the Creative Commons Attribution 4.0 License.
the Creative Commons Attribution 4.0 License.
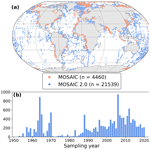
The Modern Ocean Sediment Archive and Inventory of Carbon (MOSAIC): version 2.0
Kai Nakajima
Tessa S. Van der Voort
Hannah Gies
Aline Wildberger
Thomas M. Blattmann
Lisa Bröder
Timothy I. Eglinton
Marine sediments play a crucial role in the global carbon cycle by acting as the ultimate sink of both terrestrial and marine organic carbon. To understand the spatiotemporal variability in the content, sources, and dynamics of organic carbon in marine sediments, a curated and harmonized database of organic carbon and associated parameters is needed, which has prompted the development of the Modern Ocean Sediment Archive and Inventory of Carbon (MOSAIC) database (http://mosaic.ethz.ch/, last access: 26 July 2023; https://doi.org/10.5281/zenodo.8322094, Paradis, 2023; https://doi.org/10.5168/mosaic019.1, Van der Voort et al., 2019). MOSAIC version 2.0 has expanded the spatiotemporal coverage of the original database by >400 % and now holds data from more than 21 000 individual sediment cores from different continental margins on a global scale. Additional variables have also been incorporated into MOSAIC v.2.0 that are crucial to interpret the quantity, origin, and age of organic carbon in marine sediments globally. Sedimentological parameters (e.g. grain size fractions and mineral surface area) help understand the effect of hydrodynamic sorting and mineral protection on the distribution of organic carbon, while molecular biomarker signatures (e.g. lignin phenols, fatty acids, and alkanes) can help constrain the specific origin of organic matter. MOSAIC v.2.0 also stores data on specific sediment and molecular fractions, which provide further insight into the processes that affect the degradation and ageing of organic carbon in marine sediments. Data included within MOSAIC are continuously expanding, and version control will allow users to benefit from updated versions while ensuring reproducibility of their findings.
- Article
(10094 KB) - Full-text XML
-
Supplement
(3242 KB) - BibTeX
- EndNote
Marine sediments are the ultimate sink of particulate organic carbon (OC) and play a fundamental role in the global carbon cycle. Understanding the functioning of the carbon cycle requires investigations of the distribution, composition, and dynamics of OC in marine sediments on different spatial and temporal scales. However, given resource and time limitations, studies prioritize either their spatial breadth and/or the factors and parameters measured (e.g. Bao et al., 2016; Mollenhauer et al., 2004; Smeaton et al., 2021). This leads to dispersed and unstandardized datasets that are often specific to individual research questions and/or laboratories, hindering broader global assessments.
Proper harmonization of marine sedimentary data is especially important given the logistical challenges and costs of retrieving samples at sea. Compiling a global dataset of OC and its geochemical composition is crucial to understand large-scale patterns that affect its distribution. The first global maps of the distribution of organic matter in surface sediments were presented by Premuzic et al. (1982) and Romankevich (1984), but these essentially highlighted general patterns in the global distribution of OC. With the advance of geostatistical techniques, a more precise distribution of surficial OC content was performed by Seiter et al. (2004) using over 5500 data points. However, geostatistical techniques can only infer the OC content in areas where data are available since they rely on neighbouring data points to perform a kriging interpolation (Oliver and Webster, 1990). The onset of spatial machine learning techniques in the field of geosciences has allowed the prediction of OC contents in unsampled areas that present similar explanatory features (i.e. surface ocean primary productivity, oxygen concentrations, sedimentation rates, etc.). This was first undertaken by Lee et al. (2019) using a slightly expanded dataset of 5600 data points and was revisited by Atwood et al. (2020) using 11 500 data points. While all of these studies agree that higher OC contents are found on continental margins in comparison to the open ocean, these margins are highly complex and temporally heterogenous, which is why more efforts should be directed towards compiling data in these areas. With the exception of Seiter et al. (2004), these studies do not report their raw data, which prevents any assessment of the reproducibility of their findings and does not adhere to the findability, accessibility, interoperability, and reusability (FAIR) data principles (Wilkinson et al., 2016).
The availability of a harmonized database of OC in marine sediments is crucial to refine estimates of the carbon stocks of maritime nations (Avelar et al., 2017; Luisetti et al., 2020; Smeaton et al., 2021) and marine protected areas (Atwood et al., 2020). Furthermore, compositional information of sedimentary organic matter, such as its isotopic (13C and 14C) and elemental composition, can help define spatial patterns in the distribution of the source and age of OC in marine sediments (Galy et al., 2007; Kao et al., 2014; Van der Voort et al., 2018), as well as determine its reactivity (DeMaster et al., 2021). With this in mind, compiling and harmonizing diverse variables in both surficial and downcore sediment are fundamental to understand the spatiotemporal variations in the content, source, and composition of OC in marine sediments. Hence, the Modern Ocean Sedimentary Archive and Inventory of Carbon (MOSAIC) was constructed, with data on the content (% OC), stable carbon isotope (δ13C), radiocarbon (Δ14C), and elemental (C:N ratio) composition of OC, as well as on the biogenic silica and CaCO3 contents of marine sediments, in 4460 locations worldwide (van der Voort et al., 2021). While MOSAIC can be used to model the global distribution of OC content (Atwood et al., 2020) and to identify vulnerable sites of OC disturbance (Clare et al., 2023), it can also provide a global context of the geochemical characteristics of a specific study area (Bruni et al., 2022) and can even be used to locate suitable sites and samples that can answer specific research questions (Nina Golombek, personal communication, 2023).
Since the publication of the initial MOSAIC database, new metadata-reporting strategies have stressed the importance of standardizing measurement techniques (Morrill et al., 2021). Indeed, different measurement techniques yield different values that can be up to 25 % different depending on the method employed (Byers et al., 1978; Celia Magno et al., 2018; Hoogsteen et al., 2018; Schubert and Nielsen, 2000), which could jeopardize proper comparability between studies and laboratories (Wilkinson et al., 2016). To maximize the comparability of data, the metadata reported in MOSAIC were revised and updated. Moreover, to further understand the role of hydrodynamic sorting and mineral protection in the distribution of OC (Ausín et al., 2021; Bao et al., 2019; Bruni et al., 2022; Hemingway et al., 2019), as well as to assess the dispersal of specific sources of terrigenous OC in marine sediments (Gordon and Goñi, 2004; Hou et al., 2020; Yu et al., 2021) and to contrast the age of OC compound classes (Hou et al., 2021; Kusch et al., 2021), the MOSAIC database was expanded to incorporate sedimentological properties (e.g. grain size distribution, mineral-specific surface area, and porosity) and biomarker concentrations (e.g. lignin phenols, fatty acids, and alkenones), as well as data from the analyses on specific components (i.e. grain size or density fractions and specific organic compounds). Finally, the spatiotemporal coverage of the database has more than quintupled since the publication of the first MOSAIC iteration (van der Voort et al., 2021).
These changes have prompted us to publish a new version of MOSAIC (v.2.0), with an updated metadata structure and automated ingestion pipeline (see Sect. 2), additional variables (see Sect. 3), and expanded spatiotemporal coverage (see Sect. 4).
With the purpose of expanding the database's breadth and utility, the content in MOSAIC was revised and expanded, the database schema was restructured, and the pipeline for the incorporation of new data was improved. For instance, it is common for marine studies to present previously published data to provide greater spatiotemporal contextualization of the new findings (i.e. Bao et al., 2016; Goñi et al., 2006; Gordon and Goñi, 2003; Kao et al., 2014). However, if data are independently added to the database, this can lead to large amounts of duplicate data entries, which in turn can skew the global dataset. Indeed, a careful re-examination of the first MOSAIC database revealed that ∼20 % of the OC data and ∼25 % of the 14C data were duplicated. Similarly, the same sediment samples can be analysed for different parameters (e.g. OC content, sediment grain size, and specific biomarkers), and the results of these different analyses are often presented in separate studies (e.g. Bröder et al., 2016; Vonk et al., 2012). When ingesting the data separately, they would be registered as separate samples, and therefore, comparison of relationships between these variables would be hindered. Finally, inherent limitations of the length and precision of certain data types led to the loss of data (i.e. when surpassing maximum varying characters or maximum integer length), whereas coordinates of certain samples were found to be incorrect.
To overcome these issues, the structure and ingestion pipeline of the database were amended such that, to the best of our knowledge, data are properly georeferenced, data duplication and data loss are minimized, and data comparability is improved. Given the ever-expanding body of data that continues to be published, we acknowledge that the structure and pipeline of the database will require further tuning and revisions based on user feedback and our experience.
2.1 MOSAIC v.2.0 structure
MOSAIC is a normalized relational database with separate tables that are related to each other to avoid redundancies and to store data efficiently. For instance, instead of storing data related to the sampling location in every subsample of a sediment core, it is stored only once in a separate table. The relations within the MOSAIC database follow a hierarchical list of tables that can be grouped into article and author (source metadata), geopoints (location and sampling metadata), and samples (analyses) (Fig. 1). For this new iteration, the database was migrated from MySQL to PostgreSQL, which holds more advanced and efficient geographical information system (GIS) functions through its PostGIS extension. To increase the data storage efficiency, several many-to-many relationships were built, such as between articles and authors (Fig. 1). In addition to these minor modifications, MOSAIC v.2.0 has additional tables to incorporate new variables (Fig. 1) (see Sect. 3). To increase the utility of the database, a few changes were made in the reported metadata to overcome the conundrum of sharing unstandardized data (Borgman, 2012). Some of the issues addressed in this new database are outlined below.
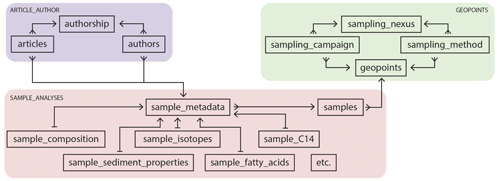
Figure 1Schematic representation of the structure of MOSAIC v.2.0, its tables, and inter-relationships, omitting primary keys and foreign keys for simplicity.
Despite relying on spatial data analysis, some studies in the field of geoscience still report their sample locations only through maps rather than providing their coordinates in tabular format (e.g. Guo et al., 2021; Hu et al., 2013; Pedersen et al., 1992; Zuo et al., 1991), hindering their addition to a harmonized database and contributing to the long tail of lost data in marine geoscience. With the improvement of map georeferencing tools (Hackeloeer et al., 2014), the locations of some of these sampled cores can be salvaged, resulting in an increase in the number of sediment cores included in MOSAIC v.2.0 by >1000 (Fig. S1 in the Supplement). However, the accuracy of these georeferenced data points may be less precise than those directly reported, so it is important to distinguish the source of the coordinates (“georeferenced_coordinates” column in the geopoints table). Similarly, studies rarely specify the exact sampling date of their samples, hindering a proper analysis of the temporal variations in sediment characteristics. The inconsistent reporting strategy of sampling date complicates the storage of this information in the database. One way to overcome this issue is separating the sampling date into year, month, and day to allow users to add as much temporal detail as possible, which has been done in other geospatial databases (Ke et al., 2022). For a full list of metadata attributes available for geopoints, see Table S1 in the Supplement. Finally, like for the previous version of MOSAIC, this new version also stores general categories of the sampling method of each geopoint since, depending on the sampling technique, marine sediment may be homogenized (e.g. dredges, grabs), may provide undisturbed sediment–water interfaces (e.g. box cores, multicores), or may lose surficial sediment through its deployment (e.g. gravity cores).
Following sample metadata-reporting strategies of analyses in other fields of geoscience (Morrill et al., 2021), the new version of the database includes a hierarchical explanation of the measurement technique used to obtain the data: a first high-level category classifies the general method employed to obtain the data, while the second level allows a free-text entry of specific details used in the method (Table S2 and Dataset S1 in the Supplement). The category of the methods for each variable was established and discussed through extensive bibliographic research and with the guidance of experts in the field. This categorical variable allows for a quick comparison of data obtained using different methods or for us to filter data based on the general method employed. For instance, data of OC content can be obtained through an elemental analyser, mass loss through combustion, coulometry, titration, or manometric measurements, while information in the second category would allow a more detailed explanation of the sample pre-treatment, the specific equipment model employed, or its instrumental settings (Fig. S2 in the Supplement). In the case of grain size analysis, the most commonly used methods are sieving, particle-settling time using Stokes' law, and laser diffraction, which can provide variable results depending on the characteristics of the sediment (Celia Magno et al., 2018), while the user could specify the sample pre-treatment (i.e. combustion, wet oxidation) in the method details (Fig. S2). In the case of biomarker data, such as for alkanes and fatty acids, we encourage the user to add the measured homologues or chain lengths in the method details in order to facilitate comparability between studies. Since this database is a collaborative effort, if an important category of the analytical method is not included in MOSAIC, we urge researchers to contact us so that it may be incorporated into future versions.
MOSAIC v.2.0 allows the specification of the material or fraction analysed for each measurement conducted (e.g. bulk sediment, OC, grain size, density, and specific compound class or fraction) with “material_analyzed” (Table S3 in the Supplement). This enables efficient storage and query of the analyses performed on the same sample but on different fractions, leading to a quick comparison of the OC content and isotopic composition in the different grain size or sediment density fractions to be assessed. For instance, the effect of hydrodynamic processes can be assessed by analysing different grain size or density fractions (Ausín et al., 2021; Bao et al., 2019; Bruni et al., 2022), while analysing the radiocarbon contents of different compounds can provide insight into the origin, reactivity, and/or transit time of OC through the system (Eglinton et al., 2021; Kusch et al., 2021). This specification of the material analysed allows for an easy incorporation of Δ14C values analysed into specific fractions, such as bulk OC in marine sediments, as well as bulk CaCO3 or foraminiferal carbonate.
MOSAIC v.2.0 also improves the link of the reference (e.g. DOI) for each analysis. In the previous database structure, a sample was associated with one reference. Consequently, if the same sample was presented in two different studies with complementary analyses (e.g. sedimentological and biomarker properties), they would be assigned different sample identifiers, hindering the assessment of the relationships between these analyses. To overcome this and incorporate the additional metadata (methods, material analysed), a new sample metadata table was devised that allowed the pairing of different analyses together while retaining their respective reference, measurement method, and material analysed (Table S2). In this metadata table, each row represents a specific measurement conducted on a specific sample, material (fraction) analysed, method, reference, and replicate, if applicable. This structure allows the same sample to be assigned different references based on the type of analysis conducted, method employed, and/or material analysed. Finally, an additional column indicates whether the value was provided by the user or was calculated through harmonization techniques (see Sect. 2.2.3).
2.2 MOSAIC v.2.0 data ingestion pipeline
The pipeline for the incorporation of data into MOSAIC v.2.0 follows a similar format as in the previous version, with a first step of data ingestion, followed by a quality check, then its population to the database, and finally its incorporation into a user-friendly website where data can be visualized and extracted. In this section, we outline the changes applied to each step of the pipeline and the reasons that motivated these changes. The data ingestion template and scripts that automate the quality check and database population can be found in the GitHub repository (Paradis et al., 2023).
2.2.1 Data ingestion
As with the previous iteration of MOSAIC, an Excel template workbook is provided, with separate sheets based on the type of data to be submitted (article where data are stored, information about the sampling location, and analyses conducted). With the expansion of the number of variables included in MOSAIC v.2.0, the previous spreadsheet file needed to be modified to avoid an excessive number of columns. Instead, the variables are classified based on their tables (Dataset S2 in the Supplement), and a drop-down menu allows users to select which variables they want to provide, allowing users to personalize the template according to their dataset. Once the user has chosen a variable to be ingested, another drop-down menu appears with the list of general methods that the database accepts, as well as the material or fraction analysed (see Sect. 2.1). This data ingestion workbook can be downloaded either from its GitHub repository (Paradis et al., 2023) or from the MOSAIC website (http://mosaic.ethz.ch, last access: 26 July 2023). The GitHub repository also provides a tutorial on how to fill in the template, along with an example workbook (Paradis et al., 2023).
2.2.2 Data quality check
The previous quality check structure simply determined whether the data provided for each variable were within a specified (i.e. plausible) range. In this new ingestion pipeline, the Python script was expanded to raise an error if the data are not in a specified format or are not within a specified range. If specified, the algorithm also compares the data with data stored in other columns and raises an error if the criteria are not met. For instance, this comparison ensures that the error values are lower than the variable value itself or that the sum of certain variables equals a value (i.e. the sum of grain size fractions cannot be greater than 100 %) when appropriate. A warning message is raised if the data are not within a common range so that the curator can assess and, if necessary, correct the values. The full list of quality check parameters is provided in Dataset S1.
The script not only checks the values of the variables but also inspects if all required fields (i.e. core name, sample name, exclusivity clause, etc.) are provided. In the case of article information, the script automatically extracts corresponding metadata stored in Cross-Ref using the article's digital object identifier (DOI), if provided. To prevent errors in the geographical positioning, the algorithm checks if the cores are located in the ocean using the NOAA high-resolution coastline GSHH v.2.3.7 product (Wessel and Smith, 1996) and adds complementary geospatial information such as its sea (Flanders Marine Institute, 2018), exclusive economic zone (EEZ) (Flanders Marine Institute, 2019), Longhurst province (Longhurst et al., 1995), and MARCAT code (Laruelle et al., 2013). If the water depth is not provided, the algorithm extracts data from the GEBCO bathymetric database (GEBCO Compilation Group, 2022) and specifies this in the geopoint metadata (Table S2).
2.2.3 Data population
To upload data in the previous iteration of MOSAIC, a Python script separated the input template based on the individual SQL tables, which then had to be manually uploaded to the database (van der Voort et al., 2021). However, this process is tedious and does not check if the data already exist in the database, potentially leading to duplicate entries. In MOSAIC v.2.0, a new Python script automates the data ingestion while querying the database to prevent duplicate data. This process is explained in more detail below for each level of the database's hierarchy (Fig. 2).
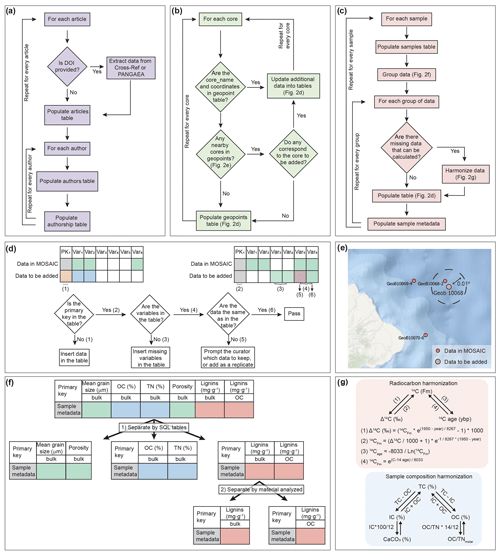
Figure 2Schematic diagrams of data population in MOSAIC v.2.0. Population workflow for (a) article information, (b) geopoint locations, and (c) sample analyses. (d) Population workflow of tables to avoid duplicates and to link different analyses to the same sample. Colours in the tables indicate similarities in the values between each row (data in MOSAIC vs. data to be added). (e) Database query for nearby cores. (f) Sample analysis table grouping to populate each individual table. (g) Example of harmonization workflow of radiocarbon analyses and sample composition.
The population workflow of the article and author sheet is summarized in Fig. 2a. This population is best achieved when the DOI is provided since the script extracts the standardized metadata from Cross-Ref, a repository of research objects that use DOIs, or PANGAEA (Diepenbroek et al., 2002), the biggest Earth science repository. This also allows automatic population of all the co-authors of each study without requiring the user to upload this information, which can be tedious if the manuscript has many co-authors. However, if the DOI is not provided, the script can still populate all the provided data. This data population is done for each row and iterates through the sheet by first populating the information related to the research article (title, year of publication, journal, and DOI) and assigning it an identifier. The script then iterates through the authors, assigns an identifier to each author, and finally creates the authorship table that stores data of this many-to-many relationship (Fig. 2a). Throughout this population, the script queries the database to ensure that the information that is being added is not already in it.
Population of the data associated with each location (geopoint) is similarly managed (Fig. 2b) but requires overcoming an additional handicap. As mentioned previously, given the high costs and complicated logistics of oceanographic cruises, these sampling campaigns are often conducted to achieve several research goals. Hence, sediment cores are often collected to conduct different analyses on the same samples, leading to the publication of data originating from the same sediment core in independent research articles (e.g. Palanques et al., 2022; Paradis et al., 2021b). Unfortunately, the same sediment core may be published using different naming conventions (e.g. Goñi et al., 2006; Gordon and Goñi, 2003), which complicates assignment of the correct geopoint identifier to the new sediment core. Unfortunately, this may not be circumvented by matching either the coordinates or the sampling date since studies report their coordinates in different units (decimal degrees, decimal minutes, or decimal minutes seconds) and with different precision, whereas the sampling date is often not fully provided (e.g. only the year or month is often reported). For instance, the outcomes of the EUROSTRATAFORM project were published in different studies, with different coordinate precisions and slight variations in the naming convention (Kiriakoulakis et al., 2011; Masson et al., 2010; de Stigter et al., 2007). To account for this, the new population algorithm first queries the database to check if the exact same core name and coordinates are already available. If not, rather than assuming that the core is not in the database, the algorithm then queries if there are any nearby cores within 0.6 arcmin (∼1 km at the Equator), and if this should be the case, it prompts the curator to check whether any of these nearby cores are actually the same (Fig. 2e).
The matching of geopoints allows different analyses presented in separate studies to be linked, enhancing the scientific richness of the database. A similar protocol is applied to the sample analysis data (Fig. 2c), but this is further complicated by the complexity of corresponding metadata given that the structure of this new database allows the specification of the material that is analysed (i.e. bulk sediment, OC, grain size fractions, and compound-specific analyses), as well as of the method employed. Hence, before populating the database, the data are first separated based on the material analysed and methods employed to allow for efficient storage of this metadata (Fig. 2f and Table S2). To further enrich MOSAIC v.2.0, automatic calculations harmonize and expand the database during the ingestion (Fig. 2g and Table S4 in the Supplement). For instance, this new version implements calculations to harmonize radiocarbon data between fraction modern (F14C), Δ14C, and radiocarbon age, as defined by Stuiver and Polach (1977) and as specified in the previous MOSAIC version (van der Voort et al., 2021). The sample metadata are then stored according to whether they were calculated through this data harmonization step (calculated data) or whether they were provided in the publication (reported data). This harmonization is performed for each group of data and then populates the SQL table. Since all the variables stored in a table are not always provided in a study, the data population workflow should allow complementary analyses to be added to the same sample. To do so, the population algorithm goes through a series of steps, adding complementary analyses if these are missing in the database or prompting the curator to take action (replace or assign as replicate) if the data to be added differ from the data that are already in the database (Fig. 2c). These data population workflows enable the linkage of different datasets with complementary analyses in them, allowing researchers to provide datasets that do not necessarily include carbon measurements but include variables that can provide a deeper understanding of the processes that affect the fate of OC in marine sediments (isotopic compositions, sedimentological properties, and biomarker concentrations; see Sect. 3).
The number of variables stored in MOSAIC v.2.0 increased by a factor of 10 in comparison to the previous iteration (Fig. S3 in the Supplement), which only contained data of OC, total and organic nitrogen, CaCO3, biogenic silica, and the isotopic composition of OC (13C and 14C) (van der Voort et al., 2021). These initial variables are crucial to understand variations in the geochemical signature due to the degradation and ageing processes of OC (Fig. 3a) or its sources since contrasting fractionation processes and radioactive decay lead to distinct isotopic signatures of OC depending on its source and history (Fig. 3b). As many more factors can also affect the distribution of OC in marine sediments (Bianchi et al., 2018; Blair and Aller, 2012), MOSAIC v.2.0 incorporates additional variables, including sedimentological parameters (Sect. 3.1) and specific biomarkers (Sect. 3.2).
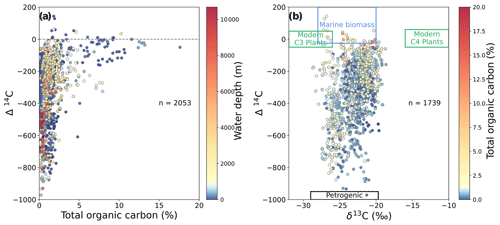
Figure 3(a) Relationship between OC content and Δ14C based on water depth. Note that, in shallow environments (<200 m), there is a wide variability of OC content and age, whereas in deeper settings, OC and radiocarbon content converge to lower content and older ages. (b) Scatter plot of the isotopic composition (13C and 14C) of OC in surface sediment stored in MOSAIC v.2.0 and the isotopic signatures of the main endmembers: marine biomass (Verwega et al., 2021), C3 and C4 plants (Bender, 1971; Farquhar et al., 1989), and petrogenic organic carbon (Copard et al., 2022; Hilton et al., 2010; Walinsky et al., 2009).
3.1 Sedimentological properties
One of the additions in MOSAIC v.2.0 was the incorporation of variables related to the sedimentological properties, such as sediment dry bulk density, porosity, grain size parameters, and mineral surface area. These additional variables are key for understanding the underlying reasons affecting the distribution of OC in continental margins. In general, OC is preferentially adsorbed to finer-grained sediments with a higher mineral-specific surface area (Keil et al., 1998; Mayer, 1994) (Fig. 4). Moreover, its mineral binding can also serve as a protective matrix that prevents the degradation of OC (Hedges and Keil, 1995; Hemingway et al., 2019). Hence, the hydrodynamic sorting of mineral particles due to differences in grain size affects the transport of OC while regulating its ageing and degradation (Ausín et al., 2021; Bao et al., 2019).
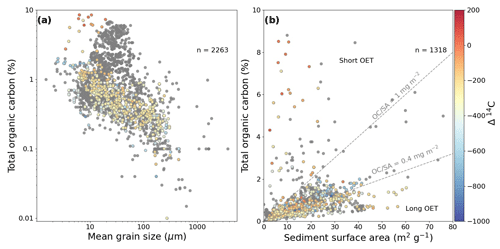
Figure 4Relationship between OC content and (a) sediment grain size fraction and (b) sediment surface area in marine sediments. The colour bar shows the ranges of Δ14C, if available (grey symbols indicate samples lacking concomitant 14C data). Lines in (b) show the specific ranges of the surface loading of different sedimentary environments (>1, 0.4–1, <0.4 mg m−2) based on the relative oxygen exposure time (OET), as explained by Mayer (1994).
During the data compilation and harmonization, we noted that different laboratories use contrasting definitions for clay fraction. While some laboratories define clay as particles that are smaller than 2 µm (e.g. Bruni et al., 2022; Schwab et al., 2021), others define it as the sediment fraction that is smaller than 4 µm (e.g. Hastings et al., 2012; Khan et al., 2020). To avoid confusion, two variables were created for the clay fraction, defining which threshold was used to define it (<4 µm, <2 µm). Moreover, during the data ingestion, grain size fractions are harmonized, calculating missing grain size fractions whenever possible to enrich the dataset (see Sect. 2.2.3 for more details).
Since different grain size fractions and density fractions present distinct sediment transport properties and may protect OC differently, several studies also analyse OC and its geochemical composition in different grain size or density fractions, which can be efficiently stored in MOSAIC v.2.0 by specifying the fraction analysed (“material_analyzed” column in the sample metadata table). For instance, the data stored in MOSAIC v.2.0 show the dual effect of organo-mineral associations, where the easily resuspended coarser silt fraction (20–63 µm) undergoes greater degradation and ageing of OC, while mineral surfaces in finer size fractions promote the protection of OC associated with these fractions (Fig. 5), a global process that occurs in all continental margins with different intensities depending on their depositional environments (Ausín et al., 2021; Bao et al., 2016, 2019; Bruni et al., 2022; Coppola et al., 2007).
3.2 Specific biomarkers
MOSAIC v.2.0 was also expanded to incorporate several groups of widely used biomarker compounds to better constrain the origin and degree of the degradation of organic matter in continental margins worldwide. Although the variety of biomarkers measured in marine sediments is vast, we have focused on those that derive into lignin-derived phenols, long-chain alkanoic (fatty) acids, alkanes and alcohols, and alkenones, given their wealth of existing data. Numerous prior contributions provide a full description of the origin and distribution of these biomarkers (e.g. Bianchi et al., 2018; Blair and Aller, 2012; Diefendorf and Freimuth, 2017; Sachse et al., 2012; Thevenot et al., 2010).
Lignin phenols
Lignin is a structural molecule that is almost exclusively found in the tissue of vascular (land) plants, and thus, it is used as a tracer of terrestrial biogenic organic matter in marine sediments (Bröder et al., 2016; Goñi and Hedges, 1990; Gordon and Goñi, 2003; Hedges and Mann, 1979; Prahl et al., 1994; Tesi et al., 2007). Lignin-derived phenols produced from oxidative alkaline hydrolysis of samples (Hedges and Ertel, 1982) can be separated into three main compound classes based on their molecular structure and origin: vanillyl (or guaiacyl) phenols (VP – angiosperms and gymnosperms), syringyl phenols (SP – gymnosperms), and cinnamyl phenols (CP – non-woody grasses) (Hedges and Mann, 1979). In addition to lignin phenols, cutin acids are also important tracers of terrestrial OC since they are only present in non-woody grasses and leaves (Goñi and Hedges, 1990). Given their distinct origin, ratios of the different phenols (SP/VP and CP/VP) can help elucidate the origin of plant sources (Goñi et al., 1998, 2000), although this can also be affected by hydrodynamic sorting of particles enriched in SP and CP relative to VP (Bianchi et al., 2002; Pasqual et al., 2013). Similarly, the different proportions of acid and aldehydes within the vanillyl and syringyl phenolic groups can also provide an indication of the degree of degradation of terrestrial organic matter given the higher reactivity of aldehydes with respect to acids (Gordon and Goñi, 2004; Tesi et al., 2012).
Long-chain n-alkyl lipids
Fatty acids, alkanes, and alcohols are naturally present in both marine and terrestrial organic matter but with variable carbon chain lengths. In general, fatty acids, alkanes, and alcohols produced by marine organisms tend to be comprised of less than 24 carbon atoms and are often referred to as low-molecular-weight (LMW) lipids. In contrast, terrestrial vascular plant leaf waxes are typically characterized by longer chain lengths (≥24 carbon atoms) and are hence referred to as being of high molecular weight (HMW) (Eglinton and Hamilton, 1967). These compounds have been used to elucidate the sources of organic matter in marine sediments, as well as to determine the contribution of organic matter from anthropogenic activities (Bai et al., 2021; Feng et al., 2013; French et al., 2018; Mead and Goñi, 2006).
Alkenones
Long-chain (typically >C35) unsaturated ketones (alkenones) are produced by a specific kind of marine phytoplankton, coccolithophores, and are well preserved in marine sediments. These compounds serve as useful proxies of marine primary productivity (Raja and Rosell-Melé, 2021), as well as being useful for the reconstruction of past sea surface temperatures (Eglinton et al., 2001; Marlowe et al., 1984; Tierney and Tingley, 2018; Volkman et al., 1980). These biomarkers are stored in separate tables based on their general compound classes. Since the characteristics of total long-chain n-alkyl lipids (e.g. fatty acids, alkanes, and alcohols) depend on the specific carbon chain lengths measured, we specify the measured homologues in the method details to improve the comparability of data. In addition, MOSAIC v.2.0 stores the individual concentrations of specific homologues (e.g. C16 fatty acids, C18 fatty acids, and C29 alkanes) to allow researchers to calculate the total concentrations within specific carbon chain lengths (e.g. HMW or LMW).
Abundances of these biomarkers are often reported based on bulk sediment concentration (as µg per g dry weight sediment) or normalized by the OC content of the sample (as µg per g OC), which is specified in the metadata stored in MOSAIC v.2.0 as the material analyzed (bulk sediment, OC, sediment fractions, etc.). Hence, concentrations of the biomarkers can be provided in either format. This data storage architecture also allows for an efficient storage of data from compound-specific stable isotope analysis (CSIA) or compound-specific radiocarbon analysis (CSRA), providing a detailed overview of the sources and pathways of organic matter deposited in marine sediments (Feng et al., 2013; French et al., 2018; Gibbs et al., 2020; Gustafsson et al., 2011; Hahn et al., 2017; Huang et al., 2000; Kusch et al., 2010; Tao et al., 2016; Wakeham and McNichol, 2014; Yu et al., 2022).
For instance, since individual biomarkers have distinct sources, transit times, and reactivities, CSRA is useful for determining the diagenetic state of organic matter and depositional processes of specific biomarkers, which would be masked when analysing only the bulk OC (Eglinton et al., 1997; Kusch et al., 2021). The initial compilation of compound-specific 14C data for lignin phenols, fatty acids, alkanes, alcohols, and alkenones stored in MOSAIC v.2.0. underlines the contrasting radiocarbon age offsets due to the different origins and reactivities (Fig. 6). For instance, while bulk OC analyses show Δ14C values that range between ‰ and ∼50 ‰, Δ14C values of terrestrial biomarkers are significantly lower than for marine biomarkers, indicating older radiocarbon ages of terrestrial organic matter depositing in marine sediments. This trend generally occurs since terrestrial OC has a longer transit time from its production in terrestrial environments until its deposition in marine sediments in comparison to marine OC, whose biomarkers tend to retain the 14C signal from the surface ocean, which is closely coupled with the atmospheric signal. In the case of terrestrial biomarkers, there is a progressive 14C depletion among HMW (plant wax) compound classes, following the order of , which is attributed to the contrasting reactivity of these compounds (see review by Kusch et al., 2021). Similarly, different marine biomarkers show different OC ageing due to their different reactivities and transit times prior to deposition on the seafloor (Bröder et al., 2018; Feng et al., 2013; Hu et al., 2014; Mollenhauer and Eglinton, 2007; Tao et al., 2016; Wakeham and McNichol, 2014; Yu et al., 2022). These contrasting radiocarbon ages in biomarkers help shed light on the origin and biogeochemical processes affecting the distribution of OC, which would be masked if only the bulk sediment was analysed.
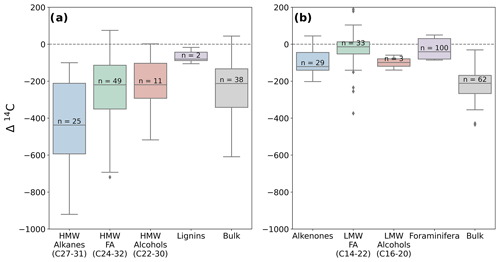
Figure 6Box plot of compound-specific radiocarbon analyses (shown as Δ14C values) for different terrestrial (a) and marine (b) biomarkers. Radiocarbon analyses of bulk OC in marine sediments, as well as in planktonic foraminifera, are also provided to contextualize molecular Δ14C values. The number of independent samples measured for each compound class is annotated in each box plot. Note that the box plots may encompass Δ14C values of several individual compounds from the same sample. FA refers to fatty acids.
3.3 Future expansions
MOSAIC v.2.0 considerably broadened the range of variables, including those that could account for the effect of hydrodynamic processes and mineral protection of OC (Sect. 3.1), as well as specific biomarkers that could further refine its sources (Sect. 3.2). However, future versions of MOSAIC will expand the breadth of variables even further to improve our understanding of the processes affecting the fate of OC in marine sediments. For instance, the inclusion of additional variables such as clay mineralogy and the concentrations of major (e.g. Al) and trace (e.g. Nd) metals, as well as their isotopes, can provide additional insights into sediment provenance and transport pathways along continental margins (Blanchet, 2019; Fagel, 2007; Jeandel et al., 2007; Li et al., 2023; Liu et al., 2010; Schwab et al., 2021). Additional source-specific biomarkers such as glycerol dialkyl glycerol tetraethers (GDGTs) (Damsté et al., 2002; Koga et al., 1993), long-chain alkyl diols (de Bar et al., 2020), and sterols (Tao et al., 2022) could further define the origin of organic matter, while other biomarkers such as algal-derived pigments, biopolymeric fraction of carbon, amino acids, and carbohydrates can determine its degree of reactivity (Burdige and Martens, 1988; Dauwe and Middelburg, 1998; Pusceddu et al., 2009; Raja and Rosell-Melé, 2022). Additionally, new proxies are continuously being proposed that can further disentangle the source of organic matter (Lattaud et al., 2021) and help refine the use of biomarker proxy calibration (Tierney and Tingley, 2014, 2018), which can be affected by sediment redistribution and degradation processes (Ausín et al., 2022; Lattaud et al., 2022). Future efforts will be directed into including these variables in MOSAIC to gain a holistic understanding of the fate of organic matter in marine sediments.
Although the main spatial focus of MOSAIC is the continental margin to understand the processes that affect carbon cycling and burial in these heterogenous and complex areas, its spatial coverage extends to marine sediments on a global scale. This includes samples collected in estuaries, inner and outer shelves, slopes, abyssal plains, and sediment from the open ocean but currently excludes intertidal areas and complex blue-carbon ecosystems such as mangroves and salt marshes.
The number of individual locations in which data are stored in MOSAIC v.2.0 is now 21 539 compared to ∼4000 in the first iteration, filling in data gaps such as in the Chukchi Sea, the Bering Strait, the Gulf of Mexico, the Greenland and Norwegian seas, the North Atlantic, the southwestern Atlantic, the Mediterranean, the Persian Gulf, the Bay of Bengal, the Gulf of Thailand, the South China Sea, and Australasia (Fig. 7a). Despite more than quadrupling the number of sampling locations stored in this new iteration, there remain substantial gaps in certain areas, such as the continental margins of eastern Africa and Madagascar (western Indian Ocean) and in Mesoamerica (Fig. 7a). This lack of an independent and identical distribution of data (i.e. an even spread of data points) of marine sediments on a global scale can skew spatial analyses to perform well in oversampled areas but poorly in underrepresented areas (Meyer and Pebesma, 2022). While data exploration and compilation remain far from complete and are ongoing, we emphasize that more effort should be made to sample unrepresented sites if we want to produce reliable maps of the global distribution of OC and other geochemical properties (Fig. 7a).
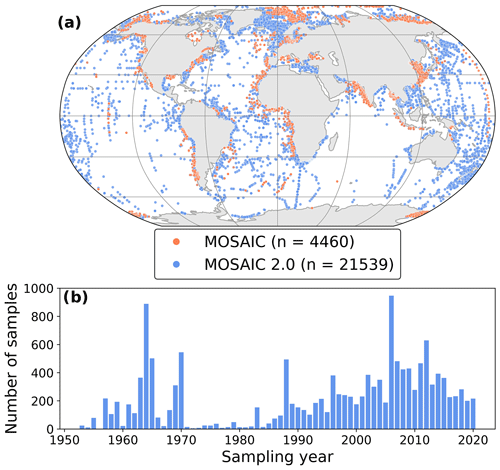
Figure 7(a) Spatial distribution of sampling locations stored in the first iteration of MOSAIC (red) and additional data in MOSAIC v.2.0 (blue). Note the increase in spatial coverage for MOSAIC v.2.0. (b) Temporal distribution of the data points in MOSAIC v.2.0. The previous iteration of MOSAIC did not store information of the sampling year.
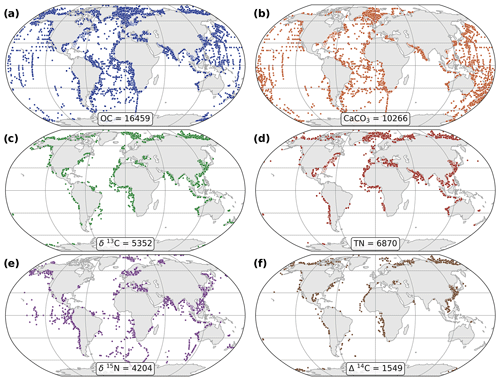
Figure 8Spatial distribution of sampling locations with surface and/or downcore data of (a) OC, (b) CaCO3, (c) δ13C of OC, (d) TN, (e) δ15N in acidified or non-acidified sediment, and (f) Δ14C of OC.
However, these sediment cores were collected in different years. Current global modelling approaches combine data collected in different decades in order to increase spatial coverage but ignore evidence that OC in surficial sediment has been changing in recent years due to direct (e.g. demersal fisheries or mining; Keil, 2017; Paradis et al., 2019, 2021a; Clare et al., 2023) or indirect (e.g. land use changes and climate change; Bröder et al., 2021) anthropogenic impacts, which impact virtually the entire marine environment (Halpern et al., 2008). In order to study temporal variations in the geochemical composition of OC in surficial marine sediments over the last century, we have included the sampling date in MOSAIC v.2.0. Out of the 21 539 sediment cores stored in the database, 68 % provide information of the sampling year, spanning from the 1950s until 2020 (Fig. 7b). According to the data available in MOSAIC v.2.0, the number of sediment cores collected during the last decades increased drastically, from ∼1000 sediment cores during the 1950s to >10 000 since the 1990s. However, the greater availability of published data and its digitalization in recent decades likely generate an inaccurate impression that more sampling has occurred over the last decades and highlight the need to continue to digitize hard-copy data collected prior to the 1990s. Nevertheless, the large number of sediment cores collected over these decades underlines the need for greater efforts to build and maintain curated databases and to ensure harmonized and machine-readable data, which represent a significant return in investment in terms of these numerous oceanographic cruises (Lee et al., 2023). Hence, this is an invaluable dataset that will allow us not only to understand the spatial distribution of OC and its composition but also to assess how these have been changing over the last decades.
Despite the widespread geographical distribution of sampling locations in MOSAIC v.2.0, the spatiotemporal coverage of the variables stored in the database is relatively limited given the specificity of their analyses and the limited number of laboratories that can conduct each analysis. We are aware that the spatial extension of certain variables would substantially increase by performing a thorough systematic review of the available literature, and future versions of MOSAIC will be focused on this. In addition, we propose the further expansion of the spatiotemporal coverage of these less represented parameters by recovering legacy samples and performing additional analyses, circumventing the high costs and logistics of organizing and executing oceanographic cruises. Finally, we are also aware that the data stored in MOSAIC originate from English-based scientific journals and reports, which bias the availability of data. Future efforts should be directed towards retrieving what is likely to be a wealth of data residing in journals, reports, and data repositories written in other languages.
Below, we outline the spatiotemporal coverage of the main subgroups of variables: bulk and isotopic compositions, sedimentological properties, and biomarkers.
4.1 Distribution of bulk and isotopic compositions
As expected, the majority of the sampling locations stored in MOSAIC v.2.0 have data of sedimentary OC content, covering nearly all continental margins (Fig. 8a). In contrast, sediment cores with data of CaCO3 and total nitrogen (TN) contents and the isotopic composition of organic matter (δ15N, δ13C, and Δ14C) are less extensive. Despite the reduced spatial distribution of these variables, the number of locations has increased substantially since the last iteration of MOSAIC (van der Voort et al., 2021).
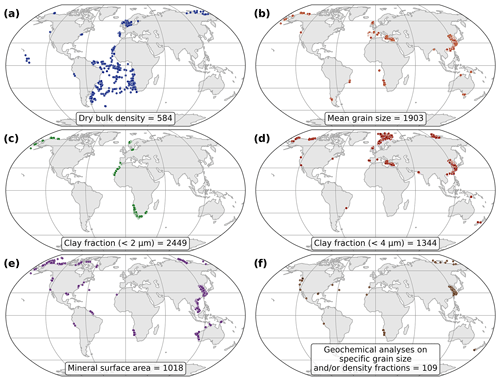
Figure 9Spatial distribution of sampling locations with surface and/or downcore data of (a) dry bulk density, (b) mean grain size, (c) clay fraction (<2 µm), (d) clay fraction (<4 µm), (e) mineral surface area, and (f) geochemical analyses in specific grain sizes and density fractions.
Nearly half of the sediment cores in MOSAIC v.2.0 have CaCO3 content data, partly due to the harmonization techniques that calculate variables when sufficient information is provided (see Sect. 2.2.3 for more details). Sediment cores with TN and δ13C generally have a similar spatial distribution, with good spatial coverage in the Arctic, Asian, Arabian, American, west African, and Australian margins, though this is to a lesser extent than with OC and CaCO3 and with much fewer sampling points in the open ocean (Fig. 8c and d). Finally, sediment with δ15N data is distributed along the western South American, North American, African, Asian, and Australian margins, as well as in the Mediterranean Sea, (Fig. 8f).
Given the high costs and the limited number of laboratories that can analyse 14C, the spatial coverage of radiocarbon data is the least comprehensive (Fig. 8f). Given the radiocarbon-centric nature of this database, substantial efforts have been made to compile published radiocarbon analyses performed in marine sediments, and the spatial distribution of this variable has increased the most in this new iteration of the database, more than doubling from ∼500 data points to ∼1500, filling in regions surrounding the African, American, Asian, and Arctic continental margins that were not represented in the previous iteration of MOSAIC. However, more efforts are needed to expand the spatiotemporal coverage of 14C given its invaluableness as a tool for understanding both the origin and age of OC deposited in marine sediments.
The lower representation of isotopic analyses in sediment cores stored in MOSAIC highlights that more efforts are needed to recover legacy sediment cores from unrepresented margins and conduct additional analyses in these samples. Analysing TN, δ15N, δ13C, and Δ14C in these legacy samples would help constrain the origin and age of organic matter, shedding light on the biogeochemical processes occurring along continental margins.
4.2 Distribution of sedimentological properties
As mentioned in Sect. 3, the previous iteration of MOSAIC did not hold any data regarding the sedimentological properties of samples. Despite efforts to compile sedimentological data, the spatial coverage of sedimentological parameters in MOSAIC v.2.0 remains limited in comparison to that of OC (Figs. 8–9). Further efforts will focus on compiling and harmonizing these data from continental margins since there is clear spatial bias in the data available.
For instance, dry bulk density is a crucial parameter to calculate the OC stock in marine sediment, but this variable is seldom reported (Fig. 9a), forcing researchers to infer it from other variables (Atwood et al., 2020; Diesing et al., 2017, 2021; Smeaton et al., 2021). We therefore urge researchers to measure and report dry bulk density in order to properly calculate carbon stocks. Similarly, mineral surface area is only reported in relatively few studies, which limits the ability to assess the role of mineral surfaces in the stabilization of OC.
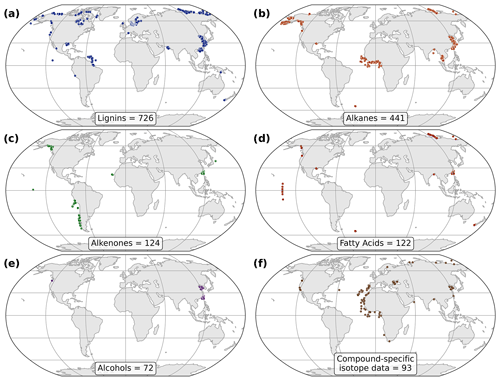
Figure 10Spatial distribution of sampling locations with surface and/or downcore data of (a) lignins, (b) alkanes, (c) alkenones, (d) fatty acids, (e) alcohols, and (f) compound-specific stable isotopic or radiocarbon analyses in any of the above-mentioned compounds.
Regarding variables related to sediment grain size, although the majority of studies measure grain size distributions, there is no consensus on how such data should be reported. While some studies only report mean (or median) grain size values (Fig. 9b), others report the relative proportion of different grain size classes (e.g. sand, silt, clay). However, as mentioned earlier, there are contrasting definitions for clay sizes (Fig. 9c and d). These different reporting strategies for grain size data lead to a broad spatial coverage of grain size analyses which cannot easily be harmonized. Similarly, some studies report mineral surface area (Fig. 9e), which is linked to both grain size and mineralogy; thus, a harmonization between these variables should be explored to further expand the richness of this database.
Finally, as an effort to understand the effect of hydrodynamic sorting in the distribution of OC in marine sediments, MOSAIC v.2.0 also includes the possibility of adding geochemical analyses performed on specific grain sizes and density fractions. However, studies assessing this are currently very limited and only cover the East China Sea (Bao et al., 2018, 2019; Wang et al., 2015), the North American margin (Ausín et al., 2021; Coppola et al., 2007; Wakeham et al., 2009), and the Namibian margin (Ausín et al., 2021; Bruni et al., 2022), with a few additional sampling locations on the Peruvian, Iberian, north African, and New Zealand margins (Ausín et al., 2021; Bergamaschi et al., 1997; Cui et al., 2016) (Fig. 9f). In order to understand the global effect of grain size sorting and mineral protection, there is a need to expand these analyses to continental margins with different environmental conditions.
4.3 Distribution of biomarkers
As with variables related to sedimentological properties, the previous iteration of MOSAIC did not hold any biomarker data. Despite ongoing efforts to compile data of biomarkers, its spatial coverage in MOSAIC v.2.0 remains very limited, largely due to the time-intensive nature of compiling this complex multivariate data for ingestion into the database (see Sect. 3.2). Instead of an exhaustive literature search for biomarker data, we highlight locations where data of these biomarkers are currently available in MOSAIC v.2.0. We recognize that extensive studies on many continental margins have been undertaken, and efforts are ongoing to compile and harmonize this data into future versions of MOSAIC. Nevertheless, the goal in MOSAIC v2.0 is to develop a framework for ingestion and organization of biomarker data such that the community can contribute to and provide feedback on its subsequent expansion.
Of the biomarkers included in MOSAIC v.2.0, lignin phenols have the greatest spatial distribution, with data available from the North American margin, offshore the Amazon basin, the Arctic margins, the Baltic Sea, and the east Asian margin and in some areas of the European, Arabian, Indonesian, and New Zealand seas (Fig. 10a). Despite the greatest coverage of lignin phenols, these data almost exclusively derive from the Northern Hemisphere, limiting a proper understanding of controls on a global scale of this group of biomarker compounds, and efforts are ongoing to compile these data together. Alkanes have been extensively analysed along the Alaskan, east Asian, and equatorial west African margins, as well as in the Gulf of Mexico and South Georgia islands, but the database does not yet include any data from other continental margins (Fig. 10b). Included alkenone data are currently distributed along the eastern South American margin, in the Alaskan margin, and in the east Asian margin. Some isolated data points have been analysed as well in the northwestern African and Japanese margins and in the Pacific (Fig. 10c). Alcohols and fatty acids are the least represented biomarkers in MOSAIC v.2.0. The database only has data for fatty acids in the east Asian margin, in certain sites in the North American and Arctic margins, and in the South Georgia islands (Fig. 10d). In contrast, the database has data of alcohols only in the east Asian margin (Fig. 10e). Finally, data on compound-specific stable isotope and compound-specific radiocarbon analyses in MOSAIC v.2.0 are thus far only available along the North American, Iberian, west African, Arabian, Indian, east Asian, and Arctic margins, as well as in the Baltic and Black seas (Fig. 10f).
The limited data coverage of the targeted groups of biomarkers that have been ingested thus far in MOSAIC v.2.0 highlights the need to compile and harmonize published biomarker data from all continental margins, as well as to analyse available samples from undersampled or underreported regions in order to add this valuable source of information on the origin of OC in continental margins and the biogeochemical processes that occur along them. As mentioned earlier, future versions of MOSAIC will be focused on including more biomarkers (see Sect. 3.3) and linking existing databases of marine biomarker proxies (de Bar et al., 2020; Tierney and Tingley, 2015, 2018) while expanding their spatiotemporal coverage in MOSAIC.
As in the previous MOSAIC version (van der Voort et al., 2021), the SQL data can be found in the Supplement (https://doi.org/10.5281/zenodo.8322094, Paradis, 2023) and through the website (https://doi.org/10.5168/mosaic019.1, Van der Voort et al., 2019; http://mosaic.ethz.ch/, last access: 26 July 2023), where users can interactively visualize the data in plots, as well as download a subset of the database (Fig. S4 in the Supplement). Since its publication, the website presents further functionalities that will promote the user's interaction with the database: (1) downloading the whole spatial extension of the database in a spreadsheet, (2) downloading the input template, and (3) uploading the template with new data to contribute to the database. Given the complexity of the new data stored in MOSAIC v.2.0, only selected analyses are available on the website, but users can download the full SQL database from the Supplement (https://doi.org/10.5281/zenodo.8322094, Paradis, 2023).
We present here a new version of MOSAIC, v.2.0, which, compared to the initial version, has expanded its spatiotemporal coverage by >400 % and incorporates additional variables (e.g. sedimentological properties and biomarkers) to facilitate a more comprehensive understanding of the processes that affect the distribution and degradation of organic carbon from different sources in marine sediments. In addition, this new database includes richer metadata that maximize the comparability of data, complying with FAIR data principles. We urge researchers to provide sufficient metadata in their studies (e.g. sampling technique, sampling dates, and details of analytical methods) in order to enhance the quality and utility of this database. While this new version of the database includes data from more than 21 000 individual sediment cores, further efforts are needed to compile and harmonize data from thus-far-unsampled areas to better understand the distribution of OC in marine sediments on a global scale and to apply novel machine learning techniques to identify different depositional environments and the factors that affect the distribution of organic carbon in marine sediments. Since this database is a collaborative effort, we urge the scientific community to continue to contribute to this growing database, which will further enhance its value and the research outputs it can provide.
The supplement related to this article is available online at: https://doi.org/10.5194/essd-15-4105-2023-supplement.
SP, TSVdV, and TE discussed the expansion of the MOSAIC database. SP, KN, and AW collected data and contributed to the discussion of the data ingestion. SP restructured the database and perfected the ingestion pipeline through automated Python scripts. SP and TSVdV adapted the RShiny website for this expansion. HG, TMB, LB, and TE contributed to the discussion of appropriate metadata stored for each variable. SP wrote the paper with the help of all the co-authors.
The contact author has declared that none of the authors has any competing interests.
Publisher's note: Copernicus Publications remains neutral with regard to jurisdictional claims in published maps and institutional affiliations.
We would like to thank all the researchers who provided their data in tabular format: Meixun Zhao, Hu Limin, Yu Fengling, Ge Chendong, Kostas Kariakoulakis, Henko de Stigter, Rut Pedrosa-Pàmies, Miguel Goñi, Chun Zhu, Markus Diesing, Jonathan Nuwer, Craig Smeaton, and Helen Bostock. Their input contributed greatly to increasing the spatiotemporal content of this database. Special thanks goes to Matthias Brakebusch, whose discussions provided great insight into the database's structure.
This work received support from the Swiss National Science Foundation “Climate and Anthropogenic PerturbationS of Land-Ocean Carbon TracKs” project (CAPS-LOCK3, SNF200020_184865/1; CAPS-LOCK+, SNF200020_215163).
This paper was edited by Xingchen (Tony) Wang and reviewed by Helen Bostock and one anonymous referee.
Atwood, T. B., Witt, A., Mayorga, J., Hammill, E., and Sala, E.: Global Patterns in Marine Sediment Carbon Stocks, Front. Mar. Sci., 7, 165, https://doi.org/10.3389/fmars.2020.00165, 2020.
Ausín, B., Bruni, E., Haghipour, N., Welte, C., Bernasconi, S. M., and Eglinton, T. I.: Controls on the abundance, provenance and age of organic carbon buried in continental margin sediments, Earth Planet. Sc. Lett., 558, 116759, https://doi.org/10.1016/j.epsl.2021.116759, 2021.
Ausín, B., Haghipour, N., Bruni, E., and Eglinton, T.: The influence of lateral transport on sedimentary alkenone paleoproxy signals, Biogeosciences, 19, 613–627, https://doi.org/10.5194/bg-19-613-2022, 2022.
Avelar, S., van der Voort, T. S., and Eglinton, T. I.: Relevance of carbon stocks of marine sediments for national greenhouse gas inventories of maritime nations, Carbon Balance Manag., 12, 10, https://doi.org/10.1186/s13021-017-0077-x, 2017.
Bai, Y., Hu, L., Wu, B., Qiao, S., Fan, D., Liu, S., Yang, G., Liu, J., Kornkanitnan, N., Khokiattiwong, S., and Shi, X.: Impact of Source Variability and Hydrodynamic Forces on the Distribution, Transport, and Burial of Sedimentary Organic Matter in a Tropical Coastal Margin: The Gulf of Thailand, J. Geophys. Res.-Biogeo., 126, e2021JG006434, https://doi.org/10.1029/2021JG006434, 2021.
Bao, R., McIntyre, C., Zhao, M., Zhu, C., Kao, S.-J., and Eglinton, T. I.: Widespread dispersal and aging of organic carbon in shallow marginal seas, Geology, 44, 791–794, https://doi.org/10.1130/G37948.1, 2016.
Bao, R., van der Voort, T. S., Zhao, M., Guo, X., Montluçon, D. B., McIntyre, C., and Eglinton, T. I.: Influence of Hydrodynamic Processes on the Fate of Sedimentary Organic Matter on Continental Margins, Global Biogeochem. Cy., 32, 1420–1432, https://doi.org/10.1029/2018GB005921, 2018.
Bao, R., Blattmann, T. M., McIntyre, C., Zhao, M., and Eglinton, T. I.: Relationships between grain size and organic carbon 14C heterogeneity in continental margin sediments, Earth Planet. Sc. Lett., 505, 76–85, https://doi.org/10.1016/j.epsl.2018.10.013, 2019.
Bender, M. M.: Variations in the ratios of plants in relation to the pathway of photosynthetic carbon dioxide fixation, Phytochemistry, 10, 1239–1244, https://doi.org/10.1016/S0031-9422(00)84324-1, 1971.
Bergamaschi, B. A., Tsamakis, E., Keil, R. G., Eglinton, T. I., Montluçon, D. B., and Hedges, J. I.: The effect of grain size and surface area on organic matter, lignin and carbohydrate concentration, and molecular compositions in Peru Margin sediments, Geochim. Cosmochim. Ac., 61, 1247–1260, https://doi.org/10.1016/S0016-7037(96)00394-8, 1997.
Bianchi, T. S., Mitra, S., and McKee, B. A.: Sources of terrestrially-derived organic carbon in lower Mississippi River and Louisiana shelf sediments: implications for differential sedimentation and transport at the coastal margin, Mar. Chem., 77, 211–223, https://doi.org/10.1016/S0304-4203(01)00088-3, 2002.
Bianchi, T. S., Cui, X., Blair, N. E., Burdige, D. J., Eglinton, T. I., and Galy, V.: Centers of organic carbon burial and oxidation at the land-ocean interface, Org. Geochem., 115, 138–155, https://doi.org/10.1016/j.orggeochem.2017.09.008, 2018.
Blair, N. E. and Aller, R. C.: The Fate of Terrestrial Organic Carbon in the Marine Environment, Annu. Rev. Mar. Sci., 4, 401–423, https://doi.org/10.1146/annurev-marine-120709-142717, 2012.
Blanchet, C. L.: A database of marine and terrestrial radiogenic Nd and Sr isotopes for tracing earth-surface processes, Earth Syst. Sci. Data, 11, 741–759, https://doi.org/10.5194/essd-11-741-2019, 2019.
Borgman, C. L.: The conundrum of sharing research data, J. Am. Soc. Inf. Sci. Tec., 63, 1059–1078, https://doi.org/10.1002/asi.22634, 2012.
Bröder, L., Tesi, T., Salvadó, J. A., Semiletov, I. P., Dudarev, O. V., and Gustafsson, Ö.: Fate of terrigenous organic matter across the Laptev Sea from the mouth of the Lena River to the deep sea of the Arctic interior, Biogeosciences, 13, 5003–5019, https://doi.org/10.5194/bg-13-5003-2016, 2016.
Bröder, L., Tesi, T., Andersson, A., Semiletov, I., and Gustafsson, Ö.: Bounding cross-shelf transport time and degradation in Siberian-Arctic land-ocean carbon transfer, Nat. Commun., 9, 806, https://doi.org/10.1038/s41467-018-03192-1, 2018.
Bröder, L., Keskitalo, K., Zolkos, S., Shakil, S., Tank, S. E., Kokelj, S. V, Tesi, T., Van Dongen, B.E., Haghipour, N., and Eglinton, T.: Preferential export of permafrost-derived organic matter as retrogressive thaw slumping intensifies, Environ. Res. Lett., 16, 054059, https://doi.org/ 10.1088/1748-9326/abee4b, 2021.
Bruni, E. T., Blattmann, T. M., Haghipour, N., Louw, D., Lever, M., and Eglinton, T. I.: Sedimentary Hydrodynamic Processes Under Low-Oxygen Conditions: Implications for Past, Present, and Future Oceans, Front. Earth Sci., 10, 886395, https://doi.org/10.3389/feart.2022.886395, 2022.
Burdige, D. J. and Martens, C. S.: Biogeochemical cycling in an organic-rich coastal marine basin: 10. The role of amino acids in sedimentary carbon and nitrogen cycling, Geochim. Cosmochim. Ac., 52, 1571–1584, https://doi.org/10.1016/0016-7037(88)90226-8, 1988.
Byers, S. C., Mills, E. L., and Stewart, P. L.: A comparison of methods of determining organic carbon in marine sediments, with suggestions for a standard method, Hydrobiologia, 58, 43–47, https://doi.org/10.1007/BF00018894, 1978.
Celia Magno, M., Venti, F., Bergamin, L., Gaglianone, G., Pierfranceschi, G., and Romano, E.: A comparison between Laser Granulometer and Sedigraph in grain size analysis of marine sediments, Measurement, 128, 231–236, https://doi.org/10.1016/j.measurement.2018.06.055, 2018.
Clare, M., Lichtschlag, A., Paradis, S., and Barlow, N. L. M.: Assessing the impact of the global subsea telecommunications network on sedimentary organic carbon stocks, Nat. Commun., 14, 2080, https://doi.org/10.1038/s41467-023-37854-6, 2023.
Copard, Y., Eyrolle, F., Grosbois, C., Lepage, H., Ducros, L., Morereau, A., Bodereau, N., Cossonnet, C., and Desmet, M.: The unravelling of radiocarbon composition of organic carbon in river sediments to document past anthropogenic impacts on river systems, Sci. Total Environ., 806, 150890, https://doi.org/10.1016/j.scitotenv.2021.150890, 2022.
Coppola, L., Gustafsson, Ö., Andersson, P., Eglinton, T. I., Uchida, M., and Dickens, A. F.: The importance of ultrafine particles as a control on the distribution of organic carbon in Washington Margin and Cascadia Basin sediments, Chem. Geol., 243, 142–156, https://doi.org/10.1016/j.chemgeo.2007.05.020, 2007.
Cui, X., Bianchi, T. S., Hutchings, J. A., Savage, C., and Curtis, J. H.: Partitioning of organic carbon among density fractions in surface sediments of Fiordland, New Zealand, J. Geophys. Res.-Biogeo., 121, 1016–1031, https://doi.org/10.1002/2015JG003225, 2016.
Damsté, J. S. S., Schouten, S., Hopmans, E. C., van Duin, A. C. T., and Geenevasen, J. A. J.: Crenarchaeol, J. Lipid Res., 43, 1641–1651, https://doi.org/10.1194/jlr.M200148-JLR200, 2002.
Dauwe, B. and Middelburg, J. J.: Amino acids and hexosamines as indicators of organic matter degradation state in North Sea sediments, Limnol. Oceanogr., 43, 782–798, https://doi.org/10.4319/lo.1998.43.5.0782, 1998.
de Bar, M. W., Weiss, G., Yildiz, C., Rampen, S. W., Lattaud, J., Bale, N. J., Mienis, F., Brummer, G.-J. A., Schulz, H., Rush, D., Kim, J.-H., Donner, B., Knies, J., Lückge, A., Stuut, J.-B. W., Sinninghe Damsté, J. S., and Schouten, S.: Global temperature calibration of the Long chain Diol Index in marine surface sediments, Org. Geochem., 142, 103983, https://doi.org/10.1016/j.orggeochem.2020.103983, 2020.
DeMaster, D. J., Taylor, R. S., Smith, C. R., Isla, E., and Thomas, C. J.: Using Radiocarbon to Assess the Abundance, Distribution, and Nature of Labile Organic Carbon in Marine Sediments, Global Biogeochem. Cy., 35, e2020GB006676, https://doi.org/10.1029/2020GB006676, 2021.
de Stigter, H. C., Boer, W., de Jesus Mendes, P. A., Jesus, C. C., Thomsen, L., van den Bergh, G. D., and van Weering, T. C. E. E.: Recent sediment transport and deposition in the Nazaré Canyon, Portuguese continental margin, Mar. Geol., 246, 144–164, https://doi.org/10.1016/j.margeo.2007.04.011, 2007.
Diefendorf, A. F. and Freimuth, E. J.: Extracting the most from terrestrial plant-derived n-alkyl lipids and their carbon isotopes from the sedimentary record: A review, Org. Geochem., 103, 1–21, https://doi.org/10.1016/j.orggeochem.2016.10.016, 2017.
Diepenbroek, M., Grobe, H., Reinke, M., Schindler, U., Schlitzer, R., Sieger, R., and Wefer, G.: PANGAEA – an information system for environmental sciences, Comput. Geosci., 28, 1201–1210, https://doi.org/10.1016/S0098-3004(02)00039-0, 2002.
Diesing, M., Kröger, S., Parker, R., Jenkins, C., Mason, C., and Weston, K.: Predicting the standing stock of organic carbon in surface sediments of the North–West European continental shelf, Biogeochemistry, 135, 183–200, https://doi.org/10.1007/s10533-017-0310-4, 2017.
Diesing, M., Thorsnes, T., and Bjarnadóttir, L. R.: Organic carbon densities and accumulation rates in surface sediments of the North Sea and Skagerrak, Biogeosciences, 18, 2139–2160, https://doi.org/10.5194/bg-18-2139-2021, 2021.
Eglinton, G. and Hamilton, R. J.: Leaf Epicuticular Waxes, Science, 156, 1322–1335, https://doi.org/10.1126/science.156.3780.1322, 1967.
Eglinton, T. I., Benitez-Nelson, B. C., Pearson, A., McNichol, A. P., Bauer, J. E., and Druffel, E. R. M.: Variability in Radiocarbon Ages of Individual Organic Compounds from Marine Sediments, Science, 277, 796–799, https://doi.org/10.1126/science.277.5327.796, 1997.
Eglinton, T. I., Conte, M. H., Eglinton, G., and Hayes, J. M.: Proceedings of a workshop on alkenone-based paleoceanographic indicators, Geochem. Geophy. Geosy., 2, 2000GC000122, https://doi.org/10.1029/2000GC000122, 2001.
Eglinton, T. I., Galy, V. V., Hemingway, J. D., Feng, X., Bao, H., Blattmann, T. M., Dickens, A. F., Gies, H., Giosan, L., Haghipour, N., Hou, P., Lupker, M., McIntyre, C. P., Montluçon, D. B., Peucker-Ehrenbrink, B., Ponton, C., Schefuß, E., Schwab, M. S., Voss, B. M., Wacker, L., Wu, Y., and Zhao, M.: Climate control on terrestrial biospheric carbon turnover, P. Natl. Acad. Sci. USA, 118, e2011585118, https://doi.org/10.1073/pnas.2011585118, 2021.
Fagel, N.: Chapter Four Clay Minerals, Deep Circulation and Climate, Developments in Marine Geology, 1, 139–184, https://doi.org/10.1016/S1572-5480(07)01009-3, 2007.
Farquhar, G. D., Ehleringer, J. R., and Hubick, K. T.: Carbon Isotope Discrimination and Photosynthesis, Annu. Rev. Plant Phys., 40, 503–537, https://doi.org/10.1146/annurev.pp.40.060189.002443, 1989.
Feng, X., Benitez-Nelson, B. C., Montluçon, D. B., Prahl, F. G., McNichol, A. P., Xu, L., Repeta, D. J., and Eglinton, T. I.: 14C and 13C characteristics of higher plant biomarkers in Washington margin surface sediments, Geochim. Cosmochim. Ac., 105, 14–30, https://doi.org/10.1016/j.gca.2012.11.034, 2013.
Flanders Marine Institute: IHO Sea Areas, version 3, https://doi.org/10.14284/323, 2018.
Flanders Marine Institute: Maritime Boundaries Geodatabase: Maritime Boundaries and Exclusive Economic Zones (200NM), version 11, https://doi.org/10.14284/386, 2019.
French, K. L., Hein, C. J., Haghipour, N., Wacker, L., Kudrass, H. R., Eglinton, T. I., and Galy, V.: Millennial soil retention of terrestrial organic matter deposited in the Bengal Fan, Sci. Rep.-UK, 8, 11997, https://doi.org/10.1038/s41598-018-30091-8, 2018.
Galy, V., France-Lanord, C., Beyssac, O., Faure, P., Kudrass, H., and Palhol, F.: Efficient organic carbon burial in the Bengal fan sustained by the Himalayan erosional system, Nature, 450, 407–410, https://doi.org/10.1038/nature06273, 2007.
GEBCO Compilation Group: GEBCO 2022 Grid, https://doi.org/10.5285/e0f0bb80-ab44-2739-e053-6c86abc0289c, 2022.
Gibbs, M., Leduc, D., Nodder, S. D., Kingston, A., Swales, A., Rowden, A. A., Mountjoy, J., Olsen, G., Ovenden, R., Brown, J., Bury, S., and Graham, B.: Novel Application of a Compound-Specific Stable Isotope (CSSI) Tracking Technique Demonstrates Connectivity Between Terrestrial and Deep-Sea Ecosystems via Submarine Canyons, Front. Mar. Sci., 7, 608, https://doi.org/10.3389/fmars.2020.00608, 2020.
Goñi, M. A. and Hedges, J. I.: Cutin-derived CuO reaction products from purified cuticles and tree leaves, Geochim. Cosmochim. Ac., 54, 3065–3072, https://doi.org/10.1016/0016-7037(90)90122-2, 1990.
Goñi, M. A., Ruttenberg, K. C., and Eglinton, T. I.: A reassessment of the sources and importance of land-derived organic matter in surface sediments from the Gulf of Mexico, Geochim. Cosmochim. Ac., 62, 3055–3075, https://doi.org/10.1016/S0016-7037(98)00217-8, 1998.
Goñi, M. A., Yunker, M. B., Macdonald, R. W., and Eglinton, T. I.: Distribution and sources of organic biomarkers in arctic sediments from the Mackenzie River and Beaufort Shelf, Mar. Chem., 71, 23–51, https://doi.org/10.1016/S0304-4203(00)00037-2, 2000.
Goñi, M. A., Gordon, E. S., Monacci, N. M., Clinton, R., Gisewhite, R., Allison, M. A., and Kineke, G.: The effect of Hurricane Lili on the distribution of organic matter along the inner Louisiana shelf (Gulf of Mexico, USA), Cont. Shelf Res., 26, 2260–2280, https://doi.org/10.1016/j.csr.2006.07.017, 2006.
Gordon, E. S. and Goñi, M. A.: Sources and distribution of terrigenous organic matter delivered by the Atchafalaya River to sediments in the northern Gulf of Mexico, Geochim. Cosmochim. Ac., 67, 2359–2375, https://doi.org/10.1016/S0016-7037(02)01412-6, 2003.
Gordon, E. S. and Goñi, M. A.: Controls on the distribution and accumulation of terrigenous organic matter in sediments from the Mississippi and Atchafalaya river margin, Mar. Chem., 92, 331–352, https://doi.org/10.1016/J.MARCHEM.2004.06.035, 2004.
Guo, J., Yuan, H., Song, J., Li, X., Duan, L., Li, N., and Wang, Y.: Evaluation of Sedimentary Organic Carbon Reactivity and Burial in the Eastern China Marginal Seas, J. Geophys. Res.-Oceans, 126, e2021JC017207, https://doi.org/10.1029/2021JC017207, 2021.
Gustafsson, Ö., van Dongen, B. E., Vonk, J. E., Dudarev, O. V., and Semiletov, I. P.: Widespread release of old carbon across the Siberian Arctic echoed by its large rivers, Biogeosciences, 8, 1737–1743, https://doi.org/10.5194/bg-8-1737-2011, 2011.
Hackeloeer, A., Klasing, K., Krisp, J. M., and Meng, L.: Georeferencing: a review of methods and applications, Ann. GIS, 20, 61–69, https://doi.org/10.1080/19475683.2013.868826, 2014.
Hahn, A., Schefuß, E., Andò, S., Cawthra, H. C., Frenzel, P., Kugel, M., Meschner, S., Mollenhauer, G., and Zabel, M.: Southern Hemisphere anticyclonic circulation drives oceanic and climatic conditions in late Holocene southernmost Africa, Clim. Past, 13, 649–665, https://doi.org/10.5194/cp-13-649-2017, 2017.
Halpern, B. S., Walbridge, S., Selkoe, K. A., Kappel, C. V., Micheli, F., D'Agrosa, C., Bruno, J. F., Casey, K. S., Ebert, C., Fox, H. E., Fujita, R., Heinemann, D., Lenihan, H. S., Madin, E. M. P, Perry, M. T., Selig, E. R., Spalding, M., Steneck, R., and Watson, R.: A global map of human impact on marine ecosystems, Science, 319, 948–952, https://doi.org/10.1126/science.1149345, 2008.
Hastings, R. H., Goñi, M. A., Wheatcroft, R. A., and Borgeld, J. C.: A terrestrial organic matter depocenter on a high-energy margin: The Umpqua River system, Oregon, Cont. Shelf Res., 39–40, 78–91, https://doi.org/10.1016/j.csr.2012.04.002, 2012.
Hedges, J. I. and Ertel, J. R.: Characterization of lignin by gas capillary chromatography of cupric oxide oxidation products, Anal. Chem., 54, 174–178, https://doi.org/10.1021/ac00239a007, 1982.
Hedges, J. I. and Keil, R. G.: Sedimentary organic matter preservation: an assessment and speculative synthesis, Mar. Chem., 49, 81–115, https://doi.org/10.1016/0304-4203(95)00008-F, 1995.
Hedges, J. I. and Mann, D. C.: The characterization of plant tissues by their lignin oxidation products, Geochim. Cosmochim. Ac., 43, 1803–1807, https://doi.org/10.1016/0016-7037(79)90028-0, 1979.
Hemingway, J. D., Rothman, D. H., Grant, K. E., Rosengard, S. Z., Eglinton, T. I., Derry, L. A., and Galy, V. V.: Mineral protection regulates long-term global preservation of natural organic carbon, Nature, 570, 228–231, https://doi.org/10.1038/s41586-019-1280-6, 2019.
Hilton, R. G., Galy, A., Hovius, N., Horng, M.-J., and Chen, H.: The isotopic composition of particulate organic carbon in mountain rivers of Taiwan, Geochim. Cosmochim. Ac., 74, 3164–3181, https://doi.org/10.1016/j.gca.2010.03.004, 2010.
Hoogsteen, M. J. J., Lantinga, E. A., Bakker, E. J., and Tittonell, P. A.: An Evaluation of the Loss-on-Ignition Method for Determining the Soil Organic Matter Content of Calcareous Soils, Commun. Soil Sci. Plan., 49, 1541–1552, https://doi.org/10.1080/00103624.2018.1474475, 2018.
Hou, P., Yu, M., Zhao, M., Montluçon, D. B., Su, C., and Eglinton, T. I.: Terrestrial Biomolecular Burial Efficiencies on Continental Margins, J. Geophys. Res.-Biogeo., 125, e2019JG005520, https://doi.org/10.1029/2019JG005520, 2020.
Hou, P., Eglinton, T. I., Yu, M., Montluçon, D. B., Haghipour, N., Zhang, H., Jin, G., and Zhao, M.: Degradation and Aging of Terrestrial Organic Carbon within Estuaries: Biogeochemical and Environmental Implications, Environ. Sci. Technol., 55, 10852–10861, https://doi.org/10.1021/acs.est.1c02742, 2021.
Hu, B., Li, J., Zhao, J., Wei, H., Yin, X., Li, G., Liu, Y., Sun, Z., Zou, L., Bai, F., Dou, Y., Wang, L., and Sun, R.: Late Holocene elemental and isotopic carbon and nitrogen records from the East China Sea inner shelf: Implications for monsoon and upwelling, Mar. Chem., 162, 60–70, https://doi.org/10.1016/j.marchem.2014.03.008, 2014.
Hu, L., Shi, X., Guo, Z., Wang, H., and Yang, Z.: Sources, dispersal and preservation of sedimentary organic matter in the Yellow Sea: The importance of depositional hydrodynamic forcing, Mar. Geol., 335, 52–63, https://doi.org/10.1016/j.margeo.2012.10.008, 2013.
Huang, Y., Dupont, L., Sarnthein, M., Hayes, J. M., and Eglinton, G.: Mapping of C4 plant input from North West Africa into North East Atlantic sediments, Geochim. Cosmochim. Ac., 64, 3505–3513, https://doi.org/10.1016/S0016-7037(00)00445-2, 2000.
Jeandel, C., Arsouze, T., Lacan, F., Téchiné, P., and Dutay, J.-C.: Isotopic Nd compositions and concentrations of the lithogenic inputs into the ocean: A compilation, with an emphasis on the margins, Chem. Geol., 239, 156–164, https://doi.org/10.1016/j.chemgeo.2006.11.013, 2007.
Kao, S.-J., Hilton, R. G., Selvaraj, K., Dai, M., Zehetner, F., Huang, J.-C., Hsu, S.-C., Sparkes, R., Liu, J. T., Lee, T.-Y., Yang, J.-Y. T., Galy, A., Xu, X., and Hovius, N.: Preservation of terrestrial organic carbon in marine sediments offshore Taiwan: mountain building and atmospheric carbon dioxide sequestration, Earth Surf. Dynam., 2, 127–139, https://doi.org/10.5194/esurf-2-127-2014, 2014.
Ke, Y., Calmels, D., Bouchez, J., and Quantin, C.: MOdern River archivEs of Particulate Organic Carbon: MOREPOC, Earth Syst. Sci. Data, 14, 4743–4755, https://doi.org/10.5194/essd-14-4743-2022, 2022.
Keil, R.: Anthropogenic Forcing of Carbonate and Organic Carbon Preservation in Marine Sediments, Ann. Rev. Mar. Sci., 9, 151–172, https://doi.org/10.1146/annurev-marine-010816-060724, 2017.
Keil, R. G., Tsamakis, E., Giddings, J. C., and Hedges, J. I.: Biochemical distributions (amino acids, neutral sugars, and lignin phenols) among size-classes of modern marine sediments from the Washington coast, Geochim. Cosmochim. Ac., 62, 1347–1364, https://doi.org/10.1016/S0016-7037(98)00080-5, 1998.
Khan, A. A., Haredy, R., and Inam, A.: Geochemistry and Sedimentary Sources of the Surface Sediments from the Continental Shelf off the Indus Delta, Pakistan, Thalassas, 36, 61–74, https://doi.org/10.1007/s41208-019-00168-w, 2020.
Kiriakoulakis, K., Blackbird, S., Ingels, J., Vanreusel, A., and Wolff, G. A.: Organic geochemistry of submarine canyons: The Portuguese Margin, Deep-Sea Res. Pt. II, 58, 2477–2488, https://doi.org/10.1016/j.dsr2.2011.04.010, 2011.
Koga, Y., Nishihara, M., Morii, H., and Akagawa-Matsushita, M.: Ether polar lipids of methanogenic bacteria: structures, comparative aspects, and biosyntheses, Microbiol. Rev., 57, 164–182, https://doi.org/10.1128/mr.57.1.164-182.1993, 1993.
Kusch, S., Rethemeyer, J., Schefuß, E., and Mollenhauer, G.: Controls on the age of vascular plant biomarkers in Black Sea sediments, Geochim. Cosmochim. Ac., 74, 7031–7047, https://doi.org/10.1016/j.gca.2010.09.005, 2010.
Kusch, S., Mollenhauer, G., Willmes, C., Hefter, J., Eglinton, T. I., and Galy, V.: Controls on the age of plant waxes in marine sediments – A global synthesis, Org. Geochem., 157, 104259, https://doi.org/10.1016/j.orggeochem.2021.104259, 2021.
Laruelle, G. G., Dürr, H. H., Lauerwald, R., Hartmann, J., Slomp, C. P., Goossens, N., and Regnier, P. A. G.: Global multi-scale segmentation of continental and coastal waters from the watersheds to the continental margins, Hydrol. Earth Syst. Sci., 17, 2029–2051, https://doi.org/10.5194/hess-17-2029-2013, 2013.
Lattaud, J., De Jonge, C., Pearson, A., Elling, F. J., and Eglinton, T. I.: Microbial lipid signatures in Arctic deltaic sediments – Insights into methane cycling and climate variability, Org. Geochem., 157, 104242, https://doi.org/10.1016/j.orggeochem.2021.104242, 2021.
Lattaud, J., Eglinton, T. I., Tallon, M., Bröder, L., Erdem, Z., and Ausín, B.: Grain size controls on long-chain diol distributions and proxy signals in marine sediments, Front. Mar. Sci., 9, 1004096, https://doi.org/10.3389/fmars.2022.1004096, 2022.
Lee, T. R., Wood, W. T., and Phrampus, B. J.: A Machine Learning (kNN) Approach to Predicting Global Seafloor Total Organic Carbon, Global Biogeochem. Cy., 33, 37–46, https://doi.org/10.1029/2018GB005992, 2019.
Lee, T. R., Phrampus, B. J., and Obelcz, J.: The necessary optimization of the data lifecycle: Marine geosciences in the big data era, Front. Earth Sci., 10, 1089112, https://doi.org/10.3389/feart.2022.1089112, 2023.
Li, Q., Qiao, S., Shi, X., Chen, Y., Astakhov, A., Zhang, H., Hu, L., Yang, G., Bosin, A., Vasilenko, Y., and Dong, L.: Sr, Nd, and Pb isotope provenance of surface sediments on the East Siberian Arctic Shelf and implications for transport pathways, Chem. Geol., 618, 121277, https://doi.org/10.1016/j.chemgeo.2022.121277, 2023.
Liu, Z., Colin, C., Li, X., Zhao, Y., Tuo, S., Chen, Z., Siringan, F. P., Liu, J. T., Huang, C.-Y., You, C.-F., and Huang, K.-F.: Clay mineral distribution in surface sediments of the northeastern South China Sea and surrounding fluvial drainage basins: Source and transport, Mar. Geol., 277, 48–60, https://doi.org/10.1016/j.margeo.2010.08.010, 2010.
Longhurst, A., Sathyendranath, S., Platt, T., and Caverhill, C.: An estimate of global primary production in the ocean from satellite radiometer data, J. Plankton Res., 17, 1245–1271, https://doi.org/10.1093/plankt/17.6.1245, 1995.
Luisetti, T., Ferrini, S., Grilli, G., Jickells, T. D., Kennedy, H., Kröger, S., Lorenzoni, I., Milligan, B., van der Molen, J., Parker, R., Pryce, T., Turner, R. K., and Tyllianakis, E.: Climate action requires new accounting guidance and governance frameworks to manage carbon in shelf seas, Nat. Commun., 11, 4599, https://doi.org/10.1038/s41467-020-18242-w, 2020.
Marlowe, I. T., Brassell, S. C., Eglinton, G., and Green, J. C.: Long chain unsaturated ketones and esters in living algae and marine sediments, Org. Geochem., 6, 135–141, https://doi.org/10.1016/0146-6380(84)90034-2, 1984.
Masson, D. G., Huvenne, V. A. I., de Stigter, H. C., Wolff, G. A., Kiriakoulakis, K., Arzola, R. G., and Blackbird, S.: Efficient burial of carbon in a submarine canyon, Geology, 38, 831–834, https://doi.org/10.1130/G30895.1, 2010.
Mayer, L. M.: Surface area control of organic carbon accumulation in continental shelf sediments, Geochim. Cosmochim. Ac., 58, 1271–1284, https://doi.org/10.1016/0016-7037(94)90381-6, 1994.
Mead, R. and Goñi, M. A.: A lipid molecular marker assessment of sediments from the Northern Gulf of Mexico before and after the passage of Hurricane Lili, Org. Geochem., 37, 1115–1129, https://doi.org/10.1016/j.orggeochem.2006.04.010, 2006.
Meyer, H. and Pebesma, E.: Machine learning-based global maps of ecological variables and the challenge of assessing them, Nat. Commun., 13, 2208, https://doi.org/10.1038/s41467-022-29838-9, 2022.
Mollenhauer, G. and Eglinton, T. I.: Diagenetic and sedimentological controls on the composition of organic matter preserved in California Borderland Basin sediments, Limnol. Oceanogr., 52, 558–576, https://doi.org/10.4319/lo.2007.52.2.0558, 2007.
Mollenhauer, G., Schneider, R. R., Jennerjahn, T., Müller, P. J., and Wefer, G.: Organic carbon accumulation in the South Atlantic Ocean: its modern, mid-Holocene and last glacial distribution, Global Planet. Change, 40, 249–266, https://doi.org/10.1016/j.gloplacha.2003.08.002, 2004.
Morrill, C., Thrasher, B., Lockshin, S. N., Gille, E. P., McNeill, S., Shepherd, E., Gross, W. S., and Bauer, B. A.: The Paleoenvironmental Standard Terms (PaST) Thesaurus: Standardizing Heterogeneous Variables in Paleoscience, Paleoceanogr. Paleoclimatology, 36, e2020PA004193, https://doi.org/10.1029/2020PA004193, 2021.
Oliver, M. A. and Webster, R.: Kriging: a method of interpolation for geographical information systems, Int. J. Geogr. Inf. Syst., 4, 313–332, https://doi.org/10.1080/02693799008941549, 1990.
Palanques, A., Paradis, S., Puig, P., Masqué, P., and Lo Iacono, C.: Effects of bottom trawling on trace metal contamination of sediments along the submarine canyons of the Gulf of Palermo (southwestern Mediterranean), Sci. Total Environ., 814, 152658, https://doi.org/10.1016/j.scitotenv.2021.152658, 2022.
Paradis, S.: sarah-paradis/MOSAIC: Modern Ocean Sediment Archive and Inventory of Carbon (v2.0), Zenodo [data set], https://doi.org/10.5281/zenodo.8322094, 2023.
Paradis, S., Pusceddu, A., Masqué, P., Puig, P., Moccia, D., Russo, T., and Lo Iacono, C.: Organic matter contents and degradation in a highly trawled area during fresh particle inputs (Gulf of Castellammare, southwestern Mediterranean), Biogeosciences, 16, 4307–4320, https://doi.org/10.5194/bg-16-4307-2019, 2019.
Paradis, S., Goñi, M., Masqué, P., Durán, R., Arjona-Camas, M., Palanques, A., and Puig, P.: Persistence of Biogeochemical Alterations of Deep-Sea Sediments by Bottom Trawling, Geophys. Res. Lett., 48, e2020GL091279, https://doi.org/10.1029/2020GL091279, 2021a.
Paradis, S., Lo Iacono, C., Masqué, P., Puig, P., Palanques, A., and Russo, T.: Evidence of large increases in sedimentation rates due to fish trawling in submarine canyons of the Gulf of Palermo (SW Mediterranean), Mar. Pollut. Bull., 172, 112861, https://doi.org/10.1016/j.marpolbul.2021.112861, 2021b.
Pasqual, C., Goñi, M. A., Tesi, T., Sanchez-Vidal, A., Calafat, A., and Canals, M.: Composition and provenance of terrigenous organic matter transported along submarine canyons in the Gulf of Lion (NW Mediterranean Sea), Prog. Oceanogr., 118, 81–94, https://doi.org/10.1016/j.pocean.2013.07.013, 2013.
Pedersen, T. F., Shimmield, G. B., and N. B.: Lack of enhanced preservation of organic matter in sediments under the oxygen minimum on the Oman Margin, Geochim. Cosmochim. Ac., 56, 545–551, https://doi.org/10.1016/0016-7037(92)90152-9, 1992.
Prahl, F. G., Ertel, J. R., Goñi, M. A., Sparrow, M. A., and Eversmeyer, B.: Terrestrial organic carbon contributions to sediments on the Washington margin, Geochim. Cosmochim. Ac., 58, 3035–3048, https://doi.org/10.1016/0016-7037(94)90177-5, 1994.
Premuzic, E. T., Benkovitz, C. M., Gaffney, J. S., and Walsh, J. J.: The nature and distribution of organic matter in the surface sediments of world oceans and seas, Org. Geochem., 4, 63–77, https://doi.org/10.1016/0146-6380(82)90009-2, 1982.
Pusceddu, A., Dell'Anno, A., Fabiano, M., and Danovaro, R.: Quantity and bioavailability of sediment organic matter as signatures of benthic trophic status, Mar. Ecol. Prog. Ser., 375, 41–52, https://doi.org/10.3354/meps07735, 2009.
Raja, M. and Rosell-Melé, A.: Appraisal of sedimentary alkenones for the quantitative reconstruction of phytoplankton biomass, P. Natl. Acad. Sci. USA, 118, e2014787118, https://doi.org/10.1073/pnas.2014787118, 2021.
Raja, M. and Rosell-Melé, A.: Quantitative Link Between Sedimentary Chlorin and Sea-Surface Chlorophyll-a, J. Geophys. Res.-Biogeo., 127, e2021JG006514, https://doi.org/10.1029/2021JG006514, 2022.
Romankevich, E. A.: Geochemistry of Organic Matter in the Ocean, Springer Berlin Heidelberg, Berlin, Heidelberg, https://doi.org/10.1007/978-3-642-49964-7, 1984.
Sachse, D., Billault, I., Bowen, G. J., Chikaraishi, Y., Dawson, T. E., Feakins, S. J., Freeman, K. H., Magill, C. R., McInerney, F. A., van der Meer, M. T. J., Polissar, P., Robins, R. J., Sachs, J. P., Schmidt, H.-L., Sessions, A. L., White, J. W. C., West, J. B., and Kahmen, A.: Molecular Paleohydrology: Interpreting the Hydrogen-Isotopic Composition of Lipid Biomarkers from Photosynthesizing Organisms, Annu. Rev. Earth Pl. Sc., 40, 221–249, https://doi.org/10.1146/annurev-earth-042711-105535, 2012.
Schubert, C. J. and Nielsen, B.: Effects of decarbonation treatments on δ13C values in marine sediments, Mar. Chem., 72, 55–59, https://doi.org/10.1016/S0304-4203(00)00066-9, 2000.
Schwab, M. S., Rickli, J. D., Macdonald, R. W., Harvey, H. R., Haghipour, N., and Eglinton, T. I.: Detrital neodymium and (radio)carbon as complementary sedimentary bedfellows? The Western Arctic Ocean as a testbed, Geochim. Cosmochim. Ac., 315, 101–126, https://doi.org/10.1016/j.gca.2021.08.019, 2021.
Seiter, K., Hensen, C., Schröter, J., and Zabel, M.: Organic carbon content in surface sediments – defining regional provinces, Deep-Sea Res. Pt. I, 51, 2001–2026, https://doi.org/10.1016/j.dsr.2004.06.014, 2004.
Smeaton, C., Hunt, C. A., Turrell, W. R., and Austin, W. E. N.: Marine Sedimentary Carbon Stocks of the United Kingdom's Exclusive Economic Zone, Front. Earth Sci., 9, 593324, https://doi.org/10.3389/feart.2021.593324, 2021.
Stuiver, M. and Polach, H. A.: Discussion Reporting of 14C Data, Radiocarbon, 19, 355–363, https://doi.org/10.1017/S0033822200003672, 1977.
Tao, S., Eglinton, T. I., Montluçon, D. B., McIntyre, C., and Zhao, M.: Diverse origins and pre-depositional histories of organic matter in contemporary Chinese marginal sea sediments, Geochim. Cosmochim. Ac., 191, 70–88, https://doi.org/10.1016/j.gca.2016.07.019, 2016.
Tao, S., Liu, J. T., Wang, A., Blattmann, T. M., Yang, R. J., Lee, J., Xu, J. J., Li, L., Ye, X., Yin, X., and Wang, L.: Deciphering organic matter distribution by source-specific biomarkers in the shallow Taiwan Strait from a source-to-sink perspective, Front. Mar. Sci., 9, 969461, https://doi.org/10.3389/fmars.2022.969461, 2022.
Tesi, T., Miserocchi, S., Goñi, M. A., Langone, L., Boldrin, A., and Turchetto, M.: Organic matter origin and distribution in suspended particulate materials and surficial sediments from the western Adriatic Sea (Italy), Estuar. Coast. Shelf S., 73, 431–446, https://doi.org/10.1016/j.ecss.2007.02.008, 2007.
Tesi, T., Langone, L., Goñi, M. A., Wheatcroft, R. A., Miserocchi, S., and Bertotti, L.: Early diagenesis of recently deposited organic matter: A 9-yr time-series study of a flood deposit, Geochim. Cosmochim. Ac., 83, 19–36, https://doi.org/10.1016/j.gca.2011.12.026, 2012.
Thevenot, M., Dignac, M.-F., and Rumpel, C.: Fate of lignins in soils: A review, Soil Biol. Biochem., 42, 1200–1211, https://doi.org/10.1016/j.soilbio.2010.03.017, 2010.
Tierney, J. E. and Tingley, M. P.: A Bayesian, spatially-varying calibration model for the TEX86 proxy, Geochim. Cosmochim. Ac., 127, 83–106, https://doi.org/10.1016/j.gca.2013.11.026, 2014.
Tierney, J. E. and Tingley, M. P.: A TEX86 surface sediment database and extended Bayesian calibration, Sci. Data, 2, 150029, https://doi.org/10.1038/sdata.2015.29, 2015.
Tierney, J. E. and Tingley, M. P.: BAYSPLINE: A New Calibration for the Alkenone Paleothermometer, Paleoceanogr. Paleoclimatology, 33, 281–301, https://doi.org/10.1002/2017PA003201, 2018.
Van der Voort, T. S., Mannu, U., Blattmann, T. M., Bao, R., Zhao, M., and Eglinton, T. I.: Deconvolving the Fate of Carbon in Coastal Sediments, Geophys. Res. Lett., 45, 4134–4142, https://doi.org/10.1029/2018GL077009, 2018.
Van der Voort, T. S., Loeffler, T. J., Montlucon, D., Blattmann, T. M., and Eglinton, T.: MOSAIC – database of Modern Ocean Sediment Archive and Inventory of Carbon, ETH Zürich [data set], https://doi.org/10.5168/mosaic019.1, 2019.
Van der Voort, T. S., Blattmann, T. M., Usman, M., Montluçon, D., Loeffler, T., Tavagna, M. L., Gruber, N., and Eglinton, T. I.: MOSAIC (Modern Ocean Sediment Archive and Inventory of Carbon): a (radio)carbon-centric database for seafloor surficial sediments, Earth Syst. Sci. Data, 13, 2135–2146, https://doi.org/10.5194/essd-13-2135-2021, 2021.
Verwega, M.-T., Somes, C. J., Schartau, M., Tuerena, R. E., Lorrain, A., Oschlies, A., and Slawig, T.: Description of a global marine particulate organic carbon-13 isotope data set, Earth Syst. Sci. Data, 13, 4861–4880, https://doi.org/10.5194/essd-13-4861-2021, 2021.
Volkman, J., Eglinton, G., Corner, E., and Sargent, J.: Novel unsaturated straight-chain C37–C39 methyl and ethyl ketones in marine sediments and a coccolithophore Emiliania huxleyi, in: Advances in Organic Geochemistry 1979, edited by: Douglas, A. and Maxwell, J., Pergamon, Oxford, 219–227, https://doi.org/10.1016/0079-1946(79)90106-X, 1980.
Vonk, J. E., Sánchez-García, L., van Dongen, B. E., Alling, V., Kosmach, D., Charkin, A., Semiletov, I. P., Dudarev, O. V., Shakhova, N., Roos, P., Eglinton, T. I., Andersson, A., and Gustafsson, Ö.: Activation of old carbon by erosion of coastal and subsea permafrost in Arctic Siberia, Nature, 489, 137–140, https://doi.org/10.1038/nature11392, 2012.
Wakeham, S. G. and McNichol, A. P.: Transfer of organic carbon through marine water columns to sediments – insights from stable and radiocarbon isotopes of lipid biomarkers, Biogeosciences, 11, 6895–6914, https://doi.org/10.5194/bg-11-6895-2014, 2014.
Wakeham, S. G., Canuel, E. A., Lerberg, E. J., Mason, P., Sampere, T. P., and Bianchi, T. S.: Partitioning of organic matter in continental margin sediments among density fractions, Mar. Chem., 115, 211–225, https://doi.org/10.1016/j.marchem.2009.08.005, 2009.
Walinsky, S. E., Prahl, F. G., Mix, A. C., Finney, B. P., Jaeger, J. M., and Rosen, G. P.: Distribution and composition of organic matter in surface sediments of coastal Southeast Alaska, Cont. Shelf Res., 29, 1565–1579, https://doi.org/10.1016/j.csr.2009.04.006, 2009.
Wang, J., Yao, P., Bianchi, T. S., Li, D., Zhao, B., Cui, X., Pan, H., Zhang, T., and Yu, Z.: The effect of particle density on the sources, distribution, and degradation of sedimentary organic carbon in the Changjiang Estuary and adjacent shelf, Chem. Geol., 402, 52–67, https://doi.org/10.1016/j.chemgeo.2015.02.040, 2015.
Wessel, P. and Smith, W. H. F.: A global, self-consistent, hierarchical, high-resolution shoreline database, J. Geophys. Res.-Sol. Ea., 101, 8741–8743, https://doi.org/10.1029/96JB00104, 1996.
Wilkinson, M. D., Dumontier, M., Aalbersberg, I. J., Appleton, G., Axton, M., Baak, A., Blomberg, N., Boiten, J.-W., da Silva Santos, L. B., Bourne, P. E., Bouwman, J., Brookes, A. J., Clark, T., Crosas, M., Dillo, I., Dumon, O., Edmunds, S., Evelo, C. T., Finkers, R., Gonzalez-Beltran, A., Gray, A. J. G., Groth, P., Goble, C., Grethe, J. S., Heringa, J., 't Hoen, P. A. ., Hooft, R., Kuhn, T., Kok, R., Kok, J., Lusher, S. J., Martone, M. E., Mons, A., Packer, A. L., Persson, B., Rocca-Serra, P., Roos, M., van Schaik, R., Sansone, S.-A., Schultes, E., Sengstag, T., Slater, T., Strawn, G., Swertz, M. A., Thompson, M., van der Lei, J., van Mulligen, E., Velterop, J., Waagmeester, A., Wittenburg, P., Wolstencroft, K., Zhao, J., and Mons, B.: The FAIR Guiding Principles for scientific data management and stewardship, Sci. Data, 3, 160018, https://doi.org/10.1038/sdata.2016.18, 2016.
Yu, M., Eglinton, T. I., Haghipour, N., Montluçon, D. B., Wacker, L., Hou, P., Ding, Y., and Zhao, M.: Contrasting fates of terrestrial organic carbon pools in marginal sea sediments, Geochim. Cosmochim. Ac., 309, 16–30, https://doi.org/10.1016/j.gca.2021.06.018, 2021.
Yu, M., Eglinton, T. I., Haghipour, N., Dubois, N., Wacker, L., Zhang, H., Jin, G., and Zhao, M.: Persistently high efficiencies of terrestrial organic carbon burial in Chinese marginal sea sediments over the last 200 years, Chem. Geol., 606, 120999, https://doi.org/10.1016/j.chemgeo.2022.120999, 2022.
Zuo, Z., Eisma, D., and Berger, G. W.: Determination of sediment accumulation and mixing rates in the Gulf of Lions, Mediterranean Sea, Oceanol. Acta, 14, 253–262, 1991.