the Creative Commons Attribution 4.0 License.
the Creative Commons Attribution 4.0 License.
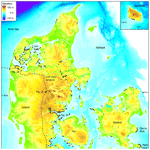
A national landslide inventory for Denmark
Anders A. Bjørk
Marie Keiding
Aart Kroon
Landslides are a frequent natural hazard occurring globally in regions with steep topography. Additionally, landslides play an important role in landscape evolution by transporting sediment downslope. Landslide inventory mapping is a common technique to assess the spatial distribution and extent of landslides in an area of interest. High-resolution digital elevation models (DEMs) have proven to be useful databases to map landslides in large areas across different land covers and topography. So far, Denmark had no national landslide inventory. Here, we create the first comprehensive national landslide inventory for Denmark derived from a 40 cm resolution DEM from 2015 supported by several 12.5 cm resolution orthophotos. The landslide inventory is created based on a manual expert-based mapping approach, and we implemented a quality control mechanism to assess the completeness of the inventory. Overall, we mapped 3202 landslide polygons in Denmark with a level of completeness of 87 %. The complete landslide inventory is freely available for download at https://doi.org/10.6084/m9.figshare.16965439.v2 (Svennevig and Luetzenburg, 2021) or as a web map (https://data.geus.dk/landskred/, last access: 6 June 2022) for further investigations.
- Article
(17913 KB) - Full-text XML
- BibTeX
- EndNote
Landslides can be a serious natural hazard, existing worldwide and causing high numbers of fatalities and damage to property every year (Froude and Petley, 2018). Identifying areas with frequent occurrences of landslides and designating areas with high landslide probabilities is important to protect human life and economic interest (Colombo et al., 2005; Ludwig et al., 2018). Under the generic term “landslide”, a variety of types can be distinguished based on the process and the material involved (Cruden and Varnes, 1996). Several landslide classifications exist that have been refined over the years (Highland and Bobrowsky, 2008; Hungr et al., 2014). When investigating a landslide, gaining knowledge about the spatial occurrence of landslides can further improve our understanding of the underlying processes causing landslides (Malamud et al., 2004).
The study of landslides reaches from site-specific field investigations to global datasets of landslides and from event-based inspections to long-term monitoring for several years (Alberti et al., 2020; Coe, 2020; Mateos et al., 2020; Svennevig et al., 2020a). Among the different spatial and temporal approaches of landslide studies, landslide inventory mapping is a common method to investigate the spatial occurrence of landslides (Guzzetti et al., 2012; Galli et al., 2008; Hao et al., 2020). Landslide inventory mapping can be performed remotely, covering large areas and with the option to validate the dataset in the field (Zieher et al., 2016). Traditionally, landslide inventories are based on aerial imagery and optical satellite images (Brardinoni et al., 2003; Fiorucci et al., 2011). With the emergence of digital elevation data and hill shading, the quality and quantity of landslide inventories have improved substantially (Morgan et al., 2013; Kakavas and Nikolakopoulos, 2021). New areas can be investigated (e.g., forests) and volumes of displaced mass can be calculated (Cavalli and Marchi, 2008). Landslide inventories often contain information about the landslide location, geometry, date of occurrence and damage caused by the landslide (Rosi et al., 2017; Palma et al., 2020).
National elevation mapping efforts and satellite campaigns are extending the areas that are covered by elevation models (Crosby, 2012; EEA, 2016). Advances in sensor technologies and satellite orbit repeat rates are improving the spatial and temporal resolution of the available data, both for optical images and elevation data (e.g., Shugar et al., 2021). Remote sensing data provide powerful information for landslide mapping, but a combination of different datasets such as digital elevation models (DEMs) and multispectral satellite images are necessary to overcome the limitations of each individual dataset (Lissak et al., 2020). The quality of manually mapped landslide inventories strongly depends on the mapping expert's knowledge about the area of investigation (Van Den Eeckhaut et al., 2005). Evaluating the quality of landslide inventories is not straightforward and most mapping efforts do not implement quality controls in their inventory (Guzzetti et al., 2012; Pellicani and Spilotro, 2014; Hao et al., 2020).
Landslide inventories exist on regional, national, international, and global scales (Kirschbaum et al., 2009; Trigila et al., 2010; Damm and Klose, 2015; Herrera et al., 2017). Within Europe, Denmark does not have a national landslide inventory, nor a legislation framework to incorporate landslides and landslide-related damages into national law (Mateos et al., 2020). Landslides are considered a predominant natural hazard in the Nordic countries (Nadim et al., 2008), and a number of case studies investigated landslides in Denmark (Hutchinson, 2002; Prior, 1977). Pedersen et al. (1989) state that Denmark is not a country with a serious landslide problem. However, a recent paper raised concerns that the geo-hazard posed by landslides in Denmark is underestimated (Svennevig et al., 2020b).
With this paper and dataset, we present the first comprehensive landslide inventory for Denmark.
Denmark consists of the Jutland peninsula and an archipelago of 394 islands encompassing 43 938 km2 in total, with 8750 km of coastline (Fig. 1). The landscape is characterized by a low relief with the highest point 171 m above sea level in central Jutland. A long history of agricultural land use has shaped the landscape. Today, around 61 % of the area is agriculturally used, 13 % are forests, another 13 % are transport routes and built up areas, and the remaining land is covered with open habitats and water bodies (Denmark, 2019).
Today's Danish landscape was shaped by numerous glaciations, dominated almost entirely by the two latest ones, the Saalian, ending ca. 130 kyr BP and the most recent Weichselian, ending ca. 16 kyr BP, which lead into the Holocene (Houmark-Nielsen, 1999, 2011). The current landscape configuration is primarily dominated by the last glacial maximum (LGM) extent reached during the Weichselian at ca. 22 kyr BP, where a glacial advance from the northeast reached mid-Jutland, leaving two distinct surface sedimentation regimes: (1) the ice-free west was dominated by sandy glacio-fluvial outwash plains surrounding older glacial deposits from the Saalian and (2) the ice overridden eastern part of Denmark was dominated by glacial processes depositing tills with a high clay content. The landscape here was mainly shaped during the LGM advance and the numerous re-advances up until ca. 16 kyr BP (Houmark-Nielsen, 1999, 2011). Postglacial isostatic rebound has affected especially the northern part of Denmark, which has been uplifted by up to 13 m relative to the local sea level, exposing raised beaches and marine terraces.
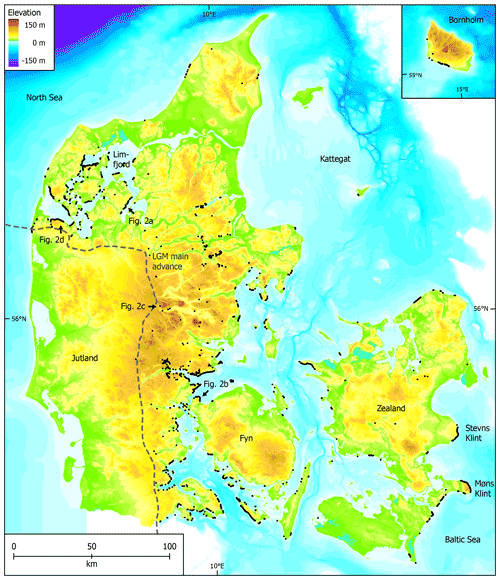
Figure 1Landslide inventory plotted over a land and sea elevation map of Denmark. The black dots show 3202 mapped landslides. The dashed line indicates the last glacial maximum (LGM) main advance from the northeast during the Weichsel glaciation (Houmark-Nielsen, 2011). Place names mentioned in the text along with positions of panels in Fig. 2 are shown.
Open waters occur in many places in Denmark (Fig. 1) and the glacial landscape is often eroded along its fringes by coastal processes. Waves induce large swash run-up on the beaches and cause erosion of the glacial landscape, forming coastal cliffs. These relatively steep cliffs are susceptible to landslides if the conditioning geology is present. The landslides in the coastal cliffs are presumably sensitive to a combination of water infiltration and specific runoff patterns over impermeable layers in the substrate and to wave erosion of the cliff toe by swash run-up during high water levels under storm conditions (Schou, 1949). The eroded sediment of the coastal cliffs and specifically the landslides in the cliffs are further transported towards deeper water in a cross-shore direction, or along the shores by wave-driven longshore currents forming accreted forms like barrier islands and spits (Kabuth et al., 2013; Kabuth and Kroon, 2014).
3.1 Data sources
The main datasets used in this study are a freely available high-resolution DEM from 2015 and orthophotos provided by the Danish Agency for Data Supply and Infrastructure. The national DEM is produced from airborne lidar scans with a spatial resolution of 40 cm and is freely available (Geodatastyrelsen, 2015b). Several multi-temporal nationwide orthophotos with a resolution of 12.5 cm complement the mapping effort for visual validation of landslide features in the landscape (Geodatastyrelsen, 2015a). Table 1 shows a complete list of the datasets used to map landslides in this study.
3.2 Landslide mapping
A detailed description of the method is given in Svennevig et al. (2020b). The nationwide freely available 40 cm resolution DEM from 2015 is visualized as a multidirectional hillshade model. Landslides are mapped based on their morphological expression in the multidirectional hillshade model when a scarp and a displaced unit are observed (Fig. 2). The identification of a landslide in the multidirectional hillshade model is supported by additional morphological features, such as a crown, transverse cracks, main body or foot in many cases. Coastal erosion makes it difficult to separate the source area from the main deposit and the landslide foot is often partly removed by wave erosion. Therefore, landslides are mapped in a single polygon and the mapping did not distinguish between the source area and landslide deposit. Subsequent landslides in the same area are mapped as overlapping independent polygons when it was possible to clearly differentiate between varying morphological features. Along the coast, landslide morphologies occurred in sequences next to each other. When it was not possible to separate single landslides in the hillshade model, succession rates of the vegetation, visible in the orthophotos, were used to distinguish between morphologies. Landslides that originate from before the last glaciation are not included in the database due to the high uncertainty of the morphological expression in the DEM. Mapped landslides are classified into coastal (<300 m to the shore) or inland (>300 m from the shore) landslides and categorized by their type of movement (fall, slide, flow spread), following the classification from Hungr et al. (2014). Several 12.5 cm resolution orthophotos annually from 2014–2019 support the investigation (Table 1). The method applied here is similar to Svennevig (2019) and is simplified from Slaughter et al. (2017) and Burns and Madin (2009).
3.3 Quality control
Two experts mapped landslides in about half of Denmark each. After completion of the initial mapping, a verification of the mapped polygons was performed by the other expert. This included adding landslide polygons to the database and refining existing polygon shapes. Afterwards, an additional validation of the landslide inventory was performed by a third expert independently mapping landslides in a randomly selected subsample area. They evaluated the completeness of the inventory and estimated the bias of the initial mapping. To achieve this, the area of investigation was subdivided into 658 tiles with a size of 10×10 km. Out of the 658 tiles, 192 tiles were randomly selected, creating a subsample with a confidence level of 90 % and an error of 5 % (Fig. 3). The third mapper used the same datasets and applied the same criteria for mapping a landslides like the two initial mappers, but had no knowledge about the already mapped landslides in the subset area. The quality control mapper mapped landslide points, and an agreement between the two initial mappers and the third mapper was reached when the quality control point fell within the initial landslide polygon. After estimating the completeness of the inventory based on the comparison of the two independent mappings, landslides that were detected by the third mapper but not the first two mappers were added to the inventory. However, in some cases, the first two mappers did not agree with the third mapper and not all landslides were added to the final database.
The landslide inventory consists of 3202 unique polygons of mapped landslides. The count of types of movement and the number of coastal and inland landslides are shown in Table 2. Alongside the polygonal shape, every landslide is associated with a unique identifier. The planar area (m2) and perimeter length (m) of every landslide are provided as are the x and y coordinates of the center point. By planar area, the largest slides comprise 327 000 m2. Landslides were mapped to a minimum area of 25 m2. An analysis of the mapped landslides shows that most landslides in Denmark are shallow rotational slides. The database underrepresents processes with indistinguishable morphologies and expressions in the DEM, such as rockfalls and mudflows. However, there are only a few areas in Denmark with the geological preconditions facilitating rockfalls. The vast majority of landslides recorded are located in landscapes covered in glacial till. Although all mapped landslides must have occurred after the last glaciation, as their morphological expression would have been erased by the activity of the ice sheet, there are no data available in the landslide database when individual landslides emerged. Landscapes that were not covered by ice during the LGM are almost entirely absent of landslides today (Fig. 1).
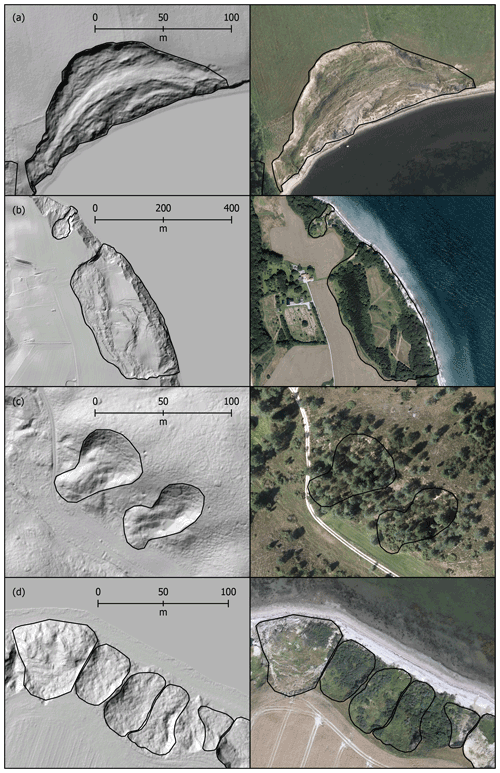
Figure 2Examples of mapped landslide polygons in the hillshade model (left) and orthophoto from 2015 (right) (Geodatastyrelsen, 2015a, b). Shallow coastal slide (a), coastal flow and deep-seated slide partly obscured by agricultural land use (b), two shallow inland slides visible in the hillshade model but covered by vegetation in the orthophoto (c) and the sequence of coastal landslides with different ages and succession rates of vegetation.
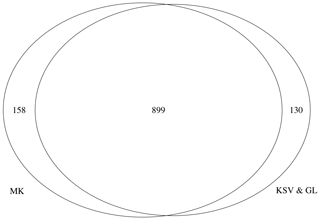
Figure 3Venn diagram with the number of mapped landslides in the randomly selected tiles by the two initial experts and quality control (MK, KSV & GL: 899), the number of landslides only mapped by the quality control (MK: 158) and the number of landslides mapped only by the two initial experts (KSV & GL: 130).
We interpret most of the mapped landslides as single events with process durations that span from an instantaneous event to several decades or even centuries and, thus, some are still active while others are inactive landforms today. Landslides that are clearly not a single event are mapped as separate polygons. The present landslide inventory only represents a snapshot of the landslide activity in Denmark at the time of recording from the 2015 DEM. However, the landslide inventory does not contain any information about current or past activity or inactivity. In some cases, landslide areas overlap each other, making it more difficult to distinguish individual landslide morphologies. Without dating every single landslide, a further distinction is not possible in these cases. The datasets and morphological criteria used for the mapping are not suitable for mapping slides with small volumes or faint morphologies, such as rockfalls and mudslides. Thus, these types are expected to be underrepresented in the database. Land use such as farming and infrastructure development may have led to an underrepresentation of landslides in these areas due to intensive cultivation and site development, especially in the inland areas. Nevertheless, around 85 % of the mapped landslides are in coastal environments, often on a cliff at the edge of agriculturally used land. Farmers usually avoid those steep slopes with their heavy and expensive equipment. In some areas along the coastal cliffs, abandoned quarries show morphological expressions similar to landslides in the DEM. The absence of landslide deposits can be the only distinction between the morphological expression of a coastal quarry and a landslide in the DEM. Occasionally, quarries may have been mistakenly mapped as landslides during the mapping. In some cases, landslides evolved on the steep slopes of a quarry, sliding into the former pit and, in other cases, quarry activity may have overprinted landslides.
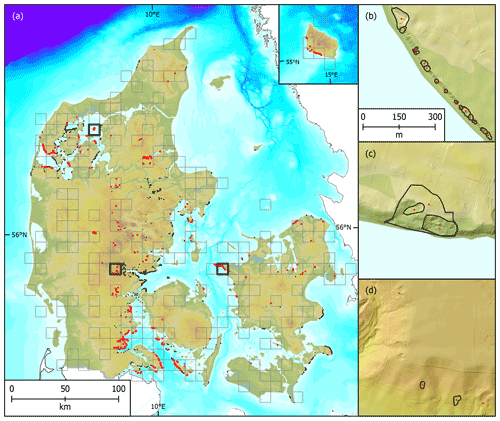
Figure 4Landslide inventory quality control with 192 randomly selected tiles across Denmark. The black dots show the 1057 landslides mapped by the third mapper (a), sequence of mapped landslide polygons along the coast with a high accordance of quality control points (b), nested landslides with quality control points for each polygon (c) and mapped inland landslides with quality control points that show additional landslides that were missed by the initial mappers (d).
Within the area of the subsample plots for quality control, the two experts had initially mapped 1029 landslides and the quality control mapped 1057 landslides, a difference of 2.7 %. However, 899 of those landslides were identical, 130 landslides were only mapped during the initial mapping and 158 only during the quality control (Fig. 3). Provided that the combined landslide mapping effort of the initial investigation and the quality control detected the true number of landslides (1187), the initial effort discovered 87 % and the quality control 89 % of all landslides. Furthermore, 151 (4.7 %) landslides in the entire study area were validated by visiting the landslides in the field or by mentions in other resources such as previous publications or newspaper articles.
Based on the careful observation of the entire study area and the implemented quality control, the landslide inventory can be considered 87 % complete with a confidence level of 90 % and an error of 5 % for the 2015 DEM. However, a few landslides always remain undetected and new landslides will have emerged since the DEM was recorded. According to the landslide inventory protocol from Burns and Madin (2009), we only mapped landslides with a moderate to high confidence. The high confidence level, in combination with the high quality of the input datasets, lets us conclude that all landslides included in the database are actually landslides.
The landslide dataset and a document with metadata are freely available from https://doi.org/10.6084/m9.figshare.16965439.v2 (Svennevig and Luetzenburg, 2021) and can also be viewed through a web map environment (https://data.geus.dk/landskred/, last access: 6 June 2022) where layers such as the hillshade model, soil map, pre-Quaternary geology, etc. can be displayed for context. The landslide dataset is provided in the form of an Environmental Systems Research Institute (ESRI) shapefile including the following attributes: landslide ID, area, perimeter length, center point coordinates, coastal or inland, movement type, field validation, quality control confirmation, original mapper and modifying mapper. The definitions of each attribute are provided in an additional metadata text document. The DEM is available for download in 10 km tiles (https://datafordeler.dk/dataoversigt/?emne=landkort%20og%20geografi, last access: 6 June 2022; Geodatastyrelsen, 2015b).
The motivation for creating and freely providing this landslide inventory is twofold:
-
The first national landslide inventory for Denmark is an important step towards a more comprehensive hazard and risk framework for Denmark. Making the inventory available enables local, regional and national stakeholders to implement landslides into their risk reduction strategies. Furthermore, a legislative framework implementing landslide risk and damage may build upon this dataset. With the expected increase in global landslide activities due to climate change, a landslide risk reduction strategy is now more important than ever before (Gariano and Guzzetti, 2016). In Denmark, a combination of increases in frequency and magnitude of heavy precipitation events, ground water level rises, storm surges and a general increase in relative sea level make higher landslide activity in the future very likely. Therefore, it is crucial to better understand the underlying processes causing landslides and develop effective risk reduction strategies to protect human lives and property.
-
Providing an expert-based, high-quality and scientifically evaluated landslide inventory to scientific communities like the modeling, landslide prediction, machine and deep learning research communities. The landslide dataset is validated and extends the availability of urgently needed training datasets for automated mapping methods. The consistently high amount of time required to manually compile landslide inventories stands in contrast to the increase in data available for landslide mapping. Future challenges in landslide inventory mapping lie in developing methods to reliably automate the process. The present dataset provides a valuable resource to train and develop future algorithms for this task. Especially in combination with the freely available DEM, automated mapping methods can include the elevation data into their investigation. Additionally, this is one of the few landslide inventories providing a statistical error estimation of the completeness of the number of mapped landslides.
KS conceptualized the study, GL and KS curated the data and performed the formal analysis, GL wrote the original draft and visualized the data, MK quality controlled the data, and all authors discussed the dataset, reviewed and edited the manuscript.
The contact author has declared that none of the authors has any competing interests.
Publisher's note: Copernicus Publications remains neutral with regard to jurisdictional claims in published maps and institutional affiliations.
Gregor Luetzenburg has received funding from the European Union's Horizon 2020 research and innovation program under the Marie Skłodowska-Curie grant (grant no. 801199).
This paper was edited by Kirsten Elger and reviewed by Cees van Westen and one anonymous referee.
Alberti, S., Senogles, A., Kingen, K., Booth, A., Castro, P., DeKoekkoek, J., Glover-Cutter, K., Mohney, C., Olsen, M., and Leshchinsky, B.: The Hooskanaden Landslide: historic and recent surge behavior of an active earthflow on the Oregon Coast, Landslides, 17, 2589–2602, https://doi.org/10.1007/s10346-020-01466-8, 2020.
Brardinoni, F., Slaymakerl, O., and Hassan, M. A.: Landslide inventory in a rugged forested watershed: a comparison between air-photo and field survey data, Geomorphology, 54, 179–196, https://doi.org/10.1016/S0169-555X(02)00355-0, 2003.
Burns, W. J. and Madin, I. P.: Protocol for Inventroy Mapping of Landslide Deposits from Light Detection and Ranging (LiDAR) Imagery, Oregon Department of Geology and Mineral Industries, 2009.
Cavalli, M. and Marchi, L.: Characterisation of the surface morphology of an alpine alluvial fan using airborne LiDAR, Nat. Hazards Earth Syst. Sci., 8, 323–333, https://doi.org/10.5194/nhess-8-323-2008, 2008.
Coe, J. A.: Bellwether sites for evaluating changes in landslide frequency and magnitude in cryospheric mountainous terrain: a call for systematic, long-term observations to decipher the impact of climate change, Landslides, 17, 2483–2501, https://doi.org/10.1007/s10346-020-01462-y, 2020.
Colombo, A., Lanteri, L., Ramasco, M., and Troisi, C.: Systematic GIS-based landslide inventory as the first step for effective landslide-hazard management, Landslides, 2, 291–301, https://doi.org/10.1007/s10346-005-0025-9, 2005.
Crosby, C. J., Whitmeyer, S. J., Bailey, J. E., De Paor, D. G., and Ornduff, T.: Lidar and Google Earth: Simplifying access to high-resolution topography data, in: Google Earth and Virtual Visualizations in Geoscience Education and Research, Geological Society of America, 0, https://doi.org/10.1130/2012.2492(03), 2012.
Cruden, D. M. and Varnes, D. J.: Landslide Types and Processes, Transportation Research Board, 36–75, 1996.
Damm, B. and Klose, M.: The landslide database for Germany: Closing the gap at national level, Geomorphology, 249, 82–93, https://doi.org/10.1016/j.geomorph.2015.03.021, 2015.
Denmark, S.: Denmark in Figures, Statistics Denmark, 2019.
EEA: European Digital Elevation Model (EU-DEM), version 1.1, European Environment Agency , 2016.
Fiorucci, F., Cardinali, M., Carla, R., Rossi, M., Mondini, A. C., Santurri, L., Ardizzone, F., and Guzzetti, F.: Seasonal landslide mapping and estimation of landslide mobilization rates using aerial and satellite images, Geomorphology, 129, 59–70, https://doi.org/10.1016/j.geomorph.2011.01.013, 2011.
Froude, M. J. and Petley, D. N.: Global fatal landslide occurrence from 2004 to 2016, Nat. Hazards Earth Syst. Sci., 18, 2161–2181, https://doi.org/10.5194/nhess-18-2161-2018, 2018.
Galli, M., Ardizzone, F., Cardinali, M., Guzzetti, F., and Reichenbach, P.: Comparing landslide inventory maps, Geomorphology, 94, 268–289, https://doi.org/10.1016/j.geomorph.2006.09.023, 2008.
Gariano, S. L. and Guzzetti, F.: Landslides in a changing climate, Earth-Sci. Rev., 162, 227–252, https://doi.org/10.1016/j.earscirev.2016.08.011, 2016.
Geodatastyrelsen: Geodanmark 2015 12.5 cm Styrelsen for Dataforsyning og Effektivisering, WMS Service, 2015a.
Geodatastyrelsen: Denmark's Elevation Model, Styrelsen for Dataforsyning og Effektivisering, WMS Service, https://datafordeler.dk/dataoversigt/?emne=landkort%20og%20geografi (last access: 6 June 2022), 2015b.
Guzzetti, F., Mondini, A. C., Cardinali, M., Fiorucci, F., Santangelo, M., and Chang, K.-T.: Landslide inventory maps: New tools for an old problem, Earth-Sci. Rev., 112, 42–66, https://doi.org/10.1016/j.earscirev.2012.02.001, 2012.
Hao, L., Rajaneesh A., van Westen, C., Sajinkumar K. S., Martha, T. R., Jaiswal, P., and McAdoo, B. G.: Constructing a complete landslide inventory dataset for the 2018 monsoon disaster in Kerala, India, for land use change analysis, Earth Syst. Sci. Data, 12, 2899–2918, https://doi.org/10.5194/essd-12-2899-2020, 2020.
Herrera, G., Mateos, R. M., García-Davalillo, J. C., Grandjean, G., Poyiadji, E., Maftei, R., Filipciuc, T.-C., Jemec Auflič, M., Jež, J., Podolszki, L., Trigila, A., Iadanza, C., Raetzo, H., Kociu, A., Przyłucka, M., Kułak, M., Sheehy, M., Pellicer, X. M., McKeown, C., Ryan, G., Kopačková, V., Frei, M., Kuhn, D., Hermanns, R. L., Koulermou, N., Smith, C. A., Engdahl, M., Buxó, P., Gonzalez, M., Dashwood, C., Reeves, H., Cigna, F., Liščák, P., Pauditš, P., Mikulėnas, V., Demir, V., Raha, M., Quental, L., Sandić, C., Fusi, B., and Jensen, O. A.: Landslide databases in the Geological Surveys of Europe, Landslides, 15, 359–379, https://doi.org/10.1007/s10346-017-0902-z, 2017.
Highland, L. M. and Bobrowsky, P.: The Landslide Handbook – A Guide to Understanding Landslides, USGS, https://pubs.usgs.gov/circ/1325/ (last access: 26 July 2022), 2008.
Houmark-Nielsen, M.: A lithostratigraphy of Weichselian glacial and interstadial deposits in Denmark, B. Geol. Soc. Denmark, 46, 101–114, https://doi.org/10.37570/bgsd-1999-46-09, 1999.
Houmark-Nielsen, M.: Pleistocene Glaciations in Denmark: A Closer Look at Chronology, Ice Dynamics and Landforms, in: Quaternary Glaciations – Extent and Chronology – A Closer Look, Developments in Quaternary Sciences, 15, 47–58, https://doi.org/10.1016/B978-0-444-53447-7.00005-2, 2011.
Hungr, O., Leroueil, S., and Picarelli, L.: The Varnes classification of landslide types, an update, Landslides, 11, 167–194, https://doi.org/10.1007/s10346-013-0436-y, 2014.
Hutchinson, J. N.: Chalk flows from the coastal cliffs of northwest Europe, in: Catastrophic landslides: Effects, occurrence, and mechanisms, edited by: Evans, S. G. and DeGraff, J. V., Geological Society of America Reviews in Engineering Geology, Boulder, Colorado, 257–302, 2002.
Kabuth, A. K. and Kroon, A.: Wave energy fluxes and multidecadal shoreline changes in two coastal embayments in Denmark, Ocean Dynam., 64, 741–754, https://doi.org/10.1007/s10236-014-0709-6, 2014.
Kabuth, A. K., Kroon, A., and Pedersen, J. B. T.: Multidecadal Shoreline Changes in Denmark, J. Coastal Res., 30, 714–728, https://doi.org/10.2112/JCOASTRES-D-13-00139.1, 2013.
Kakavas, M. P. and Nikolakopoulos, K. G.: Digital Elevation Models of Rockfalls and Landslides: A Review and Meta-Analysis, Geosciences, 11, 6, https://doi.org/10.3390/geosciences11060256, 2021.
Kirschbaum, D. B., Adler, R., Hong, Y., Hill, S., and Lerner-Lam, A.: A global landslide catalog for hazard applications: method, results, and limitations, Nat. Hazards, 52, 561–575, https://doi.org/10.1007/s11069-009-9401-4, 2009.
Lissak, C., Bartsch, A., De Michele, M., Gomez, C., Maquaire, O., Raucoules, D., and Roulland, T.: Remote Sensing for Assessing Landslides and Associated Hazards, Surv. Geophys., 41, 1391–1435, https://doi.org/10.1007/s10712-020-09609-1, 2020.
Ludwig, K. A., Ramsey, D. W., Wood, N. J., Pennaz, A. B., Godt, J. W., Plant, N. G., Luco, N., Koenig, T. A., Hudnut, K. W., Davis, D. K., and Bright, P. R.: Science for a risky world – A U.S. Geological Survey plan for risk research and applications, Reston, VA, Report 1444, https://doi.org/10.3133/cir1444, 2018.
Malamud, B. D., Turcotte, D. L., Guzzetti, F., and Reichenbach, P.: Landslide inventories and their statistical properties, Earth Surf. Process. Landf., 29, 687–711, https://doi.org/10.1002/esp.1064, 2004.
Mateos, R. M., Lopez-Vinielles, J., Poyiadji, E., Tsagkas, D., Sheehy, M., Hadjicharalambous, K., Liscak, P., Podolski, L., Laskowicz, I., Iadanza, C., Gauert, C., Todorovic, S., Auflic, M. J., Maftei, R., Hermanns, R. L., Kociu, A., Sandic, C., Mauter, R., Sarro, R., Bejar, M., and Herrera, G.: Integration of landslide hazard into urban planning across Europe, Landscape Urban Plan., 196, 103740, https://doi.org/10.1016/j.landurbplan.2019.103740, 2020.
Morgan, A. J., Chao, D., Froese, C. R., Martin, C. D., and Kim, T. H.: LiDAR based landslide inventory and spatial analysis, Peace River, Alberta, Energy Resources Conservation Board, 22 pp., 2013.
Nadim, F., Pedersen, S. A. S., Schmidt-Thome, P., Sigmundsson, F., and Engdahls, M.: Natural hazards in Nordic countries, Episodes, 31, 176–184, https://doi.org/10.18814/epiiugs/2008/v31i1/024, 2008.
Palma, A., Garrill, R., Brook, M. S., Richards, N., and Tunnicliffe, J.: Reactivation of coastal landsliding at Sunkist Bay, Auckland, following ex-Tropical Cyclone Debbie, 5 April 2017, Landslides, 17, 2659–2669, https://doi.org/10.1007/s10346-020-01474-8, 2020.
Pedersen, S. A. S., Foged, N., and Frederiksen, J.: Extent and economic significance of landslides in Denmark, Faroe Islands and Greenland, in: Landslides: Extent and Economic Significance, Brabb & Harrod, Rotterdam, 1989.
Pellicani, R. and Spilotro, G.: Evaluating the quality of landslide inventory maps: comparison between archive and surveyed inventories for the Daunia region (Apulia, Southern Italy), B. Eng. Geol. Env., 74, 357–367, https://doi.org/10.1007/s10064-014-0639-z, 2014.
Prior, D. B.: Coastal Mudslide Morphology and Processes on Eocene Clays in Denmark, Geografisk Tidsskrift-Danish Journal of Geography, 76, 14–33, https://doi.org/10.1080/00167223.1977.10649071, 1977.
Rosi, A., Tofani, V., Tanteri, L., Tacconi Stefanelli, C., Agostini, A., Catani, F., and Casagli, N.: The new landslide inventory of Tuscany (Italy) updated with PS-InSAR: geomorphological features and landslide distribution, Landslides, 15, 5–19, https://doi.org/10.1007/s10346-017-0861-4, 2017.
Schou, A.: Danish Coastal Cliffs in Glacial Deposits, Geografiska Annaler, 31, 357–364, 1949.
Shugar, D. H., Jacquemart, M., Shean, D., Bhushan, S., Upadhyay, K., Sattar, A., Schwanghart, W., McBride, S., de Vries, M. V. W., Mergili, M., Emmer, A., Deschamps-Berger, C., McDonnell, M., Bhambri, R., Allen, S., Berthier, E., Carrivick, J. L., Clague, J. J., Dokukin, M., Dunning, S. A., Frey, H., Gascoin, S., Haritashya, U. K., Huggel, C., Kaab, A., Kargel, J. S., Kavanaugh, J. L., Lacroix, P., Petley, D., Rupper, S., Azam, M. F., Cook, S. J., Dimri, A. P., Eriksson, M., Farinotti, D., Fiddes, J., Gnyawali, K. R., Harrison, S., Jha, M., Koppes, M., Kumar, A., Leinss, S., Majeed, U., Mal, S., Muhuri, A., Noetzli, J., Paul, F., Rashid, I., Sain, K., Steiner, J., Ugalde, F., Watson, C. S., and Westoby, M. J.: A massive rock and ice avalanche caused the 2021 disaster at Chamoli, Indian Himalaya, Science, 373, 300–306, https://doi.org/10.1126/science.abh4455, 2021.
Slaughter, S. L., Burns, W. J., Mickelson, K. A., Jacobacci, K. E., Biel, A., and Contreras, T. A.: Protocol for Landslide Inventory Mapping from LiDAR Data in Washington State, Washington Geological Survey Bulletin, 82, 2017.
Svennevig, K.: Preliminary landslide mapping in Greenland, Geol. Surv. Den. Greenl., 43, https://doi.org/10.34194/GEUSB-201943-02-07, 2019.
Svennevig, K. and Luetzenburg, G.: Danish landslide inventory 211104, Figshare [data set], https://doi.org/10.6084/m9.figshare.16965439.v1, 2021.
Svennevig, K., Dahl-Jensen, T., Keiding, M., Merryman Boncori, J. P., Larsen, T. B., Salehi, S., Munck Solgaard, A., and Voss, P. H.: Evolution of events before and after the 17 June 2017 rock avalanche at Karrat Fjord, West Greenland – a multidisciplinary approach to detecting and locating unstable rock slopes in a remote Arctic area, Earth Surf. Dynam., 8, 1021–1038, https://doi.org/10.5194/esurf-8-1021-2020, 2020a.
Svennevig, K., Luetzenburg, G., Keiding, M. K., and Pedersen, S. A. S.: Preliminary landslide mapping in Denmark indicates an underestimated geohazard, Geus Bulletin, 44, https://doi.org/10.34194/geusb.v44.5302, 2020b.
Trigila, A., Iadanza, C., and Spizzichino, D.: Quality assessment of the Italian Landslide Inventory using GIS processing, Landslides, 7, 455–470, https://doi.org/10.1007/s10346-010-0213-0, 2010.
Van Den Eeckhaut, M., Poesen, J., Verstraeten, G., Vanacker, V., Moeyersons, J., Nyssen, J., and van Beek, L. P. H.: The effectiveness of hillshade maps and expert knowledge in mapping old deep-seated landslides, Geomorphology, 67, 351–363, https://doi.org/10.1016/j.geomorph.2004.11.001, 2005.
Zieher, T., Perzl, F., Rossel, M., Rutzinger, M., Meissl, G., Markart, G., and Geitner, C.: A multi-annual landslide inventory for the assessment of shallow landslide susceptibility – Two test cases in Vorarlberg, Austria, Geomorphology, 259, 40–54, https://doi.org/10.1016/j.geomorph.2016.02.008, 2016.