the Creative Commons Attribution 4.0 License.
the Creative Commons Attribution 4.0 License.
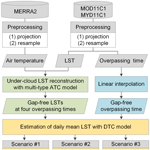
A global dataset of spatiotemporally seamless daily mean land surface temperatures: generation, validation, and analysis
Falu Hong
Frank-M. Göttsche
Zihan Liu
Pan Dong
Huyan Fu
Fan Huang
Xiaodong Zhang
Daily mean land surface temperatures (LSTs) acquired from polar orbiters are crucial for various applications such as global and regional climate change analysis. However, thermal sensors from polar orbiters can only sample the surface effectively with very limited times per day under cloud-free conditions. These limitations have produced a systematic sampling bias (ΔTsb) on the daily mean LST (Tdm) estimated with the traditional method, which uses the averages of clear-sky LST observations directly as the Tdm. Several methods have been proposed for the estimation of the Tdm, yet they are becoming less capable of generating spatiotemporally seamless Tdm across the globe. Based on MODIS and reanalysis data, here we propose an improved annual and diurnal temperature cycle-based framework (termed the IADTC framework) to generate global spatiotemporally seamless Tdm products ranging from 2003 to 2019 (named the GADTC products). The validations show that the IADTC framework reduces the systematic ΔTsb significantly. When validated only with in situ data, the assessments show that the mean absolute errors (MAEs) of the IADTC framework are 1.4 and 1.1 K for SURFRAD and FLUXNET data, respectively, and the mean biases are both close to zero. Direct comparisons between the GADTC products and in situ measurements indicate that the MAEs are 2.2 and 3.1 K for the SURFRAD and FLUXNET datasets, respectively, and the mean biases are −1.6 and −1.5 K for these two datasets, respectively. By taking the GADTC products as references, further analysis reveals that the Tdm estimated with the traditional averaging method yields a positive systematic ΔTsb of greater than 2.0 K in low-latitude and midlatitude regions while of a relatively small value in high-latitude regions. Although the global-mean LST trend (2003 to 2019) calculated with the traditional method and the IADTC framework is relatively close (both between 0.025 to 0.029 K yr−1), regional discrepancies in LST trend do occur – the pixel-based MAE in LST trend between these two methods reaches 0.012 K yr−1. We consider the IADTC framework can guide the further optimization of Tdm estimation across the globe, and the generated GADTC products should be valuable in various applications such as global and regional warming analysis. The GADTC products are freely available at https://doi.org/10.5281/zenodo.6287052 (Hong et al., 2022).
- Article
(21367 KB) - Full-text XML
- BibTeX
- EndNote
Land surface temperature (LST) is one of the most important variables of land–atmosphere interaction (Jin and Dickinson, 2010). Currently, satellite thermal remote sensing provides the only way to obtain long-term and regular LST over extensive areas. The archived long-term satellite-derived LST datasets have been widely used in various fields such as land cover change detection (Lambin and Ehrlich, 1997; Muro et al., 2018), radiation flux simulation (Alcântara et al., 2010; Anderson et al., 2007), drought monitoring (Karnieli et al., 2010; Mildrexler et al., 2017), vegetation change analysis (Julien and Sobrino, 2009; Julien et al., 2006; Still et al., 2019), permafrost thawing monitoring (Westermann et al., 2011), and global LST trend investigation (Jin, 2004; Jin and Dickinson, 2002; Yan et al., 2020).
According to the satellite onboard duration and spatiotemporal resolution (Tomlinson et al., 2011), satellite-derived LST products used for long-term time-series analysis can be divided into two categories: (1) the LSTs obtained from high-orbit geostationary satellite sensors with a coarse spatial resolution (3–5 km), e.g., the MSG-SEVIRI (the Spinning Enhanced Visible and Infrared Imager onboard Meteosat Second Generation) and GOES (Geostationary Operational Environmental Satellite), and (2) the LSTs from low-orbit polar-orbiting satellite sensors. The second category of satellite sensors can be further divided into (1) the narrow-swath polar-orbiting satellite sensors with a relatively high spatial resolution (around 100 m), e.g., Landsat and ASTER (Advanced Spaceborne Thermal Emission and Reflection Radiometer) and (2) the polar-orbiting satellite sensors with a moderate spatial resolution (around 1 km), e.g., AVHRR (Advanced Very High-Resolution Radiometer), SLSTR (Sea and Land Surface Temperature Radiometer), and MODIS (Moderate Resolution Imaging Spectroradiometer).
The geostationary satellite thermal sensors are characterized by a very high temporal resolution (1 h or finer). However, they are relatively difficult to provide global consistent LST products due to the limited coverage of a single geostationary satellite and the systematic errors among different satellites (Freitas et al., 2013). The Landsat (or similar polar orbiters) has been providing thermal observations since the 1980s, but the relatively long revisiting period (e.g., 16 d for Landsat) makes it challenging to capture the daily and hourly continuous LST dynamics (Fu and Weng, 2016). By contrast, wide-swath polar-orbiting sensors (e.g., MODIS) can sample the earth surface at least twice a day with a relatively high spatial resolution (around 1.0 km). The feature makes the MODIS-like sensors overcome the limitations of the Landsat-like satellites (with a long revisiting period) and geostationary satellite sensors (with a restricted global coverage). Therefore, the LSTs obtained from wide-swath polar-orbiting sensors (e.g., MODIS and AVHRR) have been widely used in capturing the long-term global LST dynamics (Sobrino et al., 2020a; Mildrexler et al., 2011). Among these, the MODIS LST data have been used the most (Eleftheriou et al., 2018; Fu, 2019; Heck et al., 2019; Potter and Coppernoll-Houston, 2019; Quan et al., 2016; Sobrino et al., 2020a; Yan et al., 2020; Zhao et al., 2019, 2021). This is mainly because, especially when compared with the AVHRR data, (1) MODIS LST observations are less affected by the orbit drift effect (Julien and Sobrino, 2012; Latifovic et al., 2012; Ma et al., 2020; Gutman, 1999); (2) the MODIS LST products can offer more details about the diurnal LST dynamics with four observations per day (Crosson et al., 2012; Hong et al., 2018); and (3) the MODIS LST retrieval algorithm has been continuously improved, and the associated LSTs products are comparably more mature and have been extensively validated (Duan et al., 2018, 2019; Wan, 2014).
However, most previous studies employed temporally aggregated results (8 d or monthly mean) of instantaneous cloud-free LSTs for long-term LST time-series analysis (Mao et al., 2017; Sobrino et al., 2020a, b; Xing et al., 2021), instead of continuous daily mean LST (termed as Tdm) on a day-to-day basis. Compared with the continuous daily Tdm, temporally aggregated results of instantaneous cloud-free LSTs lack the information of under-cloud thermal observations and insufficiently sample the LST diurnal dynamics (Ermida et al., 2019; Hu et al., 2020; Westermann et al., 2012). Such a direct temporal aggregation approach can produce a systematic sampling bias (termed as ΔTsb) (Hong et al., 2021), which affects the accuracy of Tdm directly and the associated trend analysis indirectly (Zhou and Wang, 2016). To estimate accurate Tdm, Hong et al. (2021) designed the ADTC-based framework that combines an annual temperature cycle (ATC) model and a diurnal temperature cycle (DTC) model. Based on the MODIS LST product and some auxiliary data such as the reanalysis data, the ADTC-based framework first uses an ATC model to reconstruct the instantaneous under-cloud LSTs and then simulates the diurnal LST dynamics with a four-parameter DTC model to solve the issue of under-sampling with only four observations per day. Validations showed that the ADTC-based framework can reduce the ΔTsb significantly and produce the spatiotemporally seamless Tdm (Hong et al., 2021).
However, the original ADTC-based framework (termed the OADTC framework) has only been tested over a relatively small region. In other words, the performance of the OADTC framework over complicated situations across global land surfaces has not been studied. Currently a global spatiotemporally seamless daily mean LST product is still unavailable to the satellite thermal remote-sensing community; furthermore, the spatial distribution of ΔTsb and its impact on the LST trend over global land surfaces also remains unclear. There are two further limitations when applying the OADTC framework to the actual generation of global seamless Tdm: (1) the selected ATC model in the OADTC framework uses a single sinusoidal function to describe the intra-annual variation of solar radiation, which becomes less suitable for equatorial and polar regions (Z. Liu et al., 2019); (2) the DTC model used may fail around sunrise with no-solution or extreme solution and cause an underestimation and even outliers of the daily mean LST (Hong et al., 2021; Hu et al., 2020).
Facing these issues, this study intends to formulate an improved version of the original ADTC-based framework (hereafter termed the IADTC framework) using an advanced multi-type ATC model as well as a DTC model optimized for estimating Tdm. With the IADTC framework, we then generate a global spatiotemporally seamless 0.5∘ Tdm product (termed the GADTC product; refer to Sect. 3.1 for the detailed description) for the period from 2003 to 2019. Based on the GADTC product, we then analyze the global spatial distribution of ΔTsb as well as LST trends, which are compared with those obtained with the traditional method. We consider that the IADTC framework and the associated GADTC product should be useful for various applications such as analysis of global climate change and assessment of reanalysis data.
The MODIS LST products and MERRA2 (the Modern-Era Retrospective analysis for Research and Applications version 2) reanalysis dataset were required as input data for the IADTC framework. We also employed in situ LST measurements from the SURFRAD and FLUXNET to validate the IADTC framework and the GADTC product.
2.1 MODIS LST products
The MODIS LST products, including both the MOD11C1 and MYD11C1 LST products in Collection 6 from 2003 to 2019 (available at https://ladsweb.nascom.nasa.gov/, last access: 1 March 2020), were used to help the generation of Tdm. The MODIS LSTs were retrieved with a refined generalized split-window algorithm, and their accuracies are mostly within 1.0 K over homogeneous surfaces (Zhengming and Zhao-Liang, 1997; Duan et al., 2019; Wan, 2014). The MOD11C1 and MYD11C1 LST products cover the global land surfaces four times per day with a spatial resolution of 0.05∘. At low-latitude and midlatitude regions, MOD11C1 LSTs are obtained around 10:30 and 22:30 (local solar time), and MYD11C1 LSTs are around 01:30 and 13:30 (local solar time) with a time interval of around 1.5 h. At high-latitude regions, due to the convergence of satellite orbit (Fig. A1), the overpass times possess a significant shift from those at low-latitude and midlatitude regions (Østby et al., 2014). More details on the time shift and its impact on the estimation of Tdm with the IADTC framework are provided in Sects. 3.1.3 and 5.2.
2.2 Reanalysis data
Surface air temperatures (SATs) are used to drive the ATC model for the reconstruction of under-cloud LSTs (see Sect. 3.1). We employed the SATs from the MERRA2 reanalysis dataset (the specific collection name is inst1_2d_lfo_Nx, obtained from https://disc.gsfc.nasa.gov/datasets/M2I1NXLFO_V5.12.4/summary, last access: 9 June 2020) from 2003 to 2019 (Gelaro et al., 2017; GMAO, 2015). The spatial and temporal resolutions of these reanalysis SAT data are and 1 h, respectively.
2.3 In situ data
The in situ LST measurements from 133 globally distributed stations (Fig. 1) were used to validate the IADTC framework at site level (see Sect. 3.2.1) as well as to evaluate the GADTC product (see Sect. 3.2.2). They include seven SURFRAD (Surface Radiation Budget Network) sites (Augustine et al., 2000) and 126 FLUXNET sites from FLUXNET2015 datasets (Pastorello et al., 2020). These two datasets have been widely used for validating satellite-derived LSTs due to their extensive distribution, rigorous quality control, and long-term availability (Guillevic et al., 2018; Martin et al., 2019; Duan et al., 2019).
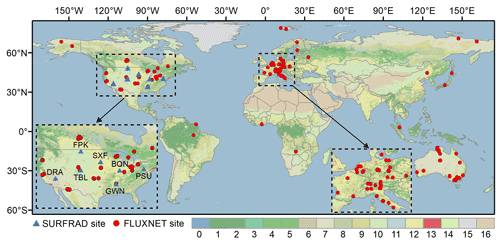
Figure 1Geolocation of the stations used for validation. The red circles and blue triangles represent the locations of the FLUXNET and SURFRAD sites, respectively. The numbers “0” to “16” at the bottom represent the background land cover type as defined by the International Geosphere-Biosphere Programme (IGBP) (Friedl et al., 2002).
2.3.1 SURFRAD data
We employed observations from the seven SURFRAD sites during the period of 2003–2019 (available at https://www.esrl.noaa.gov/gmd/grad/surfrad/, last access: 1 April 2020). The seven SURFRAD sites have relatively heterogeneous surfaces, and their land cover types include grassland, cropland, and bare soil. Broadband hemispherical radiances are measured with pyrgeometers (Eppley Precision Infrared Radiometer) with a wavelength range of 4–50 µm. Sensors at each site are installed at 10 m height with a spatial representativeness of approximately 70×70 m2 (Guillevic et al., 2014). More detailed information on these sites is given in Table 1 in Sect. 4.2. In situ LSTs were estimated with the measured upward and downward longwave radiances with the following formula:
where L↑ and L↓ are the upward and downward longwave radiation, respectively; εb is the broadband emissivity estimated with the MODIS narrowband emissivities ε31 and ε32 in MODIS Channels 31 and 32, respectively (Liang et al., 2013); and σ is the Stefan–Boltzmann constant ( W m−2 K−4). To reduce the impacts of short-term LST fluctuations on validation, we aggregated minutely observations into hourly values.
2.3.2 FLUXNET data
We further employed the FLUXNET 2015 datasets (available at https://fluxnet.org/data/fluxnet2015-dataset/, last access: 1 April 2020) to evaluate the GADTC product (Pastorello et al., 2020). The FLUXNET 2015 datasets include more than 200 sites covering multiple ecosystem types across the globe and provide hourly upwelling and downwelling longwave radiation observations of two pyrgeometers (spectral range 3.5–50.0 µm) that can be used to retrieve LST (Guillevic et al., 2018). Removing the sites without upwelling longwave radiation observations resulted in a total of 126 sites for the period from 2003–2015 (Fig. 1). The in situ LSTs were calculated and preprocessed using the same method as for the SURFRAD data.
3.1 Generation of global gap-free daily mean LST with the IADTC framework
The OADTC framework consists of two steps to generate Tdm (Hong et al., 2021): (1) reconstruction of instantaneous under-cloud LSTs with an ATC model to ensure the availability of four valid LSTs at the four daily overpass times and (2) simulation of diurnal LST dynamics using a four-parameter DTC model and estimation of Tdm. This study improved the OADTC framework using a more advanced ATC model as well as by optimizing the estimation of Tdm with the DTC model. The generation of global gap-free Tdm with this improved framework (termed the IADTC framework) includes four steps (Fig. 2): data preprocessing (Sect. 3.1.1), under-cloud LST reconstruction with an advanced ATC model (Sect. 3.1.2), linear interpolation of MODIS overpass time (Sect. 3.1.3), and Tdm estimation with a DTC model (Sect. 3.1.4).
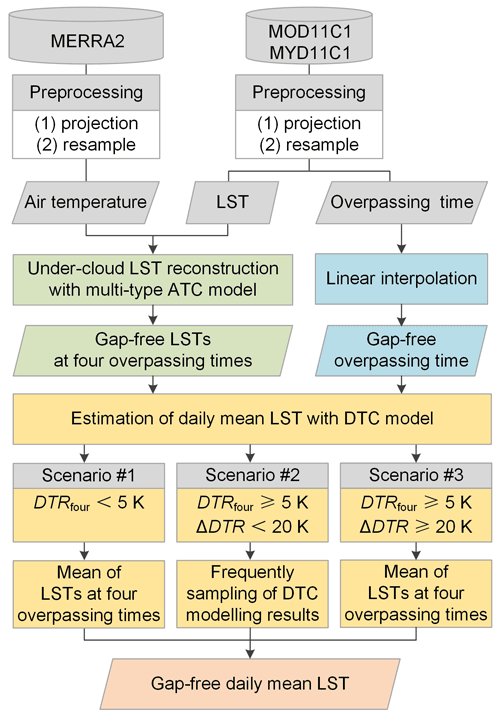
Figure 2Flowchart of the IADTC framework. DTRfour refers to diurnal temperature range (DTR) calculated as the maximum minus the minimum from the gap-free LSTs at the four overpass times; DTRDTC refers to the DTR calculated from the hourly LSTs modeled with the DTC model. ΔDTR refers to the absolute difference between DTRfour and DTRDTC.
3.1.1 Data preprocessing
We generated the global Tdm product with a spatial resolution of 0.5×0.5∘ rather than a higher resolution (e.g., 1 km), mainly because of the following two aspects. First, our study aims at analyzing the spatial pattern of ΔTsb and the LST trend at the global scale, i.e., to perform a LST climatology analysis for which a spatial resolution of 0.5∘ is adequate. Second, the Tdm generation is conducted on a daily and pixel-by-pixel basis on the global scale, which requires a huge amount of computational resources on a higher spatial resolution. Consequently, the MOD11C1 and MYD11C1 products were resampled to a spatial resolution of 0.5∘; the MERRA2 reanalysis hourly air temperature data were resampled to daily values with the same resolution.
3.1.2 Under-cloud LST reconstruction with multi-type ATC model
The general formula of ATC model is displayed in Eq. (2). The single-type ATC model in the OADTC framework uses a single sinusoidal function (M=1 in Eq. 2) to model the intra-annual LST variations driven by solar radiation change and incorporates surface air temperatures to help simulate the LST fluctuations induced by synoptic conditions (Zou et al., 2018; Z. Liu et al., 2019). The use of a single sinusoidal function is generally acceptable for midlatitude regions. However, a single sinusoidal is no longer suitable for low latitudes because there are two solar radiation peaks within a yearly cycle of low-latitude regions (Xing et al., 2020; Bechtel, 2015; Cao and Sanchez-Azofeifa, 2017); it is also inadequate for high-latitude regions where polar days and nights occur (Østby et al., 2014; Z. Liu et al., 2019; Westermann et al., 2012). Therefore, the use of the single-type ATC model in the OADTC framework is less suitable to generate Tdm at the global scale (Fig. 3). To overcome this limitation, the IADTC framework uses different versions of ATC model (termed the multi-type ATC model) to reconstruct under-cloud LSTs over the low-latitude, midlatitude, and high-latitude regions, respectively. The details are given as follows:
-
Low-latitude regions (23.5∘ N–23.5∘ S).
The solar radiation possesses two peaks within a yearly cycle over low-latitude regions (Fig. 3a). We therefore employed the ATC model with two sinusoidal functions (M=2 in Eq. 2) to reconstruct the daily LST dynamics within an annual cycle (Z. Liu et al., 2019; Xing et al., 2020).
-
Midlatitude regions (23.5∘–66.5∘ N/S).
The solar radiation peaks once in summer during an annual cycle. We therefore employed the ATC model with a single sinusoidal function (M=1 in Eq. 2) to reconstruct the daily LST dynamics (Fig. 3b).
-
High-latitude regions (66.5∘–90∘ N/S).
The polar day/night phenomena occur over high-latitude regions, and the duration increases with latitude. Theoretically, over these regions, the ATC model with multiple sinusoidal functions should be the best choice. However, the number of cloud-free MODIS observations is limited, and additional model complexity can lead to over-fitting and weaken the generalization ability of the ATC model (Z. Liu et al., 2019). To balance model accuracy and generalization ability, the ATC model with two sinusoidal functions was selected for high-latitude regions (see Fig. 3c).
where TATCM (d) denotes the daily LST variations simulated with the ATC model; M is the number of harmonic components used; d and N are the day of year (DOY) and number of days in a year, respectively; ΔTair (d) is the difference between the daily SATs (i.e., Tair (d), obtained from MERRA2 reanalysis data) and the modeled air temperatures with the original ATC model (TATCO (d)); and T0, Am, θm, and k are the parameters that need to be solved with the cloud-free daily LSTs and SATs, usually through the least-squares method.
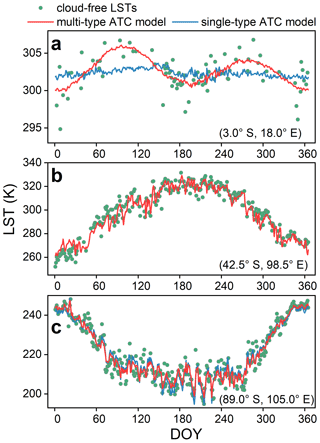
Figure 3Comparison of reconstructing under-cloud LSTs with multi-type and single-type ATC models at different latitudes. Panels (a, b, c) show three examples of ATC modeling at low-latitudes, midlatitudes, and high-latitudes for cloud-free Terra-day LST in 2019. The green circles, blue lines, and red lines denote the cloud-free observations and LSTs simulated by the single- and multi-type ATC models, respectively. Note that for (b), the results of the single- and multi-type ATC models are identical since they both use the ATC model with a single sinusoidal function.
3.1.3 Interpolation of overpass times
The under-cloud LST reconstruction with the ATC model ensures that there are four valid LSTs within a diurnal cycle. However, there are still missing values for the corresponding four overpass times. We used linear interpolation to reconstruct the missing overpass times based on the valid overpass times on the 2 adjacent days with valid values. For example, if the overpass times from 10 to 20 July for Aqua day are missing, the linear interpolation was used to fill the missing values during this period using the valid values on the 2 adjacent days with valid values (i.e., 9 and 21 July). The uncertainties of linear interpolation are expected to be within the range associated with local overpass time fluctuations. For the low-latitude and midlatitude regions where the overpass time fluctuations are relatively small (less than 1.5 h), the uncertainties using linear interpolation are relatively minor. However, for the high-latitude regions where the overpass times fluctuate significantly (Fig. A1), linear interpolation holds a larger error and might affect the estimation of Tdm. More discussions in terms of the uncertainties of the linear interpolation are provided in Sect. 5.2.
3.1.4 Estimation of daily mean LST with DTC model
The under-cloud LST reconstruction (Sect. 3.1.2) and linear interpolation of overpass time (Sect. 3.1.3) ensure that there are four valid LSTs and the associated overpass times per day. These provide the foundation for estimating Tdm with a four-parameter DTC model. This study selected the four-parameter GOT09-dT-τ model, which has been shown to have the highest accuracy among a variety of four-parameter DTC models (Hong et al., 2018). Further details related to the formulae and the associated parameters of the GOT09-dT-τ model are provided in Göttsche and Olesen (2009) and Hong et al. (2018).
For the generation of global products, the GOT09-dT-τ model can face the issues of no solution or extreme solution, under which the estimated Tdm can be significantly biased due to the reduced capability to model LST around sunrise (Hu et al., 2020) (Fig. 4c). The failed simulations can be associated with the following two reasons: (1) there are four daily MODIS LSTs per daily cycle but no observation around sunrise (Hong et al., 2018); (2) the DTC model is subject to the clear-sky hypothesis (Göttsche and Olesen, 2009). Therefore, to avoid outliers caused by failed simulations, under certain conditions, Tdm was estimated directly by averaging the four LSTs per daily cycle. We introduced two criteria to determine whether to use the DTC model for estimating Tdm or not (Fig. 2, Scenario no. 1 to no. 3).
The first criterion is based on the diurnal temperature range (DTR), which was calculated as the maximum minus the minimum LSTs within a diurnal cycle. Specifically, the DTR calculated by four LSTs within the diurnal cycle (termed DTRfour) was used (Fig. 2). Here these four daily LSTs can consist of both cloud-free observations (Tin_cloud_free, the green circles in Fig. 4) and under-cloud LSTs reconstructed by the ATC model (Tin_ATC, the blue triangles in Fig. 4). For relatively small DTRfour, e.g., on overcast days with heavy clouds or on days with low incoming solar radiation (e.g., polar nights), Tdm can be directly estimated as the mean of the four daily LSTs per daily cycle (Fig. 4a). In this case, the DTC model would be unnecessary. We empirically set the DTRfour threshold as 5.0 K (see Sect. 5.1 for detailed discussions). In other words, when the DTRfour is less than 5.0 K (see Scenario no. 1 in Figs. 2 and 4a), Tdm estimated with the IADTC framework (termed Tdm_IADTC) was obtained by averaging the four LSTs within a diurnal cycle (termed Tdm_ATC_four).
When DTRfour is greater than 5.0 K, the DTC model would be used to simulate the diurnal LST dynamics. However, for the global generation of Tdm, the simulation can still fail for cases with complicated diurnal LST dynamics (Fig. 4c). To avoid this issue, we introduced the second criterion to determine whether to use the DTC model or not. This was done by comparing the DTRfour and the DTR calculated by the DTC model (termed DTRDTC). This comparison can be used to identify the failed simulations of the DTC model because the DTRDTC would be abnormal once the LSTs modeled by the DTC model are significantly underestimated around sunrise. Therefore, we employed the absolute difference between DTRDTC and DTRfour (termed as ΔDTR) as the second threshold to further determine whether to use the DTC model or not. This study empirically set the ΔDTR threshold as 20.0 K. More discussions on this are provided in Sect. 5.1.
In the practical generation of Tdm, when DTRfour≥5.0 K and ΔDTR <20.0 K (Scenario no. 2 in Fig. 2), the DTC modeling results (Tin_ATC_DTC; see the blue line in Fig. 4b) are satisfactory and were then used to estimate Tdm. The Tdm_IADTC was then calculated as the average of instantaneous hourly LSTs (Tin_ATC_DTC). When DTRfour≥5.0 K and ΔDTR ≥20.0 K (Scenario no. 3 in Fig. 2), the DTC model may fail (Fig. 4c) as the Tdm estimate based on the DTC modeling (i.e., Tdm_ATC_DTC) is considerably lower than the true Tdm. In this case, the error of Tdm_ATC_DTC can be even larger than that of Tdm estimated as the average of the four LSTs within the day (i.e., Tdm_ATC_four; refer to Fig. 11 in Sect. 5.1). Therefore, in this case, Tdm_IADTC was directly calculated as Tdm_ATC_four. In summary, for Scenarios no. 1 and 3, Tdm_IADTC was calculated as Tdm_ATC_four, while it was calculated as Tdm_ATC_DTC for Scenario no. 2.
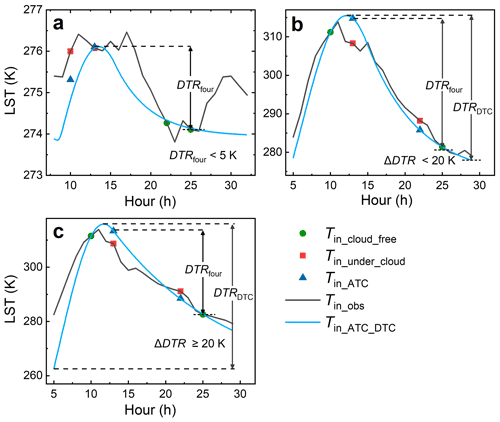
Figure 4Estimation of Tdm under different conditions. Panel (a) displays an example of estimating Tdm by averaging Tin_cloud_free and Tin_ATC when DTRfour is less than 5.0 K (i.e., Scenario no. 1); panel (b) displays an example of estimating Tdm based on the DTC modeling results (i.e., Scenario no. 2); panel (c) displays an example of estimating Tdm by averaging Tin_cloud_free and Tin_ATC when ΔDTR is equal or greater than 20.0 K (i.e., Scenario no. 3). The green circles, red rectangles, and blue triangles denote the instantaneous cloud-free LST observations, under-cloud LST observations, and under-cloud LSTs reconstructed by the ATC model, respectively. The black lines denote the in situ LST observations, while the blue lines show the DTC-modeled values based on the cloud-free LST observations and ATC-modeled under-cloud LSTs. Note that hours larger than 24 along the x axis correspond to the next day.
3.2 Validations
The GADTC products were validated from the following two aspects: (1) validating the IADTC framework indirectly with single-source in situ measurements at the site level and (2) validating the GADTC products directly by comparing with in situ measurements. These two aspects complement each other and allow us to assess the applicability of the IADTC framework and the accuracy of the generated GADTC products. The direct comparison of the GADTC product with in situ measurements (SURFRAD and FLUXNET measurements for this study) provides information on the accuracy of the IADTC framework, especially over homogeneous areas (Guillevic et al., 2018). However, such direct validations can be affected by uncertainties beyond the IADTC framework, e.g., a mismatch of spatial scale between satellite and in situ measurements, different observation angles, and uncertainties from the LST retrieval algorithm (Ermida et al., 2014; Guillevic et al., 2014; Li et al., 2014). Therefore, direct comparisons may not fully reflect the true accuracy of the IADTC framework. To address this issue and assess the applicability of IADTC framework, we validated the IADTC framework indirectly by driving it with in situ measurement and then using hourly measurements for validation. This strategy avoids the mismatch issue of multi-source data and can, therefore, better reflect the accuracy of the IADTC framework (Hong et al., 2021).
3.2.1 Validation of the IADTC framework with in situ measurements
The IADTC framework was validated with in situ hourly measurements obtained exclusively from SURFRAD and FLUXNET data. During this validation process, the MERRA2 air temperature at the corresponding station location, instead of the air temperature from in situ measurements, was used to drive the ATC model, which is identical to the actual generation of the GADTC products.
The approach used the cloud-free in situ measurements at each MODIS overpass time and MERRA2 air temperatures to drive the ATC model and the corresponding under-cloud in situ measurements (Tin_under_cloud, red rectangles in Fig. 4) to evaluate the accuracy of the under-cloud LSTs reconstructed by the ATC model (Tin_ATC). The accuracy of the Tdm estimated with the IADTC framework (Tdm_IADTC) was evaluated against “true” Tdm (termed Tdm_true), i.e., the average of the hourly in situ measurements (Tin_obs, gray line in Fig. 4). We also provided the sampling bias (ΔTsb) of the traditional method based on cloud-free observations (i.e., the average of Tin_cloud_free), which here is termed Tdm_cloud_free. Therefore, the accuracy improvements of Tdm_IADTC compared to Tdm_cloud_free are reflected in the corresponding reduction of ΔTsb. We further provide Tdm estimated with the OADTC framework (termed Tdm_OADTC) to illustrate the improvement achieved by the IADTC framework.
3.2.2 Validation of the GADTC product directly with in situ measurements
After matching the geolocation and observation time, we directly compared the GADTC product with in situ Tdm measurements from SURFRAD and FLUXNET. Note that outliers in the in situ measurements were removed before performing the accuracy evaluation; here outliers are defined as the Tdm differences between in situ measurements and GADTC products deviating by more than 3σ (3 standard deviations) from the mean (Göttsche et al., 2016; Zhang et al., 2020).
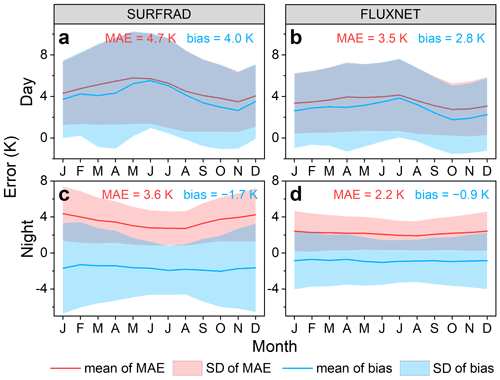
Figure 5Validations of reconstructed under-cloud LSTs at Aqua and Terra day and night overpass times based exclusively on in situ data. The under-cloud LSTs were reconstructed with the ATC model. Panels (a) and (b) show monthly mean errors obtained for daytime overpasses (including Aqua day and Terra day) for SURFRAD and FLUXNET data, respectively; (c) and (d) show the same for the nighttime overpasses (including Aqua night and Terra night).
3.3 Analysis of the GADTC product
We analyzed the difference in LST values and trends between Tdm_cloud_free (the daily mean LST estimated by the traditional method) and the GADTC products. For the difference in LST values, we present the global spatial distribution of ΔTsb using the GADTC product as the reference (see Sect. 4.3). For the difference in LST trends, the seasonal Mann–Kendall test and Theil–Sen slope were used to diagnose the warming/cooling trend of LST and describe its slope, respectively (see Sect. 4.4). The seasonal Mann–Kendall test is a nonparametric test suitable to detect LST warming/cooling trends and to quantify the associated significance level in LST time series (Hirsch et al., 1982; Hussain and Mahmud, 2019), while the Theil–Sen slope reduces the impact of outliers on LST trend analysis (Sen, 1968; Theil, 1950). We conducted a seasonal Mann–Kendall test for both the Tdm_cloud_free and the GADTC product and compared their Theil–Sen slopes in describing global LST trends.
4.1 Validation of the IADTC framework with in situ measurements
The validations using the SURFRAD measurements show that the MAE and bias of the ATC model for the day are 4.7 and 4.0 K, respectively, while those for the night are 3.6 and −1.6 K, respectively (Fig. 5a and c). Although the results for the ATC model are less satisfactory, the Tdm accuracies estimated with the IADTC framework are generally acceptable: the MAEs of Tdm_IADTC at the daily and monthly scales are 1.4 and 0.6 K, respectively and the corresponding biases are both −0.2 K (Fig. 6). By contrast, the MAEs of the Tdm_cloud_free are 4.1 and 2.5 K at the daily and monthly scales, respectively; i.e., they indicate a significantly lower accuracy compared to that of Tdm_IADTC.
The proportions of the three scenarios were 0.2 %, 95.0 %, and 4.8 %, respectively. In Scenarios no. 1 and no. 3 under which the accuracies were improved compared with the OADTC framework, the IADTC framework improves the MAE of estimated Tdm by around 0.45 K (from 2.80 to 2.35 K; see Fig. B1a). The accuracy improvement results mainly from two aspects: (1) the IADTC framework reduces the systematic negative bias caused by cases for which the DTC-modeled LSTs are significantly underestimated around sunrise; and (2) the outliers due to failed DTC simulations are avoided. The overall accuracies for all three scenarios show that the IADTC framework improves the bias from −0.38 to −0.18 K, while the MAE improvement is relatively small. The relatively slight increase in the overall accuracy is attributed to the relatively small proportion of Scenarios no. 1 and no. 3 (around 5 %).
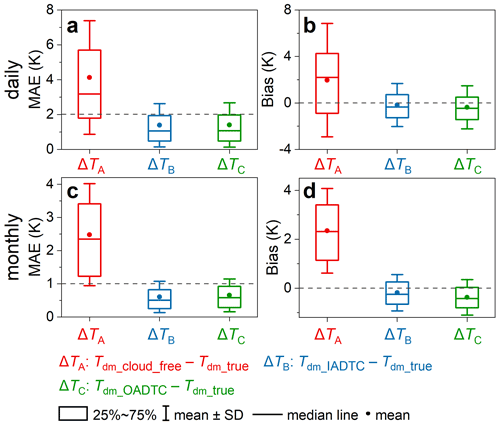
Figure 6Validations of daily mean LST (Tdm) estimation with SURFRAD data. Box plots show the errors for the traditional Tdm estimation method (Tdm_cloud_free), the IADTC framework (Tdm_ATC_DTC), and the OADTC framework (Tdm_OADTC). Panels (a) and (b) display the MAE and bias at the daily scale, respectively, and panels (c) and (d) display the MAE and bias at the monthly scale, respectively.
The validations using the FLUXNET data are similar to those with the SURFRAD data: (1) the IADTC framework significantly reduces the ΔTsb of Tdm_cloud_free; (2) the MAEs of Tdm_IADTC are 1.1 and 0.5 K at the daily and monthly scales, respectively; and (3) the biases are both close to zero (Fig. 7). The validations again indicate that the under-cloud LSTs reconstructed by the ATC model are systematically positive during the day (the MAE and bias are 3.5 and 2.8 K, respectively) and systematically negative during the night (the MAE and bias are 2.2 and −0.9 K, respectively) (Fig. 5b and d).
The proportion of each scenario is 10.2 %, 82.5 %, and 7.3 %, respectively. Compared with the OADTC framework, in Scenarios no. 1 and no. 3 (the proportion is 17.4 %) under which the accuracies are considerably improved, the IADTC framework improved the MAE of the estimated Tdm by around 0.78 K (from 1.95 to 1.17 K; refer to Fig. B1b). However, for all the three scenarios, the overall MAE and bias improvements of the IADTC framework are around 0.15 and 0.30 K, respectively (Fig. 7).
4.2 Evaluation of the GADTC product with in situ measurements
The comparison between the GADTC products and in situ measurements (SURFRAD and FLUXNET datasets) shows that the MAEs of the GADTC products are 3.0 and 2.6 K at the daily and monthly scales, respectively, and the mean bias on both scales is −1.5 K (Fig. 8). The MAE and bias are larger than those of the IADTC framework at site level (Fig. 6). This is thought to be due to inconsistencies between MODIS cloud-free observations and in situ measurements, i.e., errors of MODIS cloud-free observations propagating into the GADTC products. The mismatch in spatial resolution between the GADTC products and in situ measurements can also lead to lower accuracies.
The validation with the SURFRAD measurements show that the MAE of the GADTC products is 2.2 and 1.6 K at the daily and monthly scales, respectively, and the bias is around −1.6 K at both scales (Fig. 8a and d). These accuracies of daily mean LST are generally on a par with those of instantaneous LSTs in studies comparing instantaneous MODIS cloud-free observations and SURFRAD measurements (Duan et al., 2019; Martin et al., 2019). Across the different SURFRAD sites, the MAEs of the GADTC products are relatively similar (around 2.2 K; see Table 1).
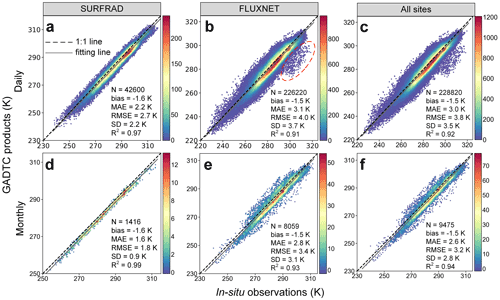
Figure 8GADTC products versus in situ observations. Panels (a), (b), and (c) compare the daily mean LST over the SURFRAD, FLUXNET and combined sites, respectively, and panels (d), (e), and (f) show the corresponding results for monthly mean LST. The biases were calculated by the GADTC products minus the in situ measurements. The red ellipse in (b) highlights the cases with notably large errors.
Table 1Validation results obtained over the seven SURFRAD sites.
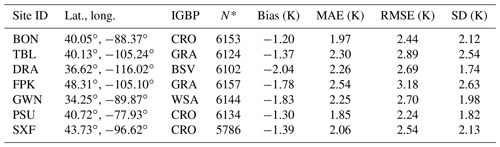
* N denotes the number of days used for validation.
The direct comparison between the GADTC products and FLUXNET measurements shows that the MAEs are 3.1 and 2.8 K at the daily and monthly scales, respectively, and the bias at these two timescales is −1.5 K (Fig. 8b and e). Compared with the validations over the SURFRAD sites, the accuracies over the FLUXNET sites decrease slightly, and the standard deviations increase. The relatively larger errors at several FLUXNET sites (e.g., AU-Wac, SJ-Adv, and CH-Fru sites, with MAEs larger than 8.0 K; refer to the red ellipse in Fig. 8e) partly account for the lower accuracy. The relatively large errors at these sites might be related to the erroneous in situ measurements as well as the high spatial heterogeneity around these sites. However, the accuracies at most FLUXNET sites are acceptable.
The validations over the FLUXNET sites show that the MAEs vary from 2.6 to 4.8 K and depend on land cover type. Relatively lower accuracies of the GADTC products (MAE larger than 3.5 K) are observed over IGBP land cover types OSH (open shrublands) and SNO (snow and ice) (Table 2). This may be related to unusually large measurement errors and the relatively high spatial heterogeneity at some sites as well as the limited number of sites representing a particular land cover type. For example, the accuracy assessment over the SNO land cover type is performed with a single site, and there are only three sites of the OSH land cover type (e.g., the RU-Cok with MAE as large as 4.6 K).
4.3 Analysis of the GADTC product
The validations based exclusively on in situ LST measurements (Fig. 6) show that the IADTC framework can reduce the sampling bias (ΔTsb, i.e., Tdm_cloud_free−Tdm_true) significantly, especially at the monthly scale. ΔTsb directly affects the value of Tdm and may further influence the LST trend. Therefore, based on the GADTC products, we analyzed the global distribution of ΔTsb (calculated by Tdm_cloud_free−Tdm_IADTC) at the monthly scale (Sect. 4.3.1) and compared the LST trend differences between monthly averaged Tdm_cloud_free and Tdm_IADTC to study the impact of ΔTsb on LST trends (Sect. 4.3.2).
4.3.1 Global distribution of the sampling bias ΔTsb
The global distribution of the averaged ΔTsb from 2003 to 2019 shows that the global-mean ΔTsb is 1.8 K (Fig. 9). At low-latitude and midlatitude regions, ΔTsb is generally around 2.0 K, yet it can exceed 4.0 K in some regions, e.g., deserts. At high-latitude regions, ΔTsb is close to or slightly less than zero. ΔTsb also varies with month or season (Fig. C1). For example, the average ΔTsb for September–October–November (2.0 K) is larger than that for December–January–February (1.5 K). We further observe that ΔTsb is sensitive to land cover type and that DTR can partially explain ΔTsb. For instance, regions with a large DTR (e.g., deserts or bare soils) usually have a greater ΔTsb (Sharifnezhadazizi et al., 2019; Hong et al., 2021; Jin and Dickinson, 2010).
Apart from the DTR, in high-latitude regions, ΔTsb can also be affected by the drift of MODIS overpass time. The DTR is relatively small in high-latitude regions where the angle of the incident solar radiation is low and the LST observations across a diurnal cycle are often already close to the true Tdm, leading to a relatively small ΔTsb. The time drift at high-latitude regions can also contribute to the relatively small ΔTsb. At low-latitude and midlatitude regions, MODIS samples the surface near 10:30, 13:30, 22:30, and 01:30 (local solar time) (Fig. A1): the systematic positive ΔTsb is then mostly due to the under-sampling of the nighttime cooling until the sunrise of the next day (Hong et al., 2021). At high-latitude regions, the time drift effect allows MODIS observations to be conducted at other than these four times and alleviates the under-sampling of nighttime cooling, thereby reducing ΔTsb.
4.3.2 Analysis of global LST trends from 2003 to 2019
The LST trends determined for Tdm_cloud_free and Tdm_IADTC show similar global patterns; i.e., both can show comparable warming/cooling trends (Fig. 10a and b). For example, they both display an overall increasing LST trend over the globe as well as an accelerated surface warming trend over the Arctic and Europe (Fig. 10), which is consistent with most previous studies (Mao et al., 2017; Sobrino et al., 2020a, b).
However, the slopes of the LST trends are significantly different between Tdm_cloud_free and Tdm_IADTC with a MAE of 0.012 K yr−1 (Fig. 10e). The slope difference is related to the variation of ΔTsb, which can be affected by the cloud percentage and cloud duration among different months. When taking the slope of Tdm_IADTC as reference, the slope of Tdm_cloud_free underestimates the global LST warming rate by 0.004 K yr−1. With the original MODIS LST observations (i.e., Tdm_cloud_free) as reference, the warming LST trends would be underestimated over South America, Africa, Asia, and Oceania. They would be overestimated over Europe and relatively similar to the trends obtained with Tdm_IADTC over North America and Antarctica.
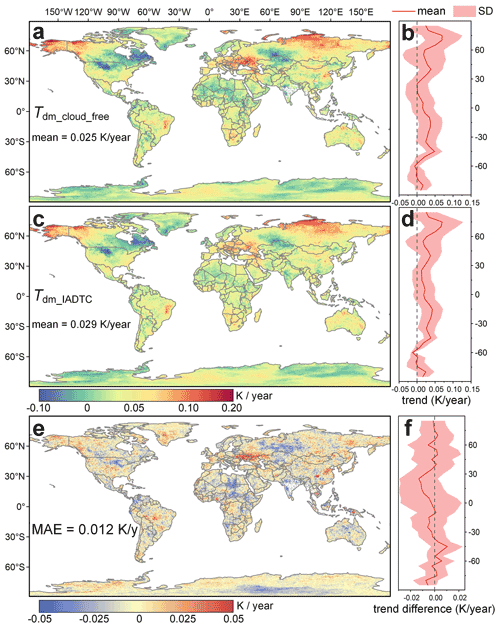
Figure 10Global LST trends from 2003 to 2019. Panels (a) and (b) display the global LST trends based on Tdm_cloud_free and their averaged results for 5∘ longitudinal intervals, respectively; panels (c) and (d) show the corresponding results for Tdm_IADTC; and panels (e) and (f) show the global LST trend differences between Tdm_cloud_free and Tdm_IADTC and their averages for 5∘ longitudinal intervals, respectively.
5.1 Empirical determination of the threshold for optimizing the Tdm estimation with DTC model
To determine the threshold for the first criterion (i.e., the threshold for the DTRfour; see Fig. 2), we analyzed the variations in the error of Tdm_ATC_four depending on DTRfour using SURFRAD and FLUXNET data (Fig. 11). The assessments show that the errors of Tdm_ATC_four generally increase with DTRfour. The linear fitting lines show that the error of Tdm_ATC_four is relatively low when DTRfour is small. In other words, the direct average of the four LSTs per daily cycle (Tdm_ATC_four) is a good estimate of Tdm when the DTRfour is small. Based on the linear fits in Fig. 11a, b, and c, we therefore chose the DTRfour threshold of the first criterion to be 5.0 K.
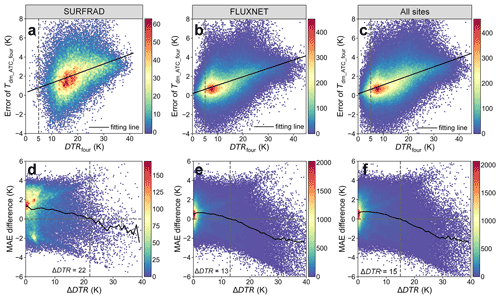
Figure 11Threshold determination for the two criteria in Fig. 2. Panels (a), (b), and (c) display the errors of Tdm_ATC_four (Tdm_ATC_four minus Tdm_true) depending on DTRfour for SURFRAD, FLUXNET, and combined data, respectively; and panels (d), (e), and (f) display the MAE differences between Tdm_ATC_four and Tdm_ATC_DTC (i.e., the MAE of Tdm_ATC_four minus the MAE of Tdm_ATC_DTC) depending on the ΔDTR for SURFRAD, FLUXNET, and combined data, respectively. The black lines in (d), (e), and (f) denote the averaged MAE difference within every unit along the x axis.
The second criterion uses the ΔDTR to filter cases for which Tdm is significantly underestimated. To determine the optimal threshold for ΔDTR, we analyzed the MAE differences between Tdm_ATC_four and Tdm_ATC_DTC (i.e., the MAE of Tdm_ATC_four minus the MAE of Tdm_ATC_DTC) and their dependence on ΔDTR for SURFRAD and FLUXNET data (Fig. 11d and e). The assessments show that ΔDTR is generally less than 10 K, and the accuracy of Tdm_ATC_DTC is better than that of Tdm_ATC_four. However, with the increase of ΔDTR, the overall accuracy of Tdm_ATC_four can be superior to Tdm_ATC_DTC. For SURFRAD data, the overall accuracy of Tdm_ATC_four is better than that of Tdm_ATC_DTC once ΔDTR exceeds 22.0 K (i.e., the ΔDTR threshold is 22.0 K), while this threshold is 13.0 K for FLUXNET data. With the further increase of ΔDTR, the accuracy of Tdm_ATC_DTC can be even lower than that of Tdm_ATC_four, e.g., by up to 2.0 K in Fig. 11d and e. In other words, Tdm can be estimated more accurately with Tdm_ATC_four than Tdm_ATC_DTC once ΔDTR is relatively large (i.e., Scenario no. 3).
Note that the optimal threshold of ΔDTR for the SURFRAD data (22.0 K) differs from that for the FLUXNET data (13.0 K). Here, we set the ΔDTR threshold as 20.0 K, which is close to that determined for the SURFRAD data, mostly because of the following factors: (1) the SURFRAD sites have been managed uniformly by NOAA (National Oceanic and Atmospheric Administration) for over 15 years, and the associated radiance measurements have been consistently quality-controlled (Augustine et al., 2000); and (2) the land cover types of the SURFRAD sites are not limited to vegetation. We acknowledge that using a single threshold of 20.0 K may not be optimal for all climate zones and land cover types across the globe, but use of a single threshold effectively avoids outliers due to failed simulations while keeping the simplicity in the global generation of Tdm products.
With the thresholds given as above, we provide the percentage of each scenario within each 10∘ latitude zone (Fig. 12). In low-latitude and midlatitude regions, the percentage of Scenario no. 2 (i.e., DTRfour≥5.0 & ΔDTR <20.0 K) reaches over 80 %, indicating that the IADTC framework mainly uses the DTC-modeled results to estimate Tdm in those regions. With the increase of latitude, the percentage of Scenario no. 1 (i.e., DTRfour<5.0 K) gradually increases, mostly due to a decrease in DTR with the weakened incoming solar radiation over higher-latitude regions. The percentage of Scenario no. 1 reaches around 60 % in the Arctic and Antarctic, which echoes well with the small ΔTsb in high-latitude regions (Fig. 9). The percentage of Scenario no. 3 (i.e., DTRfour≥5.0 & ΔDTR ≥20.0 K) remains relatively stable at around 10 % over most regions across the globe, but it can increase to 20 % in the equatorial zone (10∘ N–10∘ S) and Antarctic, which indicates the relatively poor performance of the DTC model over these regions. The lower performance of the DTC model in the equatorial zone may be related to the high cloud percentage, while over the Antarctic, it reflects the expected difficulties over polar regions (see Sect. 5.2 for more discussions).
5.2 Possible uncertainty sources of GADTC product
GADTC products uncertainties arise from four main sources: (1) MODIS data quality or LST retrieval error, (2) cloud cover and contamination; (3) overpass time drift and linear interpolation, and (4) uncertainties associated with the IADTC framework. These four uncertainty sources can affect the under-cloud LST reconstruction with the ATC model as well as the diurnal LST dynamics modeling with the DTC model and, consequently, affect the accuracy of the GADTC products. In addition, these uncertainties can influence each other via error propagation. In the following, we discuss the four error sources and their effect in more detail.
The ATC and DTC models use cloud-free LST observations to estimate Tdm. Therefore, retrieval errors of MODIS LSTs affect the results of ATC and DTC models and the accuracies of the estimated Tdm. Figure A2a shows that the quality of MODIS LSTs in the equatorial regions is lower than that in the other regions. This suggests that GADTC products should have larger uncertainties in equatorial regions where, consequently, the IADTC framework may need further improvements.
Cloud percentage can also impact the accuracies of the GADTC products. In regions with a higher cloud percentage, e.g., the equatorial regions (Fig. A2b), more under-cloud LSTs need to be reconstructed with the ATC model. However, errors of reconstructed under-cloud LSTs are larger than those of cloud-free LSTs. Therefore, regions with a higher cloud percentage are also associated with larger errors from ATC modeling and, consequently, DTC modeling and the estimated Tdm. In polar regions, the cloud detection algorithm has larger uncertainties due to the spectral similarities between clouds and snow (Østby et al., 2014; Westermann et al., 2012), which introduces additional uncertainties to the GADTC products.
The impact of the overpass time drift mainly occurs over high-latitude regions where the time drift is larger. On the one hand, the cloud-free observations used for solving the free parameters of the ATC model come from significantly different times within a daily cycle (Fig. A1), which affects the under-cloud LST reconstruction. On the other hand, approximately 30 % of the Tdm over high-latitude regions were estimated with the DTC modeling results (i.e., Scenario no. 2; refer to Fig. 12), and the time drift can affect the shape of the DTC curve and, therefore, the estimated Tdm. Temporal normalization methods can adjust the LST observations at fluctuated overpass time to the fixed time, which can eliminate the uncertainties in the under-cloud LST reconstruction and diurnal LST dynamics modeling (Ma et al., 2022; Z. Liu et al., 2019; Duan et al., 2014).
The uncertainties of the GADTC products derived with the IADTC framework mainly include three parts: the reconstruction error of the ATC model, the fitting error of the DTC model, and the choice of the two thresholds. First, the currently used ATC model reconstructs under-cloud LSTs during the day (night) with small positive (negative) biases (Fig. 5), even though information on under-cloud air temperature has been incorporated (Z. Liu et al., 2019). Additionally, the errors in the ATC model can affect the determination of scenarios and, consequently, the way to calculate the Tdm. Second, the DTC model assumes clear-sky conditions and is less capable of simulating under-cloud LST dynamics accurately, which introduces additional uncertainties, especially under some complex situations (Hong et al., 2021). Third, the two fixed thresholds for DTRfour and ΔDTR were determined empirically (Fig. 11): the threshold for DTRfour may introduce additional uncertainty over high-latitude regions with small DTRs, while the threshold for ΔDTR may still miss some cases with unrealistic modeling results.
It is difficult to distinguish and quantify the individual contributions of these four uncertainty sources to the estimated Tdm, as they can affect the ATC and DTC modeling individually and interactively. We are therefore unable to provide a quality control flag for each pixel of the GADTC products. The validations have shown that the accuracies of the GADTC products are generally acceptable over most areas across the globe. However, there are relatively larger uncertainties over equatorial and polar regions, where further validations of the GADTC products and an optimization of the IADTC framework are required.
5.3 Future perspectives
Further improvements of the GADTC product can focus on the following three aspects:
-
More extensive validation and inter-comparison of the GADTC products. The GADTC products have been evaluated with FLUXNET and SURFRAD datasets, which include in situ measurements from most climate zones. However, the number of sites is very limited in regions where the uncertainties of the GADTC products are largest (e.g., equatorial and polar regions; refer to Fig. 1). It is therefore hard to validate the IADTC framework as well as its improvements over these regions, e.g., the use of a multi-type ATC model instead of a single-type ATC model. The current in situ data are also insufficient to verify the accuracies of the GADTC products over these regions. It is therefore necessary to obtain more in situ measurements over these regions to validate the accuracy of IADTC framework as well as the GADTC product more completely. Furthermore, reanalysis data, which provide long-term spatiotemporally seamless LSTs and have been widely used in relevant studies (Simmons et al., 2017), can be used to assess the GADTC products (Trigo et al., 2015).
-
Rapid generation of high-resolution spatiotemporally seamless Tdm product. Considering the limited computing resources as well as the aim of this study to obtain the spatial distribution of ΔTsb and LST trends on a global scale, the spatiotemporally seamless daily Tdm values were generated at a spatial resolution of 0.5∘. However, the current IADTC framework is equally suitable to generate spatiotemporally seamless daily 1 km Tdm. For local-scale studies, the IADTC framework can probably be applied directly, while for large-scale (continent-scale or even global-scale) studies or applications, the generation of 1 km spatiotemporally seamless daily Tdm could be computationally unaffordable. Under this circumstance, apart from using as many computation resources as possible, we can resort to three strategies to substantially reduce computational complexity.
First, the similarity of the ATC and DTC model parameters among neighboring pixels can be utilized to accelerate the calculation speed considerably (Hong et al., 2021; Hu et al., 2020; Zhan et al., 2016). Second, the physically based IADTC framework can also be integrated with some statistical or empirical estimation strategies (both on Tdm or on ΔTsb) to help improve the computational efficiency (Xing et al., 2021). This is reasonable as ΔTsb (and Tdm) is generally related to local surface properties (Figs. 9 and 11). For example, for large-scale or global high-resolution generation of spatiotemporally seamless daily 1 km Tdm, the IADTC framework can be run in some chosen sample regions to obtain adequate training samples of Tdm (or ΔTsb). Based on these samples, statistical relationships between Tdm (ΔTsb) and the related variables such as the four daily LSTs, latitude, land cover type, elevation, and cloud percentage can be obtained to help estimate the Tdm (ΔTsb) across the globe efficiently. Furthermore, the training samples of Tdm (ΔTsb) can also be from geostationary satellite data, which can help reduce the computational complexity of the DTC modeling. Third, other highly efficient under-cloud LST reconstruction methods, such as statistical interpolation, spatiotemporal fusion, and the passive microwave-based method (Wu et al., 2021; Hong et al., 2021), or the generated under-cloud LST products (Zhang et al., 2022; Zhao et al., 2020) can replace the ATC model in the Tdm generation framework. Similarly, more efficient diurnal LST dynamics modeling methods can also replace the DTC model (Jia et al., 2022).
-
Generation of Tdm with a longer time span. The GADTC products can only date back to 2003 because the IADTC framework requires four observations per day to estimate Tdm, while MODIS started to provide four daily observations in 2003. However, daily mean LSTs with a longer time span are strongly required for relevant studies such as climate change analysis (Jin and Dickinson, 2010; Simmons et al., 2017). AVHRR data provide global LST observations before 2000, and recent studies have achieved tremendous progress in the correction of orbit drift in order to generate more accurate AVHRR LST datasets (Julien and Sobrino, 2012; Latifovic et al., 2012; Ma et al., 2020; X. Liu et al., 2019). However, the current IADTC framework is not applicable to AVHRR since it only samples the surface twice per day. It is therefore imperative to develop a framework for Tdm estimation that also suits AVHRR-like LSTs. Apart from polar orbiters, geostationary satellites and reanalysis data deliver LST over similar time spans. Although reanalysis data are still limited by their coarse spatial resolution and geostationary satellite data have a limited spatial coverage, especially over polar regions, the fusion of these datasets has great potential to help generate Tdm with a longer time span (Long et al., 2020; Quan et al., 2018).
The generated GADTC products are organized yearly and are freely available at https://doi.org/10.5281/zenodo.6287052 (Hong et al., 2022). Each file contains the global day-to-day spatiotemporal seamless daily mean land surface temperature in units of kelvin.
MODIS LST products have been widely used for long-term time-series analyses. However, due to the missing LSTs caused by clouds and under-sampling of the diurnal LST dynamics, currently there is still no global dataset of spatiotemporally seamless daily mean LST (Tdm) with an acceptable systematic sampling bias (ΔTsb), which is caused by averaging only instantaneous cloud-free observations. To resolve this issue, we proposed the IADTC framework by using a more advanced ATC model as well as by optimizing the estimation of Tdm with the DTC model and generated global spatiotemporally seamless Tdm products (i.e., the GADTC products) from 2003 to 2019. Based on SURFRAD and FLUXNET in situ measurements, the IADTC framework was validated with in situ measurements at the site level, and the GADTC products were directly compared with in situ Tdm observations. The validations with the SURFRAD and FLUXNET measurements reveal that the IADTC framework is able to reduce the systematic positive sampling bias (ΔTsb) of Tdm_cloud_free, avoid the outliers caused by failed simulation, and provide relatively accurate estimates of spatiotemporally seamless Tdm. Based on the GADTC products, we analyzed the global distribution of ΔTsb and examined the similarities and differences between the GADTC products and Tdm_cloud_free (daily mean LST based on cloud-free observations).
Our major conclusions are as follows: (1) the validations of the IADTC framework based exclusively on in situ measurements at the site level show MAEs of 1.4 and 1.1 K for the SURFRAD and FLUXNET measurements, respectively; the biases for these two datasets are both close to zero. (2) The comparisons between the GADTC satellite products and in situ Tdm observations show that the MAEs for the SURFRAD and FLUXNET measurements are 2.2 and 3.1 K, respectively; the associated biases for these two datasets are −1.6 and −1.5 K, respectively. (3) The global-mean sampling bias ΔTsb is 1.8 K; it is usually larger than 2.0 K over low-latitude and midlatitude regions and close to zero over high-latitude regions. (4) Global-mean LST trends derived with the GADTC product and the traditional direct-averaging method are similar (both between 0.025 to 0.029 K yr−1 from 2003 to 2019), while the pixel-based MAE in LST trend derived with these two methods is 0.012 K yr−1. Despite its limitations, the proposed IADTC framework allows for the practical generation of global spatiotemporally seamless Tdm and provides insights for generating global long-term high-resolution (e.g., 1 km) Tdm products. The generated GADTC product should be helpful for relevant applications such as climate change analysis and thermal environment investigations.
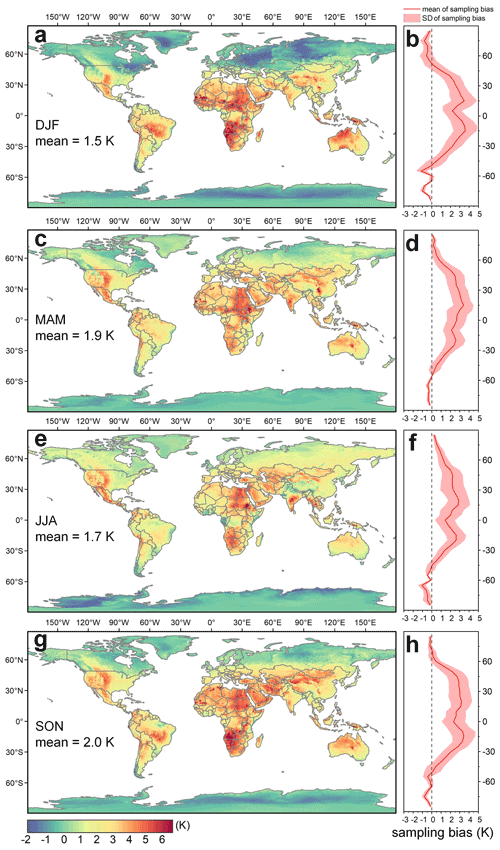
Figure C1Average sampling bias ΔTsb for indicated 3-month interval between 2003 and 2019. Panel (a) displays the ΔTsb for December–January–February (DJF), and (b) displays the corresponding results averaged over 5∘ longitudinal intervals. Similarly, (c) and (d), (e) and (f), and (g) and (h) display the corresponding results for March–April–May (MAM), June–July–August (JJA), and September–October–November (SON), respectively.
Acronyms | |
ASTER | Advanced Spaceborne Thermal Emission and Reflection Radiometer |
ATC | annual temperature cycle |
AVHRR | Advanced Very High-Resolution Radiometer |
BSV | barren sparse vegetation |
CRO | croplands |
DBF | deciduous broadleaf forests |
DOY | day of year |
DTC | diurnal temperature cycle |
DTR | daily temperature range |
EBF | evergreen broadleaf forests |
ENF | evergreen needleleaf forests |
GADTC | global daily mean LST product generated with the improved ADTC-based framework |
GOES | Geostationary Operational Environmental Satellite |
GRA | grasslands |
IADTC | framework improved ADTC-based framework |
IGBP | International Geosphere-Biosphere Programme |
LST | land surface temperature |
MAE | mean absolute error |
MERRA-2 | Modern-Era Retrospective analysis for Research and Applications version 2 |
MF | mixed forests |
MODIS | Moderate-Resolution Imaging Spectroradiometer |
MSG-SEVIRI | the Spinning Enhanced Visible and Infrared Imager onboard Meteosat Second Generation |
OADTC framework | original ADTC-based framework |
OSH | open shrublands |
SAV | savannas |
SAT | surface air temperature |
SNO | snow and ice |
SURFRAD | Surface Radiation Budget Network |
WET | permanent wetlands |
WSA | woody savannas |
Symbol representation | |
DTRfour | diurnal temperature range calculated by the four LSTs which include the cloud-free |
LSTs and ATC-reconstructed LSTs | |
DTRDTC | diurnal temperature range calculated by the DTC model |
ΔDTR | the difference between DTRDTC and DTRfour |
Tdm | daily mean LST |
Tdm_ATC_DTC | daily mean LST calculated by frequently sampling diurnal LST dynamics modeled by |
DTC model with cloud-free LST observations and under-cloud LSTs reconstructed by ATC model | |
Tdm_ATC_four | daily mean LST calculated by averaging cloud-free LST observations and under-cloud |
LSTs reconstructed by ATC model | |
Tdm_cloud_free | daily mean LST calculated by averaging cloud-free LST observations |
Tdm_IADTC | daily mean LST estimated with the IADTC framework |
Tdm_OADTC | daily mean LST estimated with the OADTC framework |
Tdm_true | true daily mean LST for validation |
Tin_ATC | instantaneous under-cloud LSTs reconstructed by ATC model |
Tin_ATC_DTC | diurnal LST dynamics modeled by DTC model with cloud-free LST observations and |
under-cloud LSTs reconstructed by ATC model | |
Tin_cloud_free | instantaneous cloud-free LST observations |
Tin_obs | hourly LST observations |
Tin_under_cloud | instantaneous under-cloud LST observations |
ΔTsb | sampling bias |
FH and WZ designed the research. FH implemented the research and wrote the original manuscript. WZ supervised the research. All co-authors revised the manuscript and contributed to the writing.
The contact author has declared that none of the authors has any competing interests
Publisher's note: Copernicus Publications remains neutral with regard to jurisdictional claims in published maps and institutional affiliations.
The authors wish to thank the following organizations for providing the data to support this study, including (1) the Global Radiation group of the Earth System Research Laboratory Global Monitoring Division managed by the National Oceanic and Atmospheric Administration (NOAA) for providing SURFRAD data, (2) the Land Processes Distributed Active Archive Center (LP DAAC) managed by the National Aeronautics and Space Administration (NASA) Earth Science Data and Information System (ESDIS) project for providing MOD11C1 and MYD11C1 products, (3) the FLUXNET network hosted by the Lawrence Berkeley National Laboratory for providing the FLUXNET2015 dataset, and (4) NASA's Goddard Space Flight Center for providing MERRA-2 data.
This research has been supported by the National Natural Science Foundation of China (grant no. 42171306), the Natural Science Foundation of Jiangsu Province (grant no. BK20180009), and the National Youth Talent Support Program of China.
This paper was edited by Nellie Elguindi and reviewed by three anonymous referees.
Alcântara, E. H., Stech, J. L., Lorenzzetti, J. A., Bonnet, M. P., Casamitjana, X., Assireu, A. T., and Novo, E. M. L. d. M.: Remote sensing of water surface temperature and heat flux over a tropical hydroelectric reservoir, Remote Sens. Environ., 114, 2651–2665, https://doi.org/10.1016/j.rse.2010.06.002, 2010.
Anderson, M. C., Norman, J. M., Mecikalski, J. R., Otkin, J. A., and Kustas, W. P.: A climatological study of evapotranspiration and moisture stress across the continental United States based on thermal remote sensing: 2. Surface moisture climatology, J. Geophys. Res.-Atmos., 112, D11112, https://doi.org/10.1029/2006JD007507, 2007.
Augustine, J. A., DeLuisi, J. J., and Long, C. N.: SURFRAD–A National Surface Radiation Budget Network for Atmospheric Research, B. Am. Meteorol. Soc., 81, 2341–2358, https://doi.org/10.1175/1520-0477(2000)081<2341:SANSRB>2.3.CO;2, 2000.
Bechtel, B.: A new global climatology of annual land surface temperature, Remote Sens., 7, 2850–2870, https://doi.org/10.3390/rs70302850, 2015.
Cao, S. and Sanchez-Azofeifa, A.: Modeling seasonal surface temperature variations in secondary tropical dry forests, Int. J. Appl. Earth Obs., 62, 122–134, https://doi.org/10.1016/j.jag.2017.06.008, 2017.
Crosson, W. L., Al-Hamdan, M. Z., Hemmings, S. N. J., and Wade, G. M.: A daily merged MODIS Aqua–Terra land surface temperature data set for the conterminous United States, Remote Sens. Environ., 119, 315–324, https://doi.org/10.1016/j.rse.2011.12.019, 2012.
Duan, S.-B., Li, Z.-L., Tang, B.-H., Wu, H., and Tang, R.: Generation of a time-consistent land surface temperature product from MODIS data, Remote Sens. Environ., 140, 339–349, https://doi.org/10.1016/j.rse.2013.09.003, 2014.
Duan, S.-B., Li, Z.-L., Wu, H., Leng, P., Gao, M., and Wang, C.: Radiance-based validation of land surface temperature products derived from Collection 6 MODIS thermal infrared data, Int. J. Appl. Earth Obs., 70, 84–92, https://doi.org/10.1016/j.jag.2018.04.006, 2018.
Duan, S.-B., Li, Z.-L., Li, H., Göttsche, F.-M., Wu, H., Zhao, W., Leng, P., Zhang, X., and Coll, C.: Validation of Collection 6 MODIS land surface temperature product using in situ measurements, Remote Sens. Environ., 225, 16–29, https://doi.org/10.1016/j.rse.2019.02.020, 2019.
Eleftheriou, D., Kiachidis, K., Kalmintzis, G., Kalea, A., Bantasis, C., Koumadoraki, P., Spathara, M. E., Tsolaki, A., Tzampazidou, M. I., and Gemitzi, A.: Determination of annual and seasonal daytime and nighttime trends of MODIS LST over Greece – climate change implications, Sci. Total Environ., 616–617, 937–947, https://doi.org/10.1016/j.scitotenv.2017.10.226, 2018.
Ermida, S. L., Trigo, I. F., DaCamara, C. C., Göttsche, F. M., Olesen, F. S., and Hulley, G.: Validation of remotely sensed surface temperature over an oak woodland landscape – The problem of viewing and illumination geometries, Remote Sens. Environ., 148, 16–27, https://doi.org/10.1016/j.rse.2014.03.016, 2014.
Ermida, S. L., Trigo, I. F., DaCamara, C. C., Jiménez, C., and Prigent, C.: Quantifying the clear-sky bias of satellite land surface temperature using microwave-based estimates, J. Geophys. Res.-Atmos., 124, 844–857, https://doi.org/10.1029/2018JD029354, 2019.
Freitas, S. C., Trigo, I. F., Macedo, J., Barroso, C., Silva, R., and Perdigão, R.: Land surface temperature from multiple geostationary satellites, Int. J. Remote Sens., 34, 3051–3068, https://doi.org/10.1080/01431161.2012.716925, 2013.
Friedl, M. A., McIver, D. K., Hodges, J. C. F., Zhang, X. Y., Muchoney, D., Strahler, A. H., Woodcock, C. E., Gopal, S., Schneider, A., Cooper, A., Baccini, A., Gao, F., and Schaaf, C.: Global land cover mapping from MODIS: Algorithms and early results, Remote Sens. Environ., 83, 287–302, https://doi.org/10.1016/S0034-4257(02)00078-0, 2002.
Fu, P.: Responses of vegetation productivity to temperature trends over continental United States from MODIS Imagery, IEEE J. Sel. Top. Appl., 12, 1085–1090, https://doi.org/10.1109/JSTARS.2019.2903080, 2019.
Fu, P. and Weng, Q.: Consistent land surface temperature data generation from irregularly spaced Landsat imagery, Remote Sens. Environ., 184, 175–187, https://doi.org/10.1016/j.rse.2016.06.019, 2016.
Gelaro, R., McCarty, W., Suárez, M. J., Todling, R., Molod, A., Takacs, L., Randles, C. A., Darmenov, A., Bosilovich, M. G., Reichle, R., Wargan, K., Coy, L., Cullather, R., Draper, C., Akella, S., Buchard, V., Conaty, A., da Silva, A. M., Gu, W., Kim, G.-K., Koster, R., Lucchesi, R., Merkova, D., Nielsen, J. E., Partyka, G., Pawson, S., Putman, W., Rienecker, M., Schubert, S. D., Sienkiewicz, M., and Zhao, B.: The Modern-Era Retrospective Analysis for Research and Applications, Version 2 (MERRA-2), J. Climate, 30, 5419–5454, https://doi.org/10.1175/JCLI-D-16-0758.1, 2017.
Global Modeling and Assimilation Office (GMAO): MERRA-2 inst1_2d_lfo_Nx: 2d,1-Hourly, Instantaneous, Single-Level, Assimilation, Land Surface Forcings V5.12.4, Greenbelt, MD, USA, Goddard Earth Sciences Data and Information Services Center (GES DISC), https://doi.org/10.5067/RCMZA6TL70BG, 2015.
Göttsche, F. M. and Olesen, F. S.: Modelling the effect of optical thickness on diurnal cycles of land surface temperature, Remote Sens. Environ., 113, 2306–2316, https://doi.org/10.1016/j.rse.2009.06.006, 2009.
Göttsche, F. M., Olesen, F. S., Trigo, I. F., Bork-Unkelbach, A., and Martin, M. A.: Long term validation of land surface temperature retrieved from MSG/SEVIRI with continuous in-situ measurements in Africa, Remote Sens., 8, 410, https://doi.org/10.3390/rs8050410, 2016.
Guillevic, P., Göttsche, F., Nickeson, J., Hulley, G., Ghent, D., Yu, Y., Trigo, I., Hook, S., Sobrino, J., Remedios, J., and Camacho, F.: Land surface temperature product validation best practice protocol, Version 1.1, in: Best practice for satellite-derived land product validation (p. 58): Land product validation subgroup (WGCV/CEOS), edited by: Guillevic, P., Göttsche, F., Nickeson, J., and Román, M., https://doi.org/10.5067/doc/ceoswgcv/lpv/lst.001, 2018.
Guillevic, P. C., Biard, J. C., Hulley, G. C., Privette, J. L., Hook, S. J., Olioso, A., Göttsche, F. M., Radocinski, R., Román, M. O., Yu, Y., and Csiszar, I.: Validation of land surface temperature products derived from the Visible Infrared Imaging Radiometer Suite (VIIRS) using ground-based and heritage satellite measurements, Remote Sens. Environ., 154, 19–37, https://doi.org/10.1016/j.rse.2014.08.013, 2014.
Gutman, G. G.: On the monitoring of land surface temperatures with the NOAA/AVHRR: Removing the effect of satellite orbit drift, Int. J. Remote Sens., 20, 3407–3413, https://doi.org/10.1080/014311699211435, 1999.
Heck, E., de Beurs, K. M., Owsley, B. C., and Henebry, G. M.: Evaluation of the MODIS collections 5 and 6 for change analysis of vegetation and land surface temperature dynamics in North and South America, ISPRS J. Photogramm., 156, 121–134, https://doi.org/10.1016/j.isprsjprs.2019.07.011, 2019.
Hirsch, R. M., Slack, J. R., and Smith, R. A.: Techniques of trend analysis for monthly water quality data, Water Resour. Res., 18, 107–121, https://doi.org/10.1029/WR018i001p00107, 1982.
Hong, F., Zhan, W., Göttsche, F.-M., Liu, Z., Zhou, J., Huang, F., Lai, J., and Li, M.: Comprehensive assessment of four-parameter diurnal land surface temperature cycle models under clear-sky, ISPRS J. Photogramm., 142, 190–204, https://doi.org/10.1016/j.isprsjprs.2018.06.008, 2018.
Hong, F., Zhan, W., Göttsche, F.-M., Lai, J., Liu, Z., Hu, L., Fu, P., Huang, F., Li, J., Li, H., and Wu, H.: A simple yet robust framework to estimate accurate daily mean land surface temperature from thermal observations of tandem polar orbiters, Remote Sens. Environ., 264, 112612, https://doi.org/10.1016/j.rse.2021.112612, 2021.
Hong, F., Zhan W., Göttsche, Frank.-M., Liu, Z., Dong, P., Fu, H., Huang, F., and Zhang, X: A global spatiotemporally seamless daily mean land surface temperature from 2003 to 2019 (v1.0), Zenodo, https://doi.org/10.5281/zenodo.6287052, 2022.
Hu, L., Sun, Y., Collins, G., and Fu, P.: Improved estimates of monthly land surface temperature from MODIS using a diurnal temperature cycle (DTC) model, ISPRS J. Photogramm., 168, 131–140, https://doi.org/10.1016/j.isprsjprs.2020.08.007, 2020.
Hussain, M. M., and Mahmud, I.: pyMannKendall: a python package for non parametric Mann Kendall family of trend tests, J. Open Source Softw., 4, 1556, https://doi.org/10.21105/joss.01556, 2019.
Jia, A., Liang, S., and Wang, D.: Generating a 2-km, all-sky, hourly land surface temperature product from Advanced Baseline Imager data, Remote Sens. Environ., 278, 113105, https://doi.org/10.1016/j.rse.2022.113105, 2022.
Jin, M.: Analysis of land skin temperature using AVHRR observations, B. Am. Meteorol. Soc., 85, 587–600, https://doi.org/10.1175/BAMS-85-4-587, 2004.
Jin, M. and Dickinson, R. E.: New observational evidence for global warming from satellite, Geophys. Res. Lett., 29, 39-31–39-34, https://doi.org/10.1029/2001GL013833, 2002.
Jin, M. and Dickinson, R. E.: Land surface skin temperature climatology: benefitting from the strengths of satellite observations, Environ. Res. Lett., 5, 044004, https://doi.org/10.1088/1748-9326/5/4/044004, 2010.
Julien, Y. and Sobrino, J. A.: The Yearly Land Cover Dynamics (YLCD) method: An analysis of global vegetation from NDVI and LST parameters, Remote Sens. Environ., 113, 329–334, https://doi.org/10.1016/j.rse.2008.09.016, 2009.
Julien, Y. and Sobrino, J. A.: Correcting AVHRR Long Term Data Record V3 estimated LST from orbital drift effects, Remote Sens. Environ., 123, 207–219, https://doi.org/10.1016/j.rse.2012.03.016, 2012.
Julien, Y., Sobrino, J. A., and Verhoef, W.: Changes in land surface temperatures and NDVI values over Europe between 1982 and 1999, Remote Sens. Environ., 103, 43–55, https://doi.org/10.1016/j.rse.2006.03.011, 2006.
Karnieli, A., Agam, N., Pinker, R. T., Anderson, M., Imhoff, M. L., Gutman, G. G., Panov, N., and Goldberg, A.: Use of NDVI and land surface temperature for drought assessment: merits and limitations, J. Climate, 23, 618–633, https://doi.org/10.1175/2009JCLI2900.1, 2010.
Lambin, E. F. and Ehrlich, D.: Land-cover changes in sub-saharan Africa (1982–1991): Application of a change index based on remotely sensed surface temperature and vegetation indices at a continental scale, Remote Sens. Environ., 61, 181–200, https://doi.org/10.1016/S0034-4257(97)00001-1, 1997.
Latifovic, R., Pouliot, D., and Dillabaugh, C.: Identification and correction of systematic error in NOAA AVHRR long-term satellite data record, Remote Sens. Environ., 127, 84–97, https://doi.org/10.1016/j.rse.2012.08.032, 2012.
Li, H., Sun, D., Yu, Y., Wang, H., Liu, Y., Liu, Q., Du, Y., Wang, H., and Cao, B.: Evaluation of the VIIRS and MODIS LST products in an arid area of Northwest China, Remote Sens. Environ., 142, 111–121, https://doi.org/10.1016/j.rse.2013.11.014, 2014.
Liang, S., Li, X., and Wang, J.: Quantitative Remote Sensing: Concepts and Algorithms, Science Press, Beijing, China, 2013.
Liu, X., Tang, B.-H., Yan, G., Li, Z.-L., and Liang, S.: Retrieval of global orbit drift corrected land surface temperature from long-term AVHRR data, Remote Sens., 11, 2843, https://doi.org/10.3390/rs11232843, 2019.
Liu, Z., Zhan, W., Lai, J., Hong, F., Quan, J., Bechtel, B., Huang, F., and Zou, Z.: Balancing prediction accuracy and generalization ability: A hybrid framework for modelling the annual dynamics of satellite-derived land surface temperatures, ISPRS J. Photogramm., 151, 189–206, https://doi.org/10.1016/j.isprsjprs.2019.03.013, 2019.
Long, D., Yan, L., Bai, L., Zhang, C., Li, X., Lei, H., Yang, H., Tian, F., Zeng, C., Meng, X., and Shi, C.: Generation of MODIS-like land surface temperatures under all-weather conditions based on a data fusion approach, Remote Sens. Environ., 246, 111863, https://doi.org/10.1016/j.rse.2020.111863, 2020.
Ma, J., Zhou, J., Göttsche, F.-M., Liang, S., Wang, S., and Li, M.: A global long-term (1981–2000) land surface temperature product for NOAA AVHRR, Earth Syst. Sci. Data, 12, 3247–3268, https://doi.org/10.5194/essd-12-3247-2020, 2020.
Ma, J., Shen, H., Wu, P., Wu, J., Gao, M., and Meng, C.: Generating gapless land surface temperature with a high spatio-temporal resolution by fusing multi-source satellite-observed and model-simulated data, Remote Sens. Environ., 278, 113083, https://doi.org/10.1016/j.rse.2022.113083, 2022.
Mao, K. B., Ma, Y., Tan, X. L., Shen, X. Y., Liu, G., Li, Z. L., Chen, J. M., and Xia, L.: Global surface temperature change analysis based on MODIS data in recent twelve years, Adv. Space Res., 59, 503–512, https://doi.org/10.1016/j.asr.2016.11.007, 2017.
Martin, M. A., Ghent, D., Pires, A. C., Göttsche, F.-M., Cermak, J., and Remedios, J. J.: Comprehensive in situ validation of five satellite land surface temperature data sets over multiple stations and years, Remote Sens., 11, 479, https://doi.org/10.3390/rs11050479, 2019.
Mildrexler, D. J., Zhao, M., and Running, S. W.: A global comparison between station air temperatures and MODIS land surface temperatures reveals the cooling role of forests, J. Geophys. Res.-Biogeo., 116, G03025, https://doi.org/10.1029/2010JG001486, 2011.
Mildrexler, D. J., Zhao, M., Cohen, W. B., Running, S. W., Song, X. P., and Jones, M. O.: Thermal anomalies detect critical global land surface changes, J. Appl. Meteorol. Clim., 57, 391–411, https://doi.org/10.1175/JAMC-D-17-0093.1, 2017.
Muro, J., Strauch, A., Heinemann, S., Steinbach, S., Thonfeld, F., Waske, B., and Diekkrüger, B.: Land surface temperature trends as indicator of land use changes in wetlands, Int. J. Appl. Earth Obs., 70, 62–71, https://doi.org/10.1016/j.jag.2018.02.002, 2018.
Østby, T. I., Schuler, T. V., and Westermann, S.: Severe cloud contamination of MODIS Land Surface Temperatures over an Arctic ice cap, Svalbard, Remote Sens. Environ., 142, 95–102, https://doi.org/10.1016/j.rse.2013.11.005, 2014.
Pastorello, G., Trotta, C., Canfora, E., Chu, H., Christianson, D., Cheah, Y.-W., Poindexter, C., Chen, J., Elbashandy, A., Humphrey, M., Isaac, P., Polidori, D., Ribeca, A., van Ingen, C., Zhang, L., Amiro, B., Ammann, C., Arain, M. A., Ardö, J., Arkebauer, T., et al.: The FLUXNET2015 dataset and the ONEFlux processing pipeline for eddy covariance data, Sci. Data, 7, 225, https://doi.org/10.1038/s41597-020-0534-3, 2020.
Potter, C. and Coppernoll-Houston, D.: Controls on land surface temperature in deserts of southern california derived from MODIS satellite time series analysis, 2000 to 2018, Climate, 7, 32, https://doi.org/10.3390/cli7020032, 2019.
Quan, J., Zhan, W., Chen, Y., Wang, M., and Wang, J.: Time series decomposition of remotely sensed land surface temperature and investigation of trends and seasonal variations in surface urban heat islands, J. Geophys. Res.-Atmos., 121, 2638–2657, https://doi.org/10.1002/2015JD024354, 2016.
Quan, J., Zhan, W., Ma, T., Du, Y., Guo, Z., and Qin, B.: An integrated model for generating hourly Landsat-like land surface temperatures over heterogeneous landscapes, Remote Sens. Environ., 206, 403–423, https://doi.org/10.1016/j.rse.2017.12.003, 2018.
Sen, P. K.: Estimates of the regression coefficient based on Kendall's tau, J. Am. Stat. Assoc., 63, 1379–1389, https://doi.org/10.1080/01621459.1968.10480934, 1968.
Sharifnezhadazizi, Z., Norouzi, H., Prakash, S., Beale, C., and Khanbilvardi, R.: A Global Analysis of Land Surface Temperature Diurnal Cycle Using MODIS Observations, J. Appl. Meteorol. Clim., 58, 1279–1291, https://doi.org/10.1175/JAMC-D-18-0256.1, 2019.
Simmons, A. J., Berrisford, P., Dee, D. P., Hersbach, H., Hirahara, S., and Thépaut, J. N.: A reassessment of temperature variations and trends from global reanalyses and monthly surface climatological datasets, Q. J. Roy. Meteor. Soc., 143, 101–119, https://doi.org/10.1002/qj.2949, 2017.
Sobrino, J. A., García-Monteiro, S., and Julien, Y.: Surface temperature of the planet Earth from satellite data over the period 2003–2019, Remote Sens., 12, 2036, https://doi.org/10.3390/rs12122036, 2020a.
Sobrino, J. A., Julien, Y., and García-Monteiro, S.: Surface temperature of the planet Earth from satellite data, Remote Sens., 12, 218, https://doi.org/10.3390/rs12020218, 2020b.
Still, C., Powell, R., Aubrecht, D., Kim, Y., Helliker, B., Roberts, D., Richardson, A. D., and Goulden, M.: Thermal imaging in plant and ecosystem ecology: applications and challenges, Ecosphere, 10, e02768, https://doi.org/10.1002/ecs2.2768, 2019.
Theil, H.: A rank-invariant method of linear and polynominal regression analysis (Parts 1-3), Ned. Akad. Wetensch. Proc. Ser. A, 53, 1397–1412, 1950.
Tomlinson, C. J., Chapman, L., Thornes, J. E., and Baker, C.: Remote sensing land surface temperature for meteorology and climatology: a review, Meteorol. Appl., 18, 296–306, https://doi.org/10.1002/met.287, 2011.
Trigo, I. F., Boussetta, S., Viterbo, P., Balsamo, G., Beljaars, A., and Sandu, I.: Comparison of model land skin temperature with remotely sensed estimates and assessment of surface-atmosphere coupling, J. Geophys. Res.-Atmos., 120, 12096–12111, https://doi.org/10.1002/2015JD023812, 2015.
Wan, Z.: New refinements and validation of the collection-6 MODIS land-surface temperature/emissivity product, Remote Sens. Environ., 140, 36–45, https://doi.org/10.1016/j.rse.2013.08.027, 2014.
Westermann, S., Langer, M., and Boike, J.: Spatial and temporal variations of summer surface temperatures of high-arctic tundra on Svalbard – Implications for MODIS LST based permafrost monitoring, Remote Sens. Environ., 115, 908–922, https://doi.org/10.1016/j.rse.2010.11.018, 2011.
Westermann, S., Langer, M., and Boike, J.: Systematic bias of average winter-time land surface temperatures inferred from MODIS at a site on Svalbard, Norway, Remote Sens. Environ., 118, 162–167, https://doi.org/10.1016/j.rse.2011.10.025, 2012.
Wu, P., Yin, Z., Zeng, C., Duan, S.-B., Gottsche, F.-M., Ma, X., Li, X., Yang, H., and Shen, H.: Spatially continuous and high-resolution land surface temperature product generation: A review of reconstruction and spatiotemporal fusion techniques, IEEE Geoscience and Remote Sensing Magazine, 9, 112–137, https://doi.org/10.1109/mgrs.2021.3050782, 2021.
Xing, Z., Yu, Y., Duan, S., Li, Z., Gao, M., Leng, P., Zhang, X., and Shang, G.: Modeling year-to-year variations of clear-sky land surface temperature using Aqua/MODIS data, IEEE Access, 8, 114541–114553, https://doi.org/10.1109/ACCESS.2020.3003990, 2020.
Xing, Z., Li, Z.-L., Duan, S.-B., Liu, X., Zheng, X., Leng, P., Gao, M., Zhang, X., and Shang, G.: Estimation of daily mean land surface temperature at global scale using pairs of daytime and nighttime MODIS instantaneous observations, ISPRS J. Photogramm., 178, 51–67, https://doi.org/10.1016/j.isprsjprs.2021.05.017, 2021.
Yan, Y., Mao, K., Shi, J., Piao, S., Shen, X., Dozier, J., Liu, Y., Ren, H.-l., and Bao, Q.: Driving forces of land surface temperature anomalous changes in North America in 2002–2018, Sci. Rep.-UK, 10, 6931, https://doi.org/10.1038/s41598-020-63701-5, 2020.
Zhan, W., Huang, F., Quan, J., Zhu, X., Gao, L., Zhou, J., and Ju, W.: Disaggregation of remotely sensed land surface temperature: A new dynamic methodology, J. Geophys. Res.-Atmos., 121, 10538–10554, https://doi.org/10.1002/2016JD024891, 2016.
Zhang, T., Zhou, Y., Zhu, Z., Li, X., and Asrar, G. R.: A global seamless 1 km resolution daily land surface temperature dataset (2003–2020), Earth Syst. Sci. Data, 14, 651–664, https://doi.org/10.5194/essd-14-651-2022, 2022.
Zhang, X., Zhou, J., Liang, S., Chai, L., Wang, D., and Liu, J.: Estimation of 1-km all-weather remotely sensed land surface temperature based on reconstructed spatial-seamless satellite passive microwave brightness temperature and thermal infrared data, ISPRS J. Photogramm., 167, 321–344, https://doi.org/10.1016/j.isprsjprs.2020.07.014, 2020.
Zhao, B., Mao, K., Cai, Y., Shi, J., Li, Z., Qin, Z., Meng, X., Shen, X., and Guo, Z.: A combined Terra and Aqua MODIS land surface temperature and meteorological station data product for China from 2003 to 2017, Earth Syst. Sci. Data, 12, 2555–2577, https://doi.org/10.5194/essd-12-2555-2020, 2020.
Zhao, W., He, J., Yin, G., Wen, F., and Wu, H.: Spatiotemporal variability in land surface temperature over the mountainous region affected by the 2008 Wenchuan earthquake from 2000 to 2017, J. Geophys. Res.-Atmos., 124, 1975–1991, https://doi.org/10.1029/2018jd030007, 2019.
Zhao, W., Yang, M., Chang, R., Zhan, Q., and Li, Z.-L.: Surface warming trend analysis based on MODIS/Terra land surface temperature product at Gongga Mountain in the southeastern Tibetan Plateau, J. Geophys. Res.-Atmos., 126, e2020JD034205, https://doi.org/10.1029/2020JD034205, 2021.
Zhou, C. and Wang, K.: Spatiotemporal divergence of the warming hiatus over land based on different definitions of mean temperature, Sci. Rep.-UK, 6, 31789, https://doi.org/10.1038/srep31789, 2016.
Zhengming, W. and Zhao-Liang, L.: A physics-based algorithm for retrieving land-surface emissivity and temperature from EOS/MODIS data, IEEE T. Geosci. Remote, 35, 980–996, https://doi.org/10.1109/36.602541, 1997.
Zou, Z., Zhan, W., Liu, Z., Bechtel, B., Gao, L., Hong, F., Huang, F., and Lai, J.: Enhanced modeling of annual temperature cycles with temporally discrete remotely sensed thermal observations, Remote Sens., 10, 650, https://doi.org/10.3390/rs10040650, 2018.
- Abstract
- Introduction
- Datasets
- Methodology
- Results
- Discussion
- Data availability
- Conclusions
- Appendix A: Statistics on the original MODIS MXDC1 V6 products
- Appendix B: Mean absolute errors of Tdm_IADTC and Tdm_OADTC in Scenarios no. 1 and no. 3 at the site level
- Appendix C: Distribution of average sampling bias per season
- Appendix D: Nomenclature
- Author contributions
- Competing interests
- Disclaimer
- Acknowledgements
- Financial support
- Review statement
- References
- Abstract
- Introduction
- Datasets
- Methodology
- Results
- Discussion
- Data availability
- Conclusions
- Appendix A: Statistics on the original MODIS MXDC1 V6 products
- Appendix B: Mean absolute errors of Tdm_IADTC and Tdm_OADTC in Scenarios no. 1 and no. 3 at the site level
- Appendix C: Distribution of average sampling bias per season
- Appendix D: Nomenclature
- Author contributions
- Competing interests
- Disclaimer
- Acknowledgements
- Financial support
- Review statement
- References