the Creative Commons Attribution 4.0 License.
the Creative Commons Attribution 4.0 License.
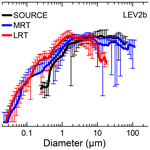
Large synthesis of in situ field measurements of the size distribution of mineral dust aerosols across their life cycles
Paola Formenti
Claudia Di Biagio
Mineral dust aerosol is important in the Earth system, and the correct representation of its size distribution is fundamental for shaping the current state and evolution of the climate. Despite many observational dust size data that are available in the literature, using this body of information to properly guide the development and validation of climate models and remote sensing retrievals remains challenging. In this study we collect, evaluate, harmonize, and synthesize 58 size distribution data from the past 50 years of in situ field observations with the aim of providing a consistent dataset to the community for use in constraining the representation of dust size across its life cycle. Four levels (LEVs) of data treatment are defined, going from original data (LEV0), data interpolated and normalized on a standardized diameter grid (LEV1), and data in which original particle diameters are converted to a common geometrical definition under both spherical (LEV2a) and aspherical (LEV2b) assumptions. Size distributions are classified as emission or source (SOURCE, <1 d from emission; number of datasets in this category N=12), mid-range transport (MRT, 1–4 d of transport; N=36), and long-range transport (LRT, >4 d of transport; N=10). The harmonized dataset shows consistent features suggesting the conservation of airborne particles with time and a decrease in the main coarse-mode diameter from a value on the order of 10 µm (in volume) for SOURCE dust to a value on the order of 1–2 µm for LRT conditions. An additional mode becomes evident below 0.4 µm for MRT and LRT dust. Data for the three levels (LEV1, LEV2a, and LEV2b) and the three categories (SOURCE, MRT, and LRT), together with statistical metrics (mean, median, 25th and 75th percentiles, and standard deviation), are available as follows:
-
SOURCE (https://doi.org/10.57932/58dbe908-9394-4504-9099-74a3e77140e9, Formenti and Di Biagio, 2023a);
-
MRT (https://doi.org/10.57932/31f2adf7-74fb-48e8-a3ef-059f663c47f1, Formenti and Di Biagio, 2023b);
-
LRT (https://doi.org/10.57932/17dc781c-3e9d-4908-85b5-5c99e68e8f79, Formenti and Di Biagio, 2023c).
- Article
(1711 KB) - Full-text XML
-
Supplement
(1980 KB) - BibTeX
- EndNote
Airborne mineral dust aerosols emitted by the eolian erosion of bare soils contribute in a major way to Earth's radiative budget and environmental processes, including human health. Because of their native mineralogical composition and size distribution, they interact with solar and infrared radiation, influence the formation and brightness of liquid and ice clouds, and affect the composition of the atmosphere and the ocean while also transporting pollutants, viruses, and bacteria across the continents and the oceans (Knippertz and Stuut, 2014, and the many references therein).
As a consequence, a large effort has started in the last few decades to include the representation of those properties in climate and air quality models. Indeed, the complex mineralogy of mineral dust, depending on that of the parent soils (Claquin et al., 1999; Journet et al., 2014; Gonçalves Ageitos et al., 2023), is now accounted for in models (Scanza et al., 2015; Perlwitz et al., 2015a, b; Menut et al., 2020; Kok et al., 2017; Di Biagio et al., 2020; Gómez Maqueo Anaya et al., 2024) and is starting to be retrieved by remote sensing (Green et al., 2020; Zhou et al., 2020; Di Biagio et al., 2023).
On the other hand, representing the span and the variability in time and space of the dust aerosol size distribution remains a challenge.
The particle size distribution of mineral dust extends over several orders of magnitude. Iron-rich particles as small as 14 nm in diameter were observed from deflating soils in the laboratory by Baddock et al. (2013). During a sandstorm in Algeria, Gomes et al. (1990) measured an increase in the mass concentration of particles between 100 nm and 1 µm and attributed this to clays disaggregated by sandblasting. Measurements of the size-resolved vertical dust flux by Gillette et al. (1972, 1974) and Gillette (1974) based on microscopy analyses of samples from Texas and Nebraska showed the presence of particles of up to several microns in dust emissions.
The representation of the accumulation and coarse modes in mineral dust has long been based on the columnar measurements by the sun or sky photometers of the AErosol RObotic NETwork (AERONET), which provide (with normalized size distributions of mineral dust considered to be chemically homogeneous particles) the 0.1–30 µm optically equivalent diameter (Dubovik et al., 2002, 2006) and which, incidentally, also serve as the look-up tables of the remote sensing retrievals of dust from space (e.g., Cuesta et al., 2015; Zhou et al., 2020).
Nevertheless, in situ observations at ground-based stations and on aircraft in more recent years have shown that particles of several tens, sometimes hundreds, of microns are airborne at emission and remain so after several days of transport (J. S. Reid et al., 2003; Formenti et al., 2003; Rajot et al., 2008; Chou et al., 2008; Kandler et al., 2007, 2009; Wagner et al., 2009; Klaver et al., 2011; Ryder et al., 2013a, 2015; Rosenberg et al., 2014; Denjean et al., 2016a, b; Weinzierl et al., 2017; van der Does et al., 2018).
These observations have been instrumental in a number of advances. Using them as an ensemble dataset to smooth local atmospheric variability, they have served as a basis for a new classification of the dust size distribution in four modes, i.e., fine dust (diameter ≤2.5 µm), coarse dust (2.5 < diameter ≤10 µm), super-coarse dust (10 < diameter ≤62.5 µm), and giant dust (diameter >62.5 µm), extending above the size range retrieved by AERONET (Adebiyi et al., 2023). Additionally, they have fostered the revision of the numerical schemes of emissions and deposition and identified the numerous processes and properties (nonspherical shapes of particles, electric forces, atmospheric turbulence) that could counteract the size-selective removal by gravitational settling and keep particles airborne longer than expected (Kok, 2011; Huneeus et al., 2011; Mahowald et al., 2011; Kok et al., 2017; Di Biagio et al., 2020; Zhao et al., 2022; Adebiyi and Kok, 2020; Adebiyi et al., 2020; Huang et al., 2021; Meng et al., 2022; Adebiyi et al., 2023).
In support of those activities, in this paper we present a large and standardized compilation of in situ observations of the particle size distribution of mineral dust made during the past 50 years of research. This dataset extends the currently published compilations of measurements (Meng et al., 2022; Adebiyi et al., 2020, 2023) to provide the state of the art in current knowledge in support of the development of models and ground-based and satellite remote sensing. Analysis of this dataset may provide an integrated view of the size distribution of dust particles across their life cycle to evaluate their impacts on the Earth or human system.
2.1 Constitution of the dataset
The data presented in this paper come from in situ ground-based and aircraft observations of airborne dust conducted during field campaigns in the past 50 years of dust research. Data from deposition samples (e.g., van der Does et al., 2018, or Varga et al., 2021) are not considered in this analysis.
Only datasets published and properly referenced in the open peer-reviewed literature were retained. We also favored datasets for which the methodology of acquisition, calibration, and data treatment was well described so that the data quality could be assessed. Finally, we searched for data that, as far as possible, were representative of different source and transport regions of the world.
The observations contributing to the dataset are listed in Table S1 in the Supplement, and the spellings of the abbreviations of the field campaigns are reported in Sect. S1 in the Supplement. Data are geo-localized in Fig. 1, where they are classified with respect to their time after emission. Geographical coordinates are reported in Table S2 in the Supplement.
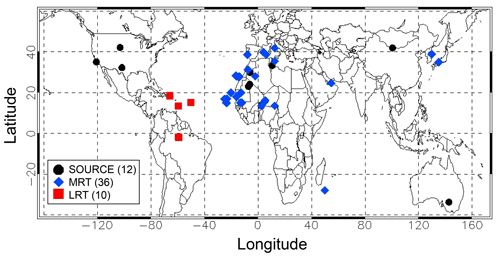
Figure 1Geographical location of the datasets contributing to size distribution observations for the SOURCE, mid-range transport (MRT), and long-range transport (LRT) categories. The legend indicates the line style used in the plot. The number of data for each category is indicated in the parentheses in the legend.
Observations obtained at the time of dust emission or within 1 d after emission are classified as SOURCE. Observations corresponding to 1 to 4 d after emission and/or geographically acquired near source regions (for example, offshore of northern Africa) are classified as mid-range transport (MRT). Observations at times exceeding 4 d after emission or geographically distant from source regions (for example, observations in the Caribbean) are classified as long-range transport (LRT). Note that potential uncertainties may arise in this classification, in particular for datasets lying at the boundaries of the SOURCE, MRT, and LRT categories, and we acknowledge this aspect as a source of error in our analysis. We invite the reader to refer to the Supplement (Sect. S4) for a thorough description of the assumptions made in some cases to associate each dataset with a category.
The SOURCE dataset (Fig. 1, black points) consists of 12 observations in northern Africa, North America, and Asia as well as one dataset in Australia. It includes works by Gillette et al. (1972, 1974), Gillette (1974), Fratini et al. (2007), Rajot et al. (2008), Sow et al. (2009), Shao et al. (2011), Ryder et al. (2013a, b), Rosenberg et al. (2014), Huang et al. (2019), and Khalfallah et al. (2020); a set of data recently used by Kok et al. (2017), Di Biagio et al. (2020), and Huang et al. (2021) to constrain the shape of the dust size distribution at emission in model studies; and the most recent work by Gónzales-Flórez et al. (2023). The MRT class (Fig. 1, blue points) is contributed by 36 datasets from field campaigns (ACE2, ACE-Asia, ADRIMED, AER-D, AMMA, DABEX, DARPO, DIAPASON, DODO1-2, FENNEC, GAMARF, GERBILS, INDOEX, NAMMA, RHaMBLe, SALTRACE, SAMUM1-2, TRACE-P, and UAE2) in western Africa, Cabo Verde, the Mediterranean basin, the eastern tropical Atlantic, Saudi Arabia, Japan, and the Indian Ocean, which are downwind sources either over the ocean or over desert areas. Additional datasets from studies performed in the Sahara, the Atlantic Ocean, the Canary Islands, and Japan (Schütz et al., 1981; D'Almeida, 1987; Maring et al., 2000; Kobayashi et al., 2007) are added to the dataset. The LRT class (Fig. 1, red points) is based on 10 datasets of observations across the Atlantic Ocean and South America and is contributed by observations from the Bacex, CLAIRE, Dust-Attack, Go-Amazon, PRIDE, and SALTRACE campaigns and intercontinental dust transport data from Schütz et al. (1981).
2.2 Instrumentation contributing to the in situ dataset
The natural dynamical range of the particle size and concentration of mineral dust can only be represented by a combination of instruments based on different intrinsic particle properties such as density, electrical charge, shape, and composition (e.g., E. A. Reid et al., 2003; Formenti et al., 2011; Wendisch and Brenguier, 2013; Mahowald et al., 2014; Adebiyi et al., 2023). As a consequence, the datasets considered in this paper are contributed by different in situ instruments (also described in Sect. S2 in the Supplement) listed as follows:
-
Optical particle counters (OPCs) use the dependence of light scattering on particle size and provide the particle concentration as a function of the optical equivalent diameter (e.g., J. S. Reid et al., 2003; Clarke et al., 2004; Osborne et al., 2008; Formenti et al., 2011; Ryder et al., 2013a, 2018; Khalfallah et al., 2020).
-
Particle collection by filtration or impaction is followed by individual particle characterization by transmission electron microscopy (TEM) and/or scanning electron microscopy (SEM) sizing particles as a function of their equivalent projected-area diameter and Coulter geometric sizing methods (e.g., Gillette et al., 1972, 1974; Gillette, 1974; E. A. Reid et al., 2003; Kobayashi et al., 2007; Kandler et al., 2009; Chou et al., 2008).
-
Multistage filtration or impaction sampling coupled with gravimetric or chemical analysis provides the mass size distribution as the equivalent aerodynamic diameter (e.g., Formenti et al., 2001; J. S. Reid et al., 2003).
-
Differential and scanning mobility particle sizers (DMPSs and SMPSs) provide the sizes of particles in the submicron range as the electrical mobility equivalent diameter of a charged particle moving in a static electric field (e.g., Maring et al., 2000, 2003; Bates et al., 2002; Müller et al., 2010; Denjean et al., 2016a, b).
-
Aerodynamic particle sizers (APSs) measure the equivalent aerodynamic diameter of a sphere of unit density with the same terminal velocity in an accelerated airflow as the irregularly shaped dust particles (e.g., Maring et al., 2003; J. S. Reid et al., 2003, 2008; Struckmeier et al., 2016).
Each of these instrument type size particles on an equivalent diameter (optical, projected area, aerodynamic, and mobility) depends on its respective working principle. Converting these operational size definitions to a homogenized one is part of the treatment applied in this work, which follows the theory proposed and discussed in the literature and benefits from recent progress in characterizing or synthesizing dust properties relevant to these treatments (e.g., Hinds, 1999; DeCarlo et al., 2004; Mahowald et al., 2014; Di Biagio et al., 2019; Huang et al., 2021). Diameter definitions and formulas for converting each of them to a geometrical diameter, under the assumption of both spherical and aspherical dust, are provided in Sect. S3 and summarized in Table S3 in the Supplement.
Section S4 presents relevant information on each dataset considered in the present analysis. This includes a brief description of the field operations, the experimental conditions, the type of original data (number, volume or mass concentration size distribution, and size-resolved emission fluxes), the instrumentation, and the data treatment applied to the measurements (averages and diameter corrections) in the original publication. Original data were obtained, as far as possible, through personal contact with the data providers or from the original publications. This is also indicated in Sect. S4.
2.3 Data treatment, harmonization, and synthesis
The original observations were treated to provide a harmonized dataset in terms of the definition of the particle diameter, and the data were normalized to remove differences in the sampled number concentrations. The four levels of data treatment are defined as described below:
- 1.
Level-0 (LEV0) original data are taken at the native resolution or the resolution from the digitization process and are then converted to the volume distribution assuming spherical particles (), where D is the particle diameter used in the publication and is the particle number concentration. To remove differences in concentration and in the absence of information on the original bin width, LEV0 data are normalized to the maximum of the volume size distribution.
- 2.
Level-1 (LEV1) data from LEV0 are interpolated over a common size range of equi-logarithmically spaced diameters (dlog D = 0.05) encompassing the original diameter range for each dataset and normalized so that the integral is equal to 1 over a common diameter range. The diameter range for integral normalization was set to be as large as possible and covered by more than 90 % of the datasets in each category. For the SOURCE data this resulted in the diameter range for common integral normalization being between 1.58 and 7.1 µm, and for MRT and LRT it is between 0.71 and 8.9 µm.
- 3.
Level-2a (LEV2a) data. Based on LEV1, the LEV2a data treatment aims to harmonize the size distributions by converting the operational original particle diameters, which depend on the physical principle of each instrument, to a commonly defined sphere-equivalent geometric diameter. Data from LEV1 are treated as in the following with respect to their diameter corrections:
- –
Data already provided as geometrical diameters (from Coulter counters, i.e., only one dataset in our study) are left unchanged. Data provided as projected-area diameters (i.e., from microscopy) are left unchanged.
- –
Data provided as aerodynamic diameters (from APS or cascade impactors) are corrected assuming a shape factor (χ) of 1 (under spherical assumption). Therefore, a size-invariant conversion factor of 1.58 (see Eq. S2 in the Supplement) is applied to the dataset assuming a dust density of 2.5 g cm−3 (). If the original aerodynamic diameter data have already been converted to the geometrical diameter, we replace the original correction with a conversion factor of 1.58. Since the correction is a multiplicative factor, the dlog D of the bins remains unchanged.
- –
Data provided as optical diameters (from OPCs) are converted to sphere-equivalent geometric diameters by applying the optical-to-geometrical corrections by assuming homogeneous spherical particles and a value of the complex refractive index (CRI) of 1.53–0.003i. This CRI value is the average of the dust refractive indices reported in the 370–950 nm spectral range in Di Biagio et al. 2019) for dust of global origin. Data for applying the correction to the different models of the OPCs considered were taken from Formenti et al. (unpublished data), and the conversion factors were recalculated at the dlog D path of 0.05 assumed in the interpolated sizes. For GRIMM 1.108, we used the data taken from Formenti et al. (2011) interpolated at the 0.05 dlog D path of our diameters. In order to avoid discontinuities appearing and because of the new dlog D not significantly differing on average from the value of 0.05 for Dgeom calculated from Dopt interpolated data, we do not update the dlog D, so that the conversion only implies a shift in the diameter. More details on the choices applied for corrections in the different cases are provided in Sect. S4. The original datasets already converted to the geometrical diameter are left unchanged. However, it is worth noting that the ensemble of data already applying an optical-to-geometrical correction uses CRIs varying between 1.53 and 1.55 for the real part and between 0.001 and 0.004 for the imaginary part. It works under the hypothesis of homogeneous spherical particles (Mie theory), which is therefore consistent with our treatment. Exceptions are Khalfallah et al. (2020) using a CRI of 1.43–0.00i for quartz particles and González-Flórez et al. (2023) using a CRI of 1.49–0.0015i, also applying calculations under an ellipsoidal assumption instead of Mie theory. The only dataset not theoretically submitted for optical-to-geometrical correction is the one provided by Renard et al. (2018) using an OPC built with a specific geometry, making the measurement sensitivity to CRI calibration very low.
- –
- 4.
Level 2b (LEV2b). Based on LEV1, the LEV2b data treatment aims to harmonize the size distributions by converting the operational original particle diameters to a commonly defined geometrical diameter by taking into account the fact that mineral dust is aspherical. Data from LEV1 are treated as follows with respect to their diameter corrections:
- –
Data already provided as geometrical diameters from Coulter counters are left unchanged. This technique is in fact only slightly affected by shape effects, as discussed by Kobayashi et al. (2007).
- –
Data provided as projected-area diameters are corrected using the size-invariant correction factor of 1.56 from Huang et al. (2021) () (see Eq. S1 in the Supplement).
- –
Data provided as aerodynamic diameters are corrected assuming a size-invariant conversion factor of 1.45 following Huang et al. (2021) () (see Eq. S2).
- –
Data provided as optical diameters and already treated as for LEV2a data are further corrected by applying a size-dependent aspherical-to-spherical ratio (ASR(Dgeom)) correction function, ASR, to take into account nonsphericity effects in optical-to-geometrical conversion. The ASR function (Fig. S1 in the Supplement) is obtained by combining the optical-to-geometrical diameter conversion factors for different OPCs calculated by Formenti et al. (unpublished data) and Huang et al. (2021), both under the assumption of spherical homogeneous particles (Dgeom)spherical and triaxial ellipsoid dust (Dgeom)aspherical. More details are provided in Sect. S3. The original datasets derived from OPC measurements already provided as geometrical diameters but under an assumption of sphericity are also corrected by applying the ASR(Dgeom) conversion function. The only exceptions are González-Flórez et al. (2023), who already applied triaxial ellipsoid calculations in their optical-to-geometrical conversion, and Renard et al. (2018), who did not require optical-to-geometrical conversion.
- –
As for LEV1, the LEV2a and LEV2b data, for which a known interpolation path is used, are normalized so that the integral of the volume size distribution is 1 over the common diameter ranges (1.58–7.1 µm for SOURCE and 0.71–8.9 µm for MRT and LRT).
For each category (SOURCE, MRT, and LRT) and for each data level (LEV1, LEV2a, and LEV2b), the mean, median, and standard deviation of the particle volume concentration per size class are calculated where at least two datasets are available in the diameter range. Additionally, the 25th and 75th percentiles are calculated while keeping in mind their limited representativeness given the reduced number of samples in the datasets, especially for the SOURCE and LRT classes.
2.4 Limitations of the chosen approach
Some precisions should be given when considering the LEV2a and LEV2b treatments reported in this work. First, the implicit assumption when applying LEV2a and LEV2b dataset corrections is that dust is the dominant aerosol species, and possible effects due to internal or external mixing of dust with other aerosol types are not taken into consideration (i.e., in the complex refractive index or shape factor assumptions). Second, for those datasets that are obtained from the combination of different techniques, i.e., DMPS + APS (Bates et al., 2002; Maring et al., 2000, 2003; Müller et al., 2010), OPC + APS (Chen et al., 2011), SMPS + OPC (de Reus et al., 2000; Otto et al., 2007; Denjean et al., 2016a, b), DMPS + APS + microscopy (Kandler et al., 2011), or multiple OPC instruments (J. S. Reid et al., 2003; McConnell et al., 2008; Johnson and Osborne, 2011; Ryder et al., 2013a, b, 2018; Rosenberg et al., 2014; Weinzierl et al., 2009, 2011, 2017), the choice is to apply artifact corrections for the dominant instrument, often the one in the extended coarse-mode range, and to consider this correction to be applicable to the whole diameter range. This is because, when multiple instruments are used to build a size distribution, it is not easy to reconstruct the steps of the data analysis and to merge from the original work. The subsequent considerations follow:
-
The corrections applied for the aerodynamic and projected-area diameters apply a constant size-invariant scaling factor to the ensemble of the size distribution data. In this approximation, if the SMPS or DMPS is combined with aerodynamic or microscopy data, a correction factor between 1.45 and 1.58, depending on the level and the technique as detailed in the previous section, is applied in place of the factor of 1 (spherical assumption) or 1.19 (aspherical assumption) (see Eq. S3 in the Supplement) that is expected to convert the mobility diameter to the geometrical diameter in the LEV2a and LEV2b data. As a consequence, the submicron size is 20 to 58 % finer than expected, which is only due to the mobility-to-geometrical conversion.
-
A similar approach is used to correct datasets where an OPC is the main technique used to bring dust particles together with the SMPS. For the LEV2a data the Mie correction is applied to the full size distribution, but since the size-dependent correction is mostly inactive for submicron particles (i.e., Dgeom∼Dopt for most OPCs), the approach is mostly equivalent to considering a mobility diameter correction with a shape factor of 1. For the LEV2b data, using OPC corrections induces a limited right shifting of the size distribution compared to the one that would be obtained from mobility conversion because of the magnitude of the ASR function (Fig. S1) compared to the shape factor of 1.19 assumed for aspherical dust.
-
When datasets rely on multiple OPC measurements, the assumption is that the “dominant” OPC, i.e., the OPC covering the largest range and the coarsest sizes in particular, is considered. Given that optical-to-geometrical corrections are not relevant for submicron particles and that the magnitude of the correction typically increases for increasing sizes, this assumption is not expected to determine significant biases in the data. Additionally, a general ambiguity of the optical-to-geometrical correction exists around a diameter of 1 µm, where a plateau in the scattering calibration function for several OPC models can be found (i.e., Formenti et al., unpublished data).
More details on the specific assumptions and choices made for each dataset are provided in Sect. S4.
Further, for the LEV2a and LEV2b data for which corrections are applied, caution is needed at the boundary of the size distribution and when the first and/or last bin of the corrected size show significant divergence. These data are removed from the dataset.
An additional source of error is the individual measurement uncertainty, which varies with the specific setup, instrument, and spatial and temporal extent of the measurement.
Illustration of the data for different levels is provided in Fig. 2. Figure 3 presents the synthesis of the LEV2b data and the comparison of the SOURCE, MRT, and LRT distributions. The contribution of the different size classes to the total particle number, surface, and volume is summarized in Table 1. The size classes have been defined according to the classification of Adebiyi et al. (2023) defining fine dust (D≤2.5 µm), coarse dust ( µm), super-coarse dust ( µm), and giant dust (D>62.5 µm). Within the fine-dust class, we further calculate the fractions of particles smaller than 0.4 µm.
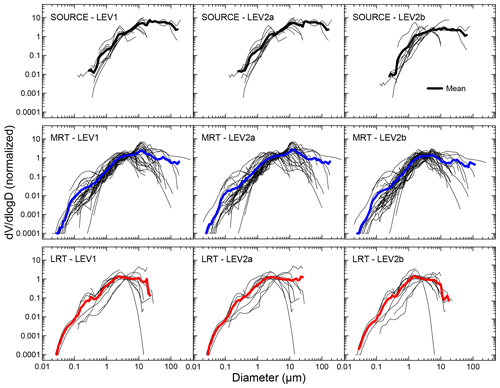
Figure 2Data for SOURCE, MRT, and LRT dust at levels 1, 2a, and 2b as described in Sect. 2.3 (labeled LEV1, LEV2a, and LEV2b, respectively). Single datasets, all normalized at the integral of 1, are plotted as black lines. The means (thick black, blue, and red lines for SOURCE, MRT, and LRT, respectively) are shown at all the levels. Note that the mean is calculated only where at least two datasets are available in the diameter range.
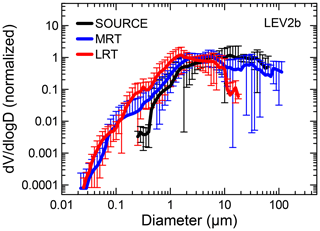
Figure 3Comparison of the normalized mean volume size distribution for the SOURCE, MRT, and LRT categories in our study reported as LEV2b data (mean ±standard deviation). For the sake of comparison, and differently from the data in Fig. 2, the SOURCE, MRT, and LRT synthesis datasets reported here are normalized at the integral of 1 over a common diameter range corresponding to 0.35–17.8 µm. This is done to remove differences linked to the different integration ranges for SOURCE data compared to MRT and LRT.
Table 1Percentages of number, surface, and volume size distribution in the diameter ranges D≤0.4 µm, D≤2.5 µm, µm, µm, and D>62.5 µm for the mean of the sizes obtained for the SOURCE, MRT, and LRT LEV2b datasets.
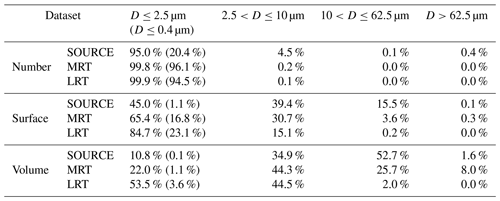
As shown in Figs. 2 and 3, the shape of the dust size distribution at emission and along transport shows the main consistent features. The main mode located at ∼10 µm (in volume) is observed for dust at emission and close to the sources, as based on the few studies allowing us to measure up to the coarse fraction. The main dust modes decrease to ∼5 and ∼2 µm for MRT and LRT conditions, respectively. Below 0.4 µm the dust volume size shows an additional mode that is particularly visible for MRT and LRT. As a matter of fact, the sparse datasets measuring very fine particles at SOURCE show that particles with diameters below 0.4 µm (measured however only down to 0.2 µm, as shown in Fig. 2) represent approximately 20 % of the total particle number, increasing to more than 90 % in MRT and LRT. Instruments such as the SMPS and DMPS used in MRT and LRT studies measure particles as small as 0.02 µm in diameter. Previous single-particle compositional observations show that the particle number concentration in the size range between 0.1 and 0.4 µm is largely contributed by aluminosilicate dust particles at emission, while internal or external mixing with aerosols other than dust gains importance with time and altitude of transport (Chou et al., 2008; Kandler et al., 2007, 2009; Weinzierl et al., 2009, 2017; Klaver et al., 2011; Denjean et al., 2016a, b).
The normalized size distribution of dust particles between 0.4 and 10 µm is rather consistent and invariant along the dust cycle. This is true in particular when restricting them to the 2.5 to 10 µm size range, where differences are minimal and the contribution to the total volume is between 34.9 % and 44.5 %. Below that range, which is between 0.4 and 2.5 µm, the contribution of particles to LRT is significantly higher (53.5 % in volume) than for SOURCE (10.8 %) and MRT (22.0 %), likely because, due to the normalization, it compensates for the decrease in particles larger than 10 µm.
The magnitude of the particle volume above 10 µm remains unchanged almost up to 100 µm for both the SOURCE and MRT conditions, which also present similar particle volumes. This mode decreases very strongly for LRT conditions, where it represents only 2 % of the total volume compared to almost 55 % and 34 % for SOURCE and MRT, respectively.
The dataset presented in this work, synthesizing available in situ observations, allows evaluation of the natural variability of dust size distributions along their life cycles. It should be emphasized, however, that while consistent differences in the mean size distribution curves are obtained when going from SOURCE to LRT, as shown in Fig. 3, the inherent range of variability for each category, represented by the standard deviation of the data, is also non-negligible and reflects the large range of documented size distributions together with the limited statistics that are available. This is particularly true for both super-coarse and giant dust at MRT and LRT. Lower variability is identified below 0.4 µm because of the restricted number of datasets available for MRT and LRT conditions, and there is an absence of data for SOURCE dust below this size range.
Finally, to facilitate the use of these data within models and remote sensing schemes, Table 2 provides the parameters of lognormal size distributions fitting the LEV2a and LEV2b mean values of the three dust categories. Lognormal functions are set to reproduce the main shape of the dust distribution above 0.4 µm, neglecting the specific features below this diameter, where there is less information and the size is affected by particle mixing with other compounds, especially for MRT and LRT. We found that a single broad mode can be employed to represent the main features of the volume size distributions above 0.4 µm. Plots of the fitting functions are provided in Fig. S4 in the Supplement. Because there is an inherent level of subjectivity in the choice of the number of modes and their parameters, we invite the individual researchers using the data to implement the parameterizations in accordance with their scientific needs.
Table 2Parameters: total number and volume concentration, Ntot ( cm−3), Vtot (nm3 cm−3), number and volume median diameter NMD and VMD (µm), and geometric standard deviation σ for the lognormal modes used to parameterize the LEV2b volume size distributions of the SOURCE, MRT, and LRT categories. The parameters refer to the following equations: and .
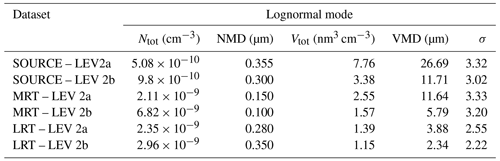
The LEV1, LEV2a, and LEV2b datasets discussed in this paper are available in the EaSy data repository (https://www.easydata.earth/#/public/home, last access: 1 June 2024) maintained by the National French DATA TERRA research infrastructure. Their respective DOIs are summarized here below:
-
SOURCE_LEV1.dat, SOURCE_LEV2a.dat, and SOURCE_LEV2b.dat: https://doi.org/10.57932/58dbe908-9394-4504-9099-74a3e77140e9 (Formenti and Di Biagio, 2023a);
-
MRT_LEV1.dat, MRT_LEV2a.dat, and MRT_LEV2b.dat: https://doi.org/10.57932/31f2adf7-74fb-48e8-a3ef-059f663c47f1 (Formenti and Di Biagio, 2023b);
-
LRT_LEV1.dat, LRT_LEV2a.dat, and LRT_LEV2b.dat: https://doi.org/10.57932/17dc781c-3e9d-4908-85b5-5c99e68e8f79 (Formenti and Di Biagio, 2023c).
The figures of the individual datasets (including LEV0) are provided upon request.
The data from the images in the published paper were digitized with the online WebplotDigitizer software available at https://automeris.io/WebPlotDigitizer/ (last access: 23 October 2024; Marin et al., 2017).
In this paper we present the most comprehensive synthesis possible of in situ observations of the particle size distribution of atmospheric dust aerosols. This compilation reflects the current state of the art and represents a standardized and synthetic benchmark to constrain and evaluate models and satellite retrievals. We highlight the differences and commonalities of the dust volume distribution as a function of time in the atmosphere in terms of both the main identified modes and the relative contribution of dust in different size ranges.
We did this based on high statistics of data to permit the retrieval of robust information between 0.4 and 10 µm, where most of the observations exist, while above and below this size range observations are rare. Dust particles below 0.4 µm in diameter are seldom measured close to source regions but are found in observations under mid- and long-range transport conditions. Their presence at emission, their size-segregated composition, and their state of mixing should be better documented and understood. The dynamics of the coarse mode above 10 µm, its invariance from the source to mid-range transport, and its decline afterwards are reported and can challenge the models.
We acknowledge the evidence that the compilation of a reference dataset is, almost by definition, a subjective and incomplete exercise which must be revised continuously with the emergence of new datasets, new field campaigns, and the improvement of sampling techniques. We henceforth encourage colleagues to provide us with new or revised datasets to feed and update the dataset in the future.
The supplement related to this article is available online at: https://doi.org/10.5194/essd-16-4995-2024-supplement.
PF and CDB designed the research, compiled and analyzed the dataset, and wrote the manuscript.
The contact author has declared that none of the authors has any competing interests.
Publisher's note: Copernicus Publications remains neutral with regard to jurisdictional claims made in the text, published maps, institutional affiliations, or any other geographical representation in this paper. While Copernicus Publications makes every effort to include appropriate place names, the final responsibility lies with the authors.
Paola Formenti and Claudia Di Biagio acknowledge Jean-Louis Rajot, Cyrielle Denjean, Adeyemi A. Adebiyi, Daniela Meloni, Claire Ryder, and Jasper Kok for providing the original data from their publications. The authors would like to thank Greg Schuster (NASA/Langley), Ron Miller (NASA/Giss), and an anonymous referee for their effort in improving the paper and the data access. The help of Guillaume Brissebrat (CNRS/DATA TERRA/Aeris), Hélène Bressan, and Sylvain Grellet (GaiaData BRGM) in creating the DOIs for the different datasets and solving the access issues is gratefully acknowledged.
This research is funded by the DustClim project, which is part of ERA4CS, an ERA-NET project initiated by JPI Climate and funded by FORMAS (SE), DLR (DE), BMWFW (AT), IFD (DK), MINECO (ES), and ANR (FR) with co-funding from the European Union's Horizon program (grant no. 690462).
This paper was edited by Iolanda Ialongo and reviewed by Ron L. Miller, Gregory L. Schuster, and one anonymous referee.
Adebiyi, A. A. and Kok, J. F.: Climate models miss most of the coarse dust in the atmosphere, Sci. Adv., 6, eaaz9507, https://doi.org/10.1126/sciadv.aaz9507, 2020.
Adebiyi, A. A., Kok, J. F., Wang, Y., Ito, A., Ridley, D. A., Nabat, P., and Zhao, C.: Dust Constraints from joint Observational-Modelling-experiMental analysis (DustCOMM): comparison with measurements and model simulations, Atmos. Chem. Phys., 20, 829–863, https://doi.org/10.5194/acp-20-829-2020, 2020.
Adebiyi, A. A., Kok, J., Murray, B. J., Ryder, C. L., Stuut, J.-B. W., Kahn, R. A., Knippertz, P., Formenti, P., Mahowald, N. M., Pérez García-Pando, C., Klose, M., Ansmann, A., Samset, B. H., Ito, A., Balkanski, Y., Di Biagio, C., Romanias, M. N., Huang, Y., and Meng, J.: A review of coarse mineral dust in the Earth system, Aeol. Res., 60, 100849, https://doi.org/10.1016/j.aeolia.2022.100849, 2023.
Baddock, M., Boskovic, L., Strong, C., McTainsh, G., Bullard, J., Agranovski, I., and Cropp, R.: Iron–rich nanoparticles formed by aeolian abrasion of desert dune sand, Geochem. Geophys. Geosyst., 14, 3720–3729, https://doi.org/10.1002/ggge.20229, 2013.
Bates, T. S., Coffman, D. J., Covert, D. S., and Quinn, P. K.: Regional marine boundary layer aerosol size distributions in the Indian, Atlantic, and Pacific Oceans: A comparison of INDOEX measurements with ACE–1, ACE–2, and Aerosols99, J. Geophys. Res., 107, INX2 25–1–INX2 25–15, https://doi.org/10.1029/2001JD001174, 2002.
Chen, G., Ziemba, L. D., Chu, D. A., Thornhill, K. L., Schuster, G. L., Winstead, E. L., Diskin, G. S., Ferrare, R. A., Burton, S. P., Ismail, S., Kooi, S. A., Omar, A. H., Slusher, D. L., Kleb, M. M., Reid, J. S., Twohy, C. H., Zhang, H., and Anderson, B. E.: Observations of Saharan dust microphysical and optical properties from the Eastern Atlantic during NAMMA airborne field campaign, Atmos. Chem. Phys., 11, 723–740, https://doi.org/10.5194/acp-11-723-2011, 2011.
Chou, C., Formenti, P., Maille, M., Ausset, P., Helas, G., Harrison, M., and Osborne, S.: Size distribution, shape, and composition of mineral dust aerosols collected during the African Monsoon Multidisciplinary Analysis Special Observation Period 0: Dust and Biomass-Burning Experiment field campaign in Niger, J. Geophys. Res., 113, D00C10, https://doi.org/10.1029/2008JD009897, 2008.
Claquin, T., Schulz, M., and Balkanski, Y.: Modeling the mineralogy of atmospheric dust sources, J. Geophys. Res., 104, 22243–22256, 1999.
Clarke, A. D., Shinozuka, Y., Kapustin, V. N., Howell, S., Huebert, B., Doherty, S., Anderson, T., Covert, D., Anderson, J., Hua, X., Moore, K. G., McNaughton, C., Carmichael, G., and Weber, R.: Size distributions and mixtures of dust and black carbon aerosol in Asian outflow: Physiochemistry and optical properties, J. Geophys. Res., 109, D15S09, https://doi.org/10.1029/2003JD004378, 2004.
Cuesta, J., Eremenko, M., Flamant, C., Dufour, G., Laurent, B., Bergametti, G., Hopfner, M., Orphal, J., and Zhou, D.: Three-dimensional distribution of a major desert dust outbreak over East Asia in March 2008 derived from IASI satellite observations, J. Geophys. Res., 120, 7099–7127, 2015.
d'Almeida, G. A.: On the variability of desert aerosol radiative characteristics, J. Geophys. Res.-Atmos., 92, 3017–3026, https://doi.org/10.1029/JD092iD03p03017, 1987.
DeCarlo, P. F., Slowik, J. G., Worsnop, D. R., Davidovits, P., and Jimenez, J. L.: Particle Morphology and Density Characterization by Combined Mobility and Aerodynamic Diameter Measurements. Part 1: Theory, Aerosol Sci. Technol., 38, 1185–1205, https://doi.org/10.1080/027868290903907, 2004.
Denjean, C., Cassola, F., Mazzino, A., Triquet, S., Chevaillier, S., Grand, N., Bourrianne, T., Momboisse, G., Sellegri, K., Schwarzenbock, A., Freney, E., Mallet, M., and Formenti, P.: Size distribution and optical properties of mineral dust aerosols transported in the western Mediterranean, Atmos. Chem. Phys., 16, 1081–1104, https://doi.org/10.5194/acp-16-1081-2016, 2016a.
Denjean, C., Formenti, P., Desboeufs, K., Chevaillier, S., Triquet, S., Maillé, M., Cazaunau, M., Laurent, B., Mayol-Bracero, O. L., Vallejo, P., Quiñones, M., Gutierrez-Molina, I. E., Cassola, F., Prati, P., Andrews, E., and Ogren, J.: Size distribution and optical properties of African mineral dust after intercontinental transport, J. Geophys. Res., 121, 7117–7138, https://doi.org/10.1002/2016JD024783, 2016b.
de Reus, M., Dentener, F., Thomas, A., Borrmann, S., Ström, J., and Lelieveld, J.: Airborne observations of dust aerosol over the North Atlantic Ocean during ACE 2: Indications for heterogeneous ozone destruction, J. Geophys. Res., 105, 15263–15275, https://doi.org/10.1029/2000JD900164, 2000.
Di Biagio, C., Formenti, P., Balkanski, Y., Caponi, L., Cazaunau, M., Pangui, E., Journet, E., Nowak, S., Andreae, M. O., Kandler, K., Saeed, T., Piketh, S., Seibert, D., Williams, E., and Doussin, J.-F.: Complex refractive indices and single-scattering albedo of global dust aerosols in the shortwave spectrum and relationship to size and iron content, Atmos. Chem. Phys., 19, 15503–15531, https://doi.org/10.5194/acp-19-15503-2019, 2019.
Di Biagio, C., Balkanski, Y., Albani, S., Boucher, O., and Formenti, P.: Direct radiative effect by mineral dust aerosols constrained by new microphysical and spectral optical data, Geophys. Res. Lett., 47, e2019GL086186, https://doi.org/10.1029/2019GL086186, 2020.
Di Biagio, C., Doussin, J. F., Cazaunau, M., Pangui, E., Cuesta, J., Sellitto, P., Rodenas, M., and Formenti, P.: Infrared optical signature reveals the source–dependency and along–transport evolution of dust mineralogy as shown by laboratory study, Sci. Rep., 13, 13252, https://doi.org/10.1038/s41598-023-39336-7, 2023.
Dubovik, O., Holben, B. N., Eck, T. F., Smirnov, A., Kaufman, Y. J., King, M. D., Tanre, D., and Slutsker, I.: Variability of absorption and optical properties of key aerosol types observed in worldwide locations, J. Atmos. Sci., 59, 590–608, https://doi.org/10.1175/1520-0469(2002)059<0590:VOAAOP>2.0.CO;2, 2002.
Dubovik, O., Sinyuk, A., Lapyonok, T., Holben, B. N., Mishchenko, M., Yang, P., Eck, T. F., Volten, H., Muñoz, O., Veihelmann, B., van der Zande, W. J., Leon, J.-F., Sorokin, M., and Slutsker, I.: Application of spheroid models to account for aerosol particle nonsphericity in remote sensing of desert dust, J. Geophys. Res., 111, D11208, https://doi.org/10.1029/2005JD006619, 2006.
Formenti, P. and Di Biagio, C.: Large synthesis of in situ field measurements of the size distribution of mineral dust aerosols across their lifecycle-SOURCE, EaSy Data [data set], https://doi.org/10.57932/58dbe908-9394-4504-9099-74a3e77140e9, 2023a.
Formenti, P. and Di Biagio, C.: Large synthesis of in situ field measurements of the size distribution of mineral dust aerosols across their lifecycle-MRT, EaSy Data [data set], https://doi.org/10.57932/31f2adf7-74fb-48e8-a3ef-059f663c47f1, 2023b.
Formenti, P. and Di Biagio, C.: Large synthesis of in situ field measurements of the size distribution of mineral dust aerosols across their lifecycle-LRT, EaSy Data [data set], https://doi.org/10.57932/17dc781c-3e9d-4908-85b5-5c99e68e8f79, 2023c.
Formenti, P., Andreae, M. O., Lange, L., Roberts, G., Cafmeyer, J., Rajta, I., Maenhaut, W., Holben, B. N., Artaxo, P., and Lelieveld, J.: Saharan dust in Brazil and Suriname during the Large–Scale Biosphere–Atmosphere Experiment in Amazonia (LBA) – Cooperative LBA Regional Experiment (CLAIRE) in March 1998, J. Geophys. Res., 106, 14919–14934, https://doi.org/10.1029/2000JD900827, 2001.
Formenti, P., Elbert, W., Maenhaut, W., Haywood, J., and Andreae, M. O.: Chemical composition of mineral dust aerosol during the Saharan Dust Experiment (SHADE) airborne campaign in the Cape Verde region, September 2000, J. Geophys. Res., 108, 8576, https://doi.org/10.1029/2002JD002648, 2003.
Formenti, P., Rajot, J. L., Desboeufs, K., Saïd, F., Grand, N., Chevaillier, S., and Schmechtig, C.: Airborne observations of mineral dust over western Africa in the summer Monsoon season: spatial and vertical variability of physico-chemical and optical properties, Atmos. Chem. Phys., 11, 6387–6410, https://doi.org/10.5194/acp-11-6387-2011, 2011.
Fratini, G., Ciccioli, P., Febo, A., Forgione, A., and Valentini, R.: Size-segregated fluxes of mineral dust from a desert area of northern China by eddy covariance, Atmos. Chem. Phys., 7, 2839–2854, https://doi.org/10.5194/acp-7-2839-2007, 2007.
Gillette, D. A.: On the production of soil wind erosion having the potential for long range transport, J. Rech. Atmos., 8, 734–744, 1974.
Gillette, D. A., Blifford, I. H., and Fenster, C. R.: Measurements of Aerosol Size Distributions and Vertical Fluxes of Aerosols on Land Subject to Wind Erosion, J. Appl. Meteor., 11, 977–987, https://doi.org/10.1175/1520-0450(1972)011<0977:MOASDA>2.0.CO;2, 1972.
Gillette, D. A., Blifford, I. H., and Fryrear, D. W.: The influence of wind velocity on the size distributions of aerosols generated by the wind erosion of soils, J. Geophys. Res., 79, 4068–4075, https://doi.org/10.1029/JC079i027p04068, 1974.
Gomes, L., Bergametti, G., Coudé-Gaussen, G., and Rognon, P.: Submicron Desert Dusts: A Sandblasting Process, J. Geophys. Res., 95, 927–940, 1990.
Gómez Maqueo Anaya, S., Althausen, D., Faust, M., Baars, H., Heinold, B., Hofer, J., Tegen, I., Ansmann, A., Engelmann, R., Skupin, A., Heese, B., and Schepanski, K.: The implementation of dust mineralogy in COSMO5.05-MUSCAT, Geosci. Model Dev., 17, 1271–1295, https://doi.org/10.5194/gmd-17-1271-2024, 2024.
González-Flórez, C., Klose, M., Alastuey, A., Dupont, S., Escribano, J., Etyemezian, V., Gonzalez-Romero, A., Huang, Y., Kandler, K., Nikolich, G., Panta, A., Querol, X., Reche, C., Yus-Díez, J., and Pérez García-Pando, C.: Insights into the size-resolved dust emission from field measurements in the Moroccan Sahara, Atmos. Chem. Phys., 23, 7177–7212, https://doi.org/10.5194/acp-23-7177-2023, 2023.
Gonçalves Ageitos, M., Obiso, V., Miller, R. L., Jorba, O., Klose, M., Dawson, M., Balkanski, Y., Perlwitz, J., Basart, S., Di Tomaso, E., Escribano, J., Macchia, F., Montané, G., Mahowald, N. M., Green, R. O., Thompson, D. R., and Pérez García-Pando, C.: Modeling dust mineralogical composition: sensitivity to soil mineralogy atlases and their expected climate impacts, Atmos. Chem. Phys., 23, 8623–8657, https://doi.org/10.5194/acp-23-8623-2023, 2023.
reen, R. O., Mahowald, N., Ung, C., Thompson, D. R., Bator, L., and Bennet, M.: The earth surface mineral dust source investigation: an earth science imaging spectroscopy mission, in: 2020 IEEE Aerospace Conference, 1–15, https://doi.org/10.1109/AERO47225.2020.9172731, 2020.
Hinds, W. C.: Aerosol technology: properties, behavior, and mea- surement of airborne particles, John Wiley & Sons, Chichester, 504 pp., 1999.
Huang, Y., Kok, J. F., Martin, R. L., Swet, N., Katra, I., Gill, T. E., Reynolds, R. L., and Freire, L. S.: Fine dust emissions from active sands at coastal Oceano Dunes, California, Atmos. Chem. Phys., 19, 2947–2964, https://doi.org/10.5194/acp-19-2947-2019, 2019.
Huang, Y., Adebiyi, A. A., Formenti, P., and Kok, J. F.: Linking the different diameter types of aspherical desert dust indicates that models underestimate coarse dust emission, Geophys. Res. Lett., 48, e2020GL092054, https://doi.org/10.1029/2020GL092054, 2021.
Huneeus, N., Schulz, M., Balkanski, Y., Griesfeller, J., Prospero, J., Kinne, S., Bauer, S., Boucher, O., Chin, M., Dentener, F., Diehl, T., Easter, R., Fillmore, D., Ghan, S., Ginoux, P., Grini, A., Horowitz, L., Koch, D., Krol, M. C., Landing, W., Liu, X., Mahowald, N., Miller, R., Morcrette, J.-J., Myhre, G., Penner, J., Perlwitz, J., Stier, P., Takemura, T., and Zender, C. S.: Global dust model intercomparison in AeroCom phase I, Atmos. Chem. Phys., 11, 7781–7816, https://doi.org/10.5194/acp-11-7781-2011, 2011.
Johnson, B. T. and Osborne, S. R.: Physical and optical properties of mineral dust aerosol measured by aircraft during the GERBILS campaign, Q. J. Roy. Meteor. Soc., 137, 1117–1130, https://doi.org/10.1002/qj.777, 2011.
Journet, E., Balkanski, Y., and Harrison, S. P.: A new data set of soil mineralogy for dust-cycle modeling, Atmos. Chem. Phys., 14, 3801–3816, https://doi.org/10.5194/acp-14-3801-2014, 2014.
Kandler, K., Benker, N., Bundke, U., Cuevas, E., Ebert, M., Knippertz, P., Rodriguez, S., Schütz, L., and Weinbruch, S.: Chemical composition and complex refractive index of Saharan mineral dust at Izana, Tenerife (Spain) derived by electron microscopy, Atmos. Environ., 41, 8058–8074, 2007.
Kandler, K., Schütz, L., Deutscher, C., Ebert, M., Hofmann, H., Jäckel, S., Jaenicke, R., Knippertz, P., Lieke, K., Massling, A., Petzold, A., Schladitz, A., Weinzierl, B., Wiedensohler, A., Zorn, S., and Weinbruch, S.: Size distribution, mass concentration, chemical and mineralogical composition and derived optical parameters of the boundary layer aerosol at Tinfou, Morocco, during SAMUM 2006, Tellus B, 61, 32–50, https://doi.org/10.1111/j.1600-0889.2008.00385.x, 2009.
Kandler, K., Schütz, L., Jäckel, S., Lieke, K., Emmel, C., Müller–Ebert, D., Ebert, M., Scheuvens, D., Schladitz, A., Šegvić, B., Wiedensohler, A., and Weinbruch, S.: Ground-based off-line aerosol measurements at Praia, Cape Verde, during the Saharan Mineral Dust Experiment: microphysical properties and mineralogy, Tellus B, 63, 459–474, https://doi.org/10.1111/j.1600-0889.2011.00546.x, 2011.
Khalfallah, B., Bouet, C., Labiadh, M. T., Alfaro, S. C., Bergametti, G., Marticorena, B., Lafon, S., Chevaillier, S., Féron, A., Hease, P., Tureaux, T. H. des, Sekrafi, S., Zapf, P., and Rajot, J. L.: Influence of Atmospheric Stability on the Size Distribution of the Vertical Dust Flux Measured in Eroding Conditions Over a Flat Bare Sandy Field, J. Geophys. Res.-Atmos., 125, e2019JD031185, https://doi.org/10.1029/2019JD031185, 2020.
Klaver, A., Formenti, P., Caquineau, S., Chevaillier, S., Ausset, P., Calzolai, G., Osborne, S., Johnson, B., Harrison, M., and Dubovik, O.: Physico-chemical and optical properties of Sahelian and Saharan mineral dust: in situ measurements during the GERBILS campaign, Q. J. Roy. Meteor. Soc., 137, 1193–1210, https://doi.org/10.1002/qj.889, 2011.
Knippertz, P. and Stuut, J.-B. W. (Eds.): Mineral Dust: A Key Player in the Earth System, Springer Netherlands, https://doi.org/10.1007/978-94-017-8978-3, 2014.
Kobayashi, H., Arao, K., Murayama, T., Iokibe, K., Koga, R., and Shiobara, M.: High–Resolution Measurement of Size Distributions of Asian Dust Using a Coulter Multisizer, J. Atmos. Ocean. Tech., 24, 194–205, https://doi.org/10.1175/JTECH1965.1, 2007.
Kok, J. F.: A scaling theory for the size distribution of emitted dust aerosols suggests climate models underestimate the size of the global dust cycle, P. Natl. Acad. Sci. USA, 108, 1016–1021, 2011.
Kok, J. F., Ridley, D. A., Zhou, Q., Miller, R. L., Zhao, C., Heald, C. L., Ward, D. S., Albani, S., and Haustein, K.: Smaller desert dust cooling effect estimated from analysis of dust size and abundance, Nat. Geo., 10, 274–278, https://doi.org/10.1038/ngeo2912, 2017.
Mahowald, N., Lindsay, K., Rothenberg, D., Doney, S. C., Moore, J. K., Thornton, P., Randerson, J. T., and Jones, C. D.: Desert dust and anthropogenic aerosol interactions in the Community Climate System Model coupled-carbon-climate model, Biogeosciences, 8, 387–414, https://doi.org/10.5194/bg-8-387-2011, 2011.
Mahowald, N., Albani, S., Kok, J. F., Engelstaeder, S., Scanza, R., Ward, D. S., and Flanner, M. G.: The size distribution of desert dust aerosols and its impact on the Earth system, Aeolian Res., 15, 53–71, 2014.
Marin, F., Rohatgi, A., and Charlot, S.: WebPlotDigitizer, a polyvalent and free software to extract spectra from old astronomical publications: application to ultraviolet spectropolarimetry, arXiv [preprint] arXiv:1708.02025, https://doi.org/10.48550/arXiv.1708.02025, 2017 (code available at: https://automeris.io/WebPlotDigitizer/, last access: 23 October 2024).
Maring, H., Savoie, D. L., Izaguirre, M. A., McCormick, C., Arimoto, R., Prospero, J. M., and Pilinis, C.: Aerosol physical and optical properties and their relationship to aerosol composition in the free troposphere at Izaña, Tenerife, Canary Islands, during July 1995, J. Geophys. Res., 105, 14677–14700, https://doi.org/10.1029/2000JD900106, 2000.
Maring, H., Savoie, D. L., Izaguirre, M. A., Custals, L., and Reid, J. S.: Mineral dust aerosol size distribution change during atmospheric transport, J. Geophys. Res., 108, 8592, https://doi.org/10.1029/2002JD002536, 2003.
McConnell, C. L., Highwood, E. J., Coe, H., Formenti, P., Anderson, B., Osborne, S., Nava, S., Desboeufs, K., Chen, G., and Harrison, M. A. J.: Seasonal variations of the physical and optical characteristics of Saharan dust: Results from the Dust Outflow and Deposition to the Ocean (DODO) experiment, J. Geophys. Res., 113, D14S05, https://doi.org/10.1029/2007JD009606, 2008.
Meng, J., Huang, Y., Leung, D. M., Li, L., Adebiyi, A. A., Ryder, C. L., Mahowald, N. M., and Kok, J. F.: Improved Parameterization for the Size Distribution of Emitted Dust Aerosols Reduces Model Underestimation of Super Coarse Dust, Geophys. Res. Lett., 49, e2021GL097287, https://doi.org/10.1029/2021GL097287, 2022.
Menut, L., Siour, G., Bessagnet, B., Couvidat, F., Journet, E., Balkanski, Y., and Desboeufs, K.: Modelling the mineralogical composition and solubility of mineral dust in the Mediterranean area with CHIMERE 2017r4, Geosci. Model Dev., 13, 2051–2071, https://doi.org/10.5194/gmd-13-2051-2020, 2020.
Müller, K., Lehmann, S., van Pinxteren, D., Gnauk, T., Niedermeier, N., Wiedensohler, A., and Herrmann, H.: Particle characterization at the Cape Verde atmospheric observatory during the 2007 RHaMBLe intensive, Atmos. Chem. Phys., 10, 2709–2721, https://doi.org/10.5194/acp-10-2709-2010, 2010.
Osborne, S. R., Johnson, B. T., Haywood, J. M., Baran, A. J., Harrison, M. A. J., and McConnell, C. L.: Physical and optical properties of mineral dust aerosol during the Dust and Biomass–burning Experiment, J. Geophys. Res., 113, D00C03, https://doi.org/10.1029/2007JD009551, 2008.
Otto, S., de Reus, M., Trautmann, T., Thomas, A., Wendisch, M., and Borrmann, S.: Atmospheric radiative effects of an in situ measured Saharan dust plume and the role of large particles, Atmos. Chem. Phys., 7, 4887–4903, https://doi.org/10.5194/acp-7-4887-2007, 2007.
Perlwitz, J. P., Pérez García-Pando, C., and Miller, R. L.: Predicting the mineral composition of dust aerosols – Part 1: Representing key processes, Atmos. Chem. Phys., 15, 11593–11627, https://doi.org/10.5194/acp-15-11593-2015, 2015a.
Perlwitz, J. P., Pérez García-Pando, C., and Miller, R. L.: Predicting the mineral composition of dust aerosols – Part 2: Model evaluation and identification of key processes with observations, Atmos. Chem. Phys., 15, 11629–11652, https://doi.org/10.5194/acp-15-11629-2015, 2015b.
Rajot, J. L., Formenti, P., Alfaro, S., Desboeufs, K., Chevaillier, S., Chatenet, B., Gaudichet, A., Journet, E., Marticorena, B., Triquet, S., Maman, A., Mouget, N., and Zakou, A.: AMMA dust experiment: An overview of measurements performed during the dry season special observation period (SOP0) at the Banizoumbou (Niger) supersite, J. Geophys. Res., 113, D00C14, https://doi.org/10.1029/2008JD009906, 2008.
Reid, E. A., Reid, J. S., Meier, M. M., Dunlap, M. R., Cliff, S. S., Broumas, A., Perry, K., and Maring, H.: Characterization of African dust transported to Puerto Rico by individual particle and size segregated bulk analysis, J. Geophys. Res., 108, 8591, https://doi.org/10.1029/2002JD002935, 2003.
Reid, J. S., Jonsson, H. H., Maring, H. B., Smirnov, A., Savoie, D. L., Cliff, S. S., Reid, E. A., Livingston, J. M., Meier, M. M., Dubovik, O., and Tsay, S.-C.: Comparison of size and morphological measurements of coarse mode dust particles from Africa, J. Geophys. Res., 108, 8593, https://doi.org/10.1029/2002JD002485, 2003.
Reid, J. S., Reid, E. A., Walker, A., Piketh, S., Cliff, S., Mandoos, A. A., Tsay, S.–C., and Eck, T. F.: Dynamics of southwest Asian dust particle size characteristics with implications for global dust research, J. Geophys. Res., 113, D14212, https://doi.org/10.1029/2007JD009752, 2008.
Renard, J.-B., Dulac, F., Durand, P., Bourgeois, Q., Denjean, C., Vignelles, D., Couté, B., Jeannot, M., Verdier, N., and Mallet, M.: In situ measurements of desert dust particles above the western Mediterranean Sea with the balloon-borne Light Optical Aerosol Counter/sizer (LOAC) during the ChArMEx campaign of summer 2013, Atmos. Chem. Phys., 18, 3677–3699, https://doi.org/10.5194/acp-18-3677-2018, 2018.
Rosenberg, P. D., Parker, D. J., Ryder, C. L., Marsham, J. H., Garcia-Carreras, L., Dorsey, J. R., Brooks, I. M., Dean, A. R., Crosier, J., McQuaid, J. B., and Washington, R.: Quantifying particle size and turbulent scale dependence of dust flux in the Sahara using aircraft measurements, J. Geophys. Res.-Atmos., 119, 7577–7598, https://doi.org/10.1002/2013JD021255, 2014.
Ryder, C. L., Highwood, E. J., Lai, T. M., Sodemann, H., and Marsham, J. H.: Impact of atmospheric transport on the evolution of microphysical and optical properties of Saharan dust, Geophys. Res. Lett., 40, 2433–2438, https://doi.org/10.1002/grl.50482, 2013a.
Ryder, C. L., Highwood, E. J., Rosenberg, P. D., Trembath, J., Brooke, J. K., Bart, M., Dean, A., Crosier, J., Dorsey, J., Brindley, H., Banks, J., Marsham, J. H., McQuaid, J. B., Sodemann, H., and Washington, R.: Optical properties of Saharan dust aerosol and contribution from the coarse mode as measured during the Fennec 2011 aircraft campaign, Atmos. Chem. Phys., 13, 303–325, https://doi.org/10.5194/acp-13-303-2013, 2013b.
Ryder, C. L., McQuaid, J. B., Flamant, C., Rosenberg, P. D., Washington, R., Brindley, H. E., Highwood, E. J., Marsham, J. H., Parker, D. J., Todd, M. C., Banks, J. R., Brooke, J. K., Engelstaedter, S., Estelles, V., Formenti, P., Garcia-Carreras, L., Kocha, C., Marenco, F., Sodemann, H., Allen, C. J. T., Bourdon, A., Bart, M., Cavazos-Guerra, C., Chevaillier, S., Crosier, J., Darbyshire, E., Dean, A. R., Dorsey, J. R., Kent, J., O'Sullivan, D., Schepanski, K., Szpek, K., Trembath, J., and Woolley, A.: Advances in understanding mineral dust and boundary layer processes over the Sahara from Fennec aircraft observations, Atmos. Chem. Phys., 15, 8479–8520, https://doi.org/10.5194/acp-15-8479-2015, 2015.
Ryder, C. L., Marenco, F., Brooke, J. K., Estelles, V., Cotton, R., Formenti, P., McQuaid, J. B., Price, H. C., Liu, D., Ausset, P., Rosenberg, P. D., Taylor, J. W., Choularton, T., Bower, K., Coe, H., Gallagher, M., Crosier, J., Lloyd, G., Highwood, E. J., and Murray, B. J.: Coarse-mode mineral dust size distributions, composition and optical properties from AER-D aircraft measurements over the tropical eastern Atlantic, Atmos. Chem. Phys., 18, 17225–17257, https://doi.org/10.5194/acp-18-17225-2018, 2018.
Scanza, R. A., Mahowald, N., Ghan, S., Zender, C. S., Kok, J. F., Liu, X., Zhang, Y., and Albani, S.: Modeling dust as component minerals in the Community Atmosphere Model: development of framework and impact on radiative forcing, Atmos. Chem. Phys., 15, 537–561, https://doi.org/10.5194/acp-15-537-2015, 2015.
Schütz, L., Jaenicke, R. and Pietrek, H., Saharan Dust Transport over the North Atlantic Ocean, in: Desert Dust, edited by: Péwé, T. L., Geological Society of America, Boulder, Special Paper, vol. 186, 87–100, https://doi.org/10.1130/SPE186-p87, 1981.
Shao, Y., Ishizuka, M., Mikami, M., and Leys, J. F.: Parameterization of size-resolved dust emission and validation with measurements, J. Geophys. Res., 116, D08203, https://doi.org/10.1029/2010JD014527, 2011.
Sow, M., Alfaro, S. C., Rajot, J. L., and Marticorena, B.: Size resolved dust emission fluxes measured in Niger during 3 dust storms of the AMMA experiment, Atmos. Chem. Phys., 9, 3881–3891, https://doi.org/10.5194/acp-9-3881-2009, 2009.
Struckmeier, C., Drewnick, F., Fachinger, F., Gobbi, G. P., and Borrmann, S.: Atmospheric aerosols in Rome, Italy: sources, dynamics and spatial variations during two seasons, Atmos. Chem. Phys., 16, 15277–15299, https://doi.org/10.5194/acp-16-15277-2016, 2016.
Varga, G., Dagsson-Waldhauserová, P., Gresina, F., and Helgadottir, A.: Saharan dust and giant quartz particle transport towards Iceland, Sci. Rep., 11, 11891, https://doi.org/10.1038/s41598-021-91481-z, 2021.
van der Does, M., Knippertz, P., Zschenderlein, P., Giles Harrison, R., and Stuut, J.-B. W.: The mysterious long-range transport of giant mineral dust particles, Sci. Adv., 4, eaau2768, https://doi.org/10.1126/sciadv.aau2768, 2018.
Wagner, F., Bortoli, D., Pereira, S., Costa, M. Jo., Silva, A. M., Weinzierl, B., Esselborn, M., Petzold, A., Rasp, K., Heinold, B., and Tegen, I.: Properties of dust aerosol particles transported to Portugal from the Sahara desert, Tellus B, 61, 297–306, https://doi.org/10.1111/j.1600-0889.2008.00393.x, 2009.
Weinzierl, B., Petzold, A., Esselborn, M., Wirth, M., Rasp, K., Kandler, K., Schütz, L., Koepke, P., and Fiebig, M.: Airborne measurements of dust layer properties, particle size distribution and mixing state of Saharan dust during SAMUM 2006, Tellus B, 61, 96–117, https://doi.org/10.1111/j.1600-0889.2008.00392.x, 2009.
Weinzierl, B., Sauer, D., Esselborn, M., Petzold, A., Veira, A., Rose, M., Mund, S., Wirth, M., Ansmann, A., Tesche, M., Gross, S., and Freudenthaler, V.: Microphysical and optical properties of dust and tropical biomass burning aerosol layers in the Cape Verde region – an overview of the airborne in situ and lidar measurements during SAMUM–2, Tellus B, 63, 589–618, https://doi.org/10.1111/j.1600-0889.2011.00566.x, 2011.
Weinzierl, B., Ansmann, A., Prospero, J. M., Althausen, D., Benker, N., Chouza, F., Dollner, M., Farrell, D., Fomba, W. K., Freudenthaler, V., Gasteiger, J., Groß, S., Haarig, M., Heinold, B., Kandler, K., Kristensen, T. B., Mayol–Bracero, O. L., Müller, T., Reitebuch, O., Sauer, D., Schäfler, A., Schepanski, K., Spanu, A., Tegen, I., Toledano, C., and Walser, A.: The Saharan Aerosol Long-Range Transport and Aerosol–Cloud-Interaction Experiment: Overview and Selected Highlights, B. Am. Meteorol. Soc., 98, 1427–1451, https://doi.org/10.1175/BAMS-D-15-00142.1, 2017.
Wendisch, M. and Brenguier, J.-L. (Eds.): Airborne measurements for environmental research: methods and instruments, Wiley-VCH Verlag GmbH & Co. KGaA, Weinheim, Germany, ISBN 978-3-527-40996-9, 2013.
Zhao, A., Ryder, C. L., and Wilcox, L. J.: How well do the CMIP6 models simulate dust aerosols?, Atmos. Chem. Phys., 22, 2095–2119, https://doi.org/10.5194/acp-22-2095-2022, 2022.
Zhou, Y., Levy, R. C., Remer, L. A., Mattoo, S., Shi, Y., and Wang, C.: Dust Aerosol Retrieval over the Oceans with the MODIS/VIIRS Dark Target algorithm. Part I: Dust Detection, Earth Space Sci., e2020EA001221, https://doi.org/10.1029/2020EA001221, 2020.