the Creative Commons Attribution 4.0 License.
the Creative Commons Attribution 4.0 License.
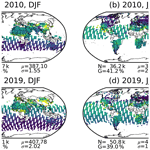
An 11-year record of XCO2 estimates derived from GOSAT measurements using the NASA ACOS version 9 retrieval algorithm
Thomas E. Taylor
Christopher W. O'Dell
David Crisp
Akhiko Kuze
Hannakaisa Lindqvist
Paul O. Wennberg
Abhishek Chatterjee
Michael Gunson
Annmarie Eldering
Brendan Fisher
Matthäus Kiel
Robert R. Nelson
Aronne Merrelli
Greg Osterman
Frédéric Chevallier
Paul I. Palmer
Liang Feng
Nicholas M. Deutscher
Manvendra K. Dubey
Dietrich G. Feist
Omaira E. García
David W. T. Griffith
Frank Hase
Laura T. Iraci
Rigel Kivi
Cheng Liu
Martine De Mazière
Isamu Morino
Justus Notholt
Young-Suk Oh
Hirofumi Ohyama
David F. Pollard
Markus Rettinger
Matthias Schneider
Coleen M. Roehl
Mahesh Kumar Sha
Kei Shiomi
Kimberly Strong
Ralf Sussmann
Voltaire A. Velazco
Mihalis Vrekoussis
Thorsten Warneke
Debra Wunch
The Thermal And Near infrared Sensor for carbon Observation – Fourier Transform Spectrometer (TANSO-FTS) on the Japanese Greenhouse gases Observing SATellite (GOSAT) has been returning data since April 2009. The version 9 (v9) Atmospheric Carbon Observations from Space (ACOS) Level 2 Full Physics (L2FP) retrieval algorithm (Kiel et al., 2019) was used to derive estimates of carbon dioxide (CO2) dry air mole fraction (XCO2) from the TANSO-FTS measurements collected over its first 11 years of operation. The bias correction and quality filtering of the L2FP XCO2 product were evaluated using estimates derived from the Total Carbon Column Observing Network (TCCON) as well as values simulated from a suite of global atmospheric inversion systems (models) which do not assimilate satellite-derived CO2. In addition, the v9 ACOS GOSAT XCO2 results were compared with collocated XCO2 estimates derived from NASA's Orbiting Carbon Observatory-2 (OCO-2), using the version 10 (v10) ACOS L2FP algorithm.
These tests indicate that the v9 ACOS GOSAT XCO2 product has improved throughput, scatter, and bias, when compared to the earlier v7.3 ACOS GOSAT product, which extended through mid 2016. Of the 37 million soundings collected by GOSAT through June 2020, approximately 20 % were selected for processing by the v9 L2FP algorithm after screening for clouds and other artifacts. After post-processing, 5.4 % of the soundings (2×106 out of 37×106) were assigned a “good” XCO2 quality flag, as compared to 3.9 % in v7.3 (<1 ×106 out of 24×106). After quality filtering and bias correction, the differences in XCO2 between ACOS GOSAT v9 and both TCCON and models have a scatter (1σ) of approximately 1 ppm for ocean-glint observations and 1 to 1.5 ppm for land observations. Global mean biases against TCCON and models are less than approximately 0.2 ppm. Seasonal mean biases relative to the v10 OCO-2 XCO2 product are of the order of 0.1 ppm for observations over land. However, for ocean-glint observations, seasonal mean biases relative to OCO-2 range from 0.2 to 0.6 ppm, with substantial variation in time and latitude.
The ACOS GOSAT v9 XCO2 data are available on the NASA Goddard Earth Science Data and Information Services Center (GES-DISC) in both the per-orbit full format (https://doi.org/10.5067/OSGTIL9OV0PN, OCO-2 Science Team et al., 2019b) and in the per-day lite format (https://doi.org/10.5067/VWSABTO7ZII4, OCO-2 Science Team et al., 2019a). In addition, a new set of monthly super-lite files, containing only the most essential variables for each satellite observation, has been generated to provide entry level users with a light-weight satellite product for initial exploration (CaltechDATA, https://doi.org/10.22002/D1.2178, Eldering, 2021). The v9 ACOS Data User's Guide (DUG) describes best-use practices for the GOSAT data (O'Dell et al., 2020). The GOSAT v9 data set should be especially useful for studies of carbon cycle phenomena that span a full decade or more and may serve as a useful complement to the shorter OCO-2 v10 data set, which begins in September 2014.
- Article
(17347 KB) - Full-text XML
- BibTeX
- EndNote
A new era of dedicated satellite observations of greenhouse gases began in 2009, with the successful launch of GOSAT (Kuze et al., 2009). Each day, GOSAT's Thermal And Near infrared Sensor for carbon Observation – Fourier Transform Spectrometer (TANSO-FTS) acquires approximately 10 thousand high-spectral-resolution measurements of reflected sunlight (≃ 36.5×106 in 10 years). Soundings that are determined to be sufficiently clear of clouds and aerosols are processed by retrieval algorithms to produce estimates of XCO2. Both the quality of the GOSAT TANSO-FTS spectra and the derived XCO2 estimates have been continually refined over the past 12 years. While the official GOSAT L2 products are available from the National Institute for Environmental Studies (NIES; http://www.gosat.nies.go.jp/en/about_5_products.html, last access: 10 January 2022; Yoshida et al., 2013) a number of independent research institutes have developed their own products (e.g., Butz et al., 2011; Crisp et al., 2012; Cogan et al., 2012; Heymann et al., 2015).
One of these groups, the Atmospheric CO2 Observations from Space (ACOS) team, used a Level 2 Full Physics (L2FP) retrieval algorithm developed for the NASA Orbiting Carbon Observatory (OCO) to derive estimates of XCO2 from the GOSAT data (O'Dell et al., 2012; Crisp et al., 2012). Early XCO2 estimates from these efforts had large biases and random errors when compared to XCO2 estimates from the Total Carbon Column Observing Network (TCCON) and other standards. For example, the v2.8 ACOS GOSAT L2FP product had biases of 7 to 8 ppm relative to TCCON (Crisp et al., 2012). These biases were reduced to 1–2 ppm in the v2.9 product. The next major release was v3.5 in 2014, which spanned approximately 4 years. This data product showed additional reductions in bias and scatter against TCCON, as well as reasonable agreement in seasonal cycle phase and amplitude (Lindqvist et al., 2015; Kulawik et al., 2016).
These early space-based XCO2 products were rapidly adopted by the carbon cycle science community. Early studies based on GOSAT ACOS retrievals included Basu et al. (2013), Deng et al. (2014), Chevallier et al. (2014), and Feng et al. (2016). These studies provided the first comprehensive insights into regional flux estimates from space-based observations of carbon dioxide. Houweling et al. (2015) conducted an extensive inter-comparison of the early GOSAT-based atmospheric inversion system studies and reported a reduction in the global land sink for CO2 and a shift in the terrestrial net uptake of carbon from the tropics to the extratropics. However, these studies also highlighted the role of spatiotemporal systematic errors in the satellite retrievals and the negative impact they can have on estimation of CO2 sources and sinks using atmospheric inversion systems.
Motivated by these early studies, as well as the launch of the OCO-2 sensor in July 2014, the ACOS team continued to refine the L2FP retrieval algorithm. In 2016, the ACOS GOSAT v7.3 product was distributed. No formal results of the XCO2 estimates were published by the algorithm team, although internal analysis showed small improvement over v3.5, as well as an extension of the record to 7 years. A number of atmospheric inversion studies were published using the v7.3 product. For example, Chatterjee et al. (2017) and Liu et al. (2017) used v7.3 to define the climatological background in their studies of the impact of the 2015–2016 El Niño on the tropical carbon cycle. Palmer et al. (2019) used this data product in a global study, concluding that the tropical land regions were a net annual source of CO2 emissions, including unexpectedly large net emissions from northern tropical Africa. Wang et al. (2019) found that the ACOS GOSAT v7.3 XCO2 yielded a stronger carbon land sink than the v7 OCO-2 product. Byrne et al. (2020) used the ACOS GOSAT 7.3 product to study interannual variability in the carbon cycle across North America, and Jiang et al. (2021) investigated interannual variability of the carbon cycle across the globe with v7.3.
Most recently, the v9 ACOS L2FP retrieval algorithm, first applied to OCO-2 (Kiel et al., 2019), was used to generate estimates of XCO2 from an 11-year record of GOSAT measurements, spanning April 2009 through June 2020. This both extends the time record over v7.3 and produces an ACOS GOSAT product that is more directly comparable to the newest OCO-2 product, which is now using version 10.
The paper is organized as follows: Sect. 2 discusses the GOSAT TANSO-FTS instrument and measurements as related to the ACOS XCO2 estimates. In Sect. 3, updates to the ACOS v9 L2FP algorithm are detailed, and an assessment is given of the v9 XCO2 data product volume. The XCO2 quality filtering and bias correction procedures, specific to ACOS GOSAT v9, are also discussed. Section 4 provides an evaluation of the v9 XCO2 product using estimates of XCO2 from TCCON and from a suite of four atmospheric inversion systems (models). In addition, a comparison to collocated XCO2 estimates derived from NASA's OCO-2 sensor is presented. A summary of the results is provided in Sect. 6.
The GOSAT mission is a joint project between the Japan Aerospace Exploration Agency (JAXA), the National Institute for Environmental Studies (NIES), and the Ministry of the Environment (MOE) (Kuze et al., 2009). GOSAT was launched on 23 January 2009 into a sun-synchronous orbit with a local overpass time of approximately 12:49 and a 3 d ground repeat cycle. Its TANSO-FTS collects high-resolution spectra of reflected sunlight that can be analyzed to yield estimates of carbon dioxide (CO2) (Yoshida et al., 2011, 2013).
2.1 GOSAT TANSO-FTS instrument
TANSO-FTS collects high-resolution spectra of reflected sunlight in the near-infrared (NIR) and shortwave-infrared (SWIR) spectral ranges that include the oxygen A-band near 0.76 µm (ABO2 band) at approximately 0.36 cm−1 spectral resolution, and weak and strong CO2 absorption features near 1.6 µm (WCO2 band) and 2.0 µm (SCO2 band), respectively, at 0.27 cm−1 spectral resolution. All three channels simultaneously measure two orthogonal components of polarization approximately every 4.6 s.
Each GOSAT sounding has a circular ground footprint with a diameter of approximately 10.5 km when viewing the local nadir. An agile, two-axis pointing system allows cross-track and along-track motions of ± 35∘ and ± 20∘, respectively. Before August 2010, a five-point cross-track scan was used, yielding footprints that were separated by approximately 150 km in both the down-track and along-track dimensions. Since that time, a three-point cross-track scan has been used, yielding footprint separation of approximately 260 km (Kuze et al., 2016).
Over water, the TANSO-FTS scan mechanism targets the field of view to collect observations in the direction of the local glint spot, where sunlight is specularly reflected from the surface. Early in the mission, glint observations were collected only within ± 20∘ of the sub-solar latitude. In May 2013, to increase the latitudinal extent of the GOSAT ocean measurements, the scanning strategy was improved to better track the actual specular glint spot, which varies by latitude and season. The latitude range for glint observation was further extended three times in increments of 3∘ in September 2014, June 2015, and January 2016, by not only tracking the exact specular point but also tracking along the principal plane of the specular reflection when the glint spot was out of range of the scan mechanism. In addition, more observations over fossil fuel emission target sites such as mega-cities and power plants have been made in recent years, allowing for detailed emission source studies (e.g., Kuze et al., 2020). Daily observation patterns can be found at https://www.eorc.jaxa.jp/GOSAT/currentStatus_10.html (last access: 10 January 2022).
The TANSO-FTS detectors can be read out using independent medium-gain and high-gain signal chains. Most measurements over land use the instrument's high-gain signal chain (H-gain), while brighter land surfaces are measured using the medium-gain signal chain (M-gain) to avoid saturating the detectors. Over oceans, which appear dark in the SWIR spectral bands, measurements are collected using the high-gain signal chain to maximize the signal.
During the first 7 years of GOSAT operations (2009–2015), data acquisition was temporarily suspended due to one spacecraft and two instrument anomalies, as highlighted in Kuze et al. (2016). A rotation failure of a solar paddle in 2014 resulted in a data loss of 6 d. A switch from the primary to secondary pointing mirror in January 2015 resulted in a data loss of approximately 6 weeks, while a temporary shutdown of the cryocooler in August 2015 resulted in a data loss of 13 d.
Since 2015, three additional anomalies interrupted data acquisition. An unexpected shutdown of the instrument occurred in May 2018, resulting in the loss of a week of data. A failure of the second solar panel caused a significant loss of data spanning more than a month in November and December of 2018, and an anomaly of the FTS alignment laser caused a loss of a week of data in June of 2020. In all these cases, the system was able to recover full functionality either through utilization of on-board back-up systems, or through mitigation strategies, and as of the summer of 2021, TANSO-FTS continues to collect science data.
2.2 ACOS GOSAT v9 L1b measurements
The JAXA L1b algorithm, which has been updated more than 10 times over the 11-year data record, produces an internally consistent set of geometrically, radiometrically, and spectrally calibrated TANSO-FTS radiances. The raw spectral measurements are interferograms, which are calibrated and Fourier transformed to yield spectra. The version 205/210 Level 1b (L1b) geolocated and calibrated radiances provided by JAXA have been used for the ACOS v9 reprocessing. A list of L1b updates for v205/210 can be found in Table 3 of the ACOS v9 Data Users Guide (DUG) (O'Dell et al., 2020). Note that while the current L1b version is now 230, the only differences between this version and 205/210 are in the thermal infrared band (5.6–14.3 µm), which is not used in the ACOS XCO2 retrieval.
After obtaining the calibrated L1b product from JAXA, the ACOS team converts the files to the format needed as input to the ACOS L2 algorithms. The L2FP algorithm uses a simple average of the S and P linear polarizations to produce an approximation of the total measured intensity. Due to cooperation agreements between JAXA and the California Institute of Technology, the distribution of the ACOS GOSAT L1b product is restricted and therefore not publicly available on the NASA DISC. However, the data may be procured by submitting a request to the GOSAT project.
The ACOS Level 2 full physics (L2FP) retrieval algorithm is well documented, most recently in O'Dell et al. (2018) for v8 and in Kiel et al. (2019) for v9. A Bayesian optimal estimation framework is used to derive estimates of XCO2 from spectral measurements of reflected solar radiation. A post-processing step assigns a simple good/bad quality flag (QF) to each XCO2 value based on successful L2FP algorithm convergence and a series of empirically derived filters. An empirical bias correction (BC) to the estimated XCO2 values, derived from comparisons with TCCON-derived XCO2 and CO2 fields from a suite of atmospheric inversion systems, is included in the Lite File product. Here we provide a summary of the recent evolution of the ACOS algorithm and discuss retrieval parameters and setup specific to GOSAT.
3.1 ACOS L2FP algorithm updates
Table 1 summarizes the evolution of the ACOS L2FP retrieval algorithm from v7 to v10. A similar table, complete through v8, can be found in O'Dell et al. (2018). The trace gas absorption coefficient tables (ABSCO) were updated from v4.2 (Thompson et al., 2012) in ACOS v7 to ABSCO v5.0 (Oyafuso et al., 2017) in ACOS v8/9. The ACOS v9 L2FP algorithm is unmodified relative to v8 (Kiel et al., 2019). However, changes were made in v9 regarding the sampling of the meteorological prior, which does affect ACOS GOSAT estimates of XCO2. The source of the prior meteorology was switched from the European Center for Medium-range Weather Forecast (ECMWF) in ACOS v7, to the NASA Goddard Modeling and Assimilation Office (GMAO) Goddard Earth Observing System (GEOS) Forward Processing – Instrument Team (FP-IT) product for ACOS v8/9. Both v7 and v8/9 used aerosol priors based on a simple monthly 1∘ latitude by 1∘ longitude climatology constructed from the output aerosol fields of the GMAO Modern-Era Retrospective analysis for Research and Applications (MERRA) product (Rienecker et al., 2011). However, between v7 and v8/9, an additional stratospheric aerosol layer was introduced, as described in Sect. 3.1.1 of O'Dell et al. (2018). In addition, the prior value of the aerosol optical depth (AOD) for each retrieved aerosol type was lowered from 0.0375 in ACOS v7 to 0.0125 in ACOS v8/9 based on extensive testing. There was no change in the source of the CO2 prior from ACOS v7 to v8/9; both versions adopted the prior developed by the TCCON team for use in the ggg2014 algorithm (Wunch et al., 2015). An additional change from ACOS v7 to v8/9 was a switch from a purely Lambertian land surface model to a more sophisticated bi-directional reflectance distribution function (BRDF) model.
Several important components of the v9 ACOS L2FP retrieval configured for GOSAT have not changed from v7.3: (i) the surface pressure prior constraint remains set at ±2 hPa, (ii) three empirical orthogonal functions (EOFs) are fit in each spectral band (see Sect. 3.3 in O'Dell et al., 2018 for a full discussion of ACOS EOFs), and (iii) a zero level offset (ZLO) is fit in the state vector to account for non-linearity in the ABO2 signal chain on GOSAT TANSO-FTS (Crisp et al., 2012).
To support comparisons of the ACOS GOSAT v9 XCO2 product with the OCO-2 v10 product, Table 1 includes the most recent updates to the ACOS v10 L2FP algorithm. For v10, the ABSCO tables were again updated from v5.0 to v5.1 (Payne et al., 2020). The aerosol prior was updated from the MERRA monthly climatology to daily GEOS-FT-IT values, with a tightened prior uncertainty (Nelson and O'Dell, 2019). Finally, the CO2 priors developed by the TCCON team for use in ggg2014 were updated to a revised set of priors developed for use in ggg2020.
3.2 ACOS GOSAT v9 L2FP sounding selection and convergence
GOSAT data from 20 April 2009 through 30 June 2020 were passed through the ACOS L2FP algorithm pipeline, which includes a series of stages where soundings can be rejected or selected for further processing. The throughput of each of these stages for ACOS GOSAT v9 is summarized in Table 2 and Fig. 1. The pipeline begins with a series of preprocessing steps, which reject corrupted spectra and screen the remainder to eliminate those with optically thick clouds and/or aerosols (Taylor et al., 2016). From the full set of measurements (panel a of Fig. 1), the remaining soundings are accepted by the L2FP algorithm (18.8 % of the 37.4×106 measured soundings contained in the ACOS GOSAT v9 record) (panel b of Fig. 1) and a retrieval of XCO2 is attempted. The majority of the selected soundings successfully converge to a valid solution: 87 % for ACOS GOSAT v9 (16.4 % of the total measured soundings). Soundings can fail to converge for a variety of reasons, including (i) producing non-physical values, such as negative gas mixing ratios or surface pressures (3.9 % of the selected), (ii) converging too slowly and exceeding a predefined number of iterations (3.2 % of the selected), or (iii) having more diverging steps than the predefined maximum (5.9 % of the selected). The 6.1×106 valid soundings were then run through the quality filtering and bias correction procedure discussed in the next section.
Table 2Accounting of the soundings in the 11-year-long GOSAT ACOS v9 data set at each stage of the data processing chain. The final line summarizes the number of good-quality XCO2 soundings used in the evaluation section of this work.
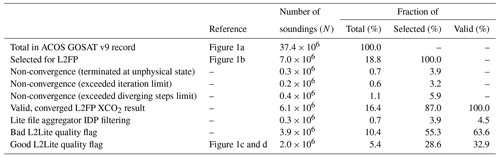
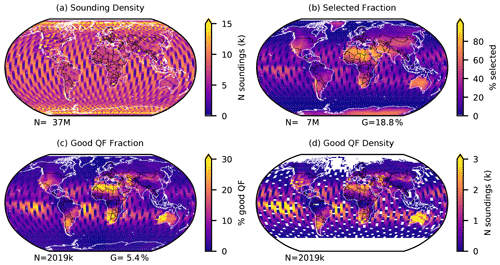
Figure 1The total measured sounding density per 2.5∘ by 5∘ latitude–longitude grid cell in the 11-year (April 2009–June 2020) ACOS GOSAT v9 data record (a). The fraction of the total soundings selected to run through the L2FP algorithm (b). The fraction of the total soundings that converged in the L2FP and were assigned a good L2FP QF (c). The sounding density of the good QF data per 2.5∘ by 5∘ latitude–longitude grid cell (d).
3.3 ACOS GOSAT v9 XCO2 quality filtering and bias correction
All GOSAT soundings that converged to a valid XCO2 value within the L2FP retrieval were input to the quality filtering and bias correction procedure. A modest fraction (4.5 % of the valid soundings) were removed from the final L2Lite product based on screening via the IMAP-DOAS Preprocessor (IDP) CO2 ratio, which indicated the presence of clouds or aerosols. Based on a series of screening criteria derived from comparisons with TCCON and modeled CO2 fields, each sounding that converged within the L2FP is assigned either a “good” (=0) or “bad” (=1) XCO2 quality flag. Generally, for global or regional studies, it is recommended that users retain only the “good” quality soundings, as the soundings flagged as “bad” quality are likely to include biases that compromise their utility for some applications. A global map of the ACOS GOSAT v9 “good” XCO2 sounding density is provided in panels C and D of Fig. 1. A subset of data variables from the per-orbit L2Std files (OCO-2 Science Team et al., 2019b), along with the quality filter flag and bias-corrected XCO2, are repackaged into the daily aggregated L2Lite NetCDF files (OCO-2 Science Team et al., 2019a).
A fundamental aspect of the quality filtering and bias correction procedures (QF/BC) is the need for XCO2 truth metrics with which to compare the satellite-derived estimates (O'Dell et al., 2018). The development of ACOS GOSAT v9 used XCO2 truth metrics derived from both TCCON measurements and the median CO2 distributions determined from a suite of four atmospheric inversion systems, which do not assimilate satellite CO2 measurements.
TCCON is a well-established validation transfer standard for space-based estimates of XCO2 (Wunch et al., 2011a, 2017b). For the ACOS GOSAT v9 QF/BC, estimates of XCO2 derived from TCCON measurements using the ggg2014 retrieval algorithm were used (Wunch et al., 2015). Individual GOSAT soundings were compared to TCCON daily mean XCO2 values. TCCON data were included if (i) they were flagged as good (flag = 0), (ii) they fell within 3 standard deviations of a daily quadratic fit against time (to remove outliers, e.g., due to unscreened cloud), (iii) they covered at least 15 min within a given day, (iv) there were at least three good soundings within the day, and (v) the standard deviation of the good soundings for the day was less than 3 ppm. In the GOSAT-TCCON comparisons described here, an averaging kernel correction was applied to each TCCON XCO2 estimate following Nguyen et al. (2014), prior to calculating the daily mean value.
Following the criteria defined in Wunch et al. (2017b), the spatial collocation criteria for GOSAT soundings were those falling within ±2.5∘ latitude and ±5∘ longitude of a TCCON station for most sites. For the Southern Hemisphere (SH) sites poleward of 25∘ S latitude, where the variation of CO2 is low, the spatial box was increased to ±10∘ latitude by 20∘ longitude to increase the number of collocations. For the Edwards TCCON station, which lies in an arid region just north of the polluted Los Angeles metropolitan area, a very specific collocation box of [34.68, 37.46] latitude and [−127.88, −112.88] longitude was used to avoid contamination from the city. Similarly, for the Caltech site, located in Pasadena, California, a latitude box of [33.38, 34.27] and longitude box of [−118.49, −117.55] were used. This avoids collocating GOSAT soundings measured over ocean, the San Gabriel Mountains, and regions too far outside of the Los Angeles basin with the Caltech TCCON data. Finally, only GOSAT soundings acquired within ±2 h of the mean TCCON measurement time were considered. For the quality filtering and bias correction procedure, single sounding level collocations are used to maximize the number of fit points.
Estimates of CO2 from atmospheric inversion systems, or models, provide a useful metric for evaluating satellite-based estimates of XCO2 (O'Dell et al., 2018). In this work, a suite of four models (CarbonTracker, CAMS, CarboScope, and University of Edinburgh) were sampled at the GOSAT sounding times and locations. Brief descriptions of each, along with references, are provided in Table 3. The models use a variety of land biosphere prior fluxes, inverse solvers and transport models, and assimilate CO2 data only from flasks and continuous analyzers on a wide variety of platforms, e.g., observatories, towers, aircraft, and ships. Specifically, no data from GOSAT, OCO-2, or TCCON are assimilated. The CO2 concentration fields of the models capture the known features of the global atmospheric CO2 distribution, including seasonality, time trends, and inter-annual variability (IAV) due to El Niño–Southern Oscillation. For each GOSAT sounding, the vertical profiles of CO2 from the corresponding grid box of each of the four models are spatiotemporally interpolated (linear in latitude, longitude, and time) to the GOSAT observation point, and the GOSAT averaging kernel is applied to each vertical profile to produce a modeled XCO2 as if viewed from the satellite.
For each GOSAT sounding, a multi-model median (MMM) XCO2 was calculated from the models having a valid XCO2 estimate for that location and time. Unless otherwise noted, the model XCO2 is taken to be that which a perfect OCO-2 would have observed, XCO2,ak; that is, an averaging kernel correction is applied to account for differences between the model profile of CO2 and the ACOS prior in the unmeasured part of the profile:
where hi is the pressure weighting function on the i=1…20 ACOS model levels, a is the normalized ACOS averaging kernel for CO2, um is the model profile of CO2, and ua is the ACOS prior profile of CO2.
To exclude outliers, models with XCO2 that deviated more than ±1.5 ppm from the initial MMM for that sounding were not included. The sounding was then rejected if more than one of the four models had been excluded, or if the standard deviation amongst the valid models was > 1 ppm. Approximately 85 % of the GOSAT v9 soundings with a good L2FP quality flag had a valid MMM XCO2 value for analysis. The regions with the highest fraction of rejections occur along the Southern Ocean (latitude −60∘), the Amazon and Congo rain forests, and a broad region across northern Asia. Table 4 lists the model version numbers used for the QF/BC procedure, as well as that used in the evaluation of the final good-quality XCO2 product that will be presented later.
Peters et al. (2007)CarbonTracker (2021)Rödenbeck (2005); Rödenbeck et al. (2018)CarboScope (2021)Chevallier et al. (2010)CAMS (2021)Feng et al. (2009)UoE (2021)Table 3Carbon inversion systems used for ACOS GOSAT v9. NOAA – National Oceanic Atmospheric Administration, CASA – Carnegie–Ames–Stanford Approach, TM5 – Transport Model 5, TM3 – Transport Model 3, EnKF – ensemble Kalman filter, 4D-Var – 4-Dimensional Variation, ORCHIDEE – Organising Carbon and Hydrology In Dynamic Ecosystems, LMDZ – Laboratoire de Météorologie Dynamique, UoE – University of Edinburgh, GEOS-Chem – Goddard Earth Observing System Chemistry.
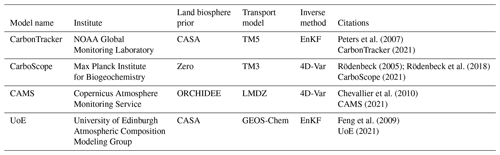
Table 5 lists the quality filtering variables used for ACOS GOSAT v9 and their corresponding thresholds. Many of the same variables (18 out of 31) were also used in the OCO-2 v9 quality filtering, as seen in Table 5 of Kiel et al. (2019). This includes the IDP CO2 and H2O ratios (Frankenberg et al., 2005), and the A-band preprocessor dP, i.e., the difference between the retrieved and prior surface pressure from the oxygen A band (Taylor et al., 2016). Another common variable used for quality filtering is the perturbation in the L2FP CO2 vertical profile relative to the prior, a quantity called “CO2 grad del” (δ ), as defined in Eq. (5) of O'Dell et al. (2018). A number of aerosol-related retrieval parameters are also used, similar to OCO-2 v9. Section 2.5 of the ACOS GOSAT v9 DUG provides additional details on the quality filtering (O'Dell et al., 2020).
Table 5ACOS GOSAT v9 L2FP quality filtering variables and thresholds. Descriptions of the variables can be found in the DUG (O'Dell et al., 2020). Soundings falling outside of the data ranges are assigned a bad XCO2 quality flag. The second column identifies variables that were also used for OCO-2 v9 quality filtering, as taken from Table 5 of Kiel et al. (2019).
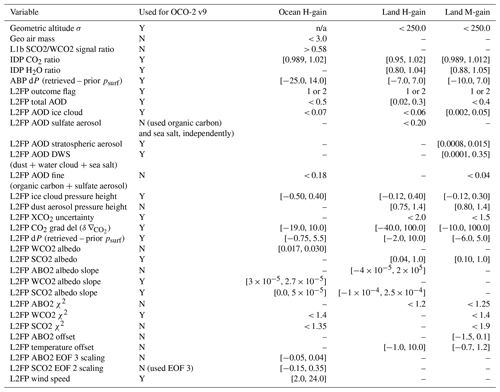
n/a – not applicable
Spurious correlations in the estimates of XCO2 with other retrieval variables due to inadequacies in the modeled physics motivate the application of a bias correction (Wunch et al., 2011b; O'Dell et al., 2018). Generally such spurious correlations are found with state vector elements such as retrieved surface pressure, various aerosol parameters, and δ . For each sensor there are also typically offsets by viewing mode, e.g., land glint versus ocean glint, which are accounted for via the bias correction. A general discussion of the ACOS XCO2 bias correction methodology is provided in Sect. 4 of O'Dell et al. (2018), where the fundamental equation is defined as
where CP is the mode-dependent parametric bias, CF is a footprint-dependent bias for footprints 1…8, and C0 represents a mode-dependent global scaling factor. Note that for GOSAT there is no footprint-dependent bias correction term, as is necessary for OCO due to low-level calibration errors across the detector frame. Further, to be consistent with previous ACOS GOSAT data versions, the global divisor is replaced by an additive offset, which is effectively the same because the range of XCO2 variability (∼ 20 ppm) is small relative to the mean XCO2 (∼ 400 ppm).
The explicit formula for application of the ACOS GOSAT v9 correction is provided in Sect. 2.5.6 of the DUG (O'Dell et al., 2020). For both land H-gain and M-gain, a set of five BC variables are used, while ocean H-gain uses only three variables. The difference between the H-gain and M-gain bias correction over land is minor. New for ACOS GOSAT v9 is the use of a correction against time, which is made possible with an 11-year data record; the corrections are 0.05 ppm/yr over land and 0.10 ppm/yr over water. The source of this spurious drift in the bias-corrected XCO2 is currently unclear and is the subject of ongoing study. Although there is some commonality in the quality filtering and bias correction variables used for ACOS GOSAT v9 (compare Tables 5 and 6), they do differ somewhat, as is typically the case with each sensor and data version.
Table 6 compares the bias correction variables used for ACOS GOSAT v9 with the variables used in the previous ACOS GOSAT v7.3, as well as with OCO-2 v9 and v10. The same few variables have appeared in all recent versions, including L2FP δ , L2FP dP, and L2FP DWS for land soundings. For ocean soundings the bias correction variables have evolved, with the only common one being δ .
Kiel et al. (2019)Table 7 summarizes the effect of the quality filtering and bias correction on the ACOS GOSAT XCO2 for v7.3 and v9. For ocean H-gain soundings, the v9 quality flag is substantially more restrictive compared to v7.3, i.e., ≃ 57 % pass rate compared to ≃ 78 %. This is mostly driven by the more extensive latitudinal coverage in the v9 record, which tends to include more soundings with high solar zenith angles (SZA) and low signal-to-noise ratio (SNR), which are more challenging for the L2FP. For H-gain land observations, the two versions have quite similar QF pass rates (≃ 35 %–45 %). The QF pass rate for v9 M-gain land data is ≃ 39 % when compared against models but ≃ 56 % against TCCON. In all cases there is a significant reduction in the scatter of the XCO2 after application of the QF/BC: by a factor of ≃ 2 for ocean H-gain and land M-gain and a factor of 3 for land H-gain. The QF/BC scatter is always slightly lower for v9 compared to v7.3, even though the number of soundings is greater by 1.5 to 10 times for the various scenarios.
Table 7Comparison of ACOS GOSAT v7.3 versus v9 sounding throughput and XCO2 scatter against truth metrics before and after filtering and bias correction.
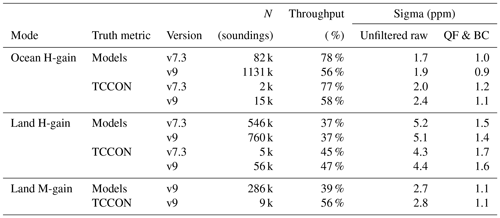
Figure 2 shows the relative magnitudes of the bias correction on the good-quality soundings by season, aggregated to 2.5∘ latitude by 5∘ longitude. The global median bias of −1.8 ppm has been removed for clarity. This highlights gradients and contrasts in the bias correction, which are of importance as gradients in CO2 concentrations are the primary driver of CO2 fluxes in atmospheric inversion systems. In general, the bias correction is necessary to remove spurious contrasts between land and ocean XCO2 values. The strongest relative bias corrections are positive adjustments over the bright land surfaces in M-gain viewing mode, specifically the Sahara in DJF and JJA and Australia in DJF. The land H-gain observations have a mix of relative bias correction values, ranging from mildly negative over high northern latitudes in JJA to moderately positive over northern mid-latitudes in JJA in the western United States and the Middle East. Most of the ocean H-gain observations have a mildly negative relative bias correction, with some mild positive values in the southern tropical oceans in DJF.
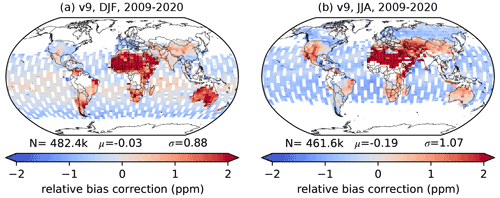
Figure 2Maps of ACOS GOSAT v9 relative bias correction for DJF (a) and JJA (b) for good QF soundings in the 11-year data record at 2.5∘ by 5∘ latitude–longitude, after removal of the global, median bias of −1.8 ppm. Grid cells with fewer than five GOSAT soundings are not colored. The total number of soundings (N), and the mean bias (μ) and standard deviation of the bias (σ) in each grid cell are given.
The ACOS GOSAT v9 XCO2 record was characterized in five ways: (i) an analysis of the XCO2 “good-quality” data volume, (ii) a spatiotemporal analysis of the XCO2 estimates, (iii) a validation against XCO2 estimates from TCCON, (iv) a comparison to XCO2 derived from models, and (v) a comparison with collocated XCO2 estimates from the OCO-2 v10 product.
4.1 ACOS GOSAT v9 “good-quality” data volume
It is instructive to compare the ACOS GOSAT v9 product to the earlier v7.3 product to highlight similarities and differences in the quality filter screening. A time series histogram of the monthly throughput of the good-quality-filtered soundings for the v9 product compared to v7.3 is shown in Fig. 3. The soundings have been binned by month, with the three GOSAT observation modes displayed by color. The v7.3 product did not contain any land M-gain data in the L2Lite files (red in the figure) as the quality filtering and bias correction were not developed for that gain mode in v7.3 due to some unreconciled differences. An important feature of the v9 data record is the extension in time, which runs through June 2020, compared to a termination date of June 2016 for v7.3. Even for the overlapping v7.3 and v9 time period (2009 through mid 2016), there are some differences in the data volume for land H-gain and ocean H-gain observations. This is due to changes in both the details of the QF procedure, including changes in the variable thresholds used to assign QF = good/bad, and to some differences in the convergence characteristics of the L2FP retrieval. Generally, v9 is producing up to 60 % more good-quality data than v7.3 near the end of the overlap period in 2016. There was a substantial increase in the number of good QF soundings from 2010 to 2019, due to the increased latitudinal range of the ocean observations as a result of improvements in the GOSAT pointing strategy, as well as improvements in the sounding selection for ACOS L2FP v9.
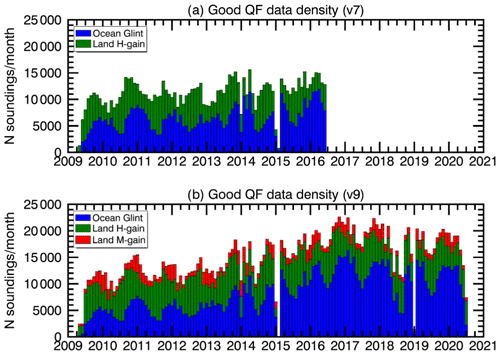
Figure 3Time series histograms of the monthly number of good QF GOSAT soundings for v7.3 (a) and v9 (b) spanning the 11-year record. The large data gaps in early 2015 and 2019 were caused by a switch from the primary to secondary pointing mirror and a solar panel failure, respectively.
Figure 4 shows sounding density Hovmöller plots comparing ACOS GOSAT v7.3 (a) to v9 (b) with the three GOSAT observation modes combined. Again, the extended time period covered by v9 is evident. The increase in sounding density in the SH beginning in 2016 due to optimization of the GOSAT viewing strategy is prominent in the v9 product. This feature is also seen in the spatial maps showing the fraction of good-quality soundings and the density per grid box, in panels (c) and (d) of Fig. 1, which was introduced in Sect. 3.2. Persistently clear regions, such as the Sahara and the western part of Australia, have as many as 30 % of the observations assigned a good-quality flag. Large regions of the tropical Pacific and Atlantic also contain a relatively high fraction of good-quality soundings. On the other hand, tropical forests and high latitudes in general have low yields of good-quality soundings. This is largely a combination of cloud contamination, dark surfaces at shortwave infrared wavelengths, and low solar illumination conditions, all three of which are problematic for retrieving CO2 from space using reflected sunlight.
4.2 ACOS GOSAT v9 XCO2 spatiotemporal analysis
There has been a steady increase in the atmospheric burden of CO2 since the onset of the industrial age due mainly to the burning of fossil fuels (e.g., Keeling et al., 1995). In May of 2009, at the beginning of the GOSAT mission, the mean global value of XCO2 reported by the NOAA Global Monitoring Laboratory was 387.95 ppm, while by May of 2020, the mean global value had risen to 413.81 ppm (Dlugokencky and Tans, 2021). This yields a secular increase of ≃ 2.35 ppm/yr. For comparison, Fig. 5 shows the ACOS GOSAT v9 bias-corrected and quality-filtered XCO2 as a function of latitude (15∘ increments) and time (30 d increments) for combined land M and H-gain observations (a) and ocean H-gain observations (b). Using the monthly mean XCO2 values (combined land and ocean) for May 2009 (386.50 ppm) and May 2020 (411.82 ppm), the ACOS GOSAT v9 record has a secular increase of ≃ 2.30 ppm/yr over the 11-year record. This small disagreement in secular trend of approximately 2 % is understandable, given the significant differences in the spatiotemporal sampling of the two data sets. For the interested reader, a thorough comparison of satellite- and surface-derived growth rates in atmospheric CO2 is given in Buchwitz et al. (2018).
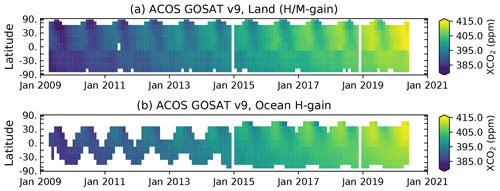
Figure 5ACOS GOSAT v9 bias-corrected and quality-filtered XCO2 as a function of latitude (15∘ increments) and time (30 d increments) for combined land M-gain and H-gain observations (a), and ocean H-gain observations (b). Grid cells with fewer than 10 GOSAT soundings are not colored.
The maps in Fig. 6 show the spatial distribution of XCO2 at 2.5∘ latitude by 5∘ longitude resolution for 2010 (top) and 2019 (bottom), for DJF (left) and JJA (right). The dynamic range of the color scale in each case is 6 ppm. However, due to the secular increase in global CO2 of ≃ 2.3 ppm per year, the scale is centered ≃ 20 ppm higher in 2019 compared to 2010. The strong latitudinal gradients in XCO2 are similar in these two seasons, while the zonal gradient tends to be weakest in MAM (not shown), just before the summer drawdown of CO2 by the land biosphere begins. The increase in the number of ocean H-gain soundings in the later part of the data record is also evident in these maps.
Qualitatively, the patterns in the maps look quite similar from 2010 to 2019, but with increased data coverage. In general, the highest concentrations of XCO2 for the two selected seasons are observed by GOSAT in the Northern Hemisphere (NH) during DJF, especially over northern tropical Africa (between 0 and 15∘ N latitude), large portions of China, and the eastern United States. This stands to reason, as the atmospheric burden of CO2 increases towards a peak during NH winter due to inactivity of the land biosphere, coupled with strong anthropogenic CO2 emissions. During DJF the ACOS GOSAT v9 XCO2 exhibits relatively low concentrations across the entire SH, as would be expected if the Southern Ocean were a strong carbon sink (e.g., Gruber et al., 2019). In JJA, the XCO2 is reduced over the mid-latitude and boreal forests, also expected behavior due to strong photosynthetic uptake of CO2 during this season (e.g., Ciais et al., 2019).
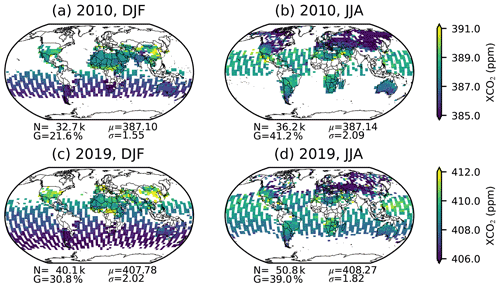
Figure 6ACOS GOSAT v9 bias-corrected XCO2 for the good QF soundings at 2.5∘ latitude by 5∘ longitude resolution for DJF 2009–2010 (a), JJA 2010 (b), DJF 2018–2019 (c), and JJA 2019 (d). The dynamic range of the color scale in each case is 6 ppm. However, due to the secular increase in global CO2 of ≃ 2.2 ppm per year, the scale is centered ≃20 ppm higher in 2019 compared to 2010. Grid cells with fewer than five GOSAT soundings are not colored.
A quantification of differences in the bias-corrected ACOS GOSAT v9 XCO2 data product relative to the v7.3 product is given in Fig. 7 for the overlapping period. The top row (panels a through c) shows results for the land H-gain observations, while the lower row (panels d through f) shows results for the ocean H-gain observations. Only soundings that were present in both data sets and assigned a good-quality L2FP flag were used in this comparison. This restricts the analysis to April 2009 through June 2016 and also eliminates the v9 land M-gain data, as no M-gain data exist in the v7.3 product. The mean and standard deviation of the ΔXCO2 for the approximately 311 k land soundings at the single sounding level are −0.18 and 0.72 ppm, respectively, as shown in panel (a). When gridded and mapped at 2.5∘ latitude by 5∘ longitude resolution, as shown in panel (b), the majority of the negative signal (v9 XCO2 lower than v7.3) occurs at latitudes greater than approximately 45∘. Most of the land mass at latitudes less than 45∘ has ΔXCO2 values closer to zero, with the largest positive signals appearing over equatorial forests. Furthermore, when the data are gridded and viewed versus time and latitude in 30 d by 15∘ increments, respectively, as in panel (b), we see that the ΔXCO2 signal has an increasing tendency in time; i.e., the v9 XCO2 increases more rapidly in time than v7.3. The cause of this effect is currently unknown, but it is partially due to the implementation in v9 of a time-dependent bias correction term of +0.05 ppm/yr for land observations. This translates into an expected change of about 0.35 ppm in the v9 record over the 2009 to 2016 time span.
For the ocean H-gain observations at the single sounding level (panel d), the mean and standard deviation of the ΔXCO2 are +0.28 and 0.79 ppm, respectively. When gridded and mapped at 2.5∘ latitude by 5∘ longitude resolution (panel e), the spatial distribution is fairly smooth, i.e., low variation in both latitude and longitude. Finally, when the data are gridded versus time and latitude (panel f), the modest variation in latitude is confirmed, but a substantial time dependence is observed, with the ΔXCO2 signal beginning negative in 2009 (v9 XCO2 lower than v7.3), and switching to a positive ΔXCO2 signal by 2016 (v9 XCO2 higher than v7.3). The time-dependent bias correction term for ocean H-gain observations was +0.1 ppm/yr. This translates into an expected change of about 0.7 ppm over the 2009 to 2016 time span in the v9 record, accounting for some but not all of this time-dependent difference between v9 and v7.3.
This direct comparison between the v9 and v7.3 XCO2 product only allows for statements as to their differences. It does not allow one to deduce which is closer to truth. Therefore, an analysis of the v9 XCO2 data product against truth metrics follows. Furthermore, it is difficult to accurately determine the effect that the new v9 XCO2 product will have on atmospheric inversion system results relative to v7.3 without further detailed study.
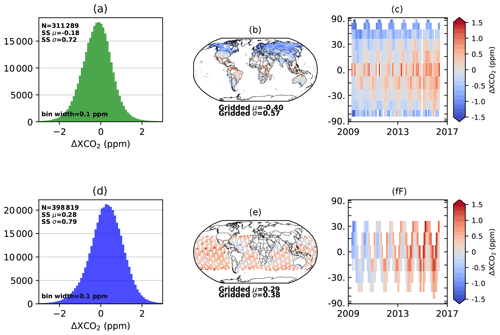
Figure 7Analysis of the ACOS GOSAT calculated ΔXCO2 (v9 minus v7.3) for the quality-filtered and bias-corrected soundings for the overlapping period spanning April 2009 through May 2016. Panels (a) through (c) show results for the land H-gain observations, while panels (d) through (f) show results for ocean H-gain observations. Panels (a) and (d) show the single sounding frequency distribution of ΔXCO2 in 0.1 ppm bins. Panels (b) and (e) show the spatially gridded ΔXCO2 at 2.5∘ latitude by 5∘ longitude resolution. Panels (c) and (f) show the ΔXCO2 as a function of time and latitude in 30 d by 15∘ increments, respectively. The statistics in panels (a) and (d) were calculated at the single sounding level, while those reported in panels (b) and (e) were calculated on the grid box means.
4.3 ACOS GOSAT v9 XCO2 versus TCCON
A list of TCCON stations used in this work, including basic physical information and data citations, is given in Table 8. For the evaluation against the ACOS v9 XCO2 data, the single sounding collocations described in Sect. 3.3 were aggregated into overpass mean values. Essentially the same TCCON data set was used for both the QFBC procedure as for the evaluation, as no hold-over data were maintained. Also, as described in Sect. 3.3, an averaging kernel correction was applied to the TCCON data in order to fairly compare to the satellite data. A one-to-one linear regression of the XCO2 provides a simple quantification of the agreement, as shown in Fig. 8.
Strong et al. (2019)Kivi et al. (2014)Wunch et al. (2017a)Deutscher et al. (2019)Notholt et al. (2019)Hase et al. (2015)Te et al. (2014)Warneke et al. (2019)Sussmann and Rettinger (2018a)Sussmann and Rettinger (2018b)Wennberg et al. (2017)Morino et al. (2016)Iraci et al. (2016b)Wennberg et al. (2016b)Dubey et al. (2014); Lindenmaier et al. (2014)Goo et al. (2014)Morino et al. (2018a)Petri et al. (2020)Iraci et al. (2016a)Wennberg et al. (2016a)Wennberg et al. (2016a)Wennberg et al. (2015)Kawakami et al. (2014)Liu et al. (2018)Blumenstock et al. (2017)Morino et al. (2018b)Feist et al. (2014)Griffith et al. (2014a)De Mazière et al. (2017)Griffith et al. (2014b)Pollard et al. (2019)Table 8List of TCCON stations used in the BCQF and XCO2 evaluation of ACOS GOSAT v9, along with the data citations.
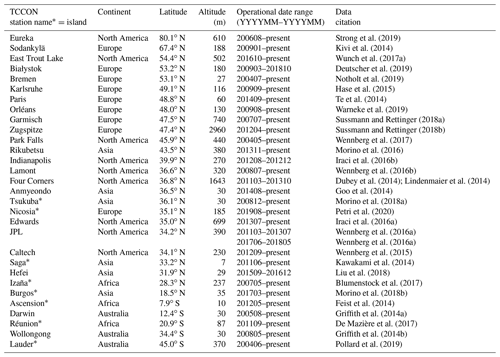
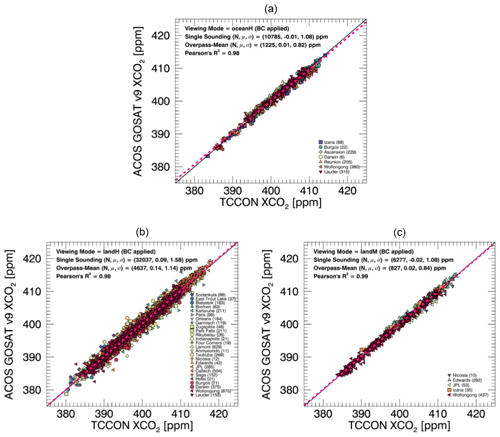
Figure 8Quality-filtered and bias-corrected ACOS GOSAT v9 vs. collocated TCCON XCO2 for ocean H-gain (a), land H-gain (b), and land M-gain (c). Each point represents an overpass mean, which typically contain 5–10 GOSAT soundings per overpass. The legend in the lower right indicates the number of collocated overpass means for individual TCCON stations. Summary statistics for all stations combined are reported in the upper left of each panel for both single sounding and overpass means.
For ocean H-gain observations (Fig. 8a), the mean (μ) of the differences in XCO2 (ΔXCO = GOSAT − TCCON) is essentially zero: −0.01 ppm for the single-sounding (SS) results and +0.01 ppm for the overpass mean (OPM) results. The corresponding standard deviations (σ) are 1.08 and 0.82 ppm for the SS and OPM results, respectively. This indicates that roughly half of the SS error variance is a result of instrument noise or other random high-frequency error sources (1.082=1.2 ppm versus 0.822=0.7 ppm).
For land H-gain observations (Fig. 8b), ppm and +0.14 ppm for the SS and OPM, respectively. The land H-gain σ values are higher than for ocean H-gain: 1.58 and 1.14 ppm for SS and OPM, respectively. Larger variations in ΔXCO are expected for land H-gain due to variability in topography and surface brightness, as well as higher likelihood of contamination by cloud and aerosol, all of which are more challenging for the ACOS retrieval. Further, biology and atmospheric transport cause CO2 signals to vary more over land regions, and in addition, instrument noise is higher because the SNRs tend to be lower.
Land M-gain observations have near-zero bias (.02 ppm and +0.02 ppm for SS and OPM, respectively) and scatter similar to that for ocean H-gain (σ=1.08 and 0.84 ppm for SS and OPM, respectively), likely driven by lower variability in surface topography and brightness compared to land H-gain observations, as well as higher SNRs over these bright land surfaces.
The correlation in the XCO2 between the data sets in all observation modes is high, with Pearson R2=0.98, 0.98, and 0.99 for ocean H-gain, land H-gain, and land M-gain, respectively. Overall, these results indicate excellent agreement between the bias-corrected and quality-filtered ACOS GOSAT v9 XCO2 product and collocated estimates from TCCON.
Figure 9 shows the mean absolute error (MAE) between the overpass mean collocated GOSAT and TCCON XCO2, organized by latitude bins, season, and observation mode for v7.3 (panels a and b) and v9 (panels c, d, e). The calculation of the MAE and error bars follow the procedure reported in Chatterjee et al. (2013) (Eqs. 3 and 4). The error bars on the MAE represent the scatter around the mean. A smaller error bar, or a lower scatter, implies that the MAE values are more consistent across a group of TCCON stations within a latitude band and season. The MAEs tend to be lower for v9 compared to v7.3, with smaller error bars and increased number of collocations. This is especially true for the SH ocean H-gain data, where the MAE ranges from 0.4 to 0.7 ppm in v9 for all seasons, in contrast to v7.3, which had higher MAE ranging from 0.5 to 0.85 ppm in that region. In the v9 land H-gain data, the MAE is roughly a function of latitude, with the highest values (≃ 1.0 ppm) seen between 60–90∘ N and the lowest values (≃ 0.7 ppm) seen from 30–60∘ S. This stands to reason as lower variability of XCO2 in the SH tends to yield better agreement between satellite- and ground-based observations. The error bars on the v9 land H-gain estimates in the 30–60∘ N latitude range are very small, due in part to the large number of collocations. There are very limited land data between 0 and 30∘ N (approximately 25 % of Earth's surface), due to the sparsity of TCCON stations in this latitude band. Only Burgos (18.5∘ N) and Izaña (28.3∘ N) are located in this range (reference Table 8), and many of the collocations from these sites are from GOSAT observations made in ocean H-gain viewing.
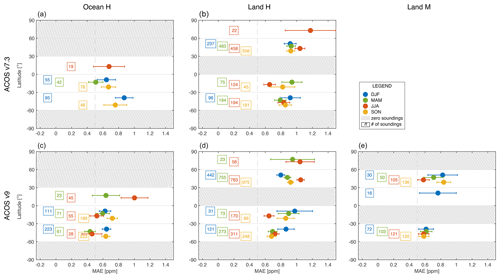
Figure 9Mean absolute error (MAE; ppm) between ACOS GOSAT v9 XCO2 and collocated TCCON observations for v7.3 (a, b) and v9 (c, d, e), binned by latitude for ocean H-gain (a, c), land H-gain (b, d) and land M-gain (e). Recall that there were no land M-gain data generated for v7.3. The error bars on the MAE represent the standard error of the mean. The calculation of the MAE statistic and error bars follow the procedure reported in Chatterjee et al. (2013) (Eqs. 3 and 4). The total number of independent observations available within each latitude band and for each season are reported in the colored boxes. Latitude bands that do not have any collocated soundings are shaded in gray.
Knowledge of the average XCO2 seasonal cycle can be used to disentangle the CO2 growth rate from the seasonal variability, as well as for quantifying potential seasonal biases between satellite- and ground-based XCO2 estimates. Lindqvist et al. (2015) fitted a skewed sine wave (see Eq. 1 of Lindqvist et al., 2015) to the ACOS GOSAT v3.5 XCO2 time series and the TCCON estimates of XCO2 at 16 stations, spanning April 2009 through December 2013. They found that ACOS GOSAT v7.3 captured the seasonal cycle within approximately 1 ppm of the TCCON estimates for all but the European sites and that the satellite- and ground-based CO2 growth rates agreed generally better than 0.2 ppm per year. Here, we provide an update to those results using the 11-year ACOS GOSAT v9 XCO2 data record. For this part of the analysis, a slightly more restrictive set of collocation criteria were implemented, compared to that described in Sect. 3.3 for the BC/QF procedure and to that used to generate Fig. 8. The seasonal cycle analysis required that the TCCON record spanned at least one contiguous year (a full seasonal cycle) and that a minimum of 20 collocations with GOSAT occurred. In addition, the three GOSAT observation modes (ocean H-gain, land M-gain, land H-gain) were combined for each site, and satellite overpass means of XCO2 were aggregated into daily means. This resulted in approximately 7700 daily averages at 26 TCCON stations over the 11-year GOSAT data record.
Figure 10 shows the results of the seasonal cycle fit for the Lamont, Oklahoma, TCCON station. The one-to-one scatter of the 896 daily averaged XCO2 values (a) indicates a bias of −0.27 ppm for the GOSAT product relative to TCCON, with a standard deviation of 1.25 ppm and a Pearson's R2 of 0.99. The seasonal cycle fits (b) indicate excellent agreement in the secular CO2 increase at this site: 2.34 ppm for both GOSAT and TCCON. The mean seasonal amplitudes indicate a slight disagreement of a few tenths of a ppm, with TCCON showing a slightly higher fitted peak XCO2 value during the spring maximum phase, compared to GOSAT. This is similar to the results for this site reported in Fig. 4 of Lindqvist et al. (2015). The time series of the calculated difference in satellite- and ground-based estimated XCO2 (GOSAT − TCCON), shown in (c), highlights the magnitude of the scatter about the mean bias and suggests that there is no observable time drift in the data at this site.
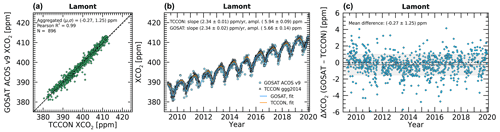
Figure 10Seasonal cycle analysis of ACOS GOSAT v9 XCO2 versus collocated TCCON for the Lamont, Oklahoma, station, following the methodology of Lindqvist et al. (2015). Panel (a) shows the one-to-one scatter plot of the bias-corrected daily mean XCO2 for GOSAT versus TCCON. Panel (b) shows the time series of GOSAT XCO2 (blue circles) with fit (blue line) and the TCCON XCO2 (gray triangles) with fit (orange line) over the 11-year data record. Panel (c) shows the time series of calculated ΔXCO (GOSAT – TCCON), with the mean difference (horizontal solid black line) and ±1 standard deviation (gray shading). The three GOSAT observation modes have been combined into daily mean averages to provide the maximum number of collocations possible for the seasonal fits.
A summary of the data from each station that met the seasonal cycle collocation criteria is provided in Table 9. In addition, the full complement of plots is presented in Appendix A. Overall, the seasonal cycle analysis at most sites is in agreement, to within the estimated uncertainties. The standard deviation of the mean XCO2 biases for the 26 sites is 0.41 ppm for the ACOS GOSAT v9 record. This compares to a value of 0.51 ppm at 23 stations for ACOS GOSAT v7.3, suggesting an improvement in the quality of the v9 XCO2 product.
Table 9Evaluation of the daily mean bias-corrected ACOS GOSAT v9 XCO2 (all viewing modes combined) against collocated TCCON estimates for individual stations. There were 7741 d total for the 26 stations. The following sites/instruments were excluded from this part of the analysis due to inadequate time series or seasonal cycle coverage: Eureka, Four Corners, Indianapolis (Influx), JPL2007, Lauder1, Lauder3, Manaus, and Ny-Ålesund. The mean, standard deviation, and Pearson correlation coefficient (μ, σ, R2) of the linear fit between GOSAT and TCCON are given in columns 3–5. The remaining columns quantify the seasonal cycle fit following the methodology described in Lindqvist et al. (2015). The bottom row provides mean summary statistics for the linear fit.
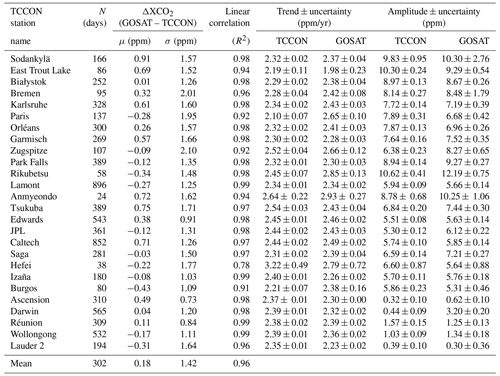
4.4 ACOS GOSAT v9 XCO2 versus models
The collocation and calculation of the multi-model-mean (MMM) is described in Sect. 3.3. Although the model data used for evaluation were very similar to those used in the QF/BC procedure, some minor version updates and extensions in time were included, as indicated in Table 4. It is important to be aware that there can be a considerable delay between performing the QF/BC procedure and the full generation of the final product, during which time the models are often updated.
Seasonal maps of ΔXCO (GOSAT v9 minus MMM) are shown in Fig. 11 for the 11-year data record binned at 2.5∘ latitude by 5∘ longitude. Generally, the agreement between the model-derived values and the satellite estimates is quite good, with an annual mean difference of .15 ppm and binned scatter ≃ 0.5 ppm. For ocean H-gain observations, the ΔXCO tends to be negative (positive) in the Northern (Southern) Hemisphere. Land observations exhibit several distinct sub-continental-scale disagreements, including a strong positive signal over northern tropical Africa in DJF (GOSAT XCO2 higher than MMM).
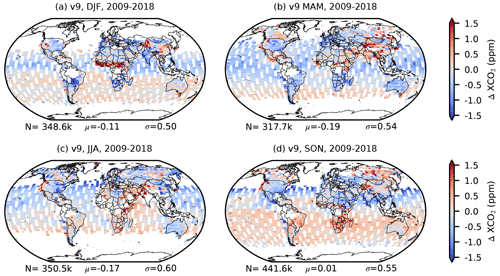
Figure 11Seasonal maps of the mean ΔXCO (GOSAT – MMM) spanning 2009 through 2018 for DJF (a), MAM (b), JJA (c), and SON (d) at 2.5∘ latitude by 5∘ longitude resolution. Grid boxes containing fewer than 10 collocations are colored white.
Figure 12 shows Hovmöller plots of the ΔXCO ocean H-gain data at 30 d by 15∘ latitude resolution for v7.3 (a) and v9 (a). The extension in time of v9 is evident, as well as the expansion in the latitude range of the ocean H-gain observations since 2015. A direct comparison between the v7.3 and v9 ΔXCO values for the overlapping time period, April 2009 through June 2016, reveals a global mean bias and standard deviation of −0.54 and 1.0 ppm for the v7.3 product, and −0.20 and 0.84 ppm for v9, underscoring the improvement.
Of particular note are the strong positive ΔXCO values in the v9 SH ocean H-gain observations for the latter part of 2014, persisting through most of 2015. This feature is not seen in the v7.3 product, due to a paucity of SH ocean H-gain data. It approximately coincides with the strong 2015–2016 El Ninõ event, where ΔXCO signals were also seen in the OCO-2 v7 ocean H-gain data, as reported in Chatterjee et al. (2017). It has been hypothesized that the 2015–2016 El Ninõ produced an anomalously strong carbon release from tropical land regions due to higher temperature and below average precipitation (Liu et al., 2017). In contrast to the positive SH signal, negative ΔXCO values (GOSAT lower than MMM) have been observed in the v9 NH oceans since 2016. It is unclear why the satellite and models disagree over such large spatial and temporal scales, but recent work by Müller et al. (2021) suggests that the ACOS v7.3 (and to a lesser extent v9) XCO2 values are in fact biased low by approximately 1 to 1.5 ppm, as compared to a new independent evaluation data set generated from combined ship and aircraft measurements over the open oceans. Further investigation into the source of the ACOS GOSAT biases against models is warranted.
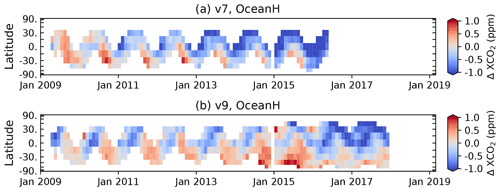
Figure 12Time series of ΔXCO (ACOS GOSAT v9 – MMM) versus latitude at 30 d by 15∘ resolution for ocean H-gain observations for v7.3 (a) and v9 (b). Grid cells containing fewer than 10 collocations are colored white.
Figure 13 shows spatial maps of ΔXCO for the truncated time span 2010 through 2015 comparing v7.3 (a and c) and v9 (b and d) for DJF (a and b) and JJA (c and d). There is significant decrease in scatter in the v9 product (≃ 0.5 ppm) relative to v7.3 (≃ 0.75 ppm). The low bias in DJF tropical Pacific vanishes in v9, and the positive bias in JJA extratropical land regions is reduced. The expanded latitudinal extent of the ocean H-gain observations is evident in the v9 maps. One feature that is robust in both v7.3 and v9 is the large positive signal over northern tropical Africa in DJF. This feature was also observed in the OCO-2 v7 and v8 comparisons to a MMM (O'Dell et al., 2018) and in v10 XCO2 anomaly maps (Hakkarainen et al., 2019).
4.5 ACOS GOSAT v9 XCO2 versus OCO-2
NASA's Orbiting Carbon Observatory-2 (OCO-2) has been collecting science data since September, 2014 from a near-polar low-Earth orbit (705 km altitude), with an afternoon Equator crossing time of ≃ 13:30 local time (Crisp et al., 2017). Like GOSAT, OCO-2 takes measurements of reflected solar radiation in the oxygen A-band (0.76 µm) and the weak and strong carbon dioxide bands (1.6 and 2.0 µm, respectively), which are used to estimate XCO2 using the ACOS L2FP retrieval algorithm (Eldering et al., 2017; O'Dell et al., 2018). However, due to differences in the orbit parameters of the two sensors, e.g., a 3 d repeat cycle for GOSAT versus a 16 d repeat cycle for OCO-2 (see Table 2 of Kataoka et al., 2017), the number of collocated soundings is somewhat limited. Therefore, some criteria must be defined in order to identify soundings that can be compared in a meaningful way. The underlying assumption of the collocation is that on scales of a few hundred kilometers and several hours, the natural variance in XCO2 is not detectable in satellite-derived estimates from the ACOS L2FP algorithm.
For this study, the coincidence criteria to match OCO-2 soundings to individual GOSAT soundings were as follows: (i) falling within 2∘ latitude and 3∘ longitude, (ii) with a maximum spatial separation of 300 km, and (iii) acquired within ±2 h. Due to the dense nature of the OCO-2 soundings relative to the sparseness of the GOSAT soundings, there are typically between zero and several hundred matched OCO-2 soundings per GOSAT footprint. A lower limit of 10 and an upper limit of 100 (randomly selected) OCO-2 soundings that meet the coincidence criteria were set in order to retain the GOSAT sounding for analysis. The individual L2FP quality flags are applied for both GOSAT and OCO-2 during the collocation procedure, and then the mean value of XCO2 from the 10 to 100 collocated OCO-2 soundings is calculated and subtracted from the corresponding GOSAT XCO2 to produce ΔXCO.
Here we compare ACOS GOSAT v9 against OCO-2 v10 (rather than to the deprecated v9), since we assume that science users will adopt the newest OCO-2 product. Major updates to the version 10 ACOS L2FP algorithm (Osterman et al., 2020) are discussed in Sect. 3.1.
One complexity in comparing ACOS GOSAT v9 and OCO-2 v10 is the fact that the two versions of the algorithm used different CO2 priors. Typically, models which assimilate satellite CO2 data take into account the unmeasured part of the prior CO2 profile (specified via the retrieval's averaging kernel) via an averaging kernel correction, as given in Eq. (1). Therefore, in order to fairly compare these two data sets as models would assimilate them, we need to remove their difference due to the unmeasured part of the CO2 profile, as follows:
where h is the XCO2 pressure weighting function, a is the normalized XCO2 averaging kernel, ua is the ACOS v9 CO2 prior profile used for GOSAT, and is the ACOS v10 CO2 prior profile used for OCO-2. The summation takes place over the 20 vertical levels defined in the ACOS code. In summary, the total adjustment to the ACOS GOSAT XCO2 value is calculated as the contribution of the difference in the vertical CO2 priors at each level weighted by the one minus the averaging kernel at that level. The global mean adjustment due to the CO2 prior correction was approximately 0.2 ppm, with 95 % of corrections between −0.1 and +0.5 ppm.
Spatial maps of ΔXCO (GOSAT v9 – OCO-2 v10) for the prior-corrected, collocated soundings are shown in Figs. 14 and 15 for land and ocean, respectively. In each figure the maps are shown by season at 2.5∘ latitude by 5∘ longitude resolution. In all seasons, higher scatter in ΔXCO is observed over land (≃1 ppm) than over ocean (< 0.7 ppm), likely due to variability of land surface features and/or lower signal-to-noise ratios of the radiance measurements. The annual global mean ΔXCO for land is near zero (0.06 ppm) and exhibits little variation with season. For ocean H-gain, the global mean ΔXCO is larger (−0.40 ppm) and varies more significantly by season from −0.2 (DJF) to −0.6 ppm (JJA). The disagreements in the ocean H-gain data tend to be spatially coherent, with a notably large negative difference in the NH in most seasons. Currently, the underlying cause of these disagreements is unknown and could stem from instrument calibration or sampling-related issues, differences in retrieval algorithm versions, or even collocation issues.
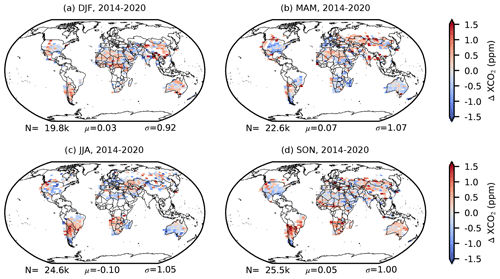
Figure 14Spatial distribution of the bias-corrected ΔXCO (GOSAT v9 minus OCO-2 v10) for the good QF land soundings for DJF (a), MAM (b), JJA (c), and SON (D) for the overlapping period August 2014 through June 2020. The spatiotemporal requirements for matched soundings are a maximum separation of 300 km, and observation time within ±2 h. Maps are gridded at 2.5∘ latitude by 5∘ longitude resolution, and only grid boxes with at least 10 matched soundings are shown.
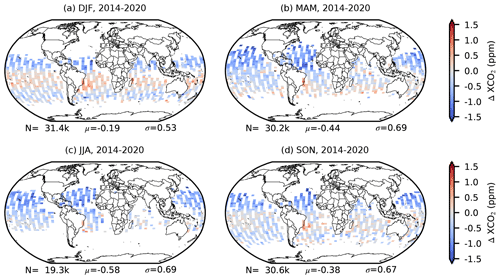
Figure 15Same as Fig. 14, but for ocean H-gain observations.
The disagreement in XCO2 for ocean H-gain between ACOS GOSAT v9 and OCO-2 v10 is highlighted in panel (a) of Fig. 16, which shows ΔXCO for the period September 2014 through December 2020 at 30 d by 15∘ latitude resolution for the ocean H-gain observations. Panel B shows the number of collocated soundings in each bin. A large SH positive difference in ΔXCO (GOSAT higher than OCO-2 by ≃ 0.5 ppm) is observed for the first 2 years of the time record. Then, in early 2016, there is what appears to be an abrupt jump to a large negative difference (GOSAT lower than OCO-2 by 0.5 ppm) in the NH. From this point forward, ΔXCO appears to be reasonably stable in time, although there is a persistent low difference in the NH for the remainder of the record.
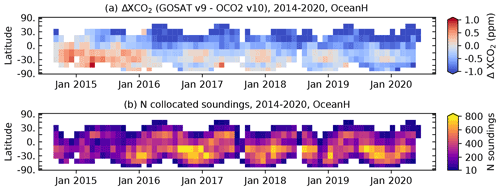
Figure 16Difference in XCO2 between ACOS GOSAT v9 and OCO-2 v10 (ΔXCO) as a function of time and latitude at 30 d by 15∘ latitude resolution for ocean H-gain observations (a). Panel (b) shows the sounding density of the collocated soundings. Grid cells containing fewer than 10 collocations are colored white.
Figure 17 shows the ΔXCO data for the combined land H-gain and M-gain data, similar to Fig. 16. The main feature here is that the overall variability is larger compared to the ocean H-gain data, which we attribute to biases introduced by variations in both topography and surface albedo. A slightly positive (red) signal is observed during the September to December months in the SH, especially in 2014, 2018, and 2019. Although additional investigation into such signals is warranted, it is beyond the scope of the current work.
A set of summary statistics for the ACOS GOSAT v9 versus OCO-2 v10 XCO2 product is given in Table 10. The values reported here are on the individual collocations by year and season, rather than the spatially gridded averages as given in Figs. 14 and 15. For the land observations, there has been a very slight upward trend in time of the ΔXCO to slightly more positive values (GOSAT v9 larger than OCO-2 v10 XCO2). On the other hand, for ocean H-gain observations, the general trend has been an increasingly more negative ΔXCO in time, as is seen in Fig. 16. Additional investigation will be required to determine the root cause(s) of these differences.
Table 10A set of summary statistics for the comparison of the ACOS GOSAT v9 XCO2 to the OCO-2 v10 product. Individual collocations for each year and season are given by N, while the mean ΔXCO2 and the standard deviation from the mean are given by μ and σ, respectively, both in units ppm. The top portion of the table is for land observations, while the bottom is for ocean H-gain (OceanH).
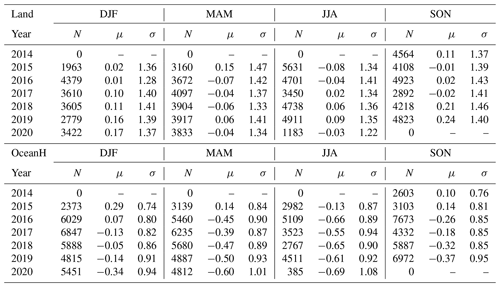
The ACOS GOSAT v9 XCO2 data are available on the NASA Goddard Earth Science Data and Information Services Center (GES-DISC) in both the per-orbit full format (OCO-2 Science Team et al., 2019b, https://doi.org/10.5067/OSGTIL9OV0PN) and in the per-day lite format (OCO-2 Science Team et al., 2019a, https://doi.org/10.5067/VWSABTO7ZII4). The monthly super-lite files, containing only the most essential variables for each satellite observation, are available at CaltechDATA (https://doi.org/10.22002/D1.2178, Eldering, 2021). The OCO-2 v10 L2Lite files containing the bias-corrected and quality-filtered XCO2 data are also available on the GES-DISC (OCO-2 Science Team et al., 2020, https://doi.org/10.5067/E4E140XDMPO2). The TCCON data for individual stations are available on the CaltechDATA site (see citations listed in Table 8). The CarbonTracker data are available on the NOAA GML site (https://carbontracker.noaa.gov, CarbonTracker, 2021). The CarboScope model data are available at http://www.bgc-jena.mpg.de/CarboScope (CarboScope, 2021). The CAMS model data are available at https://atmosphere.copernicus.eu/data (CAMS, 2021). The UoL model data are available at https://www.geos.ed.ac.uk/~lfeng/ (UoE, 2021).
The v9 ACOS GOSAT XCO2 product, spanning February 2009 through June 2020, has been compared to XCO2 estimates from TCCON, a suite of atmospheric inversion systems (models), and with collocated OCO-2 v10 data. The ACOS GOSAT v9 product is an improvement over ACOS GOSAT v7.3 relative to these standards. The v9 product provides a significant extension of the data record and contains data in M-gain viewing mode over bright land surfaces.
Of the 37.4×106 estimates of XCO2 contained in the ACOS GOSAT v9 data record, approximately 80 % were prefiltered due to contamination by cloud and/or aerosol, or due to insufficient SNR. Of the 7.0×106 that were selected to run through the ACOS L2FP algorithm, approximately 6.1×106 returned valid estimates of XCO2. However, only ≃ 2×106 of those were identified as being of “good” quality. This represents 5.4 % of the total recorded soundings. The quality filtering and bias correction variables used for ACOS GOSAT v9 were similar to those used in previous product versions, and similar to those used for OCO-2 v9 and v10, but include for the first time, a correction to account for a small temporal drift in the data.
Comparisons with collocated estimates of XCO2 from TCCON indicate overpass mean biases of ≃ 0.1 to 0.2 ppm and standard deviations of ≃ 1.5 ppm. The mean squared error against TCCON is highest for land observations in the northern mid-latitudes (30–60∘ N), and lowest for ocean H-gain and SH land M-gain observations. The statistics show improvement when compared to the results for v7.3, which spanned a shorter time period (April 2009 to June 2016). Specifically, the standard deviation of the mean station bias for the 26 sites is 0.41 ppm for the ACOS GOSAT v9 record, compared to 0.51 ppm at 23 stations for ACOS GOSAT v7.3.
Comparisons with collocated XCO2 derived from a suite of four atmospheric inversion systems (models) suggest annual global mean differences of ≃ 0.15 ppm and standard deviation of ≃ 0.5 ppm. Hemispherical differences in XCO2 estimates over oceans were observed, as well as robust subcontinental-scale land features. Results indicate better agreement with models in the ACOS v9 product (.20 ppm, σ=0.8 ppm) compared to v7.3 (.54 ppm, σ=1.0 ppm) for the overlapping period April 2009 through June 2016, but further investigation is required to explain the remaining disagreement over large spatial and temporal scales.
Comparisons with collocated OCO-2 v10 XCO2 data show low bias but relatively high scatter for land observations (μ=0.06 ppm, σ=1.0 ppm, when averaged across seasons). Increased scatter over land is expected due to XCO2 bias introduced by variability in topography and surface albedo. However, for ocean H-gain observations, although the XCO2 scatter is lower than that for land as expected (σ=0.7 ppm), the global mean bias is relatively high (.4 ppm, when averaged across seasons). These are issues that must be resolved in order for GOSAT v9 and OCO-2 v10 data to provide consistent information to atmospheric inversion systems for assessing fluxes of CO2.
Global estimates of CO2 derived from satellite measurements provide coverage in traditionally data-sparse regions where ground-based measurements are difficult. The assimilation of satellite XCO2 into atmospheric inversion systems to quantify the spatiotemporal variations of carbon fluxes is a promising, but challenging, area of research. This research continues to benefit from various improvements in transport models, atmospheric inversion systems, and satellite retrievals. The role of the GOSAT record in this field remains unique due to its exceptional 11-year length and its coverage of nearly 5.5 years of the carbon cycle prior to the launch of OCO-2. The ACOS GOSAT v9 L2Std and L2Lite file products are both available on the NASA GES DISC (OCO-2 Science Team et al., 2019b, a).
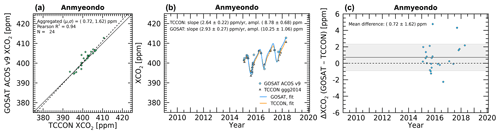
Figure A1Daily averaged bias-corrected ACOS GOSAT v9 versus collocated TCCON XCO2 at Anmyeondo, South Korea. Left panel (a) shows the one-to-one scatter plot of the daily mean XCO2 for GOSAT versus TCCON. Middle panel (b) shows the time series of daily mean GOSAT XCO2 (blue circles) with fit (blue line) and the TCCON XCO2 (gray triangles) with fit (orange line) over the 11-year data record. Right panel (c) shows the time series of calculated ΔXCO, with the mean difference (horizontal solid black line) and ±1 standard deviation (gray shading). In these plots, the three GOSAT observation modes have been combined in order to provide the maximum number of collocations possible for the seasonal fits.
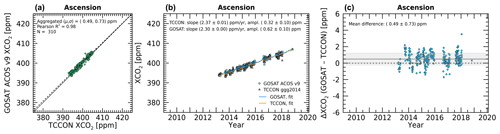
Figure A2Same as Fig. A1, but for Ascension Island, located in the Pacific Ocean off the west coast of Africa.
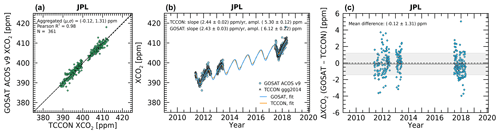
Figure A13Same as Fig. A1, but for Jet Propulsion Laboratory (JPL), Pasadena, California. This site has been used occasionally for the simultaneous operation of a TCCON instrument during the thermal vacuum testing of OCO-2 (Frankenberg et al., 2015) and OCO-3.
TET and CWO conceptualized the study and performed formal analysis. Additional formal analysis was performed by HL and AC. Funding acquisition was provided by DC, AK, POW, MG, and AE. Algorithm development was provided by TET, CWO, DC, POW, MG, AE, BF, MK, RRN, AM, and GO. Model data were provided by FC, PIP, and LF. TCCON stations were maintained and data provided by POW, NMD, MKD, DGF, OEG, DWTG, FH, LTI, RK, CL, MDM, IM, JN, YSO, HO, DFP, MR, CMR, MS, MKS, KS, KS, RS, YT, VAV, MW, TW, and DW. Paper writing of the draft was performed by TET, CWO, and DC. All authors contributed to editing the final version of the manuscript.
The contact author has declared that neither they nor their co-authors have any competing interests.
Publisher’s note: Copernicus Publications remains neutral with regard to jurisdictional claims in published maps and institutional affiliations.
Thomas E. Taylor acknowledges assistance from Peter Somkuti and Heather Cronk at CSU/CIRA with Python map plotting. CarbonTracker results were provided by NOAA ESRL, Boulder, Colorado, USA, from the website at http://carbontracker.noaa.gov (last access: 17 January 2022). The GEOS data used in this study were provided by the Global Modeling and Assimilation Office (GMAO) at NASA Goddard Space Flight Center.
The CSU contribution to this work was supported by JPL subcontract 1439002. Hannakaisa Lindqvist is supported by the Academy of Finland (project 331829). Aronne Merrelli's contributions to this work were supported by JPL subcontract 1577173. Paul I. Palmer and Liang Feng were supported by the UK National Centre for Earth Observation funded the National Environment Research Council (NE/R016518/1). The TCCON stations at Rikubetsu, Tsukuba, and Burgos are supported in part by the GOSAT series project. Local support for Burgos is provided by the Energy Development Corporation (EDC, Philippines). The TCCON site at Réunion has been operated by the Royal Belgian Institute for Space Aeronomy with financial support since 2014 by the EU project ICOS-Inwire and the ministerial decree for ICOS (FR/35/IC1 to FR/35/C6) and local activities supported by LACy/UMR8105 and by OSU-R/UMS3365 – Université de La Réunion. The TCCON stations at Garmisch and Zugspitze have been supported by the European Space Agency (ESA) under grant 4000120088/17/I-EF and by the German Bundesministerium für Wirtschaft und Energie (BMWi) via the DLR under grant 50EE1711D as well as by the Helmholtz Society via the research program ATMO. The observations in Bremen are supported by the German Bundesministerium für Wirtschaft und Energie (BMWi) via the DLR under grant 50EE1711B. The Paris TCCON site has received funding from Sorbonne Université, the French research center CNRS, the French space agency CNES, and Région Île-de-France. The Ascension Island TCCON station has been supported by the European Space Agency (ESA) under grant 4000120088/17/I-EF and by the German Bundesministerium für Wirtschaft und Energie (BMWi) under grants 50EE1711C and 50EE1711E. We thank the ESA Ariane Tracking Station at North East Bay, Ascension Island, for hosting and local support. The Anmyeondo TCCON station was funded by the Korea Meteorological Administration Research and Development Program “Development of Monitoring and Analysis Techniques for Atmospheric Composition in Korea” under grant no. KMA 2018-00522. Nicholas M. Deutscher is supported by an Australian Research Council (ARC) Future Fellowship, FT180100327. The Darwin and Wollongong TCCON sites have been supported by a series of ARC grants, including DP160100598, DP140100552, DP110103118, DP0879468 and LE0668470, and NASA grants NAG5-12247 and NNG05-GD07G. The Eureka measurements were made at the Polar Environment Atmospheric Research Laboratory (PEARL) by the Canadian Network for the Detection of Atmospheric Change (CANDAC), primarily supported by the Natural Sciences and Engineering Research Council of Canada, Environment and Climate Change Canada, and the Canadian Space Agency. Manvendra K. Dubey thanks the LANL LDRD program for support operating the Four Corners TCCON site. The TCCON Nicosia site has received additional support from the European Union's Horizon 2020 research and innovation program under grant agreement no. 856612 and the Cyprus Government, and by the University of Bremen. The Anmyeondo TCCON station is funded by the Korea Meteorological Administration Research and Development Program “Development of Monitoring and Analysis Techniques for Atmospheric Composition in Korea” under grant no. KMA 2018-00522.
This paper was edited by David Carlson and reviewed by two anonymous referees.
Basu, S., Guerlet, S., Butz, A., Houweling, S., Hasekamp, O., Aben, I., Krummel, P., Steele, P., Langenfelds, R., Torn, M., Biraud, S., Stephens, B., Andrews, A., and Worthy, D.: Global CO2 fluxes estimated from GOSAT retrievals of total column CO2, Atmos. Chem. Phys., 13, 8695–8717, https://doi.org/10.5194/acp-13-8695-2013, 2013. a
Blumenstock, T., Hase, F., Schneider, M., Garcia, O. E., and Sepulveda, E.: TCCON data from Izaña (ES), Release GGG2014R1, TCCON data archive, hosted by CaltechDATA [data set], https://doi.org/10.14291/TCCON.GGG2014.IZANA01.R1, 2017. a
Buchwitz, M., Reuter, M., Schneising, O., Noël, S., Gier, B., Bovensmann, H., Burrows, J. P., Boesch, H., Anand, J., Parker, R. J., Somkuti, P., Detmers, R. G., Hasekamp, O. P., Aben, I., Butz, A., Kuze, A., Suto, H., Yoshida, Y., Crisp, D., and O'Dell, C.: Computation and analysis of atmospheric carbon dioxide annual mean growth rates from satellite observations during 2003–2016, Atmos. Chem. Phys., 18, 17355–17370, https://doi.org/10.5194/acp-18-17355-2018, 2018. a
Butz, A., Guerlet, S., Hasekamp, O., Schepers, D., Galli, A., Aben, I., Frankenberg, C., Hartmann, J., Tran, H., Kuze, A., Aleks, G. K., Toon, G., Wunch, D., Wennberg, P., Deutscher, N., Griffith, D., Macatangay, R., Messerschmidt, J., Notholt, J., and Warneke, T.: Toward accurate CO2 and CH4 observations from GOSAT, Geophys. Res. Lett., 38, L14812, https://doi.org/10.1029/2011GL047888, 2011. a
Byrne, B., Liu, J., Lee, M., Baker, I., Bowman, K. W., Deutscher, N. M., Feist, D. G., Griffith, D. W. T., Iraci, L. T., Kiel, M., Kimball, J. S., Miller, C. E., Morino, I., Parazoo, N. C., Petri, C., Roehl, C. M., Sha, M. K., Strong, K., Velazco, V. A., Wennberg, P. O., and Wunch, D.: Improved constraints on northern extratropical CO2 fluxes obtained by combining surface-based and space-based atmospheric CO2 measurements, J. Geophys. Res.-Atmos., 125, e2019JD032 029, https://doi.org/10.1029/2019JD032029, 2020. a
CAMS: Copernicus Atmosphere Monitoring Service, available at: https://atmosphere.copernicus.eu/sites/default/files/2019-08/CAMS73_2018SC1_D73.1.4.1-2018-v1_201907_v1.pdf (last access: 10 January 2022), 2021. a, b
CarbonTracker: CarbonTracker, available at: https://carbontracker.noaa.gov (last access: 10 January 2022), 2021. a, b
CarboScope: CarboScope, available at: http://www.bgc-jena.mpg.de/CarboScope (last access: 10 January 2022), 2021. a, b
Chatterjee, A., Engelen, R. J., Kawa, S. R., Sweeney, C., and Michalak, A. M.: Background error covariance estimation for atmospheric CO2 data assimilation, J. Geophys. Res.-Atmos., 118, 10140–10154, https://doi.org/10.1002/jgrd.50654, 2013. a, b
Chatterjee, A., Gierach, M. M., Sutton, A. J., Feely, R. A., Crisp, D., Eldering, A., Gunson, M. R., O'Dell, C. W., Stephens, B. B., and Schimel, D. S.: Influence of El Niño on atmospheric CO2 over the tropical Pacific Ocean: Findings from NASA's OCO-2 mission, Science, 358, eaam5776, https://doi.org/10.1126/science.aam5776, 2017. a, b
Chevallier, F., Ciais, P., Conway, T. J., Aalto, T., Anderson, B. E., Bousquet, P., Brunke, E. G., Ciattaglia, L., Esaki, Y., Fröhlich, M., Gomez, A., Gomez-Pelaez, A. J., Haszpra, L., Krummel, P. B., Langenfelds, R. L., Leuenberger, M., Machida, T., Maignan, F., Matsueda, H., Morguí, J. A., Mukai, H., Nakazawa, T., Peylin, P., Ramonet, M., Rivier, L., Sawa, Y., Schmidt, M., Steele, L. P., Vay, S. A., Vermeulen, A. T., Wofsy, S., and Worthy, D.: CO2 surface fluxes at grid point scale estimated from a global 21 year reanalysis of atmospheric measurements, J. Geophys. Res.-Atmos., 115, D21307, https://doi.org/10.1029/2010JD013887, 2010. a
Chevallier, F., Palmer, P. I., Feng, L., Boesch, H., O'Dell, C. W., and Bousquet, P.: Toward robust and consistent regional CO2 flux estimates from in situ and spaceborne measurements of atmospheric CO2, Geophys. Res. Lett., 41, 1065–1070, https://doi.org/10.1002/2013GL058772, 2014. a
Ciais, P., Tan, J., Wang, X., Roedenbeck, C., Chevallier, F., Piao, S.-L., Moriarty, R., Broquet, G., LeQuéré, C., Canadell, J., Peng, S., Poulter, B., Liu, Z., and Tans, P.: Five decades of northern land carbon uptake revealed by the interhemispheric CO2 gradient, Nature, 568, 221–225, https://doi.org/10.1038/s41586-019-1078-6, 2019. a
Cogan, A. J., Boesch, H., Parker, R. J., Feng, L., Palmer, P. I., Blavier, J.-F. L., Deutscher, N. M., Macatangay, R., Notholt, J., Roehl, C., Warneke, T., and Wunch, D.: Atmospheric carbon dioxide retrieved from the Greenhouse gases Observing SATellite (GOSAT): Comparison with ground-based TCCON observations and GEOS-Chem model calculations, J. Geophys. Res.-Atmos., 117, D21301, https://doi.org/10.1029/2012JD018087, 2012. a
Crisp, D., Fisher, B. M., O'Dell, C., Frankenberg, C., Basilio, R., Bösch, H., Brown, L. R., Castano, R., Connor, B., Deutscher, N. M., Eldering, A., Griffith, D., Gunson, M., Kuze, A., Mandrake, L., McDuffie, J., Messerschmidt, J., Miller, C. E., Morino, I., Natraj, V., Notholt, J., O'Brien, D. M., Oyafuso, F., Polonsky, I., Robinson, J., Salawitch, R., Sherlock, V., Smyth, M., Suto, H., Taylor, T. E., Thompson, D. R., Wennberg, P. O., Wunch, D., and Yung, Y. L.: The ACOS CO2 retrieval algorithm – Part II: Global XCO2 data characterization, Atmos. Meas. Tech., 5, 687–707, https://doi.org/10.5194/amt-5-687-2012, 2012. a, b, c, d
Crisp, D., Pollock, H. R., Rosenberg, R., Chapsky, L., Lee, R. A. M., Oyafuso, F. A., Frankenberg, C., O'Dell, C. W., Bruegge, C. J., Doran, G. B., Eldering, A., Fisher, B. M., Fu, D., Gunson, M. R., Mandrake, L., Osterman, G. B., Schwandner, F. M., Sun, K., Taylor, T. E., Wennberg, P. O., and Wunch, D.: The on-orbit performance of the Orbiting Carbon Observatory-2 (OCO-2) instrument and its radiometrically calibrated products, Atmos. Meas. Tech., 10, 59–81, https://doi.org/10.5194/amt-10-59-2017, 2017. a
De Mazière, M., Sha, M. K., Desmet, F., Hermans, C., Scolas, F., Kumps, N., Metzger, J.-M., Duflot, V., and Cammas, J.-P.: TCCON data from Réunion Island (RE), Release GGG2014.R1, TCCON data archive, hosted by CaltechDATA [data set], https://doi.org/10.14291/TCCON.GGG2014.REUNION01.R1, 2017. a
Deng, F., Jones, D. B. A., Henze, D. K., Bousserez, N., Bowman, K. W., Fisher, J. B., Nassar, R., O'Dell, C., Wunch, D., Wennberg, P. O., Kort, E. A., Wofsy, S. C., Blumenstock, T., Deutscher, N. M., Griffith, D. W. T., Hase, F., Heikkinen, P., Sherlock, V., Strong, K., Sussmann, R., and Warneke, T.: Inferring regional sources and sinks of atmospheric CO2 from GOSAT XCO2 data, Atmos. Chem. Phys., 14, 3703–3727, https://doi.org/10.5194/acp-14-3703-2014, 2014. a
Deutscher, N. M., Notholt, J., Messerschmidt, J., Weinzierl, C., Warneke, T., Petri, C., and Grupe, P.: TCCON data from Bialystok (PL), Release GGG2014.R2, TCCON data archive, CaltechDATA [data set], https://doi.org/10.14291/TCCON.GGG2014.BIALYSTOK01.R2, 2019. a
Dlugokencky, E. and Tans, P.: Trends in atmospheric carbon dioxide, NOAA/GML, available at: http://gml.noaa.gov/ccgg/trends/ (last access: 10 January 2022), 2021. a
Dubey, M. K., Lindenmaier, R., Henderson, B. G., Green, D., Allen, N. T., Roehl, C. M., Blavier, J.-F., Butterfield, Z. T., Love, S., Hamelmann, J. D., and Wunch, D.: TCCON data from Four Corners (US), Release GGG2014.R0, TCCON data archive, CaltechDATA [data set], https://doi.org/10.14291/TCCON.GGG2014.FOURCORNERS01.R0/1149272, 2014. a
Eldering, A.: ACOS GOSAT v9 Super Lite XCO2 data (1.0), CaltechDATA [data set], https://doi.org/10.22002/D1.2178, 2021. a, b
Eldering, A., O'Dell, C. W., Wennberg, P. O., Crisp, D., Gunson, M. R., Viatte, C., Avis, C., Braverman, A., Castano, R., Chang, A., Chapsky, L., Cheng, C., Connor, B., Dang, L., Doran, G., Fisher, B., Frankenberg, C., Fu, D., Granat, R., Hobbs, J., Lee, R. A. M., Mandrake, L., McDuffie, J., Miller, C. E., Myers, V., Natraj, V., O'Brien, D., Osterman, G. B., Oyafuso, F., Payne, V. H., Pollock, H. R., Polonsky, I., Roehl, C. M., Rosenberg, R., Schwandner, F., Smyth, M., Tang, V., Taylor, T. E., To, C., Wunch, D., and Yoshimizu, J.: The Orbiting Carbon Observatory-2: first 18 months of science data products, Atmos. Meas. Tech., 10, 549–563, https://doi.org/10.5194/amt-10-549-2017, 2017. a
Feist, D. G., Arnold, S. G., John, N., and Geibel, M. C.: TCCON data from Ascension Island (SH), Release GGG2014.R0, TCCON data archive, CaltechDATA [data set], https://doi.org/10.14291/TCCON.GGG2014.ASCENSION01.R0/1149285, 2014. a
Feng, L., Palmer, P. I., Bösch, H., and Dance, S.: Estimating surface CO2 fluxes from space-borne CO2 dry air mole fraction observations using an ensemble Kalman Filter, Atmos. Chem. Phys., 9, 2619–2633, https://doi.org/10.5194/acp-9-2619-2009, 2009. a
Feng, L., Palmer, P. I., Parker, R. J., Deutscher, N. M., Feist, D. G., Kivi, R., Morino, I., and Sussmann, R.: Estimates of European uptake of CO2 inferred from GOSAT XCO2 retrievals: sensitivity to measurement bias inside and outside Europe, Atmos. Chem. Phys., 16, 1289–1302, https://doi.org/10.5194/acp-16-1289-2016, 2016. a
Frankenberg, C., Platt, U., and Wagner, T.: Iterative maximum a posteriori (IMAP)-DOAS for retrieval of strongly absorbing trace gases: Model studies for CH4 and CO2 retrieval from near infrared spectra of SCIAMACHY onboard ENVISAT, Atmos. Chem. Phys., 5, 9–22, https://doi.org/10.5194/acp-5-9-2005, 2005. a
Frankenberg, C., Pollock, R., Lee, R. A. M., Rosenberg, R., Blavier, J.-F., Crisp, D., O'Dell, C. W., Osterman, G. B., Roehl, C., Wennberg, P. O., and Wunch, D.: The Orbiting Carbon Observatory (OCO-2): spectrometer performance evaluation using pre-launch direct sun measurements, Atmos. Meas. Tech., 8, 301–313, https://doi.org/10.5194/amt-8-301-2015, 2015. a
Goo, T.-Y., Oh, Y.-S., and Velazco, V. A.: TCCON data from Anmeyondo (KR), Release GGG2014.R0, TCCON data archive, CaltechDATA [data set], https://doi.org/10.14291/TCCON.GGG2014.ANMEYONDO01.R0/1149284, 2014. a
Griffith, D. W., Deutscher, N. M., Velazco, V. A., Wennberg, P. O., Yavin, Y., Aleks, G. K., Washenfelder, R. a., Toon, G. C., Blavier, J.-F., Murphy, C., Jones, N., Kettlewell, G., Connor, B. J., Macatangay, R., Roehl, C., Ryczek, M., Glowacki, J., Culgan, T., and Bryant, G.: TCCON data from Darwin (AU), Release GGG2014R0, TCCON data archive, CaltechDATA [data set], https://doi.org/10.14291/tccon.ggg2014.darwin01.R0/1149290, 2014a. a
Griffith, D. W., Velazco, V. A., Deutscher, N. M., Murphy, C., Jones, N., Wilson, S., Macatangay, R., Kettlewell, G., Buchholz, R. R., and Riggenbach, M.: TCCON data from Wollongong (AU), Release GGG2014R0, TCCON data archive, CaltechDATA [data set], https://doi.org/10.14291/tccon.ggg2014.wollongong01.R0/1149291, 2014b. a
Gruber, N., Landschützer, P., and Lovenduski, N. S.: The variable southern ocean carbon sink, Ann. Rev. Mar. Sci., 11, 159–186, https://doi.org/10.1146/annurev-marine-121916-063407, 2019. a
Hakkarainen, J., Ialongo, I., Maksyutov, S., and Crisp, D.: Analysis of four years of global XCO2 anomalies as seen by Orbiting Carbon Observatory-2, Remote Sensing, 11, 850, https://doi.org/10.3390/rs11070850, 2019. a
Hase, F., Blumenstock, T., Dohe, S., Gross, J., and Kiel, M.: TCCON data from Karlsruhe (DE), Release GGG2014R1, TCCON data archive, CaltechDATA [data set], https://doi.org/10.14291/tccon.ggg2014.karlsruhe01.R1/1182416, 2015. a
Heymann, J., Reuter, M., Hilker, M., Buchwitz, M., Schneising, O., Bovensmann, H., Burrows, J. P., Kuze, A., Suto, H., Deutscher, N. M., Dubey, M. K., Griffith, D. W. T., Hase, F., Kawakami, S., Kivi, R., Morino, I., Petri, C., Roehl, C., Schneider, M., Sherlock, V., Sussmann, R., Velazco, V. A., Warneke, T., and Wunch, D.: Consistent satellite XCO2 retrievals from SCIAMACHY and GOSAT using the BESD algorithm, Atmos. Meas. Tech., 8, 2961–2980, https://doi.org/10.5194/amt-8-2961-2015, 2015. a
Houweling, S., Baker, D., Basu, S., Boesch, H., Butz, A., Chevallier, F., Deng, F., Dlugokencky, E. J., Feng, L., Ganshin, A., Hasekamp, O., Jones, D., Maksyutov, S., Marshall, J., Oda, T., O'Dell, C. W., Oshchepkov, S., Palmer, P. I., Peylin, P., Poussi, Z., Reum, F., Takagi, H., Yoshida, Y., and Zhuravlev, R.: An intercomparison of inverse models for estimating sources and sinks of CO2 using GOSAT measurements, J. Geophys. Res.-Atmos., 120, 5253–5266, https://doi.org/10.1002/2014JD022962, 2015. a
Iraci, L. T., Podolske, J., Hillyard, P. W., Roehl, C., Wennberg, P. O., Blavier, J.-F., Allen, N., Wunch, D., Osterman, G. B., and Albertson, R.: TCCON data from Edwards (US), Release GGG2014R1, TCCON data archive, CaltechDATA [data set], https://doi.org/10.14291/tccon.ggg2014.edwards01.R1/1255068, 2016a. a
Iraci, L. T., Podolske, J. R., Hillyard, P. W., Roehl, C., Wennberg, P. O., Blavier, J.-F., Landeros, J., Allen, N., Wunch, D., Zavaleta, J., Quigley, E., Osterman, G. B., Barrow, E., and Barney, J.: TCCON data from Indianapolis (US), Release GGG2014.R1, TCCON data archive, CaltechDATA [data set], https://doi.org/10.14291/TCCON.GGG2014.INDIANAPOLIS01.R1/1330094, 2016b. a
Jiang, F., Wang, H., Chen, J. M., Ju, W., Tian, X., Feng, S., Li, G., Chen, Z., Zhang, S., Lu, X., Liu, J., Wang, H., Wang, J., He, W., and Wu, M.: Regional CO2 fluxes from 2010 to 2015 inferred from GOSAT XCO2 retrievals using a new version of the Global Carbon Assimilation System, Atmos. Chem. Phys., 21, 1963–1985, https://doi.org/10.5194/acp-21-1963-2021, 2021. a
Kataoka, F., Crisp, D., Taylor, T. E., O’Dell, C. W., Kuze, A., Shiomi, K., Suto, H., Bruegge, C., Schwandner, F. M., Rosenberg, R., Chapsky, L., and Lee, R. A. M.: The cross-calibration of spectral radiances and cross-validation of CO2 estimates from GOSAT and OCO-2, Remote Sensing, 9, 1158, https://doi.org/10.3390/rs9111158, 2017. a
Kawakami, S., Ohyama, H., Arai, K., Okumura, H., Taura, C., Fukamachi, T., and Sakashita, M.: TCCON data from Saga (JP), Release GGG2014R0, TCCON data archive, CaltechDATA [data set], https://doi.org/10.14291/tccon.ggg2014.saga01.R0/1149283, 2014. a
Keeling, C., Whorf, T., Wahlen, M., and van der Plichtt, J.: Interannual extremes in the rate of rise of atmospheric carbon dioxide since 1980, Nature, 375, 666–670, https://doi.org/10.1038/375666a0, 1995. a
Kiel, M., O'Dell, C. W., Fisher, B., Eldering, A., Nassar, R., MacDonald, C. G., and Wennberg, P. O.: How bias correction goes wrong: measurement of XCO2 affected by erroneous surface pressure estimates, Atmos. Meas. Tech., 12, 2241–2259, https://doi.org/10.5194/amt-12-2241-2019, 2019. a, b, c, d, e, f, g
Kivi, R., Heikkinen, P., and Kyrö, E.: TCCON data from Sodankylä (FI), Release GGG2014.R0, TCCON data archive, CaltechDATA [data set], https://doi.org/10.14291/tccon.ggg2014.sodankyla01.R0/1149280, 2014. a
Kulawik, S., Wunch, D., O'Dell, C., Frankenberg, C., Reuter, M., Oda, T., Chevallier, F., Sherlock, V., Buchwitz, M., Osterman, G., Miller, C. E., Wennberg, P. O., Griffith, D., Morino, I., Dubey, M. K., Deutscher, N. M., Notholt, J., Hase, F., Warneke, T., Sussmann, R., Robinson, J., Strong, K., Schneider, M., De Mazière, M., Shiomi, K., Feist, D. G., Iraci, L. T., and Wolf, J.: Consistent evaluation of ACOS-GOSAT, BESD-SCIAMACHY, CarbonTracker, and MACC through comparisons to TCCON, Atmos. Meas. Tech., 9, 683–709, https://doi.org/10.5194/amt-9-683-2016, 2016. a
Kuze, A., Suto, H., Nakajima, M., and Hamazaki, T.: Thermal and near infrared sensor for carbon observation Fourier-transform spectrometer on the Greenhouse Gases Observing Satellite for greenhouse gases monitoring, Appl. Optics, 48, 6716–6733, https://doi.org/10.1364/AO.48.006716, 2009. a, b
Kuze, A., Suto, H., Shiomi, K., Kawakami, S., Tanaka, M., Ueda, Y., Deguchi, A., Yoshida, J., Yamamoto, Y., Kataoka, F., Taylor, T. E., and Buijs, H. L.: Update on GOSAT TANSO-FTS performance, operations, and data products after more than 6 years in space, Atmos. Meas. Tech., 9, 2445–2461, https://doi.org/10.5194/amt-9-2445-2016, 2016. a, b
Kuze, A., Kikuchi, N., Kataoka, F., Suto, H., Shiomi, K., and Kondo, Y.: Detection of Methane Emission from a Local Source Using GOSAT Target Observations, Remote Sensing, 12, https://doi.org/10.3390/rs12020267, 2020. a
Lindenmaier, R., Dubey, M. K., Henderson, B. G., Butterfield, Z. T., Herman, J. R., Rahn, T., and Lee, S.-H.: Multiscale observations of CO2, 13CO2, and pollutants at Four Corners for emission verification and attribution, P. Natl. Acad. Sci. USA, 111, 8386–8391, https://doi.org/10.1073/pnas.1321883111, 2014. a
Lindqvist, H., O'Dell, C. W., Basu, S., Boesch, H., Chevallier, F., Deutscher, N., Feng, L., Fisher, B., Hase, F., Inoue, M., Kivi, R., Morino, I., Palmer, P. I., Parker, R., Schneider, M., Sussmann, R., and Yoshida, Y.: Does GOSAT capture the true seasonal cycle of carbon dioxide?, Atmos. Chem. Phys., 15, 13023–13040, https://doi.org/10.5194/acp-15-13023-2015, 2015. a, b, c, d, e, f
Liu, C., Wang, W., and Sun, Y.: TCCON data from Hefei (PRC), Release GGG2014.R0, TCCON data archive, CaltechDATA [data set], https://doi.org/10.14291/TCCON.GGG2014.HEFEI01.R0, 2018. a
Liu, J., Bowman, K. W., Schimel, D. S., Parazoo, N. C., Jiang, Z., Lee, M., Bloom, A. A., Wunch, D., Frankenberg, C., Sun, Y., O'Dell, C. W., Gurney, K. R., Menemenlis, D., Gierach, M., Crisp, D., and Eldering, A.: Contrasting carbon cycle responses of the tropical continents to the 2015–2016 El Niño, Science, 358, eaam5690, https://doi.org/10.1126/science.aam5690, 2017. a, b
Morino, I., Yokozeki, N., Matzuzaki, T., and Horikawa, M.: TCCON data from Rikubetsu (JP), Release GGG2014R1, TCCON data archive, CaltechDATA [data set], https://doi.org/10.14291/TCCON.GGG2014.RIKUBETSU01.R1/1242265, 2016. a
Morino, I., Matsuzaki, T., and Horikawa, M.: TCCON data from Tsukuba (JP), 125HR, Release GGG2014R2, TCCON data archive, CaltechDATA [data set], https://doi.org/10.14291/TCCON.GGG2014.TSUKUBA02.R2, 2018a. a
Morino, I., Velazco, V. A., Hori, A., Uchino, O., and Griffith, D. W. T.: TCCON data from Burgos, Ilocos Norte (PH), Release GGG2014.R0, TCCON data archive, CaltechDATA [data set], https://doi.org/10.14291/TCCON.GGG2014.BURGOS01.R0, 2018b. a
Müller, A., Tanimoto, H., Sugita, T., Machida, T., Nakaoka, S., Patra, P. K., Laughner, J., and Crisp, D.: New approach to evaluate satellite-derived XCO2 over oceans by integrating ship and aircraft observations, Atmos. Chem. Phys., 21, 8255–8271, https://doi.org/10.5194/acp-21-8255-2021, 2021. a
Nelson, R. R. and O'Dell, C. W.: The impact of improved aerosol priors on near-infrared measurements of carbon dioxide, Atmos. Meas. Tech., 12, 1495–1512, https://doi.org/10.5194/amt-12-1495-2019, 2019. a
Nguyen, H., Osterman, G., Wunch, D., O'Dell, C., Mandrake, L., Wennberg, P., Fisher, B., and Castano, R.: A method for colocating satellite XCO2 data to ground-based data and its application to ACOS-GOSAT and TCCON, Atmos. Meas. Tech., 7, 2631–2644, https://doi.org/10.5194/amt-7-2631-2014, 2014. a
Notholt, J., Petri, C., Warneke, T., Deutscher, N. M., Palm, M., Buschmann, M., Weinzierl, C., Macatangay, R. C., and Grupe, P.: TCCON data from Bremen (DE), Release GGG2014.R1, TCCON data archive, CaltechDATA [data set], https://doi.org/10.14291/TCCON.GGG2014.BREMEN01.R1, 2019. a
OCO-2 Science Team, Gunson, M., and Eldering, A.: ACOS GOSAT/TANSO-FTS Level 2 bias-corrected XCO2 and other select fields from the full-physics retrieval aggregated as daily files V9r, Goddard Earth Sciences Data and Information Services Center (GES DISC) [data set], Greenbelt, MD, USA, https://doi.org/10.5067/VWSABTO7ZII4, 2019a. a, b, c, d
OCO-2 Science Team, Gunson, M., and Eldering, A.: ACOS GOSAT/TANSO-FTS Level 2 Full Physics Standard Product V9r, Goddard Earth Sciences Data and Information Services Center (GES DISC) [data set], Greenbelt, MD, USA, https://doi.org/10.5067/OSGTIL9OV0PN, 2019b. a, b, c, d
OCO-2 Science Team, Gunson, M., and Eldering, A.: OCO-2 Level 2 bias-corrected XCO2 and other select fields from the full-physics retrieval aggregated as daily files V10r, Goddard Earth Sciences Data and Information Services Center (GES DISC) [data set], Greenbelt, MD, USA, https://doi.org/10.5067/E4E140XDMPO2, 2020. a
O'Dell, C., Osterman, G., Eldering, A., Cheng, C., Crisp, D., Frankenberg, C., and Fisher, B.: Orbiting Carbon Observatory-2 & 3 Retrievals of carbon dioxide from GOSAT using the atmospheric CO2 observations from space (ACOS) algorithm; Level 2 standard product and lite data product data user's guide, v9, Tech. rep., Jet Propulsion Laboratory, available at: https://docserver.gesdisc.eosdis.nasa.gov/public/project/OCO/ACOS_v9_DataUsersGuide.pdf (last access: 10 January 2022), 2020. a, b, c, d, e
O'Dell, C. W., Connor, B., Bösch, H., O'Brien, D., Frankenberg, C., Castano, R., Christi, M., Eldering, D., Fisher, B., Gunson, M., McDuffie, J., Miller, C. E., Natraj, V., Oyafuso, F., Polonsky, I., Smyth, M., Taylor, T., Toon, G. C., Wennberg, P. O., and Wunch, D.: The ACOS CO2 retrieval algorithm – Part 1: Description and validation against synthetic observations, Atmos. Meas. Tech., 5, 99–121, https://doi.org/10.5194/amt-5-99-2012, 2012. a
O'Dell, C. W., Eldering, A., Wennberg, P. O., Crisp, D., Gunson, M. R., Fisher, B., Frankenberg, C., Kiel, M., Lindqvist, H., Mandrake, L., Merrelli, A., Natraj, V., Nelson, R. R., Osterman, G. B., Payne, V. H., Taylor, T. E., Wunch, D., Drouin, B. J., Oyafuso, F., Chang, A., McDuffie, J., Smyth, M., Baker, D. F., Basu, S., Chevallier, F., Crowell, S. M. R., Feng, L., Palmer, P. I., Dubey, M., García, O. E., Griffith, D. W. T., Hase, F., Iraci, L. T., Kivi, R., Morino, I., Notholt, J., Ohyama, H., Petri, C., Roehl, C. M., Sha, M. K., Strong, K., Sussmann, R., Te, Y., Uchino, O., and Velazco, V. A.: Improved retrievals of carbon dioxide from Orbiting Carbon Observatory-2 with the version 8 ACOS algorithm, Atmos. Meas. Tech., 11, 6539–6576, https://doi.org/10.5194/amt-11-6539-2018, 2018. a, b, c, d, e, f, g, h, i, j, k
Osterman, G., O'Dell, C., Eldering, A., Fisher, B., Crisp, D., Cheng, C., Frankenberg, C., Lambert, A., Gunson, M., Mandrake, L., and Wunch, D.: Orbiting Carbon Observatory-2 & 3 Data Product User's Guide, v10, Tech. rep., Jet Propulsion Laboratory, available at: https://docserver.gesdisc.eosdis.nasa.gov/public/project/OCO/OCO2_OCO3_B10_DUG.pdf (last access: 10 January 2022), 2020. a
Oyafuso, F., Payne, V. H., Drouin, B. J., Devi, V. M., Benner, D. C., Sung, K., Yu, S., Gordon, I. E., Kochanov, R., Tan, Y., Crisp, D., Mlawer, E. J., and Guillaume, A.: High accuracy absorption coefficients for the Orbiting Carbon Observatory-2 (OCO-2) mission: Validation of updated carbon dioxide cross-sections using atmospheric spectra, J. Quant. Spectrosc. Ra., 203, 213–223, https://doi.org/10.1016/j.jqsrt.2017.06.012, 2017. a
Palmer, P. I., Feng, L., Baker, D., Chevallier, F., Bösch, H., and Somkuti, P.: Net carbon emissions from African biosphere dominate pan-tropical atmospheric CO2 signal, Nat. Commun., 10, 3344, https://doi.org/10.1038/s41467-019-11097-w, 2019. a, b
Payne, V. H., Drouin, B. J., Oyafuso, F., Kuai, L., Fisher, B. M., Sung, K., Nemchick, D., Crawford, T. J., Smyth, M., Crisp, D., Adkins, E., Hodges, J. T., Long, D. A., Mlawer, E. J., Merrelli, A., Lunny, E., and O’Dell, C. W.: Absorption coefficient (ABSCO) tables for the Orbiting Carbon Observatories: Version 5.1, J. Quant. Spectrosc. Ra., 255, 107217, https://doi.org/10.1016/j.jqsrt.2020.107217, 2020. a
Peters, W., Jacobson, A. R., Sweeney, C., Andrews, A. E., Conway, T. J., Masarie, K., Miller, J. B., Bruhwiler, L. M. P., Pétron, G., Hirsch, A. I., Worthy, D. E. J., van der Werf, G. R., Randerson, J. T., Wennberg, P. O., Krol, M. C., and Tans, P. P.: An atmospheric perspective on North American carbon dioxide exchange: CarbonTracker, P. Natl. Acad. Sci. USA, 104, 18925–18930, https://doi.org/10.1073/pnas.0708986104, 2007. a
Petri, C., Vrekoussis, M., Rousogenous, C., Warneke, T., Sciare, J., and Notholt, J.: TCCON data from Nicosia (CY), Release GGG2014R0, TCCON data archive, CaltechDATA [data set], https://doi.org/10.14291/tccon.ggg2014.nicosia01.R0, 2020. a
Pollard, D. F., Robinson, J., and Shiona, H.: TCCON data from Lauder (NZ), Release GGG2014.R0, TCCON data archive, CaltechDATA [data set], https://doi.org/10.14291/TCCON.GGG2014.LAUDER03.R0, 2019. a
Rienecker, M. M., Suarez, M. J., Gelaro, R., Todling, R., Bacmeister, J., Liu, E., Bosilovich, M. G., Schubert, S. D., Takacs, L., Kim, G.-K., Bloom, S., Chen, J., Collins, D., Conaty, A., da Silva, A., Gu, W., Joiner, J., Koster, R. D., Lucchesi, R., Molod, A., Owens, T., Pawson, S., Pegion, P., Redder, C. R., Reichle, R., Robertson, F. R., Ruddick, A. G., Sienkiewicz, M., and Woollen, J.: MERRA: NASA’s Modern-Era Retrospective Analysis for Research and Applications, J. Climate, 24, 3624–3648, https://doi.org/10.1175/JCLI-D-11-00015.1, 2011. a
Rödenbeck, C.: Estimating CO2 sources and sinks from atmospheric mixing ratio measurements using a global inversion of atmospheric transport, Technical Report 6, Max Planck Institute for Biogeochemistry, Jena, 2005. a
Rödenbeck, C., Zaehle, S., Keeling, R., and Heimann, M.: How does the terrestrial carbon exchange respond to inter-annual climatic variations? A quantification based on atmospheric CO2 data, Biogeosciences, 15, 2481–2498, https://doi.org/10.5194/bg-15-2481-2018, 2018. a
Strong, K., Roche, S., Franklin, J. E., Mendonca, J., Lutsch, E., Weaver, D., Fogal, P. F., Drummond, J. R., Batchelor, R., and Lindenmaier, R.: TCCON data from Eureka (CA), Release GGG2014.R3, TCCON data archive, CaltechDATA [data set], https://doi.org/10.14291/TCCON.GGG2014.EUREKA01.R3, 2019. a
Sussmann, R. and Rettinger, M.: TCCON data from Garmisch (DE), Release GGG2014R2, TCCON data archive, CaltechDATA [data set], https://doi.org/10.14291/TCCON.GGG2014.GARMISCH01.R2, 2018a. a
Sussmann, R. and Rettinger, M.: TCCON data from Zugspitze (DE), Release GGG2014.R1, TCCON data archive, CaltechDATA [data set], https://doi.org/10.14291/TCCON.GGG2014.ZUGSPITZE01.R1, 2018b. a
Taylor, T. E., O'Dell, C. W., Frankenberg, C., Partain, P. T., Cronk, H. Q., Savtchenko, A., Nelson, R. R., Rosenthal, E. J., Chang, A. Y., Fisher, B., Osterman, G. B., Pollock, R. H., Crisp, D., Eldering, A., and Gunson, M. R.: Orbiting Carbon Observatory-2 (OCO-2) cloud screening algorithms: validation against collocated MODIS and CALIOP data, Atmos. Meas. Tech., 9, 973–989, https://doi.org/10.5194/amt-9-973-2016, 2016. a, b
Te, Y., Jeseck, P., and Janssen, C.: TCCON data from Paris (FR), Release GGG2014R0, TCCON data archive, CaltechDATA [data set], https://doi.org/10.14291/tccon.ggg2014.paris01.R0/1149279, 2014. a
Thompson, D. R., , Benner, D. C., Brown, L. R., Crisp, D., Devi, V. M., Jiang, Y., Natraj, V., Oyafuso, F., Sung, K., Wunch, D., Castano, R., and Miller, C. E.: Atmospheric validation of high accuracy CO2 absorption coefficients for the OCO-2 mission, J. Quant. Spectrosc. Ra., 113, 2265–2276, https://doi.org/10.1016/j.jqsrt.2012.05.021, 2012. a
UoE: UoE, available at: https://www.geos.ed.ac.uk/~lfeng/otool.pdf (last access: 10 January 2022), 2021. a, b
Wang, H., Jiang, F., Wang, J., Ju, W., and Chen, J. M.: Terrestrial ecosystem carbon flux estimated using GOSAT and OCO-2 XCO2 retrievals, Atmos. Chem. Phys., 19, 12067–12082, https://doi.org/10.5194/acp-19-12067-2019, 2019. a
Warneke, T., Messerschmidt, J., Notholt, J., Weinzierl, C., Deutscher, N. M., Petri, C., and Grupe, P.: TCCON data from Orléans (FR), Release GGG2014R1, TCCON data archive, CaltechDATA [data set], https://doi.org/10.14291/TCCON.GGG2014.ORLEANS01.R1, 2019. a
Wennberg, P. O., Wunch, D., Roehl, C., Blavier, J.-F., Toon, G. C., and Allen, N.: TCCON data from Caltech (US), Release GGG2014R1, TCCON data archive, CaltechDATA [data set], https://doi.org/10.14291/tccon.ggg2014.pasadena01.R1/1182415, 2015. a
Wennberg, P. O., Roehl, C. M., Blavier, J.-F., Wunch, D., and Allen, N. T.: TCCON data from Jet Propulsion Laboratory (US), 2011, Release GGG2014.R1, TCCON data archive, CaltechDATA [data set], https://doi.org/10.14291/TCCON.GGG2014.JPL02.R1/1330096, 2016a. a, b
Wennberg, P. O., Wunch, D., Roehl, C., Blavier, J.-F., Toon, G. C., Allen, N., Dowell, P., Teske, K., Martin, C., and Martin., J.: TCCON data from Lamont (US), Release GGG2014R1, TCCON data archive, CaltechDATA [data set], https://doi.org/10.14291/tccon.ggg2014.lamont01.R1/1255070, 2016b. a
Wennberg, P. O., Roehl, C., Wunch, D., Toon, G. C., Blavier, J.-F., Washenfelder, R. a., Keppel-Aleks, G., Allen, N., and Ayers, J.: TCCON data from Park Falls (US), Release GGG2014R1, TCCON data archive, CaltechDATA [data set], https://doi.org/10.14291/TCCON.GGG2014.PARKFALLS01.R1, 2017. a
Wunch, D., Toon, G. C., Blavier, J.-F. L., Washenfelder, R. A., Notholt, J., Connor, B. J., Griffith, D. W. T., Sherlock, V., and Wennberg, P. O.: The Total Carbon Column Observing Network, Philos. T. Roy. Soc. A, 369, 2087–2112, https://doi.org/10.1098/rsta.2010.0240, 2011a. a
Wunch, D., Wennberg, P. O., Toon, G. C., Connor, B. J., Fisher, B., Osterman, G. B., Frankenberg, C., Mandrake, L., O'Dell, C., Ahonen, P., Biraud, S. C., Castano, R., Cressie, N., Crisp, D., Deutscher, N. M., Eldering, A., Fisher, M. L., Griffith, D. W. T., Gunson, M., Heikkinen, P., Keppel-Aleks, G., Kyrö, E., Lindenmaier, R., Macatangay, R., Mendonca, J., Messerschmidt, J., Miller, C. E., Morino, I., Notholt, J., Oyafuso, F. A., Rettinger, M., Robinson, J., Roehl, C. M., Salawitch, R. J., Sherlock, V., Strong, K., Sussmann, R., Tanaka, T., Thompson, D. R., Uchino, O., Warneke, T., and Wofsy, S. C.: A method for evaluating bias in global measurements of CO2 total columns from space, Atmos. Chem. Phys., 11, 12317–12337, https://doi.org/10.5194/acp-11-12317-2011, 2011b. a
Wunch, D., Toon, G. C., Sherlock, V., Deutscher, N. M., Liu, C., Feist, D. G., and Wennberg, P. O.: Documentation for the 2014 TCCON Data Release, Tech. rep., CaltechDATA, available at: https://doi.org/10.14291/TCCON.GGG2014.DOCUMENTATION.R0/1221662, 2015. a, b
Wunch, D., Mendonca, J., Colebatch, O., Allen, N. T., Blavier, J.-F., Springett, S., Neufeld, G., Strong, K., Kessler, R., and Worthy, D.: TCCON data from East Trout Lake, SK (CA), Release GGG2014.R0, TCCON data archive, CaltechDATA [data set], https://doi.org/10.14291/TCCON.GGG2014.EASTTROUTLAKE01.R0/1348207, 2017a. a
Wunch, D., Wennberg, P. O., Osterman, G., Fisher, B., Naylor, B., Roehl, C. M., O'Dell, C., Mandrake, L., Viatte, C., Kiel, M., Griffith, D. W. T., Deutscher, N. M., Velazco, V. A., Notholt, J., Warneke, T., Petri, C., De Maziere, M., Sha, M. K., Sussmann, R., Rettinger, M., Pollard, D., Robinson, J., Morino, I., Uchino, O., Hase, F., Blumenstock, T., Feist, D. G., Arnold, S. G., Strong, K., Mendonca, J., Kivi, R., Heikkinen, P., Iraci, L., Podolske, J., Hillyard, P. W., Kawakami, S., Dubey, M. K., Parker, H. A., Sepulveda, E., García, O. E., Te, Y., Jeseck, P., Gunson, M. R., Crisp, D., and Eldering, A.: Comparisons of the Orbiting Carbon Observatory-2 (OCO-2) XCO2 measurements with TCCON, Atmos. Meas. Tech., 10, 2209–2238, https://doi.org/10.5194/amt-10-2209-2017, 2017b. a, b
Yoshida, Y., Ota, Y., Eguchi, N., Kikuchi, N., Nobuta, K., Tran, H., Morino, I., and Yokota, T.: Retrieval algorithm for CO2 and CH4 column abundances from short-wavelength infrared spectral observations by the Greenhouse gases observing satellite, Atmos. Meas. Tech., 4, 717–734, https://doi.org/10.5194/amt-4-717-2011, 2011. a
Yoshida, Y., Kikuchi, N., Morino, I., Uchino, O., Oshchepkov, S., Bril, A., Saeki, T., Schutgens, N., Toon, G. C., Wunch, D., Roehl, C. M., Wennberg, P. O., Griffith, D. W. T., Deutscher, N. M., Warneke, T., Notholt, J., Robinson, J., Sherlock, V., Connor, B., Rettinger, M., Sussmann, R., Ahonen, P., Heikkinen, P., Kyrö, E., Mendonca, J., Strong, K., Hase, F., Dohe, S., and Yokota, T.: Improvement of the retrieval algorithm for GOSAT SWIR XCO2 and XCH4 and their validation using TCCON data, Atmos. Meas. Tech., 6, 1533–1547, https://doi.org/10.5194/amt-6-1533-2013, 2013. a, b
- Abstract
- Introduction
- The GOSAT instrument and measurements
- The ACOS v9 L2FP XCO2 retrieval algorithm
- Evaluation of ACOS GOSAT v9 XCO2
- Data availability
- Summary
- Appendix A: Seasonal cycle plots of ACOS GOSAT v9 XCO2 versus TCCON for individual stations
- Author contributions
- Competing interests
- Disclaimer
- Acknowledgements
- Financial support
- Review statement
- References
- Abstract
- Introduction
- The GOSAT instrument and measurements
- The ACOS v9 L2FP XCO2 retrieval algorithm
- Evaluation of ACOS GOSAT v9 XCO2
- Data availability
- Summary
- Appendix A: Seasonal cycle plots of ACOS GOSAT v9 XCO2 versus TCCON for individual stations
- Author contributions
- Competing interests
- Disclaimer
- Acknowledgements
- Financial support
- Review statement
- References