the Creative Commons Attribution 4.0 License.
the Creative Commons Attribution 4.0 License.
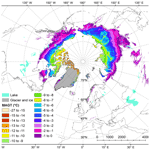
New high-resolution estimates of the permafrost thermal state and hydrothermal conditions over the Northern Hemisphere
Xin Li
Guodong Cheng
Jingxin Che
Juha Aalto
Olli Karjalainen
Jan Hjort
Miska Luoto
Huijun Jin
Jaroslav Obu
Masahiro Hori
Xiaoli Chang
Monitoring the thermal state of permafrost (TSP) is important in many environmental science and engineering applications. However, such data are generally unavailable, mainly due to the lack of ground observations and the uncertainty of traditional physical models. This study produces novel permafrost datasets for the Northern Hemisphere (NH), including predictions of the mean annual ground temperature (MAGT) at the depth of zero annual amplitude (DZAA) (approximately 3 to 25 m) and active layer thickness (ALT) with 1 km resolution for the period of 2000–2016, as well as estimates of the probability of permafrost occurrence and permafrost zonation based on hydrothermal conditions. These datasets integrate unprecedentedly large amounts of field data (1002 boreholes for MAGT and 452 sites for ALT) and multisource geospatial data, especially remote sensing data, using statistical learning modeling with an ensemble strategy. Thus, the resulting data are more accurate than those of previous circumpolar maps (bias = 0.02±0.16 ∘C and RMSE = 1.32±0.13 ∘C for MAGT; bias = 2.71±16.46 cm and RMSE = 86.93±19.61 cm for ALT). The datasets suggest that the areal extent of permafrost (MAGT ≤0 ∘C) in the NH, excluding glaciers and lakes, is approximately 14.77 (13.60–18.97) × 106 km2 and that the areal extent of permafrost regions (permafrost probability >0) is approximately 19.82×106 km2. The areal fractions of humid, semiarid/subhumid, and arid permafrost regions are 51.56 %, 45.07 %, and 3.37 %, respectively. The areal fractions of cold ( ∘C), cool (−3.0 ∘C to −1.5 ∘C), and warm ( ∘C) permafrost regions are 37.80 %, 14.30 %, and 47.90 %, respectively. These new datasets based on the most comprehensive field data to date contribute to an updated understanding of the thermal state and zonation of permafrost in the NH. The datasets are potentially useful for various fields, such as climatology, hydrology, ecology, agriculture, public health, and engineering planning. All of the datasets are published through the National Tibetan Plateau Data Center (TPDC), and the link is https://doi.org/10.11888/Geocry.tpdc.271190 (Ran et al., 2021a).
- Article
(12078 KB) - Full-text XML
- BibTeX
- EndNote
Permafrost is defined as ground that remains at or below 0 ∘C for at least 2 consecutive years (Van Everdingen, 2005). As a key component of the cryosphere in the Northern Hemisphere (NH), permafrost is sensitive to disturbances from climate change, environmental disturbances, and human activities (Shur and Jorgenson, 2007). Changes in the thermal state of permafrost (TSP) have multiple important impacts on infrastructure safety, hydrological cycles, ecosystems, and climate systems (Yoshikawa and Hinzman, 2003; Cheng, 2005; Cheng and Wu, 2007; Schuur et al., 2009; Tarnocai et al., 2009; Cheng and Jin, 2013; Gao et al., 2016; Hjort et al., 2018; Ran et al., 2018; Xie et al., 2019; Li et al., 2020a, b).
Over the past half century, hundreds of permafrost maps have been compiled at local to global scales (e.g., Heginbottom, 2002; Ran et al., 2012; Cao et al., 2019; Zhelezniak et al., 2021). On a global scale, the first permafrost map, the Circum-Arctic Map of Permafrost and Ground Ice Conditions, was compiled by the International Permafrost Association (IPA) using a manual delineation method by integrating all (readily) available data and regional permafrost maps in the 1990s (Heginbottom et al., 1993; Brown et al., 2002). The IPA permafrost map indicates that the permafrost region underlies an area spanning approximately 22.79×106 km2 in the NH, while the estimated areal extent actually underlain by permafrost ranges from 12.21×106 to 16.98×106 km2 (Zhang et al., 2000, 2008). The IPA permafrost map represents the best understanding of the permafrost distribution in the NH in the 1990s and has been frequently used for model evaluation or validation and even to perform derivative permafrost simulations (e.g., Chadburn et al., 2017). However, its quality and consistency vary because it is derived from the experience and knowledge of experts and depends on very limited observations at varied scales and accuracies and limited integration methods of mapping.
Since the release of the IPA permafrost map, great advances have been made in monitoring and modeling permafrost. In terms of data, the number of both ground-based observations and remote sensing data have remarkably increased in the past 30 years. Global and regional observation networks have been gradually established worldwide, strengthening permafrost monitoring in many regions, including Russia (e.g., Dvornikov et al., 2016), North America (e.g., Romanovsky and Osterkamp, 2001; Smith et al., 2005, 2010), Central Asia and China (e.g., Zhao et al., 2010b, 2021), and Europe (e.g., Harris et al., 2001; Mair et al., 2011; Kellerer-Pirklbauer et al., 2016). As a result of these regional networks, the Global Terrestrial Network for Permafrost (GTN-P) was established by the IPA in 1999 (Brown et al., 2000). The GTN-P monitors the TSP and active layer thickness (ALT) through the TSP and the Circumpolar Active Layer Monitoring (CALM) programs (Romanovsky et al., 2010; Biskaborn et al., 2015, 2019). At present, at a global scale, data on ground temperature measured at approximately 700 boreholes and ALT data from more than 200 sites can be freely downloaded from the GTN-P website (http://gtnp.arcticportal.org, last access: 20 March 2020). The data at some monitoring sites have been accumulating for decades (e.g., Biskaborn et al., 2015, 2019; Luo et al., 2016). Remote sensing observations are unprecedentedly abundant, and some variables related to permafrost, such as land surface temperature (LST), vegetation cover, and snow cover, can be retrieved from remote sensing sensors with high accuracy and spatial–temporal resolution (e.g., Justice et al., 2002; Zhao et al., 2013). The most significant methodological advances in mapping permafrost mainly include the expansion of permafrost models to applications of various spatial domains (Riseborough et al., 2008; Obu et al., 2019) and the extensive applications of machine learning to infer the occurrence and TSP (Aalto et al., 2018; Ran et al., 2021b).
With data accumulation and technical advances, several new hemispheric-scale permafrost maps have been compiled. Gruber (2012) proposed a simple semiempirical function relationship between the mean annual ground temperature (MAGT) and mean annual air temperature (MAAT) for estimating the global permafrost zonation at a 1 km scale using downscaled MAAT data. The map indicates an areal extent of regions actually underlain by permafrost in the NH of approximately 12.9×106 to 17.7×106 km2. Aalto et al. (2018) produced a distribution map of the circum-Arctic MAGT at the depth of zero annual amplitude (DZAA) using statistical forecasting models, with an estimated areal extent of regions actually underlain by permafrost of approximately km2, excluding the areal extent of permafrost to the south of 30∘ N in the NH. The performance of statistical models depends heavily on the in situ MAGT data used as a training set and the adopted predictors. The in situ data used in Aalto et al. (2018) were limited to those from the Qinghai–Tibet Plateau and Northeast China, while the predictor variables were mainly derived from downscaled monthly averages of climate data, which probably limited prediction accuracy from the perspective of permafrost mapping. Obu et al. (2019) employed an equilibrium state model for the temperature at the top of the permafrost (TTOP) for the 2000–2016 period, driven by remotely sensed LSTs, downscaled ERA-Interim climate reanalysis data, and a land cover map. The error of the modeled TTOP is ±2 ∘C in comparison with the data obtained from permafrost boreholes. This discrepancy is probably due to the inherent differences in the TTOP and the MAGT (at the DZAA) and the shortcomings of the model structure, which lead to large uncertainties, especially in regions of warm ( ∘C) permafrost, such as the Qinghai–Tibet Plateau (e.g., Wu et al., 2002; Riseborough, 2007; Zhao et al., 2017). The modeled areal extent of near-surface permafrost (TTOP <0 ∘C) covers an area of 13.9×106 km2 (Obu et al., 2019). The European Space Agency (ESA) Climate Change Initiative (CCI) also provides permafrost products including MAGT, ALT, and permafrost probability that are derived from a remote-sensing-driven CryoGrid model (Obu et al., 2021). The annual ALT and ground temperature at various depths during 1997–2019 with 1 km resolution are uniquely available for the permafrost and climate science communities. The abovementioned mapping efforts provide new-generation permafrost maps. However, the accuracy of these maps is still limited, especially at middle and low latitudes and at high elevations, due to the limitations of the data and models. These limitations may be overcome by integrating more ground observations and remote sensing data using statistical or machine-learning models that can improve upon physical-based models by automatically identifying better solutions (Bergen et al., 2019).
On the other hand, the maps of both the IPA and Obu et al. (2019) present permafrost zonation based on areal continuity. Areal-continuity-based map systems effectively reflect the permafrost distribution characteristics in high-latitude areas, but such systems are not suitable for describing high-altitude permafrost because the areal continuity of permafrost distribution is relative and scale-dependent (Nelson, 1989; Ran et al., 2012, 2021b). With a sufficiently high spatial resolution, all permafrost can be considered continuous or absent. Therefore, how to define the areal continuity of permafrost and the spatial resolution per se remains controversial and practically daunting. Additionally, continuity-based systems ignore the vertical distribution and longitudinal zonation of permafrost and thus cannot effectively reflect the hydrothermal conditions of permafrost, which are important for comprehensively understanding the characteristics and vulnerability of permafrost, especially at the regional scale (Cheng, 1984; Jin et al., 2014; Ran et al., 2021b). From a thermal stability perspective, for a given thermal condition and temperature increase in the air, permafrost temperature often responds more quickly in arid regions than in humid regions because of the much greater thermal inertia of wetter soils (Abu-Hamdeh, 2003). Dry soil can reduce evaporation heat consumption and increase the incident radiation on the soil (Pan and Mahrt, 1987). In addition, under different soil hydrological conditions, the response of permafrost to precipitation of various types may differ substantially (Trenberth and Shea, 2005). In humid regions, increased precipitation may heat the air and subsequently the active layer and permafrost, in contrast to that in arid regions. The difference in hydrothermal conditions is also reflected in the responses of ecosystems to precipitation because precipitation may alter or modify the effects of temperature on ecosystems (Zhao et al., 2018). Furthermore, ecosystems are very important for the thermal stability of permafrost under a changing climate (Shur and Jorgenson, 2007), although the interactions among climate, permafrost, and ecosystems are complex.
In short, the current statement of permafrost maps requires addressing the need for more comprehensive and integrated efforts to map the thermal state and hydrothermal zonation of permafrost. Thus, the objectives of this paper are as follows: (1) to develop and release the MAGT and ALT datasets as a baseline at 1 km scale in the NH, (2) to provide revised zonal statistics of the thermal state and distribution of permafrost in the NH, and (3) to present a permafrost zonation map that features the detailed hydrothermal conditions of permafrost.
We first compiled a ground measurement database for MAGT and ALT in the NH (see the workflow in Fig. 1). Then, the MAGT and ALT predictions with a 1 km resolution in the NH were produced using ensemble statistical forecasting by integrating the remotely sensed freezing degree days (FDD) and thawing degree days (TDD) (i.e., the total annual degree days below and above 0 ∘C, respectively), leaf area index (LAI), snow cover duration (SCD), precipitation (mm), solar radiation (kJ m−2 d−1), soil organic content (g kg−1), soil bulk density (kg m−3), coarse fragment content (volumetric %), and the compiled ground measurement data. Correspondingly, a dataset for the probability of permafrost occurrence was also produced. Finally, the hydrothermal conditions of permafrost were produced by combining the MAGT and aridity index using a rule-based decision-making process.
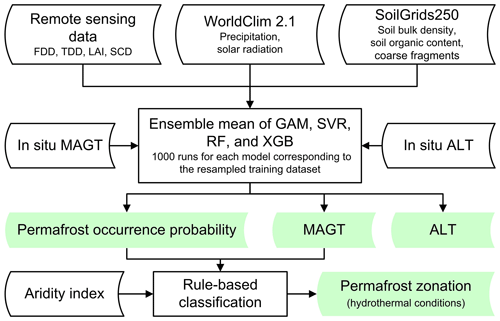
Figure 1The data processing workflow used to compile the permafrost datasets (FDD, freezing degree days; TDD, thawing degree days; LAI, leaf area index; SCD, snow cover duration; MAGT, mean annual ground temperature; ALT, active layer thickness; GAM, generalized additive model; SVR, support vector regression; RF, random forest; and XGB, extreme gradient boosting).
2.1 Field measurement data
The standardized measurements of MAGT at or near DZAA (approximately 3 to 25 m) from 1002 boreholes and those of ALT from 452 sites were compiled mainly based on the ground measurement data used in Aalto et al. (2018), but the density of data points on the Qinghai–Tibet Plateau, in the Tian Shan, and in Northeast China was greatly increased (Fig. 2). Additional MAGT measurements from 253 boreholes are used mainly from the sources in Ran et al. (2021b), which were compiled mostly from existing literature on the Qinghai–Tibet Plateau (Wu et al., 2007; Yu et al., 2008; Sheng et al., 2010; Li et al., 2011, 2016; Zhang et al., 2011; Sun et al., 2013; Wang et al., 2013; Liu et al., 2015; Qiao et al., 2015; Wu et al., 2015; Qin et al., 2017; Cao et al., 2017; Luo, 2012; Luo et al., 2018a; Wani et al., 2020; Zhao et al., 2021) and the Tian Shan (Yu et al., 2013; Liu et al., 2015). Other additional MAGT measurements from 19 boreholes in Northeast China are from Li et al. (2019) and Chang (2011). Additional ALT measurements from 149 sites were mainly compiled from permafrost studies on the Qinghai–Tibet Plateau and the Tian Shan (Zhao et al., 2010a; Luo, 2012, 2018b; Yu et al., 2013; Wu et al., 2015; Cao et al., 2017, 2018; Ali et al., 2018; Wani et al., 2020) and Northeast China (Chang, 2011; He et al., 2018; Li et al., 2020b). Only the measurement data from the undisturbed (natural) sites were used. Incomplete or inaccurate location information in some studies was corrected and checked carefully via communications with the authors. The elevations of these boreholes range from 0 to 5428 m above sea level (a.s.l.), and 99 % of these measurements were made during 2000–2016.
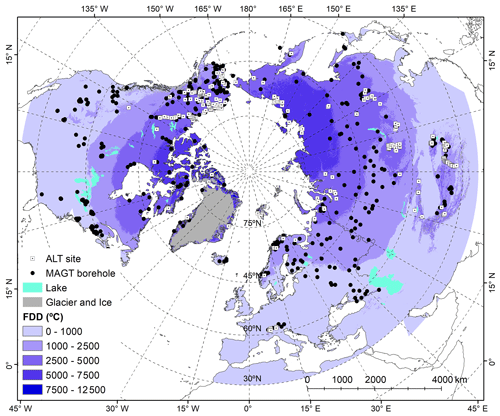
Figure 2The distribution of the boreholes (1002) for monitoring the mean annual ground temperature (MAGT) and the sites (452) for monitoring the active layer thickness (ALT) used in this study. FDD refers to the freezing degree days (∘C) derived from gap-filled daily MODIS land surface temperature.
For MAGT, to reduce the potential overrepresentation of MAGT observations around 0 ∘C, MAGT measurements in Aalto et al. (2018) both within and beyond the permafrost region were used (Karjalainen et al., 2019) but excluded the sites with MAGT ∘C (21 sites) to ensure the comparability of positive and negative temperature ranges, i.e., −15.5 and 15.5 ∘C. For some boreholes, exact DZAA values were not available, but multilayer time series measurement data were available; in these cases, DZAA was manually determined by examining the annual temperature variations (<0.1 ∘C) at various depths assuming that averaged year-round temperature measurements can be used to derive more representative annual means than single measurements (Aalto et al., 2018; Karjalainen et al., 2019). For other boreholes where exact DZAA values were not available but where single-time measurement data were available, the single-time temperature measurements at depths generally greater than 8 m (most approximately 15 m) below the ground surface were used, assuming that the depth was near the DZAA.
Overall, the proportion of MAGTs at or near DZAA was quite large when we considered only permafrost sites (84 %). However, for all 1002 sites, only 79 % of MAGTs were at or near the DZAA (Table A1 in Appendix A). This was due to the inclusion of non-permafrost sites for which there often was no information to determine the DZAA.
To reduce the potential overfitting due to residual autocorrelation, we resampled the training data 1000 times by excluding the sampling points within a distance of less than 3 km following Ran et al. (2021b). This resulted in using an average of 776 MAGT measurements and 276 ALT measurements for model training, and an average of 76 MAGT measurements and 30 ALT measurements were used for model evaluation per cross-validation run.
2.2 Environmental and climate data
Nine environmental and climate variables were selected as predictors in a statistical learning model to estimate the permafrost thermal state based on previous studies (Aalto et al., 2018; Ran et al., 2021b). These variables were derived from high-quality datasets available at present (Table 1).
Table 1Environmental and climate variable datasets used in this study to predict MAGT (mean annual ground temperature) and ALT (active layer thickness). MODIS, Moderate Resolution Imaging Spectroradiometer; LST, land surface temperature; AVHRR, Advanced Very High Resolution Radiometer; GLASS, Global Land Surface Satellite.
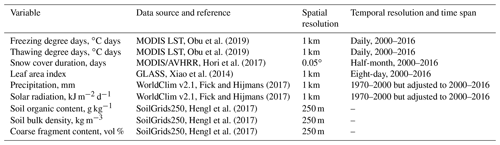
2.2.1 FDD and TDD
The multiannual average FDD and TDD with a spatial resolution of 1 km2 were derived from four times daily Moderate Resolution Imaging Spectroradiometer (MODIS) LST (MOD11A1 and MYD11A1 version 6) from 2000 to 2016. Due to cloud contamination, gap-filling processing was applied to the MODIS LST time series using the downscaled ERA-Interim near-surface air temperature data (Dee et al., 2011; Obu et al., 2019) based on the assumption of the similarity of air and surface temperatures under cloudy skies (Gallo et al., 2011). More details about the gap-filling processing can be found in Obu et al. (2019).
2.2.2 Snow cover duration
The annual SCD data were estimated from satellite-derived bimonthly global snow cover extent (SCE) products obtained from the Japan Aerospace Exploration Agency (JAXA) Satellite Monitoring for Environmental Studies website. The SCE products with 0.05∘ resolution were derived from polar-orbiting satellite-borne optical sensors (AVHRR and MODIS). Snow cover was identified using radiance data at five spectral bands from visible to thermal-infrared wavelength regions the same as those of AVHRR. The overall accuracy of the snow/no-snow-cover classification was estimated to be 0.82–0.99. The detailed analysis method and accuracies of the SCE product can be found in Hori et al. (2017). The SCD is the annual sum of the temporal fraction of snow cover within each bimonthly period. Then, the multiannual average values of SCD from 2000 to 2016 were used in this study.
2.2.3 Leaf area index
The multiannual average values of LAI from 2000 to 2016 were derived from the Global Land Surface Satellite (GLASS), an 8 d, 1 km resolution LAI product. The GLASS LAI product was proposed based on the integration of MODIS and CYCLOPES LAI products, and the remaining cloud contamination values were removed using general regression neural networks (Xiao et al., 2014). The validation results show a higher accuracy for the GLASS LAI product in comparison with the MODIS and CYCLOPES LAI products (Xiang et al., 2014).
2.2.4 Soil data
The soil organic content, bulk density, and coarse fragment content source from SoilGrids250 (https://soilgrids.org, last access: 14 May 2020) were used in this study. With a spatial resolution of 250 m, the SoilGrids250 is a global gridded soil map developed by the International Soil Reference and Information Center (ISRIC) using machine-learning algorithms by integrating 230 000 soil profiles and remote sensing environmental variables (Hengl et al., 2017). The soil organic content, bulk density, and coarse fragment content were derived for seven standard depths (0, 5, 15, 30, 60, 100, and 200 cm) with a depth interval weighted average process and then aggregated to 30 arcsec resolution using the nearest resampling technique.
2.2.5 Downscaled climate data
The WorldClim v2.1 climate variables with a 1 km resolution (http://worldclim.org, last access: 4 March 2020), including solar radiation (kJ m−2 d−1) and precipitation (mm) data (Fick and Hijmans, 2017), were used in this study. Solar radiation data were directly used, but the precipitation data for 1970–2000 were temporally adjusted to those for 2000–2016 based on WorldClim historical monthly weather data from 2000 to 2016 by using their locally smoothed (3×3 pixels) difference following Aalto et al. (2018).
2.3 Statistical learning model
The four statistical learning modeling techniques used in this study include the generalized additive model (GAM) (Hastie and Tibshirani, 2017), support vector regression (SVR) (Vapnik, 1995), random forest (RF) (Breiman, 2001), and extreme gradient boosting (XGB) (Friedman, 2001; Chen and Guestrin, 2016). The techniques were implemented based on the R packages mgcv (Wood, 2011) for GAM, randomForest (Liaw and Wiener, 2002) for RF, e1071 (Karatzoglou et al., 2006) for SVR, and xgboost (Chen and Guestrin, 2016) for XGB. The GAM is a semiparametric extension of the generalized linear model in which a smooth function is specified to fit the nonlinear function of multiple predictors to the response variable at the same time (Aalto et al., 2018). In this study, the maximum smoothing function was set to three, and the thin plate regression spline was used as the smoothing function. The RF is an ensemble learning algorithm for building and aggregating multiple decision trees. In this study, the number of trees is set to 400. Each tree is built using a randomly selected training dataset and three environmental variables to split each tree node. SVR features nonlinear kernel transformation, sparse solution, and maximal margin control (Awad and Khanna, 2015). It assumes that the maximum deviation (ε) (maximal margin) between the predicted and measured values can be tolerated, and thus an ε-insensitive loss function can be found to minimize the prediction error. The output model of the nonparametric technique depends on kernel functions. In this study, the default radial kernel function was used, and the grid search method based on 10-fold cross-validation was used to select the model parameters. A normalization method was used to avoid overfitting. XGB is an efficient implementation of a gradient boosted regression tree, which is an ensemble learning algorithm, and ensembles are built by merging multiple decision trees (Chen and Guestrin, 2016). The learning corrects the prediction errors from the prior models using an optimization algorithm by sequentially adding a tree. Here, XGB was implemented using the xgboost function through 50 rounds of 10-fold cross-validation, and maximum depth was set as 3.
To account for the uncertainty of a single run and single model, an ensemble average of the 1000 runs for the four model means was used to represent the distribution of MAGT and ALT in this study.
2.4 The probability of permafrost occurrence
The probability of permafrost occurrence is obtained by calculating the fraction of predicted MAGT ≤0 ∘C based on the multimodel ensemble results with 1000 runs. The areal extent of the permafrost region is defined as the regions where the probability of permafrost occurrence is greater than 0.
2.5 Hydrothermal-condition-based permafrost zonation
We propose a classification system based on the hydrothermal condition of permafrost by synthesizing the thermal condition system proposed by Cheng (1984) and later modified by Ran et al. (2018) and by taking into account the aridity system proposed by Jin et al. (2014) and UNEP (1997). This system divides permafrost into nine categories using a two-level hierarchical index system, i.e., with two criteria consisting of the MAGT and climate aridity index (CAI). At the first level, permafrost is divided into cold (MAGT ∘C), cool (−3.0 ∘C < MAGT ∘C), and warm (MAGT ∘C) using MAGT as the sole indicator. At the second level, permafrost is divided into humid (CAI >0.65), semiarid/subhumid (0.65≥ CAI >0.20), and arid (CAI ≤0.20) using the CAI, the ratio of mean annual precipitation to potential evapotranspiration (P/PET). CAI data with 1 km resolution were used, sourced from the global aridity index database (Trabucco and Zomer, 2019) derived from precipitation data, and potential evapotranspiration was modeled using the Penman–Monteith equation based on WorldClim 2.1 climate data for the 1970–2000 period (http://worldclim.org, last access: 4 March 2020). Then, majority statistics processes with rectangular 5×5 neighborhoods and boundary cleaning tools were used to remove the numerous small inclusions of permafrost zonation. Finally, glacier and lake areas were excluded. The extent of glaciers was sourced from the ESA CCI land cover map for 2010 (ESA, 2017), and the extent of lakes was sourced from the global lakes and wetlands database, level 1 (Lehner and Döll, 2004), which comprises large lakes (area ≥50 km2) and large reservoirs (storage capacity ≥0.5 km3).
2.6 Accuracy and uncertainty assessment
Model performance was assessed on the basis of the root-mean-square error (RMSE), bias, and square of the correlation coefficient (R2) computed by distance-blocked 10-fold cross-validation with 1000 repetitions. The uncertainty of the permafrost area was quantified using the 97.5th and 2.5th percentiles of the multimodel-ensemble-simulated MAGT with 1000 runs.
The cross-validation indicates that the ensemble average of four statistical techniques (GAM, SVR, RF, and XGB) achieved the highest accuracy for MAGT (RMSE = 1.32±0.13 ∘C, bias = 0.02±0.16 ∘C), but the RF model was significantly more accurate (RMSE = 85.47±20.39 cm, bias cm) than the ensemble mean (RMSE = 86.93±19.61 cm, bias = 2.71±16.46 cm) for ALT prediction (p≤0.001, paired sample t test, n=1000) (Table 2). The accuracy of the predicted MAGT in permafrost regions, with field measurements in permafrost sites (MAGT ≤0 ∘C) used as reference, was significantly higher (RMSE =1.06 ∘C, bias ∘C) than that in non-permafrost regions (RMSE = 1.56 ∘C, bias = 0.88 ∘C).
Based on the datasets (named NIEER in reference to the Northwest Institute of Eco-Environment and Resources, Chinese Academy of Sciences), we analyzed the distribution characteristics of MAGT, ALT, and the permafrost area/region changes along latitude, elevation, and aridity index transects and the hydrothermal conditions in the permafrost regions.
Table 2The predictive performance (mean ± SD) of the mean annual ground temperature (MAGT) and active layer thickness (ALT) for the four statistical learning models and their ensemble means.
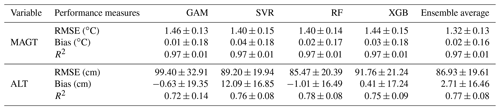
GAM: generalized additive model; SVR: support vector regression; RF: random forest; and XGB: extreme gradient boosting.
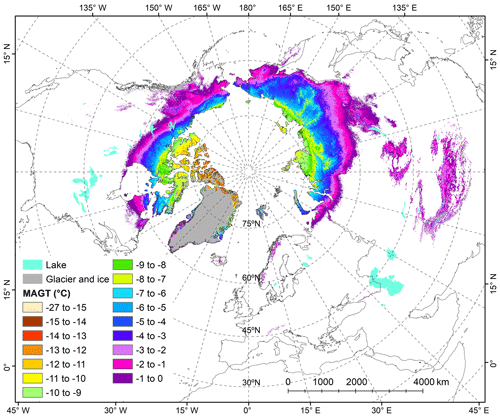
Figure 3Distribution of the mean annual ground temperature (MAGT) at the zero annual amplitude depth (approximately 3 to 25 m) average in the Northern Hemisphere for the period of 2000–2016.
3.1 Mean annual ground temperature and active layer thickness in the NH
Figure 3 shows the distribution of MAGT in the NH displaying an obvious latitudinal gradient from the zone of extremely cold ( ∘C) permafrost in the High Arctic to the zone of warm ( ∘C) permafrost in alpine and high-plateau regions at low latitudes, such as the Qinghai–Tibet and Mongolian plateaus, as well as the Yablonovy and Stanovoy mountains in southeastern Russia. In the Arctic permafrost region, the MAGT shows a clear distribution pattern of Arctic mountain permafrost in the eastern Siberian lowlands, on the Central Siberian Plateau, in the Ural Mountains, in Scandinavia in Europe, and in the upper Yukon River Basin in western Canada. The average MAGT is approximately ∘C in the Third Pole, which is cored by the Qinghai–Tibet Plateau, while that in the Arctic is approximately ∘C; the average MAGT is especially cold in the High Arctic, where it is close to −9.5 ∘C. The pattern of ALT in the NH is generally similar to that of MAGT, but the details vary markedly (Fig. 4). The regional average ALT varies from 76.95±21.69 cm in the High Arctic to 232.40±47.95 cm in the alpine and high-plateau permafrost regions at low latitudes with a narrow transition zone in the Mongolian Plateau and Northeast China.
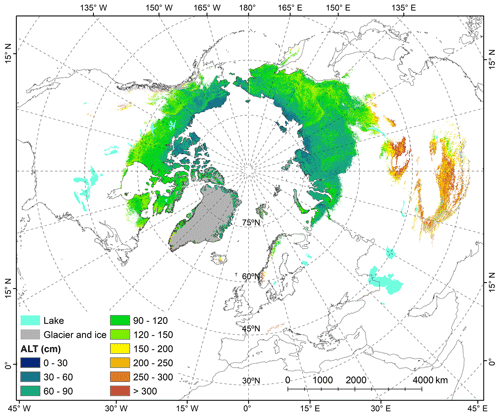
Figure 4Distribution of the average active layer thickness (ALT) in the Northern Hemisphere for the period of 2000–2016.
The three-dimensional ground thermal regimes across the NH are investigated on the basis of the simulated MAGT and ALT. Figure 5a illustrates the latitudinal distributive patterns of MAGT and ALT, which show some comparable and contrasting features between those of MAGT and ALT. Regional average MAGT is generally stable at approximately −1.5 ∘C from 28∘ N in the Himalayas to 60∘ N in the subarctic with a stable standard deviation. From 60∘ N northwards, with rising latitudes, the MAGT decreases linearly, but its variation increases. In contrast, the regional average ALT is nearly stable at 80 cm between 56 and 84∘ N, and there is a narrow transitional zone between 56 and 46∘ N, where the ALT enlarges rapidly from approximately 100 to 250 cm. These trends are considered to be predominantly associated with the transition from alpine/high-elevation plateau permafrost to lowland permafrost with generally more protective ecosystem properties (e.g., thicker overburdens). The contrasting latitudinal zonation in MAGT and ALT may indicate their varied geoenvironmental impacts on MAGT and ALT at different scales. The ALT represents the hydrothermal state near the ground surface with more spatiotemporal heterogeneity than the MAGT, which represents the thermal state of the relatively deeper ground. The vulnerability of the near-surface ground to external disturbances associated with the inconsistency of the ALT measurement method may be one of the reasons for the large uncertainty in the prediction of the ALT. Of course, the uncertainty of ALT is considerable, especially in the vast area of western Siberia where the training data are sparse. The low spatial representativeness of training data may lead to an overestimation in several Siberian mountain regions and underestimation near the lower boundary of permafrost. This highlights the importance and urgency of strengthening global coordinated ALT observation networks. Overall, the complexity of predictive models of ALT needs further investigation.
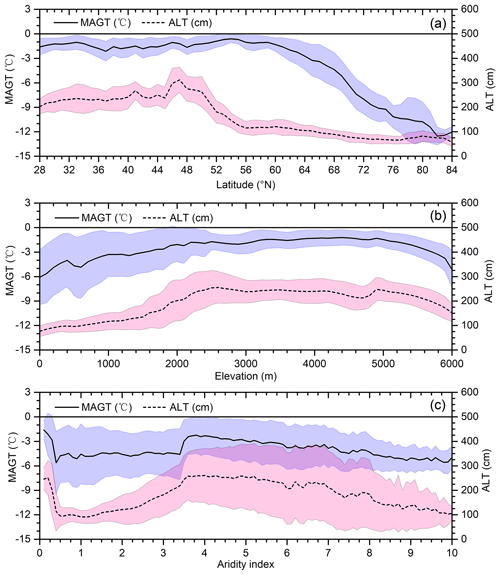
Figure 5Mean annual ground temperature (MAGT) and active layer thickness (ALT) along latitude (a), elevation (b), and aridity index (P/PET) (c) transects in the Northern Hemisphere (the black solid line is mean MAGT, and the light blue shaded area is its standard deviation; the dashed line is mean ALT, and the pink shaded area is its standard deviation; the dark pink area in panel c is the overlapped shaded area between ALT and MAGT).
The elevational effects on MAGT and ALT are modified by latitudinal effects at the hemispheric scale because mountains are widely distributed in the NH, with more in the lower latitudes. The combined effect of latitude and elevation differs among different elevation ranges. From 0 to 2500 m a.s.l., contrary to our understanding of the elevational effect at the regional scale, MAGT and ALT increase with increasing elevation, with large variations (Fig. 5b). However, the variations show opposite trends within this elevation range: with increasing elevation, the variation in MAGT declines, whereas that in ALT increases. In this elevation range, the elevational effect may be completely dominated by the latitudinal effect. The combined effect of latitude and elevation is moderate between 2500 and 5000 m a.s.l., where MAGT is stable at approximately −2 ∘C, with minor variation, and ALT is stable at approximately 220 cm. At elevations >5000 m a.s.l., the effect of elevation appears. MAGT and ALT both decline monotonically with increasing elevation.
The trend of MAGT corresponds well to that of ALT along the rising aridity index (Fig. 5c). For CAI values from 0.2 to 0.5, the MAGT and ALT both rapidly decrease with increasing CAI (moisture). However, in the range of CAI values from 0.5 to 3.6, MAGT and ALT both increase with increasing CAI. Then, above values of 3.6 on the CAI, both MAGT and ALT are nearly stable or slightly decreasing.
The three-dimensional zonal (latitudinal, elevation, and aridity) characteristics of MAGT and ALT discussed above probably indicate different controlling factors for MAGT and ALT and their variations. Multilinear regression analysis shows that the contributions of precipitation and soil bulk density to MAGT are statistically significant (p<0.01), but those to ALT are insignificant, and the contribution of the coarse fragment content of soils to MAGT is significant (p<0.01), whereas that to ALT is insignificant. The FDD, TDD, SCD, LAI, soil organic content, and solar radiation variables all contribute significantly to both MAGT and ALT (p<0.05). These differences may also be affected by the data gaps and uncertainty and analysis scales. In general, at the hemisphere scale, latitude dominates the distributive patterns and trends of MAGT and ALT. However, elevation and aridity become the main factors at the regional scale. For example, in mountainous areas of subarctic and low-latitude permafrost regions, elevation becomes the controller, especially above 5000 m a.s.l. (Fig. 5b). In plateau areas, such as the Qinghai–Tibet Plateau, which are dominated by semiarid/subhumid and arid hydrologic conditions, aridity becomes the main controller of ALT (p<0.05). This role of aridity has not received due attention in the literature, and further research is very much needed. This highlights the necessity of the preliminary hydrothermal zonation of permafrost in Sect. 3.3.
In addition, the DZAA represented by predicted MAGT varies in the NH (approximately 3 to 25 m). In general, in the continuous permafrost region in the Arctic and Qinghai–Tibet Plateau, the range in annual air temperature is large, and the zero annual amplitude is reached only at depths of 10 to 25 m below the surface (Ran et al., 2021b). These depths are greater than those in discontinuous permafrost and midlatitude regions, where the DZAA occurs at 5–10 m or less. This pattern is notably different from those of other MAGT products for specific depths.
3.2 Permafrost distribution in the NH
For permafrost distribution, two terms, i.e., permafrost area and permafrost region, should be distinguished. In general, the permafrost area is defined as the area with MAGT ≤0 ∘C, while the permafrost region is defined as the area where the permafrost probability is greater than 0 (Zhang et al., 2000). According to the ensemble average of MAGT, the permafrost area over the NH, excluding glaciers (approximately 0.64×106 km2) and lakes (approximately 0.3×106 km2), is approximately 14.77 km2. The range in permafrost area was quantified by using the 97.5th and 2.5th percentiles of the multimodel-ensemble-simulated MAGT with 1000 runs. This result is generally consistent with those of existing studies (Zhang et al., 2008; Gruber, 2012; Chadburn et al., 2017; Aalto et al., 2018; Obu et al., 2019) and logically consistent with the ESA CCI permafrost area defined according to MAGT at 2 m (Obu et al., 2021). Our results contain permafrost in discontinuous zones, while ESA CCI data contain no permafrost, because permafrost still may occur in deeper layers but has thawed at shallower depths. This difference is substantial particularly in the pan-Arctic. The new data indicate that the areal extent of the permafrost region is approximately 19.82×106 km2 with different occurrence probabilities (Fig. 6). This area is slightly less than that estimated in a recent report (Obu et al., 2019) and approximately 2.59×106 km2 less than that estimated in the IPA circum-Arctic permafrost map under the same conditions (i.e., excluding glaciers and water bodies). The differences in permafrost region between the NIEER map proposed in this study and the IPA map are distributed mainly in the southern/lower boundary of permafrost, such as in the Mongolian Plateau and Qinghai–Tibet Plateau (Fig. 7). This difference mainly reflects the difference in cartography technology between the two eras. The IPA map is a mechanical compilation of national maps, with each nation having their own mapping standard, which introduces multiple errors and uncertainties. Furthermore, the permafrost region of the new map uses permafrost probability (>0), based on multimodel-ensemble-simulated MAGT (≤0 ∘C), as the boundary. This probability mainly reflects the spatial variation of uncertainty of the ensemble model and differs from the definition of permafrost occurrence probability in Gruber (2012) and Cao et al. (2019). In the real world, MAGT (≤0 ∘C) is a very strict standard due to the effect of thermal offset within the active layer, and permafrost may exist between the DZAA and the depth of seasonal maximum thaw (i.e., the ALT).
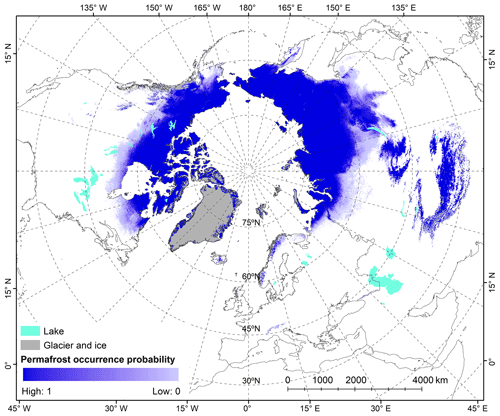
Figure 6The present-day probability of permafrost occurrence in the Northern Hemisphere for the period of 2000–2016 (the probability is defined by the fraction of predicted MAGT ≤0 ∘C based on the multimodel ensemble results with 1000 runs).
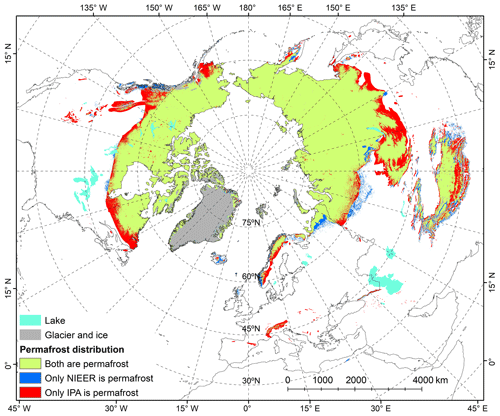
Figure 7The spatial difference of the permafrost region proposed in this study (defined as the regions where the probability of permafrost occurrence is greater than 0) with the circum-Arctic permafrost map from International Permafrost Association (Brown et al., 2002).
In general, at a global scale, latitude, elevation, and aridity/longitude mainly govern the distribution of permafrost (Cheng, 1984; Noetzli et al., 2017). The latitudinal dependence of the permafrost distribution is based on the latitudinal variation in insolation (incoming solar radiation) and surface energy balance, and thus on the subsequent latitudinal zonation of climate, soil, and vegetation. The dependence of permafrost distribution on elevation is due to the dependence of air temperature, soil, and vegetation on elevation and the strong lateral water–heat fluxes that occur at different scales from the latitudinal effect (Noetzli et al., 2017). At a global scale, climate aridity affects the distribution of permafrost primarily by climatic continentality. This reflects the vulnerability of permafrost dependence on the annual mean temperature range and variation in the net effect of precipitation and evapotranspiration. Here, we investigate the distribution of the permafrost region and permafrost area along latitude, elevation, and aridity transects in the NH (Fig. 8).
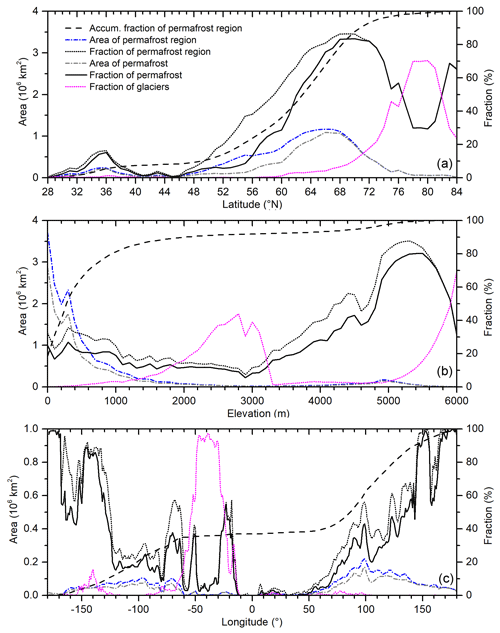
Figure 8Distributions of the permafrost region (permafrost probability >0) and permafrost area (MAGT ≤0 ∘C) along northern latitude (a), elevation (b), and longitude (c) transects in the Northern Hemisphere (blue short dash–dot line: area of permafrost region; grey short dash–dot line: area of permafrost region; black dotted line: fraction of permafrost region; black dashed line: accumulation fraction of permafrost region; black solid line: fraction of permafrost area; magenta dotted line: fraction of glacier area).
The permafrost distribution depends strongly on latitude. In the NH, permafrost occurs from 28∘ N in the Himalayas to north of 75∘ N in Greenland and the Canadian Arctic Archipelago, and more than 90 % is distributed in the regions north of 46∘ N, predominantly between 52 and 74∘ N (Fig. 8a). Approximately 8 % of the existing permafrost regions are distributed in the regions south of 45∘ N, mainly in the high-elevation regions of Asia, such as the Qinghai–Tibet Plateau between 28 and 40∘ N. With increasing latitude, the fraction of permafrost regions in the currently exposed land surface increases until approximately 70∘ N, where the fraction begins to decrease with the sharp increase in Arctic oceans, glaciers, and ice sheets. The profile of the permafrost area is generally consistent with that of the permafrost region. The decrease in the differences in the distributive trends and patterns of the permafrost region and permafrost area with rising northern latitude indicates the latitudinal dependence of permafrost areal continuity (PEC). The PEC is greater than 90 % north of approximately 66∘ N; i.e., terrestrial permafrost becomes continuous north of 66∘ N.
The permafrost distribution also clearly depends on elevation, especially in the mountainous and high-plateau regions at middle and low latitudes. Approximately 80 % of permafrost occurs below 1000 m a.s.l., and less than 10 % of permafrost occurs above 3000 m a.s.l. In the areas below 3000 m a.s.l., the area of permafrost decreases with increasing elevation, but its fraction of the land surface is approximately stable. In the areas above 3000 m a.s.l., the area of permafrost and its fraction of the exposed land surface both increase with rising elevation (Fig. 8b). This may indicate that latitude is the main controller of permafrost distribution below 3000 m a.s.l., while elevation is a more significant controller above 3000 m a.s.l.
Regarding the profile of permafrost distribution along northern longitude (Fig. 8c), our study results are generally consistent with those of existing studies (Zhang et al., 2008) but provide more spatial detail.
3.3 Permafrost hydrothermal conditions
The hydrothermal conditions of permafrost differ remarkably over the NH, from the warm–arid type dominating on the Qinghai–Tibet Plateau to the cold–humid type dominating in the High Arctic. Figure 9 shows the distribution of permafrost hydrothermal conditions in the NH. In the Eurasian Arctic, permafrost occurs mainly as the cold–humid type in western Siberia and as the cold-semiarid/subhumid type in central and eastern Siberia. While cold–semiarid/subhumid permafrost prevails in the western Canadian Arctic, the eastern Canadian Arctic contains cold–humid permafrost. The cold–humid type is dominant in Greenland. In the Alaskan Arctic, there is cold–semiarid/subhumid permafrost. In the permafrost regions at middle and low latitudes, such as the Qinghai–Tibet and Mongolian plateaus, permafrost occurs mainly as the warm–arid type. Warm–humid permafrost mainly occurs to the south of the continuous permafrost zone in western Siberia and eastern Canada.
In general, regarding moisture conditions, permafrost in the NH is dominated by the humid type, which accounts for approximately 51.56 % (10.22×106 km2) of the permafrost region. The areas of semiarid/subhumid and arid permafrost regions account for 45.07 % (8.93×106 km2) and 3.37 % (0.67×106 km2) of the region, respectively (Table 3). Regarding thermal conditions, the areas of cold, cool, and warm permafrost regions are approximately 7.49×106 (37.80 %), 2.84×106 (14.30 %), and 9.49×106 km2 (47.90 %), respectively. The hydrothermal conditions are closely related to the ecosystem and climate. Thus, it is helpful to understand the environmental changes in permafrost regions and their interaction with permafrost degradation.
Table 3The zonal areas of different permafrost hydrothermal conditions (106 km2) over the currently exposed land surface in the Northern Hemisphere.
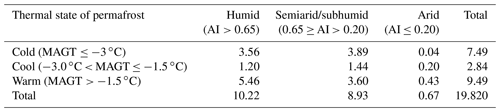
The NIEER datasets generated by this study (Table 4) are publicly available and can be downloaded at the National Tibetan Plateau Data Center (TPDC) (http://data.tpdc.ac.cn/en, last access: 16 December 2021) via https://doi.org/10.11888/Geocry.tpdc.271190 (Ran et al., 2021a). The MAGT, ALT, and permafrost probability in GeoTIFF format and the permafrost hydrothermal zonation map in ESRI shapefile format can be used with GIS software.
This study produced MAGT at or near the DZAA and ALT datasets with 1 km resolution for the period of 2000–2016 in the NH (named the NIEER map). The datasets integrate unprecedentedly large amounts of ground/field measurement data (1002 boreholes for MAGT and 452 sites for ALT) and multisource spatial data, including remotely sensed FDD and TDD, LAI, SCD, precipitation, solar radiation, soil organic content, soil bulk density, and coarse fragment content, using a multiple-statistical/machine-learning model with 1000 runs. The cross-validation shows that the accuracy of the MAGT (bias ∘C, RMSE = 1.32±0.13 ∘C) and ALT (bias = 2.71±16.46 cm, RMSE = 86.93±19.61 cm) data is likely greater than that in previous studies. The NIEER map suggests that the areal extent of permafrost (MAGT ≤0 ∘C) in the NH, excluding glaciers and lakes, is approximately 14.77 km2, which is generally consistent with recent reports. Furthermore, the area of permafrost regions (permafrost probability >0) (approximately 19.82×106 km2) in the NIEER map is less than that estimated in the IPA circum-Arctic permafrost map. This difference likely reflects the difference in cartography technology between the two eras. Based on the new permafrost classification system proposed in this study, a hydrothermal-condition-based permafrost zonation map was produced by combining the permafrost probability map with MAGT and the aridity index to describe the hydrothermal characteristics of permafrost in the NH. The results show that the areal fractions of humid, semiarid/subhumid, and arid permafrost regions are 51.56 %, 45.07 %, and 3.37 %, respectively. The areal fractions of cold ( ∘C), cool (−3.0 to −1.5 ∘C), and warm ( ∘C) permafrost regions are 37.80 %, 14.30 %, and 47.90 %, respectively. Based on high-quality datasets, this study has provided a more comprehensive and accurate understanding of the permafrost distribution in the NH through analyses of changes in the MAGT, ALT, and permafrost area/region along latitude, elevation, and aridity index transects. The model-predicted MAGT and ALT as well as the corresponding permafrost probability map and hydrothermal zonation map are potentially valuable for studies of cold regions and the Arctic in a variety of fields, such as climatology, hydrology, ecology, agriculture, public health, and engineering planning in the NH. Additionally, as baselines, these datasets are also important for predicting and rebuilding changes in permafrost features over the NH in the future and in the past.
YR, XL, and GC designed the study. YR prepared the datasets, wrote the manuscript, plotted the figures, and performed the analysis. YR designed the methodology, and JC implemented the statistical learning. JA, OK, JH, ML, QY, and XC contributed parts of the field data. JO provided the TDD and FDD data. XL, JA, OK, JH, ML, and HJ improved the writing and structure of the paper.
The contact author has declared that neither they nor their co-authors have any competing interests.
Publisher's note: Copernicus Publications remains neutral with regard to jurisdictional claims in published maps and institutional affiliations.
This article is part of the special issue “Extreme environment datasets for the three poles”. It is not associated with a conference.
This study was jointly supported by the Strategic Priority Research Program of the Chinese Academy of Sciences (grant no. XDA19070204) and the National Natural Science Foundation of China (grant no. 42071421). Olli Karjalainen and Jan Hjort acknowledge support from the Academy of Finland (grant no. 315519). FDD and TDD data were generated by the European Space Agency GlobPermafrost project (grant no. 4000116196/15/I-NB).
This research has been supported by the Strategic Priority Research Program of the Chinese Academy of Sciences (grant no. XDA19070204), the National Natural Science Foundation of China (grant no. 42071421), and the Academy of Finland (grant no. 315519).
This paper was edited by Kirsten Elger and reviewed by Stepan Varlamov and two anonymous referees.
Aalto, J., Karjalainen, O., Hjort, J., and Luoto, M.: Statistical forecasting of current and future Circum-Arctic ground temperatures and active layer thickness, Geophys. Res. Lett., 45, 4889–4898, 2018.
Abu-Hamdeh, N. H.: Thermal properties of soils as affected by density and water content, Biosyst Eng., 86, 97–102, 2003.
Ali, S. N., Quamar, M. F., Phartiyal, B., and Sharma, A.: Need for permafrost researches in Indian Himalaya, J. Clim. Chang., 4, 33–36, 2018.
Allard, M., Sarrazin, D., and L'Hérault, E.: Borehole and near-surface ground temperatures in northeastern Canada, Version 1.3 (1988–2014), Nordicana D [data set], https://doi.org/10.5885/45291SL-34F28A9491014AFD, 2015.
Awad, M. and Khanna, R.: Support Vector Regression, in: Efficient Learning Machines, Apress, Berkeley, CA, https://doi.org/10.1007/978-1-4302-5990-9_4, 2015.
Bergen, K. J., Johnson, P. A., Maarten, V., and Beroza, G. C.: Machine learning for data-driven discovery in solid Earth geoscience, Science, 363, eaau0323, https://doi.org/10.1126/science.aau0323, 2019.
Biskaborn, B. K., Lanckman, J.-P., Lantuit, H., Elger, K., Streletskiy, D. A., Cable, W. L., and Romanovsky, V. E.: The new database of the Global Terrestrial Network for Permafrost (GTN-P), Earth Syst. Sci. Data, 7, 245–259, https://doi.org/10.5194/essd-7-245-2015, 2015.
Biskaborn, B. K., Smith, S. L., Noetzli, J., Matthes, H., Vieira, G., Streletskiy, D. A., and Allard, M.: Permafrost is warming at a global scale, Nat. Commun., 10, 264, https://doi.org/10.1038/s41467-018-08240-4, 2019.
Blackett, R.: Utah Temperature-Depth Log Compilation, Utah Geological Survey [data set], http://search.geothermaldata.org/dataset/utah-temperature-depth-log-compilation (last access: 20 August 2015), 2013.
Breiman, L.: Random forests, Mach. Learn., 45, 5–32, 2001.
Brown, J., Ferrians, O., Heginbottom, J. A., and Melnikov, E.: Circum-Arctic Map of Permafrost and Ground-Ice Conditions, Version 2, Boulder, Colorado USA, NSIDC: National Snow and Ice Data Center [data set], https://doi.org/10.7265/skbg-kf16, 2002.
Brown, J., Hinkel, K. M., and Nelson, F. E.: The circumpolar active layer monitoring (CALM) program: research designs and initial results, Polar Geogr., 24, 166–258, 2000.
Cao, B., Gruber, S., Zhang, T., Li, L., Peng, X., Wang, K., Zheng, L., Shao, W., and Guo, H.: Spatial variability of active layer thickness detected by ground-penetrating radar in the Qilian Mountains, Western China, J. Geophys. Res. Earth. Surf., 122, 574–591, 2017.
Cao, B., Zhang, T., Peng, X., Mu, C., Wang, Q., Zheng, L., and Zhong, X.: Thermal characteristics and recent changes of permafrost in the upper reaches of the Heihe River basin, Western China, J. Geophys. Res.-Atmos., 123, 7935–7949, 2018.
Cao, B., Zhang, T., Wu, Q., Sheng, Y., Zhao, L., and Zou, D.: Permafrost zonation index map and statistics over the Qinghai-Tibet Plateau based on field evidence, Permafrost Periglac. Process., 30, 178–194, 2019.
Chadburn, S. E., Burke, E. J., Cox, P. M., Friedlingstein, P., Hugelius, G., and Westermann, S.: An observation-based constraint on permafrost loss as a function of global warming, Nat. Clim. Change, 7, 340–344, 2017.
Chang, X. L.: Thermal effect of vegetation and snow cover on the underlying permafrost and soils in the active layer in the northern Da Xiang'anling Mountains, Northeastern China, PhD thesis, Univ. Chinese Acad. Sci., 1–138, https://d.wanfangdata.com.cn/thesis/Y2031477 (last access: 18 July 2020), 2011 (in Chinese, English abstract).
Chen, T. Q. and Guestrin, C.: XGBoost: A Scalable Tree Boosting System, in: Proceedings of the 22nd ACM SIGKDD International Conference on Knowledge Discovery and Data Mining, ACM, New York, NY, USA, 785–794, https://doi.org/10.1145/2939672.2939785, 2016.
Cheng, G.: Problems on zonation of high-altitude permafrost, Acta. Geogr. Sin., 39, 185–193, 1984 (in Chinese).
Cheng, G.: A roadbed cooling approach for the construction of Qinghai–Tibet Railway, Cold Reg. Sci. Technol., 42, 169–176, 2005.
Cheng, G. and Jin, H. J.: Permafrost and groundwater on the Qinghai-Tibet Plateau and in northeast China, Hydrogeol. J., 21, 5–23, 2013.
Cheng, G. and Wu, T.: Responses of permafrost to climate change and their environmental significance, Qinghai-Tibet Plateau, J. Geophys. Res. Earth. Surf., 112, 93–104, 2007.
Crow, H. L., Good, R. L., Hunter, J. A., Burns, R. A., Reman, A., and Russell, H. A. J.: Borehole geophysical logs in unconsolidated sediments across Canada, Geological Survey of Canada [data set], Open File 7591, https://doi.org/10.4095/295753, 2015.
Curran, J., Rancan, H., and French, M.: New Jersey Well Logs, New Jersey Geological and Water Survey [data set], http://search.geothermaldata.org/dataset/new-jersey-well-logs (last access: 20 January 2016), 2013.
Czajkowski, J.: Washington Well Logs. Washington Division of Geology and Earth Resources, Department of Natural Resources [data set], http://search.geothermaldata.org/dataset/washington-well-logs (last access: 10 January 2016), 2012.
Dee, D. P., Uppala, S. M., Simmons, A. J., Berrisford, P., Poli, P., Kobayashi, S., Andrae, U., Balmaseda, M. A., Balsamo, G., Bauer, P., Bechtold, P., Beljaars, A. C. M., van de Berg, L., Bidlot, J., Bormann, N., Delsol, C., Dragani, R., Fuentes, M., Geer, A. J., Haimberger, L., Healy, S. B., Hersbach, H., Hólm, E. V., Isaksen, L., Kållberg, P., Köhler, M., Matricardi, M., McNally, A. P., Monge-Sanz, B. M., Morcrette, J.-J., Park, B.-K., Peubey, C., de Rosnay, P., Tavolato, C., Thépaut, J.-N., and Vitart, F.: The ERA-Interim reanalysis: configuration and performance of the data assimilation system, Q. J. Roy. Meteorol. Soc., 137, 553–597, 2011.
Dvornikov, Y., Leibmann, M., Heim, B., Bartsch, A., Haas, A., Khomutov, A., Gubarkov, A., Mikhaylova, M., Mullanurov, D., Widhalm, B., Skorospekhova, T., and Fedorova, I.: Geodatabase and WebGIS project for long-term permafrost monitoring at the Vaskiny Dachi research station, Yamal, Russia, Polarforschung, 85, 107–115, 2016.
Ednie, M., Chartrand, J., Smith, S. L., Duchesne, C., and Riseborough, D. W.: Report on 2011 Field Activities and Collection of Ground Thermal and Active Layer Data in the Mackenzie Corridor Completed Under Northwest Territories Science Licence #14918, Geological Survey of Canada [data set], Open File 7231, https://doi.org/10.4095/291982, 2012.
ESA: Land Cover CCI Product User Guide Version 2.0, http://maps.elie.ucl.ac.be/CCI/viewer/download/ESACCI-LC-Ph2-PUGv2_2.0.pdf, (last access: 4 March 2021), 2017.
Fick, S. E. and Hijmans, R. J.: WorldClim 2: new 1km spatial resolution climate surfaces for global land areas, Int. J. Climatol., 37, 4302–4315, 2017.
Friedman, J. H.: Greedy function approximation: a gradient boosting machine, Ann. Stat., 29, 1189–1232, 2001.
Gallo, K., Hale, R., Tarpley, D., and Yu, Y.: Evaluation of the relationship between air and land surface temperature under clear-and cloudy-sky conditions, J. Appl. Meteorol. Climatol., 50, 767–775, 2011.
Gao, T., Zhang, T., Cao, L., Kang, S., and Sillanpää, M.: Reduced winter runoff in a mountainous permafrost region in the northern Tibetan Plateau, Cold Reg. Sci. Technol., 126, 36–43, 2016.
Geological Survey of Norway (NGU): Permafrost Svalbard, The NORPERM Permafrost Database [data set], http://geo.ngu.no/kart/permafrost_svalbard (last access: 23 April 2017), 2016.
Geophysical Institute Permafrost Laboratory (GIPL): Site Information and Historical Data Access, http://permafrost.gi.alaska.edu/sites_list (last access: 26 January 2016), 2010.
Gosnold, W.: Nebraska Temperature-Depth Data and Profiles, University of North Dakota [data set], http://search.geothermaldata.org/dataset/nebraska-temperature-depth-data-and-profiles (last access: 10 March 2016), 2013.
Gruber, S.: Derivation and analysis of a high-resolution estimate of global permafrost zonation, The Cryosphere, 6, 221–233, https://doi.org/10.5194/tc-6-221-2012, 2012.
Harris, C., Haeberli, W., Vonder Mühll, D., and King, L.: Permafrost monitoring in the high mountains of Europe: the PACE project in its global context, Permafrost Periglac. Process., 12, 3–11, 2001.
Harrison III, W. B.: Michigan Well Log Observation Data, Western Michigan University – Geosciences Department [data set], http://search.geothermaldata.org/dataset/michigan-well-log-observation-data (last access: 10 March 2016), 2012.
Hastie, T. J. and Tibshirani, R. J.: Generalized additive models, Routledge, https://doi.org/10.1201/9780203753781, 2017.
He, R., Jin, H., Chang, X., Wang, Y., and Wang, L.: Freeze-thaw processes of active-layer soils in the Nanweng'he River National Natural Reserve in the Da Xing'anling Mountains, northern Northeast China, Sci. Cold. Arid. Reg., 10, 104–113, 2018.
Heginbottom, J. A.: Permafrost mapping: a review, Prog. Phys. Geogr., 26, 623–642, 2002.
Heginbottom, J. A., Brown, J., Melnikov, E. S., and Ferrians Jr, O. J.: Circumarctic map of permafrost and ground ice conditions, Proc. Int. Conf. Permafrost. Natl. Snow Ice Data Cent./World Data Cent. Glaciol., Boulder, CO, 2, 1132–1136, 1993.
Hengl, T., Mendes de Jesus, J., Heuvelink, G. B., Ruiperez Gonzalez, M., Kilibarda, M., Blagotić, A., Shangguan, W., Wright, M. N., Geng, X., Bauer-Marschallinger, B., Guevara, M. A., Vargas, R., MacMillan, R. A., Batjes, N. H., Leenaars, J. G., and Ribeiro, E., Wheeler, I., Mantel, S., and Kempen, B.: SoilGrids250m: Global gridded soil information based on machine learning, PLoS One, 12, https://doi.org/10.1371/journal.pone.0169748, 2017.
Hjort, J., Karjalainen, O., Aalto, J., Westermann, S., Romanovsky, V. E., Nelson, F. E., Etzelmüller, B., and Luoto, M.: Degrading permafrost puts Arctic infrastructure at risk by mid-century, Nat. Commun., 9, 5147, https://doi.org/10.1038/s41467-018-07557-4, 2018.
Hori, M., Sugiura, K., Kobayashi, K., Aoki, T., Tanikawa, T., Kuchiki, K., Niwano, M., and Enomoto, H.: A 38-year (1978–2015) Northern Hemisphere daily snow cover extent product derived using consistent objective criteria from satellite-borne optical sensors, Remote Sens. Environ., 191, 402–418, 2017.
Huang, S., Pollack, H. N., and Shen, P.-Y.: Temperature trends over the past five centuries reconstructed from borehole temperatures, Nature, 403, 756–758, 2000.
Jin, H., Lü, L., and He, R.: A new aridity-based classification of permafrost zones on the Tibetan Plateau, J. Glaciol. Geocryol., 36, 1049–1057, 2014 (in Chinese, English abstract).
Justice, C. O., Townshend, J. R. G., Vermote, E. F., Masuoka, E., Wolfe, R. E., Saleous, N., Roy, D. P., and Morisette, J. T.: An overview of MODIS Land data processing and product status, Remote Sens. Environ., 83, 3–15, 2002.
Karatzoglou, A., Meyer, D., and Hornik, K.: Support vector machines in R, J. Stat. Softw., 15, 1–28, 2006.
Karjalainen, O., Aalto, J., Luoto, M., Westermann, S., Romanovsky, V. E., Nelson, F. E., Etzelmüller, B., and Hjort, J.: Circumpolar permafrost maps and geohazard indices for near-future infrastructure risk assessments, Sci. Data, 6, 1–16, 2019.
Kellerer-Pirklbauer, A., Bartsch, A., Gitschthaler, C., Reisenhofer, S., Weyss, G., Riedl, C., and Avian, M.: A national strategy for a long-term monitoring of permafrost and periglacial processes and their relationship to natural hazard prevention in Austria, EGUGA, 18, EPSC2016-15245, 2016.
Kelley, S.: New Mexico Temperature-Depth Logs and Graphic Profiles, New Mexico Bureau of Geology & Mineral Resources [data set], http://search.geothermaldata.org/dataset/new-mexico-temperature-depth-logs-and-graphic-profiles (last access: 1 April 2016), 2011.
Lehner, B. and Döll, P.: Development and validation of a global database of lakes, reservoirs and wetlands, J. Hydrol., 296, 1–22, 2004.
Li, J., Sheng, Y., Chen, J., Zhang, B., Wu, J., and Zang, X.: Characteristics of ground temperatures and influencing factors of permafrost development and distribution in the source region of Datong River, Prog. Geogr., 30, 827–836, 2011 (in Chinese, English abstract).
Li, J., Sheng, Y., and Wu, J.: Mapping frozen soil distribution and modeling permafrost stability in the Source Area of the Yellow River, Sci. Geogr. Sin., 36, 588–596, 2016 (in Chinese, English abstract).
Li, X., Jin, H., He, R., Huang, Y., Wang, H., Luo, D., Jin, X., Lv, L., Wang, L., Li, W., Wei, C., Chang, X., Yang, S., and Yu, S.: Effects of forest fires on the permafrost environment in the northern Da Xing'anling (Hinggan) mountains, Northeast China, Permafrost Periglac. Process., 30, 163–177, 2019.
Li, X., Che, T., Li, X., Wang, L., Duan, A., Shangguan, D., Pan, X., Fang, M., and Bao, Q.: CASEarth Poles: Big data for the Three Poles, B. Am. Meteorol. Soc., 101, E1475–E1491, 2020a.
Li, X., Jin, H., Wang, H., Wu, X., Huang, Y., He, R., Luo, D., and Jin, X.: Distributive features of soil carbon and nutrients in permafrost regions affected by forest fires in northern Da Xing'anling (Hinggan) Mountains, NE China, Catena, 185, 104304, https://doi.org/10.1016/j.catena.2019.104304, 2020b.
Liaw, A. and Wiener, M.: Classification and regression by randomForest, R News, 2, 18–22, 2002.
Liu, G. Y., Wang. W., Zhao, L., Chen, J., Pang, Q. Q., Wang, Z. W., and Du, E. J.: Using transient electromagnetic method to sound permafrost depth in the West Kunlun Mountains, J. Glaciol. Geocryol., 37, 38–48, 2015 (in Chinese, English abstract).
Luo, D., Wu, Q., Jin, H., Marchenko, S. S., Lü, L., and Gao, S.: Recent changes in the active layer thickness across the northern hemisphere, Environ. Earth Sci., 75, 555, https://doi.org/10.1007/s12665-015-5229-2, 2016.
Luo, D., Jin, H., Jin, X., He, R., Li, X., Muskett, R. R., and Romanovsky, V. E.: Elevation-dependent thermal regime and dynamics of frozen ground in the Bayan Har Mountains, northeastern Qinghai-Tibet Plateau, southwest China, Permafrost Periglac. Process., 29, 257–270, 2018a.
Luo, D., Jin, H., Wu, Q., Bense, V. F., He, R., Ma, Q., and Lü, L.: Thermal regime of warm-dry permafrost in relation to ground surface temperature in the Source Areas of the Yangtze and Yellow rivers on the Qinghai-Tibet Plateau, SW China, Sci. Total Environ., 618, 1033–1045, 2018b.
Luo, D. L.: Monitoring, mapping and modeling of permafrost and active layer processes in the Sources Areas of the Yellow River (SAYR) on Northeastern Qinghai-Tibet Plateau, PhD thesis, Univ. Chinese Acad. Sci., 1–119, 2012 (in Chinese, English abstract).
Maine Geological Survey: Maine Well Headers, National Geothermal Data System [data set], http://search.geothermaldata.org/dataset/maine-well-headers (last access: 16 April 2016), 2014.
Mair, V., Zischg, A., Lang, K., Tonidandel, D., Krainer, K., Kellerer-Pirklbauer, A., Deline, P., Schoeneich, P., Cremonese, E., Pogliotti, P., Gruber, S., and Böckli, L.: PermaNET-Permafrost Long-term Monitoring Network: Synthesis report, Int. Res. Soc. INTERPRAEVENT, 2011.
Nelson, F. E.: Permafrost zonation in eastern Canada: a review of published maps, Phys. Geogr., 10, 231–246, 1989.
Nevada Bureau of Mines and Geology: Nevada Borehole Temperatures, National Geothermal Data System [data set], http://search.geothermaldata.org/dataset/nevada-borehole-temperatures (last access: 20 April 2016), 2014.
Niewendorp, C. A.: Oregon Well Logs, Oregon Department of Geology and Mineral Industries [data set], http://search.geothermaldata.org/dataset/oregon-well-logs (last access: 11 April 2016), 2012.
Noetzli, J., Gruber, S., Kohl, T., Salzmann, N., and Haeberli, W.: Three-dimensional distribution and evolution of permafrost temperatures in idealized high-mountain topography, J. Geophys. Res., 112, F02S13, https://doi.org/10.1029/2006JF000545, 2017.
NSF Arctic Data Center: Network of Permafrost Observatories in Western Alaska, Arctic Data Center [data set], https://doi.org/10.18739/A2D934, 2014.
Obu, J., Westermann, S., Bartsch, A., Berdnikov, N., Christiansen, H. H., Dashtseren, A., Delaloye, R., Elberling, B., Etzelmüller, B., Kholodov, A., Khomutov, A., Kääb, A., Leibman, M. O., Lewkowicz, A. G., Panda, S. K., Romanovsky, V., Way, R. G., Westergaard-Nielsen, A., Wu, T., Yamkhin, J., and Zou, D.: Northern Hemisphere permafrost map based on TTOP modelling for 2000–2016 at 1 km2 scale, Earth-Sci. Rev., 193, 299–316, 2019.
Obu, J., Westermann, S., Barboux, C., Bartsch, A., Delaloye, R., Grosse, G., Heim, B., Hugelius, G., Irrgang, A., Kääb, A. M., Kroisleitner, C., Matthes, H., Nitze, I., Pellet, C., Seifert, F. M., Strozzi, T., Wegmüller, U., Wieczorek, M., and Wiesmann, A.: ESA Permafrost Climate Change Initiative (Permafrost_cci): Permafrost Ground Temperature for the Northern Hemisphere, v3.0, NERC EDS Centre for Environmental Data Analysis, https://doi.org/10.5285/b25d4a6174de4ac78000d034f500a268, 2021.
Ødegård, R. S., Isaksen, K., Eiken, T., and Sollid, J. L.: MAGST in Mountain Permafrost, Dovrefjell, Southern Norway, 2001–2006, Ninth International Conference on Permafrost, University of Alaska Fairbanks, USA, in: Proceedings Volume 2, edited by: Kane D. L. and Hinkel, K. M., Institute of Northern Engineering, University of Alaska Fairbanks, 1311–1315, ISBN 978-0-9800179-3-9, 2008.
Paetzhold, R. F.: Monthly Summaries of Soil Temperature and Soil Moisture at Sites in China, Boulder, Colorado USA, NSIDC: National Snow and Ice Data Center [data set], https://doi.org/10.7265/67qf-zj58, 2003.
Pan, H. L. and Mahrt, L.: Interaction between soil hydrology and boundary-layer development, Bound. Lay. Meteorol., 38, 185–202, 1987.
Peter, M.: Modeling of permafrost temperatures in the Lena River Delta, Siberia, based on remote sensing products, MS thesis, University of Leipzig, hdl: 10013/epic.45589.d001, https://epic.awi.de/id/eprint/38041/ (last access: 28 July 2019), 2015.
Qiao, Y., Zhao, L., Pang, Q., Chen, J., Zou, D., and Gao, Z.: Characteristics of permafrost in Gerze county on the Tibetan Plateau, J. Glaciol. Geocryol., 37, 1453–1460, 2015 (in Chinese, English abstract).
Qin, Y., Wu, T., Zhao, L., Wu, X., Li, R., Xie, C., and Liu, G.: Numerical modeling of the active layer thickness and permafrost thermal state across Qinghai-Tibetan Plateau, J. Geophys. Res.-Atmos., 122, 11–604, 2017.
Ran, Y., Li, X., Cheng, G., Zhang, T., Wu, Q., Jin, H., and Jin, R.: Distribution of permafrost in China: an overview of existing permafrost maps, Permafrost Periglac. Process., 23, 322–333, 2012.
Ran, Y., Li, X., and Cheng, G.: Climate warming over the past half century has led to thermal degradation of permafrost on the Qinghai–Tibet Plateau, The Cryosphere, 12, 595–608, https://doi.org/10.5194/tc-12-595-2018, 2018.
Ran, Y., Li, X., Cheng, G., Che, J., Aalto, J., Karjalainen, O., Hjort, J., Luoto, M., Jin, H., Obu, J., Hori, M., Yu, Q., and Chang, X.: High-resolution datasets of permafrost thermal state and hydrothermal zonation in the Northern Hemisphere, National Tibetan Plateau Data Center [data set], https://doi.org/10.11888/Geocry.tpdc.271190, 2021a.
Ran, Y., Li, X., Cheng, G., Nan, Z., Che, J., Sheng, Y., Wu, Q., Jin, H., Luo, D., Tang, Z., and Wu, X.: Mapping the permafrost stability on the Tibetan Plateau for 2005–2015, Sci. China: Earth Sci., 64, 62–79, 2021b.
Riseborough, D.: The effect of transient conditions on an equilibrium permafrost-climate model, Permafrost Periglac. Process., 18, 21–32, 2007.
Riseborough, D., Shiklomanov, N., Etzelmüller, B., Gruber, S., and Marchenko, S.: Recent advances in permafrost modelling, Permafrost Periglac. Process., 19, 137–156, 2008.
Romanovsky, V. E. and Osterkamp, T. E.: Permafrost monitoring system in Alaska: structure and results, Kriosfera Zemli, 5, 59–68, 2001 (in Russian).
Romanovsky, V. E., Smith, S. L., and Christiansen, H. H.: Permafrost thermal state in the polar Northern Hemisphere during the international polar year 2007–2009: a synthesis, Permafrost Periglac. Process., 21, 106–116, 2010.
Schuur, E. A., Vogel, J. G., Crummer, K. G., Lee, H., Sickman, J. O., and Osterkamp, T. E.: The effect of permafrost thaw on old carbon release and net carbon exchange from tundra, Nature, 459, 556–559, 2009.
Sheng, Y., Li, J., Wu, J. C., Ye, B. S., and Wang, J.: Distribution patterns of permafrost in the upper area of Shule River with the application of GIS technique, J. China Univ. Min. Technol., 39, 32–39, 2010.
Sherstiukov, A.: Dataset of daily soil temperature up to 320 cm depth based on meteorological stations of Russian Federation, RIHMI-WDC, 176, 224–232, 2012.
Shur, Y. L. and Jorgenson, M. T.: Patterns of permafrost formation and degradation in relation to climate and ecosystems, Permafrost Periglac. Process., 18, 7–19, 2007.
Smith, S. L. and Ednie, M.: Ground thermal data collection along the Alaska Highway easement (KP 1559-1895) Yukon, summer 2014, Geological Survey of Canada [data set], Open File 7762, https://doi.org/10.4095/295974, 2015.
Smith, S. L., Burgess, M. M., Riseborough, D., and Mark Nixon, F.: Recent trends from Canadian permafrost thermal monitoring network sites, Permafrost Periglac. Process., 16, 19–30, 2005.
Smith, S. L., Romanovsky, V. E., Lewkowicz, A. G., Burn, C. R., Allard, M., Clow, G. D., Yoshikawa, K., and Throop, J.: Thermal state of permafrost in North America: a contribution to the international polar year, Permafrost Periglac. Process., 21, 117–135, 2010.
Smith, S. L., Riseborough, D. W., Ednie, M., and Chartrand, J.: A Map and Summary Database of Permafrost Temperatures in Nunavut, Canada, Geological Survey of Canada [data set], Open File 7393, https://doi.org/10.4095/292615, 2013.
Streletskiy, D. A., Tananaev, N. I., Opel, T., Shiklomanov, N. I., Nyland, K. E., Streletskaya, I. D., and Shiklomanov, A. I.: Permafrost hydrology in changing climatic conditions: seasonal variability of stable isotope composition in rivers in discontinuous permafrost, Environ. Res. Lett., 10, 095003, https://doi.org/10.1088/1748-9326/10/9/095003, 2015.
Sun, Z. Z., Ma, W., Dang, H. M., Yun, H. B., and Wu, G. L.: Characteristics and causes of embankment deformation for Qinghai-Tibet Railway in permafrost regions, Rock Soil Mech., 34, 2667–2671, 2013 (in Chinese, English abstract).
Tarnocai, C., Canadell, J. G., Schuur, E. A. G., Kuhry, P., Mazhitova, G., and Zimov, S.: Soil organic carbon pools in the northern circumpolar permafrost region, Global Biogeochem. Cy., 23, https://doi.org/10.1029/2008GB003327, 2009.
Trabucco, A. and Zomer, R.: Global Aridity Index and Potential Evapotranspiration (ET0) Climate Database v2, figshare [data set], https://doi.org/10.6084/m9.figshare.7504448.v3, 2019.
Trenberth, K. E. and Shea, D. J.: Relationships between precipitation and surface temperature, Geophys. Res. Lett., 32, https://doi.org/10.1029/2005GL022760, 2005.
UNEP (United Nations Environment Programme), World atlas of desertification 2ED, Arnold, London, 182, ISBN 0340691662, 1997.
University of North Dakota: Temperature at Depth Database, NGDS [data set], http://geothermal.smu.edu/static/DownloadFilesButtonPage.htm (last access: 15 May 2016), 2014.
Van Everdingen, R. O.: Multi-Language Glossary of Permafrost and Related Ground-Ice Terms, International Permafrost Association, https://globalcryospherewatch.org/reference/glossary_docs/Glossary_of_Permafrost_and_Ground-Ice_IPA_2005.pdf (last access: 2 April 2019), 2005.
Vapnik, V.: The Nature of Statistical Learning Theory, https://doi.org/10.1007/978-1-4757-2440-0, Springer, NY, 1995.
Virginia Division of Geology and Mineral Resources: Georgia Well Logs, NGDS [data set], http://search.geothermaldata.org/dataset/georgia-well-logs (last access: 11 May 2016), 2012a.
Virginia Division of Geology and Mineral Resources: Virginia Well Logs, NGDS [data set], http://search.geothermaldata.org/dataset/virginia-well-logs (last access: 11 May 2016), 2012b.
Wang, Q., Zhang, T., Wu, J., Peng, X., Zhong, X., Mu, C., and Cheng, G.: Investigation on permafrost distribution over the upper reaches of the Heihe River in the Qilian Mountains, J. Glaciol. Geocryol., 35, 19–25, 2013 (in Chinese, English abstract).
Wani, J. M., Thayyen, R. J., Gruber, S., Ojha, C. S. P., and Stumm, D.: Single-year thermal regime and inferred permafrost occurrence in the upper Ganglass catchment of the cold-arid Himalaya, Ladakh, India, Sci. Total Environ., 703, 134631, https://doi.org/10.1016/j.scitotenv.2019.134631, 2020.
Wolfe, S. A., Smith, S. L., Chartrand, J., Kokelj, S., Palmer, M., and Stevens, C. W.: Geotechnical Database and Descriptions of Permafrost Monitoring Sites Established 2006–10 in the Northern Mackenzie Corridor, Northwest Territories, Geological Survey of Canada [data set], Open File 6677, https://doi.org/10.4095/287167, 2010.
Wood, S. N.: Fast stable restricted maximum likelihood and marginal likelihood estimation of semiparametric generalized linear models, J. R. Stat. Soc. Ser. B, 73, 3–36, 2011.
Wu, J., Yu, S., Yu, H., and Li, J.: Permafrost in the middleeast section of Qilian Mountains (II): Characters of permafrost, J. Glaciol. Geocryol., 29, 426–432, 2007 (in Chinese with English abstract).
Wu, Q., Zhu, Y., and Liu, Y.: Application of the permafrost table temperature and thermal offset forecast model in the Tibetan Plateau, J. Glaciol. Geocryol., 24, 614–617, 2002 (in Chinese with English abstract).
Wu, Q., Hou, Y., Yun, H., and Liu, Y.: Changes in active-layer thickness and near-surface permafrost between 2002 and 2012 in alpine ecosystems, Qinghai–Xizang (Tibet) Plateau, China, Glob. Planet. Change, 124, 149–155, 2015.
Xiang, Y., Xiao, Z. Q., Ling, S. L., Wang, J. D., and Song, J. L.: Validation of Global LAnd Surface Satellite (GLASS) leaf area index product, Int. J. Remote Sens., 18, 573–584, 2014.
Xiao, Z., Liang, S., Wang, J., Chen, P., Yin, X., Zhang, L., and Song, J.: Use of general regression neural networks for generating the GLASS leaf area index product from time-series MODIS surface reflectance, IEEE T. Geosci. Remote, 52, 209–223, 2014.
Xie, Y. L., Yu, Q. H., You, Y. H., Zhang, Z. Q., and Gou, T. T.: The changing process and trend of ground temperature around tower foundations of Qinghai-Tibet Power Transmission line, Sci. Cold Arid Reg., 11, 13–20, 2019.
Yoshikawa, K. and Hinzman, L. D.: Shrinking thermokarst ponds and groundwater dynamics in discontinuous permafrost near Council, Alaska, Permafrost Periglac. Process., 14, 151–160, 2003.
Yu, H., Wu, Q. B., and Liu, Y. Z.: The long-term monitoring system on permafrost regions along the Qinghai-Tibet Railway, J. Glaciol. Geocryol., 30, 475–481, 2008 (in Chinese, English abstract).
Yu, Q., You, Y., Yan, H., and Liu X.: Distribution and characteristics of permafrost in Nalati Mountain, western Tianshan Mountains in China, J. Glaciol. Geocryol., 35, 10–18, 2013 (in Chinese, English abstract).
Zhang, T., Heginbottom, J. A., Barry, R. G., and Brown, J.: Further statistics on the distribution of permafrost and ground ice in the Northern Hemisphere, Polar Geogr., 24, 126–131, 2000.
Zhang, T., Barry, R. G., Knowles, K., Heginbottom, J. A., and Brown, J.: Statistics and characteristics of permafrost and ground-ice distribution in the Northern Hemisphere, Polar Geogr., 31, 47–68, 2008.
Zhang, X. M., Nan, Z. T., Wu, J. C., Du, E. J., Wang, T., and You, Y. H.: Modeling permafrost distribution in Wenquan Area over Qinghai-Tibet Plateau by using multivariate adaptive regression splines, J. Glaciol. Geocryol., 33, 1088–1097, 2011 (in Chinese, English abstract).
Zhao, J., Luo, T., Li, R., Wei, H., Li, X., Du, M., and Tang, Y.: Precipitation alters temperature effects on ecosystem respiration in Tibetan alpine meadows, Agric. For. Meteorol., 252, 121–129, 2018.
Zhao, L., Zou, D., Hu, G., Wu, T., Du, E., Liu, G., Xiao, Y., Li, R., Pang, Q., Qiao, Y., Wu, X., Sun, Z., Xing, Z., Sheng, Y., Zhao, Y., Shi, J., Xie, C., Wang, L., Wang, C., and Cheng, G.: A synthesis dataset of permafrost thermal state for the Qinghai–Tibet (Xizang) Plateau, China, Earth Syst. Sci. Data, 13, 4207–4218, https://doi.org/10.5194/essd-13-4207-2021, 2021.
Zhao, L., Liu, G. Y., Jiao, K. Q., Li, R., Qiao, Y. P., and Ping, C. L.: Variation of the permafrost in the Headwaters of the Urumqi River in the Tianshan Mountains since 1991, J. Glaciol. Geocryol., 32, 223–230, 2010a.
Zhao, L., Wu, Q., Marchenko, S. S., and Sharkhuu, N.: Thermal state of permafrost and active layer in Central Asia during the International Polar Year, Permafrost Periglac. Process., 21, 198–207, 2010b.
Zhao, S. P., Nan, Z. T., Huang, Y. B., and Zhao, L.: The application and evaluation of simple permafrost distribution models on the Qinghai–Tibet Plateau, Permafrost Periglac. Process., 28, 391–404, 2017.
Zhao, X., Liang, S., Liu, S., Yuan, W., Xiao, Z., Liu, Q., Cheng, J., Zhang, X., Tang, H., Zhang, X., Liu, Q., Zhou, G., Xu, S., and Yu, K.: The Global Land Surface Satellite (GLASS) remote sensing data processing system and products, Remote Sens., 5, 2436–2450, 2013.
Zhelezniak, M., Wu, Q. B., Kirillin, A., Wen, Z., Zhirkov, A., and Zhizhin, W. Permafrost distribution and temperature in the Elkon Horst, Russia, Sci. Cold Arid Reg., 13, 107–122, 2021.
- Abstract
- Introduction
- Materials and methods
- Results and discussion
- Data availability
- Conclusions
- Appendix A: List of mean annual ground temperature (MAGT) ground measurement data sources
- Author contributions
- Competing interests
- Disclaimer
- Special issue statement
- Acknowledgements
- Financial support
- Review statement
- References
- Abstract
- Introduction
- Materials and methods
- Results and discussion
- Data availability
- Conclusions
- Appendix A: List of mean annual ground temperature (MAGT) ground measurement data sources
- Author contributions
- Competing interests
- Disclaimer
- Special issue statement
- Acknowledgements
- Financial support
- Review statement
- References