balance to oceanic and atmospheric forcings: insights with the regional climate model MAR, PhD thesis, University of Liège, Liège, http://hdl.handle.net/2268/258491 (last access: 28 May 2022), 2021.
Kittel, C., Amory, C., Agosta, C., Jourdain, N. C., Hofer, S., Delhasse, A., Doutreloup, S., Huot, P.-V., Lang, C., Fichefet, T., and Fettweis, X.: Diverging future surface mass balance between the Antarctic ice shelves and grounded ice sheet, The Cryosphere, 15, 1215–1236, https://doi.org/10.5194/tc-15-1215-2021, 2021.
Knuth, S. L., Tripoli, G. J., Thom, J. E., Weidner, G. A., The influence of blowing snow and precipitation on snow depth change across the Ross Ice Shelf and Ross Sea regions of Antarctica, J. Appl. Meteorol. Clim., 49, 1306–1321, https://doi.org/10.1175/2010JAMC2245.1, 2010.
Lazzara, M. A., Weidner, G. A., Keller, L. M., Thom, J. E., and Cassano, J. J.: Antarctic automatic weather station program: 30 years of polar observation, B. Am. Meteorol. Soc., 93, 1519–1537, https://doi.org/10.1175/BAMS-D-11-00015.1, 2012.
Lazzara, M. A., Welhouse, L. J., Thom, J. E., Cassano, J. J., DuVivier, A. K., Weidner, G. A., Keller, L. M., and Kalnajs, L.: Automatic Weather Station (AWS) Program operated by the University of Wisconsin-Madison during the 2011–2012 field season, Antarctic Record, 57, 125–135, https://doi.org/10.15094/00009683, 2013.
Ma, Y. and Bian, L.: A Surface Climatological Validation of ERA-interim Reanalysis and NCEP FNL Analysis over East Antarctic, Chinese Journal of Polar Research, 26, 469–480, https://doi.org/10.13679/j.jdyj.2014.4.469, 2014.
Ma, Y., Bian, L., Xiao, C., and Allison, I.: Correction of snow accumulation impacted on air temperature from automatic weather station on the Antarctic Ice Sheet, Advance in Polar Science, 20, 299–309, 2008.
Martin, P. and Peel, D.: The Spatial Distribution of 10 m Temperatures in the Antarctic Peninsula, J. Glaciol., 20, 311–317, https://doi.org/10.3189/S0022143000013861, 1978.
Mottram, R., Hansen, N., Kittel, C., van Wessem, J. M., Agosta, C., Amory, C., Boberg, F., van de Berg, W. J., Fettweis, X., Gossart, A., van Lipzig, N. P. M., van Meijgaard, E., Orr, A., Phillips, T., Webster, S., Simonsen, S. B., and Souverijns, N.: What is the surface mass balance of Antarctica? An intercomparison of regional climate model estimates, The Cryosphere, 15, 3751–3784, https://doi.org/10.5194/tc-15-3751-2021, 2021.
Reijmer, C. H. and Oerlemans, J.: Temporal and spatial variability of the surface energy balance in Dronning Maud Land, East Antarctica, J. Geophys. Res.-Atmos., 107, 4759, https://doi.org/10.1029/2000JD000110, 2002.
Renfrew, I. A. and Anderson, P. S.: The surface climatology of an ordinary katabatic wind regime in Coats Land, Antarctica, Tellus A, 54, 463–484, https://doi.org/10.3402/tellusa.v54i5.12162, 2002.
Reusch, D. B. and Alley, R. B.: A 15-year West Antarctic climatology from six automatic weather station temperature and pressure records, J. Geophys. Res.-Atmos., 109, D04103, https://doi.org/10.1029/2003JD004178, 2004.
Rodrigo, J. S., Buchlin, J-M., van Beeck J., Lenaerts, J. T. M., and van den Broeke, M. R.: Evaluation of the antarctic surface wind climate from ERA reanalyses and RACMO2/ANT simulations based on automatic weather stations, Clim. Dynam., 40, 353–376, https://doi.org/10.1007/s00382-012-1396-y, 2013.
Rignot, E., Mouginot, J., Scheuchl, B., and Morlighem, M.: Four decades of Antarctic Ice Sheet mass balance from 1979–2017, P. Natl. Aacad. Sci. USA, 116, 1095–1103, https://doi.org/10.1073/pnas.1812883116, 2019.
Seefeldt, M. W., Cassano, J. J., and Parish, T. R.: Dominant regimes of the Ross Ice Shelf surface wind field during austral autumn 2005, J. Appl. Meteorol. Clim., 46, 1933–1955, https://doi.org/10.1175/2007JAMC1442.1, 2007.
Shuman, C. A. and Stearns, C. R.: Decadal-length composite inland West Antarctic temperature records, J. Climate, 14, 1977–1988, https://doi.org/10.1175/1520-0442(2001)014<1977:DLCIWA>2.0.CO;2, 2001.
Smeets, P. C., Kuipers Munneke, P., Van As, D., van den Broeke, M. R., Boot, W., Oerlemans, H., Snellen, H., Reijmer, C. H., and van de Wal, R. S.: The K-transect in west Greenland: Automatic weather station data (1993–2016), Arct. Antarct. Alp. Res., 50, S100002, https://doi.org/10.1080/15230430.2017.1420954, 2018.
Stearns, C. R. and Wendler, G.: Research results from Antarctic automatic weather stations, Rev. Geophys., 26, 45–61, https://doi.org/10.1029/RG026i001p00045, 1988.
Stearns, C. R., Keller, L. M., Weidner, G. A., and Sievers, M.: Monthly mean climatic data for Antarctic automatic weather stations, Antarctic meteorology and climatology: studies based on automatic weather stations, American Geophysical Union, 61, 1–21, https://doi.org/10.1029/AR061p0001, 1993.
Steig, E. J., Schneider, D. P., Rutherford, S. D., Mann, M. E., Comiso, J. C., and Shindell, D. T.: Warming of the Antarctic ice-sheet surface since the 1957 International Geophysical Year, Nature, 457, 459–462, https://doi.org/10.1038/nature07669, 2009.
Summerhayes, C. P.: International collaboration in Antarctica: The International Polar Years, the International Geophysical Year, and the Scientific Committee on Antarctic Research, Polar Rec., 44, 321–334, https://doi.org/10.1017/S0032247408007468, 2008.
Tastula, E. M., Vihma, T., and Andreas, E. L.: Evaluation of Polar WRF from Modeling the Atmospheric Boundary Layer over Antarctic Sea Ice in Autumn and Winter, Mon. Weather Rev., 140, 3919–3935, https://doi.org/10.1175/MWR-D-12-00016.1, 2012.
Turner, J., Colwell, S. R., Marshall, G. J., Lachlan-Cope, T. A., Carleton, A. M., Jones, P. D., Lagun, V., Reid, P. A., and Iagovkina, S.: The SCAR READER project: Toward a high-quality database of mean Antarctic meteorological observations, J. Climate, 17, 2890–2898, https://doi.org/10.1175/1520-0442(2004)017<2890:TSRPTA>2.0.CO;2, 2004.
Van den Broeke, M. R. and Van Lipzig, N. P. M.: Factors controlling the near-surface wind field in Antarctica, Mon. Weather Rev., 131, 733–743, https://doi.org/10.1175/1520-0493(2003)131<0733:FCTNSW>2.0.CO;2, 2003.
Van den Broeke, M. R., Van Lipzig, N. P. M., and Van Meijgaard, E.: Momentum budget of the East Antarctic atmospheric boundary layer: Results of a regional climate model, J. Atmos. Sc., 59, 3117–3129, https://doi.org/10.1175/1520-0469(2002)059<3117:MBOTEA>2.0.CO;2, 2002.
van Wessem, J. M., Reijmer, C. H., Lenaerts, J. T. M., van de Berg, W. J., van den Broeke, M. R., and van Meijgaard, E.: Updated cloud physics in a regional atmospheric climate model improves the modelled surface energy balance of Antarctica, The Cryosphere, 8, 125–135, https://doi.org/10.5194/tc-8-125-2014, 2014.
Wang, Y., Wang, M., and Zhao, J.: A comparison of MODIS LST retrievals with in situ observations from AWS over the Lambert Glacier Basin, East Antarctica, Int. J. Geosci., 4, 611–617, https://doi.org/10.4236/ijg.2013.43056, 2013.
Wang, Y., Ding, M., Reijmer, C. H., Smeets, P. C. J. P., Hou, S., and Xiao, C.: The AntSMB dataset: a comprehensive compilation of surface mass balance field observations over the Antarctic Ice Sheet, Earth Syst. Sci. Data, 13, 3057–3074, https://doi.org/10.5194/essd-13-3057-2021, 2021.
Wang, Y., Zhang, X., Ning, W., Lazzara, M. A., Ding, M., Reijmer C., Smeets P., Grigioni, P., Thomas, E. R., Zhai Z., Sun Y., and Hou, S.: AntAWS Dataset: A compilation of Antarctic automatic weather station observations, Version 1.0, AMRDC Data Repository [data set], https://doi.org/10.48567/key7-ch19, 2022.
Wille, J. D., Favier, V., Jourdain, N. C., Kittel, C., Turton, J. V., Agosta, C., Gorodetskaya, I. V., Picard, G., Codron, F., Santos, C. L-D., Amory, C., Fettweis, X., Blanchet, J., Vincent Jomelli, V., and Berchet, A.: Intense atmospheric rivers can weaken ice shelf stability at the Antarctic Peninsula, Commun. Earth Environ., 3, 90, https://doi.org/10.1038/s43247-022-00422-9, 2022.
World Meteorological Organization: Guide to Instruments and Methods of Observation Volume 1–Measurement of Meteorological Variables, Geneva, Switzerland, 8, ISBN 978-92-63-10008-5, 2018.
- Abstract
- Introduction
- Automatic weather station system
- Data processing
- Description of the AntAWS dataset
- Spatiotemporal characteristics of the AntAWS dataset
- Station documentation
- Code and data availability
- Conclusions
- Author contributions
- Competing interests
- Disclaimer
- Acknowledgements
- Financial support
- Review statement
- References
- Supplement
- Abstract
- Introduction
- Automatic weather station system
- Data processing
- Description of the AntAWS dataset
- Spatiotemporal characteristics of the AntAWS dataset
- Station documentation
- Code and data availability
- Conclusions
- Author contributions
- Competing interests
- Disclaimer
- Acknowledgements
- Financial support
- Review statement
- References
- Supplement