the Creative Commons Attribution 4.0 License.
the Creative Commons Attribution 4.0 License.
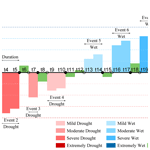
A multi-scale daily SPEI dataset for drought characterization at observation stations over mainland China from 1961 to 2018
Jingyu Zeng
Junyu Qi
Xuesong Zhang
Yue Zeng
Wei Shui
Zhanghua Xu
Rongrong Zhang
Xiaoping Wu
Jiang Cong
The monthly standardized precipitation evapotranspiration index (SPEI) can
be used to monitor and assess drought characteristics with 1-month or
longer drought duration. Based on data from 1961 to 2018 at 427
meteorological stations across mainland China, we developed a daily SPEI
dataset to overcome the shortcoming of the coarse temporal scale of monthly
SPEI. Our dataset not only can be used to identify the start and end dates
of drought events, but also can be used to investigate the meteorological,
agricultural, hydrological, and socioeconomic droughts with a different timescales. In the present study, the SPEI data with 3-month (about 90 d)
timescale were taken as a demonstration example to analyze spatial distribution
and temporal changes in drought conditions for mainland China. The SPEI
data with a 3-month (about 90 d) timescale showed no obvious intensifying
trends in terms of severity, duration, and frequency of drought events from
1961 to 2018. Our drought dataset serves as a unique resource with daily
resolution to a variety of research communities including meteorology,
geography, and natural hazard studies. The daily SPEI dataset developed is
free, open, and publicly available from this study. The dataset
with daily SPEI is publicly available via the figshare portal (Wang et al.,
2020c), with https://doi.org/10.6084/m9.figshare.12568280.
Highlights. A multi-scale daily SPEI dataset was developed across mainland China
from 1961 to 2018.
The daily SPEI dataset can be used to identify the start and end days of the
drought event.
The developed daily SPEI dataset in this study is free, open, and
publicly available.
- Article
(2502 KB) - Full-text XML
- BibTeX
- EndNote
Drought is one of the most destructive natural hazards worldwide. It can lead to adverse effects to the ecological system, industrial production, agricultural practices, drinking water availability, hydrological processes, and water quality (Bussi and Whitehead, 2020; Lai et al., 2019; Vicente-Serrano et al., 2012; Wang et al., 2014, 2017). Drought caused ca. USD 221 billion in loss from 1960 to 2016 reported by the International Disaster Database (EM-DAT), and the drought events in South Asia influenced over 60 million residents from 1998 to 2001 (Agrawala et al., 2001). Unfortunately, drought is expected to increase in frequency and intensity due to the future warming air temperature (Trenberth et al., 2014; Zambrano et al., 2018). The exacerbated drought conditions have promoted some national legislation (such as drought preparedness and planning) to carry out risk management and adaptive strategy for drought disasters (Garrick et al., 2017).
The various drought types result in the difficulty of drought characterization and assessment. Drought definition is not unique. Some studies proposed defining drought according to the water deficit (Wilhite and Glantz, 1985), while others defined drought based on the period of abnormal arid conditions (Eslamian et al., 2017). The most common drought can be classified into four types including (1) meteorological, (2) agricultural, (3) hydrological, and (4) socioeconomic (Mishra and Singh, 2010). Meteorological drought results from precipitation deficit or evaporation increases (McKee et al., 1993). Meteorological drought can propagate into agricultural drought with lower soil moisture availability, and it also can lead to hydrological drought with lower streamflow and socioeconomic drought with lower water availability (Barella-Ortiz and Quintana-Seguí, 2019; Gevaert et al., 2018). In general, drought indices are normally used to monitor and assess the condition or spatial–temporal characteristics of drought.
Many drought indices have been developed for drought characterization and assessment, such as the Palmer drought severity index (PDSI) (Dai et al., 2004), standardized precipitation index (SPI) (McKee et al., 1993), vegetation water supply index (VWSI) (Carlson et al., 1994), vegetation health index (VHI) (Kogan, 2002), vegetation temperature condition index (VTCI) (Wan et al., 2004), and other drought indices (Men-xin and Hou-quan, 2016; Wang et al., 2015, 2017). PDSI and SPI are the most popular drought studies worldwide (Dai et al., 2004; McKee et al., 1993); however, they have some limitations. PDSI is only suitable to the agricultural drought through characterization of the soil water deficit, and it cannot identify the meteorological, hydrological, and socioeconomic droughts (Feng and Su, 2019). In addition, PDSI limits the spatial comparability of drought due to the fact that it is heavily dependent on data calibration (Sheffield et al., 2009; Yu et al., 2014). Although the SPI can be used to monitor and assess different drought types on multiple spatial scales at a monthly time step, it only considers the precipitation factor and neglects effects of evaporation stemming from temperature and other meteorological factors (Wang et al., 2014, 2017; Yang et al., 2018). To solve the above problems, the standardized precipitation evapotranspiration index (SPEI), which considers the advantages of both PDSI and SPI, was developed to monitor and assess droughts (Vicente-Serrano et al., 2010). It not only accounts for the effect of evaporation on drought, but also has the capability of spatial comparability and characterization of different drought types with multiple timescales (Feng and Su, 2019; Wang et al., 2015). SPEI can be used to delineate spatial–temporal evolution of drought, drought characteristics, and impacts of drought at the regional and global scales (Mallya et al., 2016; Wang et al., 2014).
However, the commonly used SPEI fails to identify droughts with a duration less than 1 month (Van der Schrier et al., 2011; Vicente-Serrano et al., 2010). With future climate change, flash droughts have been recently categorized as a type of extreme climate event. Flash droughts occur along with sudden onset, rapid aggravation, and sudden end of drought and could lead to severe consequences (Pendergrass et al., 2020). It is imperative to characterize flash droughts with short-term duration (e.g., several days). To use the sub-month-resolution drought index, we have developed the daily SPEI for the first time, and our daily SPEI has been used to assess the drought and its impacts in previous studies (Wang et al., 2015, 2017). The new SPEI can not only identify drought with a duration of 1 month and more than 1 month, but also monitor drought with a duration of several days. In addition, our new daily SPEI has filled the gap in the capability to monitor the onset and duration of droughts. Our daily SPEI has similar principles as the commonly used monthly SPEI in terms of time accumulation effects (Vicente-Serrano et al., 2010; Wang et al., 2015; Yu et al., 2014). The daily SPEI data with different timescales can also meet the requirements of characterizing and assessing different drought types (meteorological drought, agricultural drought, and hydrological drought) at multiple timescales (Wang et al., 2014).
The SPEI can be calculated by the difference between daily precipitation and daily potential evapotranspiration (PET) (Vicente-Serrano et al., 2012). Precipitation can generally be directly obtained by the meteorological observation stations (Wang et al., 2015). But PET can only be estimated by drivers of meteorological data or remote sensing data (Wang et al., 2018, 2017). Although there are at least 50 methods to calculate the PET, the methods estimate the inconsistent and different values due to diverse assumptions, data inputs, and climatic regions (Grismer et al., 2002; Lu et al., 2005). PET plays an important role in understanding fluxes of the heat and mass of the atmospheric system at local and global scales (Thomas, 2000). Thus, it is necessary to choose a suitable method to estimate PET. The choice of candidate probability distributions for SPEI calculation is also very important (Vicente-Serrano et al., 2010, 2012). The chosen distribution for SPEI generally needs a location parameter because climatic water balance may have negative values (when PET is greater than precipitation in certain periods) (Wang et al., 2015, 2017). Distributions for SPEI normalization have a generalized logistic distribution, Pearson type III distribution, normal distribution, and generalized extreme value (GEV) distribution (Stagge et al., 2015). The four candidate SPEI distributions have the best goodness of fit for the accumulated climatic water balance (Stagge et al., 2015; Wang et al., 2015; Wang et al., 2017). However, the GEV distribution has the best performance among all four probability distributions across all of continental Europe, because of the lower rejection frequencies of GEV using several tests (Kolmogorov–Smirnov (K–S), Anderson–Darling (A–D), and Shapiro–Wilk (S–W)) (Stagge et al., 2015). Therefore, we choose the GEV distribution fitting the accumulated climatic water balance to calculate SPEI. The SPEI is suited to investigate the effects of climate change and global warming on drought severity. SPEI has been widely used in diverse studies on drought variability and impact and drought monitoring systems (Boroneant et al., 2011; Fuchs et al., 2012; Potop et al., 2014; Sohn et al., 2013).
The aim of this study, therefore, is to produce a long (1961–2018) daily drought index dataset for all of mainland China. Specifically, we used the new daily SPEI algorithm to produce the multi-timescale drought dataset at a daily time resolution. Meteorological data with 427 stations including multiple factors (daily precipitation, daily average air temperature, daily minimum air temperature, daily maximum air temperature, and sunshine) are used. The developed drought dataset at the national scale has the potential to be used to monitor and assess droughts and their impacts for the sectors including the agricultural sector, forest sector, hydrological sector, ecological sector, environmental sector, and so on.
2.1 Data sources
Daily meteorological data from 1960 to 2018 were collected from the National Meteorological Science Data Sharing Service Platform (http://data.cma.cn/, last access: 15 July 2020). The data, which have gone through quality controlling, have been used in many studies on drought (Li et al., 2019; Wang et al., 2019). In total, there are 839 stations with public data. To ensure continuous and complete data records, 427 meteorological stations are chosen for our study by removing stations with missing data exceeding 30 d over the whole period. Meteorological variables include the minimum and maximum air temperature (∘C), precipitation (mm), and sunshine duration (h). The sunshine duration was converted to solar radiation based on the Ångström function (Chen et al., 2010; Wang et al., 2015). The station location is shown in Fig. 1.
2.2 Daily SPEI calculation
The daily SPEI can be calculated by the difference between daily precipitation and daily potential evapotranspiration. Because air temperature and solar radiation explained at least 80 % of evapotranspiration variability (Martí et al., 2015; Priestley and Taylor, 1972), the Hargreaves model based on temperature and solar radiation can be used to estimate the daily potential evapotranspiration (Hargreaves and Samani, 1982; Mendicino and Senatore, 2013; Wang et al., 2015). The daily potential evapotranspiration can be obtained by the following formula:
where Tmean is the daily average air temperature (∘C); Tmax and Tmin are the daily maximum and minimum air temperatures (∘C), respectively; and Ra is the daily net radiation on the land surface (MJ m−2 d−1).
SPEI calculation depends on the accumulating deficit or surplus (Di) of water balance at different timescales. Di can be determined based on precipitation (P) and PET formula given day i:
The obtained Di values are summed at different timescales, following the same procedure as that for the commonly used SPEI. The on a given day j and year i depends on the chosen timescale k (days). For example, the accumulated difference for 1 d in a particular year i with a 30 d timescale (or other timescales) is calculated using
We also need to normalize the water balance into a probability distribution to get the SPEI series. The best distribution for SPEI calculation is the generalized extreme value (GEV) distribution (Stagge et al., 2015), which can overcome the limitation of the original SPEI through generalized logistic distribution for short accumulation (1–2-month) periods (Stagge et al., 2015; Vicente-Serrano et al., 2010). Therefore, we adopted the GEV distribution to standardize the D series into SPEI data series (Monish and Rehana, 2020). The GEV probability density function is
where
where ξ, σ, and μ are the shape, scale, and location parameters, respectively. The cumulative distribution function F(x) of GEV can be calculated by the following equation:
where
Thus, the probability distribution function of the D series is given by
With F(x), the SPEI can easily be obtained as the standardized values of F(x). Following the classical approximation of Abramowitz and Stegun (1965),
where for P≤0.5 and P is the probability of exceeding a determined D value, . If P>0.5, then P is replaced by 1−P and the sign of the resultant SPEI is reversed. The constants are C0=2.515517, C1=0.802853, C2=0.010328, d1=1.432788, d2=0.189269, and d3=0.001308.
2.3 Drought analysis method
The daily SPEI dataset was calculated in five accumulating periods (30, 90, 180, 360, 720 d) based on the water balance (difference between precipitation and PET). The classifications for the SPEI drought classes are presented in Table 1.
We used the method described by Yevjevich (1967) to define the drought characteristics (severity, duration, and intensity). A drought event can first be determined by drought start and end dates, and its duration and severity were then assigned. Thus, we accounted for the continuity of drought propagation. The continuous days with SPEI values less than the threshold (such as −0.5, −1.0, −1.5, −2) are defined as the duration of a drought event. The severity is the integral area between absolute value of the SPEI with value and the horizontal axis (SPEI =0) from the drought start day to the drought end day. The drought frequency is the total number of drought events in a period. The drought event and its characteristics (severity, duration, and intensity) can be seen in Fig. 2.
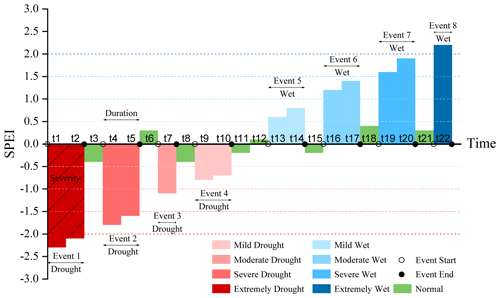
Figure 2Schematic diagram of drought and wet events (the red shaded area denotes the drought events; the blue shaded area denotes the wet events).
The SPEI data based on 90 d (3-month) timescales can be used to identify soil moisture or agriculture droughts (Wang et al., 2014). Due to its important applications, we selected the SPEI data with the 90 d timescales as the example data for analysis in the present study. To investigate the spatial–temporal characteristics of the example data, we defined three variables including annual total drought severity (ATDS), annual total drought duration (ATDD), and annual total drought frequency (ATDF). The three variables were obtained by summing the severity, duration, and frequency of all the drought events in each year at 427 stations.
We also used the non-parametric Mann–Kendall (MK) test to detect monotonic trends (Kendall, 1948; Mann, 1945), because the MK test does not require data normality (Mann, 1945; Wang et al., 2020a, b). We computed slopes for ATDS, ATDD, and ATDF using the Sen method (Sen, 1968). These statistical methods are commonly used in analyses of water resources, climate, and ecology data. For the MK test, the global trend for the entire series is significant when P value <0.05.
3.1 Spatial distribution of drought characteristics
The ATDS can be used to identify hot spots with more severe drought conditions. Figure 3 shows the calculated ATDS values across mainland China. We categorized ATDS values into two main groups, with higher ATDS values indicating more severe drought conditions. The distribution of ATDS values shows that, in general, the northeastern parts of China had more severe drought conditions than the southern parts. However, our results also indicate that the humid climate zone in the south also experienced severe drought conditions, though not as much as for northern parts of China (Fig. 3).
Figure 4 shows that ATDD values ranged from 100 to 110 d for most stations across mainland China. This indicates that there was nearly one-third of a year when most stations were experiencing drought conditions. More stations with ATDD values ranging from 100 to 110 were found compared with stations with ATDD values of 120–130 (Fig. 4). For drought years, the duration days of drought events are expected to be longer. The ATDD had similar spatial distribution characteristics to those of the ATDS, indicating that droughts also occurred in the humid climate zone.
Figure 5 shows the spatial distribution of ATDF values across mainland China. In general, most stations had four to six annual drought events. There were fewer stations with six to eight annual drought events compared with stations with two to four annual drought events. We also detected that drought events could occur in both arid and humid regions based on spatial distributions of ATDF values (Fig. 5). Since the ATDF indicated only the annual average drought events, we could expect that for the more severe drought years the ATDF would have greater values for different stations.
3.2 Trends in drought characteristics
The changing trends of ATDS can be used to detect whether drought severity is weakening or intensifying with time. Figure 6 shows the spatial distribution of changing trends of ATDS from 1961 to 2018 across mainland China. In general, there were more stations with weakening trends in drought severity than those with intensifying trends across all stations (Fig. 6). It seems that both weakening and intensifying absolute values were largest in the northeast, northwest, and central China compared with other parts. However, after scrutiny, we found that drought severity tended to weaken in the northeast, northwest, and central China, with more stations having significant weakening trends according to statistical tests (P value <0.05; Fig. 6). For southern China, most stations had no significant trends in either weakening or intensifying of drought severity (P value >0.05; Fig. 6).
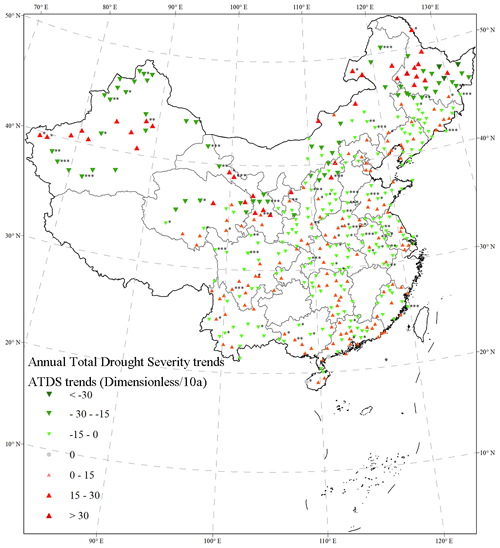
Figure 6The spatial distribution of the changing trends of ATDS (the red and green triangles indicate increasing and decreasing trends, respectively. “***” denotes P value <0.001, “**” denotes P value <0.01, and “*” denotes P value <0.05).
The changing trends of ATDD can be used to detect whether drought duration is getting shorter or longer. Figure 7 shows the spatial distribution of changing trends for the ATDD across all stations. In general, stations in the southeast demonstrated downward trends with shortening drought duration, while stations in the northwest had upward trends for the ATDD with increasing drought duration (Fig. 7). Note that the increasing or decreasing trends for ATDD were significant (P value <0.05) for stations across central China, indicating that the central China regions were suffering dramatic changes of drought conditions.
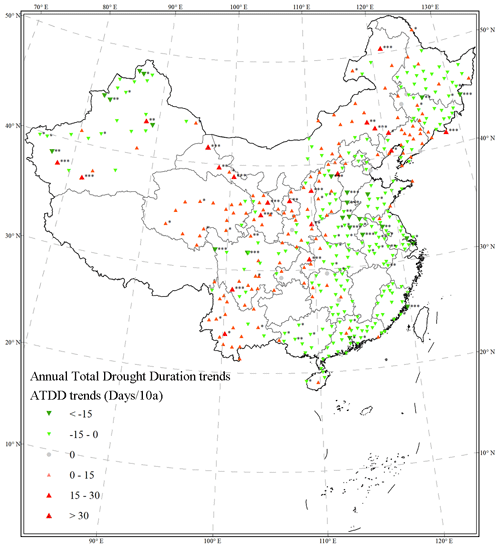
Figure 7The spatial distribution of the changing trends of ATDD (the red and green triangles indicate increasing and decreasing trends, respectively. “***” denotes P value <0.001, “**” denotes P value <0.01, and “*” denotes P value <0.05).
The changing trends of ATDF can be used to detect whether the frequency of drought events is increasing or decreasing with time. Figure 8 shows the spatial distribution of changing trends of ATDF across all stations. Most stations demonstrated no significant trend in the frequency of drought events, except for dozens of stations in western China having significant upward trends (P value <0.05) with increasing frequency in drought events, and stations in northeastern China demonstrated significant downward trends (P value <0.05) with decreasing frequency of drought events.
The reason for selecting a 90 d (3-month) timescale to assess spatial and temporal characteristics of drought conditions across mainland China is because the SPEI with the 90 d (3-month) timescale can indicate the agricultural drought (or soil moisture) (Van der Schrier et al., 2011; Wang et al., 2014, 2017), and its results are comparable with the PDSI (Dai et al., 2004; Van der Schrier et al., 2011) and other drought indices including the surface water supply index (SWSI) and moisture adequacy index (MAI) (Doesken et al., 1991; McGUIRE and Palmer, 1957). The commonly used monthly SPEI has been used to assess drought characteristics and their impacts worldwide from the regional scale to the global scale (Stagge et al., 2015; Vicente-Serrano et al., 2010; Wang et al., 2014). The SPEI with different timescales is relevant for meteorological drought (1-month timescale), agricultural drought (3–6-month timescale, about 90–180 d), hydrological drought (12-month timescale, about 360 d), and socioeconomic drought (24-month timescale, about 720 d), respectively (Homdee et al., 2016; Potop et al., 2014; Tirivarombo et al., 2018; Vicente-Serrano et al., 2010).
Our new SPEI dataset with multiple timescales was developed and compiled using the daily SPEI algorithm in the previous study (Wang et al., 2015). The daily SPEI has been used in drought characterization and assessment and was validated by drought characterization and assessment (Jevšenak, 2019; Jia et al., 2018; Salvador et al., 2019; Wang et al., 2015, 2017). The global SPEI database with monthly temporal resolution and 0.5∘ spatial resolution is available (https://spei.csic.es/database.html, last access: 25 July 2017). The database covers the period between January 1901 and December 2018. Although the database can be used effectively for meteorological, agricultural, hydrological, and socioeconomic droughts, it cannot identify and detect flash drought with less than 1-month duration. In addition, the monthly database can only detect the start month and end month of drought events, and therefore it fails to determine the start and end dates of a drought event (Kassaye et al., 2020; Vicente-Serrano et al., 2010; Wang et al., 2014). Our newly developed daily SPEI can compensate for the shortcomings of monthly SPEI in drought characterization and assessment. In addition, we used the well-received GEV probability distribution for the SPEI calculation for our dataset (Stagge et al., 2015).
Although the daily SPEI has better performance in drought characterization and assessment (Jevšenak, 2019; Wang et al., 2017), the uncertainty of daily SPEI still needs to be evaluated in future works. Our daily SPEI dataset used the simple Hargreaves model based on temperature and solar radiation to estimate daily potential evapotranspiration (Hargreaves and Samani, 1982; Wang et al., 2017). We will further investigate effects of various evapotranspiration models (such as the CRAE model, Penman algorithm, Thornthwaite algorithm, Makkink algorithm, and Priestley–Taylor algorithm) on the calculation of SPEI (Makkink, 1957; Morton, 1983; Penman, 1948; Priestley and Taylor, 1972; Thornthwaite, 1944). We only chose SPEI based on the 90 d (3-month) timescale as an example to analyze drought characteristics, and the results demonstrated that there were no obvious intensifying trends for drought across mainland China, which is consistent with other studies (Han et al., 2020). Meanwhile, our newly developed daily SPEI will be further validated in other regions of the world. In addition, SPEI values at different timescales can be used as a proxy for other types of droughts, but it lacks the complete picture (no soil moisture condition, streamflow, etc.) (Zargar et al., 2011).
Our long-term daily SPEI dataset has contributed significantly to our understanding of drought evolution, especially flash drought. The dataset can be used to monitor and assess different drought types (meteorological drought, agricultural drought, and hydrological drought) through different timescale data. It can also identify the start and end dates for drought. The dataset is valuable to meteorological research and natural hazard communities for various purposes such as assessment of extreme climate or drought effect evaluation.
All daily SPEI datasets including data and their description at 427 observed meteorological stations are provided open access via figshare (Wang et al., 2020c), available at https://doi.org/10.6084/m9.figshare.12568280. This depository includes the five files of the daily SPEI data with five scales (30 d about 1 month, 90 d about 3 months, 180 d about 6 months, 360 d about 12 months, 720 d about 24 months) and station information for 427 meteorological stations.
In the present study, we have produced a daily SPEI dataset from 1960 to 2018 at 427 meteorological stations across mainland China. Our open-access dataset is an important contribution to drought assessment, and it can overcome the disadvantages of the commonly used monthly SPEI database. Our daily dataset can help monitor and assess the spatial and temporal characteristics of droughts. It can be used to assess the impacts of droughts on ecological systems, hydrological processes, and other natural resources. Our daily multi-timescale SPEI dataset can be widely used in studies on meteorological drought (1-month timescale), agricultural drought (3–6-month timescale), hydrological drought (12-month timescale), and socioeconomic drought (24-month timescale). The dataset will reduce the time spent on research and avoid the duplication of efforts, which will be highly attractive to meteorological, geographical, and natural hazard researchers and researchers from other areas.
QW led the study, developed the method, and wrote the manuscript with input from all the authors. JQ and XZ discussed the results and revised the manuscript. All the authors contributed to the final paper. QW, JY, RZ, XW, and XZ collected and analyzed data over time, providing statistics and material (figures and tables) for the paper.
The authors declare that they have no conflict of interest.
This research received financial support from the National Natural Science Foundation of China (41601562), the Strategic Priority Research Program of the Chinese Academy of Sciences (XDA13020506), and the China Scholarship Council (201806655014). The authors sincerely thank James Howard Stagge for his help on the codes and calculation of SPEI. Special thanks go to the meteorological data provider from the China Meteorological Administration (http://cdc.cma.gov.cn/, last access: 15 July 2020).
This research received financial support from the National Natural Science Foundation of China (grant no. 41601562), the Strategic Priority Research Program of the Chinese Academy of Sciences (grant no. XDA13020506), and the China Scholarship Council (grant no. 201806655014).
This paper was edited by Ge Peng and reviewed by five anonymous referees.
Abramowitz, M. and Stegun, I.: Handbook of Mathematical Functions, with Formulas, Graphs, and Mathematical Tables, New York, NY, Dover, 1046 pp., 1965.
Agrawala, S., Barlow, M., Cullen, H., and Lyon, B.: The drought and humanitarian crisis in Central and Southwest Asia: a climate perspective, IRI special report N. 01-11, International Research Institute for Climate Prediction, Palisades, 24, https://doi.org/10.7916/D8NZ8FHQ, 2001.
Barella-Ortiz, A. and Quintana-Seguí, P.: Evaluation of drought representation and propagation in regional climate model simulations across Spain, Hydrol. Earth Syst. Sci., 23, 5111–5131, https://doi.org/10.5194/hess-23-5111-2019, 2019.
Boroneant, C., Ionita, M., Brunet, M., and Rimbu, N.: CLIVAR-SPAIN contributions: seasonal drought variability over the Iberian Peninsula and its relationship to global sea surface temperature and large scale atmospheric circulation, WCRP OSC: Climate Research in Service to Society, Denver, USA, available at: https://www.wcrp-climate.org/conference2011/posters/C4/C4_Boroneant_TH197A_0.pdf (last access: 9 February 2021), 24–28 October 2011.
Bussi, G. and Whitehead, P. G.: Impacts of droughts on low flows and water quality near power stations, Hydrol. Sci. J., 65, 898–913, 2020.
Carlson, T. N., Gillies, R. R., and Perry, E. M.: A method to make use of thermal infrared temperature and NDVI measurements to infer surface soil water content and fractional vegetation cover, Remote Sens. Rev., 9, 161–173, 1994.
Chen, C., Wang, E., and Yu, Q.: Modelling the effects of climate variability and water management on crop water productivity and water balance in the North China Plain, Agr. Water Manage., 97, 1175–1184, 2010.
Dai, A., Trenberth, K. E., and Qian, T.: A global dataset of Palmer Drought Severity Index for 1870–2002: Relationship with soil moisture and effects of surface warming, J. Hydrometeorol., 5, 1117–1130, 2004.
Doesken, N. J., McKee, T. B., and Garen, D. : Drought monitoring in the western United States using a Surface Water Supply Index, 7th Conf. AppI. Climatology, Proc., American Meteorological Society, Boston, Mass., 10–13, 1991.
Eslamian, S., Ostad-Ali-Askari, K., Singh, V. P., Dalezios, N. R., Ghane, M., Yihdego, Y., and Matouq, M.: A review of drought indices, Int. J. Constr. Res. Civ. Eng., 3, 48–66, 2017.
Feng, K. and Su, X.: Spatiotemporal Characteristics of Drought in the Heihe River Basin Based on the Extreme-Point Symmetric Mode Decomposition Method, Int. J. Dis. Risk Sci., 10, 591–603, 2019.
Fuchs, B., Svoboda, M., Nothwehr, J., Poulsen, C., Sorensen, W., and Guttman, N.: A new national drought risk Atlas for the US from the National Drought Mitigation Center, National Drought Mitigation Center, Univ. of Nebraska, Lincoln, NE, USA, 2012.
Garrick, D. E., Hall, J. W., Dobson, A., Damania, R., Grafton, R. Q., Hope, R., Hepburn, C., Bark, R., Boltz, F., and De Stefano, L.: Valuing water for sustainable development, Science, 358, 1003–1005, 2017.
Gevaert, A. I., Veldkamp, T. I. E., and Ward, P. J.: The effect of climate type on timescales of drought propagation in an ensemble of global hydrological models, Hydrol. Earth Syst. Sci., 22, 4649–4665, https://doi.org/10.5194/hess-22-4649-2018, 2018.
Grismer, M., Orang, M., Snyder, R., and Matyac, R.: Pan evaporation to reference evapotranspiration conversion methods, J. Irrig. Drain. E., 128, 180–184, 2002.
Han, X., Wu, J., Zhou, H., Liu, L., Yang, J., Shen, Q., and Wu, J.: Intensification of historical drought over China based on a multi-model drought index, Int. J. Climatol., 40, 5407–5419, https://doi.org/10.1002/joc.6527, 2020.
Hargreaves, G. H. and Samani, Z. A.: Estimating potential evapotranspiration, J. Irrig. Drain. E., 108, 225–230, 1982.
Homdee, T., Pongput, K., and Kanae, S.: A comparative performance analysis of three standardized climatic drought indices in the Chi River basin, Thailand, Agr. Nat. Resour., 50, 211–219, 2016.
Jevšenak, J.: Daily climate data reveal stronger climate-growth relationships for an extended European tree-ring network, Quaternary Sci. Rev., 221, 105868, https://doi.org/10.1016/j.quascirev.2019.105868, 2019.
Jia, Y., Zhang, B., and Ma, B.: Daily SPEI reveals long-term change in drought characteristics in Southwest China, Ch. Geogr. Sci., 28, 680–693, 2018.
Kassaye, A. Y., Shao, G., Wang, X., and Wu, S.: Quantification of drought severity change in Ethiopia during 1952–2017, Environ. Dev. Sustain., 1–26, https://doi.org/10.1007/s10668-020-00805-y, 2020.
Kendall, M. G.: Rank correlation methods, Charles Griffin, London, p. 202, 1948.
Kogan, F.: World droughts in the new millennium from AVHRR-based vegetation health indices, T. AGU, 83, 557–563, 2002.
Lai, C., Zhong, R., Wang, Z., Wu, X., Chen, X., Wang, P., and Lian, Y.: Monitoring hydrological drought using long-term satellite-based precipitation data, Sci. Total Environ., 649, 1198–1208, 2019.
Li, Y., Yuan, X., Zhang, H., Wang, R., Wang, C., Meng, X., Zhang, Z., Wang, S., Yang, Y., and Han, B.: Mechanisms and early warning of drought disasters: Experimental drought meteorology research over China, B. Am. Meteorol. Soc., 100, 673–687, 2019.
Lu, J., Sun, G., McNulty, S. G., and Amatya, D. M.: A Comparison of Six Potential Evapotranspiration Methods for Regional Use in the Southeastern United States 1, J. Am. Water Resour. As., 41, 621–633, 2005.
Makkink, G. F.: Testing the Penman formula by means of lysimeters, J. Inst. Water Eng., 11, 277–288, 1957.
Mallya, G., Mishra, V., Niyogi, D., Tripathi, S., and Govindaraju, R. S.: Trends and variability of droughts over the Indian monsoon region, Weather Climate Extremes, 12, 43–68, 2016.
Mann, H.: Non-Parametric Tests against Trend, Econmetrica, 13, 245–259, 1945
Martí, P., Zarzo, M., Vanderlinden, K., and Girona, J.: Parametric expressions for the adjusted Hargreaves coefficient in Eastern Spain, J. Hydrol., 529, 1713–1724, 2015.
McGuire, J. K. and Palmer, W. C.: The 1957 drought in the eastern United States, Mon. Weather Rev., 85, 305–314, 1957.
McKee, T. B., Doesken, N. J., and Kleist, J.: The relationship of drought frequency and duration to time scales Eighth Conference on Applied Climatology, American Meteorological Society, Boston, Eighth Conf. Appl. Climatol., available at: https://climate.colostate.edu/pdfs/relationshipofdroughtfrequency.pdf (last access: 9 February 2021), 1993.
Mendicino, G. and Senatore, A.: Regionalization of the Hargreaves coefficient for the assessment of distributed reference evapotranspiration in Southern Italy, J. Irrig. Drain. Eng., 139, 349–362, 2013.
Men-Xin, W. and Hou-Quan, L.: A modified vegetation water supply index (MVWSI) and its application in drought monitoring over Sichuan and Chongqing, China, J. Integr. Agr., 15, 2132–2141, 2016.
Mishra, A. K. and Singh, V. P.: A review of drought concepts, J. Hydrol., 391, 202–216, 2010.
Monish, N. and Rehana, S.: Suitability of distributions for standard precipitation and evapotranspiration index over meteorologically homogeneous zones of India, J. Earth Syst. Sci., 129, 2132–2141, 2020.
Morton, F. I.: Operational estimates of areal evapotranspiration and their significance to the science and practice of hydrology, J. Hydrol., 66, 1–76, 1983.
Pendergrass, A. G., Meehl, G. A., Pulwarty, R., Hobbins, M., Hoell, A., AghaKouchak, A., Bonfils, C. J., Gallant, A. J., Hoerling, M., and Hoffmann, D.: Flash droughts present a new challenge for subseasonal-to-seasonal prediction, Nat. Clim. Change, 10, 191–199, 2020.
Penman, H. L.: Natural evaporation from open water, bare soil and grass, Proc. R. Soc. Lon. Ser.-A, 193, 120–145, 1948.
Potop, V., Boroneanţ, C., Možný, M., Štěpánek, P., and Skalák, P.: Observed spatiotemporal characteristics of drought on various time scales over the Czech Republic, Theor. Appl. Climatol., 115, 563–581, 2014.
Priestley, C. H. B. and Taylor, R.: On the assessment of surface heat flux and evaporation using large-scale parameters, Mon. Weather Rev., 100, 81–92, 1972.
Salvador, C., Nieto, R., Linares, C., Diaz, J., and Gimeno, L.: Effects on daily mortality of droughts in Galicia (NW Spain) from 1983 to 2013, Sci. Total Environ., 662, 121–133, 2019.
Sen, P. K.: Estimates of the regression coefficient based on Kendall's tau, J. Am. Stat. Assoc., 63, 1379–1389, 1968.
Sheffield, J., Andreadis, K., Wood, E. F., and Lettenmaier, D.: Global and continental drought in the second half of the twentieth century: severity–area–duration analysis and temporal variability of large-scale events, J. Climate, 22, 1962–1981, 2009.
Sohn, S. J., Ahn, J. B., and Tam, C. Y.: Six month–lead downscaling prediction of winter to spring drought in South Korea based on a multimodel ensemble, Geophys. Res. Lett., 40, 579–583, 2013.
Stagge, J. H., Tallaksen, L. M., Gudmundsson, L., Van Loon, A. F., and Stahl, K.: Candidate distributions for climatological drought indices (SPI and SPEI), Int. J. Climatol., 35, 4027–4040, 2015.
Thomas, A.: Spatial and temporal characteristics of potential evapotranspiration trends over China, Int. J. Climatol., 20, 381–396, 2000.
Thornthwaite, C.: Report of the Committee on Transpiration and Evaporation 1943-44, T. AGU, 25, 683–693, 1944.
Tirivarombo, S., Osupile, D., and Eliasson, P.: Drought monitoring and analysis: standardised precipitation evapotranspiration index (SPEI) and standardised precipitation index (SPI), Phys. Chem. Earth, 106, 1–10, 2018.
Trenberth, K. E., Dai, A., Van Der Schrier, G., Jones, P. D., Barichivich, J., Briffa, K. R., and Sheffield, J.: Global warming and changes in drought, Nat. Clim. Change, 4, 17–22, 2014.
Van der Schrier, G., Jones, P., and Briffa, K.: The sensitivity of the PDSI to the Thornthwaite and Penman-Monteith parameterizations for potential evapotranspiration, J. Geophys. Res.- Atmos., 116, https://doi.org/10.1029/2010JD015001, 2011.
Vicente-Serrano, S. M., Beguería, S., and López-Moreno, J. I.: A multiscalar drought index sensitive to global warming: the standardized precipitation evapotranspiration index, J. Climate, 23, 1696–1718, 2010.
Vicente-Serrano, S. M., López-Moreno, J. I., Beguería, S., Lorenzo-Lacruz, J., Azorin-Molina, C., and Morán-Tejeda, E.: Accurate computation of a streamflow drought index, J. Hydrol. Eng. 17, 318–332, 2012.
Wan, Z., Wang, P., and Li, X.: Using MODIS land surface temperature and normalized difference vegetation index products for monitoring drought in the southern Great Plains, USA, Int. J. Remote Sens., 25, 61–72, 2004.
Wang, Q., Wu, J., Lei, T., He, B., Wu, Z., Liu, M., Mo, X., Geng, G., Li, X., and Zhou, H.: Temporal-spatial characteristics of severe drought events and their impact on agriculture on a global scale, Quatern. Int., 349, 10–21, 2014.
Wang, Q., Shi, P., Lei, T., Geng, G., Liu, J., Mo, X., Li, X., Zhou, H., and Wu, J.: The alleviating trend of drought in the Huang-Huai-Hai Plain of China based on the daily SPEI, Int. J. Climatol., 35, 3760–3769, 2015.
Wang, Q., Wu, J., Li, X., Zhou, H., Yang, J., Geng, G., An, X., Liu, L., and Tang, Z.: A comprehensively quantitative method of evaluating the impact of drought on crop yield using daily multi-scale SPEI and crop growth process model, Int. J. Biometeorol., 61, 685–699, 2017.
Wang, Q., Tang, J., Zeng, J., Qu, Y., Zhang, Q., Shui, W.,Wang, W., Yi, L., and Leng, S.: Spatial-temporal evolution of vegetation evapotranspiration in Hebei Province, China, J. Integr. Agr., 17, 2107–2117, 2018.
Wang, Q., Qi, J., Li, J., Cole, J., Waldhoff, S. T., and Zhang, X.: Nitrate loading projection is sensitive to freeze-thaw cycle representation, Water Res., 186, 116355, https://doi.org/10.1016/j.watres.2020.116355, 2020a.
Wang, Q., Qi, J., Wu, H., Zeng, Y., Shui, W., Zeng, J., and Zhang, X.: Freeze-Thaw cycle representation alters response of watershed hydrology to future climate change, Catena, 195, 104767, https://doi.org/10.1016/j.catena.2020.104767, 2020b.
Wang, Q., Zeng J., Qi J., Zhang, X., Zeng, Y., Shui, W., Xu. Z., Zhang, R., and Wu, X.: muliti-scale daily SPEI dataset over the Mainland China from 1961–2018 (version June 2020), dataset, Figshare, https://doi.org/10.6084/m9.figshare.12568280, 2020c.
Wang, Y., Zhao, W., Zhang, Q., and Yao, Y.-B.: Characteristics of drought vulnerability for maize in the eastern part of Northwest China, Sci. Rep.-UK, 9, 1–9, 2019.
Wilhite, D. A. and Glantz, M. H.: Understanding: the drought phenomenon: the role of definitions, Water Int., 10, 111–120, 1985.
Yang, P., Xia, J., Zhang, Y., Zhan, C., and Qiao, Y.: Comprehensive assessment of drought risk in the arid region of Northwest China based on the global palmer drought severity index gridded data, Sci. Total Environ., 627, 951–962, 2018.
Yevjevich, V. M.: Objective approach to definitions and investigations of continental hydrologic droughts, Hydrology papers (Colorado State University), no. 23, https://doi.org/10.1016/0022-1694(69)90110-3, 1967.
Yu, M., Li, Q., Hayes, M. J., Svoboda, M. D., and Heim, R. R.: Are droughts becoming more frequent or severe in China based on the standardized precipitation evapotranspiration index: 1951–2010?, Int. J. Climatol., 34, 545–558, 2014.
Zambrano, F., Vrieling, A., Nelson, A., Meroni, M., and Tadesse, T.: Prediction of drought-induced reduction of agricultural productivity in Chile from MODIS, rainfall estimates, and climate oscillation indices, Remote Sens. Environ., 219, 15–30, 2018.
Zargar, A., Sadiq, R., Naser, B., and Khan, F. I.: A review of drought indices, Environ. Rev., 19, 333–349, 2011.