the Creative Commons Attribution 4.0 License.
the Creative Commons Attribution 4.0 License.
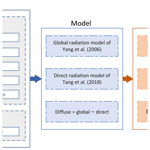
A dense station-based, long-term and high-accuracy dataset of daily surface solar radiation in China
Wenjun Tang
Junmei He
Jingwen Qi
The lack of long-term and high-quality solar radiation data has been an obstacle for scientific and industrial fields. In this study, a dense station-based, long-term and high-accuracy dataset of daily surface solar radiation was developed using two surface radiation models. One is the model developed by Yang et al. (2006) for global radiation estimation, and the other is the model developed by Tang et al. (2018) for direct radiation estimation. The main inputs for the development of the dataset are surface pressure, air temperature, relative humidity, horizontal visibility and sunshine duration, which are the routine meteorological variables observed at the 2743 China Meteorological Administration (CMA) weather stations. Validation against in situ observations and comparisons with two satellite-based radiation products shows that our station-based radiation dataset clearly outperforms the satellite-based radiation products at both daily and monthly scales. In addition, our dataset is available for more than 60 years and includes three radiation components of global, direct and diffuse radiation, which is not possible with satellite products. This station-based radiation dataset will contribute to the climate change research and solar energy engineering applications in the future. The station-based dataset is now available at https://doi.org/10.11888/Atmos.tpdc.300461 (Tang, 2023).
- Article
(7729 KB) - Full-text XML
- BibTeX
- EndNote
Solar radiation provides energy to everything on Earth; drives the water, energy and carbon cycles of the Earth's climate system; and largely determines the climatic conditions of human habitats (Wild, 2009). Therefore, solar radiation information at the Earth's surface is crucial in research of agriculture, hydrology, ecology and climate change and in simulations of land surface processes (Wang et al., 2012). Solar radiation reaching the horizontal surface is referred to as total solar radiation or global radiation (Rg) and is composed of direct radiation (Rdir) and diffuse radiation (Rdif). Global radiation is an important component of the surface energy budgets (Wild et al., 2015), and accurate global radiation data will contribute to the simulation of land-surface-related processes, such as ecological, hydrological, agricultural and glacial simulations (Tang et al., 2019b). In addition, both direct and diffuse radiation provide energy for plant photosynthesis and transpiration and are essential for hydrological and agricultural studies (Lee et al., 2017). Due to its multidirectional nature, diffuse radiation penetrates the vegetation canopy more than direct radiation, and more diffuse radiation can increase the light energy use efficiency of the canopy (Mercado et al., 2009; Yang et al., 2019). For example, under the same global radiation condition, increasing the proportion of diffuse radiation can increase the light energy use efficiency of different vegetation types by 6 %–180 % (Gu et al., 2002; Alton et al., 2007).
In addition to basic scientific research, the distribution and intensity of surface solar radiation is urgently needed for solar energy applications. All three components of solar radiation, i.e. global radiation, direct radiation and diffuse radiation, are prerequisites for the siting, design, evaluation and optimisation of different solar energy systems (Karakoti et al., 2011; Mellit et al., 2010). For example, photovoltaic (PV) power systems rely on global radiation to generate electricity, and global radiation on arbitrarily oriented PV panels can be calculated by direct and diffuse radiation, while concentrated solar power systems use only direct radiation to generate electricity (Boland et al., 2013; Tang et al., 2018). In addition, recently developed bifacial PV panels also use the backside of the PV panel to generate electricity, and the source of solar radiation on the backside is diffuse radiation (Rodríguez-Gallegos et al., 2018; Pelaez et al., 2019).
In situ measurements are considered to be the most effective and direct means of obtaining surface solar radiation data. However, the number of radiation observation stations is very low due to high maintenance and calibration costs. For example, radiation fluxes measured at about 2500 stations worldwide are stored in the Global Energy Balance Archive (GEBA), a database that stores the different components of the surface energy budget from different data sources, such as national meteorological services, various experimental observation networks and project reports (Wild et al., 2017). Among the GEBA, there are only about 100 radiation stations (Jiang et al., 2020a), which are provided by the China Meteorological Administration (CMA). Another issue is that the radiation observation stations are very unevenly distributed, with most of radiation stations being located in flat and densely populated areas and with a very small number of stations being located in complex-terrain areas and sparsely populated areas. In addition, almost all radiation stations include global radiation observations, but most of them do not include direct radiation and diffuse radiation observations. In addition, radiation measurements are considered to be more prone to problems and unreliable data than those of other meteorological variables. For example, erroneous or spurious data were frequently found in the CMA radiation observations (Shi et al., 2008; Tang et al., 2011). Therefore, the lack of long-term and dense solar radiation observations has become a tough challenge.
Satellites can provide continuous spatiotemporal observations, and retrieval based on satellite remote sensing is considered to be one of the most effective and commonly used means to fill the gap in ground-based radiation measurements (Lu et al., 2011; Zhang et al., 2014; Huang et al., 2019; Wang et al., 2020; Letu et al., 2021). In the past, satellite-based retrieval algorithms and corresponding radiation products emerged, and the retrieval accuracy of the corresponding products improved (Huang et al., 2019). In particular, retrievals applied to the new generation of geostationary satellites have improved in terms of temporal resolution, spatial resolution and accuracy to varying degrees (Tang et al., 2019a; Letu et al., 2020). In particular, Li et al. (2023) produced a high-spatiotemporal-resolution radiation product based on the new generation of geostationary satellites from the United States and Japan, with accuracy higher than other existing satellite products. In general, the accuracy of the satellite-based radiation product is higher than that of the reanalysis product (Jiang et al., 2020b). In addition, Hao et al. (2020) developed a global radiation product based on the unique Deep Space Climate Observatory (DSCOVR) satellite, whose orbit is at the Lagrange point. Despite the obvious advantages, there are still several problems with satellite-based radiation products. First, the time series of satellite radiation products are generally not long enough to meet the demand for long-time-series data, such as for climate change studies. Second, the updating of satellite sensors and the fusion of multi-source satellites would lead to inconsistent data quality and introduce large uncertainties into the analysis of long-term variations (Feng and Wang, 2021a; Shao et al., 2022). Third, most satellite radiation products only provide global radiation but do not include direct and diffuse radiation because the uncertainty in algorithms for estimating direct and diffuse radiation is much larger than the uncertainty in global radiation.
Alternatively, estimation based on meteorological variables observed at routine weather stations is another effective solution that can overcome the scarcity of radiation data since the number of routine weather stations is much denser than that of radiation stations (Feng and Wang, 2021b). For example, the number of radiation stations maintained by the CMA is only about 100, but the number of routine weather stations with long-term observations is much denser, exceeding 2400 stations. Empirical models for estimating global radiation using surface meteorological variables were usually found in the hydrological and agricultural fields (Wang et al., 2016), and these models can be broadly classified into three types: air-temperature-based models, sunshine-duration-based models and cloud-cover-based models (Liu et al., 2009; Ehnberg and Bollen, 2005). In general, a well-calibrated sunshine-duration-based model was considered to perform better than the other two types of models (Pohlert, 2004). Although the above empirical models, especially the Ångström–Prescott relationships (Ångström, 1924; Prescott, 1940), have been widely used and have achieved great success, these models require ground radiation observations for calibration, and the calibrated parameters are usually site dependent, which poses significant risks and challenges when applied in areas with sparse or no radiation observations.
Yang et al. (2001) developed a hybrid model to estimate daily global radiation by combining pure physical processes under clear skies and a parametrisation formula for cloud transmission under cloudy skies. The hybrid model has been shown to work well without local calibration (Yang et al., 2006, 2010). Tang et al. (2013) used this hybrid model to construct a dataset of daily global radiation at 716 CMA weather stations during 1961–2010 and also found that its accuracy was generally higher than that of locally calibrated empirical models and satellite-based retrievals. However, few studies have focused on the development of empirical models for direct and diffuse radiation estimates. Fortunately, Tang et al. (2018) also developed a similar hybrid model to estimate daily direct radiation by adopting the strategy of Yang et al. (2001) to estimate global radiation. Therefore, these two hybrid models provide us with an opportunity to construct daily surface solar radiation at routine weather stations once the observations of meteorological variables are available. The constructed dataset will contribute to simulations of land surface processes, climate change analysis and solar energy applications.
In this study, based on our previous study (Tang et al., 2013) for global radiation estimation, we expanded the number of stations, radiation elements and time length and finally developed a dense station-based, long-term and high-accuracy daily surface solar radiation dataset in China, which includes three elements: global radiation, direct radiation and diffuse radiation. This long-term dataset will contribute to the analysis of long-term variations in surface process simulations and solar energy applications, such as the assessment of solar energy potential, the determination of the optimal angle for solar PV panels and their long-term variation analysis, as well as the assessment of historical extreme events in solar energy systems. The rest of the paper is organised as follows. The methods used to estimate daily global, direct and diffuse radiation at weather stations are presented in Sect. 2, and Sect. 3 describes the input data used to drive the station-based models, the in situ data used to validate the accuracy of the developed dataset and two satellite-based radiation products used for comparison with our products. The performance of our dataset and two satellite-based radiation products against the in situ data is evaluated in Sect. 4, and the information on data availability is described in Sect. 5. Finally, Sect. 6 presents the summary of this study.
In this study, daily global radiation and daily direct radiation were estimated using the hybrid model of Yang et al. (2006) and the method developed by Tang et al. (2018). Finally, daily diffuse radiation was calculated by subtracting direct radiation from global radiation. The methods for estimating daily global, direct and diffuse radiation can be simply described by the following five mathematical formulas:
where Rg, Rb and Rd [W m−2] are the daily all-sky global, direct and diffuse radiation at the horizontal surface, respectively. Rb,clr and Rb,clr [W m−2] are the daily clear-sky global and direct radiation at the horizontal surface, respectively. τc,g and τc,b are the cloud transmittance for the daily global and direct radiation, respectively. n and N are the actual sunshine duration and the maximum possible sunshine duration, respectively. The daily clear-sky global and direct radiation (Rb,clr and Rb,clr) at the horizontal surface are calculated with the following equations:
where I0 [W m−2] is the horizontal solar radiation at the top of the atmosphere (TOA); and t1 [h] and t2 [h] are the times of sunrise and sunset, respectively. and are the transmittances for the daily direct and diffuse radiation under clear skies, respectively. , , , and are transmittances due to ozone absorption, permanent gas absorption, water vapour absorption, Rayleigh scattering, and aerosol absorption and scattering in the atmospheric layer, respectively. m is the air mass, and l [cm] is the ozone layer thickness. mc is the pressure-corrected air mass, and w [cm] is the precipitable water. β and h [radian] are the Ångström turbidity coefficient and the solar elevation angle, respectively. p0 [Pa] is the standard atmospheric pressure, and ps [Pa] is the surface pressure.
The overall flowchart for the estimation of the station-based radiation products is shown in Fig. 1, which consists mainly of input, model and output sections. The inputs are surface pressure, air temperature, relative humidity, ozone amount, aerosol data and sunshine duration. Air temperature and relative humidity are used to estimate precipitable water. The aerosol Ångström turbidity is mainly converted from horizontal visibility observations measured at weather stations using the method of Tang et al. (2017a). The ozone amount is obtained from the zonal means of the Total Ozone Mapping Spectrometer (TOMS). The outputs are daily global, direct and diffuse radiation. For more detailed information on the above methods, we can refer to the articles by Yang et al. (2006) and Tang et al. (2018).
3.1 Input data
The inputs to the above methods for estimating daily global, direct and diffuse radiation are mainly surface pressure, air temperature, relative humidity, horizontal visibility, sunshine duration and ozone amount. Except for ozone, all input data are observed at the 2473 CMA routine meteorological stations, where the estimation methods can work well. Here, the ozone amount was used from the climatological data obtained from the TOMS zonal means. Figure 2 shows the spatial distribution of these routine meteorological stations, indicated by the small blue circles, with characteristics of a dense distribution in the eastern and southern regions of China and a sparse distribution in the western and northern regions of China.
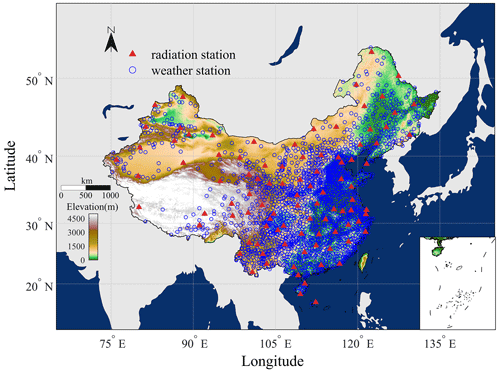
Figure 2Spatial distribution of China Meteorological Administration weather station and radiation station. Red triangles represent radiation stations, and blue circles denote weather stations without radiation observation.
Finally, in this study, a dataset of more than 60 years of daily global, direct and diffuse radiation was constructed using the methods described above at the 2473 CMA routine meteorological stations from the 1950s to 2021, with most stations covering the period of 1961–2021.
3.2 In situ radiation data
Due to the extensive renewal and replacement of radiation instruments in 1993 and because more erroneous observations were found before 1993, radiation observations during the period 1993–2010 were used to evaluate the performance of our developed station-based radiation dataset and the other two satellite-based radiation products. Among the 2473 CMA routine meteorological stations, there are about 96 radiation stations (denoted by the upper red triangle in Fig. 2) where radiation observations have been carried out in addition to routine meteorological observations since 1993. Among the 96 CMA radiation stations, there are only 19 stations where direct and diffuse radiation observations are carried out in addition to global radiation observations.
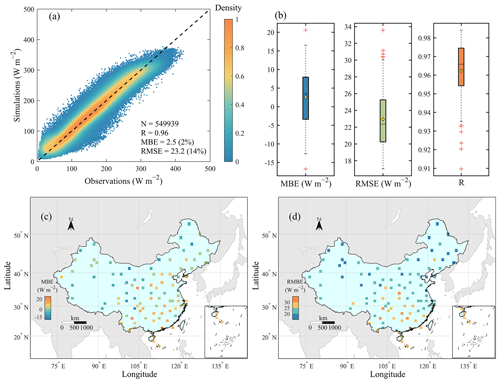
Figure 3Validation results for our station-based daily global radiation dataset against observations collected at 96 CMA radiation stations during 1993–2010. (a) Comparison of daily global radiation between our dataset and observations. (b) Boxplots of three statistical error metrics (MBE, RMSE and R). (c) Spatial distribution of MBE for individual radiation stations. (d) Spatial distribution of RMSE for individual radiation stations.
One issue to note is that we did not use the direct radiation observations as a validation basis; rather, we used the value obtained by subtracting the diffuse radiation observations from the global radiation observations because the quality of the direct radiation observations is significantly lower than that of the global and diffuse radiation observations (Tang et al., 2018). Another issue that we need to be aware of is that, obviously, erroneous or false values have usually been found in the CMA radiation data (Shi et al., 2008), although a preliminary quality check of the raw radiation observations was carried out before release. Therefore, we further applied the quality control procedures developed by Tang et al. (2010) to the raw radiation observations and filtered out the corresponding spurious and erroneous observations. More detailed information on the quality control scheme can be found in the article by Tang et al. (2010).
3.3 Satellite-based radiation products
In this study, two satellite-based radiation products (Jiang et al., 2020a; Tang et al., 2019b) were used for comparison with our station-based radiation dataset composed of estimations from 2743 CMA routine meteorological stations.
One is the product of Jiang et al. (2020a), which provides hourly global radiation and diffuse radiation in China with a spatial resolution of ∼5 km for a time span from 2007 to 2018. The product was generated using a deep learning algorithm developed by Jiang et al. (2019) to retrieve global radiation and a transfer learning approach to retrieve diffuse radiation from Multi-functional Transport Satellites (MTSAT) imagery. The algorithm successfully tackled spatial adjacency effects induced by photon transport through convolutional neural networks, resulting in excellent performance in instantaneous radiation retrieval, especially for diffuse radiation (Jiang et al., 2020c).
The other is the high-resolution global product of global radiation developed by Tang et al. (2019b) based on the latest cloud products of the International Satellite Cloud Climatology Project H-series pixel-level global (ISCCP-HXG), routine meteorological variables of the ERA5 reanalysis data, aerosol optical thickness of the MERRA-2 reanalysis data, and albedo of the MODIS and Satellite Application Facility on Climate Monitoring (CM SAF) products with the physical algorithm of Tang et al. (2017b). This global product has a spatial resolution of 10 km and a temporal resolution of 3 h, and it spans the period of July 1983 to December 2018. Global comparative validation with observations shows that the accuracy of this global radiation product is generally better than several global satellite radiation products, such as the Earth's Radiant Energy System (CERES; Kato et al., 2013), the Global Energy and Water Cycle Experiment surface radiation budget (GEWEX-SRB; Pinker and Laszlo, 1992) and the ISCCP flux dataset (ISCCP-FD; Zhang et al., 2004).
In this study, these two satellite-based radiation products were first averaged to daily and monthly means, then validated against observations collected at the CMA radiation stations, and finally compared with our station-based radiation dataset in China.
The station-based radiation dataset was first validated against the CMA radiation observations at daily and monthly scales, then it was compared with two other satellite-based radiation products, and finally, its spatial distribution characteristics were further analysed. These are described in detail in the following four subsections. In this study, we used the statistical metrics of mean bias error (MBE), relative MBE (rMBE), root mean square error (RMSE), relative RMSE (rRMSE) and correlation coefficient (R) to measure the accuracies of our station-based dataset and the other two satellite-based radiation products.
4.1 Validation at daily scale
Figure 3 shows the validation results for the daily global radiation estimates against observations from 96 CMA radiation stations over the period of 1993–2010. Overall, our station-based estimates over China perform well, with an MBE of 2.5 W m−2, an RMSE of 23.2 W m−2 and an R of 0.96 (Fig. 3a). This indicates that the accuracy of our station-based estimates is significantly higher than that of almost all of the satellite products (such as ISCCP-FD, GEWEX-SRB, CERES, GLASS and ISCCP-HXG) and reanalysis data (such as, ERA5 and MERRA-2), as well as that of other regional satellite-based radiation products from Tang et al. (2016), Jiang et al. (2020a) and Letu et al. (2021). Therefore, we would expect our station-based estimates to be more accurate than the five global radiation products mentioned by Li et al. (2021) as CERES generally performs best among them. Of course, this speculation needs to be further verified with in situ measurements collected in China in the future. In terms of MBE, there is a slight positive deviation, but the relative deviation is close to 2 %, which is within the tolerance range of the PV potential assessment.
In addition, we also calculated the statistical metrics for each radiation station, and their boxplots and spatial distributions are shown in Fig. 3b–d. It can be seen that most of the stations had an MBE between −4 and 8 W m−2, an RMSE less than 25 W m−2 and an R greater than 0.95. The stations with relatively large errors were mainly found in the southern and eastern regions of China. This is largely due to the fact that these regions have more cloud cover and overcast skies, making it difficult to use sunshine duration to accurately parametrise cloud transmission. In addition, the uncertainty in the aerosol data would also partly contribute to the large errors.
Similar evaluations for the daily direct and diffuse radiation estimates are presented in Figs. 4 and 5, respectively. Averaged over 19 CMA radiation stations, our estimates for daily direct radiation produce an MBE of 7.4 W m−2, an RMSE of 27.2 W m−2 and an R of 0.92, while our estimates for daily diffuse radiation produce an MBE of −3.3 W m−2, an RMSE of 19.2 W m−2 and an R of 0.83. The accuracies for both direct and diffuse radiation are lower than for global radiation, as can be seen from their rRMSE and rMBE. The absolute rMBE for global radiation is about 2 %, while those for direct and diffuse radiation are about 9 % and 4 %, respectively. The rRMSE for global radiation is about 14 %, while those for direct and diffuse radiation are about 33 % and 22 %, respectively. We found that there is a slight overestimation for direct radiation, with an MBE of about 7.4 W m−2. This may be due to the presence of high clouds, in which case the cloud transmission for direct radiation cannot be well parametrised by the sunshine duration (Tang et al., 2018). For direct radiation, the RMSE at most stations is less than 29 W m−2, and the R at most stations is greater than 0.91; for diffuse radiation, the RMSE at most stations is less than 21 W m−2, and the R at most stations is greater than 0.81. The largest RMSE for both direct and diffuse radiation is found at the Lhasa station, where popcorn clouds were common, posing a major challenge to the simulation of cloud transmittance with sunshine duration.
4.2 Validation at monthly scale
Based on the daily estimates, we also calculated their monthly averages and validated them against observations. Figure 6 shows the validations for the estimates of monthly global radiation against observations at the CMA radiation stations. Averaged over all stations, the monthly global radiation estimates give an MBE of 2.4 W m−2, an RMSE of 13.1 W m−2 and an R of 0.98. The rRMSE is about 8 %. From the boxplots of the error indicators, R was greater than 0.98, and RMSE was less than 15 W m−2 at most stations. The spatial distribution features of MBE and RMSE are similar to those for daily global radiation, with relatively larger errors in the southern and eastern regions of China, and the largest MBE and RMSE are both found at Panzhihua station (with latitude of 26.583∘ and longitude of 101.717∘).
Figures 7 and 8 also show the validation results for monthly direct and diffuse radiation against 19 CMA radiation stations. The rRMSE values for monthly direct and diffuse radiation are 20 % and 12 %, respectively. The MBE, RMSE and R for monthly direct radiation are 6.6 W m−2, 16.3 W m−2 and 0.94, respectively, while the MBE, RMSE and R for monthly diffuse radiation are −3.3 W m−2, 10.5 W m−2 and 0.94, respectively. Similarly to the case of the daily scale, a slight overestimation is also found for the monthly direct radiation. The RMSE for monthly direct and diffuse radiation is less than 18 and 13 W m−2, respectively, at most stations, while R for monthly direct and diffuse radiation is greater than 0.91 and 0.95, respectively, at most stations. The spatial distribution characteristics of MBE and RMSE are similar to those of daily conditions for both direct and diffuse radiation.
4.3 Comparison with satellite-based products
To demonstrate the superiority of the station-based radiation dataset developed in this study, we compared the station-based dataset with two widely used satellite-based radiation products. One is the product developed with deep learning and trained with surface radiation observations (Jiang et al., 2020a), and the other is the long-term global product of global radiation (Tang et al., 2019b), which was mainly developed based on the latest ISCCP-HXG cloud products with the improved physical algorithm (Tang et al., 2017b). The former can provide hourly global, direct and diffuse radiation, while the latter can only provide the 3-hourly global radiation. Here, we use a 3×3 spatial window to smooth the raw radiation product from Tang et al. (2019b) as its accuracy is clearly improved when upscaled to 30 km. As we only have access to the CMA radiation observations up to 2010, the time period chosen for comparison is 2000–2010. Tables 1 and 2 present the comparison of the evaluation results among the three radiation products against the observations measured at all CMA radiation stations for daily and monthly conditions, respectively.
For daily data comparisons, our station-based estimate for daily global radiation obviously outperforms the other two satellite-based radiation products, with an MBE of 2.7 W m−2, an RMSE of 23.2 W m−2 and an R of 0.96, followed by the product of Tang et al. (2019b) (with an MBE of 6.7 W m−2, an RMSE of 26.8 W m−2 and an R of 0.95) and the product of Jiang et al. (2020a) (with an MBE of 4.4 W m−2, an RMSE of 33.6 W m−2 and an R of 0.92). Our station-based estimates for daily direct radiation are also apparently more accurate than the satellite-based product of Jiang et al. (2020a), with the former producing a lower RMSE and a higher R. The RMSE of our estimates is about 9 W m−2 lower than that of the product of Jiang et al. (2020a).
For the daily diffuse radiation, our estimate is comparable to the diffuse radiation product of Jiang et al. (2020a). It should be noted that the CMA radiation observations were used to train the deep learning model of Jiang et al. (2020a), while no observations were used in our estimates. Overall, these results indicate that the station-based estimates of global, direct and diffuse radiation generally outperform those from satellite retrievals.
Table 1Accuracy comparisons of our estimates in this study against other two satellite-based products at daily scale. The units of MBE and RMSE are both in watts per square metre (W m−2). Observations of global radiation measured at 96 CMA radiation stations and observations of direct radiation and diffuse radiation measured at 19 CMA radiation stations during 2000–2010 are used.
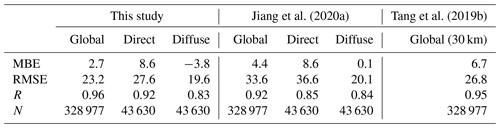
For the monthly comparisons, we also found that our estimate of monthly global radiation is clearly more accurate than that of the two satellite products. The MBE and RMSE of our estimate are 2.6 and 13.4 W m−2, respectively, which are both lower than those of the two satellite products, with MBE and RMSE values of 4.6 and 18.5 W m−2 for the Jiang et al. (2020a) product and of 6.7 and 16.3 W m−2 for the Tang et al. (2019b) product. The R of our estimate is 0.98, which is higher than that of the two satellite products. Our direct radiation estimate also outperforms the satellite product of Jiang et al. (2020a), with the former having lower RMSE and MBE and higher R. Similarly to the daily comparison, the two monthly diffuse products are comparable to each other.
Table 2Accuracy comparisons of our estimates in this study with other two satellite-based products at monthly scale. The units of MBE and RMSE are both in watts per square metre (W m−2). Observations of global radiation measured at 96 CMA radiation stations and observations of direct radiation and diffuse radiation measured at 19 CMA radiation stations during 2000–2010 are used.
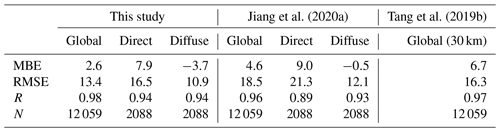
4.4 Spatial distribution features of solar radiation in China
Based on the developed station-based solar radiation dataset, Fig. 9 presents the spatial distributions of the multi-year average global, direct and diffuse radiation in China during 1961–2021. The spatial distribution of direct radiation is similar to that of global radiation, with the highest value over the Tibetan Plateau and the lowest value over the Sichuan Basin. However, the spatial distribution of diffuse radiation is very different from that of global radiation, with the highest value being found at the southernmost tip of China and the lowest value being found in northeastern China. In general, clear-sky days correspond to more direct radiation, and cloudy days correspond to more diffuse radiation, mainly because the scattering effect of aerosols is much smaller than the scattering effect of clouds. Therefore, high direct radiation is usually found in areas with frequent sunny days, such as northwest China, northern China, and inner Mongolia, while low direct radiation is usually found in areas with frequent cloud cover, such as eastern China and southern China. The opposite is true for diffuse radiation.
It should be noted that our station-based products are spatially discontinuous, especially in northwestern China, which may introduce significant uncertainty when applied to the assessment of solar power system potential. However, the uncertainty caused by spatial discontinuity in flat areas would be relatively small as the spatial representation of a station on flat ground is generally larger than 25 km (Hakuba et al., 2013). Fortunately, most solar power systems are built on land with slopes of less than 3 %. In contrast, applications over complex terrain will introduce large uncertainties. Combining station-based data with satellite products will be a good solution in the future to improve the accuracy of solar energy potential assessment.
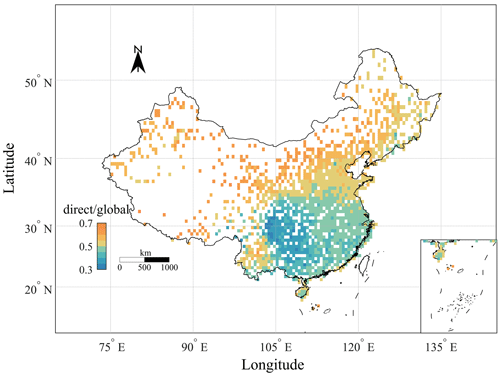
Figure 10Spatial distribution of the multi-year mean ratio of direct radiation to global radiation from the station-based radiation dataset in China during 1961–2021.
The spatial distribution of the multi-year average ratio of direct radiation to global radiation in China during the period of 1961–2021 is also shown in Fig. 10. Direct radiation is mainly greater than diffuse radiation (ratio <50 %) in northern China, northeastern China, northwestern China and the Tibetan Plateau, while diffuse radiation dominates in eastern and southern China. This information can guide the planning of solar PV power and concentrating solar power (CSP) in China. For example, regions such as Xinjiang, inner Mongolia, Gansu and the Tibetan Plateau, with high proportions of direct radiation, are suitable for the construction of CSP projects. Regions such as Hainan, Yunnan, Guangxi and Guangdong, with relatively high global and diffuse radiation, are suitable for the use of bifacial PV panels as both sides of the panels can be used to generate electricity, and the main source on the back is from diffuse radiation. Conversely, in areas with low diffuse radiation, it may not be necessary to use bifacial PV panels.
The dense station-based, long-term and high-accuracy dataset of daily global, direct and diffuse radiation is stored at the National Tibetan Plateau Data Center (https://doi.org/10.11888/Atmos.tpdc.300461, Tang, 2023), Institute of Tibetan Plateau Research, Chinese Academy of Sciences.
In this study, we have developed a dense station-based, long-term dataset of daily surface solar radiation in China with high accuracy. The dataset consists of estimates of three radiation components (global, direct and diffuse radiation) at the 2473 CMA meteorological stations during the period from the 1950s to 2021, with most stations covering the period of 1961–2021. The methods used to develop the dataset are the global radiation estimation model of Yang et al. (2006) and the direct radiation estimation model of Tang et al. (2018), and the main inputs are the five meteorological variables of surface pressure, air temperature, relative humidity, horizontal visibility and sunshine duration measured at the meteorological stations. The developed dataset was evaluated against in situ measurements collected at 96 CMA radiation stations and was further compared with another two satellite-based radiation products.
Averaged over all radiation stations and the time period of 1993–2010, the total RMSE values for daily global, direct and diffuse radiation are 23.2, 27.6 and 19.6 W m−2, respectively. At the monthly mean scale, our estimates give RMSE values of about 13.4, 16.5 and 10.9 W m−2, respectively, for monthly global, direct and diffuse radiation. These error indicators on both daily and monthly scales are generally lower than those of the satellite radiation products, especially for global and direct radiation. Comparisons with the satellite-based radiation products indicate that our station-based estimates have a clear advantage in terms of accuracy and length of time series. However, our dataset does not provide radiation data beyond the weather stations. Merging the station-based estimates with the satellite-based retrievals will have good potential to improve the accuracy of the radiation products in the future. We expect that our station-based radiation dataset will contribute significantly to relevant basic research, engineering applications and fusion with satellite-based retrievals in the future.
All the authors discussed the results and contributed to the paper. WT calculated the dataset, analysed the results and drafted the paper. JH drew the figures.
The contact author has declared that none of the authors has any competing interests.
Publisher's note: Copernicus Publications remains neutral with regard to jurisdictional claims made in the text, published maps, institutional affiliations, or any other geographical representation in this paper. While Copernicus Publications makes every effort to include appropriate place names, the final responsibility lies with the authors.
The ISCCP-HXG global product of global radiation was provided by the National Tibetan Plateau Data Center (TPDC). The radiation product of Jiang et al. (2020a) was available from Pangaea at https://doi.org/10.1594/PANGAEA.904136 (Jiang and Lu, 2019), and the CMA routine meteorological variables and radiation data were obtained from the CMA Meteorological Information Center. The authors would like to thank the staff of the data management and production organisations for their valuable work.
This work was supported by the National Natural Science Foundation of China (grant nos. 41988101 and 42171360) and the Development Program of China (grant no. 2022YFB4202104).
This paper was edited by Jing Wei and reviewed by two anonymous referees.
Alton, P. B., North, P. R., and Los, S. O.: The impact of diffuse sunlight on canopy light-use efficiency, gross photosynthetic product and net ecosystem exchange in three forest biomes, Global Change Biol., 13, 776–787, 2007.
Ångström, A.: Solar and terrestrial radiation, Q. J. Roy. Meteor. Soc., 50, 121–125, 1924.
Boland, J., Huang, J., and Ridley, B.: Decomposing global solar radiation into its direct and diffuse components. Renew. Sust. Energy Rev., 28, 749–756, https://doi.org/10.1016/j.rser.2013.08.023, 2013.
Ehnberg, J. S. G. and Bollen, M. H. J.: Simulation of global solar radiation based on cloud observations, Solar Energy, 78, 157–162, https://doi.org/10.1016/j.solener.2004.08.016, 2005.
Feng, F. and Wang, K.: Merging high-resolution satellite surface radiation data with meteorological sunshine duration observations over China from 1983 to 2017, Remote Sens., 13, 602, https://doi.org/10.3390/rs13040602, 2021a.
Feng, F. and Wang, K.: Merging ground-based sunshine duration observations with satellite cloud and aerosol retrievals to produce high-resolution long-term surface solar radiation over China, Earth Syst. Sci. Data, 13, 907–922, https://doi.org/10.5194/essd-13-907-2021, 2021b.
Gu, L. H., Baldocchi, D., Verma, S. B., Black, T. A., Vesala, T., Falge, E. V., and Dowty, P. R.: Advantages of diffuse radiation for terrestrial ecosystem productivity, J. Geophys. Res.-Atmos., 107, 4050, https://doi.org/10.1029/2001JD001242, 2002.
Hakuba, M. Z., Folini, D., Sanchez-Lorenzo, A., and Wild, M.: Spatial representativeness of ground-based solar radiation measurements, J. Geophys. Res.-Atmos., 118, 8585–8597, https://doi.org/10.1002/2017JD027261, 2013.
Hao, D., Asrar, G. R., Zeng, Y., Zhu, Q., Wen, J., Xiao, Q., and Chen, M.: DSCOVR/EPIC-derived global hourly and daily downward shortwave and photosynthetically active radiation data at 0.1∘ × 0.1∘ resolution, Earth Syst. Sci. Data, 12, 2209–2221, https://doi.org/10.5194/essd-12-2209-2020, 2020.
Huang, G., Li, Z., Li, X., Liang, S., Yang, K., Wang, D., and Zhang, Y.: Estimating surface solar irradiance from satellites: Past, present, and future perspectives, Remote Sens. Environ., 233, 111371, https://doi.org/10.1016/j.rse.2019.111371, 2019.
Jiang, H. and Lu, N.: High-resolution surface global solar radiation and the diffuse component dataset over China, PANGAEA [data set], https://doi.org/10.1594/PANGAEA.904136, 2019.
Jiang, H., Lu, N., Qin, J., Tang, W., and Yao, L.: A deep learning algorithm to estimate hourly global solar radiation from geostationary satellite data, Renew. Sustain. Energy Rev., 114, 109327, https://doi.org/10.1016/j.rser.2019.109327, 2019.
Jiang, H., Lu, N., Qin, J., and Yao, L.: Hourly 5-km surface total and diffuse solar radiation in China, 2007–2018, Sci. Data, 7, 311, https://doi.org/10.1038/s41597-020-00654-4, 2020a.
Jiang, H., Yang, Y., Bai, Y., and Wang, H.: Evaluation of the total, direct, and diffuse solar radiations from the ERA5 reanalysis data in China, IEEE T. Geosci. Remote, 17, 47–51, 2020b.
Jiang, H., Yang, Y., Wang, H., Bai, Y., and Bai, Y.: Surface diffuse solar radiation determined by reanalysis and satellite over East Asia: evaluation and comparison, Remote Sens., 12, 1387, https://doi.org/10.3390/rs12091387, 2020c.
Karakoti, I., Pande, B., and Pandey, K.: Evaluation of different diffuse radiation models for Indian stations and predicting the best fit model, Renew. Sust. Energy Rev., 15, 2378–2384, https://doi.org/10.1016/j.rser.2011.02.020, 2011.
Kato, S., Loeb, N. G., Rose, F. G., Doelling, D. R., Rutan, D. A., Caldwell, T. E., Yu, L., and Weller, R. A.: Surface irradiances consistent with CERES-derived top-of-atmosphere shortwave and longwave irradiances, J. Climate, 26, 2719–2740, 2013.
Lee, M., Hollinger, D., Keenan, T., Ouimette, A., Ollinger, S., and Richardson, A.: Model-based analysis of the impact of diffuse radiation on CO2 exchange in a temperate deciduous forest, Agr. Forest Meteorol., 249, 377–389, 2017.
Letu, H., Yang, K., Nakajima, T. Y., Ishimoto, H., Nagao, T. M., Riedi, J., Baran, A. J., Ma, R., Wang, T., Shang, H., Khatri, P., Chen, L., Shi, C., and Shi, J.: High-resolution retrieval of cloud microphysical properties and surface solar radiation using Himawari-8/AHI next-generation geostationary satellite, Remote Sens. Environ., 239, 111583, https://doi.org/10.1016/j.rse.2019.111583, 2020.
Letu, H., Nakajima, T. Y., Wang, T. X., Shang, H. Z., Ma, R., Yang, K., Baran, A. J., Riedi, J., Ishimoto, H., Yoshida, M., Shi, C., Khatri, P., Du, Y. H., Chen, L. F., and Shi, J. C.: A new benchmark for surface radiation products over the East Asia-Pacific region retrieved from the Himawari-8/AHI next-generation geostationary satellite, B. Am. Meteorol. Soc., 2021, E873–E888, 2021.
Li, R., Wang, D., and Liang, S.: Comprehensive assessment of five global daily downward shortwave radiation satellite products, Sci. Remote Sens., 4, 100028, https://doi.org/10.1016/j.srs.2021.100028, 2021.
Li, R., Wang, D., Wang, W., and Nemani, R.: A GeoNEX-based high-spatiotemporal-resolution product of land surface downward shortwave radiation and photosynthetically active radiation, Earth Syst. Sci. Data, 15, 1419–1436, https://doi.org/10.5194/essd-15-1419-2023, 2023.
Liu, X. Y., Mei, X. R., Li, Y. Z., Wang, Q., Jensen, J. R., Zhang, Y., and Porter, J. R.: Evaluation of temperature-based global solar radiation models in China, Agric. Forest Meteorol., 149, 1433–1446, 2009.
Lu, N., Qin, J., Yang, K., and Sun, J.: A simple and efficient algorithm to estimate daily global solar radiation from geostationary satellite data, Energy, 36, 3179–3188, https://doi.org/10.1016/j.energy.2011.03.007, 2011.
Mellit, A., Eleuch, H., Benghanem, M., Elaoun, C., and Pavan, A. M.: An adaptive model for predicting of global, direct and diffuse hourly solar irradiance, Energy Convers. Manage., 51, 771–782, https://doi.org/10.1016/j.enconman.2009.10.034, 2010.
Mercado, L. M., Bellouin, N., Sitch, S., Boucher, O., Huntingford, C., Wild, M., and Cox, P. M.: Impact of changes in diffuse radiation on the global land carbon sink, Nature, 458, 1014–1017, 2009.
Pelaez, S. A., Deline, C., Macalpine, S. M., Marion, B., Stein, J. S., and Kostuk, R. K.: Comparison of Bifacial Solar Irradiance Model Predictions With Field Validation, IEEE J. Photovoltaics, 9, 82–88, https://doi.org/10.1109/jphotov.2018.2877000, 2019.
Pinker, R. T. and Laszlo, I.: Modeling surface solar irradiance for satellite application on a global scale, J. Appl. Meteorol., 31, 194–211, https://doi.org/10.1175/1520-0450(1992)031<0194:MSSIFS>2.0.CO;2, 1992.
Pohlert, T.: Use of empirical global radiation models for maize growth simulation, Agric. Forest Meteorol., 126, 47–58, 2004.
Prescott, J. A.: Evaporation from a water surface in relation to solar radiation, T. Roy. Soc. Austr., 641, 114–125, 1940.
Rodríguez-Gallegos, C. D., Bieri, M., Gandhi, O., Singh, J. P., Reindl, T., and Panda, S. K.: Monofacial vs bifacial Si-based PV modules: Which one is more cost-effective?, Solar Energy, 176, 412–438, https://doi.org/10.1016/j.solener.2018.10.012, 2018.
Shao, C., Yang, K., Tang, W., He, Y., Jiang, Y., Lu, H., Fu, H., and Zheng, J.: Convolutional neural network-based homogenization for constructing a long-term global surface solar radiation dataset, Renew. Sust. Energy Rev., 169, 112952, https://doi.org/10.1016/j.rser.2022.112952, 2022.
Shi, G. Y., Hayasaka, T., Ohmura, A., Chen, Z. H., Wang, B., Zhao, J. Q., Che, H. Z., and Xu, L.: Data quality assessment and the long-term trend of ground solar radiation in China, J. Appl. Meteor. Climatol., 47, 1006–1016, 2008.
Tang, W., Yang, K., He, J., and Qin, J.: Quality control and estimation of global solar radiation in China, Solar Energy, 84, 466–475, https://doi.org/10.1016/j.solener.2010.01.006, 2010.
Tang, W., Yang, K., Qin, J., and Min, M.: Development of a 50-year daily surface solar radiation dataset over China, Sci. China Earth Sci., 56, 1555–1565m https://doi.org/10.1007/s11430-012-4542-9, 2013.
Tang, W., Qin, J., Yang, K., Liu, S., Lu, N., and Niu, X.: Retrieving high-resolution surface solar radiation with cloud parameters derived by combining MODIS and MTSAT data, Atmos. Chem. Phys., 16, 2543–2557, https://doi.org/10.5194/acp-16-2543-2016, 2016.
Tang, W., Yang, K., Qin, J., Niu, X., Lin, C., and Jing, X.: A revisit to decadal change of aerosol optical depth and its impact on global radiation over China, Atmos. Environ., 150, 106–115, 2017a.
Tang, W., Yang, K., Sun, Z., Qin, J., and Niu, X.: Global Performance of a Fast Parameterization Scheme for Estimating Surface Solar Radiation From MODIS Data, IEEE T. Geosci. Remote Sens, 55, 3558–3571, https://doi.org/10.1109/TGRS.2017.2676164, 2017b.
Tang, W., Yang, K., Qin, J., Min, M., and Niu, X.: First Effort for Constructing a Direct Solar Radiation Data Set in China for Solar Energy Applications, J. Geophys. Res.-Atmos., 123, 1724–1734, https://doi.org/10.1002/2017jd028005, 2018.
Tang, W., Li, J., Yang, K., Qin, J., Zhang, G., and Wang, Y.: Dependence of remote sensing accuracy of global horizontal irradiance at different scales on satellite sampling frequency, Solar Energy, 193, 597–603, 2019a.
Tang, W., Yang, K., Qin, J., Li, X., and Niu, X.: A 16-year dataset (2000–2015) of high-resolution (3 h, 10 km) global surface solar radiation, Earth Syst. Sci. Data, 11, 1905–1915, https://doi.org/10.5194/essd-11-1905-2019, 2019b.
Tang, W.: A dense station-based long-term and high-accuracy dataset of daily surface solar radiation in China, National Tibetan Plateau/Third Pole Environment Data Center [data set], https://doi.org/10.11888/Atmos.tpdc.300461, 2023.
Tang, W.-J., Yang, K., Qin, J., Cheng, C. C. K., and He, J.: Solar radiation trend across China in recent decades: a revisit with quality-controlled data, Atmos. Chem. Phys., 11, 393–406, https://doi.org/10.5194/acp-11-393-2011, 2011.
Wang, D., Liang, S., Zhang, Y., Gao, X., Brown, M. G., and Jia, A.: A new set of MODIS land products (MCD18): Downward shortwave radiation and photosynthetically active radiation, Remote Sensing, 12, 168, https://doi.org/10.3390/rs12010168, 2020.
Wang, K. C., Dickinson, R. E., Wild, M., and Liang, S.: Atmospheric impacts on climatic variability of surface incident solar radiation, Atmos. Chem. Phys., 12, 9581–9592, https://doi.org/10.5194/acp-12-9581-2012, 2012.
Wang, L., Kisi, O., Zounemat-Kermani, M., Salazar, G., Zhu, Z., and Gong, W.: Solar radiation prediction using different techniques: Model evaluation and comparison, Renew. Sustain. Energy Rev., 61, 384–397, https://doi.org/10.1016/j.rser.2016.04.024, 2016.
Wild, M.: Global dimming and brightening: A review, J. Geophys. Res.-Atmos., 114, D00D16, https://doi.org/10.1029/2008JD011470, 2009.
Wild, M., Folini, D., Hakuba, M. Z., Schär, C., Seneviratne, S. I., Kato, S., Rutan, D., Ammann, C., Wood, E. F., and König-Langlo, G.: The energy balance over land and oceans: an assessment based on direct observations and CMIP5 climate models, Clim. Dynam., 44, 3393–3429, 2015.
Wild, M., Ohmura, A., Schär, C., Müller, G., Folini, D., Schwarz, M., Hakuba, M. Z., and Sanchez-Lorenzo, A.: The Global Energy Balance Archive (GEBA) version 2017: a database for worldwide measured surface energy fluxes, Earth Syst. Sci. Data, 9, 601–613, https://doi.org/10.5194/essd-9-601-2017, 2017.
Yang, K., Huang, G.-W., and Tamai, N.: A hybrid model for estimating global solar radiation, Solar Energy, 70, 13–22, https://doi.org/10.1016/S0038-092X(00)00121-3, 2001.
Yang, K., Koike, T., and Ye, B.: Improving estimation of hourly, daily, and monthly downward shortwave radiation by importing global data sets, Agric. Forest Meteorol., 137, 43–55, https://doi.org/10.1016/j.agrformet.2006.02.001, 2006.
Yang, K., He, J., Tang, W., Qin, J., and Cheng, C. C. K.: On downward shortwave and longwave radiations over high altitude regions: Observation and modeling in the Tibetan Plateau, Agric. Forest Meteorol., 150, 38–46, 2010.
Yang, X., Li, J., Yu, Q., Ma, Y., Tong, X., Feng, Y., and Tong, Y.: Impacts of diffuse radiation fraction on light use efficiency and gross primary production of winter wheat in the North China Plain, Agric. Forest Meteorol., 275, 233–242, 2019.
Zhang, X., Liang, S., Zhou, G., Wu, H., and Zhao, X.: Generating Global LAnd Surface Satellite incident shortwave radiation and photosynthetically active radiation products from multiple satellite data, Remote Sens. Environ., 152, 318–332, 2014.
Zhang, Y. C., Rossow, W. B., Lacis, A. L., Valdar, O., and Michael, I. M.: Calculation of radiative fluxes from the surface to top of atmosphere based on ISCCP and other global data sets: refinements of the radiative transfer model and the input data, J. Geophys. Res., 109, D19105, https://doi.org/10.1029/2003JD004457, 2004.