the Creative Commons Attribution 4.0 License.
the Creative Commons Attribution 4.0 License.
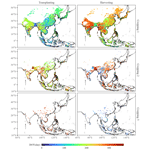
Monsoon Asia Rice Calendar (MARC): a gridded rice calendar in monsoon Asia based on Sentinel-1 and Sentinel-2 images
Xin Zhao
Haruka Izumisawa
Yuji Masutomi
Seima Osako
Shuhei Yamamoto
An accurate and spatially explicit large-scale rice calendar can enhance the understanding of agricultural practices and their ecological services, particularly in monsoon Asia. However, currently available global- or continental-scale rice calendars suffer from coarse resolution, poor recording, and outdated information; thus, they do not provide detailed and consistent information on rice phenology. To address this limitation, this study mapped a new (2019–2020) gridded (0.5°×0.5° resolution) rice calendar for monsoon Asia based on Sentinel-1 and Sentinel-2 satellite images. The novelty of this rice calendar lies in the development of a consistent optimal methodological framework that allows the spatially explicit characterization of the rice transplanting date, harvesting date, and number of rice cropping seasons. The methodological framework incorporates two steps: (1) detection of rice phenological dates and number of rice cropping seasons via the combination of a feature-based algorithm and the fitted Weibull function; (2) spatiotemporal integration of the detected transplanting and harvesting dates derived from Step 1 using von Mises maximum likelihood estimates. Results revealed that the proposed rice calendar can accurately identify the rice phenological dates for three croppings in monsoon Asia. When compared with single rice data from the census-based RiceAtlas calendar, the proposed calendar exhibited better results than the MODIS-based Rice Crop Calendar for Asia (RICA). It exhibited biases of 4 and −6 d for the transplanting and harvesting dates, respectively, with 10 and 15 d lower MAE values and 6 and 15 d lower RMSE values for the transplanting and harvesting dates, respectively. In total, the proposed rice calendar can detect single-, double-, and triple-rice-cropping seasons with an area of 0.53×106, 0.45×106, and 0.09×106 km2, respectively. This novel gridded rice calendar fills the gaps in 0.5° rice calendars across major global rice production areas, facilitating research on rice phenology that is relevant to climate change. The developed gridded Monsoon Asia Rice Calendar (MARC) is available at https://doi.org/10.17595/20230728.001 (Zhao and Nishina, 2023).
- Article
(15014 KB) - Full-text XML
-
Supplement
(2382 KB) - BibTeX
- EndNote
A rice calendar records a series of phenological dates for rice growth and indicates the number of times that rice is grown in a year (Mishra et al., 2021; Zhao et al., 2023). Rice calendars provide critical information that contributes to agricultural management, crop production prediction, and the estimation of greenhouse gas (GHG) emissions (Laborte et al., 2017; Portmann et al., 2010; Sacks et al., 2010). Specifically, concern regarding the negative impacts of rice cultivation is increasing because irrigated rice paddy fields are an important source of anthropogenic GHG emissions, contributing 8 % and 11 % of global methane and nitrous oxide emissions, respectively (Saunois et al., 2020; Jiang et al., 2019). The inundated period from the transplanting date to the harvesting date derived from a rice calendar largely determines the quantity of GHG emissions (Ito et al., 2022). To accurately estimate GHG emissions related to rice cultivation and to establish appropriate reduction measures, a detailed rice calendar that depicts rice phenology dynamics is urgently needed, especially for monsoon Asia, which accounts for 87 % of the area of harvested rice globally and for 90 % of global rice production (FAOSTAT, 2022).
Existing approaches to rice calendar mapping can be grouped into three categories: those based on census data, those based on models, and those based on remote-sensing images. The scarce global rice calendars, e.g., SAGE (Sacks et al., 2010), MIRCA2000 (Portmann et al., 2010), and RiceAtlas (Laborte et al., 2017), that are currently available rely on a compilation of statistical data at national and/or subnational scales. Model-based rice calendars (Waha et al., 2012; Elliott et al., 2015; Mathison et al., 2018; Iizumi et al., 2019) provide large-scale spatially explicit rice phenology information that is mainly based on climate data, but they are difficult to validate using Earth observation data over such scales (Mishra et al., 2021). In contrast, remote-sensing approaches that can provide consistent detection of large-scale rice phenological change over time have been used for rice calendar mapping with varying spatial coverage, e.g., global (Kotsuki and Tanaka, 2015), Asia (Mishra et al., 2021; Zhang et al., 2022), South and Southeast Asia (More et al., 2016), China (Luo et al., 2020), and Japan (Sakamoto et al., 2005).
However, many challenges hinder the production of an accurate rice calendar using remote-sensing approaches. First, use of a coarse- to moderate-resolution satellite sensor (e.g., the Advanced Very High Resolution Radiometer, AVHRR, with an approximate 5 km resolution and MODIS with a 500 m resolution) or any single sensor (optical or synthetic aperture radar – SAR) diminishes the accuracy of rice calendar mapping. The Rice Crop Calendar for Asia (RICA), produced using MODIS images, faces issues with rice paddy field sizes smaller than the 500 m sensor resolution (Mishra et al., 2021). Second, the rule-based algorithm currently in use for rice phenological date extraction depends on turning points or key nodes of vegetation indices and backscattering (Xin et al., 2020), which are affected by the smoothing method and the parameters adopted. Additionally, existing algorithms like ChinaCropPhen1km for China (Luo et al., 2020) and Enhanced-Vegetation-Index-related methods for Japan (Sakamoto et al., 2005) are limited to specific administrative areas. Some alternative algorithms like PhenoRice (Mishra et al., 2021) and Leaf-Area-Index-related approaches (Zhang et al., 2022) aim to map rice phenology over large areas but may ignore the fine heterogeneity within administrative units. Third, determination of the number of rice cropping seasons is frequently biased. For example, some recent studies have focused only on the main rice cropping (Zhang et al., 2022) or have determined rice cropping during specific time windows (Mishra et al., 2021), thereby excluding rice grown in other periods. Additionally, rice sometimes grows across years because the double- or triple-rice-cropping seasons make it difficult to determine the actual number of rice cropping seasons. Although methods have been proposed to extract the number of cropping seasons via growing season peak detection (Kotsuki and Tanaka, 2015; Yan et al., 2019), much effort is required to reduce the uncertainty of bias in the peak caused by ratoons and/or noisy data (Liu et al., 2020).
The combination of optical and SAR sensors, utilizing the high spatial (10 m) and temporal (6 d for Sentinel-1 or 5 d for Sentinel-2) resolution of Copernicus Sentinel images, benefits crop phenology monitoring by offering precise and timely information on phenological variations. A feature-based algorithm, proposed for large-scale rice phenology detection (Zhao et al., 2023), excels with respect to utilizing backscattering (VH) and vegetation indices (Enhanced Vegetation Index – EVI; Normalized Difference Yellow Index – NDYI) derived from Sentinel-1 and Sentinel-2 images to reflect features related to rice cultivation, such as flooding, maximum leaf area, and peak yellowness around transplanting, heading, and harvesting date. Additionally, this algorithm has successfully tracked rice phenological dates of different cropping seasons (single-, double-, and triple-cropping seasons) and at different spatial scales (subnational, 0.5° grid cell, and site scales) (Zhao et al., 2023). Meanwhile, a fitted six-parameter Weibull function has successfully been adopted to depict the growth development of phytoplankton (Rolinski et al., 2007) and vegetation (Maciel-Nájera et al., 2020; Muñoz-Salazar et al., 2022). As variation in greenness is a reasonable indicator of crop intensity, the ability of a fitted Weibull function to fit the beginning, peak, and end of the greenness cycle allows it to capture the number of rice cropping seasons. Different from most widely used peak greenness detection methods, which depend on thresholds, derivatives, or inflection points for detection (Xin et al., 2020; Yang et al., 2020), the fitted Weibull function omits the noisy peaks, which means it can track the shape of the vegetation index time series. Moreover, the fitted Weibull function has been packaged in the R software, making detection of the number of rice cropping seasons automatic. Therefore, a feature-based algorithm combined with a fitted Weibull function is suitable for extracting rice phenological dates and the number of rice cropping seasons.
The objective of this study was to develop a new gridded rice calendar that highlights the following features: (a) consistent detection using remote-sensing methods, (b) spatial resolution (0.5° × 0.5°), (c) large-scale coverage (monsoon Asia), and (d) the ability to extract multiple rice cropping seasons. To achieve this goal, Sentinel-1 and Sentinel-2 satellite images with high spatiotemporal resolution, spanning from 2019 to 2020, were integrated within a novel methodological framework. This framework consists of two main steps: (1) detection of rice phenological dates and the number of rice cropping seasons using the combination of a feature-based algorithm and a fitted Weibull function; (2) spatiotemporal integration of detected phenological dates using von Mises maximum likelihood estimates. The resulting rice calendar was then evaluated against existing rice calendars. The findings of this study provide valuable insight into the methodological framework and rice calendar products, benefiting both crop calendar algorithm developers and end users.
2.1 Study area
The analyzed area is located in monsoon Asia, which covers the region from 10° S to 53.5° N and from 61 to 153° E. The total area of monsoon Asia is 2106×106 ha.
Monsoon Asia accounts for the largest rice harvest area and the greatest volume of rice production globally (Zhang et al., 2020). The rice paddy fields of monsoon Asia are mainly on the Indo-Gangetic Plain, on the Yangtze Plain, in the Ayeyarwady Delta region, and in the Mekong Basin (Zhang et al., 2020). India and China have the two largest rice harvested areas covering 44×106 and 30×106 ha, respectively, followed by Bangladesh, Thailand, Vietnam, Myanmar, the Philippines, Cambodia, Pakistan, and Nepal (Fig. S1 in the Supplement) (FAOSTAT, 2022).
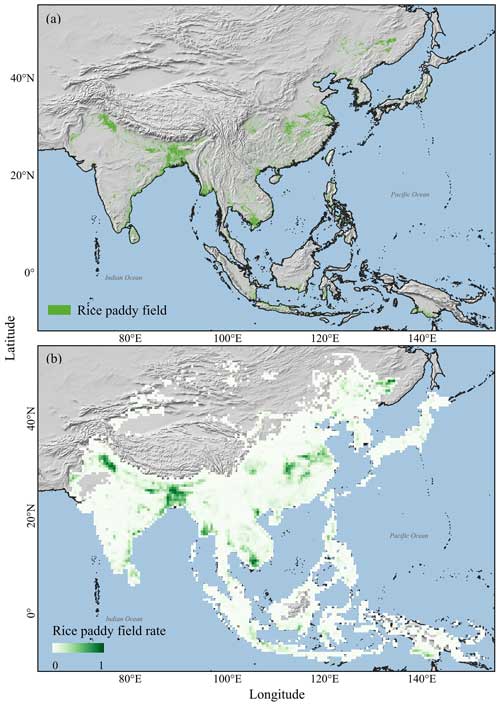
Figure 1Location of the study area and distribution of rice paddy fields in monsoon Asia. The rice paddy field distribution map in panel (a) was obtained from Zhang et al. (2020) and was produced using MODIS images. Green areas indicate rice paddy fields. The gridded rice paddy field map in panel (b) shows the percentage of rice paddy field in 0.5° grids. The green gradient indicates the variation in the percentage coverage of rice paddy fields.
2.2 Data
2.2.1 Rice paddy field distribution map and sampling method
The rice paddy field distribution map adopted in this study is from a 500 m resolution map produced using MODIS images (Fig. 1a; Zhang et al., 2020). This map effectively displays the presence and distribution of rice paddy fields over monsoon Asia. The reliability of this map is substantiated by its strong correlation with existing rice paddy field maps across diverse areas (R2 values ranging from 0.72 to 0.95) and its alignment with the area information obtained from FAOSTAT statistical data for each country (Zhang et al., 2020). In this study, this rice paddy field distribution map was aggregated to a gridded map with a 0.5° resolution (Fig. 1b).
Within each 0.5° grid, 20 rice paddy fields were randomly selected to derive the average rice phenology for that grid (Xiao et al., 2021; Zhao et al., 2023). This sampling method effectively minimizes errors caused by the misclassification of rice paddy fields by excluding outliers that deviate from the average rice phenology (Zhao et al., 2023). Additionally, this sampling size of 20 rice paddy fields is sufficient in that it saves computation time and has no effect on average rice phenology detection (Fig. S2).
2.2.2 Satellite data
All available images from Sentinel-1 and Sentinel-2 from 1 January 2019 to 31 December 2020 were used to generate backscattering or vegetation index time series via the Google Earth Engine (GEE) and the Google Colaboratory platforms. To overcome the inherent speckle noise and overlapping observations of Sentinel-1 images, 3 pixel × 3 pixel moving-window filter and incidence angle processing were performed (Inoue et al., 2020). Invalid observations in Sentinel-2 images caused by clouds and cirrus were removed using cloud filtering (> 50 %) and the cloud-score method (QA60 quality assessment band at a 60 m resolution) (Inoue et al., 2020). The VH C-band Ground Range Detected images in the Interferometric Wide swath mode were acquired at a 6 d temporal resolution. Based on the Sentinel-2 Multispectral Instrument Level-1C top-of-atmosphere reflectance images, the EVI and the NDYI, based on the blue (B2), green (B3), red (B4), and NIR (B8) spectral bands with a 10 m spatial resolution and a 5 d temporal resolution, were calculated as follows:
The locally estimated scatterplot smoothing (LOESS) method was further adopted to smooth the time-series data. The span value was assigned as 0.075 and 0.2 to depict VH and the EVI/NDYI time-series pattern.
2.2.3 Reference rice calendars
There are three widely accepted rice calendars currently available. RiceAtlas, based on a compilation of multiple census data sources, provides the start, peak, and end of the transplanting and harvesting dates as well as the number of rice cropping seasons at national or subnational scales globally (Laborte et al., 2017). RICA, generated using MODIS images, maps the rice transplanting date, harvesting date, and number of rice cropping seasons at administrative units in Asia (Mishra et al., 2021). The SAGE calendar records the gridded rice transplanting and harvesting dates of the year 2000 at a 5 min spatial resolution, but it only records two rice cropping seasons (Sacks et al., 2010). Therefore, the RiceAtlas, RICA, and SAGE rice calendars were used in this study to evaluate the number of rice cropping seasons. The RiceAtlas rice calendar, with its detailed phenological date range, was used to assess the performance of the proposed rice calendar with respect to determining the transplanting date and harvesting date, evaluating it based on the coefficient of determination (R2), bias error (Bias), mean absolute error (MAE), and root-mean-square error (RMSE) (Sect. S1 in the Supplement).
2.3 Methodology
The overall methodology for rice calendar mapping, which is summarized in Fig. 2, can be divided into two steps. The first step is the extraction of the transplanting and harvesting dates and the detection of the number of rice cropping seasons, depicted in Step 1 (Fig. 2). The transplanting and harvesting dates obtained in the first step (Step 1) require temporal and spatial integration for the generation of the rice calendar in Step 2 (Fig. 2).
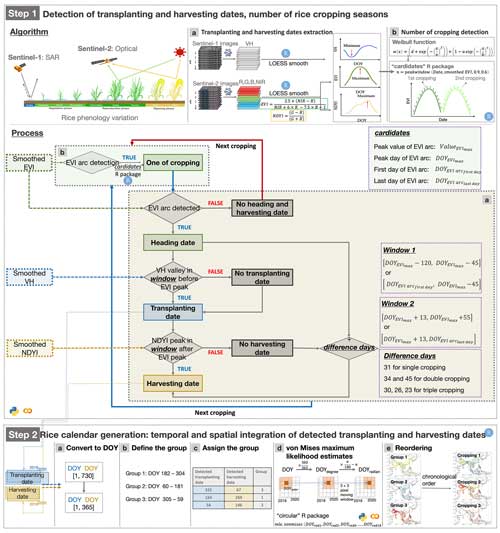
Figure 2Workflow for gridded rice calendar mapping based on satellite images. Step 1 depicts the algorithm and the process of transplanting and harvesting date extraction as well as the detection of the number of rice cropping seasons, as shown in the first box. In Step 2, the generation of the rice calendar is described, relying on the detected transplanting and harvesting dates derived from Step 1, via the temporal and spatial integration of the detected phenological dates displayed in the second box.
2.3.1 Step 1: extraction of phenological dates and the number of rice cropping seasons
The algorithm and process for the extraction of the transplanting and harvesting dates
Flooding rice cultivation, common in Asia and accounting for over 12 % of the global cropland (M. Zhang et al., 2021), presents a distinctive flooding signal that can be used for the detection of the rice transplanting date. Additionally, rice harvesting is characterized by irreversible leaf yellowing due to chlorophyll breakdown (“Algorithm” in Step 1 in Fig. 2; W. Zhang et al., 2021). These phenological characteristics of rice crops can be captured by a feature-based algorithm applied to the smoothed VH, EVI, and NDYI time-series data (Zhao et al., 2023). This algorithm's robustness has been confirmed at multiple spatial scales (subnational, 0.5° grid cell, and site scales) and for multiple cropping seasons (single-, double-, and triple-cropping seasons) in monsoon Asia (Zhao et al., 2023). The transplanting date was determined by identifying the minimum VH intensity from the shortest plants above the water surface, where VH intensity gradually increase as they interact with the radar signal (Torres et al., 2012). The harvesting date was detected using the NDYI's yellow signal, indicating the maximum yellowness at the harvesting date (“Transplanting and harvesting dates extraction” in Step 1 in Fig. 2; Zhao et al., 2023).
The minimum VH and peak NDYI were detected within the time window (“Process” box a in Step 1 in Fig. 2), indicating that only the minimum VH and the maximum NDYI values within the time window, before and after the EVI peak, can be identified as the transplanting date and harvesting date, respectively. To identify the optimal window for the detection of the transplanting and harvesting dates, the time window for detection of the minimum VH and peak NDYI were used (Table S1 in the Supplement). If the peak NDYI could not be obtained from those time windows, it was identified using the peak EVI date () plus the difference days. The difference days for each rice cropping can be found in Zhao et al. (2023) (“Process” box a in Step 1 in Fig. 2).
The method and process for detecting the number of rice cropping seasons
The six-parameter Weibull function can be used to identify the number of rice cropping seasons by depicting an arc with the shape of downward-opening patterns from the smoothed EVI time series (hereafter referred to as EVI arc) (“Number of cropping detection” in Step 1 in Fig. 2) as follows:
where a, b, c, d, e, and f are the free parameters to be fitted (Rolinski et al., 2007).
This fitted Weibull function can be implemented using the “peakwindow” function in the “cardidates” package of the R software (Rolinski et al., 2007) (Figs. S3a, 3). The rice cropping duration and its peak were determined as follows:
where y represents the variations in the smoothed EVI time-series values with respect to the date variable x.
To control the shape of the EVI arc, which represents the relative height between the neighboring peaks and valleys, for the purpose of identifying rice cropping, the parameters mincut and minpeak were set to 0.9 (Figs. S3c, S4) and 0.6 (Figs. S3d, S4), respectively.
After application of the function (Eq. 4), all available arcs of the smoothed EVI time series were labeled, including the start (start day of the detected EVI arc, ), peak (peak day of the detected EVI arc, ), and end (end day of the detected EVI arc, ) of the arc, and the peak EVI value () (“Process” box b in Step 1 in Fig. 2).
This method can detect the EVI arc, even if it does not exhibit a complete downward-opening shape (Fig. S3b). This is because rice growth spans 2 years, and some days are not within the period of study, resulting in the lack of EVI time-series data for those days. Based on the labeled EVI arc, all rice croppings were recognized, as the heading date through extraction of the .
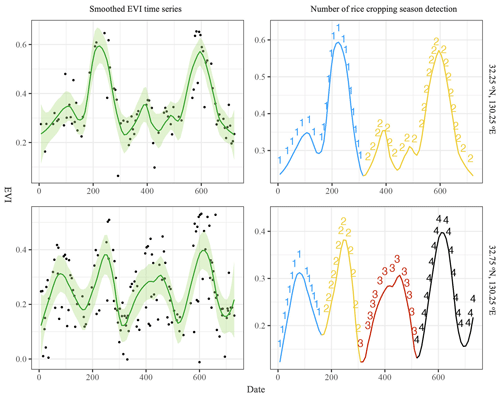
Figure 3Smoothed EVI time series and subsequent identification of the number of rice cropping seasons in adjacent grids (32.25° N, 130.25° E; 32.75° N, 130.25° E) across 2 years. Panels (a) and (c) show the smoothed EVI time series using the LOESS method. Black points and green lines indicate the EVI value at specific dates and the smoothed EVI time series, respectively. The green area indicates the 95 % confidence interval around the smoothed EVI time series. Panels (b) and (d) display the number of rice cropping seasons detected using the fitted Weibull function implemented via the cardidates package in R. Blue, yellow, red, and black lines correspond to the detected first, second, third, and fourth arcs of the smoothed EVI time series.
2.3.2 Step 2: temporal and spatial integration of the detected transplanting and harvesting dates for rice calendar generation
All of the transplanting and harvesting dates across 2 years for each grid were detected in Step 1 using the algorithms and processes described above. However, these detected transplanting and harvesting dates in each grid vary annually due to different weather conditions, the effects of climate change, adjustments in the agricultural schedule, and the availability of satellite images. Additionally, the detected transplanting and harvesting dates for a specific cropping season in a grid can differ markedly from those in neighboring grids, possibly indicating detection errors. Therefore, the temporal and spatial integration of the detected transplanting and harvesting dates, referred to as Step 2, is a necessary step in the generation of a multiyear, spatially averaged rice calendar.
To achieve this goal, the first step involved converting all of the detected transplanting and harvesting dates over 2 years into the day of the year (DOY) format, ranging from DOY 1 (1 January 2019) to DOY 730 (31 December 2020; 29 February 2020 was not considered for simplicity). Subsequently, the detected transplanting and harvesting dates that occurred in 2020 (DOY 366 to DOY 730) were converted for consistency with the first year (DOY 1 to DOY 365) by subtracting 365 (“Convert to DOY” in Step 2 in Fig. 2). Finally, all of the detected transplanting and harvesting dates were converted to DOY values from 1 to 365 (“Convert to DOY” in Step 2 in Fig. 2).
The temporal and spatial integration of phenological dates should be conducted within specific periods of time. Typically, rice is cultivated up to three times annually in most areas (Mishra et al., 2021), which serves as a meaningful basis for dividing the year into three equal periods. Thus, the year was divided into three groups: Group 1 comprised July–October (DOY 182 to DOY 304), Group 2 comprised March–June (DOY 60 to DOY 181), and Group 3 comprised November–February (DOY 305 to DOY 59) (“Define the group” in Step 2 in Fig. 2). The detected phenological dates were assigned to the corresponding group based on the maximum number of days from the transplanting date to the harvesting date falling within that group (“Assign the group” in Step 2 in Fig. 2).
The phenological date DOY values represent circular data that exhibit periodicity or cyclicity (Mahan, 1991). The designation of high and low values is arbitrary (Berens, 2009). For example, DOY 365 is almost the same as DOY 1 with a 1 d difference instead of a difference of 364 d. Adoption of statistical analysis commonly used with circular data can lead to incorrect results, whereas the von Mises distribution VM(μ, κ) can display a circular unimodal distribution (Berens, 2009). The probability density function of the von Mises distribution can be expressed as follows:
where I0 is the modified zero-order Bessel function and , κ>0.
The availability of the “circular” R package (Agostinelli and Lund, 2023) is convenient for the analysis of circular data. In the circular R package, the probability density function of the von Mises distribution can be displayed as follows:
where mu is the mean direction of the distribution, while kappa is a nonnegative numeric value representing a concentration parameter of the distribution; mu and kappa correspond to μ and κ in Eq. (5), respectively.
The circular data x in Eqs. (5) and (6) denote the phenological date shown in DOY format. The DOY was converted to an angle value (degrees) (“von Mises maximum likelihood estimates” in Step 2 in Fig. 2) (Franch et al., 2022) as follows:
where DOYdeg represents the angular value of the DOY, while 365 denotes the number of equal interval date units representing one rotation around the circle.
The angle value of the DOY (DOYdeg) was then converted to the radian value of the DOY (DOYrad) with interval [] (“von Mises maximum likelihood estimates” in Step 2 in Fig. 2) as follows:
The DOYrad was then input into the “mle.vonmises” function in the circular R package to obtain the parameters of the von Mises distribution via maximum likelihood estimates. For each group (i.e., Group 1, Group 2, and Group 3), the DOYrad values of each grid and the eight neighboring grids (3 × 3 pixel window) across the 2 years were included as input for the mle.vonmises function (“von Mises maximum likelihood estimates” in Step 2 in Fig. 2). Overall, 18 DOYrad values were used as follows:
The parameters DOYintegrated and Var were derived from the mle.vonmises function (Eq. 9), representing the value and variance of the phenological dates (DOYrad), respectively, after performing temporal and spatial integration for each grid within each group:
However, the value and variance of the phenological date (DOYintegrated and Var, respectively) are radian values that must be converted back to the DOY (DOYmu and DOYvar, respectively) as follows:
The integrated transplanting dates of all of the groups (e.g., DOYmu_G1, DOYmu_G2, and DOYmu_G3) were then reordered according to their chronological order for each grid. Finally, cropping-season-based phenological dates were obtained through the conversion of the group-based phenological dates (“Reordering” in Step 2 in Fig. 2).
3.1 Transplanting date and harvesting date
Based on the above methodological framework, rice calendars with two types of transplanting and harvesting dates were obtained: a group-based calendar (Fig. 4) and a cropping-season-based calendar (Fig. 6). The group-based rice calendar was initially produced and resulted in explicit transplanting and harvesting dates for three groups (Fig. 4). The median transplanting dates across monsoon Asia for the three rice groups are DOY 182, 77, and 325, with standard deviations of 23, 24, and 60, respectively (Fig. 4). Similarly, the median harvesting dates for the three groups are DOY 281, 172, and 67, with standard deviations of 23, 27, and 56, respectively (Fig. 4). Because the three groups divide the year equally, the transplanting date and the harvesting date both exhibit a unimodal distribution (Fig. S5). Moreover, the variance in the transplanting or harvesting dates observed in each grid originates from the analysis of 18 detected transplanting or harvesting dates from its eight neighbors across the 2 years, thereby highlighting both its temporal and spatial variation. The variance in the transplanting and harvesting dates across monsoon Asia for the three groups is shown in Fig. 5. The variance is 8, 11, and 12 d for the transplanting dates and 7, 11, and 15 d for the harvesting dates (Fig. 5). This variance arises from interannual variation and the spatial smoothing effect, and the small values indicate stability in the phenological date extraction.
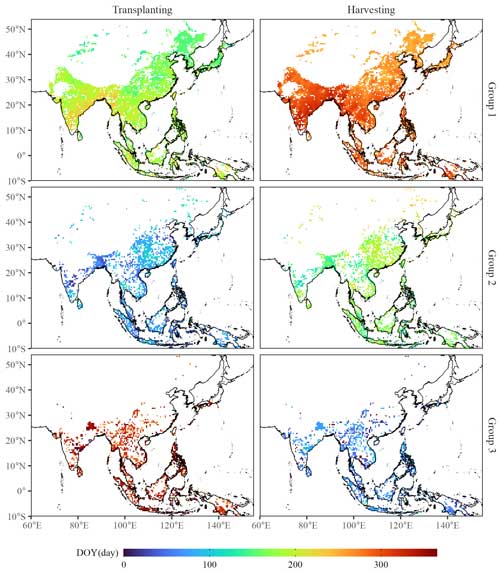
Figure 4Transplanting date and harvesting date for the three groups. The gradient from blue to red in the legend denotes the respective transplanting and harvesting dates.
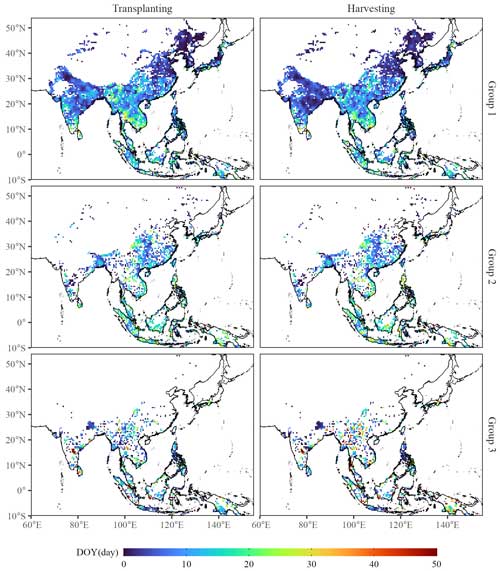
Figure 5Variance in the transplanting date and harvesting date for the three groups. The gradient from blue to red in the legend denotes the respective variance in the transplanting and harvesting dates.
The group-based transplanting and harvesting dates were then converted to the cropping-season-based format by reordering the detected transplanting dates for each grid. The cropping-season-based transplanting and harvesting dates are in a common format that facilitates comparison with those of other rice calendars. The median transplanting dates across monsoon Asia for three rice croppings are DOY 154, 208, and 327, with standard deviations of 61, 68, and 27, respectively (Fig. 6). Similarly, the median harvesting dates for three croppings are 253, 273, and 62, with standard deviations of 63, 111, and 47, respectively (Fig. 6). Owing to the large spatial coverage, the transplanting and harvesting dates vary across different croppings, exhibiting a bimodal distribution (Fig. 8). The variance in the transplanting dates for three cropping seasons across monsoon Asia is 9, 10, and 12 d, while the variance in the harvesting dates is 8, 11, and 16 d (Fig. 7).
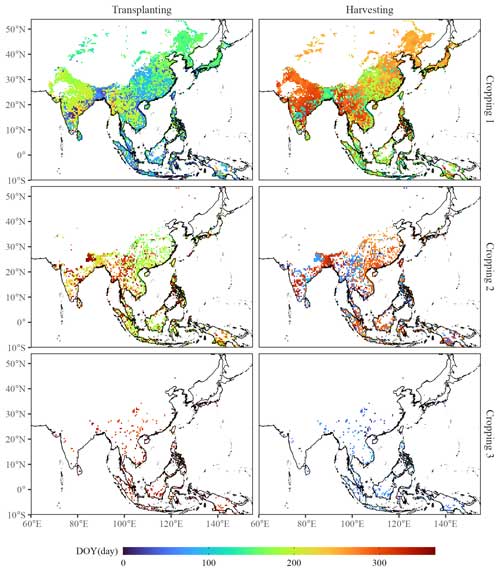
Figure 6Transplanting date and harvesting date for three rice croppings. The gradient from blue to red in the legend denotes the respective transplanting and harvesting dates.
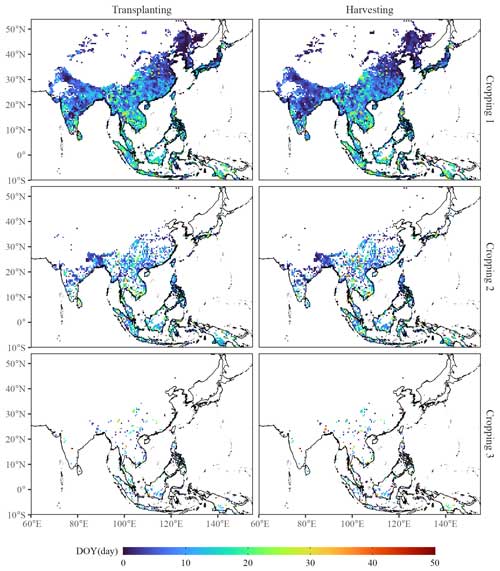
Figure 7Variance in the transplanting date and harvesting date for three rice croppings. The gradient from blue to red in the legend denotes the respective variance in the transplanting and harvesting dates.
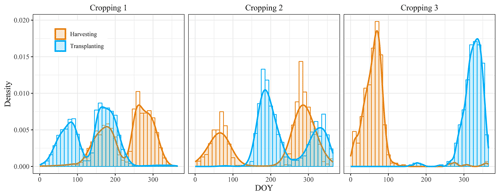
Figure 8Distribution of the transplanting and harvesting dates for three rice croppings. Blue and orange represent the transplanting date and the harvesting date, respectively.
There are difficulties in directly comparing transplanting and harvesting dates with those from other rice calendars owing to differences in the spatial resolution (grid versus administrative scale) and the identification of rice cropping sequences (Clauss et al., 2018). Thus, the RiceAtlas, which has been rasterized to the same spatial resolution as that of the proposed rice calendar (0.5°), was used to evaluate the performance in terms of single rice cropping with respect to the transplanting and harvesting dates. The transplanting dates of the proposed rice calendar are consistent with those of the RiceAtlas, with an R2 of 0.43, Bias of 3.93 d, MAE of 16.38 d, and RMSE of 27.62 d (Fig. 9). Additionally, the harvesting dates of the proposed rice calendar are correlated with those of the RiceAtlas, with an R2 of 0.44, Bias of −5.76 d, MAE of 17.87 d, and RMSE of 28.32 d (Fig. 9). However, the presence of the same transplanting or harvesting dates across large spatial areas in the RiceAtlas rice calendar (Fig. S6) reduces its accuracy. Similarly, the RiceAtlas has been used to evaluate the performance of the MODIS-based RICA (Mishra et al., 2021). Compared to the RICA in terms of accuracy, the proposed rice calendar demonstrates a smaller MAE (26.41 d in the RICA) and RMSE (34.20 d in the RICA) for transplanting dates as well as almost half the MAE (33.20 d in the RICA) and a smaller RMSE (42.72 d in the RICA) for harvesting dates (Mishra et al., 2021).
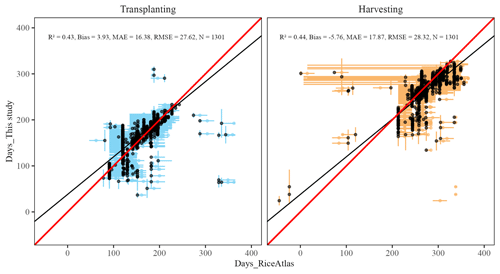
Figure 9Comparison of the transplanting date and harvesting date for single rice cropping between the proposed rice calendar and the RiceAtlas: blue and orange represent the transplanting date and harvesting date, respectively; vertical lines denote the range of the transplanting and harvesting dates of the proposed rice calendar; horizontal lines denote the range of the transplanting and harvesting dates of the RiceAtlas; and dots denote the peak of the transplanting or harvesting dates. Black dots denote the detected phenological day that falls within the transplanting or harvesting ranges from the RiceAtlas. Red and black solid lines represent the 1:1 line and the regression, respectively.
3.2 Number of rice cropping seasons
The number of rice cropping seasons in the proposed rice calendar was obtained by counting the phenological dates for the three croppings (Fig. 10a). In comparison with the RiceAtlas (Fig. 10b), RICA (Fig. 10c), and SAGE (Fig. 10d), which are based on the administrative scale, the proposed rice calendar shows the number of rice cropping seasons per grid, which cannot be paralleled by the other rice calendars. Among the total of 4811 detected grids, 2728, 1644, and 439 grids were identified as single, double, and triple rice croppings, respectively. To compare the number of each rice cropping across the rice calendars with different spatial resolutions, the area for each number of croppings was calculated (Fig. 11). In the area calculation, the variation in the area of each grid cell on the ellipsoidal Earth (Fig. S9) was considered, as was the percentage coverage of rice paddy fields in each grid (Fig. 1b).
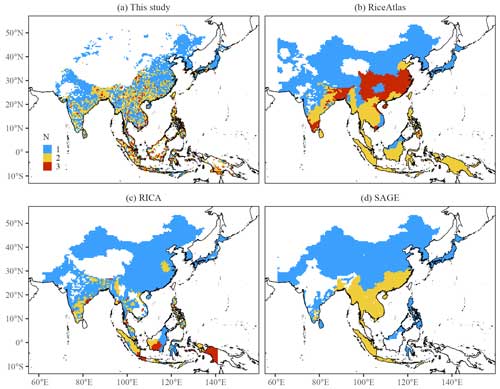
Figure 10Detected number of rice cropping seasons for (a) the proposed rice calendar, (b) the RiceAtlas, (c) the RICA, and (d) the SAGE calendar. Blue, yellow, and red represent single, double, and triple rice cropping, respectively.
The areas covered by single, double, and triple rice croppings in the proposed rice calendar are 0.53×106, 0.45×106, and 0.09×106 km2, respectively (Fig. 11). The area covered by single rice cropping falls within the range of 0.24×106 (RiceAtlas) to 0.65×106 km2 (RICA) (Fig. 11). The detection of single rice cropping in the proposed rice calendar shows reasonable performance, with consistent detection across the north of the middle to lower reaches of the Yangtze River, North Korea, South Korea, and most of Japan when compared with the other three rice calendars (Fig. 10). The regions of Haryana, Himachal Pradesh, and Punjab in India; central, midwestern, and western Nepal; and Balochistan and the historic Federally Administered Tribal Areas and North-West Frontier Province regions of Pakistan were also identified as single-rice-cropping-season areas (Fig. 10). In fact, these regions were initially identified as having double-rice-cropping seasons, but they are dominated by a rice–wheat cropping system (Abrol, 1997; Dhanda et al., 2022; Ahmad and Iram, 2023). Therefore, in this study, wheat cropping was removed in this region (Figs. 4–7, 10, 11, S10a, S10b) (Abrol, 1997; Dhanda et al., 2022).
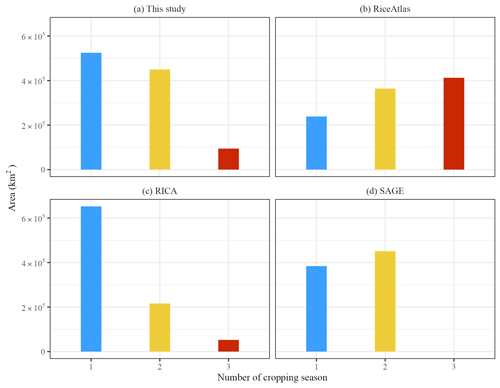
Figure 11Area of rice cropping for (a) the proposed rice calendar, (b) the RiceAtlas, (c) the RICA, and (d) the SAGE calendar. Blue, yellow, and red represent single, double, and triple rice cropping, respectively.
The region of detected double rice cropping occupies a larger area in the proposed rice calendar than in the other three rice calendars (Fig. 11a). Additionally, the area covered by a triple-rice-cropping season falls within the range of 0.05×106 km2 (RICA) to 0.4×106 km2 (RiceAtlas). The proposed rice calendar successfully detects a mix of double- and triple-rice-cropping seasons in Southeast Asia, including Vietnam, Malaysia, Indonesia, and the Philippines. The results align with real-world observations. Double- or triple-rice-cropping seasons are mostly cultivated in Vietnam (Nguyen et al., 2012; Diem et al., 2021). Two main cropping seasons are cultivated in Malaysia (Fatchurrachman et al., 2022) and the Philippines (Laborte et al., 2012). Even on Java (Indonesia), the cultivation ranges from single- to triple-rice-cropping seasons (Ramadhani et al., 2020). In contrast, fewer areas of double-rice-cropping seasons were detected in Myanmar, Thailand, Laos, and Cambodia using remote-sensing-based methods such as the proposed calendar (Fig. 10a) and the RICA (Fig. 10c), in comparison with census-based rice calendars such as the RiceAtlas (Fig. 10b) and SAGE (Fig. 10d). The presence of a large number of small but highly heterogeneous rice paddy fields limits the accurate detection of the number of rice cropping seasons via remote sensing (Mishra et al., 2021). Moreover, census-based rice calendars record the potential number of rice cropping seasons, which means that some rice croppings might overlap or that triple rice cropping is determined even though it accounts for only a small proportion of the rice cultivation activity within an administrative unit. This is a condition that does not occur in association with remote-sensing-based rice calendars.
The proposed rice calendar extracts 9 % of triple-rice-cropping seasons (Fig. 11a), which are scattered and distributed in South China, Southeast Asia, and India (Fig. 10a). This proportion is close to that of the RICA (6 % in Fig. 11c) but markedly lower than that of the RiceAtlas (41 % in Fig. 11c). However, the larger percentage of triple-rice-cropping seasons in the RiceAtlas might be overestimated, especially in Northeast India and Bangladesh in areas of the lower Gangetic Plain. The double-rice-cropping system is predominant on the lower Gangetic Plain (Wang et al., 2020). In this area, rice cultivation occurs in one to three seasons, namely the Aus rice season (March/April/May to June/July), the Aman rice season (June/July/August to November/December), and the Boro rice season (November/December/January to April/May). Among the three seasons, Aus and Aman are the dominant cropping seasons (Singha et al., 2019). Similarly, in South China, which is dominated by double-rice-cropping seasons, early rice is transplanted at the end of April and harvested at the end of July, whereas late rice is cultivated from June to October (Chen et al., 2020).
3.3 Advantages of the proposed rice calendar
The proposed rice calendar (Zhao and Nishina, 2023) successfully extracts rice transplanting and harvesting dates at a 0.5° grid cell scale across monsoon Asia by utilizing the rice-feature-based phenology algorithm (Zhao et al., 2023) with Sentinel-1 and Sentinel-2 images (“Transplanting and harvesting dates extraction” in Step 1 in Fig. 2). The detected transplanting and harvesting dates have been validated against 40 site-scale records from the literature and have shown high agreement, with an R2 of 0.90 and 0.87, Bias of 7.99 and −9.07 d, MAE of 16.32 and 19.58 d, and RMSE of 19.00 and 22.43 d for the transplanting and harvesting dates, respectively (Sect. S3.1 in the Supplement). The robustness of the site validation (Fig. S12), combined with reasonable performance compared to other rice calendars (Fig. 9), further demonstrates the efficacy of the transplanting and harvesting dates in the proposed rice calendar.
The main difference between the proposed rice calendar and other rice calendars lies in the algorithm for phenological date extraction. In contrast to census-based methods (such as the RiceAtlas), which face the issue of overlapping rice croppings, and remote-sensing-based methods (such as the RICA), which rely on constant threshold values set for large areas, this algorithm is not limited by rice variety, management, or environmental factors. It extracts the features of flooding around the transplanting date and peak yellowness during harvest from the minimum VH and peak NDYI values, respectively, without setting threshold parameters to characterize rice phenological variations. Unfortunately, due to the absence of ground-truth data, it is not possible to validate the Asian continental-scale rice calendar with correct accuracy. Instead, the validation in this study was based on observational records available in the existing literature. In this validation, it is worth noting that the proposed rice calendar showed a relatively high coefficient of determination and low RMSE compared with other rice calendars (Sect. S3.2 in the Supplement).
Detection of the number of rice cropping seasons is another important part of the methodological framework of the proposed rice calendar. The fitted Weibull function, implemented in the R package (“Number of cropping detection” in Step 1 in Fig. 2), automatically detects the number of rice cropping seasons based on the shape of the smoothed EVI time series, facilitating rapid and efficient rice calendar mapping. The shape-based detection avoids the identification of the number of rice cropping seasons based on peak detection or on the occurrence of some certain phenological date within the rice season (e.g., flowering date, as in Mishra et al., 2021). Additionally, the EVI shape-based detection allows for the identification of incomplete EVI arcs caused by noncontinuous observations as one of the rice croppings (Fig. S2b).
Temporal and spatial integration of detected transplanting and harvesting dates, as derived from Step 1, pose a great challenge due to flexible agricultural schedules and the availability of satellite imagery. This limitation restricts widespread application of remote-sensing-based rice calendars. In this study, a new algorithm (Step 2 in Fig. 2) was proposed to address this problem, which has long been a challenge in the preparation of previous rice calendars (Mishra et al., 2021). The use of von Mises maximum likelihood estimates produces the average of the transplanting and harvesting dates for 18 grids (3 × 3 grids × 2 years), taking the circular nature of phenological dates into special consideration (“von Mises maximum likelihood estimates” in Step 2 in Fig. 2). This algorithm is of great benefit for application in tropical areas where rice growth continues throughout the year, let alone temperate areas where rice growth occurs once a year. Additionally, the superiority of this algorithm lies in its ability to consider all rice croppings instead of excluding one of the rice cropping seasons through direct averaging based on administrative units. Furthermore, this algorithm improves the accuracy of the rice calendar by employing spatiotemporal integration, which reduces the presence of abnormal phenological dates.
The advantages of the abovementioned algorithms (Step 1 and Step 2 in Fig. 2) largely contribute to the production of a gridded rice calendar. The proposed rice calendar provides spatially explicit rice phenology with continental coverage via remote-sensing methods. The major difference between the proposed rice calendar and the RICA lies in the use of a feature-based algorithm with VH and the NDYI, which allows the proposed rice calendar to theoretically estimate rice phenology more accurately. Zhao et al. (2023) demonstrated that VH can accurately capture the start of paddy waterlogging and that the NDYI is a good indicator of the rice maturity stage. The proposed rice calendar presents a highly patchy map of rice phenological information (Figs. 6, 10a). The 0.5° resolution of the proposed rice calendar is finer than that of other rice calendars, including the RiceAtlas, RICA (at a subnational scale), and SAGE (derived from subnational data). This improvement greatly reduces the bias error caused by assigning average rice phenology to administrative units, as rice phenology can vary considerably within large administrative units (Franch et al., 2022). Furthermore, the proposed rice calendar displays the detailed distribution of rice paddy fields (Figs. 6, 10a), in contrast to previous rice calendars that covered entire administrative areas, irrespective of the small proportion of rice cultivation (Figs. S6–S8, 10b–d). Site-scale validation reinforces the abovementioned advantages, as the phenological dates in the proposed rice calendar are closer to the on-site records (Figs. S13, S14; Sect. S3). The relatively large bias and variance in the other three rice calendars (Fig. S14) demonstrate their limitations and uncertainties with respect to calculating the rice paddy field area, as shown in Fig. 11.
3.4 Uncertainty
Although the potential and the advantages of the proposed gridded rice calendar for monsoon Asia have been highlighted, some uncertainties remain. One challenge is the limited experimental periods on which the calendar is based, specifically during 2019–2020. While it was facilitated by the GEE and Google Colaboratory, generating detailed detection for 2 years (127×184 = 23 368 grids × 2 years) still requires high computational power. Additionally, errors can arise from the spatial and temporal integration of transplanting and harvesting dates. The grouping process, in particular, poses the risk of assigning single rice cropping seasons from 2 years into different groups, potentially overestimating the number of rice cropping seasons. The Var parameter, derived from the mle.vonmises function (Eqs. 9 and 11), is prone to bias, requiring bias-corrected estimates when the sample size is less than 16 (Best and Fisher, 1981). To address these issues, a 3 × 3 pixel window was used over 2 years to produce 18 values, highlighting the need to balance the window size and the sample size in spatiotemporal integration. The accuracy of reference rice calendars should also be considered, as they may rely on data from various sources and administrative scales (Laborte et al., 2017). Overlapping phenological dates between rice cropping seasons (Figs. S6–S8) can introduce further uncertainty. Furthermore, the complexity of multiple-crop cropping systems can lead to an overestimation of the number of rice cropping seasons. The growth of other crops exhibits a similar pattern to a unimodal EVI time series and flood irrigation before sowing, similar to rice (Ahmad and Iram, 2023). Examples include the middle rice cropping system (rice with wheat, barley, or rapeseed cropping systems) in eastern and central China (Chen et al., 2020) and the rice–wheat cropping systems on the Indo-Gangetic Plain (Abrol, 1997; Dhanda et al., 2022). Thus, detected triple-rice-cropping seasons in central China (Fig. 10a) will be biased, which must be specifically noted when using this information. Except for the rice-predominant areas, the rice–crop mixing problem can also puzzle the grids with a low rice percentage. While rice phenology extraction was obtained via the randomly selected sampling of rice paddy fields from the 500 m resolution rice distribution map (Zhang et al., 2020), grids with a low rice percentage have a higher possibility of errors with respect to wrongly classifying non-rice crops as rice, consequently resulting in the high possibility of non-rice crops being considered to be rice cultivation or one of the rice-cropping seasons. The application of a higher-resolution rice distribution map is expected to address this issue.
These uncertainties do not obscure the fact that this is a novel gridded rice calendar that provides more detailed rice phenology information that could be input into ecosystem models for GHG emission evaluation and production prediction. With the continued efforts of the research community to increase the spatiotemporal resolution of Earth observational data, integrated use of the new rice paddy field distribution map, and implementation of new tools for improved analysis of huge satellite images, it should become feasible to produce more precise rice calendars at a finer scale. Meanwhile, the methodological framework developed in this study for mapping the proposed rice calendar provides a robust reference for mapping other crop calendars.
The developed Monsoon Asia Rice Calendar (MARC) described in the paper is available from the Global Environmental Database (GED): https://doi.org/10.17595/20230728.001 (Zhao and Nishina, 2023).
The code for obtaining VH, EVI, and NDYI time-series data from Sentinel-1 and Sentinel-2 images, extracting the transplanting and harvesting dates from smoothed VH, EVI, and NDYI time-series data, and spatial and temporal integration of detected transplanting and harvesting dates can be found at https://doi.org/10.17595/20230728.001 (Zhao and Nishina, 2023).
Given the absence of an updated global- or continental-scale rice calendar that can explicitly depict the spatially gridded transplanting date and harvesting date information or the number of rice cropping seasons, this study developed a new gridded rice calendar for monsoon Asia with spatially explicit fine detail of rice phenology using a new methodological framework based on Sentinel-1 and Sentinel-2 images. The combination of a feature-based algorithm and a fitted Weibull function facilitates extraction of the transplanting and harvesting dates and detection of the number of rice cropping seasons, respectively. Subsequently, the detected transplanting and harvesting dates were subjected to temporal and spatial integration to produce the rice calendar. The proposed rice calendar was found to be sufficiently robust to map rice phenology more finely than that presented in other commonly used rice calendars, showing small Bias, MAE, and RMSE values in terms of the detection of the transplanting and harvesting dates. The proposed rice calendar could be used for global research on climate change and crop security, and the methodological framework could serve as a basis for producing large-scale mapping calendars for other crops.
The supplement related to this article is available online at: https://doi.org/10.5194/essd-16-3893-2024-supplement.
KN conceived of the research concept, acquired the funding, and supervised the project. XZ and KN developed the algorithm and curated the data. XZ, KN, and HI analyzed the data. HI, SO, and SY contributed to the satellite data analysis and algorithm implementation. XZ and KN wrote the original draft; XZ, KN, HI, YM, SO, and SY reviewed the draft.
The contact author has declared that none of the authors has any competing interests.
Publisher’s note: Copernicus Publications remains neutral with regard to jurisdictional claims made in the text, published maps, institutional affiliations, or any other geographical representation in this paper. While Copernicus Publications makes every effort to include appropriate place names, the final responsibility lies with the authors.
The authors would like to express their gratitude to the anonymous reviewers for their valuable suggestions and comments.
This study was supported by the New Energy and Industrial Technology Development Organization (NEDO; grant no. JPNP18016). This study was also partly supported by the Environment Research and Technology Development Fund of the Environmental Restoration and Conservation Agency (ERCA; grant nos. JPMEERF21S12000 and JPMEERF20235001).
This paper was edited by Xuecao Li and reviewed by four anonymous referees.
Abrol, I. P.: Sustaining rice-wheat cropping system productivity in the Indo-Gangetic Plains, in: The 4th JIRCAS International Symposium, Sustainable Agricultural Development Compatible with Environmental Conservation in Asia, 26 August 1997, 155–1651, 1997.
Agostinelli, C. and Lund, U.: R package “circular”: Circular Statistics (version 0.50), https://CRAN.R-project.org/package=_circular (last access: 8 July 2023), 2023.
Ahmad, I. and Iram, S.: Rice-wheat cropping pattern and resource conservation technologies: http://www.pakissan.com/english/agri.overview/rice.wheat.cropping.pattern.shtml, last access: 6 June 2023.
Berens, P.: CircStat: A MATLAB Toolbox for circular statistics, J. Stat. Softw., 31, 1–21, https://doi.org/10.18637/jss.v031.i10, 2009.
Best, D. and Fisher, N.: The BIAS of the maximum likelihood estimators of the von mises-fisher concentration parameters: The BIAS of the maximum likelihood estimators, Commun. Stat.-Simul. Comput., 10, 493–502, https://doi.org/10.1080/03610918108812225, 1981.
Chen, C., Groenigen, K., Yang, H., Hungate, B., Yang, B., Tian, Y., Chen, J., Dong, W., Huang, S., Deng, A., Jiang, Y., and Zhang, W.: Global warming and shifts in cropping systems together reduce China's rice production, Glob. Food Secur., 24, 100359, https://doi.org/10.1016/j.gfs.2020.100359, 2020.
Clauss, K., Ottinger, M., Leinenkugel, P., and Kuenzer, C.: Estimating rice production in the Mekong Delta, Vietnam, utilizing time series of Sentinel-1 SAR data, Int. J. Appl. Earth Obs., 73, 574–585, https://doi.org/10.1016/j.jag.2018.07.022, 2018.
Dhanda, S., Yadav, A., Yadav, D. B., and Chauhan, B. S.: Emerging issues and potential opportunities in the rice-wheat cropping system of North-Western India, Front. Plant Sci., 13, 832683, https://doi.org/10.3389/fpls.2022.832683, 2022.
Diem, P. K., Diem, N. K., and Hung, H. V.: Assessment of the efficiency of using MODIS MCD43A4 in mapping of rice transplanting calendar in the Mekong Delta, IOP Conf. Ser.-Earth Environ Sci., 652, 012015, https://doi.org/10.1088/1755-1315/652/1/012015, 2021.
Elliott, J., Müller, C., Deryng, D., Chryssanthacopoulos, J., Boote, K. J., Büchner, M., Foster, I., Glotter, M., Heinke, J., Iizumi, T., Izaurralde, R. C., Mueller, N. D., Ray, D. K., Rosenzweig, C., Ruane, A. C., and Sheffield, J.: The Global Gridded Crop Model Intercomparison: data and modeling protocols for Phase 1 (v1.0), Geosci. Model Dev., 8, 261–277, https://doi.org/10.5194/gmd-8-261-2015, 2015.
FAOSTAT: https://www.fao.org/faostat/en/#data (last access: 2 May 2023), 2022.
Fatchurrachman, Rudiyanto, Soh, N. C., Shah, R. M., Giap, S. G. E., Setiawan, B. I., and Minasny, B.: High-resolution mapping of paddy rice extent and growth stages across Peninsular Malaysia using a fusion of Sentinel-1 and 2 time series data in Google Earth Engine, Remote Sens., 14, 1875, https://doi.org/10.3390/rs14081875, 2022.
Franch, B., Cintas, J., Becker-Reshef, I., Sanchez-Torres, M. J., Roger, J., Skakun, S., Sobrino, J. A., Van Trichet, K., Degerickx, J., Gilliams, S., Koetz, B., Szantoi, Z., and Whitcraft, A.: Global crop calendars of maize and wheat in the framework of the WorldCreal project, GISci. Remote Sens., 59, 885–913, https://doi.org/10.1080/15481603.2022.2079273, 2022.
Iizumi, T., Kim, W., and Nishimori, M.: Modeling the global sowing and harvesting windows of major crops around the year 2000, J. Adv. Model. Earth Sy., 11, 99–112, https://doi.org/10.1029/2018MS001477, 2019.
Inoue, S., Ito, A., and Yonezawa, C.: Mapping paddy fields in Japan by using a Sentinel-1 SAR time series supplemented by Sentinel-2 images on Google Earth Engine, Remote Sens., 12, 1622, https://doi.org/10.3390/rs12101622, 2020.
Ito, A., Inoue, S., and Inatomi, M.: Model-based evaluation of methane emissions from paddy fields in East Asia, J. Agr. Meteorol., 78, 56–65, https://doi.org/10.2480/agrmet.D-21-00037, 2022.
Jiang, Y., Carrijo, D., Huang, S., Chen, J., Balaine, N., Zhang, W., van Groenigen K. J., and Linquist, B.: Water management to mitigate the global warming potential of rice systems: A global meta-analysis, Field Crop Res., 234, 47–54, https://doi.org/10.1016/j.fcr.2019.02.010, 2019.
Kotsuki, S. and Tanaka, K.: SACRA – a method for the estimation of global high-resolution crop calendars from a satellite-sensed NDVI, Hydrol. Earth Syst. Sci., 19, 4441–4461, https://doi.org/10.5194/hess-19-4441-2015, 2015.
Laborte, A. G., Bie, K. D., Smaling, E. M. A., Moya, P. F., Boling, A. A., and Ittersum, M. K. V.: Rice yields and yield gaps in southeast Asia: past trends and future outlook, Eur. J. Agron., 36, 9–20, https://doi.org/10.1016/j.eja.2011.08.005, 2012.
Laborte, A. G., Gutierrez, M. A., Balanza, J. G., Saito, K., Zwart, S. J., Boschetti, M., Murty, M. V. R., Villano, L., Aunario, J. K., Reinke, R., Koo, J., Hijmans, R. J., and Nelson, A.: RiceAtlas, a spatial database of global rice calendars and production, Sci. Data, 4, 170074, https://doi.org/10.1038/sdata.2017.74, 2017.
Liu, S., Chen, Y., Ma, Y., Kong, X., Zhang, X., and Zhang, D.: Mapping ratoon rice transplanting area in Central China using Sentinel-2 time stacks and the phenology-based algorithm, Remote Sens., 12, 3400, https://doi.org/10.3390/rs12203400, 2020.
Luo, Y., Zhang, Z., Chen, Y., Li, Z., and Tao, F.: ChinaCropPhen1km: a high-resolution crop phenological dataset for three staple crops in China during 2000–2015 based on leaf area index (LAI) products, Earth Syst. Sci. Data, 12, 197–214, https://doi.org/10.5194/essd-12-197-2020, 2020.
Maciel-Nájera, J. F., Hernández-Velasco, J., Gonzalez-Elizondo M. S., Hernandez-Díaz, J. C., López-Sánchez, C. A., Antúnez, P., Bailón-Soto, C. E., and Wehenkel, C.: Unexpected spatial patterns of natural regeneration in typical uneven-aged mixed pine-oak forests in the Sierra Madre Occidental, Mexico, Glob. Ecol. Conserv., 23, e01074, https://doi.org/10.1016/j.gecco.2020.e01074, 2020.
Mahan, R.: Circular statistical methods: Applications in spatial and temporal performance analysis, Special Research Report, U.S. Army Research Inst. for Behavioral and Social Sciences, Final Rep., https://apps.dtic.mil/sti/tr/pdf/ADA240751.pdf (last access: 16 July 2023), 1991.
Mathison, C., Deva, C., Falloon, P., and Challinor, A. J.: Estimating sowing and harvest dates based on the Asian summer monsoon, Earth Syst. Dynam., 9, 563–592, https://doi.org/10.5194/esd-9-563-2018, 2018.
Mishra, B., Busetto, L., Boschetti, M., Laborte, A., and Nelson, A.: RICA: A rice crop calendar for Asia based on MODIS multi year data, Int. J. Appl. Earth Obs., 103, 102471, https://doi.org/10.1016/j.jag.2021.102471, 2021.
More, R. S., Manjunath, K. R., Jain, N. K., Panigraphy, S., and Parihar, J. S.: Derivation of rice crop calendar and evaluation of crop phenometrics and latitudinal relationship for major south and south-east Asian countries: A remote sensing approach, Comput. Electron. Agr., 127, 336–350, https://doi.org/10.1016/j.compag.2016.06.026, 2016.
Muñoz-Salazar, T., LeQuesne, C., Rozas, V., Christie, D. A., and Rojas-Badilla, M.: Examining the potential of Austrocedrus chilensis tree rings as indicators of past late-spring forest events in central Chile, Dendrochronologia, 74, 125962, https://doi.org/10.1016/j.dendro.2022.125962, 2022.
Nguyen, T. T. H., De Bie, C. A. J. M., Ali, A., Smaling, E. M. A., and Chu, T. H.: Mapping the irrigated rice cropping patterns of the Mekong delta, Vietnam, through hyper-temporal SPOT NDVI image analysis, Int. J. Remote Sens., 33, 415–434, https://doi.org/10.1080/01431161.2010.532826, 2012.
Portmann, F. T., Siebert, S., and Döll, P.: MIRCA2000-Global monthly irrigated and rainfed crop areas around year 2000: A new high-resolution data set for agricultural and hydrological modelling, Global Biogeochem. Cy., 24, GB1011, https://doi.org/10.1029/2008GB003435, 2010.
Ramadhani, F., Pullanagari, R., Kereszturi, G., and Procter, J.: Automatic mapping of rice growth stages using the integration of Sentinel-2, MOD13Q1, and Sentinel-1, Remote Sens., 12, 3613, https://doi.org/10.3390/rs12213613, 2020.
Rolinski, S., Horn, H., Petzoldt, T., and Paul, L.: Identifying cardinal dates in phytoplankton time series to enable the analysis of long-term trends, Oecologia, 153, 997–1008, https://doi.org/10.1007/s00442-007-0783-2, 2007.
Sacks, W. J., Deryng, D., Foley, J. A., and Ramankutty, N.: Crop transplanting dates: An analysis of global patterns, Global Ecol. Biogeogr., 19, 607–620, https://doi.org/10.1111/j.1466-8238.2010.00551.x, 2010.
Sakamoto, T., Yokozawa, M., Toritani, H., Shibayama, M., Ishitsuka, N., and Ohno, H.: A crop phenology detection method using time-series MODIS data, Remote Sens. Environ., 96, 366–374, https://doi.org/10.1016/j.rse.2005.03.008, 2005.
Saunois, M., Stavert, A. R., Poulter, B., Bousquet, P., Canadell, J. G., Jackson, R. B., Raymond, P. A., Dlugokencky, E. J., Houweling, S., Patra, P. K., Ciais, P., Arora, V. K., Bastviken, D., Bergamaschi, P., Blake, D. R., Brailsford, G., Bruhwiler, L., Carlson, K. M., Carrol, M., Castaldi, S., Chandra, N., Crevoisier, C., Crill, P. M., Covey, K., Curry, C. L., Etiope, G., Frankenberg, C., Gedney, N., Hegglin, M. I., Höglund-Isaksson, L., Hugelius, G., Ishizawa, M., Ito, A., Janssens-Maenhout, G., Jensen, K. M., Joos, F., Kleinen, T., Krummel, P. B., Langenfelds, R. L., Laruelle, G. G., Liu, L., Machida, T., Maksyutov, S., McDonald, K. C., McNorton, J., Miller, P. A., Melton, J. R., Morino, I., Müller, J., Murguia-Flores, F., Naik, V., Niwa, Y., Noce, S., O'Doherty, S., Parker, R. J., Peng, C., Peng, S., Peters, G. P., Prigent, C., Prinn, R., Ramonet, M., Regnier, P., Riley, W. J., Rosentreter, J. A., Segers, A., Simpson, I. J., Shi, H., Smith, S. J., Steele, L. P., Thornton, B. F., Tian, H., Tohjima, Y., Tubiello, F. N., Tsuruta, A., Viovy, N., Voulgarakis, A., Weber, T. S., van Weele, M., van der Werf, G. R., Weiss, R. F., Worthy, D., Wunch, D., Yin, Y., Yoshida, Y., Zhang, W., Zhang, Z., Zhao, Y., Zheng, B., Zhu, Q., Zhu, Q., and Zhuang, Q.: The Global Methane Budget 2000–2017, Earth Syst. Sci. Data, 12, 1561–1623, https://doi.org/10.5194/essd-12-1561-2020, 2020.
Singha, M., Dong, J., Zhang, G., and Xiao, X.: High resolution paddy rice maps in cloud-prone Bangladesh and Northeast India using Sentinel-1 data, Sci. Data, 6, 1–10, https://doi.org/10.1038/s41597-019-0036-3, 2019.
Torres, R., Snoeij, P., Geudtner, D., Bibby, D., Davidson, M., Attema, E., Potin, P., Rommen, B., Floury, N., Brown, M., Traver, I. N., Deghaye, P., Duesmann, B., Rosich, B., Miranda, N., Bruno, C., L'Abbate, M., Croci, R., Pietropaolo, A., Huchler, M., and Rostan, F.: GMES Sentinel-1 mission, Remote Sens. Environ., 120, 9–24, https://doi.org/10.1016/j.rse.2011.05.028, 2012.
Waha, K., van Bussel, G. J., Müller, C., and Bondeau, A.: Climate-drive simulation of global crop sowing dates, Glob. Ecol. Biogeogr., 21, 247–259, https://doi.org/10.1111/j.1466-8238.2011.00678.x, 2012.
Wang, X., Wang, S., Li, X., Chen, B., Wang, J., Huang, M., and Rahman, A.: Modelling rice yield with temperature optima of rice productivity derived from satellite NIRv in tropical monsoon area, Agr. Forest Meteorol., 294, 108135, https://doi.org/10.1016/j.agrformet.2020.108135, 2020.
Xiao, W., Xu, S., and He, T.: Mapping paddy rice with Sentinel-1/2 phenology-, object-based algorithm-a implementation in Hangjiahu in China using GEE platform, Remote Sens., 13, 990, https://doi.org/10.3390/rs13050990, 2021.
Xin, Q., Li, J., Li, Z., Li, Y., and Zhou, X.: Evaluations and comparisons of rule-based and machine-learning-based methods to retrieve satellite-based vegetation phenology using MODIS and USA National Phenology Network data, Int. J. Appl. Earth Obs., 93, 102189, https://doi.org/10.1016/j.jag.2020.102189, 2020.
Yan, H., Liu, F., Qin, Y., Doughty, R., and Xiao, X.: Tracking the spatio-temporal change of cropping intensity in China during 2000-2015, Environ. Res. Lett., 14, 035008, https://doi.org/10.1088/1748-9326/aaf9c7, 2019.
Yang, Q., Shi, L., Han, J., Yu, J., and Huang, K.: A near real-time deep learning approach for detecting rice phenology based on UAV images, Agr. Forest Meteorol., 287, 107938, https://doi.org/10.1016/j.agrformet.2020.107938, 2020.
Zhang, G., Xiao, X., Dong, J., Xin, F., Zhang, Y., Qin, Y., Doughty, R. B., and Moore, B.: Fingerprint of rice paddies in spatial–temporal dynamics of atmospheric methane concentration in monsoon Asia, Nat. Commun., 11, 554, https://doi.org/10.1038/s41467-019-14155-5, 2020.
Zhang, J., Wu, H., Zhang, Z., Zhang, L., Luo, Y., Han, J., and Tao, F.: Asian rice calendar dynamics detected by remote sensing and their climate drivers, Remote Sens., 14, 4189, https://doi.org/10.3390/rs14174189, 2022.
Zhang, M., Wu, B., Zeng, H., He, G., Liu, C., Tao, S., Zhang, Q., Nabil, M., Tian, F., Bofana, J., Beyene, A. N., Elnashar, A., Yan, N., Wang, Z., and Liu, Y.: GCI30: a global dataset of 30 m cropping intensity using multisource remote sensing imagery, Earth Syst. Sci. Data, 13, 4799–4817, https://doi.org/10.5194/essd-13-4799-2021, 2021.
Zhang, W., Peng, K., Cui, F., Wang, D., Zhao, J., Zhang, J., Yu, N., Wang, Y., Zeng, D., Wang, Y., Cheng, Z., and Zhang, K.: Cytokinin oxidase/dehydrogenase OsCKX11 coordinates source and sink relationship in rice by simultaneous regulation of leaf senescence and grain number, Plant Biotechnol., J., 19, 335–350, https://doi.org/10.1111/pbi.13467, 2021.
Zhao, X. and Nishina, K.: Monsoon Asia Rice Calendar (MARC): a gridded rice calendar in monsoon Asia based on Sentinel-1 and Sentinel-2 images, NIES [data set], https://doi.org/10.17595/20230728.001, 2023.
Zhao, X., Nishina, K., Akitsu, T. K., Jiang, L., Masutomi, Y., and Nasahara, K. N.: Feature-based algorithm for large-scale rice phenology detection based on satellite images, Agr. Forest Meteorol., 329, 109283, https://doi.org/10.1016/j.agrformet.2022.109283, 2023.