the Creative Commons Attribution 4.0 License.
the Creative Commons Attribution 4.0 License.
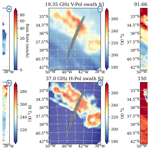
The PAZ polarimetric radio occultation research dataset for scientific applications
Estel Cardellach
Antía Paz
Santi Oliveras
Douglas C. Hunt
Sergey Sokolovskiy
Jan-Peter Weiss
Kuo-Nung Wang
F. Joe Turk
Chi O. Ao
Manuel de la Torre Juárez
Polarimetric radio occultations (PROs) represent an augmentation of the standard radio occultation (RO) technique that provides precipitation and cloud vertical information along with the standard thermodynamic products. A combined dataset that contains both the PRO observable retrievals and the RO standard retrievals, the resPrf, has been developed with the aim of fostering the use of these unique observations and fully exploiting the scientific implication of having information about vertical cloud structures with an intrinsically collocated thermodynamic state of the atmosphere. This paper describes such a dataset and provides detailed information on the processing of the observations. The procedure followed at the University Corporation for Atmospheric Research (UCAR) to combine both horizontal (H) and vertical (V) observations to generate profiles equivalent to those in standard RO missions is described in detail, and the obtained refractivity is shown to be of equivalent quality compared to that from TerraSAR-X. The steps for the processing of the PRO observations are detailed, derived products such as the top of the signal are described, and validation is provided.
Furthermore, the dataset contains the simulated ray trajectories for the PRO observation and collocated information with global satellite-based precipitation products, such as merged rain rate retrievals or passive microwave observations. These collocations are used for further validation of the PRO observations, and they are also provided within the resPrf profiles for additional use. It is also shown how accounting for external collocated information can significantly improve the effective PRO horizontal resolution, tackling one of the challenges of the technique. The resPrf dataset is publicly available at https://doi.org/10.20350/digitalCSIC/16137 (Padullés et al., 2024).
- Article
(4424 KB) - Full-text XML
- BibTeX
- EndNote
The Radio Occultation and Heavy Precipitation (ROHP) experiment aboard the PAZ satellite is providing, for the first time, polarimetric radio occultation (PRO) observations (Cardellach et al., 2014). This new observational technique represents an augmentation of the standard RO (e.g., Kursinski et al., 1997; Hajj et al., 2002) that measures the incoming Global Navigation Satellite System (GNSS) signals with a dual-polarized (H – horizontal, V – vertical) linearly orthogonal antennae instead of a right-hand circularly polarized (RHCP) one (which is used in all the other current and past standard RO missions). The advantage of collecting the H and V components of the incoming radio waves is that, in addition to the standard thermodynamic products, the phase difference between the H and V components contains information about the hydrometeors encountered along the ray path. Therefore, joint measurements of precipitation and thermodynamics are obtained.
The PAZ satellite was launched in February 2018 with the first ever payload able to collect PRO, which was activated on 10 May that same year. Roughly 1 year after the launch, the capability to detect rain was demonstrated (Cardellach et al., 2019). Validation of these observations has been performed extensively: Cardellach et al. (2019) and Padullés et al. (2020) showed the good agreement with two-dimensional precipitation products, such as NASA's Integrated Multi-satellitE Retrievals for Global Precipitation Mission (GPM, IMERG; Huffman, 2017) and the merged product of infrared brightness temperatures (TB) at 10.8 µm from geostationary satellites (Janowiak et al., 2017). In addition to precipitation, sensitivity to the upper layers of convective clouds has been demonstrated (Padullés et al., 2022), and a good climatological agreement with ice water content (IWC) retrievals is observed (Padullés et al., 2023). More recently, precise validation of the vertical structure of the signal has been performed using the US Next Generation Weather Radar (NEXRAD) polarimetric observations (Paz et al., 2024). Therefore, PRO is able to provide a view of the vertical structure of clouds and precipitation that is complementary to that from other satellite platforms, along with the intrinsically collocated thermodynamic state of the atmosphere.
Applications taking advantage of PRO capabilities have been already investigated and proposed as missions (e.g., Cardellach et al., 2017; Turk et al., 2019, 2022), as well as for potential applications in numerical weather prediction (NWP) (e.g., Murphy et al., 2019), including efforts towards an operational forward operator to assimilate PRO observables into NWP models (Hotta et al., 2024). Furthermore, interest from commercial companies in the technique has already materialized, with the Spire Global Inc. launch of three PRO-capable nano-satellites in early 2023.
Considering the fact that the ROHP-PAZ experiment a was proof-of-concept mission and the first of its kind, the equipment on PAZ was not fully optimized to collect PRO. Besides the fact that the antenna is polarimetric (able to collect H and V information independently), no other major changes were made in the receiving system. For example, the two antenna cables coming out from the H and V ports use the receiver input ports that are usually reserved for the forward and aft antenna connections. Consequently, PAZ does not carry a forward antenna and therefore does not collect rising occultations. The most important consequence of this is that the measurements in the H and V ports are not synchronized in time, but there is a slight difference in the tracking start time.
This paper aims to describe a new dataset made available at the Institut de Ciències de l'Espai – Consejo Superior de Investigaciones Científicas (ICE-CSIC), Institut d'Estudis Espacials de Catalunys (IEEC), https://paz.ice.csic.es (last access: 4 December 2024), which contains both the polarimetric and the standard thermodynamic products to facilitate the scientific exploitation of the observations (Padullés et al., 2024). The dataset is called resPrf, arising from research profiles following the well-established University Corporation for Atmospheric Research (UCAR) naming for RO missions products. It also contains valuable information on the precipitation context of the observations that has also been shown to be important when available (e.g., Turk et al., 2021). Such information is provided already interpolated into the PRO ray paths and is made up of information from IMERG, from infrared TB, and from the different channels of the GPM radiometer constellation coincident observations (Kidd et al., 2021). These data products aim to foster the scientific use of PRO and to speed up the process towards their operational use.
The resPrf files are in netCDF-4 format (Unidata, 2023) and are organized into nested groups, variables, and global attributes. Such organization is shown in Table 1. The first group, called profiles, contains the vertical profiles of the polarimetric observable ΔΦ and of several thermodynamic variables, all interpolated into the same vertical grid from 0 to 40 km altitude every 0.1 km. The information contained in the thermodynamic variables comes from UCAR's wetPf2 retrieval products, disseminated here along with the polarimetric observables for user convenience. The second group, called rays, contains the ray-traced locations of the signal trajectory between the GPS satellite and PAZ, re-gridded and sub-sampled so that the tangent point of each ray corresponds to the same vertical grid as for the vertical profiles up to 20 km (i.e., resulting in 200 rays between 0 and 20 km), plus 20 more rays reaching up to 60 km at a lower vertical resolution.
Finally, the third group, called coll, contains the collocated data from IMERG precipitation surface rain rates and the infrared TB interpolated onto the ray locations. Also, this groups contains collocated passive microwave (PMW) brightness temperatures (TB) from each of the PMW radiometers that make up the GPM satellite constellation (Kidd et al., 2021). A more detailed description of all variables is provided in Appendix A.
Table 1Overall organization of the resPrf files. A detailed description of each group is provided in Appendix A.
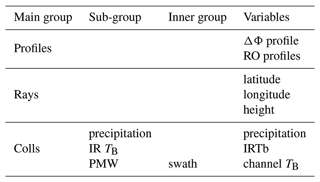
Besides describing the dataset, this paper also tackles a few other important aspects of the PRO data. The first one is to describe the methodology used to retrieve the standard thermodynamic products from the polarimetric observations, a process performed by UCAR. The quality of such retrievals is assessed since the use of a polarimetric antenna (instead of the RHCP one, which matches the emitted polarization) may result in a degraded quality of the signal. The second one is to describe the processing of the polarimetric observable and to assess its quality using the collocated precipitation information. Furthermore, derived products from the ΔΦ are also described, and a brief discussion about the horizontal resolution of the polarimetric observables is included.
The paper is organized as follows: it starts with a brief section highlighting the main aspects of the PRO technique. Sections 3, 4, and 5 describe the corresponding groups of the resPrf dataset, with details on the processing, interpolation, and collocations, respectively. Section 6 evaluates the retrievals and obtained products, such as the standard thermodynamic profiles and the ΔΦ. Further focus on the quality and applications of the ΔΦ is provided in the sub-sections within Sect. 6. Finally, Sect. 7 shows an illustrative example on how the provided ray trajectories can be used in conjunction with model outputs to mimic a 2D forward operator and simulate PRO observations. The paper ends with the conclusions. Furthermore, the Appendix contains detailed information about the data and metadata of the resPrf that would be useful for the users of the dataset.
The PRO technique was first proposed by Cardellach et al. (2014) as an extension of standard RO. The idea is to collect GNSS occulting (or rising) signals from a low Earth orbit (LEO) using a dual-polarized antenna (horizontal – H and vertical – V). The signals traveling from the GNSS satellite to the LEO cross different layers of the atmosphere, and the ray trajectories bend due to vertical refractive gradients as the radio link penetrates into the Earth's atmosphere (e.g., Kursinski et al., 1997). In the lowermost portion of the occultation event, electromagnetic (EM) signals travel nearly tangentially to the surface inside the troposphere, even reaching within the boundary layer (e.g., Sokolovskiy et al., 2006; Ao et al., 2012). Therefore, these rays are affected by precipitation if they encounter rain events along the ray paths.
The idea of PRO is to compare the phase measured at the H and V ports of the antennae to infer information about precipitation and clouds in addition to the traditional thermodynamic RO products obtained from their bending. Precipitating hydrometeors such as raindrops, snow, or frozen particles in clouds can be asymmetric and are usually horizontally oriented (e.g., Gong and Wu, 2017; Zeng et al., 2019). These induce a phase delay that is larger in the horizontal component than in the vertical component, and it can be measured by comparing the phase observations at the H and V ports of the antennae.
The observable representing the differential phase delay experienced by the EM-propagating wave per unit length is the specific differential phase shift (Kdp):
where D is the equivalent particle diameter, N(D) is the particle size distribution, fhh,vv represent the co-polar components of the scattering amplitude matrix f, and ℜ indicates its real part. λ is the wavelength (in this case, ∼0.19 m, corresponding to the L1 frequency band). It is worth noting that, in Eq. (1), an extra is included to express Kdp in units of mm km−1 to be consistent with the GNSS community and previous literature about PRO. Therefore, information about the distribution of sizes is provided through the N(D), and information about the asymmetry of the particles, their shape, and their density is contained in f.
The actual observable for PRO is then defined as the total differential phase shift, obtained for each observation time:
where ϕh,v is the phase of the EM wave measured at each port “h” or “v”, L is the length along the ray path under the influence of rain or clouds, and l is the ray path length position. In practice, Δϕ can be obtained using the excess phase observable (Φ) obtained through the standard RO processing of both H and V signals as if they were independent (see next section).
The first main group of the dataset is the profiles group. This contains the vertical profiles of Δϕ, as well as the standard RO thermodynamic retrievals from UCAR's wetPf2. All of them are interpolated into a gridded vertical coordinate between 0 and 40 km, with a resolution of 0.1 km.
3.1 Standard RO processing
The PAZ processing chain for standard RO products at the UCAR COSMIC Data Analysis and Archive Center (CDAAC) using combined H–V polarization is shown in Fig. 1. It starts by reading the data from the PAZ satellite, obtained either through Hisdesat (satellite operators) or through the data down-link at Fairbanks. These data come as IGOR binaries and are converted to opnGns at the CDAAC.
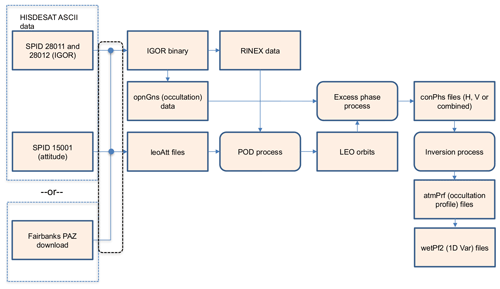
Figure 1PAZ data processing at UCAR/CDAAC. Custom H–V-combining software is included in the excess phase process.
Standard RO processing is done separately on the H and V polarization signals. The standard RO processing at UCAR/CDAAC follows these steps.
-
Start with high-rate occultation data in the opnGns format; opnGns is a custom data format defined at UCAR for quick and flexible storage of high-rate GNSS data as described in Weiss and Hunt (2022).
-
Remove orbital motion (LEO and GNSS POD data), GNSS clocks (e.g., IGS products), and LEO clocks (via differencing with a high-elevation “reference” satellite) to create an excess phase profile.
-
Compute an atmospheric Doppler model from climatology. This model is constructed using the LEO and GPS satellite positions and velocities and a climate model. It gives the expected change in signal phase due to the neutral atmosphere.
-
Integrate this model to get a phase model and then difference it with the excess phase computed above.
-
This phase angle Δθ between successive samples is now rotating slowly enough to generate meaningful I and Q components: (where SNR denotes the signal-to-noise ratio).
-
Apply GPS navigation bits to the open-loop portion of I and Q.
-
Stitch open- and closed-loop Is and Qs together.
-
Compute phase via atan2(Q,I).
-
Fix full-cycle slips by adding or subtracting 2π to minimize the difference between samples.
-
Add the phase model back in to get connected excess phase.
-
These connected L1 and L2 phase profiles are then submitted to the inversion process to compute bending angle; refractivity; and, finally, temperature and pressure profiles. This same inversion process is used by all CDAAC missions (Sokolovskiy, 2014).
-
Finally, the dry atmospheric temperature and pressure profiles are combined optimally with ECMWF weather model data in a 1D variational assimilation process (Wee et al., 2022).
The resultant H and V profiles are nearly identical, but the SNR and penetration depth can be enhanced by combining them at the excess phase level via a vector sum of the I and Q data. Here is the procedure:
-
Determine a “primary” polarization for this occultation. We use a higher SNR to choose between H and V.
-
Compute separate horizontal and vertical I and Q profiles, as in the single-polarization procedure outlined above.
-
Determine the phase alignment between H and V. For PAZ, the H and V polarization data use separate antenna channels. Thus, H and V phases have slightly different time stamps and can be out of phase alignment.
-
Line the Is and Qs up and use the primary polarization to fix cycle slips in the “secondary” polarization.
-
Find the point at which the secondary polarization signal descends into noise. This is the point at which to stop the vector combination and continue with only the primary polarization.
-
Perform a vector sum of the lined up I and Q values from the primary and secondary: .
-
Assemble the combined excess phase (as in steps 9–10 in the single-polarization processing).
-
Compute the SNR of the combined signal as . This assumes similar random noise on H and V channels.
The resultant H–V-combined profiles have higher SNR than either the H- or V-only profiles and penetrate slightly deeper into the atmosphere. In addition, the count of successful occultations when combining H and V is higher than for H or V only.
3.2 Polarimetric processing
The polarimetric processing is conducted at the ICE-CSIC, IEEC. The complete processing chain is shown in Fig. 2. The algorithm to process these data, which is detailed here, expands upon what was described in Padullés et al. (2020).
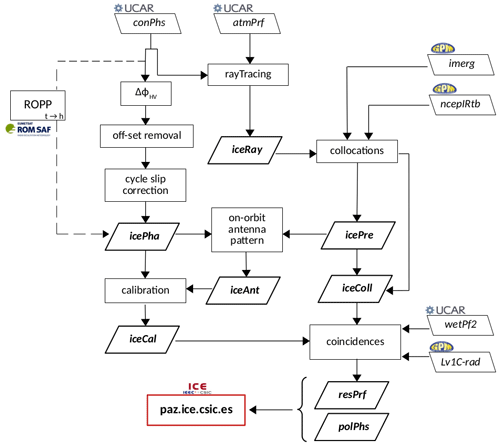
Figure 2Processing chain implemented at ICE-CSIC, IEEC, to generate the resPrf profiles. Input file types are represented as left-tilted rectangles, while output files and intermediate files generated at ICE-CSIC, IEEC, are represented as right-tilted bold rectangles. Processing steps are represented as regular rectangles. See the text for more details on each of the steps.
The processing of the polarimetric part starts with the conPhs files provided by UCAR (UCAR-COSMIC-Program, 2023a). These files contain the RO observables as a function of time, with the only difference being that, in the PAZ case, most variables are provided for each polarization (e.g., SNRH,V, excess phaseH,V). At this point, the first important step is already done by UCAR, that is, to interpolate both H and V observables to the same time stamp. This enables the straightforward differencing of the H and V excess phases to obtain the main PRO observable:
where Φ is the L1 excess phase in each polarization, and t is time. Even though RHCP should have a ΔΦ=90° since the observables used are the excess phases, the differentiation yields an arbitrary number that can be set to 0 in the higher layers of the atmosphere, where no precipitation is expected. Therefore, the rest of the ΔΦ(t) observation down below is relative to that initial value.
In order to obtain a height (h) linked to each time measurement, a modified version of the Radio Occultation Processing Package (ROPP, Culverwell et al., 2015) is used. The link between t and h is based on geometric optics, with all the limitations and consequences it may have: for example, under a strong atmospheric multipath, a large ambiguity is expected in associating a single h with a t measure since different rays may arrive at the receiver at the same time with different excess Doppler values. Estimations performed by Padullés et al. (2020) predicted an uncertainty of more than 0.5 km below 2 km altitude. Hence, altitude assignments for heights below 2 km are not to be fully trusted. Ideally, ΔΦ should be obtained through retrievals using wave optics, and work towards this is being pursued (e.g., Wang et al., 2021). Wave optics aims to disentangle the ambiguity of multi-valued time series using, e.g., radio-holographic techniques that yield one-to-one relationships between excess Doppler and impact parameters under the assumption of spherical symmetry.
After the differentiation of the ΦH,V, jumps in the Φ compatible with uncorrected cycle slips become noticeable. To correct for those, half-cycle correction is applied to the closed-loop (CL) portion of the profile, and full-cycle correction is applied to the open-loop (OL) portion (Padullés et al., 2020, Sect. 2.1). These corrected profiles are stored in internal (ICE-CSIC, IEEC) intermediate files called icePha. Those files obtained under no-rain conditions are used to build the on-orbit antenna pattern, which is used to calibrate the whole dataset (Padullés et al., 2020, Sect. 5). This calibration should also remove any effect induced by the ionosphere on ΔΦ (Padullés et al., 2020, Sect. 5). The precipitation information is stored in the intermediate files called icePre and iceColl, which are described in Sect. 5. The calibrated phase is also smoothed using a 1 s smoothing filter. The final calibrated and smoothed ΔΦ is the main PRO observable, and this is stored in the intermediate files called iceCal and, later into the processing, is copied into the resPrf. The ΔΦ provided in the resPrf is re-gridded so that it corresponds to the same height as the thermodynamic retrievals (from 0 to 40 km, every 0.1 km).
3.2.1 Height flag indication
An important attribute included in the resPrf is the height_flag. This is a parameter that aims to indicate under which height the ΔΦ is not trusted anymore in terms of the quality of the ΔΦ itself. It identifies jumps in the ΔΦ profile that were not corrected during the cycle slip correction, often associated with tracking issues and low SNR or uncorrected cycle slips that were not properly identified as such.
To perform the identification of altitudes where ΔΦ is no longer trustable, three conditions must be met by the data:
-
A first sliding window of 50 points is applied to the corrected 50 Hz ΔΦ data, where the standard deviation is computed (SD1). This SD1 must exceed a threshold of 10 mm, which would mean that a jump in the ΔΦ is detected. Such jumps may be due to noisy isolated measurements, and ΔΦ may recover rapidly. To ensure that is not the case, another condition must be met.
-
Another sliding window of 50 points is then applied to the calibrated and smoothed ΔΦ profiles, and the standard deviation is computed (SD2). Here, the threshold that SD2 must exceed is 1.5 mm. This indicates that, after smoothing, there are still some remaining issues in ΔΦ. If condition (1) above were to be met due to isolated noisy measurements, the smoothing would result in a SD2 lower than the specified threshold.
-
Finally, must exceed an arbitrary threshold of 0.4. This relative magnitude discards increases in the SD2 that are due to a sudden increase in ΔΦ caused by rapidly entering into a heavy-precipitation area.
If all three of the conditions above are met simultaneously, the height at which this happens is stored in the height_flag parameter. The profiles are considered to have good quality above that height, but users are advised against using the ΔΦ below height_flag.
The RO technique is a limb-sounding measurement. Despite the fact that, often, RO retrievals are associated with a single point location (known as the occultation point), the truth is that a larger portion of the atmosphere is contributing to the bending angle and, therefore, to refractivity and derived products. In the case of standard RO, the contribution is maximized around the tangent point, with an effective horizontal resolution of ∼100–150 km in the direction along the rays (e.g., Anthes, 2011).
For PRO, the contribution is not maximized anywhere along the ray paths; therefore, the contributions from all areas crossed by the rays must be taken into account equally. That is why an indicative representation of all ray path locations is very helpful to better interpret and understand the PRO measurements.
The resPrf files have two groups storing variables in this regard. The first one is the group rays, which contains the locations (latitude, longitude, and height) of the points of the selected rays. The group colls contains the external precipitation information interpolated into these ray point locations. Further details are provided in the subsequent subsections.
4.1 Ray tracing and interpolation
Ray-tracing software is used to simulate the actual ray path trajectories between the GPS and PAZ satellites at each time stamp of the occultation. To properly account for bending of the rays, the retrieved refractivity profile is used; therefore, ray-path trajectories are only available for those profiles whose corresponding UCAR atmPrf profile is available and passed the quality control. Each ray is computed by solving the geometrical optics equations accounting for the time-dependent locations of the satellites. Therefore, the resulting collection of rays is a slant plane that realistically represents the tangent point drift due to the relative movement between the satellites. However, since the refractivity profile is 1D, the effects of horizontal inhomogeneities are not taken into account.
Once all ray trajectories are obtained, these are sub-sampled and re-gridded so that the tangent points coincide with the vertical coordinate and resolution of the thermodynamic and ΔΦ profiles described above: that is, 200 rays are provided with tangent points between 0 and 20 km, each one 0.1 km apart. In addition, 20 more rays are included, with tangent points reaching up to 60 km and with a lower vertical resolution. Each of the 220 rays contains 301 points in the along-ray dimension, implying an along-ray resolution of about 5 km for the portion of the rays below 20 km. The interpolation of all the data available (those further explained in Sect. 5) is performed using the nearest-neighbor method, using the latitude and longitude of the rays and discarding the height dimension (since the interpolated data are 2D).
An example of the traced rays can be seen in Fig. 3, where the portion of the rays below 20 km is shown projected onto the surface, with different background images in each panel. This case corresponds to a coincidence between PAZ and the Defense Meteorological Satellite Program (DMSP) F17 satellite, where the IMERG and IR data were also available. The different background images in Fig. 3 correspond to surface rain rate, IR TB, and four different channels of the DMSP F17 PMW radiometer. More details about these collocations are provided in the following sections.
The user should become familiar with the moon slice shape of these ray projections. The longer rays correspond to rays with their tangent points at lower altitudes. As the rays' tangent points increase in altitude, a smaller portion of the rays lie below 20 km; therefore, these rays appear to be shorter in these projection maps.
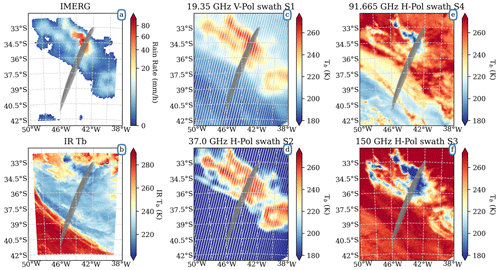
Figure 3Example of a collocation of a PAZ observation with ID no. PAZ1.2019.126.09.11.G12 using IMERG (a), IR TB (b), and different channels of the PMW radiometer SSMIS aboard DMSP F17, as indicated at the top of each panel. The gray zone represents the projection on the surface of the PRO ray portions below 20 km.
5.1 IMERG
The GPM IMERG precipitation products provide surface rain rates with global coverage, with a horizontal resolution of 0.1° and a temporal resolution of 30 min. Being a two-dimensional product, the interpolation is performed into the projection onto the surface of the PRO rays, regardless of the height dimension. A limitation is placed based on height: only the portion of the rays below 20 km is used since it is conservatively assumed that no precipitation reaches those heights.
Despite some known limitations (e.g., Watters et al., 2023; Li et al., 2022; Peinó et al., 2022; Ramadhan et al., 2022), the use of IMERG is beneficial for validating PRO observations thanks to its global coverage and temporal resolution. The IMERG data interpolated into the rays are provided in a sub-group called precipitation within the colls parent group. The rain rate at each ray point location is stored in the variable precipitation, and the group also has several attributes like the average rain rate around the occultation point.
5.2 Tb11
Like IMERG, the merged IR 10.8 µm TB product offers global coverage (±60° latitude) and a 30 min temporal resolution, with a horizontal resolution of ∼4 km. Due to the sensitivity of PRO to frozen hydrometeors and, to some extent, to the cloud structure, the use of this product helps identify the portions of the rays within clouds assuming that IR TB is informative regarding the cloud top heights. The IR TB is interpolated into the rays (also, only to the lat–long projection of the rays; no height is taken into account), and these are provided in a sub-group called IRTb within the colls parent group. The TB at the latitude and longitude of each ray point is stored in the variable IRTb, which also contains a few attributes providing additional information, like the minimum IR TB around the occultation point.
Also, this product is used to determine the cloud top height (CTH) of the clouds crossed by the PRO rays. For such determination, the coldest brightness temperature is matched with the retrieved temperature (T) vertical profile from PAZ, and the height at which both coincide is determined. Similarly, assuming that the temperature profile is valid not only at the occultation point but also in the whole area sensed by the rays, one can determine whether each ray point is below or above the corresponding cloud height by comparing both temperatures. Here, the height dimension of the ray is taken into account so that the atmospheric temperature profile is linked to each height, regardless of latitude and longitude. An example of this is shown in Fig. 4. Panel (a) shows the IR TB interpolated into the portion of each of the rays below 20 km. The representation shows the along-ray dimension on the x axis and the tangent point height linked to each ray in the y dimension. Then, the atmospheric T from each ray point (only based on height) is compared against the corresponding IR TB at the same point: if the atmospheric T is colder than IR TB, that point is considered to be above the clouds, i.e., outside the cloud. Otherwise, it is considered to be inside (or below) the cloud. The edge of the rays inside the clouds is shown with a green contour overlaid onto Fig. 4a and b. This is further exploited in Sect. 6.2.2.
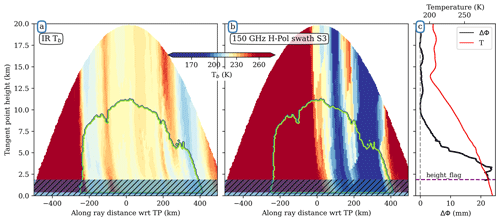
Figure 4Example of the result of the interpolation of the IR TB (a) and the 150 GHz TB from the F17 satellite (b) as function of the along-ray distance with respect to the tangent point (x axis) and the tangent point height of each ray (y axis). This example corresponds to the same case as in Fig. 3. The green contour indicates the portion of the rays inside clouds according to the procedure explained in Sect. 5.2. Panel (c) contains the vertical profiles of ΔΦ (black, bottom x axis) and atmospheric temperature (red, top x axis) as a function of height (shared axis with tangent point height from panels a and b). The hatched area in panels (a) and (b) and the dashed purple line in panel (c) indicate the height of the height_flag parameter.
5.3 Space-based radiometers
Each PAZ observation is checked against overpasses of the GPM constellation satellites carrying PMW radiometers. The time constraint for the coincidence is set to 30 min, and the distance is constrained by the size of each radiometer's swath: that is, the occultation point of the PAZ observation must be within the swath. The list of satellites with the corresponding sensor, swath widths, number of coincidences with PAZ (until 1 August 2023), and percentage of coincidences within the tropics is provided in Table 2. The number of coincidences scales almost linearly with the time allowed to consider a coincidence; e.g., when reduced to 15 min, the number of coincidences drops by 50 %–55 %. An example of a coincidence with the DMSP F17 satellite is shown in Fig. 3, where four PMW channels are selected (e.g., 19.35, 37.0, 91.665, and 150 GHz).
Table 2List of satellites with passive microwave radiometers that have coincidences in space and time with PAZ observations from 10 May 2018 until 1 August 2023. The number of coincidences and the percentage of them in the tropics are determined by the orbit of the corresponding satellite and its orbit local time of the ascending node. Note that PAZ is in a 0930/1830 orbit. More detailed information can be obtained from Turk et al. (2021). The acronyms read as follows: GPM refers to global precipitation measurement, GCOM-W1 refers to the Global Change Observing Mission for Water, NPP refers to the National Polar Orbiting Partnership, GMI refers to the GPM microwave imager, SSMIS refers to the Special Sensor Microwave Imager/Sounder, AMSR refers to the Advanced Microwave Scanning Radiometer, ATMS refers to the Advanced Technology Microwave Sounder, MHS refers to the Microwave Humidity Sounder, and SAPHIR refers to the Sounder for Probing Vertical Profiles of Humidity.
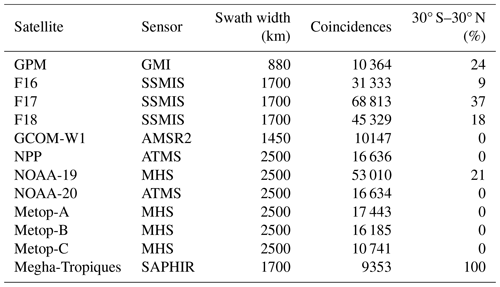
For the cases that have a collocation with one of these PMW radiometers, the TB corresponding to all channels of the radiometer is interpolated into the PRO rays. The interpolation results for the 150 GHz channel of the case example illustrated in Fig. 3 are shown in Fig. 4b. These data are provided in sub-groups named after the collocated satellite (e.g., F17, GPM-GMI, NOAA-19) within the colls parent group. Each sub-group contains an inner group corresponding to the swath mode, and each of these swath-related groups contains the data for each radiometer channel in the corresponding variables. The frequency and polarization of each variable are provided in the corresponding attributes. The view angle from cross-track-scanning PMW instruments has not been taken into account in this version of the data, and this information is going to be included in the next re-processing.
The dataset contains the standard RO retrievals and the calibrated ΔΦ. The following sub-sections are dedicated to the validation of these products.
6.1 Standard thermodynamic products
One of the challenges of the PRO technique is its ability to provide good-quality thermodynamic (or standard) retrievals with an antenna performing with a −3 dB loss. This is due to the fact that each linear polarization captures half of the power due to the polarization mismatch (with respect to a circularly polarized antenna). This could have implications for the penetration depth and quality of retrievals, especially in the lower troposphere. To quantify this effect, comparisons have been performed with TerraSAR-X (hereafter, TSX) RO observations. TSX is a rather similar satellite platform carrying a standard RO antenna and the same RO receiver as PAZ (i.e., an IGOR+, a modified version of the one used by the COSMIC-1 satellites, manufactured by Broad Reach Engineering), orbiting at the same height and same orbit inclination of 97.4° (Beyerle et al., 2011). The antenna in PAZ is similar to the one in TSX, both manufactured by Haigh-Farr, and is only modified in PAZ to capture H and V polarizations instead of RHCP.
Therefore, comparisons of PAZ PRO observations with TSX RO provide a suitable benchmark to quantify the compromise that PRO undergoes for providing precipitation. The data used for the comparison are refractivity retrievals from UCAR atmPrf and the corresponding collocated echPrf for the comparison with ECMWF model (UCAR-COSMIC-Program, 2023b).
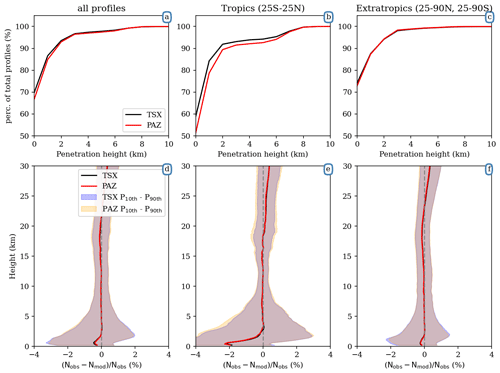
Figure 5Penetration depth and O–B comparison between TSX and PAZ refractivity retrievals N with respect to the ECMWF model for all data between 1 January 2019 and 30 June 2019. Panel (a) corresponds to the percentage of total profiles (y axis) reaching a certain penetration height (x axis) for all retrieved data. Panel (b) shows the same as panel (a) but for observations obtained in the tropics (25° S–25° N), and panel (c) shows the corresponding results for extratropical cases (25–90° S, 25–90° N). Panel (d) corresponds to the fractional differences (x axis) as a function of height (y axis) for all retrieved data. Panel (e) shows the same as panel (d) but for observations obtained in the tropics (25° S–25° N), and panel (f) shows the corresponding results for extratropical cases (25–90° S, 25–90° N). The shaded area represents the 10th to 90th percentiles of the distribution of fractional differences as a function of height.
The first thing being compared is the penetration depth. The results for the minimum height at which refractivity is being retrieved for both PAZ and TSX at UCAR CDAAC is shown in Fig. 5a–c. The figure shows the result of the percentage of profiles reaching a certain height for all PAZ and TSX observations during the first half of 2020, separated by tropical and extratropical observations. It can be seen how the penetration depth is slightly better for TSX, a difference that is larger in the tropics. In the tropics, below 2 km, the difference between TSX and PAZ is around 2.5 %, and below 1 km, it is around 4 %, increasing with decreasing altitude. Outside the tropics, the difference is negligible.
Regarding the quality of the profiles, the comparison is performed using the ECMWF model background as the reference. All TSX and PAZ refractivity profiles obtained during the first half of 2020 are compared with the refractivity obtained from the collocated ECMWF model (echPrf). The fractional refractivity difference, i.e., (), as a function of height is shown in Fig. 5d–f. No significant differences can be observed in the comparison, indicating that the quality of the retrievals is equivalent.
6.2 Polarimetric products: differential phase shift
The method used to evaluate ΔΦ is to group these profiles based on the precipitation under which they were obtained, and the results are shown in Fig. 6. The first thing to look at is the group with no precipitation. Such a group contains those profiles where the collocated IMERG precipitation is PIMERG=0 mm h−1 and should have a mean ΔΦ(h) close to 0 mm for the whole vertical profile. This is shown in Fig. 6-(right) with the solid black line. It can be seen how the solid black line follows the 0 mm line very closely. Also, Fig. 6 (left) shows the vertical profile of the standard deviation (SDΔΦ) of the group corresponding to PIMERG=0 mm h−1. It can be seen that the standard deviation is very small (e.g., SDΔΦ<0.3 mm) and that it increases with decreasing altitude. It reaches ∼1.5 mm at an approximate altitude of 2 km, which agrees very well with previous studies (e.g., Cardellach et al., 2014; Padullés et al., 2020). When precipitation increases, the corresponding group mean ΔΦ increases as well. This also corroborates previous studies (e.g., Cardellach et al., 2019; Padullés et al., 2020) and implies that ΔΦ is sensitive to precipitation intensity. Note that PIMERG used here represents an average of all rain rates interpolated into the rays (accounting only for the portion of the rays below 6 km). Since this may cover a relatively large area, values of the order of 2 mm h−1 are already representative of substantial precipitation.
It is also worthwhile noting the shape of the profiles obtained under heavy precipitation (i.e., roughly when PIMERG>2 mm h−1). At this point, it is noticeable how the maximum of the ΔΦ is achieved at altitudes around 5 km or higher. Even though, here, it is shown with the mean ΔΦ profiles in the corresponding precipitation groups, this feature is clear in individual profiles obtained under heavy precipitation. There are two main reasons behind this shape: (1) the first one is that the PRO observable ΔΦ is sensitive to frozen hydrometeors as long as they are horizontally oriented (e.g., Padullés et al., 2022, 2023), and these are placed above the freezing level; (2)the second reason is purely geometrical, namely that the PRO rays with tangent points around 5 or 6 km are traveling a long distance within clouds (rather spatially homogeneous), whereas the lower rays are crossing a greater portion of precipitation that is, in turn, more spatially inhomogeneous. Therefore, the contribution to ΔΦ is maximized at these heights.
At the time of writing, the main hypothesis was that oriented snow is the major contributor to the ΔΦ observable (e.g., Padullés et al., 2022; Hotta et al., 2024). Besides the scattering properties of such hydrometeors, their contribution is also favored by the fact that the uncertainty of the PRO measurement around ∼4–6 km is already very low and, therefore, much better than below 3 km, where the effect of liquid precipitation is maximized. Furthermore, at the lowest heights, there could be a certain ambiguity coming from atmospheric multipath due to the use of geometric optics to obtain the link between time and height (Padullés et al., 2020).
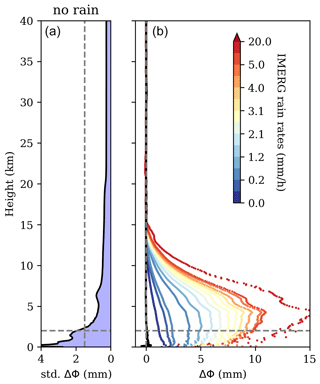
Figure 6Statistics for the ΔΦ profiles. Panel (a) shows the standard deviation as function of the tangent height of the group of ΔΦ obtained when PIMERG=0 mm h−1, representing the free-of-rain cases. Panel (b) shows the average as a function of height of the ΔΦ grouped by PIMERG when exceeding a certain value indicated in the color bar. The black line is the average for PIMERG=0 mm h−1. The horizontal dashed line indicates the 2 km height, and the vertical dashed line indicates the 1.5 mm ΔΦ standard deviation, which has been taken as a reference in previous works (e.g., Cardellach et al., 2014).
6.2.1 Polarimetric products: top of the signal
The fact that ΔΦ is sensitive to frozen hydrometeors and the fact that these are horizontally oriented globally and for different types of clouds (e.g., Gong and Wu, 2017; Zeng et al., 2019; Padullés et al., 2023) imply that the ΔΦ signature should have a relationship with the tops of the clouds. Being a measurement at the L band, it is expected that sensitivity to clouds starts below the cloud top height (as inferred from the infrared, for example). Therefore, here, the relationship between the top of the ΔΦ signal and the CTH around the observation (obtained using the IR 10.8 µm TB) is investigated.
First of all, the CTH is derived by matching the minimum IR 10.8 µm TB around the occultation point (inside a circle of 2° in diameter) with the retrieved vertical temperature profile. The height at which the temperatures match is identified as the IR-derived CTH. This value is stored in the cth_irT2deg parameter. This methodology has some caveats, such as the fact that it can fail for thin clouds or multilayered clouds, large temperature perturbations (like those induced by gravity waves), etc. However, it provides a rough estimation of the CTH that is reliable, especially in the tropics.
The top of the signal (TOS) is derived solely using the vertical ΔΦ profile. First, a mean (mΔΦ) and standard deviation (SDΔΦ) of the free-of-clouds portion of the profile, that is, above potential clouds, are obtained. This portion is defined to be between 18 and 30 km, and it is where mΔΦ and SDΔΦ are computed. Then, a threshold is defined as . Once this threshold is obtained, ΔΦ values exceeding thresh are identified. Starting from the top, the first five consecutive measurements where ΔΦ(h)>thresh are identified. TOS is then defined at the height of the first of such five consecutive measurements. The height at which this happens is stored in the deltaphi_top_height parameter. If the condition is not met, deltaphi_top_height is set to 0.1 km.
Obtained TOS values are compared with IR-derived CTH for those cases where TOS >0.1 km, and the results are shown in Fig. 7a using a density plot. In addition, the average TOS per CTH bin and the associated standard deviation are provided as well. It can be seen how, even though there exists a robust relationship showing an increase in TOS as the IR-derived CTH increases, there also exists a region (i.e., large CTH with low TOS) that separates the relationship from the perfect match. This issue is linked mainly to a geometry problem: the IR-derived CTH may be far from the tangent point.
To account for the geometry, the IR-derived CTH is now substituted by the highest point of the PRO rays that are considered to be inside the cloud, as explained in Sect. 5.2. This is an equivalent procedure that accounts for the actual ray trajectories and not only for the immediate environment. The comparison of the TOS with the IR-derived CTH when the geometry is accounted for is shown in Fig. 7b. Now the relationship is much closer to the perfect 1:1 match than the results shown in panel (a).
These results have two important implications. The first one is that the agreement between the TOS and IR-derived CTH demonstrates the sensitivity of the PRO ΔΦ to clouds. The relationship between TOS and CTH is linear, with a bias of around ∼2 km, meaning that the sensitivity is larger at the infrared than at the L band, something totally expected. Furthermore, a larger percentage of horizontally oriented hydrometeors is expected in the anvils and in the stratiform region than in the convective cores (e.g., Gong and Wu, 2017), which could also contribute to the bias. The second implication of the results in Fig. 7 is that it is very important to account for the actual trajectories and the context when interpreting the PRO ΔΦ observable. This is something similarly stated by Hotta et al. (2024), where the authors determine that the use of a 2D forward operator is crucial and that a 1D forward operator would fail to properly use ΔΦ for NWP experiments.
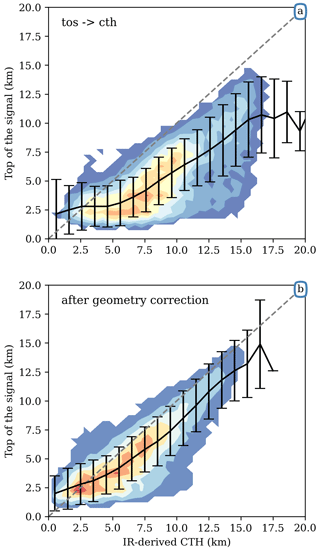
Figure 7Density plot (cold colors indicate fewer data, while warm colors indicate more data) comparing the ΔΦ top of the signal (TOS) (y axis) and the IR-derived cloud top height (CTH) (x axis) when the minimum IR TB around the occultation point is used (a) and when the geometry of the actual ray trajectories are taken into account (b). The black line represents the average, and the error bars represent the standard deviation of the TOS within each 1 km CTH bin.
6.2.2 Polarimetric products: horizontal resolution
The main challenge of the PRO ΔΦ observable is its horizontal resolution. Unlike the standard RO level-2 products, where the contribution is maximized around the tangent point, in the PRO case, the hydrometeors contribute equally all along the ray paths. Therefore, the horizontal resolution is the length the rays travel below the height at which one would expect to find clouds. Table 3 shows the effective horizontal resolution of rays with different tangent point heights depending on which height one assumes the CTH is at.
Table 3Effective horizontal resolution (in km) of a ray with a certain tangent point height depending on where the CTH is assumed to be.
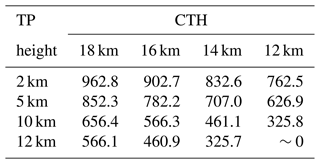
An interesting feature is that the horizontal resolution depends on height, and, therefore, different height points on the ΔΦ(h) profile have different effective horizontal resolutions. In fact, the resolution improves with increasing altitude and becomes really small when approaching the CTH. Based on the results in the previous section (Sect. 6.2.1), instead of the CTH, the TOS could be used to determine the height at which one starts to expect clouds. Another important characteristic is that the effective resolution determined by the length traveled by the rays below a certain height only provides an upper limit to the resolution, but the actual horizontal resolution is likely to be less than that.
In the cases where precipitation information like what is described in Sect. 5 is available, the actual horizontal resolution can be determined using the interpolated data. For example, in the case illustrated in Fig. 4, one can see that the CTH accounting for the geometry of the rays is approximately 11 km. This would imply an upper limit on the horizontal resolution of almost 600 km for a ray with a tangent point of 5 km. However, using the portion of the rays that are within the cloud (according to the interpolated IR TB and the retrieved temperature profile; see Sect. 5.2), it can be determined that the actual horizontal resolution is ∼540 km, as can be inferred from the green contour in Fig. 4a.
This methodology could be pushed further by using the interpolated PMW data and setting a constraint on the TB to identify portions of the cloud that are not only crossed by rays but that are actually contributing to ΔΦ. Only as an illustrative example, in the case of Fig. 4, one could assume that the only portion of the cloud that contributes to ΔΦ is the one where TB from the 150 GHz channel is below 220 K. Then, the horizontal resolution of the ray with a tangent point height of 5 km would drop to ∼300 km, as can be inferred from Fig. 4b.
This section aims to illustrate the potential use of the ray path trajectories that are provided in the resPrf files. With them, it is straightforward to know the actual locations of the rays and to use them as an input for a 2D forward operator in combination with model outputs with the aim of simulating ΔΦ profiles.
Figure 8 shows an example of the utilization of the ray path trajectories. For this illustrative case, the corresponding ERA5 reanalysis (Hersbach et al., 2020, 2023) fields are used. The fields of interest to simulate ΔΦ are the ones containing information about hydrometeors, that is, the mass of water, snow, cloud ice, and cloud liquid particles. These quantities are obtained in the fields specific rain water content, specific snow water content, specific cloud ice water content, and specific cloud liquid water content, respectively (Hersbach et al., 2023). It must be noted that these hydrometeor fields represent the average within each grid box and are obtained from the large-scale clouds generated by the cloud scheme in the ECMWF Integrated Forecasting System (IFS).
The specific water contents are converted into water content (WC) densities. These are obtained at each point of the model grid and are then interpolated into all the ray trajectories crossing that region (which are provided in the resPrf files). WC values are converted to Kdp following the same approach as in Padullés et al. (2022) and Hotta et al. (2024), that is, using a simple approximation that depends on the effective density (ρ) of the hydrometeor, the axis ratio (ar), and the amount of hydrometeors, e.g.,
where i denotes each of the hydrometeors under consideration. For each ray, is integrated along the whole ray path to obtain ΔΦi.
This is exemplified in Fig. 8. The maximum WC over the vertical column corresponding to each model grid in the whole surrounding area of the PRO event is shown in panel (a), while the interpolation of the WC corresponding to different hydrometeors into the ray trajectories is shown in panels (b), (c), and (d). The result of the integration, and therefore ΔΦi, is shown in panel (e) in comparison with the PAZ ΔΦ observation. For this case, a fixed ρ of 200 kg m−3 is being used for the frozen hydrometeors, and an axis ratio of 0.5, 0.8, and 0.7 is being used for snow, ice, and rain, respectively. The results show a good agreement between the PAZ ΔΦ and the simulated ΔΦ and emphasizes the contribution of snow. However, it must be emphasized that ERA5 does not provide the hydrometeor water content associated with cumulus parameterization, and, therefore, model underestimation is to be expected.
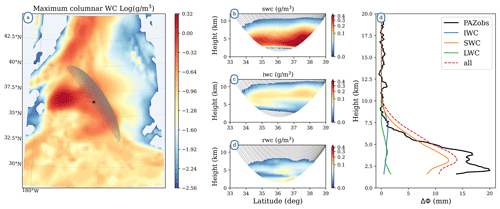
Figure 8Collocation of PAZ observation with ID no. PAZ1.2020.355.16.46.G19 and the corresponding ERA5 water content fields. Panel (a) shows the projection of the PRO ray trajectories (only portion of the rays below 20 km) on the surface, with the color background indicating the maximum columnar water content from ERA5. Panels (b), (c), and (d) show the WC fields interpolated into the ray trajectories provided by the resPrf files accounting for the 3D information for snow, ice, and rain, respectively. Panel (e) shows the ΔΦi for all considered hydrometeors, along with the total sum for all hydrometeors (dashed) and the actual PAZ observation (black).
More importantly, this example shows a straightforward way to use freely available data (e.g., resPrf and ERA5) to perform comparative analyses of ΔΦ with model outputs. The ray trajectories contained in resPrf and the relationships between Kdp and WC (those provided here and within the aforementioned references or more complex ones currently being investigated) represent a basic 2D forward operator that could be used by any user. Such a basic forward operator aims to contribute to the development of PRO data assimilation strategies into NWP systems. Similarly, PRO characteristics make this measurement technique potentially useful in the assessment of model performance, such as in microphysics schemes discrimination or scattering parameterization evaluation, among others.
The described dataset resPrf is available at https://paz.ice.csic.es/ (last access: 1 December 2024) (https://doi.org/10.20350/digitalCSIC/16137, Padullés et al., 2024). The corresponding atmPrf and wetPf2 profiles are available at https://data.cosmic.ucar.edu/gnss-ro/paz/postProc/ (last access: 1 December 2024) (https://doi.org/10.5065/k9vg-t494, UCAR-COSMIC-Program, 2023a). The TerraSAR-X data used in Sect. 6.1 are available at https://data.cosmic.ucar.edu/gnss-ro/tsx/postProc/ (last access: 1 December 2024) (https://doi.org/10.5065/fv7s-ax27, UCAR-COSMIC-Program, 2023b). The IMERG dataset (https://doi.org/10.5067/GPM/IMERG/3B-HH/07, Huffman et al., 2023), the merged IR TB products (https://doi.org/10.5067/P4HZB9N27EKU, Janowiak et al., 2017), and the PMW files (see DOIs in Table B1) are available at the NASA GES DISC (https://disc.gsfc.nasa.gov/, last access: 1 December 2024).
The resPrf dataset is created and publicly disseminated with the aim of fostering the scientific use of the PRO observations. These provide unique observations of the vertical structure of clouds and precipitation with intrinsically collocated thermodynamic soundings of the atmosphere. Since 2018, the vertical profiles of ΔΦ have been publicly provided in the polPhs files. Now, these and the future PAZ GNSS PRO observations have been reprocessed, and their output files have been expanded: the new dataset also contains the specific locations describing the full sounded slant plane, with external data interpolated into it. These external data consist of rain rate retrievals from the IMERG, IR TB observations, and radiances from PMW radiometers. These data are all provided through netCDF-4 files organized into nested groups, variables, and corresponding attributes, making the data rather self-explanatory.
The procedure to combine the H and V observations from PRO and obtained standard RO products is described in detail in Sect. 3.1. The description provides all steps so that the methodology can be reproduced and applied to other PRO missions. The obtained products have been shown to have a quality equivalent to that of the retrievals from the TerraSAR-X mission, a very similar satellite platform collecting standard ROs. However, in the results shown here, the quality of PAZ profiles is slightly lower in the tropics. Very recently, Spire Global has shown results of a similar approach (i.e., comparing standard RO retrievals with those obtained from PRO observations after combining H and V), and the quality of the combined H and V retrievals is even better than their regular standard RO retrievals (e.g., presentations in Turk et al., 2024). This implies that the results from PAZ may be affected by the fact that the PRO equipment was not fully optimized and the fact that there are artificial artifacts affecting the antenna surroundings (Padullés et al., 2020). Also, the Spire Global antennae designs, being different from those in PAZ, may play a role. Nevertheless, both sets of results encourage the use of PRO, provided that, in addition to equivalent-like standard thermodynamic products, one also obtains the precipitation information.
The description of the polarimetric processing chain is provided in Sect. 3.2. Furthermore, a detailed methodology to detect spurious portions of the ΔΦ vertical profile is provided and labeled as height_flag. The resulting ΔΦ profiles are validated using collocations with IMERG, showing results that agree with previous validation studies and that further indicate the sensitivity of PRO to precipitation intensity and to oriented frozen hydrometeors.
All the collocations from IMERG, IR TB, and PMW radiometers provide additional information on the context of the observations. In addition, these emphasize the complementarity of the limb-sounding-like observations from PRO, providing additional insight into the vertical perspective on 2D observations. This may be of special importance in complementing the observations of PMW radiometers with polarized measurements (i.e., GMI, F17) at high frequencies (e.g., >90 GHz), with vertical information on oriented hydrometeors inducing strong signals. At the same time, these collocations provide additional validation of the PRO products, as has been shown with the ΔΦ top-of-the-signal comparison with the CTH derived from IR TB.
It has been also shown how collocated data can help tackle the issue of horizontal resolution in PRO. Theoretically, the horizontal resolution of ΔΦ can be as large as the ray length, constrained within the portion traveling under the potential height of cloud tops. Using external information such as the IR TB or the PMW radiances, the effective horizontal resolution can be further constrained. Although using external information is not always desirable, products like the merged IR TB are (almost) always available within ±60° latitude and can be used routinely to constrain the upper limit of the ΔΦ horizontal resolution. Horizontal resolution can represent an issue for scientific applications, and, therefore, these kinds of solutions are worth being explored. However, in the operational use of these observations, since the use of a 2D forward operator stands as a requirement (Hotta et al., 2024), the horizontal resolution becomes less of an issue.
This fact is illustrated in Fig. 8. Besides the actual comparison between the PAZ observation and the ERA5-derived ΔΦ that has been shown, this example serves to highlight how easy is to use the ray trajectories contained in the resPrf files to perform simulations of ΔΦ. This could be of help to users who do not have a 2D forward operator available to perform assimilation studies and model performance assessments.
Overall, the presented dataset should encourage the community to continue exploring these observations, given the potential they have shown.
This Appendix provides a set of tables describing, in detail, the different groups within the resPrf data files. Table A1 describes the global attributes of the files. Table A2 describes the variables and attributes of the NetCDF group profiles. Table A3 provides the description of the variables in the group rays. Finally, Table A4 provides a description of the sub-groups and inner groups within the main group colls, along with their corresponding variables and attributes.
RP and EC led the study. EC is the PI of the ROHP-PAZ experiment. RP, EC, AP, and SO participated in the data processing at the ICE-CSIC, IEEC, and ultimately generated the resPrf products. DCH, SS, and JPW developed the processing of the standard RO products for PRO at UCAR. KNW, FJT, COA, and MdlTJ participated in the processing, testing, comparison, and collocations at NASA/JPL. All authors contributed to writing and reviewing the paper.
The contact author has declared that none of the authors has any competing interests.
Publisher's note: Copernicus Publications remains neutral with regard to jurisdictional claims made in the text, published maps, institutional affiliations, or any other geographical representation in this paper. While Copernicus Publications makes every effort to include appropriate place names, the final responsibility lies with the authors.
This publication has been supported by the grant nos. RYC2021‐033309‐I and PID2021-1264436OB-C22 provided by the MCIN/AEI (10.13039/501100011033) and the European Union “NextGenerationEU”/PRTR and “ERDF A way of making Europe”. Work performed at the ICE-CSIC was also partially supported by the program Unidad de Excelencia María de Maeztu (grant no. CEX2020-001058-M). Part of the investigations at ICE-CSIC, IEEC, were done under the EUMETSAT ROM SAF CDOP4. The work by the authors Kuo-Nung Wang, F. Joe Turk, Chi O. Ao, and Manuel de la Torre Juárez was carried out at the Jet Propulsion Laboratory of the California Institute of Technology under a contract with the National Aeronautics and Space Administration. The authors would like to thank the two anonymous reviewers for their comments and suggestions, which definitely helped to improve the paper.
This research has been supported by the Agencia Estatal de Investigación (grant nos. RYC2021-033309-I, PID2021-1264436OB-C22, and CEX2020-001058-M).
The article processing charges for this open-access publication were covered by the CSIC Open Access Publication Support Initiative through its Unit of Information Resources for Research (URICI).
This paper was edited by Graciela Raga and reviewed by two anonymous referees.
Anthes, R. A.: Exploring Earth's atmosphere with radio occultation: contributions to weather, climate and space weather, Atmos. Meas. Tech., 4, 1077–1103, https://doi.org/10.5194/amt-4-1077-2011, 2011. a
Ao, C. O., Waliser, D. E., Chan, S. K., Li, J. L., Tian, B., Xie, F., and Mannucci, A. J.: Planetary boundary layer heights from GPS radio occultation refractivity and humidity profiles, J. Geophys. Res.-Atmos., 117, 1–18, https://doi.org/10.1029/2012JD017598, 2012. a
Berg, W.: GPM SSMIS on F16 Common Calibrated Brightness Temperatures L1C 1.5 hours 12 km V07, Greenbelt, MD, USA, Goddard Earth Sciences Data and Information Services Center (GES DISC) [data set], https://doi.org/10.5067/GPM/SSMIS/F16/1C/07, 2021a. a
Berg, W.: GPM SSMIS on F17 Common Calibrated Brightness Temperatures L1C 1.5 hours 12 km V07, Greenbelt, MD, USA, Goddard Earth Sciences Data and Information Services Center (GES DISC) [data set], https://doi.org/10.5067/GPM/SSMIS/F17/1C/07, 2021b. a
Berg, W.: GPM SSMIS on F18 Common Calibrated Brightness Temperatures L1C 1.5 hours 12 km V07, Greenbelt, MD, USA, Goddard Earth Sciences Data and Information Services Center (GES DISC) [data set], https://doi.org/10.5067/GPM/SSMIS/F18/1C/07, 2021c. a
Berg, W.: GPM GMI Common Calibrated Brightness Temperatures Collocated L1C 1.5 hours 13 km V07, Greenbelt, MD, USA, Goddard Earth Sciences Data and Information Services Center (GES DISC) [data set], https://doi.org/10.5067/GPM/GMI/GPM/1C/07, 2022a. a
Berg, W.: GPM AMSR-2 on GCOM-W1 Common Calibrated Brightness Temperature L1C 1.5 hours 10 km V07, Greenbelt, MD, Goddard Earth Sciences Data and Information Services Center (GES DISC) [data set], https://doi.org/10.5067/GPM/AMSR2/GCOMW1/1C/07, 2022b. a
Berg, W.: GPM ATMS on SUOMI-NPP Common Calibrated Brightness Temperature L1C 1.5 hours 16 km V07, Greenbelt, MD, Goddard Earth Sciences Data and Information Services Center (GES DISC) [data set], https://doi.org/10.5067/GPM/ATMS/NPP/1C/07, 2022c. a
Berg, W.: GPM MHS on NOAA-19 Common Calibrated Brightness Temperatures L1C 1.5 hours 17 km V07, Greenbelt, MD, Goddard Earth Sciences Data and Information Services Center (GES DISC) [data set], https://doi.org/10.5067/GPM/MHS/NOAA19/1C/07, 2022d. a
Berg, W.: GPM ATMS on NOAA-20 Common Calibrated Brightness Temperatures L1C 1.5 hours 17 km V07, Greenbelt, MD, Goddard Earth Sciences Data and Information Services Center (GES DISC) [data set], https://doi.org/10.5067/GPM/ATMS/NOAA20/1C/07, 2022e. a
Berg, W.: GPM MHS on METOP-A Common Calibrated Brightness Temperature L1C 1.5 hours 17 km V07, Greenbelt, MD, Goddard Earth Sciences Data and Information Services Center (GES DISC) [data set], https://doi.org/10.5067/GPM/MHS/METOPA/1C/07, 2022f. a
Berg, W.: GPM MHS on METOP-B Common Calibrated Brightness Temperature L1C 1.5 hours 17 km V07, Greenbelt, MD, Goddard Earth Sciences Data and Information Services Center (GES DISC) [data set], https://doi.org/10.5067/GPM/MHS/METOPB/1C/07, 2022g. a
Berg, W.: GPM MHS on METOP-C Common Calibrated Brightness Temperature L1C 1.5 hours 17 km V07, Greenbelt, MD, Goddard Earth Sciences Data and Information Services Center (GES DISC) [data set], https://doi.org/10.5067/GPM/MHS/METOPC/1C/07, 2022h. a
Berg, W.: GPM SAPHIR on MT1 Common Calibrated Brightness Temperature L1C 1.5 hours 10 km V07, Greenbelt, MD, Goddard Earth Sciences Data and Information Services Center (GES DISC) [data set], https://doi.org/10.5067/GPM/SAPHIR/MT1/1C/07, 2022i. a
Beyerle, G., Grunwaldt, L., Heise, S., Köhler, W., König, R., Michalak, G., Rothacher, M., Schmidt, T., Wickert, J., Tapley, B. D., and Giesinger, B.: First results from the GPS atmosphere sounding experiment TOR aboard the TerraSAR-X satellite, Atmos. Chem. Phys., 11, 6687–6699, https://doi.org/10.5194/acp-11-6687-2011, 2011. a
Cardellach, E., Tomás, S., Oliveras, S., Padullés, R., Rius, A., de la Torre-Juárez, M., Turk, F. J., Ao, C. O., Kursinski, E. R., Schreiner, W. S., Ector, D., and Cucurull, L.: Sensitivity of PAZ LEO Polarimetric GNSS Radio-Occultation Experiment to Precipitation Events, IEEE T. Geosci. Remote, 53, 190–206, https://doi.org/10.1109/TGRS.2014.2320309, 2014. a, b, c, d
Cardellach, E., Padullés, R., Tomás, S., Turk, F. J., de la Torre-Juárez, M., and Ao, C. O.: Probability of intense precipitation from polarimetric GNSS radio occultation observations, Q. J. Roy. Meteor. Soc., 144, 206–220, https://doi.org/10.1002/qj.3161, 2017. a
Cardellach, E., Oliveras, S., Rius, A., Tomás, S., Ao, C. O., Franklin, G. W., Iijima, B. A., Kuang, D., Meehan, T. K., Padullés, R., de la Torre-Juárez, M., Turk, F. J., Hunt, D. C., Schreiner, W. S., Sokolovskiy, S. V., Van Hove, T., Weiss, J. P., Yoon, Y., Zeng, Z., Clapp, J., Xia-Serafino, W., and Cerezo, F.: Sensing heavy precipitation with GNSS polarimetric radio occultations, Geophys. Res. Lett., 46, 1024–1031, https://doi.org/10.1029/2018GL080412, 2019. a, b, c
Culverwell, I. D., Lewis, H. W., Offiler, D., Marquardt, C., and Burrows, C. P.: The Radio Occultation Processing Package, ROPP, Atmos. Meas. Tech., 8, 1887–1899, https://doi.org/10.5194/amt-8-1887-2015, 2015. a
Gong, J. and Wu, D. L.: Microphysical properties of frozen particles inferred from Global Precipitation Measurement (GPM) Microwave Imager (GMI) polarimetric measurements, Atmos. Chem. Phys., 17, 2741–2757, https://doi.org/10.5194/acp-17-2741-2017, 2017. a, b, c
Hajj, G. A., Kursinski, E. R., Romans, L. J., Bertiger, W. I., and Leroy, S. S.: A Technical Description of Atmospheric Sounding By Gps occultation, J. Atmos. Solar-Terr. Phy., 64, 451–469, https://doi.org/10.1016/S1364-6826(01)00114-6, 2002. a
Hersbach, H., Bell, B., Berrisford, P., Hirahara, S., Horányi, A., Muñoz-Sabater, J., Nicolas, J., Peubey, C., Radu, R., Schepers, D., Simmons, A., Soci, C., Abdalla, S., Abellan, X., Balsamo, G., Bechtold, P., Biavati, G., Bidlot, J., Bonavita, M., De Chiara, G., Dahlgren, P., Dee, D. P., Diamantakis, M., Dragani, R., Flemming, J., Forbes, R. M., Fuentes, M., Geer, A. J., Haimberger, L., Healy, S. B., Hogan, R. J., Hólm, E. V., Janisková, M., Keeley, S., Laloyaux, P., Lopez, P., Lupu, C., Radnoti, G., de Rosnay, P., Rozum, I., Vamborg, F., Villaume, S., and Thépaut, J. N.: The ERA5 global reanalysis, Q. J. Roy. Meteor. Soc., 146, 1999–2049, https://doi.org/10.1002/qj.3803, 2020. a
Hersbach, H., Bell, B., Berrisford, P., Biavati, G., Horányi, A., Muñoz Sabater, J., Nicolas, J., Peubey, C., Radu, R., Rozum, I., Schepers, D., Simmons, A., Soci, C., Dee, D. P., and Thépaut, J.-N.: ERA5 hourly data on pressure levels from 1940 to present, Copernicus Climate Change Service (C3S) Climate Data Store (CDS) [data set], https://doi.org/10.24381/cds.bd0915c6, 2023. a, b
Hotta, D., Lonitz, K., and Healy, S.: Forward operator for polarimetric radio occultation measurements, Atmos. Meas. Tech., 17, 1075–1089, https://doi.org/10.5194/amt-17-1075-2024, 2024. a, b, c, d, e
Huffman, G. J.: GPM IMERG Final Precipitation L3 Half Hourly 0.1 degree x 0.1 degree V05, Greenbelt, MD, Goddard Earth Sciences Data and Information Services Center (GES DISC) [data set], https://doi.org/10.5067/GPM/IMERG/3B-HH/05, 2017. a
Huffman, G. J., Stocker, E. F., Bolvin, D. T., Nelkin, E. J., and Tan, J.: GPM IMERG Final Precipitation L3 Half Hourly 0.1 degree x 0.1 degree V07, Greenbelt, MD, Goddard Earth Sciences Data and Information Services Center (GES DISC) [data set], https://doi.org/10.5067/GPM/IMERG/3B-HH/07, 2023. a
Janowiak, J., Joyce, B., and Xie, P.: NCEP/CPC L3 Half Hourly 4km Global (60S–60N) Merged IR V1, edited by: Savtchenko, A., Greenbelt, MD, Goddard Earth Sciences Data and Information Services Center (GES DISC) [data set], https://doi.org/10.5067/P4HZB9N27EKU, 2017. a, b
Kidd, C., Huffman, G. J., Maggioni, V., Chambon, P., and Oki, R.: The Global Satellite Precipitation Constellation. Current Status and Future Requirements, B. Am. Meteorol. Soc., 102, 1844–1861, https://doi.org/10.1175/BAMS-D-20-0299.1, 2021. a, b
Kursinski, E. R., Hajj, G. A., Schofield, J. T., Linfield, R. P., and Hardy, K. R.: Observing Earth's atmosphere with radio occultation measurements using the Global Positioning System, J. Geophys. Res., 102, 23429–23465, https://doi.org/10.1029/97JD01569, 1997. a, b
Li, Z., Tang, G., Kirstetter, P. E., Gao, S., Li, J. L., Wen, Y., and Hong, Y.: Evaluation of GPM IMERG and its constellations in extreme events over the conterminous united states, J. Hydrol., 606, 127357, https://doi.org/10.1016/j.jhydrol.2021.127357, 2022. a
Murphy, M. J., Haase, J. S., Padullés, R., Chen, S. H., and Morris, M. A.: The potential for discriminating microphysical processes in numerical weather forecasts using airborne polarimetric radio occultations, Remote Sens., 11, U2048–U2072, https://doi.org/10.3390/rs11192268, 2019. a
Padullés, R., Ao, C. O., Turk, F. J., de la Torre Juárez, M., Iijima, B., Wang, K. N., and Cardellach, E.: Calibration and validation of the Polarimetric Radio Occultation and Heavy Precipitation experiment aboard the PAZ satellite, Atmos. Meas. Tech., 13, 1299–1313, https://doi.org/10.5194/amt-13-1299-2020, 2020. a, b, c, d, e, f, g, h, i, j
Padullés, R., Cardellach, E., Turk, F. J., Ao, C. O., de la Torre-Juárez, M., Gong, J., and Wu, D. L.: Sensing Horizontally Oriented Frozen Particles With Polarimetric Radio Occultations Aboard PAZ: Validation Using GMI Coincident Observations and Cloudsat aPriori Information, IEEE T. Geosci. Remote, 60, 1–13, https://doi.org/10.1109/TGRS.2021.3065119, 2022. a, b, c, d
Padullés, R., Cardellach, E., and Turk, F. J.: On the global relationship between polarimetric radio occultation differential phase shift and ice water content, Atmos. Chem. Phys., 23, 2199–2214, https://doi.org/10.5194/acp-23-2199-2023, 2023. a, b, c
Padullés, R., Cardellach, E., and Oliveras, S.: resPrf, DIGITAL.CSIC [data set], https://doi.org/10.20350/DIGITALCSIC/16137, 2024. a, b
Paz, A., Padullés, R., and Cardellach, E.: Evaluating the Polarimetric Radio Occultation Technique Using NEXRAD Weather Radars, Remote Sens., 16, 1118, https://doi.org/10.3390/rs16071118, 2024. a
Peinó, E., Bech, J., and Udina, M.: Performance Assessment of GPM IMERG Products at Different Time Resolutions, Climatic Areas and Topographic Conditions in Catalonia, Remote Sens., 14, 5085, https://doi.org/10.3390/rs14205085, 2022. a
Ramadhan, R., Yusnaini, H., Marzuki, M., Muharsyah, R., Suryanto, W., Sholihun, S., Vonnisa, M., Harmadi, H., Ningsih, A. P., Battaglia, A., Hashiguchi, H., and Tokay, A.: Evaluation of GPM IMERG Performance Using Gauge Data over Indonesian Maritime Continent at Different Time Scales, Remote Sens., 14, 1–24, https://doi.org/10.3390/rs14051172, 2022. a
Sokolovskiy, S.: Improvements, modifications, and alternative approaches in the processing of GPS RO data, in: ECMWF/EUMETSAT ROM SAF Workshop, 16–18 June 2014, Reading, UK, https://cdaac-www.cosmic.ucar.edu/cdaac/doc/documents/Sokolovskiy_newroam.pdf (last access: 4 December 2024), 2014. a
Sokolovskiy, S. V., Kuo, Y.-H., Rocken, C., Schreiner, W. S., Hunt, D. C., and Anthes, R. A.: Monitoring the atmospheric boundary layer by GPS radio occultation signals recorded in the open-loop mode, Geophys. Res. Lett., 33, 12–15, https://doi.org/10.1029/2006GL025955, 2006. a
Turk, F. J., Padullés, R., Ao, C. O., de la Torre-Juárez, M., Wang, K.-N., Franklin, G. W., Lowe, S. T., Hristova-Veleva, S. M., Fetzer, E. J., Cardellach, E., Kuo, Y.-H., and Neelin, J. D.: Benefits of a closely-spaced satellite constellation of atmospheric polarimetric radio occultation measurements, Remote Sens., 11, 2399, https://doi.org/10.3390/rs11202399, 2019. a
Turk, F. J., Padullés, R., Cardellach, E., Ao, C. O., Wang, K.-N., Morabito, D. D., de la Torre-Juárez, M., Oyola, M., Hristova-Veleva, S. M., and Neelin, J. D.: Interpretation of the Precipitation Structure Contained in Polarimetric Radio Occultation Profiles Using Passive Microwave Satellite Observations, J. Atmos. Ocean. Tech., 38, 1727–1745, https://doi.org/10.1175/jtech-d-21-0044.1, 2021. a, b
Turk, F. J., Padullés, R., Morabito, D. D., Emmenegger, T., and Neelin, J. D.: Distinguishing Convective-Transition Moisture-Temperature Relationships with a Constellation of Polarimetric Radio Occultation Observations in and near Convection, Atmosphere, 13, 1–27, https://doi.org/10.3390/atmos13020259, 2022. a
Turk, F. J., Cardellach, E., de la Torre-Juárez, M., Padullés, R., Wang, K.-N., Ao, C. O., Kubar, T. L., Murphy, M. J., Neelin, J. D., Emmenegger, T., Wu, D. L., Nguyen, V., Kursinski, E. R., Masters, D., Kirstetter, P. E., Cucurull, L., and Lonitz, K.: Advances in the Use of Global Navigation Satellite System Polarimetric Radio Occultation Measurements for NWP and Weather Applications, B. Am. Meteorol. Soc., 105, E905–E914, https://doi.org/10.1175/BAMS-D-24-0050.1, 2024. a
UCAR-COSMIC-Program: PAZ RO Data Products, UCAR [data set], https://doi.org/10.5065/k9vg-t494, 2023a. a, b
UCAR-COSMIC-Program: TerraSar-X RO Data Products, UCAR [data set], https://doi.org/10.5065/fv7s-ax27, 2023b. a, b
Unidata: NetCDF data format Version 4, Unidata [data set], https://doi.org/10.5065/D6H70CW6, 2023. a
Wang, K.-N., Ao, C. O., Padullés, R., Turk, F. J., de la Torre-Juárez, M., and Cardellach, E.: The Effects of Heavy Precipitation on Polarimetric Radio Occultation (PRO) Bending Angle Observations, J. Atmos. Ocean. Tech., 39, 161–173, https://doi.org/10.1175/jtech-d-21-0032.1, 2021. a
Watters, D. C., Gatlin, P. N., Bolvin, D. T., Huffman, G. J., Joyce, R., Kirstetter, P., Nelkin, E. J., Ringerud, S., Tan, J., Wang, J., and Wolff, D.: Oceanic Validation of IMERG-GMI Version 6 Precipitation Using the GPM Validation Network, J. Hydrometeorol., 25, 125–142, https://doi.org/10.1175/jhm-d-23-0134.1, 2023. a
Wee, T. K., Anthes, R. A., Hunt, D. C., Schreiner, W. S., and Kuo, Y. H.: Atmospheric GNSS RO 1D-Var in Use at UCAR: Description and Validation, Remote Sens., 14, 5614, https://doi.org/10.3390/rs14215614, 2022. a
Weiss, J. P. and Hunt, D. C.: GNSS High Rate Binary Format (v2.1), Tech. rep., UCAR, https://cdaac-www.cosmic.ucar.edu/cdaac/fileFormats/GNSS_High_Rate_Binary_Format_v2.1.pdf (last access: 4 December 2024), 2022. a
Zeng, X., Skofronick-Jackson, G., Tian, L., Emory, A. E., Olson, W. S., and Kroodsma, R. A.: Analysis of the global microwave polarization data of clouds, J. Climate, 32, 3–13, https://doi.org/10.1175/JCLI-D-18-0293.1, 2019. a, b
- Abstract
- Introduction
- PRO technique in a nutshell
- Dataset description: profiles
- Dataset description: rays
- Dataset description: collocations
- Evaluation of retrievals
- Illustrative example: collocation with ERA-5
- Data availability
- Conclusions
- Appendix A
- Appendix B
- Author contributions
- Competing interests
- Disclaimer
- Acknowledgements
- Financial support
- Review statement
- References
- Abstract
- Introduction
- PRO technique in a nutshell
- Dataset description: profiles
- Dataset description: rays
- Dataset description: collocations
- Evaluation of retrievals
- Illustrative example: collocation with ERA-5
- Data availability
- Conclusions
- Appendix A
- Appendix B
- Author contributions
- Competing interests
- Disclaimer
- Acknowledgements
- Financial support
- Review statement
- References