the Creative Commons Attribution 4.0 License.
the Creative Commons Attribution 4.0 License.
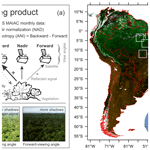
AnisoVeg: anisotropy and nadir-normalized MODIS multi-angle implementation atmospheric correction (MAIAC) datasets for satellite vegetation studies in South America
Lênio Soares Galvão
Fabien Hubert Wagner
Yhasmin Mendes de Moura
Nathan Gonçalves
Yujie Wang
Alexei Lyapustin
Yan Yang
Sassan Saatchi
Luiz Eduardo Oliveira Cruz Aragão
The AnisoVeg product consists of monthly 1 km composites of anisotropy (ANI) and nadir-normalized (NAD) surface reflectance layers obtained from the Moderate Resolution Imaging Spectroradiometer (MODIS) sensor over the entire South American continent. The satellite data were preprocessed using the multi-angle implementation atmospheric correction (MAIAC). The AnisoVeg product spans 22 years of observations (2000 to 2021) and includes the reflectance of MODIS bands 1 to 8 and two vegetation indices (VIs), namely the normalized difference vegetation index (NDVI) and enhanced vegetation index (EVI). While the NAD layers reduce the data variability added by bidirectional effects on the reflectance and VI time series, the unique ANI layers allow the use of this multi-angular data variability as a source of information for vegetation studies. The AnisoVeg product has been generated using daily MODIS MAIAC data from both Terra and Aqua satellites, normalized for a fixed solar zenith angle (SZA = 45∘), modeled for three sensor view directions (nadir, forward, and backward scattering), and aggregated to monthly composites. The anisotropy was calculated by the subtraction of modeled backward and forward scattering surface reflectance. The release of the ANI data for open usage is novel, and the NAD data are at an advanced processing level. We demonstrate the use of such data for vegetation studies using three types of forests in the eastern Amazon with distinct gradients of vegetation structure and aboveground biomass (AGB). The gradient of AGB was positively associated with ANI, while NAD values were related to different canopy structural characteristics. This was further illustrated by the strong and significant relationship between EVIANI and forest height observations from the Global Ecosystem Dynamics Investigation (GEDI) lidar sensor considering a simple linear model (R2=0.55). Overall, the time series of the AnisoVeg product (NAD and ANI) provide distinct information for various applications aiming at understanding vegetation structure, dynamics, and disturbance patterns. All data, processing codes, and results are made publicly available to enable research and the extension of AnisoVeg products for other regions outside of South America. The code can be found at https://doi.org/10.5281/zenodo.6561351 (Dalagnol and Wagner, 2022), EVIANI and EVINAD can be found as assets in the Google Earth Engine (GEE; described in the data availability section), and the full dataset is available from the open repository https://doi.org/10.5281/zenodo.3878879 (Dalagnol et al., 2022).
- Article
(6684 KB) - Full-text XML
- BibTeX
- EndNote
Anisotropy is defined as the departure from Lambertian scattering (isotropic), which is caused by the physical structure of media through which photons pass. Because most land covers are not Lambertian (isotropic), the surface reflectance measured by satellite sensors varies with the view zenith angle (VZA), view direction (backward or forward scattering), and solar zenith angle (SZA; Galvão et al., 2011). This is especially valid for images acquired over vegetated surfaces by large field-of-view (FOV) instruments such as the Moderate Resolution Imaging Spectroradiometer (MODIS; Bhandari et al., 2011). MODIS has a wide swath scanning ±55∘ from nadir on board the Terra and Aqua satellites. For example, a reflected signal coming from the backward-scattering direction of MODIS under a large VZA and close-to-zero relative azimuth angle (RAA) between the satellite and Sun (Sun behind the platform) is generally higher than that coming from the nadir (VZA = 0∘) or forward-scattering direction (platform facing the Sun at RAA = 180∘). Moreover, the SZA also varies seasonally and across geographical locations, affecting the number of shadows in the surfaces observed by satellites (Galvão et al., 2013). Such view illumination effects are dependent on the land cover types, and their magnitude relates to differences in biophysical properties of the vegetation (Galvão et al., 2004; Sims et al., 2011). Therefore, the vegetation anisotropy can be seen antagonistically as sources of noise and biophysical information in the time series analysis of vegetation indices (VIs) calculated from MODIS. As a source of noise, one may consider that the reflected signal toward the large FOV satellite sensors varies with distinct view illumination geometries of data acquisition over the same surface. As a source of information, one may highlight that the anisotropy is land-cover-type dependent, showing spectral variations that may be associated, for instance, with changes in vegetation structure across different forests.
To reduce the bidirectional effects as a source of noise, a nadir-normalized dataset can be created. We can normalize the surface reflectance of the MODIS bands to a specific set of VZA and SZA, using the bidirectional reflectance distribution function (BRDF) represented by a model such as the Ross–Thick Li–Sparse (RTLS; Wanner et al., 1995). To ensure confidence in the data analysis, we can also use the multi-angle implementation atmospheric correction (MAIAC) for atmospheric correction. MAIAC is a new generation of cloud screening and atmospheric correction algorithm that uses an adaptive time series analysis and processing of groups of pixels to derive atmospheric aerosol concentration, cloud mask, and surface reflectance without typical empirical assumptions (Lyapustin et al., 2011, 2012). By mitigating atmospheric interference and advancing the accuracy of surface reflectance over tropical vegetation by a factor of 3 to 10, MAIAC offers substantial improvement over conventional products such as the MOD09 (Hilker et al., 2012). Because of the better data quality retrieval, MAIAC is also an alternative to the MCD43A4 16 d Nadir Bidirectional Reflectance Distribution Function (BRDF)-Adjusted Reflectance (NBAR) product due to the less variable seasonal signal (3 to 10 times) over evergreen forests resultant from reduced effects of sun view geometry. While the MCD43A4 NBAR product offers view illumination correction, by using the MAIAC products, one can also correct for solar illumination effects at the same time. Due to the improvements in cloud detection, aerosol retrieval, and atmospheric correction, the MAIAC algorithm provides from 4 % to 25 % more high-quality retrievals than the traditional MOD09 product, with the largest estimate being observed for tropical regions (Lyapustin et al., 2021). Studies have used MODIS MAIAC observations with nadir-normalized geometry to assess the Amazon forest structure, functioning, and impacts of environmental and climate change (Hilker et al., 2014; Wagner et al., 2017; Anderson et al., 2018; Dalagnol et al., 2018; Fonseca et al., 2019; Bontempo et al., 2020; Gonçalves et al., 2020; Zhang et al., 2021). For instance, such products have provided a reliable time series of surface reflectance data that allowed the identification of large-scale communities of bamboo species and their dynamics in the southwestern Amazon (Dalagnol et al., 2018). Last, by improving the cloud screening and minimizing BRDF artifacts in comparison to uncorrected data, the MAIAC greatly contributed to the understanding of the long-standing debate in the Amazon over the possible existence of the green-up phenomenon observed during the dry season of each year or with severe droughts (Morton et al., 2014; Bi et al., 2015; Saleska et al., 2016; Wu et al., 2018). The existence of this phenomenon has implications on the comprehension of the resilience of tropical forests to climate change.
To use the bidirectional effects as a source of information, we generate an anisotropy dataset that is dependent on land cover types and captures the variations in sunlit and shaded canopy components viewed by the sensors (Chen et al., 2003; Gao et al., 2003). The use of multi-angular information to obtain the metrics of anisotropy and extract information on forest structure was suggested 2 decades ago (Gobron et al., 2002; Diner et al., 2005). One of the early experiments exploring the use of anisotropy to extract information about vegetation structure was conducted by calculating the ratio between backward- and forward-scattering data and generating the anisotropy index (ANIX) for studying short-stature grass-type vegetation (Sandmeier et al., 1998). Other indices have been developed and validated afterwards (Schaaf et al., 2002; Lacaze et al., 2002; Chen et al., 2005; Pocewicz et al., 2007; de Moura et al., 2015; Sharma, 2021). However, this remains an understudied topic, with limited results reported in the literature, especially in tropical regions. For instance, observations from the Multi-angle Imaging SpectroRadiometer (MISR)/Terra in the backward- and forward-scattering directions facilitated the discrimination of savanna physiognomies in Brazil (Liesenberg et al., 2007). MODIS MAIAC data from both directions were also used to calculate an anisotropic VI that explained part of the large-scale photosynthetic activity in the Amazon, where higher photosynthetic activity was associated with higher anisotropy values (de Sousa et al., 2017). De Moura et al. (2015) employed a more sophisticated approach based on scattering at backward and forward view directions using multi-temporal and multi-angular observations of MAIAC MODIS and BRDF modeling. The resultant metrics of anisotropy were further validated against field and airborne light detection and ranging (lidar) observations, showing strong linear relationship with leaf area index (LAI; R2=0.70–0.88), canopy heterogeneity (R2=0.54), and photosynthetic activity (R2=0.73–0.98; de Moura et al., 2015, 2016; Hilker et al., 2017). Although showing great potential in vegetation studies, the aforementioned anisotropy metrics were never computed over larger areas of the world as is proposed in this study for South America.
The objective of this work is to present the AnisoVeg product, and how it can be used for vegetation studies. We use MODIS Collection 6 (C6) MAIAC (Lyapustin et al., 2018) monthly data (2000–2021) generated at 1 km spatial resolution for the entire South American continent, with two different types of layers, i.e., (1) nadir-normalized (NAD) data, for the surface reflectance of MODIS bands 1 to 8 and two VIs (normalized difference vegetation index, NDVI, and enhanced vegetation index, EVI), and (2) anisotropy data (ANI), calculated from the difference between backward- and forwarding-scattering estimates of bands 1 to 8 and VIs (de Moura et al., 2015). The motivations for generating this product extend from developing applications of multi-angle observations for vegetation studies to producing analysis-ready and openly available datasets of anisotropy and nadir metrics for a larger community of users. The paper is organized in several sections to present the processing steps for generating the AnisoVeg products, a brief evaluation of data products over experimental areas, and, finally, an example of its potential application in vegetation studies.
2.1 Daily MODIS MAIAC surface reflectance data over South America
Daily surface reflectance data were obtained from the MODIS product MCD19A1 v006 (collection 6) for the tiles covering South America (Fig. 1). According to the MODIS traditional tiling system, these tiles ranged from 9–14 (horizontal) and 7–14 (vertical). The input data consisted of cross-calibrated surface reflectance from Terra and Aqua satellites on eight spectral bands (Table 1), with 1 km spatial resolution from 2000 to 2021 (Lyapustin and Wang, 2018; https://doi.org/10.5067/MODIS/MCD19A1.006). This product provides surface reflectance data corrected for atmospheric effects by the MAIAC algorithm and is controlled for cloud-free and clear-to-moderately-turbid conditions with aerosol optical depth (AOD) at 0.47 µm below 1.5 (Lyapustin et al., 2018). The MAIAC algorithm uses a time series approach for improved cloud filtering, amongst other filters such as surface reflectance change, in order to provide the most accurate surface reflectance estimates. The raw data were obtained from NASA's Level-1 and Atmosphere Archive and Distribution System (LAADS) Distributed Active Archive Center (DAAC; available at https://ladsweb.modaps.eosdis.nasa.gov/archive/allData/6/MCD19A1/, last access: 16 January 2023).
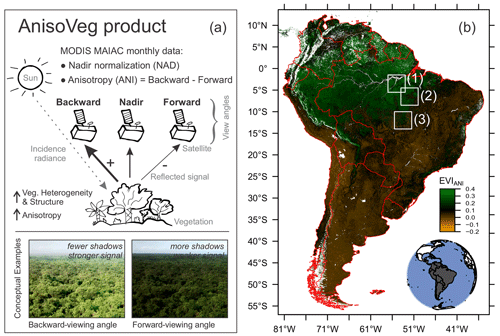
Figure 1AnisoVeg product concept and the area of coverage. (a) Schematic representation showing the observational geometry and the processing steps for producing NAD and ANI data from MODIS and to provide information on vegetation heterogeneity and structure. (b) The visualization of the anisotropy EVI (EVIANI) for South America from August 2021 at 1 km spatial resolution, showing the coverage of the product in South America and the location of three sites used to demonstrate potential applications. The sites are (1) Tapajós National Forest, (2) São Félix do Xingu, and (3) Xingu Indigenous Park. Red lines indicate the country boundaries.
2.2 The AnisoVeg product
The AnisoVeg product consists of two main types of data, spanning from 2000 to 2021, in monthly composites at 1 km spatial resolution, with (a) the nadir-normalized (NAD) data and (b) the anisotropy (ANI) data. Each data type has 10 layers, corresponding to the MODIS bands 1 to 8, and two VIs (NDVI and EVI). Additionally, the product provides auxiliary layers of backward scattering and forward scattering, including part of the bands (description in Sect. 5).
2.2.1 The nadir-normalized (NAD) data
In order to minimize the differences in sun sensor geometry between the MODIS scenes and generate the NAD dataset, the daily surface reflectance data were normalized to a fixed 45∘ SZA and to the nadir observation (VZA = 0∘) using the BRDF and the RTLS model (Wanner et al., 1995). The parameters of the RTLS BRDF model are part of the MAIAC product suite (MCD19A3 product) reported every 8 d. The MAIAC algorithm detects significant land cover changes (e.g., fire and deforestation) within the 8 d period and does not use those observations for the BRDF inversion (Lyapustin et al., 2018). A minimum of three observations in the 8 d window was required to accurately model the signal. The closest RTLS parameters in time were used to normalize the daily data. The normalized bidirectional reflectance factor (BRFn) for the NAD surface reflectance (SZA = 45∘, VZA = 0∘, and RAA = 0∘) was calculated using Eq. (1) as follows (Lyapustin et al., 2018):
where kL, kV, and kG are the BRDF isotropic, volumetric, and geometric optical kernel weights, respectively. F0V and F0G are the BRDF kernel values for the given geometry listed in Table 2. FV and FG are the kernel values of the RTLS model for the specific MODIS observation, respectively (Lyapustin et al., 2018). FV and FG values are available at 5 km cells and were resampled to 1 km using the nearest-neighbors method to match the spatial resolution of the spectral bands. This resampling step does not create spatial artifacts in the data because the geometry changes slowly over time (Lyapustin et al., 2018).
We aggregated the normalized daily data into monthly composites by keeping the median values for each pixel. During the temporal aggregation, we also calculated the per-pixel number of samples (or observations) for each monthly composite, which can be used as auxiliary data to filter pixels with a low number of observations (less reliable estimates of surface reflectance). The tiles were mosaicked for the entire South American continent and then re-projected from the original sinusoidal projection to the geographic coordinates system (datum WGS 84; EPSG: 4326). The output spatial resolution corresponded to 0.0091∘, which is approximately equivalent to 1 km in projected coordinates.
We also calculated two traditional vegetation indices, namely NDVI (Rouse et al., 1974; Eq. 2) and EVI (Huete et al., 2002; Eq. 3).
where ρ is the surface reflectance of a MODIS band, ρNIR is the NIR reflectance (band 2), ρRed is the red reflectance (band 1), and ρBlue is the blue reflectance (band 3). The constants in Eq. (3) (bands 6, 7.5, 1, and 2.5) represent the aerosol coefficient adjustment of the atmosphere for the red and blue bands, the adjustment factor for the soil, and the gain factor, respectively (Huete et al., 2002).
2.2.2 The anisotropy (ANI) data
For the ANI data, the daily surface reflectance data were first normalized to two viewing angles at the backward (SZA = 45∘, VZA = 35∘, RAA = 180∘) and forward (SZA = 45∘, VZA = 35∘, RAA = 0∘) scattering, using Eq. (1) and values from Table 2. The VZA was set to the near-hot spot (VZA = 35∘) instead of the actual hot spot (VZA = 45∘) to keep VZA closer to the actual range of MODIS observations across the South American continent and minimize errors coming from the extrapolation of the BRDF (de Moura et al., 2015). The standard deviation for this modeling was thoroughly investigated in a previous study and determined to be 10 % of the observed variation in anisotropy (de Moura et al., 2015). Furthermore, we aggregated the backward- and forward-scattering data temporally into monthly composites following the same procedures as before for the NAD data. We then calculated the NDVI and EVI for each viewing angle normalization. Finally, we obtained the difference between backward- and forward-scattering estimates for each of the eight MODIS bands, in addition to the NDVI and EVI, effectively generating the ANI layers as follows (Eq. 4; de Moura et al., 2015):
where i is the spectral band or VI selected in the calculation.
2.3 Algorithm and computation
All data processing was done in R v4.0.2 (R Core Team, 2016), and the code is available from GitHub (https://github.com/ricds/maiac_processing, last access: 16 January 2023; Dalagnol and Wagner, 2022). Besides processing the AnisoVeg product from the daily MAIAC MODIS data, the code can also generate 16 or 8 d temporal composites, mosaics, and VIs. Although we focused on South America when developing AnisoVeg, the code can readily be adapted to process data for other parts of the world and generate corresponding NAD and ANI layers. Below, we provide the computer specification for anyone who wishes to process the data independently.
For the presented dataset, the computation was performed using a HP Z840 workstation with Intel Xeon CPU E5-2640 V3 (2.60 GHz; 32 cores) and 64 GB (gigabytes) RAM. The daily MODIS data for the whole South American continent from 2000 to 2021 accounted for 6.69 TB (terabytes). Processing monthly composites is computationally intensive due to loading all of the daily data for each month at once for a given tile. Thus, the main bottlenecks are for RAM and hard drive writing speed. For the workstation with 64 GB memory, the usage of 10 cores running in parallel processing was the optimal choice. The average processing time of each monthly composite for one tile was 6 min. Therefore, it took 26.2 h to process the 262 composites (March 2000 to December 2021) for each tile. Since we had 31 tiles covering South America, the total amount of time to process one viewing normalization was approximately a month (33.8 d). Consequently, the total time spent in computation was 101.5 d for processing the three viewing normalizations (nadir, backward, and forward scattering) and generating the NAD and ANI layers. Processing can also be done with less potent computers with a minimum of 16 GB RAM memory and four processing cores.
2.4 Time series availability and uncertainty
The monthly compositing process returned a time series dataset over all of the South American continent, with an average of 242±35 out of a maximum of 262 composites (period between March 2000 and December 2021) for each pixel, with some data missing due to a lack of high-quality observations (Fig. 2). Only 34.3 % of the available pixels have the full time series (262 composites). The Amazon region shows a lower mean number of samples in the time series, with an average of 231±29 composites, which can be seen in Fig. 2. This lower number of samples is due to the innate high cloud cover (Durieux et al., 2003). It is important to note that the AnisoVeg product was strictly created to analyze the land surface and does not cover waterbodies. Moreover, the period between March 2000 and June 2002 has higher amounts of missing data because it preceded the launch of the Aqua satellite. When data from both satellites (Terra and Aqua) were combined to create the product after 2002, we had a much better pixel-level data availability to produce dense time series. Although we have a dense time series across the Amazon Rainforest (Fig. 2a), the mean number of daily observations within a month for this region is relatively lower than that observed in more dry and seasonal regions of South America (Fig. 2b). Thus, we suggest using a number of sample layers as a proxy for the uncertainty in the retrieval of monthly composites to filter out pixels with a low number of samples (e.g., less than three observations per composite). The lower the number of samples 1 pixel has, the higher the uncertainty in the data analysis. Although we use the median values to aggregate observations within months and mitigate potential land cover changes, stand-replacing changes may cause inaccurate anisotropy estimates for the given monthly estimates. Hence, we advise filtering data for land use and land cover changes before using them to obtain the most accurate anisotropy estimates.
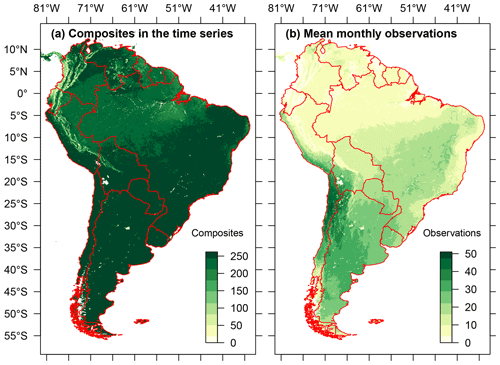
Figure 2AnisoVeg time series availability and uncertainty over South America. (a) The number of composites in the time series representing pixel availability. The maximum number of composites in the time series is 262 for the period between March 2000 and December 2021. (b) Mean number of daily observations within a month used to create the monthly composites as a proxy for uncertainty. The maximum daily observations in a composite is 60 (twice a day every day for a month).
We selected three experimental areas in the Brazilian Amazon Rainforest to show the spatial and temporal distribution of NAD and ANI data (rectangles in Fig. 1). These areas show old-growth rainforests with a distinct canopy structure and aboveground biomass (AGB) stocks. The AGB increases from semi-deciduous forests at Xingu Indigenous Park (190±19 Mg ha−1) and open ombrophilous forests with lianas at the São Félix do Xingu (241±31 Mg ha−1) to dense ombrophilous forests at the Tapajós National Forest (288±38 Mg ha−1), as estimated by the ESA/CCI (Climate Change Initiative) AGB map from 2017 (Santoro and Cartus, 2021). These are large-scale AGB estimates and may underestimate the true AGB at higher values such as in the Tapajós site. These three sites are also expected to show different phenological dynamics because their selected pixels cover distinct phenoregions in the study reported by Xu et al. (2015).
When compared to the nadir-normalized EVI (EVINAD) images (Fig. 3a–c), the anisotropy EVI (EVIANI) data showed different spatial patterns across sites (Fig. 3d–f). While the forests over the three sites showed approximately similar EVINAD values (EVINAD≈0.50; Fig. 3a–c), they showed more variability in EVIANI between the Xingu Indigenous Park (EVIANI>0.20), São Félix do Xingu (EVIANI>0.24), and Tapajós (EVIANI>0.27) sites (Fig. 3d–f). This increase in EVIANI between sites goes into the same direction of the AGB gradient observed from the Xingu Indigenous Park to the Tapajós National Forest. This result may indicate different forest canopy structures that were not captured in the EVINAD observations but were captured by the EVIANI. Overall, the EVIANI is high over forests (0.20 to 0.30) and low over pastures and crops (less than 0.10). This means large anisotropy between the reflected energy in backward- and forward-scattering MODIS directions due to the structural complexity of forest canopies. The association between anisotropy and forest canopy structure has been previously shown for the same region in a previous work (de Moura et al., 2016).
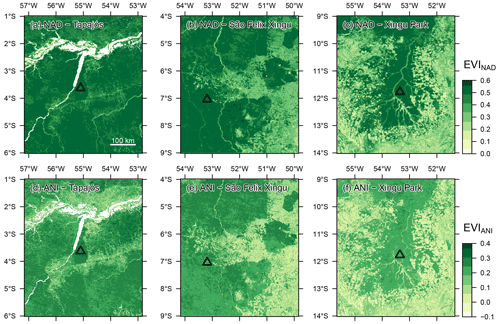
Figure 3The spatial distribution in August 2020 (dry season) of the nadir-normalized enhanced vegetation index (EVINAD) is shown in panels (a), (b), and (c) for Tapajós National Forest, São Félix do Xingu, and Xingu Indigenous Park, respectively. The corresponding results for the anisotropy EVI (EVIANI) are shown in panels (d), (e), and (f), respectively. The triangles plotted over panels (a–c) indicate the sites used to obtain the profiles in Fig. 4.
From the comparison of different sites (triangles in Fig. 3a), we observed that the mean EVINAD signal over the time period did not vary much between the selected forests, while the EVIANI varied greatly (Fig. 4) for Tapajós (mean EVINAD=0.49; mean EVIANI=0.27), São Félix do Xingu (mean EVINAD=0.51; mean EVIANI=0.24), and Xingu Indigenous Park (mean EVINAD=0.51; mean EVIANI=0.22). Moreover, EVINAD and EVIANI values were moderately positively correlated at Tapajós (), weakly correlated at São Félix do Xingu (), and moderately negatively correlated at the Xingu Indigenous Park (). The EVINAD and EVIANI seasonal variability and phase correlation changes from site to site, suggesting that different canopy dynamics processes are likely being captured by the two metrics at the three sites. Understanding exactly what those effects mean for these forests is beyond the scope of this paper. However, it indicates open avenues for studying forest functioning using these products. For example, previous studies have shown that EVINAD metrics captured different compositions of leaf ages in the canopies of the central Amazon (Gonçalves et al., 2020).
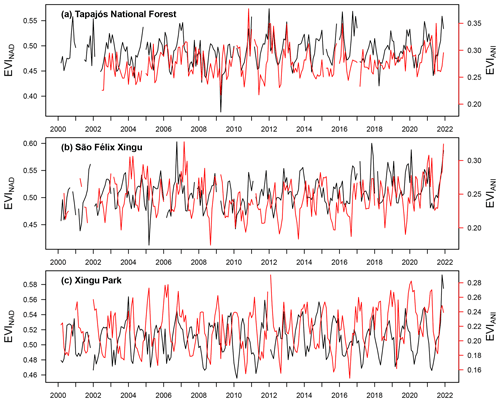
Figure 4Time series of AnisoVeg's MODIS enhanced vegetation index (EVI) from 2000 to 2021 for old-growth forests of (a) Tapajós National Forest, (b) São Félix do Xingu, and (c) Xingu Indigenous Park. The black line indicates the nadir-normalized signal (NAD layer), while the red line represents the EVI anisotropy (ANI layer). The profiles are the mean value of 3×3 pixels, whose locations are indicated by triangles in Fig. 3.
To demonstrate the potential of AnisoVeg for a large-scale forest structure inference, we compared the NAD and ANI data against forest height measurements from the Global Ecosystem Dynamics Investigation (GEDI) lidar sensor. We found that EVIANI was able to explain up to 55 % of the height variability in the Amazon forest, according to a simple linear relationship (R2=0.55; p<0.01; Fig. 5). This is a very strong predicting power for a single variable, considering a simple linear model, especially for satellite passive optical data which are often underrated for forest structure estimates in comparison to synthetic aperture radar (SAR) data. EVINAD was significantly but weakly associated with height variability (R2=0.16; p<0.01), reinforcing the increase in the explanation power owed to the anisotropy metrics built from multi-angle observations. The height data were derived from the GEDI lidar sensor aboard the International Space Station. They were obtained more specifically from the product GEDI L2A elevation and height metrics data version 2 (footprint size 25 m) acquired from April 2019 to October 2020 (available dates at the time of download). GEDI data were downloaded from Earthdata Cloud service system (https://earthdata.nasa.gov, last access: 16 January 2023). We selected the relative height metric at the 98th percentile (RH98), which represents the top canopy height. The selected RH98 metric was averaged over each 1 km grid cell and filtered using a threshold of greater than or equal to 50 shots per kilometer squared to have a high confidence of reliable height estimation representing the 1 km mean. The AnisoVeg data used for this comparison were based on the same time period as GEDI and filtered for EVINAD larger than 0.35 to exclude non-forested areas. While we only showed the plot for the strongest EVIANI : GEDI relationship in June 2019 (Fig. 5), the other months also showed significant (p<0.01) and strong relationships with R2, ranging from 0.36 to 0.55 (mean R2=0.46). Future studies should explore relationships using ANI from different months and other indices, either alone or in combination with each other, to further understand their significance for explaining forest structure. This is important to determine how the anisotropy data can contribute to aboveground biomass and carbon estimates in conjunction with other sources of data such as those from SAR sensors.
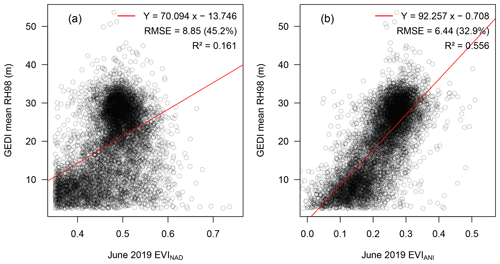
Figure 5Relationship between forest height (GEDI mean RH98) and two AnisoVeg layers obtained in June 2019 over the Amazon. (a) EVINAD and (b) EVIANI. The RH98 metric is the relative height at the 98th percentile, which represents the top of canopy height. A total of 7000 random matching pixels were used in this analysis (1 % of 700 000 total matching pixels available), resulting from the filtering of both GEDI and AnisoVeg data. The red line indicates the fitted line by a simple linear model.
Terrain illumination is a factor of spectral variability which can affect EVINAD determination and its relationship with biophysical attributes of vegetation, as shown by previous literature (Huang et al., 2010; Chen and Cao, 2012). Even at 1 km spatial resolution, EVIANI results of Figs. 3, 4, and 5 can be affected to some extent by terrain illumination effects observed locally at some sites. For instance, topographic effects on EVIANI probably occurred at the São Félix do Xingu site, where topographic roughness, observed in SRTM (Shuttle Radar Topography Mission) data (results not shown), was coincident with increased EVIANI values in Fig. 3e. Furthermore, even in relatively flat terrains, variations in topographic aspect (surface orientation to the Sun) can affect the EVI variability in MODIS data because of the different amounts of energy reflected in the NIR towards the sensor by inclined surfaces in the forward- and backward-scattering view directions. Such effects have been observed in southern Brazil with MODIS at 250 m spatial resolution and increased in magnitude at higher spatial resolution data obtained by other sensors (Galvão et al., 2016). Therefore, it may prove useful to include topographic variables in modeling exercises to offset these effects.
In a prospective analysis, we also explored the behavior of the two EVI AnisoVeg metrics over the Amazonian phenoregions mapped by Xu et al. (2015). The EVINAD and EVIANI monthly means over different phenoregions highlighted the strong heterogeneity of the Amazon forest (Fig. 6). For instance, the profiles showed strong differences between both metrics from January to September in a phenoregion with well-defined dry and wet seasons (phenoregion 1 in Fig. 6a at Xingu Indigenous Park). Large differences between EVINAD and EVIANI were also observed in some phenoregions without a very long dry season in the northwestern Amazon (phenoregion 5 in Fig. 6e). On the other hand, EVINAD and EVIANI showed temporal decoupling in phenoregion 3 located in the central–eastern Amazon (Fig. 6c). Overall, while the seasonality of EVINAD has been investigated by many studies in the past, the seasonality of EVIANI is something to be further explored with the support of auxiliary data (e.g., airborne lidar and field campaigns). This is important to better understand the differences in seasonal patterns between both AnisoVeg metrics.
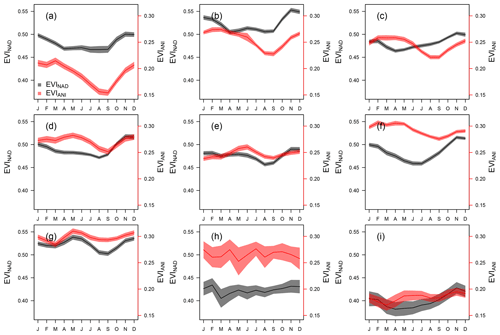
Figure 6Monthly means of EVINAD (black) and EVIANI (red) for nine phenoregions mapped by Xu et al. (2015) in the Amazon. The phenoregions are shown in increasing order from 1 to 9 in corresponding panels (a) to (i). They represent forests with similar seasonality and landscape structure. Solid lines and shaded areas represent the mean and 95 % confidence interval around the mean. The values were extracted from 20 years of data (from 2001 to 2021) for 100 random coordinates within each region and extracted from 3×3 windows of pixels.
Table 3Examples of other multi-angular anisotropy indices that can be further calculated using the layers of the AnisoVeg product. Lambda represents the selected spectral band or vegetation index. N, B, and F represent nadir view normalization, backward-scattering, and forward-scattering estimates, respectively.
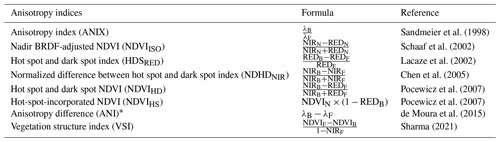
* ANI is included in the AnisoVeg product. Source adapted from Sharma (2021).
All code is available from the Zenodo repository (https://doi.org/10.5281/zenodo.6561350; Dalagnol and Wagner, 2022). The full dataset can be found at the official AnisoVeg Zenodo repository (https://doi.org/10.5281/zenodo.3878879; Dalagnol et al., 2022). The dataset was organized in compressed files (*.zip format), sub-divided by years (currently 2000–2021) and layers (bands 1–8, NDVI, and EVI) for both nadir normalization (code = NAD) and anisotropy (code = ANI). The number of sample layers (code = NO_SAMPLES) is also provided. Inside each compressed file are 12 image files (*.tif format), with one per month, except for the year 2000, which starts in March. The storage size for the whole dataset is 162.6 GB. The data have a scale factor of 10 000 to reduce the file storage size. Thus, to obtain surface reflectance values of bands or correct the range of values for indices, you should divide the layers by 10 000. The exception is the number of samples, which already shows the correct range of values (from 0 to 60 observations). It is planned to update the dataset on a yearly basis. Auxiliary data that allow the calculation of other anisotropy metrics (listed in Table 3) are included in two separate Zenodo repositories for backward (https://doi.org/10.5281/zenodo.6040300; Dalagnol, 2022a) and forward scattering (https://doi.org/10.5281/10.5281/zenodo.6048785; Dalagnol, 2022b), including the selected layers (red, NIR, NDVI, and EVI). The EVIANI and EVINAD layers were also uploaded to the GEE platform using the geeup tool v0.5.3 (Roy, 2022). They can be accessed through the GEE ImageCollection assets (projects/anisoveg/assets/evi_anisotropy and “projects/anisoveg/assets/evi_nadir), which can be found at https://code.earthengine.google.com/?asset=projects/anisoveg/assets/evi_anisotropy (last access: 16 January 2023, Dalagnol, 2022d) and https://code.earthengine.google.com/?asset=projects/anisoveg/assets/evi_nadir (last access: 16 January 2023, Dalagnol, 2022c).
The NAD layers from the AnisoVeg product have been used in previous studies to explore the climate drivers of the Amazon forest greening (Wagner et al., 2017), the large-scale Amazon forest sensitivity to drought (Anderson et al., 2018), the structure and dominance of bamboo species in the southwestern Amazon (Dalagnol et al., 2018), the productivity in a flooded forest in the eastern Amazon (Fonseca et al., 2019), the productivity and relationship with sun-induced fluorescence over the Brazilian Caatinga biome (Bontempo et al., 2020), the relationships in the leaf age demography in central Amazon (Gonçalves et al., 2020), and the relationships between fire disturbance and SAR-based vegetation optical depth in southern Amazon (Zhang et al., 2021).
The ANI layers from the AnisoVeg product have been mainly used to characterize the Amazon forest structure properties (de Moura et al., 2015, 2016). These layers now open new avenues of investigation for vegetation, including (but not limited to) the characterization of biophysical attributes of forests, including their seasonality and trends, the assessment of changes in vegetation structure due to natural disturbances or degradation (logging, fire, and edge effects), and the evaluation of forest health and productivity (greenness and browning). We expect that this dataset contributes to upscaling studies over large areas of key forest properties, such as the AGB and canopy roughness (Foody and Curran, 1994; Saatchi et al., 2008). This information is required for dynamic vegetation models to accurately represent the carbon cycle. This dataset is not limited to the study of Amazonian forests and can be used to explore other biomes of South America, such as the Atlantic Forest, savannas (Cerrado), Caatinga, Chaco, Pantanal, and Pampas. Such studies could improve our understanding of large-scale vegetation functioning, carbon storage, and cycling. Ultimately, they can contribute to refining global ecosystem models and obtaining accurate estimates of the carbon cycle in response to climate and environmental change. Furthermore, auxiliary backward- and forward-scattering data are also available with the dataset. Beyond the use of the provided ANI layers, this effectively allows the computation of several other multi-angular anisotropy indices from the literature (Table 3). The advantage (or disadvantage) of one specific anisotropy index rather than others is not established in the literature, given the range of vegetation applications and the lack of available datasets to date. We calculated and provided only ANI, due to its demonstrated relationships with Amazon forest structure and functioning (de Moura et al., 2015, 2016; Hilker et al., 2017). However, we expect other indices, including ratios and normalized differences between the backward and forward scattering components and offer additional possibilities for tropical vegetation studies which should be explored in future studies.
RD and YMdM conceived the presented idea. RD designed the methodology, with contributions from YMdM on the anisotropy method. RD conducted formal analysis and investigation, with contributions from LSG, FHW, NG, and SS. YW and AL provided the original MODIS (MAIAC) data and support for processing it. YY and SS provided the processed GEDI height data and support to analyze it. RD and FHW developed the code to process the MODIS (MAIAC) data into the products. RD conducted data curation of the products. LEOCA supervised the project. RD wrote the original draft, with support from LSG, FHW and YMdM. All authors read, reviewed, and approved the final version of the paper.
The contact author has declared that none of the authors has any competing interests.
Publisher's note: Copernicus Publications remains neutral with regard to jurisdictional claims in published maps and institutional affiliations.
Ricardo Dalagnol has been supported by the São Paulo Research Foundation (FAPESP; grant nos. 2015/22987-7 and 2019/21662-8). Fabien Hubert Wagner has been supported by FAPESP (grant no. 2015/50484-0). Part of this work was carried out at the Jet Propulsion Laboratory, California Institute of Technology, under a contract with the National Aeronautics and Space Administration (NASA). The funders had no role in the study design, data collection and analysis, including the decision to publish or prepare the paper. We thank the MODIS MAIAC team from NASA, for providing the freely available MODIS (MAIAC) daily dataset. The authors thank the comments from four anonymous reviewers who helped improve this work.
This research has been supported by the Fundação de Amparo à Pesquisa do Estado de São Paulo (grant nos. 2015/22987-7, 2019/21662-8, and 2015/50484-0). Yhasmin Mendes de Moura was supported by Royal Society, Newton International Fellowship (grant no. NF170036).
This paper was edited by Dalei Hao and reviewed by four anonymous referees.
Anderson, L. O., Ribeiro Neto, G., Cunha, A. P., Fonseca, M. G., Mendes de Moura, Y., Dalagnol, R., Wagner, F. H., and Aragão, L.: Vulnerability of Amazonian forests to repeated droughts, Philos. T. Roy. Soc. B, 373, 20170411, https://doi.org/10.1098/rstb.2017.0411, 2018.
Bhandari, S., Phinn, S., and Gill, T.: Assessing viewing and illumination geometry effects on the MODIS vegetation index (MOD13Q1) time series: implications for monitoring phenology and disturbances in forest communities in Queensland, Australia, Int. J. Remote Sens., 32, 7513–7538, https://doi.org/10.1080/01431161.2010.524675, 2011.
Bi, J., Knyazikhin, Y., Choi, S., Park, T., Barichivich, J., Ciais, P., Fu, R., Ganguly, S., Hall, F., Hilker, T., Huete, A., Jones, M., Kimball, J., Lyapustin, A. I., Mõttus, M., Nemani, R. R., Piao, S., Poulter, B., Saleska, S. R., Saatchi, S. S., Xu, L., Zhou, L., and Myneni, R. B.: Sunlight mediated seasonality in canopy structure and photosynthetic activity of Amazonian rainforests, Environ. Res. Lett., 10, 064014, https://doi.org/10.1088/1748-9326/10/6/064014, 2015.
Bontempo, E., Dalagnol, R., Ponzoni, F., and Valeriano, D.: Adjustments to SIF aid the interpretation of drought responses at the caatinga of Northeast Brazil, Remote Sens., 12, 1–29, https://doi.org/10.3390/rs12193264, 2020.
Chen, J. M., Liu, J., Leblanc, S. G., Lacaze, R., and Roujean, J. L.: Multi-angular optical remote sensing for assessing vegetation structure and carbon absorption, Remote Sens. Environ., 84, 516–525, https://doi.org/10.1016/S0034-4257(02)00150-5, 2003.
Chen, J. M., Menges, C. H., and Leblanc, S. G.: Global mapping of foliage clumping index using multi-angular satellite data, Remote Sens. Environ., 97, 447–457, https://doi.org/10.1016/j.rse.2005.05.003, 2005.
Chen, W. and Cao, C.: Topographic correction-based retrieval of leaf area index in mountain areas, J. Mountain Sci., 9, 166–174, https://doi.org/10.1007/s11629-012-2248-2, 2012.
Dalagnol, R.: Back scattering data of AnisoVeg: Anisotropy and Nadir-normalized MODIS MAIAC datasets for satellite vegetation studies in South America, Zenodo [data set], https://doi.org/10.5281/zenodo.6040300, 2022a.
Dalagnol, R.: Forward scattering data of AnisoVeg: Anisotropy and Nadir-normalized MODIS MAIAC datasets for satellite vegetation studies in South America, Zenodo, https://doi.org/10.5281/zenodo.6048785 [data set], 2022b.
Dalagnol, R.: EVI Nadir layer from the AnisoVeg dataset, https://code.earthengine.google.com/?asset=projects/anisoveg/assets/evi_nadir (last access: 16 January 2023), 2022c. Dalagnol, R.: EVI Anisotropy layer from the AnisoVeg dataset, https://code.earthengine.google.com/?asset=projects/anisoveg/assets/evi_anisotropy (last access: 16 January 2023), 2022d.
Dalagnol, R. and Wagner, F. H.: maiac_processing: Script and functions to process daily MODIS MAIAC data to BRDF-corrected 16-day and monthly mosaic composites (Version 1.0), Zenodo [code], https://doi.org/10.5281/zenodo.6561350, 2022.
Dalagnol, R., Wagner, F. H., Galvão, L. S., Nelson, B. W., and Aragão, L. E. O. E. C. D.: Life cycle of bamboo in the southwestern Amazon and its relation to fire events, Biogeosciences, 15, 6087–6104, https://doi.org/10.5194/bg-15-6087-2018, 2018.
Dalagnol, R., Galvão, L. S., Wagner, F. H., Moura, Y. M., Gonçalves, N., Wang, Y., Lyapustin, A., Yang, Y., Saatchi, S., and Aragão, L. E. O. C.: AnisoVeg: Anisotropy and Nadir-normalized MODIS MAIAC datasets for satellite vegetation studies in South America (Version v1), Zenodo [data set], https://doi.org/10.5281/zenodo.3878879, 2022.
de Moura, Y. M., Hilker, T., Lyapustin, A. I., Galvão, L. S., dos Santos, J. R., Anderson, L. O., de Sousa, C. H. R., and Arai, E.: Seasonality and drought effects of Amazonian forests observed from multi-angle satellite data, Remote Sens. Environ., 171, 278–290, https://doi.org/10.1016/j.rse.2015.10.015, 2015.
de Moura, Y. M., Hilker, T., Gonçalves, F. G., Galvão, L. S., dos Santos, J. R., Lyapustin, A., Maeda, E. E., and de Jesus Silva, C. V.: Scaling estimates of vegetation structure in Amazonian tropical forests using multi-angle MODIS observations, Int. J. Appl. Earth Obs., 52, 580–590, https://doi.org/10.1016/j.jag.2016.07.017, 2016.
de Sousa, C. H. R., Hilker, T., Waring, R., de Moura, Y. M., and Lyapustin, A.: Progress in remote sensing of photosynthetic activity over the amazon basin, Remote Sens., 9, 1–23, https://doi.org/10.3390/rs9010048, 2017.
Diner, D. J., Braswell, B. H., Davies, R., Gobron, N., Hu, J., Jin, Y., Kahn, R. A., Knyazikhin, Y., Loeb, N., Muller, J. P., Nolin, A. W., Pinty, B., Schaaf, C. B., Seiz, G., and Stroeve, J.: The value of multiangle measurements for retrieving structurally and radiatively consistent properties of clouds, aerosols, and surfaces, Remote Sens. Environ., 97, 495–518, https://doi.org/10.1016/j.rse.2005.06.006, 2005.
Durieux, L., Toledo Machado, L. A., and Laurent, H.: The impact of deforestation on cloud cover over the Amazon arc of deforestation, Remote Sens. Environ., 86, 132–140, https://doi.org/10.1016/S0034-4257(03)00095-6, 2003.
Fonseca, L. D. M., Dalagnol, R., Malhi, Y., Rifai, S. W., Costa, G. B., Silva, T. S. F., Da Rocha, H. R., Tavares, I. B., and Borma, L. S.: Phenology and Seasonal Ecosystem Productivity in an Amazonian Floodplain Forest, Remote Sens., 11, 1530, https://doi.org/10.3390/rs11131530, 2019.
Foody, G. M. and Curran, P. J.: Estimation of Tropical Forest Extent and Regenerative Stage Using Remotely Sensed Data, J. Biogeogr., 21, 223, https://doi.org/10.2307/2845527, 1994.
Galvão, L. S., Ponzoni, F. J., Epiphanio, J. C. N., Rudorff, B. F. T., and Formaggio, A. R.: Sun and view angle effects on NDVI determination of land cover types in the Brazilian Amazon region with hyperspectral data, Int. J. Remote Sen., 25, 1861–1879, https://doi.org/10.1080/01431160310001598908, 2004.
Galvão, L. S., dos Santos, J. R., Roberts, D. A., Breunig, F. M., Toomey, M., and de Moura, Y. M.: On intra-annual EVI variability in the dry season of tropical forest: A case study with MODIS and hyperspectral data, Remote Sens. Environ., 115, 2350–2359, https://doi.org/10.1016/j.rse.2011.04.035, 2011.
Galvão, L. S., Breunig, F. M., Santos, J. R. dos, and de Moura, Y. M.: View-illumination effects on hyperspectral vegetation indices in the Amazonian tropical forest, Int. J. Appl. Earth Obs., 21, 291–300, https://doi.org/10.1016/j.jag.2012.07.005, 2013.
Galvão, L. S., Breunig, F. M., Teles, T. S., Gaida, W., and Balbinot, R.: Investigation of terrain illumination effects on vegetation indices and VI-derived phenological metrics in subtropical deciduous forests, GIScience and Remote Sensing, 53, 360–381, https://doi.org/10.1080/15481603.2015.1134140, 2016.
Gao, F., Schaaf, C. B., Strahler, A. H., Jin, Y., and Li, X.: Detecting vegetation structure using a kernel-based BRDF model, Remote Sens. Environ., 86, 198–205, https://doi.org/10.1016/S0034-4257(03)00100-7, 2003.
Gobron, N., Pinty, B., Verstraete, M. M., Widlowski, J. L., and Diner, D. J.: Uniqueness of multiangular measurements – Part II: Joint retrieval of vegetation structure and photosynthetic activity from MISR, IEEE T. Geosci. Remote, 40, 1574–1592, https://doi.org/10.1109/TGRS.2002.801147, 2002.
Gonçalves, N. B., Lopes, A. P., Dalagnol, R., Wu, J., Pinho, D. M., and Nelson, B. W.: Both near-surface and satellite remote sensing confirm drought legacy effect on tropical forest leaf phenology after 2015/2016 ENSO drought, Remote Sens. Environ., 237, 111489, https://doi.org/10.1016/j.rse.2019.111489, 2020.
Hilker, T., Lyapustin, A. I., Tucker, C. J., Sellers, P. J., Hall, F. G., and Wang, Y.: Remote sensing of tropical ecosystems: Atmospheric correction and cloud masking matter, Remote Sens. Environ., 127, 370–384, https://doi.org/10.1016/j.rse.2012.08.035, 2012.
Hilker, T., Lyapustin, A. I., Tucker, C. J., Hall, F. G., Myneni, R. B., Wang, Y., Bi, J., De Moura, Y. M., and Sellers, P. J.: Vegetation dynamics and rainfall sensitivity of the Amazon, P. Natl. Acad. Sci. USA, 111, 16041–16046, https://doi.org/10.1073/pnas.1404870111, 2014.
Hilker, T., Galvão, L. S., Aragão, L. E. O. C., de Moura, Y. M., do Amaral, C. H., Lyapustin, A. I., Wu, J., Albert, L. P., Ferreira, M. J., Anderson, L. O., dos Santos, V. A. H. F., Prohaska, N., Tribuzy, E., Barbosa Ceron, J. V., Saleska, S. R., Wang, Y., de Carvalho Gonçalves, J. F., de Oliveira Junior, R. C., Cardoso Rodrigues, J. V. F., and Garcia, M. N.: Vegetation chlorophyll estimates in the Amazon from multi-angle MODIS observations and canopy reflectance model, Int. J. Appl. Earth Obs., 58, 278–287, https://doi.org/10.1016/j.jag.2017.01.014, 2017.
Huang, W., Zhang, L., Furumi, S., Muramatsu, K., Daigo, M., and Li, P.: Topographic effects on estimating net primary productivity of green coniferous forest in complex terrain using Landsat data: a case study of Yoshino Mountain, Japan, Int. J. Remote Sens., 31, 2941–2957, https://doi.org/10.1080/01431160903140829, 2010.
Huete, A., Didan, K., Miura, T., Rodriguez, E., Gao, X., and Ferreira, L.: Overview of the radiometric and biophysical performance of the MODIS vegetation indices, Remote Sens. Environ., 83, 195–213, https://doi.org/10.1016/S0034-4257(02)00096-2, 2002.
Lacaze, R., Chen, J. M., Roujean, J. L., and Leblanc, S. G.: Retrieval of vegetation clumping index using hot spot signatures measured by POLDER instrument, Remote Sens. Environ., 79, 84–95, https://doi.org/10.1016/S0034-4257(01)00241-3, 2002.
Liesenberg, V., Galvão, L. S., and Ponzoni, F. J.: Variations in reflectance with seasonality and viewing geometry: Implications for classification of Brazilian savanna physiognomies with MISR/Terra data, Remote Sens. Environ., 107, 276–286, https://doi.org/10.1016/j.rse.2006.03.018, 2007.
Lyapustin, A. and Wang, Y.: MCD19A1 MODIS/Terra+Aqua Land Surface BRF Daily L2G Global 500m, 1km and 5km SIN Grid V006, NASA EOSDIS Land Processes DAAC [data set], https://doi.org/10.5067/MODIS/MCD19A1.006, 2018.
Lyapustin, A., Martonchik, J., Wang, Y., Laszlo, I., and Korkin, S.: Multiangle implementation of atmospheric correction (MAIAC): 1. Radiative transfer basis and look-up tables, J. Geophys. Res.-Atmos., 116, D03210, https://doi.org/10.1029/2010JD014985, 2011.
Lyapustin, A., Wang, Y., Korkin, S., and Huang, D.: MODIS Collection 6 MAIAC algorithm, Atmos. Meas. Tech., 11, 5741–5765, https://doi.org/10.5194/amt-11-5741-2018, 2018.
Lyapustin, A., Zhao, F., and Wang, Y.: A Comparison of Multi-Angle Implementation of Atmospheric Correction and MOD09 Daily Surface Reflectance Products From MODIS, Front. Remote Sens., 2, 1–15, https://doi.org/10.3389/frsen.2021.712093, 2021.
Lyapustin, A. I., Wang, Y., Laszlo, I., Hilker, T., Hall, G. F., Sellers, P. J., Tucker, C. J., and Korkin, S. V.: Multi-angle implementation of atmospheric correction for MODIS (MAIAC): 3. Atmospheric correction, Remote Sens. Environ., 127, 385–393, https://doi.org/10.1016/j.rse.2012.09.002, 2012.
Morton, D. C., Nagol, J., Carabajal, C. C., Rosette, J., Palace, M., Cook, B. D., Vermote, E. F., Harding, D. J., and North, P. R. J.: Amazon forests maintain consistent canopy structure and greenness during the dry season, Nature, 506, 221–224, https://doi.org/10.1038/nature13006, 2014.
Pocewicz, A., Vierling, L. A., Lentile, L. B., and Smith, R.: View angle effects on relationships between MISR vegetation indices and leaf area index in a recently burned ponderosa pine forest, Remote Sens. Environ., 107, 322–333, https://doi.org/10.1016/j.rse.2006.06.019, 2007.
R Core Team: R: A Language and Environment for Statistical Computing (v3.3.1), Vol. 1, Issue C, R Foundation for Statistical Computing, https://www.r-project.org/ (last access: 16 January 2023), 2016.
Rouse, J. W., Hass, R. H., Schell, J. A., and Deering, D. W.: Monitoring Vegetation Systems in the Great Plains with ERTS, Third Earth Resources Technology Satellite-1 Symposium, 1, 301–317, 1974.
Roy, S.: samapriya/geeup: geeup: Simple CLI for Earth Engine Uploads (0.5.8), Zenodo [code], https://doi.org/10.5281/zenodo.7047124, 2022.
Saatchi, S., Buermann, W., ter Steege, H., Mori, S., and Smith, T. B.: Modeling distribution of Amazonian tree species and diversity using remote sensing measurements, Remote Sens. Environ., 112, 2000–2017, https://doi.org/10.1016/j.rse.2008.01.008, 2008.
Saleska, S. R., Wu, J., Guan, K., Araujo, A. C., Huete, A., Nobre, A. D., and Restrepo-Coupe, N.: Dry-season greening of Amazon forests, Nature, 531, E4–E5, https://doi.org/10.1038/nature16457, 2016.
Sandmeier, S., Müller, C., Hosgood, B., and Andreoli, G.: Physical mechanisms in hyperspectral BRDF data of grass and watercress, Remote Sens. Environ., 66, 222–233, https://doi.org/10.1016/S0034-4257(98)00060-1, 1998.
Santoro, M. and Cartus, O.: ESA Biomass Climate Change Initiative (Biomass_cci): Global datasets of forest above-ground biomass for the years 2010, 2017 and 2018 (No. 2), Centre for Environmental Data Analysis [data set], https://doi.org/10.5285/84403d09cef3485883158f4df2989b0c, 2021.
Schaaf, C. B., Gao, F., Strahler, A. H., Lucht, W., Li, X., Tsang, T., Strugnell, N. C., Zhang, X., Jin, Y., Muller, J.-P., Lewis, P., Barnsley, M., Hobson, P., Disney, M., Roberts, G., Dunderdale, M., Doll, C., Robert, P., Hu, B. L., Shunlin, P., Jeffrey, L., and Roy, D.: First operational BRDF, albedo nadir reflectance products from MODIS, Remote Sens. Environ., 83, 135–148, 2002.
Sharma, R. C.: Vegetation structure index (Vsi): Retrieving vegetation structural information from multi-angular satellite remote sensing, J. Imaging, 7, 84, https://doi.org/10.3390/jimaging7050084, 2021.
Sims, D. A., Rahman, A. F., Vermote, E. F., and Jiang, Z.: Seasonal and inter-annual variation in view angle effects on MODIS vegetation indices at three forest sites, Remote Sens. Environ., 115, 3112–3120, https://doi.org/10.1016/j.rse.2011.06.018, 2011.
Wagner, F. H., Hérault, B., Rossi, V., Hilker, T., Maeda, E. E., Sanchez, A., Lyapustin, A. I., Galvão, L. S., Wang, Y., and Aragão, L. E. O. C.: Climate drivers of the Amazon forest greening, PLoS ONE, 12, 1–15, https://doi.org/10.1371/journal.pone.0180932, 2017.
Wanner, W., Li, X., and Strahler, H.: On the derivation of kernels for kernel-driven models of bidirectional reflectance, J. Geophys. Res., 100, 21077, https://doi.org/10.1029/95JD02371, 1995.
Wu, J., Kobayashi, H., Stark, S. C., Meng, R., Guan, K., Tran, N. N., Gao, S., Yang, W., Restrepo-Coupe, N., Miura, T., Oliviera, R. C., Rogers, A., Dye, D. G., Nelson, B. W., Serbin, S. P., Huete, A. R., and Saleska, S. R.: Biological processes dominate seasonality of remotely sensed canopy greenness in an Amazon evergreen forest, New Phytologist, 217, 1507–1520, https://doi.org/10.1111/nph.14939, 2018.
Xu, L., Saatchi, S. S., Yang, Y., Myneni, R. B., Frankenberg, C., Chowdhury, D., and Bi, J.: Satellite observation of tropical forest seasonality: Spatial patterns of carbon exchange in Amazonia, Environ. Res. Lett., 10, 084005, https://doi.org/10.1088/1748-9326/10/8/084005, 2015.
Zhang, H., Hagan, D. F. T., Dalagnol, R., and Liu, Y.: Forest Canopy Changes in the Southern Amazon during the 2019 Fire Season Based on Passive Microwave and Optical Satellite Observations, Remote Sens., 13, 2238, https://doi.org/10.3390/rs13122238, 2021.
- Abstract
- Introduction
- Methodology to compute the AnisoVeg product
- Spatial and temporal distribution of NAD and ANI data across the Amazon forest
- Code and data availability
- Prospective use of the dataset
- Author contributions
- Competing interests
- Disclaimer
- Acknowledgements
- Financial support
- Review statement
- References
- Abstract
- Introduction
- Methodology to compute the AnisoVeg product
- Spatial and temporal distribution of NAD and ANI data across the Amazon forest
- Code and data availability
- Prospective use of the dataset
- Author contributions
- Competing interests
- Disclaimer
- Acknowledgements
- Financial support
- Review statement
- References