the Creative Commons Attribution 4.0 License.
the Creative Commons Attribution 4.0 License.
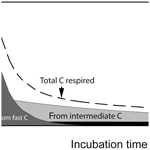
Decomposability of soil organic matter over time: the Soil Incubation Database (SIDb, version 1.0) and guidance for incubation procedures
Christina Schädel
Jeffrey Beem-Miller
Mina Aziz Rad
Susan E. Crow
Caitlin E. Hicks Pries
Jessica Ernakovich
Alison M. Hoyt
Alain Plante
Shane Stoner
Claire C. Treat
Carlos A. Sierra
The magnitude of carbon (C) loss to the atmosphere via microbial decomposition is a function of the amount of C stored in soils, the quality of the organic matter, and physical, chemical, and biological factors that comprise the environment for decomposition. The decomposability of C is commonly assessed by laboratory soil incubation studies that measure greenhouse gases mineralized from soils under controlled conditions. Here, we introduce the Soil Incubation Database (SIDb) version 1.0, a compilation of time series data from incubations, structured into a new, publicly available, open-access database of C flux (carbon dioxide, CO2, or methane, CH4). In addition, the SIDb project also provides a platform for the development of tools for reading and analysis of incubation data as well as documentation for future use and development. In addition to introducing SIDb, we provide reporting guidance for database entry and the required variables that incubation studies need at minimum to be included in SIDb. A key application of this synthesis effort is to better characterize soil C processes in Earth system models, which will in turn reduce our uncertainty in predicting the response of soil C decomposition to a changing climate. We demonstrate a framework to fit curves to a number of incubation studies from diverse ecosystems, depths, and organic matter content using a built-in model development module that integrates SIDb with the existing SoilR package to estimate soil C pools from time series data. The database will help bridge the gap between point location measurements, which are commonly used in incubation studies, and global remote-sensed data or data products derived from models aimed at assessing global-scale rates of decomposition and C turnover. The SIDb version 1.0 is archived and publicly available at https://doi.org/10.5281/zenodo.3871263 (Sierra et al., 2020), and the database is managed under a version-controlled system and centrally stored in GitHub (https://github.com/SoilBGC-Datashare/sidb, last access: 26 June 2020).
- Article
(6342 KB) - Full-text XML
-
Supplement
(233 KB) - BibTeX
- EndNote
Temperature, soil moisture, soil type, plant–microbe interactions, microbial community compositions, physical protection of organic matter (e.g., sorption on minerals and aggregation), and physical disconnection of microbes/enzymes and their substrates all control microbial decomposition processes and fluxes of greenhouse gases to the atmosphere (Conant et al., 2011; Schmidt et al., 2011). The relative importance of all these factors in controlling decomposition processes is poorly quantified but is important to understand as warming temperatures shift rates of microbial processes, potentially increasing releases of soil-stored carbon (C) to the atmosphere (Davidson and Janssens, 2006).
Research synthesis (e.g., meta-analysis) has become an increasingly important tool in science to overcome site-specific results, identify universal patterns across ecosystems and at global scales, and assess what is known and what needs further research (Gurevitch et al., 2018; Gurevitch and Hedges, 1999; Hillebrand and Gurevitch, 2013; Osenberg et al., 1999). Numerous reviews, syntheses, and meta-analyses have been performed using laboratory incubation studies (e.g., Conant et al., 2011; Hamdi et al., 2013; Schädel et al., 2014, 2016; Treat et al., 2015) to answer questions about the relative decomposability or stability of soil organic matter, the temperature response of soil respiration, and the ratio of CO2:CH4 production in anaerobic incubations. New experiments are continuously contributing to the growing body of soil incubation literature. While individual soil incubation studies are performed to answer specific research questions that may not require measuring a large variety of variables, the more details that are provided and the more comprehensive the metadata are, the greater the utility of an individual study beyond its original use (Hillebrand and Gurevitch, 2013). Metadata help to characterize these datasets, enable identification of data through relevant criteria, and provide the information needed for data archiving (Hillebrand and Gurevitch, 2013; Jiang et al., 2015), making individual incubation studies as useful as possible.
Here, we report on the development and compilation of a subset of available incubation data into a new, publicly available Soil Incubation Database (SIDb). In addition to introducing SIDb, we provide clear reporting guidance for database entry and the required variables that incubation studies need at minimum to be included in SIDb. Further, we provide guidance and associated recommendations to help inform best practices for conducting consistent, comparable soil incubation studies while retaining the adaptability required for individual research groups and projects.
A key application of this synthesis effort is to better characterize soil C processes in Earth system models, which will in turn reduce our uncertainty in predicting the response of soil C decomposition to a changing climate. Soil C decomposition is traditionally represented by a simple first-order decay function (Jenkinson, 1990) in C cycle models assuming one or more conceptual C pools (Davidson and Janssens, 2006; Parton et al., 1987; Trumbore, 1997) with fast and slower rates of C turnover. The models are described by several parameters such as the decay rate of each pool, as well as the transfer rates among pools. These parameters can be utilized to predict the evolution of CO2 one would observe in an incubation over time. Incubation time series data could therefore be used to constrain the parameters of these models by solving the corresponding inverse problem.
We demonstrate a framework to fit such curves to a number of incubation studies from diverse ecosystems, depths, and organic matter content using a built-in model development module that integrates SIDb with the existing SoilR package (Sierra et al., 2012) to estimate soil C pools from time series data. This allows users to test different model structures against their data, representing a benefit of contributing data to SIDb. We hope the database will help bridge the gap between localized measurements, which are commonly used in incubation studies, and global remote-sensed data or data products derived from models aimed at assessing global-scale rates of decomposition and C turnover (Carvalhais et al., 2014; Koven et al., 2017). This work also complements other compilations of soil-C-related datasets such as the International Soil Carbon Network (https://iscn.fluxdata.org/, last access: 26 June 2020); the open-source Continuous Soil Respiration database, COSORE (https://github.com/bpbond/cosore, last access: 26 June 2020); the Global Database of Soil Respiration Data, Version 4.0 (Bond-Lamberty and Thomson, 2018); and the International Soil Radiocarbon Database (ISRaD, http://soilradiocarbon.org/, last access: 26 June 2020; Lawrence et al., 2020).
Laboratory soil incubation studies are a commonly used method to estimate the decomposability of soil organic matter by measuring greenhouse gas release as C is mineralized from soils under controlled conditions. Results from incubation studies can inform global models about C pool sizes and rates of soil organic matter processing (mostly derived from long-term incubations) and sensitivities of process rates with respect to changes in abiotic factors such as soil temperature, moisture, pH, etc. Incubation durations may vary from less than 1 d to up to many years. Short-term incubations (a few days to a few months) provide information on how much C is readily decomposable and may be closer to the initial conditions experienced within the soil profile. Long-term incubations (months to years) may diverge from the conditions found within the profile but can give insights into the potential decomposability of slower cycling C (e.g., Schädel et al., 2014). At the beginning of laboratory incubations, respiration of fast-cycling C dominates total C respired, but it declines rapidly, whereas slow-cycling C accounts for most of the C being respired after the fast C pool is mostly depleted (Fig. 1). In this respect, laboratory incubations serve as a method to biologically partition soil C into different kinetic pools using the microbes themselves as the main partitioning agent. The time series produced is often well approximated by a sum of exponential functions, which are the solution of systems of first-order linear differential equations with constant coefficients (Metzler and Sierra, 2018). Fitting data from incubations to these types of functions has been done for individual site-level studies (e.g., Schädel et al., 2013, 2014; Sierra et al., 2017).
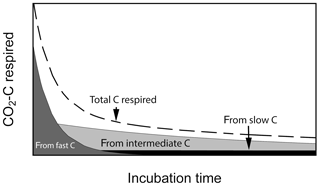
Figure 1Conceptual figure of C respiration during aerobic soil incubations. Total CO2−C flux is composed of contributions from different C pools which changes over time. Fast-cycling C dominates total CO2−C flux at the beginning of the incubation and is later replaced by slower-cycling C pools.
Like all methods, incubations have their advantages and disadvantages. Many laboratory methods exist for splitting soil C into pools of various purported stabilities (e.g., density fractionation, Sollins et al., 2006; sequential extraction, Heckman et al., 2018; and thermal analysis, Barré et al., 2016), but incubations are the only biological assay for testing soil C stability, an ultimately biological process. Carbon stability is a measure of how resistant and inaccessible organic molecules are to microbial decay.
Another distinct advantage of incubations is the high level of control they allow, compared to field methods. For example, incubations that test the temperature sensitivity of C flux (e.g., Bracho et al., 2016; Conant et al., 2008) offer a greater level of control compared to field measurements in several ways. First, in situ soil respiration is a mixture of both heterotrophic microbial respiration and autotrophic root respiration; soil incubations isolate the heterotrophic flux. Second, in situ temperatures change daily and seasonally, thereby confounding any direct effects of temperature with the phenology of C inputs such as root exudates and litter fall. At many locations, such as those under Mediterranean climate regimes, temperature is highly correlated with soil moisture so that the effects of one are impossible to disentangle from the other (Sierra et al., 2015; Subke and Bahn, 2010). With incubations, temperature and moisture effects can be tested both in isolation and with interactions. Incubations are a tractable and accessible method that can be run with minimal equipment (scale, gas-tight jars that seal, and a CO2 analyzer). Much of the utility of incubations lies in their simplicity. Lastly, as described above, the time series data collected by most incubations can be connected to soil C models (Sierra et al., 2012, 2014).
The main shortcoming of incubations is their isolation from the soil ecosystem. Incubations lack new inputs, which could otherwise prime the decomposition of the existing soil C pool (Huo et al., 2017). However, the lack of inputs simplifies the system and allows a focus on decay processes. Substrates can be added to incubations to measure the decomposability of specific compounds or materials (particularly if they are isotopically labeled), or to measure the priming effect under experimentally controlled conditions, a common extension of incubation methods (e.g., Finley et al., 2018; Pegoraro et al., 2019). Additionally, the microbial community in incubations may not reflect in situ communities. For example, constant environmental conditions in incubations may reduce the available niches and potentially result in a decline of microbial diversity – an effect that has yet to be tested. The lack of inputs can also induce changes in the microbial community as more oligotrophic microbes are favored over time. Lastly, soils used in incubations are always disturbed to varying degrees during removal from the field and often further in the laboratory: during sieving or root-picking procedures, or through rewetting prior to the start of the incubation. For example, at the time of publication, half of the studies in our database reported sieving prior to incubation, while a third do not report pre-incubation procedures. This disturbance may increase the susceptibility of occluded soil C to decay via disruption of aggregates, potentially overestimating the amount of C released during incubations relative to field conditions (Salomé et al., 2010). In general, the experimental control of incubations allows for most of these criticisms to be explicitly tested and accounted for as needed, and overall, the advantages of incubations far outweigh their drawbacks when the goal is understanding C pool structure, C stability, and C sensitivity to drivers such as temperature and moisture.
The Soil Incubation Database (SIDb) version 1.0 is an open-source software project that provides open access to data and is a platform for the development of tools for reading and analysis of data as well as documentation for future use and development. The data are freely available at https://doi.org/10.5281/zenodo.3871263 (Sierra et al., 2020), and the database is managed under a version-controlled system and centrally stored in GitHub (https://github.com/SoilBGC-Datashare/sidb, last access: 26 June 2020).
3.1 The repository
The structure of the SIDb project contains three main folders: data, docs, and Rpkg, which provide access to the database, the website (https://soilbgc-datashare.github.io/sidb/, last access: 26 June 2020), and the R package. The tree structure of the essential repository components is as follows
-
SIDb project
-
Readme.md
-
LICENSE.md
-
travis.yml
-
data
-
entry1
- –
initConditions.csv
- –
metadata.yaml
- –
timeSeries.csv
- –
-
-
docs
-
_config.yml
-
index.html
-
_layouts
-
_includes
-
assets
-
css
-
-
tests
-
testthat
- –
test_dataStructure.R
- –
-
data_test.R
-
pkg_test.sh
-
-
Rpkg
-
DESCRIPTION
-
NAMESPACE
-
R
-
data
-
inst
-
man
-
vignettes
-
3.2 The database
The open-source approach to SIDb allows data access, manipulation, analysis, and contribution to be accomplished without proprietary software. The soil incubation data are stored in the data folder. Each entry in the database consists of a folder containing three files and has the name convention AuthornameYEAR (optionally with journal name abbreviation appended) and the suffix “a” or “b” if multiple entries for one author and year exist. (1) The metadata.yaml file contains the following required sections: citation and curator information, basic site information (siteInfo), experimental setup of incubation (incubationInfo), and the metadata for the variable in the time series data (variables). The structure of the metadata file allows for flexible inclusion of many types of experimental and incubation designs. (2) The initConditions.csv file includes site, treatment, and initial soil characteristics (C content, texture conditions, etc.; Table 1). (3) The timeSeries.csv file contains measurements made over the course of the incubation. Column headers in the timeSeries.csv file are required to match the values entered for variable names in the variables section of the metadata.yaml file (e.g., V1:name, V2:name). The Readme.md file in the data folder provides a detailed explanation of how to add entries to the data folder. Note that for entries to be ingested in SIDb they must pass certain QA–QC tests (described in detail in Sect. 3.2.4 in the R package).
3.2.1 The metadata file
The metadata file is a simple text file that includes all relevant information about the incubation study. The .yaml format is both human and machine readable. YAML (YAML Ain't Markup Language) files are text files that utilize indent hierarchy to store information in iterable and query-able format. Thus, data stored under main headings may contain subcategories and arrays of information. In an array, each line is started with a hyphen, followed by a space, then the data. A heading of any level must end with a colon, followed by a new line return. The metadata.yaml file contains four sections. The first section consists of bibliographical data about the database entry, including DOI and contact information (Fig. 2). The second section, siteInfo, includes geographic data, land cover, vegetation, and soil data (Fig. 3). The third section, incubationInfo, provides data on laboratory experimental setup and sample treatment (Fig. 4). The fourth section, variables, contains metadata for the individual columns of the timeseries.csv file (Fig. 5).
One advantage of the .yaml format is the ease with which specific types of data can be grouped in a hierarchical array. For example, in Fig. 3 site is a subfield of siteInfo, and latitude is a subfield of coordinates. More subfields can be added to the siteInfo subfield as necessary; however, adding a secondary subfield beneath existing subfields should be avoided in SIDb as consistent data structure is required for data aggregation. For example, in the siteInfo section, the variables coordinates, country, MAT, MAP, landCover, vegNotes, and soilTaxonomy all need to be equal to the length of the site array Fig. 3.
In Fig. 4, the incubationInfo field has a subfield with a description on how the incubations were carried out. This is important information for documenting the experimental conditions under which the incubations were conducted. However, specific treatments and experimental conditions (temperature, moisture, etc.) should be explicitly entered under the appropriate corresponding subfields (Fig. 4).
The last fields that must be filled in are in the variables section (Fig. 5). This section consists of, in sequential order, subsections containing the metadata that correspond to the respiration time series observations (columns) of the timeSeries.csv file. The number of variables (V1–Vn) must therefore correspond to the number of columns in the timeSeries.csv file. The first column in the timeSeries file must be a vector of time (in days or other consistent unit), and thus the first variable name (V1:name) in the variables section must also be “time”. Experimental and incubation treatments listed in the incubationInfo section must be specified under each variable (V2, V3, etc.). Note that if a treatment has only one level it will be reported in the incubationInfo section and does not need to be repeated in the variables section. For example, if all incubations were conducted at the same temperature, the incubation temperature would be reported under the temperature subheading in the incubationInfo section, and the information will be automatically propagated to all of the variables (example of Crow2019a in the database). However, if a treatment has multiple levels, e.g., an incubation study utilizing three temperatures, the temperature subheading under incubationInfo would be left blank, and the temperature level would need be specified for each variable in the variables section in a subheading called “temperature” (example of Bracho2018SBB in the database).
3.2.2 Data entries
The timeSeries.csv file for each entry in the database contains the time series of incubation data in comma-separated format. The first column of the data file must contain the times at which gas measurements were taken. Subsequent columns must contain the respiration measurements. The format of the data is irrelevant (e.g., units) as long as the relevant information to identify each respiration column is described in the variables field of the metadata file.
3.2.3 The website
Documentation of the project, which includes the database and the R package, is presented on the project's website (https://soilbgc-datashare.github.io/sidb/, last access: 26 June 2020). The website is publicly served by GitHub Pages. Every time new changes are pushed to the SIDb repository, the website is rebuilt and served automatically by GitHub.
3.2.4 The R package
Data in SIDb are stored in a format that can be read in any programming language. We provide an R package to allow users to compile or read the database into R and a platform to facilitate future analyses. To install the package, open R and run
-
install.packages(”devtools”)
-
devtools::install_github('SoilBGC-Datashare/sidb/Rpkg/', build_vignettes=TRUE).
Once the R package `sidb' is installed and loaded, a browser-based html version of the available vignettes can be accessed using
-
browseVignettes('sidb').
There are currently two vignettes available: “sidbQueryReportPlot” and “Fitting data to models”. The first vignette describes a simple workflow for querying, generating reports, and plotting data with SIDb. The second vignette demonstrates the model fitting functions built into the R package “sidb”.
In the sidb R package two main functions are provided: loadEntries.R and readEntry.R. As their names suggest, loadEntries.R collects all metadata and data from all entries and produces an “R list” with the entire database. The function readEntry.R reads individual entries from the database and also produces an “R list”. The package also provides a function that “flattens” and coerces the database list object into a simpler data structure for easier querying (flatterSIDb.R), as well as stand-alone functions to query the entire database in its native list format for specific variables. For instance, the function coordinates, R extracts all latitudes and longitudes for each entry in the database. Similarly, other functions are provided to extract C and nitrogen (N) content, or the incubation duration of each entry.
Quality control is provided for code testing and data validation. A brief overview is given here and more details can be found in the Readme.md file located in the directory “sidb/tests” within the SIDb GitHub repository. Code testing can be done both locally and remotely. For local testing we have written a shell script that runs an R CMD check on the package directory (github: sidb/tests/pkg_test.sh). For remote testing, we use Travis Continuous Integration to run an R CMD check on the Rpkg directory of the SIDb GitHub repository. This ensures that any modifications to the functions or other aspects of the sidb R package are tested every time a new commit is made in the repository and that we will be notified of any errors, warnings, or issues.
For data validation, raw SIDb data (entry files that live outside the R package in the “data” directory) can be tested for conformity to SIDb standards using the file “data_test.R” (github: sidb/tests/data_test.R). This R script runs all tests in the subdirectory “testthat”. Tests can be run from the command line or directly inside R using the R package devtools. Contributors of new data or code must run these tests before contributing to SIDb and no pull requests will be accepted if any of the tests fail.
3.3 Summary statistics in SIDb version 1.0
The database is a work in progress: currently SIDb includes 31 studies with 684 time series, representing a total number of 42 545 data points (Fig. 6). Most entries contain multiple time series of CO2 fluxes. Incubations reported in SIDb were performed under temperatures ranging from 0 to 40 ∘C with the majority of incubations under normal laboratory temperature (20–25 ∘C) (Fig. 6a). Soil temperature is the most frequently reported laboratory treatment, while soil moisture is less frequently reported despite the fact that it is also a key factor in incubation studies. The omission of soil moisture data may be related to inconsistencies in reporting conventions, a topic that is discussed further in Sect. 4.3. All soils listed in our database included surface soil samples; however some studies considered soil depth as a treatment and report incubation data from soil layers as deep as 1.2 m (Fig. 6a).
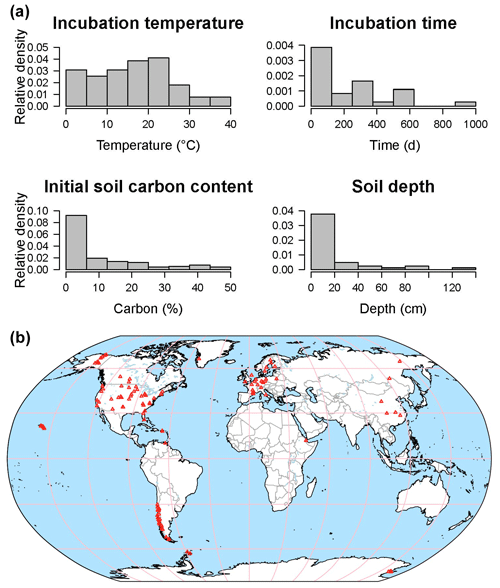
Figure 6Data distribution histograms of incubation temperature, time, initial soil C content, and soil depth for available incubation data in SIDb 1.0 (a). Map of currently available incubation studies (b).
Important geographic and ecological gaps exist in SIDb version 1.0. Coverage is highest in temperate followed by arctic regions, with only a few studies in tropical areas while the continents of Africa and Australia are barely represented (Fig. 6b). Incubation data from the tropics are currently poorly represented in SIDb despite their vulnerability and the importance of tropical regions to global C cycling and therefore should be a priority for both future ingestion into SIDb and further study. For most ecosystems, there are still many incubation studies to be included into SIDb in the future. Additionally, recent work (Fontaine et al., 2007; Hicks Pries et al., 2018; Mathieu et al., 2015) has highlighted the importance of understanding deep soil processes and potential changes due to global warming. In fact, warming effects on respiration have been observed at depths as great as 1 m (Hicks Pries et al., 2017). Incubations of deep soils thus represent a major gap in SIDb, which is reflective of the lack of deep soil incubation studies more broadly, and present a large potential for future study. It was not our intention with SIDb to produce a comprehensive database. Instead, we want to introduce SIDb's structure, tools, and the current capacity of the database to the broader scientific community, with the potential to expand.
While consistent methods across studies facilitate meta-analysis, incubation studies must remain adaptable to each research question, available resources, and soil properties. Nonetheless, in developing SIDb and the entry template, the most critical required components of incubations for making comparisons across studies emerged. On the basis of these observations, we have generated a list of variables, including information about the sites, soils, and setup of the incubation itself, that we require in order for a study to be ingested in SIDb (Table 1). Here, we discuss the issues associated with these critical variables and make suggestions for other useful variables to report that, while not required, will increase the interpretability of results and allow for broader inclusion into syntheses and meta-analyses (Table 1). In the Supplement, we also offer a limited discussion of methodologies and measurements such as incubation setup, sample preparation, additional variables to measure, and special considerations for radiocarbon incubations.
4.1 Site information
Site characteristics provide a context for the inherent conditions of the soils. General site characteristics, such as latitude and longitude, mean annual temperature, and mean annual precipitation are important in drawing out the similarities or differences between studies. Descriptions of the ecosystem and the aboveground vegetation give information on litter input and chemistry, which can be a direct link to organic matter quality. Additionally, providing information on the soil order and taxonomy helps to put findings into context with other studies (Schimel and Chadwick, 2013).
4.2 Soil characteristics
There are ultimately two essential soil variables that must be reported for incubation studies and a myriad of suggested variables that facilitate comparisons among and explorations of potential drivers. The first essential soil variable is depth, which is a major organizing factor of many soil characteristics. No matter whether an individual incubation study measured soil from a single depth increment or multiple depth increments, either the depth increment (top, bottom, and middle) or the horizon must be reported. Ideally, both depth and horizon should be reported as samples can be taken from a generic depth or from a mixture of horizons (when sampled to a certain depth). All subsequent soil characteristics should then be reported for each depth increment or horizon incubated and provided in the initConditions.csv file.
When reporting the sampling depth, it is necessary to report whether depth is in relation to the soil surface, which can be defined as the top of the mineral soil or the top of the organic horizon depending on the system, or within a specific soil horizon. Additionally, specifics of the geography and topography of the sampling locations, such as permafrost zone, active layer thickness, or water table depth in permafrost and peatlands, are crucial to report.
Table 1Required (R) and suggested (S) variables to report and measure prior to or during time series soil incubations.
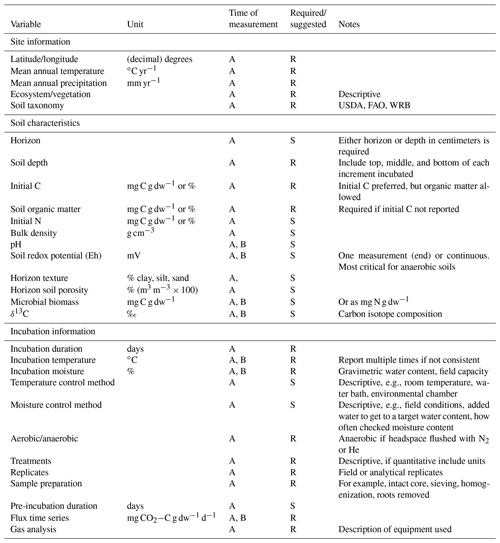
A: report once; B: can be reported multiple times during incubation.
The second required soil variable is either the initial C (reported in milligrams of carbon per gram dry weight or percent) or organic matter (which can be converted to C), which is essential for facilitating comparisons across studies and for normalizing rates of C losses during incubations. Other common and useful variables to measure are initial N (reported in milligrams of carbon or nitrogen per gram dry weight or percent), bulk density in grams per cubic centimeter, soil texture, and pH.
Most soil characteristics, as listed in Table 1, can be measured at the beginning of an incubation on a subsample of the soil being incubated, while others like pH, redox, or microbial biomass may be best measured multiple times during the course of an incubation (see Supplement for more details). For anaerobic incubations, we strongly recommend measuring redox potential because it may not be sufficient to assume that anoxic conditions (e.g., soils inundated with water and headspace filled with N2 or He) will result in the production of CH4 during the incubation as there can be a considerable lag period before CH4 production occurs (Knoblauch et al., 2018; Treat et al., 2015).
4.3 Incubation information
Details of incubation studies should be reported as they enhance the value of a primary study, but also, critically, they determine whether or not they can be included in a synthesis or meta-analysis. Thus, most of the information about how an incubation and its treatments are carried out is required in SIDb. Incubation duration, temperature, and soil moisture are among the most important details to provide because they directly affect microbial activity and therefore C flux rates (Table 1). For temperature and soil moisture, it needs to be clarified whether temperature and moisture were controlled at a single value or whether there were multiple temperature or moisture treatment levels. For temperature, details on how incubation temperature was achieved should be provided (e.g., water bath, freezer, or controlled environment chamber). For moisture, it should be specified whether the soils were all brought to the same moisture content or left at field conditions. For below-freezing incubation temperatures, unfrozen soil water can also be quantified, if possible, as temperature responses of CO2 production at subzero temperatures are influenced by water availability (Öquist et al., 2009). Moisture treatments range from fully aerobic (either drier than or at field capacity) to fully anoxic (headspace of jar flushed with N2 or helium) to fluctuating moisture conditions. In aerobic incubations, soils are often freely drained and deionized water is added over the course of the incubation to maintain constant moisture content. However, caution should be paid in order to maintain constant moisture through the incubation and not allow soils to dry out as drying and rewetting of soils can affect C mineralization rates and microbial activity (Birch, 1958; Rey et al., 2005; Unger et al., 2010). In addition, adjustments to soil moisture are ideally made at least 24–48 h prior to making measurements to minimize confounding effects of water addition (Rey et al., 2005). For anaerobic incubations it may not be necessary to add water during the course of the incubation as incubation vessels typically remain closed, but caution should be taken if water is added as it often contains dissolved oxygen. Other critical parameters to report about the incubation from the synthesis perspective include whether replicates are field (i.e., spatially different soil cores) or analytical replicates, whether soil samples were homogenized (e.g., by soil sieving), or whether roots were removed prior to incubation (see Supplement for more information). Lastly, the duration of a pre-incubation should be reported if carried out.
Flux measurements
Incubation data are most commonly published as C flux rates or cumulative C release over time for the whole incubation period. SIDb is designed around incubation studies that report respiration rates and cumulative release over time (timeSeries.csv), and time series data are required for inclusion in SIDb. Reporting only one average flux value, one maximum production value, or one single cumulative C release value for the whole incubation period may be useful for comparison of treatments within a study but omits key information about changes in C dynamics over time and precludes our ability to model dynamics of different C pools. If changes in C dynamics over time are not of interest for a specific study, time series data should be provided in supplementary material or in a data repository such as SIDb. Flux rates can be provided on a per gram of dry soil or per gram of soil C basis (mg CO2−C g dry weight−1 d−1 or mg CO2−C g−1 soil C d−1). These units can be easily converted to one another using the required initial C data (Table 1). Providing flux rates on a wet-weight soil basis or per volume of soil slurry is discouraged, as SIDb does not support this format and it precludes comparisons to other studies. If units of dry weight are not available, then soil moisture content and bulk density need to be reported so that data can be converted to standard units. Reporting C release on a per gram of C basis captures information about C decomposability and reveals information about the relative C release from a given soil that is independent of its C quantity; this is particularly useful for comparisons among soils, sites, and incubation studies (Schädel et al., 2014).
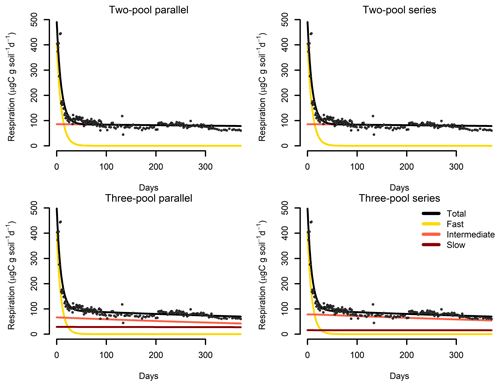
Figure 7Results from a parameter optimization procedure to soil incubation data from a native tropical forest of Hawaii. The parallel model structures do not consider transfers of C among pools, while the series model structures transfer C sequentially from fast- to slow-cycling pools. In all cases, the models fitted the data relatively well (Table 2) and identified the relative contribution of the different pools to the overall respiration flux.
Our incubation database can be easily integrated with other R packages for further analyses. For instance, it is possible to integrate soil C pool modeling from the SoilR package (Sierra et al., 2012) with parameter optimization from the FME package (Soetaert and Petzoldt, 2010). We illustrate this functionality with a simple example. The entry Crow2019a in the database contains a large number of long-term incubations (371 d). From those incubations, we selected data from a native forest in Hawaii and fitted a set of first-order models with two or three pools. Following the procedure described in Sierra et al. (2015), we optimized two- and three-pool models with parallel, series, and feedback connections among them (Fig. 7). Depending on the question asked, different criteria can be considered to select the best model (e.g., Akaike information criterion or number of parameters, Table 2), and it is beyond this paper to identify the best model; we simply show the basics of an example using SIDb.
There are two approaches to database building, which can be characterized by tradeoffs between the scope and quantity of data, the ease of data analysis, and the simplicity of data entry. SIDb has a narrow scope (i.e., incubation time series), allowing for the flexibility to incorporate studies with different variable types and experimental designs, while the data themselves are highly structured in order to facilitate data analysis. Other soil databases, such as the International Radiocarbon Database (ISRaD, Lawrence et al., 2020) or the International Soil Carbon Network (ISCN, https://iscn.fluxdata.org/, last access: 26 June 2020), have the advantage of a much larger quantity of data and a much broader scope. However, maintenance and data ingestion with these larger databases become much more challenging and require either (a) relaxing control of data structure, units of variables, and direct data oversight, such as the case with the International Soil Carbon Network, or (b) in the case of the International Radiocarbon Database, increasing the complexity of the data structure while enforcing strict variable control, e.g., allowable names, factor levels for categorical data, and numerical limits for quantitative data. Owing to the broader scope, maintaining these larger databases inevitably requires additional time and effort.
However a database is structured, establishing a common set of required measurements, metadata, and site-level data provides transparency that helps both to identify and to reduce systematic bias. The statistical power provided by the wealth of data points in a database such as SIDb is only useful as long as any potential systematic bias is identified. For example, all studies in SIDb report data at the variable level with respect to a time variable, as well as provide information about the experimental design, where the samples were collected from, who performed the study, and how to access the original data. Additionally, providing data such as geographic coordinates, land cover, mean annual temperature, mean annual precipitation, soil taxonomy, and soil C content enables leveraging of databases that may have a different scope but contain potentially useful supporting data. For example, respiration time series data from SIDb could be compared to 14C content of bulk soil or respired 14CO2 from ISRaD (Lawrence et al., 2020) by stratifying both databases along common variables, or a query could be made using geographic coordinates, DOI, or other variables.
Version 1.0 of SIDb is publicly available at https://doi.org/10.5281/zenodo.3871263 (Sierra et al., 2020). Documentation of the project and the R package are presented on the project's website (https://soilbgc-datashare.github.io/sidb/, last access: 26 June 2020).
The database is open for reuse, and the usage license follows the MIT license (https://opensource.org/licenses/MIT, last access: 26 June 2020). When using the database or R package, users should cite this definition publication and consider citing individual studies (publication or dataset).
Currently, SIDb is a compilation of a wide range of incubation studies with built in capacities to summarize the database and conduct model comparisons for fitting curves to time series data. There is great potential benefit for the soil C community through identification and ingestion of new datasets into SIDb. Every incubation study is planned and performed to answer a specific question; however, when analyzed in aggregate, syntheses of incubation studies can help answer fundamental questions about soil C pools and their stability and vulnerability to global change. Furthermore, setting up incubation studies involves several decision points, such as whether to sieve or preincubate the soil, whose consequences have not yet been tested systematically, and which may be able to be tested using SIDb.
A comprehensive collection of existing laboratory incubation data will be invaluable for the synthesis of spatial, methodological, and functional trends, as well as for identifying key gaps in our current knowledge. Individual researchers are encouraged to add individual study results to the database, thereby helping fill gaps in our broader understanding of soil C cycling in the process. A key goal for the next stages of development in SIDb will focus on expanding the geographical and ecological coverage of the entries.
SIDb is specifically designed to host incubation data with time series of respiration rates to facilitate synthesis studies. We encourage researchers to archive their data in the format presented here, but we caution that this database is not a long-term archive. SIDb not only collects data in a structured format; it also provides tools for data analysis and reporting through an R package and a website. Soil incubations are a commonly used technique for answering many different kinds of research questions, and here we provide recommendations on best practices, as well as a common data infrastructure for reporting. We expect the size of this database to grow in the future as it can be used as a standard repository for time series soil incubation data following open-source standards.
The supplement related to this article is available online at: https://doi.org/10.5194/essd-12-1511-2020-supplement.
CAS designed the database; CAS, CS, JBM, MAR, SEC, AP, CHP, SS, and AMH built and populated the database while JBM provided technical database support. CS, JE, and CT developed the first version of incubation recommendations and CS wrote up the initial draft of the SIDb manuscript. All authors contributed to the writing.
The authors declare that they have no conflict of interest.
We acknowledge support from the US Geological Survey Powell Center.
Funding for the development of the database was provided by the Max Planck Society and the German Research Foundation (SI 1953/2-1). Christina Schädel was supported by the National Science Foundation Vulnerability of Permafrost Carbon Research Coordination Network grant no. 955713 with continued support from the National Science Foundation Research Synthesis and Knowledge Transfer in a Changing Arctic: Science Support for the Study of Environmental Arctic Change grant no. 1331083. Alison M. Hoyt, Jeffrey Beem-Miller, and Shane Stoner were funded by the European Research Council (Horizon 2020 Research and Innovation Programme – grant agreement no. 695101). Susan E. Crow was supported by the USDA National Institute of Food and Agriculture project HAW01130-H.
This paper was edited by Birgit Heim and reviewed by Ben Bond-Lamberty and one anonymous referee.
Barré, P., Plante, A. F., Cécillon, L., Lutfalla, S., Baudin, F., Bernard, S., Christensen, B. T., Eglin, T., Fernandez, J. M., Houot, S., Kätterer, T., Le Guillou, C., Macdonald, A., van Oort, F., and Chenu, C.: The energetic and chemical signatures of persistent soil organic matter, Biogeochemistry, 130, 1–12, https://doi.org/10.1007/s10533-016-0246-0, 2016.
Birch, H. F.: The effect of soil drying on humus decomposition and nitrogen availability, Plant Soil, 10, 9–31, https://doi.org/10.1007/bf01343734, 1958.
Bond-Lamberty, B. P. and Thomson, A. M.: A Global Database of Soil Respiration Data, Version 4.0, ORNL DAAC, Oak Ridge, Tennessee, USA, https://doi.org/10.3334/ORNLDAAC/1578, 2018.
Bracho, R., Natali, S., Pegoraro, E., Crummer, K. G., Schädel, C., Celis, G., Hale, L., Wu, L. Y., Yin, H. Q., Tiedje, J. M., Konstantinidis, K. T., Luo, Y. Q., Zhou, J. Z., and Schuur, E. A. G.: Temperature sensitivity of organic matter decomposition of permafrost-region soils during laboratory incubations, Soil Biol. Biochem., 97, 1–14, https://doi.org/10.1016/j.soilbio.2016.02.008, 2016.
Carvalhais, N., Forkel, M., Khomik, M., Bellarby, J., Jung, M., Migliavacca, M., Mu, M., Saatchi, S., Santoro, M., Thurner, M., Weber, U., Ahrens, B., Beer, C., Cescatti, A., Randerson, J. T., and Reichstein, M.: Global covariation of carbon turnover times with climate in terrestrial ecosystems, Nature, 514, 213–217, https://doi.org/10.1038/nature13731, 2014.
Conant, R. T., Drijber, R. A., Haddix, M. L., Parton, W. J., Paul, E. A., Plante, A. F., Six, J., and Steinweg, J. M.: Sensitivity of organic matter decomposition to warming varies with its quality, Glob. Change Biol., 14, 868–877, https://doi.org/10.1111/j.1365-2486.2008.01541.x, 2008.
Conant, R. T., Ryan, M. G., Agren, G. I., Birge, H. E., Davidson, E. A., Eliasson, P. E., Evans, S. E., Frey, S. D., Giardina, C. P., Hopkins, F. M., Hyvonen, R., Kirschbaum, M. U. F., Lavallee, J. M., Leifeld, J., Parton, W. J., Steinweg, J. M., Wallenstein, M. D., Wetterstedt, J. A. M., and Bradford, M. A.: Temperature and soil organic matter decomposition rates – synthesis of current knowledge and a way forward, Glob. Change Biol., 17, 3392–3404, https://doi.org/10.1111/j.1365-2486.2011.02496.x, 2011.
Davidson, E. A. and Janssens, I. A.: Temperature sensitivity of soil carbon decomposition and feedbacks to climate change, Nature, 440, 165–173, https://doi.org/10.1038/nature04514, 2006.
Finley, B. K., Dijkstra, P., Rasmussen, C., Schwartz, E., Mau, R. L., Liu, X.-J. A., van Gestel, N., and Hungate, B. A.: Soil mineral assemblage and substrate quality effects on microbial priming, Geoderma, 322, 38–47, https://doi.org/10.1016/j.geoderma.2018.01.039, 2018.
Fontaine, S., Barot, S., Barre, P., Bdioui, N., Mary, B., and Rumpel, C.: Stability of organic carbon in deep soil layers controlled by fresh carbon supply, Nature, 450, 277–280, https://doi.org/10.1038/nature06275, 2007.
Gurevitch, J. and Hedges, L. V.: Statistical issues in ecological meta-analysis, Ecology, 80, 1142–1149, https://doi.org/10.1890/0012-9658(1999)080[1142:SIIEMA]2.0.CO;2, 1999.
Gurevitch, J., Koricheva, J., Nakagawa, S., and Stewart, G.: Meta-analysis and the science of research synthesis, Nature, 555, 175–175, 2018.
Hamdi, S., Moyano, F., Sall, S., Bernoux, M., and Chevallier, T.: Synthesis analysis of the temperature sensitivity of soil respiration from laboratory studies in relation to incubation methods and soil conditions, Soil Biol. Biochem., 58, 115–126, https://doi.org/10.1016/j.soilbio.2012.11.012, 2013.
Heckman, K., Lawrence, C. R., and Harden, J. W.: A sequential selective dissolution method to quantify storage and stability of organic carbon associated with Al and Fe hydroxide phases, Geoderma, 312, 24–35, https://doi.org/10.1016/j.geoderma.2017.09.043, 2018.
Hicks Pries, C. E., Castanha, C., Porras, R. C., and Torn, M. S.: The whole-soil carbon flux in response to warming, Science, 355, 1420–1423, https://doi.org/10.1126/science.aal1319, 2017.
Hicks Pries, C. E., Sulman, B. N., West, C., O'Neill, C., Poppleton, E., Porras, R. C., Castanha, C., Zhu, B., Wiedemeier, D. B., and Torn, M. S.: Root litter decomposition slows with soil depth, Soil Biol. Biochem., 125, 103–114, https://doi.org/10.1016/j.soilbio.2018.07.002, 2018.
Hillebrand, H. and Gurevitch, J.: Reporting standards in experimental studies, Ecol. Lett., 1419–1420, https://doi.org/10.1111/ele.12190, 2013.
Huo, C., Luo, Y., and Cheng, W.: Rhizosphere priming effect: A meta-analysis, Soil Biol. Biochem., 111, 78–84, https://doi.org/10.1016/j.soilbio.2017.04.003, 2017.
Jenkinson, D. S.: The turnover of organic carbon and nitrogen in soil, Philos. T. R. Soc. B, 329, 361–368, https://doi.org/10.1098/rstb.1990.0177, 1990.
Jiang, L.-Q., O'Connor, S. A., Arzayus, K. M., and Parsons, A. R.: A metadata template for ocean acidification data, Earth Syst. Sci. Data, 7, 117–125, https://doi.org/10.5194/essd-7-117-2015, 2015.
Knoblauch, C., Beer, C., Liebner, S., Grigoriev, M. N., and Pfeiffer, E.-M.: Methane production as key to the greenhouse gas budget of thawing permafrost, Nat. Clim. Change, 8, 309–312, https://doi.org/10.1038/s41558-018-0095-z, 2018.
Koven, C. D., Hugelius, G., Lawrence, D. M., and Wieder, W. R.: Higher climatological temperature sensitivity of soil carbon in cold than warm climates, Nat. Clim. Change, 7, 817–822, 2017.
Lawrence, C. R., Beem-Miller, J., Hoyt, A. M., Monroe, G., Sierra, C. A., Stoner, S., Heckman, K., Blankinship, J. C., Crow, S. E., McNicol, G., Trumbore, S., Levine, P. A., Vindušková, O., Todd-Brown, K., Rasmussen, C., Hicks Pries, C. E., Schädel, C., McFarlane, K., Doetterl, S., Hatté, C., He, Y., Treat, C., Harden, J. W., Torn, M. S., Estop-Aragonés, C., Asefaw Berhe, A., Keiluweit, M., Della Rosa Kuhnen, Á., Marin-Spiotta, E., Plante, A. F., Thompson, A., Shi, Z., Schimel, J. P., Vaughn, L. J. S., von Fromm, S. F., and Wagai, R.: An open-source database for the synthesis of soil radiocarbon data: International Soil Radiocarbon Database (ISRaD) version 1.0, Earth Syst. Sci. Data, 12, 61–76, https://doi.org/10.5194/essd-12-61-2020, 2020.
Mathieu, J. A., Hatté, C., Balesdent, J., and Parent, É.: Deep soil carbon dynamics are driven more by soil type than by climate: a worldwide meta-analysis of radiocarbon profiles, Glob. Change Biol., 21, 4278–4292, https://doi.org/10.1111/gcb.13012, 2015.
Metzler, H. and Sierra, C. A.: Linear Autonomous Compartmental Models as Continuous-Time Markov Chains: Transit-Time and Age Distributions, Math. Geosci., 50, 1–34, https://doi.org/10.1007/s11004-017-9690-1, 2018.
Öquist, M. G., Sparrman, T., Klemedtsson, L., Drotz, S. H., Grip, H., Schleucher, J., and Nilsson, M.: Water availability controls microbial temperature responses in frozen soil CO2 production, Glob. Change Biol., 15, 2715–2722, https://doi.org/10.1111/j.1365-2486.2009.01898.x, 2009.
Osenberg, C. W., Sarnelle, O., Cooper, S. D., and Holt, R. D.: Resolving ecological questions through meta-analysis: Goals, metrics, and models, Ecology, 80, 1105–1117, https://doi.org/10.1890/0012-9658(1999)080[1105:reqtma]2.0.co;2, 1999.
Parton, W. J., Schimel, D. S., Cole, C. V., and Ojima, D. S.: Analysis of factors controlling soil organic-matter levels in great-plains grasslands, Soil Sci. Soc. Am. J., 51, 1173–1179, 1987.
Pegoraro, E., Mauritz, M., Bracho, R., Ebert, C., Dijkstra, P., Hungate, B. A., Konstantinidis, K. T., Luo, Y., Schädel, C., Tiedje, J. M., Zhou, J., and Schuur, E. A. G.: Glucose addition increases the magnitude and decreases the age of soil respired carbon in a long-term permafrost incubation study, Soil Biol. Biochem., 129, 201–211, https://doi.org/10.1016/j.soilbio.2018.10.009, 2019.
Rey, A., Petsikos, C., Jarvis, P. G., and Grace, J.: Effect of temperature and moisture on rates of carbon mineralization in a Mediterranean oak forest soil under controlled and field conditions, Eur. J. Soil Sci., 56, 589–599, https://doi.org/10.1111/j.1365-2389.2004.00699.x, 2005.
Salomé, C., Nunan, N., Pouteau, V., Lerch, T. Z., and Chenu, C.: Carbon dynamics in topsoil and in subsoil may be controlled by different regulatory mechanisms, Glob. Change Biol., 16, 416–426, https://doi.org/10.1111/j.1365-2486.2009.01884.x, 2010.
Schädel, C., Luo, Y., Evans, D. R., Fei, S., and Schaeffer, S. M.: Separating soil CO2 efflux into C-pool-specific decay rates via inverse analysis of soil incubation data, Oecologia, 171, 721–732, https://doi.org/10.1007/s00442-012-2577-4, 2013.
Schädel, C., Schuur, E. A. G., Bracho, R., Elberling, B., Knoblauch, C., Lee, H., Luo, Y., Shaver, G. R., and Turetsky, M. R.: Circumpolar assessment of permafrost C quality and its vulnerability over time using long-term incubation data, Glob. Change Biol., 20, 641–652, https://doi.org/10.1111/gcb.12417, 2014.
Schädel, C., Bader, M. K. F., Schuur, E. A. G., Biasi, C., Bracho, R., Capek, P., De Baets, S., Diakova, K., Ernakovich, J., Estop-Aragones, C., Graham, D. E., Hartley, I. P., Iversen, C. M., Kane, E. S., Knoblauch, C., Lupascu, M., Martikainen, P. J., Natali, S. M., Norby, R. J., O/'Donnell, J. A., Chowdhury, T. R., Santruckova, H., Shaver, G., Sloan, V. L., Treat, C. C., Turetsky, M. R., Waldrop, M. P., Wickland, K. P., O'Donnell, J. A., Chowdhury, T. R., Santruckova, H., Shaver, G., Sloan, V. L., Treat, C. C., Turetsky, M. R., Waldrop, M. P., and Wickland, K. P.: Potential carbon emissions dominated by carbon dioxide from thawed permafrost soils, Nat. Clim. Change, 6, 950–953, https://doi.org/10.1038/nclimate3054, 2016.
Schimel, J. and Chadwick, O.: What's in a name? The importance of soil taxonomy for ecology and biogeochemistry, Front. Ecol. Environ., 11, 405–406, https://doi.org/10.1890/13.wb.016, 2013.
Schmidt, M. W. I., Torn, M. S., Abiven, S., Dittmar, T., Guggenberger, G., Janssens, I. A., Kleber, M., Koegel-Knabner, I., Lehmann, J., Manning, D. A. C., Nannipieri, P., Rasse, D. P., Weiner, S., and Trumbore, S. E.: Persistence of soil organic matter as an ecosystem property, Nature, 478, 49–56, https://doi.org/10.1038/nature10386, 2011.
Sierra, C. A., Müller, M., and Trumbore, S. E.: Models of soil organic matter decomposition: the SoilR package, version 1.0, Geosci. Model Dev., 5, 1045–1060, https://doi.org/10.5194/gmd-5-1045-2012, 2012.
Sierra, C. A., Müller, M., and Trumbore, S. E.: Modeling radiocarbon dynamics in soils: SoilR version 1.1, Geosci. Model Dev., 7, 1919–1931, https://doi.org/10.5194/gmd-7-1919-2014, 2014.
Sierra, C. A., Trumbore, S. E., Davidson, E. A., Vicca, S., and Janssens, I.: Sensitivity of decomposition rates of soil organic matter with respect to simultaneous changes in temperature and moisture, J. Adv. Model. Earth Sy., 7, 335–356, https://doi.org/10.1002/2014MS000358, 2015.
Sierra, C. A., Malghani, S., and Loescher, H. W.: Interactions among temperature, moisture, and oxygen concentrations in controlling decomposition rates in a boreal forest soil, Biogeosciences, 14, 703–710, https://doi.org/10.5194/bg-14-703-2017, 2017.
Sierra, C. A., Beem-Miller, J., Voelkl, H., Stoner, S., Hicks-Pries, C., Schädel, C., Crow, S. E., Plante, A. F., Hoyt, A., Tilyou, M., and Azizi-Rad, M.: The Soil Incubation Database SIDb, Zenodo, https://doi.org/10.5281/zenodo.3871263, 2020.
Soetaert, K. and Petzoldt, T.: Inverse Modelling, Sensitivity and Monte Carlo Analysis in R Using Package FME, J. Stat. Softw., 33, 1–28, https://doi.org/10.18637/jss.v033.i03, 2010.
Sollins, P., Swanston, C., Kleber, M., Filley, T., Kramer, M., Crow, S., Caldwell, B. A., Lajtha, K., and Bowden, R.: Organic C and N stabilization in a forest soil: Evidence from sequential density fractionation, Soil Biol. Biochem., 38, 3313–3324, https://doi.org/10.1016/j.soilbio.2006.04.014, 2006.
Subke, J.-A. and Bahn, M.: On the “temperature sensitivity” of soil respiration: Can we use the immeasurable to predict the unknown?, Soil Biol. Biochem., 42, 1653–1656, https://doi.org/10.1016/j.soilbio.2010.05.026, 2010.
Treat, C. C., Natali, S. M., Ernakovich, J., Iversen, C. M., Lupascu, M., McGuire, A. D., Norby, R. J., Roy Chowdhury, T., Richter, A., Šantrůčková, H., Schädel, C., Schuur, E. A. G., Sloan, V. L., Turetsky, M. R., and Waldrop, M. P.: A pan-Arctic synthesis of CH4 and CO2 production from anoxic soil incubations, Glob. Change Biol., 21, 2787–2803, https://doi.org/10.1111/gcb.12875, 2015.
Trumbore, S. E.: Potential responses of soil organic carbon to global environmental change, P. Natl. Acad. Sci. USA, 94, 8284–8291, https://doi.org/10.1073/pnas.94.16.8284, 1997.
Unger, S., Máguas, C., Pereira, J. S., David, T. S., and Werner, C.: The influence of precipitation pulses on soil respiration – Assessing the “Birch effect” by stable carbon isotopes, Soil Biol. Biochem., 42, 1800–1810, https://doi.org/10.1016/j.soilbio.2010.06.019, 2010.
- Abstract
- Introduction
- Laboratory incubations as a tool to assess soil C decomposability
- The Soil Incubation Database (SIDb)
- Required and suggested data reporting for inclusion into SIDb
- Case study: fitting time series data to pool models in SIDb version 1.0
- SIDb connections to other databases
- Data availability and user guidelines
- Conclusion
- Author contributions
- Competing interests
- Acknowledgements
- Financial support
- Review statement
- References
- Supplement
- Abstract
- Introduction
- Laboratory incubations as a tool to assess soil C decomposability
- The Soil Incubation Database (SIDb)
- Required and suggested data reporting for inclusion into SIDb
- Case study: fitting time series data to pool models in SIDb version 1.0
- SIDb connections to other databases
- Data availability and user guidelines
- Conclusion
- Author contributions
- Competing interests
- Acknowledgements
- Financial support
- Review statement
- References
- Supplement