the Creative Commons Attribution 4.0 License.
the Creative Commons Attribution 4.0 License.
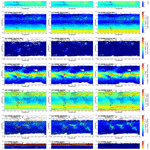
Global Ozone Monitoring Experiment-2 (GOME-2) daily and monthly level-3 products of atmospheric trace gas columns
Ka Lok Chan
Pieter Valks
Klaus-Peter Heue
Ronny Lutz
Pascal Hedelt
Diego Loyola
Gaia Pinardi
Michel Van Roozendael
François Hendrick
Thomas Wagner
Vinod Kumar
Alkis Bais
Ankie Piters
Hitoshi Irie
Hisahiro Takashima
Yugo Kanaya
Yongjoo Choi
Kihong Park
Jihyo Chong
Alexander Cede
Udo Frieß
Andreas Richter
Jianzhong Ma
Nuria Benavent
Robert Holla
Oleg Postylyakov
Claudia Rivera Cárdenas
Mark Wenig
We introduce the new Global Ozone Monitoring Experiment-2 (GOME-2) daily and monthly level-3 product of total column ozone (O3), total and tropospheric column nitrogen dioxide (NO2), total column water vapour, total column bromine oxide (BrO), total column formaldehyde (HCHO), and total column sulfur dioxide (SO2) (daily products https://doi.org/10.15770/EUM_SAF_AC_0048, AC SAF, 2023a; monthly products https://doi.org/10.15770/EUM_SAF_AC_0049, AC SAF, 2023b). The GOME-2 level-3 products aim to provide easily translatable and user-friendly data sets to the scientific community for scientific progress as well as to satisfy public interest. The purpose of this paper is to present the theoretical basis as well as the verification and validation of the GOME-2 daily and monthly level-3 products.
The GOME-2 level-3 products are produced using the overlapping area-weighting method. Details of the gridding algorithm are presented. The spatial resolution of the GOME-2 level-3 products is selected based on the sensitivity study. The consistency of the resulting level-3 products among three GOME-2 sensors is investigated through time series of global averages, zonal averages, and bias. The accuracy of the products is validated by comparison to ground-based observations. The verification and validation results show that the GOME-2 level-3 products are consistent with the level-2 data. Small discrepancies are found among three GOME-2 sensors, which are mainly caused by the differences in the instrument characteristic and level-2 processor. The comparison of GOME-2 level-3 products to ground-based observations in general shows very good agreement, indicating that the products are consistent and fulfil the requirements to serve the scientific community and general public.
- Article
(29250 KB) - Full-text XML
- BibTeX
- EndNote
Satellite remote-sensing observations provide indispensable spatiotemporal information on atmospheric composition at a global scale. Various atmospheric trace gases can be retrieved from nadir-viewing satellite spectroscopic observations in the ultraviolet (UV) and visible (VIS) spectral ranges. This type of observation has long been conducted since the Global Ozone Monitoring Experiment (GOME) mission launched in 1995 (Burrows et al., 1999). Together with other follow-up satellite-borne spectrometers, for example the SCanning Imaging Absorption SpectroMeter for Atmospheric CHartographY (SCIAMACHY; Bovensmann et al., 1999), Global Ozone Monitoring Experiment-2 (GOME-2; Callies et al., 2000), Ozone Monitoring Instrument (OMI; Levelt et al., 2006), and TROPOspheric Monitoring Instrument (TROPOMI; Veefkind et al., 2012), these observations provide a global record of earthshine radiance in the UV, VIS, and near-infrared (NIR) (UVN) spectral ranges for more than 25 years. The processing of satellite observation of the trace gas column involves two major steps, (1) conversion of raw spectral channel counts (level-0 data) to geolocation and radiometric calibrated radiance and irradiance data (level-1B data) and (2) the retrieval of trace gas columns (level-2 data) from level-1B data, which include spectral retrieval of trace gas slant columns and subsequently convert them to vertical columns. To ensure the accuracy and consistency of satellite observations, GOME-2 data are processed in stable operational environments within the framework of Satellite Application Facility on Atmospheric Composition Monitoring (AC SAF). The level-0 to level-1B conversion is processed by the European Organisation for the Exploitation of Meteorological Satellites (EUMETSAT), while the level-1B to level-2 conversion is processed by the German Aerospace Center (DLR).
The GOME-2 level-2 data are orbital-swath scientific products. A level-2 data file contains observations within a single orbit. Trace gas columns are expressed in the satellite-viewing geometry of reference using across-track and along-track positions. In addition, the satellite measurement footprint is not in a regular latitude–longitude grid, and often multiple pixels overlap at the edges of an orbit within a day. Using this kind of scientific product requires very good knowledge of the satellite product, especially when averaging multiple measurements to generate daily or monthly maps. In order to provide a user-friendly satellite product, we have projected the GOME-2 level-2 data onto a regular latitude–longitude grid to generate operational level-3 products. The purpose of this document is to present the theoretical basis, verification, and validation of the GOME-2 level-3 daily and monthly gridded products. These products include global daily and monthly means of total column ozone (O3), total and tropospheric column nitrogen dioxide (NO2), total column bromine oxide (BrO), total column water vapour (H2O), total column formaldehyde (HCHO), and total column sulfur dioxide (SO2). Compared to satellite observations from OMI and TROPOMI, which measure at noon or in the early afternoon, GOME-2 measurements in the morning provide addition information on the temporal/diurnal variation of these atmospheric trace gases.
The paper is organized as follows. Section 2.1 describes the GOME-2 instruments. A brief description of each GOME-2 level-2 trace gas product can be found in Sect. 2.2, while auxiliary data sets used for comparison are presented in Sect. 2.3. The gridding algorithm for level-2 to level-3 processing is presented in Sect. 3.1. Section 3.2 shows the selection of the best spatial resolution for GOME-2 level-3 data. The verification and validation methodology are presented in Sect. 3.3. Section 4 presents the resulting daily and monthly level-3 products. Results for the verification and validation of GOME-2 level-3 data are presented in Sect. 5. Finally, Sect. 7 summarizes our findings.
The GOME-2 instruments and the operational level-2 products of each trace gas used for the generation of gridded level-3 data are described in this section. Ground-based measurements used to validate the GOME-2 level-3 products are also presented.
2.1 GOME-2 instruments
GOME-2 instruments are passive nadir-viewing satellite-borne spectrometers on board the European Organization for the EUMETSAT Metop series of satellites. The Metop satellites orbit at an altitude of ∼ 820 km on sun-synchronous orbits with a 29 d (412-orbit) repeat cycle and a local Equator overpass time of 09:30 LT (local time) on the descending node. Metop-A, the first Metop satellite, was launched on 19 October 2006. Metop-B was launched 6 years later on 17 September 2012. The third Metop satellite, Metop-C, was launched on 7 November 2018. A more detailed introduction of the Metop series of satellites can be found in Klaes et al. (2007).
The GOME-2 instruments are optical spectrometers equipped with scanning mirrors which enable across-track scanning in nadir and sideways viewing for polar coverage (Callies et al., 2000). Each GOME-2 instrument consists of four detectors covering a wavelength range of 240–790 nm with a spectral resolution ranging from 0.26 to 0.51 nm. Additionally, two polarization components are measured with polarization measurement devices (PMDs) using 30 broadband channels covering the full spectral range at higher spatial resolution. The nominal spatial resolution of the instruments is 80 km (across-track) × 40 km (along-track) for the forward scan, and the spatial resolution is reduced to 240 km (across-track) × 40 km (along-track) for the backward scan. The scanning swath width of the GOME-2 instruments is about 1920 km. After the GOME-2 instrument on board the Metop-B satellite (referred to as GOME-2B hereafter) went into tandem operation with Metop-A in July 2013, the across-track spatial resolution of the GOME-2 instrument on board the Metop-A satellite (referred to as GOME-2A hereafter) was doubled to 40 km, with the spatial coverage of a swath reduced to 960 km. The spatial resolution and coverage of GOME-2B remain unchanged. A more detailed description of the GOME-2 instruments can be found in Munro et al. (2016). Table 1 summarizes the major characteristics of all three GOME-2 instruments.
Table 1Summary of the GOME-2 instrument characteristics.
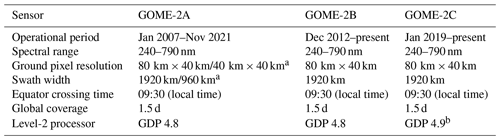
a Since tandem operation with GOME-2B on 15 July 2013. b GDP 4.9 was introduced for GOME-2C and includes updated instrument-specific retrieval settings for NO2 and SO2. For NO2, the alternative DOAS fitting window 430.2–465 nm is used (because of calibration issues for GOME-2C for wavelengths <430 nm). For SO2, improved DOAS fitting settings are used, and the wavelength region has been changed to 312–325 nm (Valks et al., 2019).
2.2 Operational GOME-2 level-2 data
2.2.1 Total column ozone
Ozone (O3) is an important trace gas in the earth’s atmosphere. In the stratosphere, ozone absorbs ultraviolet radiation from the sun, thus protecting the biosphere from harmful radiation (Eleftheratos et al., 2013; Hegglin et al., 2015). In the lower troposphere, natural ozone has an equally important beneficial role, because it initiates the chemical removal of air pollutants and greenhouse gases from the atmosphere, such as carbon monoxide (CO), nitrogen oxides (NOx), and methane (CH4). However, ozone at high concentration can also be harmful to humans, plants, and animals. In addition, ozone is a greenhouse gas that contributes to the warming of the earth’s atmosphere. In both the stratosphere and the troposphere, ozone absorbs infrared radiation emitted from the earth’s surface, trapping heat in the atmosphere. As a result, increases or decreases in stratospheric or tropospheric ozone induce a climate forcing (Hegglin et al., 2015).
The retrieval of total column ozone from GOME-2 (ir)radiance spectra is based on the typical two-step approach for weakly absorbing trace gas. The first step is to apply the differential optical absorption spectroscopy (DOAS) technique (Platt and Stutz, 2008) to the GOME-2 (ir)radiance spectra within the wavelength range of 325–335 nm to retrieve ozone slant column densities (SCDs). Ozone absorption features are prominent in this wavelength range. In addition, GOME-2 measurements have high signal-to-noise and manageable interference effects from other trace gases in this wavelength band. The DOAS fits include two ozone cross sections at 218 and 243 K. A NO2 cross section and the Ring spectrum are also included in the spectral fit.
The second step is the conversion of retrieved ozone slant column densities to vertical column densities (VCDs) using air mass factors (AMFs) (Solomon et al., 1987; Palmer et al., 2001). Vertical distribution profiles are essential a priori information used in the calculation of AMFs. The air mass factor calculation for ozone vertical column retrieval follows an iterative approach. The algorithm uses a standard ozone profile to retrieve an initial ozone vertical column. Based on the initial result of the ozone vertical column retrieval, the algorithm selects the most appropriate a priori profile from the climatology database and uses it a priori in the next iteration. The iterations end when the change in the retrieved vertical column is less than 0.1 % or when it reaches the maximum limit of iterations. For GOME-2 total column ozone retrieval, the number of iterations is in most cases smaller than 4. The radiative transfer model, LInearized Discrate Ordinate Radiative Transfer (LIDORT) (Spurr, 2008), is used for the radiative transfer calculation of AMFs with respect to the a priori ozone profile and cloud information. Cloud parameters are retrieved from GOME-2 measurements using the optical cloud recognition algorithm (OCRA) and retrieval of cloud information using neural network (ROCINN) algorithms (see Sect. 2.2.7), and the ozone absorption inside and below the cloud is treated by the intra-cloud correction term, which is a function of solar zenith angle and cloud albedo. AMFs are computed at a single wavelength of 325.5 nm (Van Roozendael et al., 2006). As the major part of ozone is in the stratosphere, which is well above clouds, all measurements (cloudy and clear-sky) are used in the level-3 product. More details on the GOME-2 total column ozone retrieval can be found in Loyola et al. (2011) and Hao et al. (2014).
2.2.2 Total and tropospheric column nitrogen dioxide
Nitrogen dioxide (NO2) plays an important role in atmospheric chemistry and air quality in the troposphere. It is an air pollutant affecting human health and ecosystems. Furthermore, NO2 in the troposphere is a major ozone precursor while being a catalyst of stratospheric ozone depletion processes, which is very important for climate change studies due to its indirect effect on the global climate (Shindell et al., 2009).
The retrieval of total and tropospheric column NO2 from GOME-2 follows the typical two-step approach for a weakly absorbing trace gas. The DOAS approach is applied to GOME-2 (ir)radiance spectra to determine NO2 slant column densities at a wavelength range of 425–450 nm (Valks et al., 2011) for GOME-2/Metop-A and GOME-2/Metop-B (GDP – GOME Data Processor – 4.8). NO2 absorption structures are prominent, and the interference effects are manageable within this spectral window. In addition, GOME-2 measurements have a high signal-to-noise ratio in this wavelength band. For GOME-2/Metop-C (GDP 4.9), an alternative fitting window of 430.2–465 nm is used, as there are systematic structures in the DOAS fitting residual for GOME-2C for wavelengths <430 nm, which result in a large positive bias of ∼ 30 %. A single NO2 reference cross-sectional spectrum at 240 K (Vandaele et al., 2002), the interfering species ozone, O4 and H2O, and the Ring spectrum are included in the DOAS spectral retrieval. The temperature dependence of the NO2 absorption cross section has been taken into account in the air mass factor calculation to improve the accuracy of the retrieved columns.
The initial total VCD is retrieved assuming an unpolluted troposphere. Therefore, the air mass factor is weighted toward stratospheric NO2, whereas the tropospheric NO2 amount is assumed to be negligible. This assumption is valid over large parts of the earth, but in areas with significant tropospheric NO2, the total column densities are underestimated and need to be corrected. The air mass factors are calculated at the mid-point of the spectral-fitting window at 437.5 nm using the radiative transfer model LIDORT. A harmonic climatology of stratospheric NO2 profiles is used in the air mass factor calculation to incorporate the seasonal and latitudinal variation of stratospheric NO2. Stratospheric NO2 columns are estimated using the spatial filtering approach (Wenig et al., 2004; Valks et al., 2011). This method masks out potentially polluted areas and then applies a low-pass filter in the zonal direction to derive the stratospheric component. The tropospheric NO2 vertical columns are then derived from the residual tropospheric slant columns using a more accurate tropospheric air mass factor which considers the effects of clouds and the NO2 profile from a chemistry transport model. The derived tropospheric columns are used to correct the initial total NO2 columns under polluted conditions to provide an estimate of total vertical columns.
Monthly average NO2 profiles during the GOME-2 overpass time from the chemistry transport model MOZART-2 are used in the tropospheric air mass factor calculations. Cloud properties derived from GOME-2 using the OCRA and ROCINN algorithms (see Sect. 2.2.7) are used in the calculation of air mass factors in the case of (partly) cloudy conditions. The Clouds-As-Layers (CAL) model included in OCRA/ROCINN has also been implemented in our prototype GOME-2 NO2 algorithm, as described in Liu et al. (2020). The new NO2 algorithm uses an improved directionally dependent Lambertian-equivalent reflectivity (DLER) for AMF calculation. It is planned to implement the prototype GOME-2 NO2 algorithm in a future version of the operational GDP. The calculation of AMFs for (partly) cloudy conditions uses the independent pixel approximation. For measurements over cloudy scenes, the cloud top is usually well above the NO2 pollution in the boundary layer. When the clouds are optically thick, the enhanced tropospheric NO2 concentrations cannot be detected by the satellite, which can result in large errors. Therefore, GOME-2 measurements with a cloud radiance fraction >50 % are flagged in the level-2 data and filtered in the computation of the level-3 tropospheric NO2 product. More details of the GOME-2 total and tropospheric column NO2 retrieval can be found in Valks et al. (2011).
2.2.3 Total column water vapour
Water vapour is one of the major components in the atmosphere, with strong impacts on climate and weather, due to its abundance in the atmosphere, making it the most important natural greenhouse gas, accounting for more than 60 % of the greenhouse effect (Clough and Iacono, 1995; Kiehl and Trenberth, 1997). The knowledge of the spatiotemporal distribution of water vapour over the globe is essential for weather prediction and climate assessments. Improving the understanding of variability and changes in water vapour is vital, especially considering that, in contrast to most other greenhouse gases, the distribution of water vapour is highly variable due to its short atmospheric lifetime.
The GOME-2 operational total column water vapour (TCWV) algorithm is based on the DOAS and AMF approaches. The DOAS retrieval of water vapour slant columns is performed in the wavelength interval of 614–683 nm. Compared to other water vapour retrieval methods, this approach focuses only on the differential absorptions and is therefore less sensitive to instrument changes or instrument degradation issues. The algorithm consists of three basic steps: (1) DOAS fit for slant column retrieval, (2) non-linearity absorption correction of slant columns, and (3) slant to vertical column conversion using AMFs (Wagner et al., 2003, 2006).
The DOAS fit for water vapour retrievals takes into account O2 and O4 cross sections, in addition to that of water vapour. Three types of vegetation spectra (Wagner et al., 2007), a synthetic Ring spectrum, and an inverse solar spectrum are included in the DOAS fit to improve the broadband filtering and to correct for possible offsets, e.g. caused by instrumental stray light. The retrieved water vapour slant columns are then corrected for the non-linearities arising from the fact that the fine-structure water vapour absorption lines are not spectrally resolved by the GOME-2 instruments. The corrected water vapour slant columns are divided by the “measured” AMFs to convert to vertical columns. The “measured” AMF is defined as the ratio between the measured O2 slant column retrieved at the same wavelength band and the known O2 vertical column from the standard atmosphere. This simple approach has the advantage that it corrects to first order for the effect of albedo variation, aerosol load, and cloud cover without the use of additional independent information. It is also important to note that the GOME-2 water vapour product does not rely on additional information, except for the use of an albedo database for the AMF correction. The surface albedo used for the correction is taken from a monthly albedo database derived from GOME-1 observations (Koelemeijer et al., 2003) for high latitudes (>50∘) and SCIAMACHY observations (Grzegorski, 2009) at mid and low latitudes (<40∘). For the transition between 40 and 50∘, both products are interpolated linearly. This serves the aim of deriving a climatologically relevant time series of TCWV measurements (Wagner et al., 2006; Lang et al., 2007; Noël et al., 2008). The current version of GOME-2 TCWV retrieval does not account for the terrain effect with elevated surface in the AMF correction, i.e. over high-mountain areas (>1000 m), and the retrieval errors in TCWV are significantly higher. Therefore, these measurements are flagged and are not used in the level-3 data processing.
GOME-2 measurements significantly contaminated by clouds are also flagged in the level-2 products and filtered in the level-3 products. The cloud flag in the water vapour product is set based on two indicators. The first cloud flag is set if the retrieved O2 slant column is below 80 % of the maximum O2 slant column for the respective solar zenith angle (roughly when about 20 % from the column to the ground is missing). Especially for low and medium-high clouds, the relative fraction of the column shielded by clouds for O2 and water vapour can be very different. The second cloud flag is set if cloud fraction and cloud-top albedo exceed 0.6. More details on the GOME-2 total column water vapour retrieval can be found in Grossi et al. (2015).
2.2.4 Total column bromine monoxide
Bromine monoxide in the lower stratosphere is involved in chain reactions that deplete ozone (Wennberg et al., 1994), while in the troposphere BrO changes the oxidizing capacity through the destruction of ozone. In particular, large amounts of BrO are often observed in the polar boundary layer during springtime (Platt and Wagner, 1998; Richter et al., 1998), known as “bromine explosion”, and lead to severe tropospheric ozone depletion by autocatalytic reactions. In addition to polar sea ice regions, enhanced BrO concentrations were also detected over salt lakes/marshes (Hebestreit et al., 1999; Hörmann et al., 2016), in the marine boundary layer, and in volcanic plumes (Theys et al., 2009; Hörmann et al., 2013).
The GOME-2 total BrO retrieval is also a typical DOAS and AMF algorithm. The DOAS retrieval of BrO slant columns is applied to the spectral range of 332–359 nm, which covers five BrO absorption peaks and minimized the interference from other trace gases, especially formaldehyde (Theys et al., 2011). This fitting window can also minimize other artefacts due to instrument noise, viewing angle dependency, and interference from incomplete ring-effect correction.
The instrumental degradation of GOME-2 has negative influences on the spectral fit and results in higher residuals, thus affecting the noise level in the BrO columns and the average slant column values. Therefore, an equatorial offset correction is applied on a daily basis to the BrO data (Richter et al., 2002). This correction reduces the influences of the instrumental degradation on the total BrO column time series. Averaged BrO slant columns in the tropical latitudinal band ±5∘ are calculated on a daily basis, assuming small equatorial BrO columns with no significant seasonal variations. These averaged slant columns are then subtracted from all slant columns, and a constant equatorial slant column offset of 7.5 × 1013 molec cm−2 is added. Corrected BrO slant columns are then converted to vertical columns by using AMFs. In the GOME-2 total column BrO retrieval, AMFs are calculated at 344 nm (mid-point of the DOAS fitting range) using the radiative transfer model LIDORT (Spurr, 2008). Monthly climatology BrO profiles from the chemistry transport model SLIMCAT (Chipperfield, 1999; Bruns et al., 2003) are used in the AMF calculations. In (partly) cloudy cases, GOME-2 cloud properties determined with the OCRA and ROCINN algorithms (see Sect. 2.2.7) are used to calculate the air mass factors in association with the independent pixel approximation. As BrO has a major contribution from the stratosphere, which is usually above clouds, all measurements (cloudy and clear-sky) are used in the level-3 product. More details on the GOME-2 total column BrO retrieval can be found in Theys et al. (2011).
2.2.5 Total column formaldehyde
Formaldehyde is an intermediate product of the oxidation of almost all volatile organic compounds (VOCs). Therefore, it is widely used as an indicator of non-methane volatile organic compounds (NMVOCs) (Fried et al., 2011). VOCs also have significant impacts on the abundance of hydroxyl (OH) radicals in the atmosphere, which is the major oxidant in the troposphere. Major HCHO sources over continents include the oxidation of VOCs emitted from plants, biomass burning, traffic, and industrial emissions. Oxidation of methane (CH4) emitted from the ocean is the main source of HCHO over water and pristine continental areas.
The GOME-2 total HCHO column retrieval algorithm also follows the two-step approach with DOAS retrieval of HCHO slant columns and subsequently converts the slant columns to vertical columns by using AMFs. To reduce the interference between HCHO and BrO absorption features, a two-step DOAS retrieval of HCHO slant columns is used (De Smedt et al., 2012). The first step is to determine BrO slant columns with a larger fitting window of 332–359 nm which includes five BrO absorption peaks and effectively minimizes the cross-correlation between BrO and HCHO. The retrieved BrO slant columns are then fixed in the subsequent DOAS retrieval of HCHO slant columns in the spectral band of 328.5–346 nm.
Although the DOAS fit settings are optimized to minimize interference from other factors, there are still unresolved spectral absorption interferences between HCHO and BrO and results with obvious zonal and seasonal dependency. In order to reduce the impact of the artefacts, an absolute normalization is applied to HCHO slant columns on a daily basis using the reference sector method (Khokhar et al., 2005). The reference sector is chosen over the Pacific Ocean (longitude: 140–160∘ W), where the only source of HCHO is the oxidation of CH4, which can be reproduced by model simulation quite well. The mean HCHO slant column density in the reference sector is determined by a polynomial fit, which is then subtracted from the retrieved slant columns on this day and replaced by a HCHO background value taken from IMAGES version-2 chemistry transport model simulations (Müller and Stavrakou, 2005).
Corrected HCHO slant columns are then converted to vertical columns by using AMFs. HCHO AMFs are calculated at 335 nm using the radiative transfer model LIDORT. Monthly averaged profiles taken from chemistry transport model (CTM) IMAGES version-2 simulation in 2007 are used as a priori HCHO profiles in the AMF calculations. The IMAGES version-2 model simulations are at a horizontal resolution of 2.0∘ (latitude) × 2.5∘ (longitude), with 40 vertical layers extending from the surface up to ∼ 44 hPa. In the case of the presence of clouds, cloud properties derived by the OCRA and ROCINN algorithms (see Sect. 2.2.7) are used to calculate the air mass factors using the independent pixel approximation. For cloudy scene measurements, clouds are usually above the boundary layer, where the major part of HCHO is located. If the clouds are optically thick, HCHO below cloud cannot be detected by the satellite and results in large uncertainties. Therefore, measurements with a cloud radiance fraction >50 % are flagged and are not used in the level-3 data processing. More details on the GOME-2 total column HCHO retrieval can be found in De Smedt et al. (2012).
2.2.6 Total column sulfur dioxide
Sulfur dioxide is an important trace species playing a key role in atmospheric chemistry on both local and global scales through the formation of sulfate aerosols and sulfuric acid. The impacts of SO2 range from short-term pollution to climate forcing. SO2 is emitted into the atmosphere through both natural and anthropogenic processes. About one-third of the global sulfur emissions originate from natural sources (volcanoes and biogenic dimethyl sulfide), and the major contributors to the total budget are anthropogenic emissions through the combustion of fossil fuels (coal and oil) and smelting.
The GOME-2 SO2 retrieval algorithm also follows the two-step approach with DOAS retrieval of SO2 slant columns and subsequently converts the slant columns to vertical columns by using AMFs. The DOAS algorithm for SO2 is based on the DOAS fit settings dedicated to ozone retrieval, with adjustments to optimize for SO2 retrieval (Rix et al., 2009, 2012). The DOAS fit for the retrieval of SO2 slant columns is applied to the wavelength ranges of 315–326 nm for GOME-2 and Metop-A and Metop-B (GDP 4.8) and 312–325 nm for GOME-2/Metop-C (GDP 4.9).
The background level of atmospheric SO2 is very low over large parts of the earth. To account for any systematic bias in the retrieved total column SO2 and to ensure geospatial consistency of the results, a background correction is applied to the data to avoid non-zero columns over regions known to have very low SO2 and at high solar zenith angles. The background correction scheme calculates offset SO2 slant columns based on latitude and surface height information. This offset is calculated on a daily basis with measurements binned to 2∘ resolution in latitude. In addition, the offset values are calculated separately at five surface altitude bins. Median offset values based on the calculated offset values in the last 2 weeks before the day of interest are used as the corresponding offset SO2 slant columns to minimize the influences from outliers or missing data in the daily data set. This latitude- and altitude-dependent value is then subtracted from the SO2 slant column derived from the DOAS retrieval.
Corrected SO2 slant columns are then converted to vertical columns by using AMFs. The major challenge in the SO2 retrieval is that the vertical distribution of SO2 in the atmosphere is usually unknown. Depending on the type of emission, SO2 can be located from the ground up to the stratosphere. In the GOME-2 SO2 retrieval, this assumes two scenarios: (1) volcanic eruption emissions and (2) anthropogenic sources. For the volcanic emission scenario, the AMFs are calculated assuming the SO2 plume follows a Gaussian profile shape with central plume heights at 15.0, 6.0, and 2.5 km above sea level. For anthropogenic emissions, the AMFs are calculated assuming a homogeneous layer from the surface up to 1 km. The GOME-2 SO2 product typically refers to the volcanic scenario with an assumed plume height at 6 km. The AMF is calculated at 320 nm (GOME-2A and GOME-2B) and 313 nm (GOME-2C) using the radiative transfer model LIDORT. For scenarios in the presence of clouds, GOME-2 cloud properties determined with the OCRA and ROCINN algorithms are used to calculate the air mass factors.
2.2.7 Cloud parameters
It is very important to derive cloud properties from GOME-2 observations as clouds significantly affect the retrieval of tropospheric trace gases. The most predominant effect of clouds in trace gas retrieval is that they shield the trace gas absorption below clouds. However, clouds can also enhance the absorption due to multiple scattering within the cloud. The GOME-2 retrieval of the trace gas vertical columns assumes independent pixel approximation (IPA) for cloudy scene measurements. For tropospheric species, i.e. tropospheric NO2, water vapour, and HCHO, especially in the case of low clouds and large cloud fractions, the presence of clouds can result in large errors. Therefore, measurements with high cloud cover are flagged in these products and are filtered in the level-3 process.
The OCRA and ROCINN algorithms (Loyola et al., 2007; Lutz et al., 2016) are used to obtain cloud information from GOME-2 observations. Clouds are treated as reflecting Lambertian surfaces in the algorithm and cloud information is reduced to the specification of three parameters: cloud fraction, cloud-top albedo, and cloud-top pressure. The radiometric cloud fraction is retrieved from the broadband polarization of UVN measurements (UV–VIS–NIR) by OCRA, while effective cloud pressure and cloud albedo are retrieved by ROCINN from observations in the oxygen–A band (O2–A) around 760 nm. The OCRA algorithm separates each measurement into two components: a cloud-free background and a residual contribution describing the influence of clouds. The key to the algorithm is the construction of a cloud-free composite that is invariant with respect to the atmosphere, to the topography, and to the solar and viewing geometries. The effective cloud fraction is determined by examining the separation between the reflectance measured by the PMDs of GOME-2 and their cloud-free composite values. Note that OCRA is also sensitive to scattering by aerosols present in a given GOME-2 scene. Therefore, the retrieved cloud fraction also includes the aerosol effects. Furthermore, the GOME-2 cloud algorithm has been improved to distinguish clouds for measurements affected by sun glint over the ocean, which is a common phenomenon that occurs at the edges of the GOME-2 swath. The detection of cloud over sun glint is achieved by analysing the broadband polarization measurements (Loyola et al., 2011; Lutz et al., 2016). Note that the OCRA/ROCINN algorithm was recently upgraded to include the retrieval of cloud-top height and cloud optical thickness using the CAL model (Loyola et al., 2018), and this new feature has been implemented to process TROPOMI data. We are also planning to implement the new feature in the GOME-2 operational GDP in the future (see Sect. 2.2.2).
The cloud fraction derived from OCRA is then ingested by ROCINN as fixed input (Loyola et al., 2007), which derived cloud-top height and cloud albedo using measurements at the O2–A band. In the radiative transfer simulations, oxygen absorption in the earthshine spectra, including the reflection from the earth's surface and cloud top, is considered in the atmospheric radiative transfer. Surfaces are assumed to be Lambertian reflectors. Black-sky albedo climatology from the MEdium Resolution Imaging Spectrometer (MERIS) is used as input for the radiative transfer and in ROCINN version 3. Radiative transfer simulations in ROCINN include Rayleigh scattering and polarization. High-resolution reflectances computed with Vector LInearized Discrate Ordinate Radiative Transfer (VLIDORT) (Spurr, 2006) are used to create a complete data set of simulated reflectance for all viewing geometries and geophysical scenarios and for various combinations of cloud fraction, cloud-top height, and cloud-top albedo. The inversion is performed using neural network techniques. The cloud-top height retrieved by ROCINN is converted to cloud-top pressure assuming the U.S. Standard Atmosphere (Anderson et al., 1986). The retrieved cloud properties are then used in the subsequent processing of trace gas column retrieval and are provided in the corresponding level-3 products.
2.3 Validation data sets
2.3.1 Brewer ozone measurements
Brewer ozone data are obtained from the World Ozone and Ultraviolet Radiation Data Center (WOUDC, http://www.woudc.org, last access: 15 March 2023). The WOUDC data centre is part of the Global Atmosphere Watch (GAW) programme of the World Meteorological Organization (WMO), providing quality-assured Brewer measurements. Brewer instruments measure intensity at several wavelength intervals in the UV band. Total column ozone is retrieved from the relative intensities among these UV channels. Brewer ozone data have long been used to validate satellite observations of ozone (Balis et al., 2007a, b; Antón et al., 2009; Loyola et al., 2011; Koukouli et al., 2012, 2015; Garane et al., 2018, 2019). In this study, we only use the direct sun Brewer observations of total column O3 for the validation of the GOME-2 level-3 product.
2.3.2 Zenith-scattered-light differential optical absorption spectroscopy and multi-axis differential optical absorption spectroscopy NO2 measurements
Zenith-scattered-light differential optical absorption spectroscopy (ZSL-DOAS) data are obtained from the Network for the Detection of Atmospheric Composition Change (NDACC). The NDACC ZSL-DOAS network provides total column NO2 observations with standardized operating procedures and harmonized retrieval methods. ZSL-DOAS data from NDACC stations are available at the NDACC data host facility (see http://www.ndacc.org, last access: 15 March 2023). ZSL-DOAS measurements during twilight periods are sensitive to stratospheric absorbers due to the geometrical enhancement of the optical path in the stratosphere. Therefore, it has long been used for the validation of satellite total NO2 observations (Ionov et al., 2008; Celarier et al., 2008). The retrieval of total column NO2 from ZSL-DOAS observations is based on the Langley method, which calculates the corresponding air mass factor according to its observation and solar geometry. As most of the ZSL-DOAS sites are located in relatively clean regions, therefore, the major contribution of total column NO2 is expected to be coming from the stratosphere. Due to the morning overpass time of GOME-2, ZSL-DOAS observations of total column NO2 during the morning twilight period are used to validate GOME-2 level-3 total NO2 products. As the measurement times of GOME-2 and ZSL-DOAS are close, therefore, these data are comparable without the need for photochemical correction.
Multi-axis differential optical absorption spectroscopy (MAX-DOAS) is a passive remote-sensing technique which uses spectroscopic observations of scattered sunlight at different viewing zenith angles to derive column densities of a trace gas. Due to its compact experimental set-up and high sensitivity to the lower troposphere, it has been widely used for the validation of satellite observations of tropospheric column NO2 (Brinksma et al., 2008; Celarier et al., 2008; Irie et al., 2008, 2009, 2012, 2016; Ma et al., 2013; Kanaya et al., 2014; Chan et al., 2015, 2018, 2019, 2020b; Drosoglou et al., 2017; Wang et al., 2017; Compernolle et al., 2020; Pinardi et al., 2020a; Verhoelst et al., 2021). Ground-based MAX-DOAS instruments are operated by various research institutes around the world, and the data are centrally managed by BIRA-IASB within the context of NItrogen Dioxide and FORmaldehyde VALidation (NIDFORVAL). The affiliation of MAX-DOAS instruments in the NDACC network is still under progress, following efforts made in NORS, QA4ECV, and the European Space Agency's (ESA's) FRM4DOAS project to harmonize and automatize data processing. In this study, MAX-DOAS observations of tropospheric column NO2 are used to validate GOME-2 level-3 tropospheric NO2 products.
2.3.3 Sun-photometer water vapour measurements
The AERosol RObotic NETwork (AERONET) uses CIMEL CE-318 sun photometers to measure direct sun and sky radiance at multiple wavelengths (Holben et al., 1998). These sun-photometer observations provide information on not only aerosol optical properties (Holben et al., 2001), but also on columnar water vapour content (Alexandrov et al., 2009). Water vapour columns are retrieved from sun-photometer observations in the NIR at 940 nm, where water vapour absorption is rather strong. The inversion of water vapour columns is based on the attenuation of radiation through the atmosphere. A more detailed description of the water vapour retrieval algorithm can be found in Alexandrov et al. (2009). In total, there are over 1000 AERONET stations around the globe, providing columnar water vapour observations, and they have been used extensively for satellite validation (Bennouna et al., 2013; Diedrich et al., 2015; Martins et al., 2019; Chan et al., 2020a; Garane et al., 2023). The AERONET water vapour product has also been validated by microwave radiometry, GPS, and radiosonde measurements (Pérez-Ramírez et al., 2014). The sun-photometer measurements in general underestimate the columnar water vapour by 6 %–9 % (Pérez-Ramírez et al., 2014). In this study, cloud-screened and quality-assured level-2 data are used to validate GOME-2 level-3 total column water vapour products.
2.3.4 ZSL-DOAS BrO measurements
The ZSL-DOAS observations at Harestua (60.22 ∘ N, 10.75 ∘ E), Norway, are used to validate the GOME-2 level-3 total column BrO product. ZSL-DOAS observations of total BrO columns are photochemically corrected to the GOME-2 overpass time (09:30 LT). The operation of the ZSL-DOAS instrument and the retrieval of the BrO column are performed by BIRA-IASB. A detailed description of the ZSL-DOAS instrument set-up and the BrO column retrieval algorithm can be found in Hendrick et al. (2007).
2.3.5 MAX-DOAS HCHO measurements
Ground-based MAX-DOAS observations are used to validate the GOME-2 level-3 total column HCHO product. MAX-DOAS observations show very good sensitivity in the troposphere, where most of the HCHO resides. Therefore, it has long been used for satellite validation (Vigouroux et al., 2009; Li et al., 2013; De Smedt et al., 2015b, 2021; Wang et al., 2017; Chan et al., 2019, 2020b; Kumar et al., 2020). The retrievals of HCHO columns from MAX-DOAS observations are performed within a wavelength range similar to the GOME-2 retrieval, i.e. 328–359 nm. Ground-based MAX-DOAS instruments are operated by various research institutes around the world, and the data are centrally managed by BIRA-IASB within the context of NIDFORVAL.
2.3.6 Pandora SO2 measurements
The Pandonia global network is a direct sun-spectrometer network used to monitor trace gas worldwide. The Pandora instrument is used to measure columnar amounts of trace gases in the atmosphere. Pandora determines trace gas amounts from direct-sun observations by using the DOAS technique with the theoretical solar spectrum as a reference. As the anthropogenic SO2 emission has been reduced significantly in recent decades, the background SO2 level is mostly zero around the globe and only a few locations with signification anthropogenic SO2 sources. Considering the low background SO2 level and the high measurement noise of SO2 data, it is more appropriate to validate the satellite observations over locations with significant variation and sources. Mexico City is one of the few places with significant anthropogenic SO2 sources. Therefore, we use the Pandora SO2 observations at Mexico City to validate GOME-2 level-3 total column SO2 products.
3.1 Gridding algorithm
GOME-2 level-3 data products are developed with the aim of providing easily translatable data sets to both facilitate scientific progress (e.g. on climate trend analysis and low-frequency climate variability) and satisfy public interest. The processing of GOME-2 level-3 data requires binning of the level-2 data onto a regular two-dimensional latitude–longitude grid.
The binning of level-2 data to a regular latitude–longitude grid includes taking the arithmetic mean and standard deviation of all level-2 data points falling onto the grid cell in a given period, i.e. a day or a month, with possible trimming of low-quality measurements due to a large spectral-fit residual and cloud contamination for tropospheric species, i.e. tropospheric NO2, water vapour, and HCHO. For all species, only forward-scan pixels are used in the gridding process. In case of cloudy measurements, most of the tropospheric gases, i.e. tropospheric NO2, water vapour, and HCHO, are mainly situated below clouds, while satellite observations could not measure the part below cloud and resulted in large uncertainties. Therefore, these measurements are not used in the production of level-3 data. For stratospheric species, i.e. total column O3, NO2, BrO, and SO2, no cloud filtering is applied.
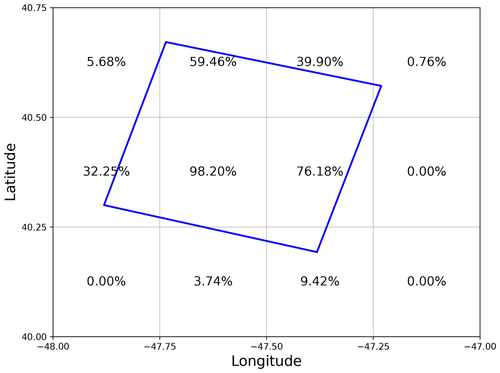
Figure 1A GOME-2A ground pixel (blue) overlaid on a 0.25∘ × 0.25∘ latitude–longitude grid (grey). The percentage of overlap (weighting) for each grid box is indicated.
Several gridding routines have been developed to create global and regional maps of the trace gas distribution, e.g. Wenig et al. (2008), Chan et al. (2012), and Kuhlmann et al. (2014). These gridding algorithms typically assume that measurement values are constant within the satellite pixel boundaries. This assumption is considered sufficient for creating global maps. A more sophisticated approach uses a parabolic spline method to interpolate adjacent satellite pixels to create high-resolution (e.g. 1 km × 1 km) regional maps (Kuhlmann et al., 2014). As GOME-2 ground pixel size is relatively large, a significant grid effect would be induced by assigning each GOME-2 measurement to a single grid cell based on their centre coordinates of the GOME-2 ground pixel without taking into account the pixel geometry and extension. Therefore, the gridding process considers the overlapping area of the GOME-2 ground pixel and the latitude–longitude grid. For grid cells partially overlapping with the satellite pixel, the percentage of overlap (a satellite pixel fully covering the entire grid cell is considered 100 % overlap) is calculated and used as weighting for the calculation of the mean value, uncertainty, and standard deviation. Figure 1 shows an example of the calculation of the weighting (percentage of overlap) for grid boxes overlapping with a GOME-2A ground pixel. The gridded columns can be expressed as Eq. (1).
where VCDg is the gridded trace gas column, while VCDi represents each individual satellite measurement (partly) overlapping with the grid cell. The weighting is denoted as w, which is the percentage of the grid cell covered by the satellite pixel. The weighting or the number of observations is also included in the level-3 product. The uncertainty of gridded columns can be expressed as Eq. (2).
where Eg is the uncertainty of the gridded trace gas column, while Ei represents the uncertainty of each individual measurement. The standard deviation of gridded columns can be expressed as Eq. (3).
where σg is the standard deviation of the gridded trace gas column.
3.2 Sampling resolution
The processing of GOME-2 level-3 data requires binning of the level-2 data onto a regular two-dimensional latitude–longitude grid. The selection of an appropriate resolution of the latitude–longitude grid is essential for the production of the level-3 products. On the one hand, it is important to preserve the original spatial features captured in the level-2 data with higher spatial resolution, but on the other hand, it is necessary to keep the data files to a reasonable size to be user-friendly.
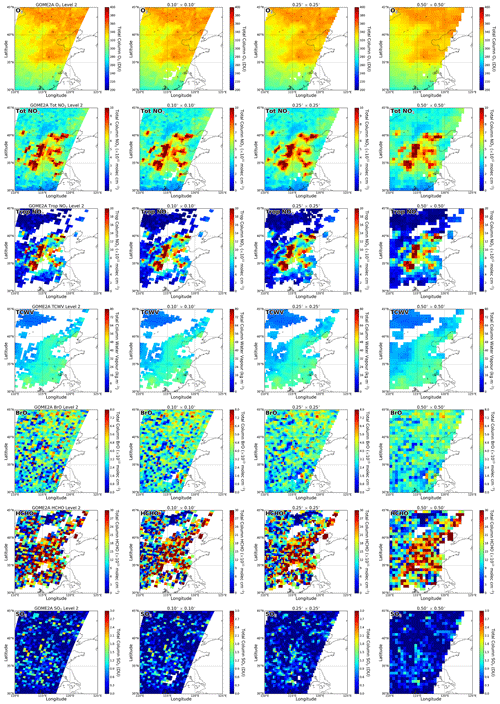
Figure 2GOME-2A observations of total column O3 (first row), total column NO2 (second row), tropospheric column NO2 (third row), total column water vapour (fourth row), total column BrO (fifth row), total column HCHO (sixth row), and total column SO2 (seventh row). Data are shown at the original instrument resolution (first column from the left), gridded with 0.1∘ × 0.1∘ resolution (second column from the left), 0.25∘ × 0.25∘ resolution (third column from the left), and 0.5∘ × 0.5∘ resolution (column on the right). GOME-2A observations on 15 July 2014 over North China are shown. Missing data are mainly due to cloudiness.
To select the best spatial resolution for the level-3 product, we have analysed the binning results with various resolutions, i.e. 0.1∘ × 0.1∘, 0.25∘ × 0.25∘, and 0.5∘ × 0.5∘. Figure 2 shows GOME-2A data of each trace gas species gridded at different resolutions and level-2 data at the original instrument resolution for an orbit over North China on 15 July 2014. Missing data are mainly due to filtering of cloudy pixels and other low-quality observations. GOME-2A data are shown due to their highest spatial resolution among all three GOME-2 instruments (GOME-2A: 40 km × 40 km after 15 July 2013, GOME-2B and GOME-2C: 40 km × 80 km). We looked into the spatial smoothing/averaging effect over North China, as this region is expected to show strong spatial gradients of tropospheric pollutants, i.e. NO2. Data at all four resolutions show very similar spatial structures. The absolute values of level-3 data are also consistent with the level-2 product. The results show that gridding GOME-2 data with a higher spatial resolution (i.e. 0.1∘ × 0.1∘) better preserves the original GOME-2 instrument footprint, while a rather strong smoothing/averaging effect is observed from data gridded with a lower spatial resolution (i.e. 0.5∘ × 0.5∘). Although gridded data with a 0.25∘ × 0.25∘ resolution show some smoothing/averaging effects, they still capture the spatial variations reasonably well.
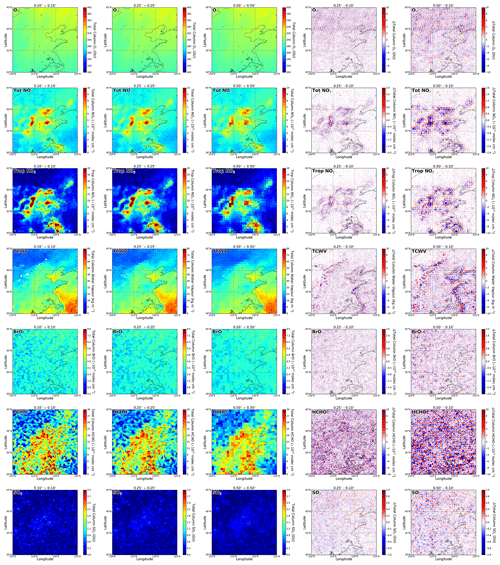
Figure 3Monthly averaged GOME-2A observations of total column O3 (first row), total column NO2 (second row), tropospheric column NO2 (third row), total column water (fourth row), vapour total column BrO (fifth row), total column HCHO (sixth row), and total column SO2 (seventh row) over North China in July 2014. Gridded data with 0.1∘ × 0.1∘ resolution (first column from the left), 0.25∘ × 0.25∘ resolution (second column from the left), and 0.5∘ × 0.5∘ resolution (third column from the left) are shown. Differences between 0.1∘, 0.25∘, and 0.5∘ are also shown for reference.
Figure 3 shows the monthly averaged GOME-2A data of each trace gas species gridded at different resolutions over North China in July 2014. Differences between data gridded with different resolutions are also shown for reference. Data gridded at all three resolutions show very similar spatial structures. Hotspots of anthropogenic pollutants, i.e. tropospheric NO2, can be clearly observed from the monthly averaged data. Species with major contributions from natural sources, e.g. O3 and water vapour, show a rather smooth appearance. Despite the fact that large numbers of observations are included in the monthly averaging process, species with lower signal-to-noise ratios, e.g. HCHO and SO2, still show rather high background noise. This is mainly due to the low column density and absorption of these species. This effect is as expected more significant for data gridded at a higher spatial resolution, i.e. 0.1∘ × 0.1∘, due to less spatial averaging. Traces of the satellite footprints can still be seen in the 0.1∘ × 0.1∘-resolution monthly averaged data, while the satellite footprints are much less significant in the 0.25∘ × 0.25∘- and 0.5∘ × 0.5∘-resolution data. The differential plots between data gridded with 0.1∘ × 0.1∘ and 0.25∘ × 0.25∘ resolution in general show only very small differences. Slightly larger discrepancies mainly appear over pollution hotspots, i.e. for tropospheric NO2. In contrast, data at 0.5∘ × 0.5∘ resolution show much bigger differences from 0.1∘ × 0.1∘-resolution data. Compared to 0.25∘ × 0.25∘-resolution data, 0.5∘ × 0.5∘-resolution data show 2 to 4 times higher underestimation of tropospheric NO2 columns over pollution hotspots. The comparison of GOME-2 data gridded at different resolutions indicates that 0.25∘ × 0.25∘ resolution is a balance to preserve the satellite resolution (GOME-2A: 40 km × 40 km, GOME-2B and GOME-2C: 40 km × 80 km) while capturing the strong spatial variations for most of the tropospheric gases, i.e. NO2, water vapour, and HCHO. In addition, the data file size of level-3 products with 0.1∘ × 0.1∘ resolution is about 6 times larger than that of 0.25∘ × 0.25∘, while the information content does not show a significant difference, especially for monthly products. Therefore, we concluded that 0.25∘ × 0.25∘ resolution is a suitable choice for GOME-2 level-3 products.
3.3 Verification and validation methods
The GOME-2 level-3 products are generated from the level-2 data sets which have already been fully validated (see the validation reports in https://acsaf.org/valreps.php, last access: 15 March 2023). Therefore, the verification and validation of the GOME-2 level-3 product mainly focus on two major aspects, the consistency among the three GOME-2 sensors and the comparison to reference ground-based measurements. Each GOME-2 level-3 product is compared to different reference ground-based measurements, and information on the reference ground-based measurements used to validate GOME-2 level-3 products is listed in Table 3.
The comparison of GOME-2 level-3 data to reference ground-based measurements requires spatial and temporal matching of the two data sets. The following criteria are applied to co-locate the GOME-2 level-3 products and ground-based reference data sets.
-
The grid cells of the level-3 GOME-2 products covering the ground-based measurement site are paired with the daily/monthly ground-based measurements.
-
For ground-based Brewer, MAX-DOAS, sun-photometer, and Pandora measurements, they are temporally averaged around the GOME-2 overpass time from 08:30 to 10:30 (local time).
-
For ZSL-DOAS measurements, morning twilight period measurement is used for comparison.
After co-locating the GOME-2 and ground-based data sets, we compare the GOME-2 level-3 products to reference ground-based data sets through a scatter plot and histogram of the differences and sort the differences/biases by year, latitude band, or measurement site as a box plot and time series to investigate the systematic bias/error.
The GOME-2 level-3 products are at two different temporal resolutions, daily and monthly. Both daily and monthly level-3 products consist of gridded trace gas columns and other auxiliary parameters, i.e. cloud, surface, and statistical parameters. The level-3 products are separated for each species (i.e. O3, NO2, water vapour, BrO, HCHO, and SO2) and each GOME-2 instrument (i.e. GOME-2A, GOME-2B, and GOME-2C). All products are at a spatial resolution of 0.25∘ × 0.25∘, with coordinates ranging from 180∘ W to 180∘ E in longitude and from 90∘ S to 90∘ N in latitude (720 (latitude) × 1440 (longitude) grid cell). The data are organized into a user-friendly and self-describing NetCDF-4 (Network Common Data Form) format, based upon the instrument/platform (GOME-2A/Metop-A, GOME-2B/Metop-B, or GOME-2C/Metop-C) and the temporal period of collection (daily or monthly data set).
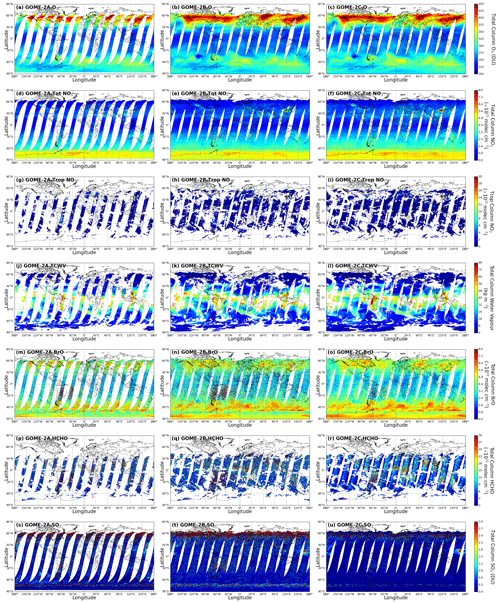
Figure 4Daily level-3 product of GOME-2A (first column), GOME-2B (second column), and GOME-2C (third column) for 15 January 2020. Total column O3 (first row), total column NO2 (second row), tropospheric column NO2 (third row), total column water vapour (fourth row), total column BrO (fifth row), total column HCHO (sixth row), and total column SO2 (seventh row) are shown.
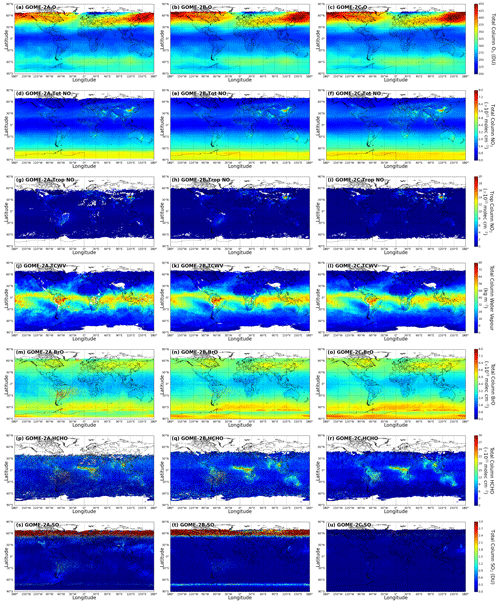
Figure 5Monthly level-3 product of GOME-2A (first column), GOME-2B (second column), and GOME-2C (third column) for January 2020. Total column O3 (first row), total column NO2 (second row), tropospheric column NO2 (third row), total column water vapour (fourth row), total column BrO (fifth row), total column HCHO (sixth row), and total column SO2 (seventh row) are shown.
Figure 4 shows examples of the daily level-3 products for all trace gases and all GOME-2 instruments, while examples of monthly level-3 data are shown in Fig. 5. Missing data are mainly due to filtering of low-quality data, e.g. cloud contamination, high solar zenith angle, and high spectral fit residual. The spatial coverage of the GOME-2A daily product is different from GOME-2B and GOME-2C due to the improvement in spatial resolution after it went into tandem operation with GOME-2B in July 2013. The noise levels of monthly GOME-2A data are significantly higher than those of GOME-2B and GOME-2C. This is mainly related to less spatial averaging and instrument aging. This effect is particularly obvious for species with a lower signal-to-noise ratio, e.g. HCHO and SO2. In addition, the stripe pattern is also more significant for GOME-2A, e.g. the water vapour product, due to the narrower swath width of GOME-2A measurements.
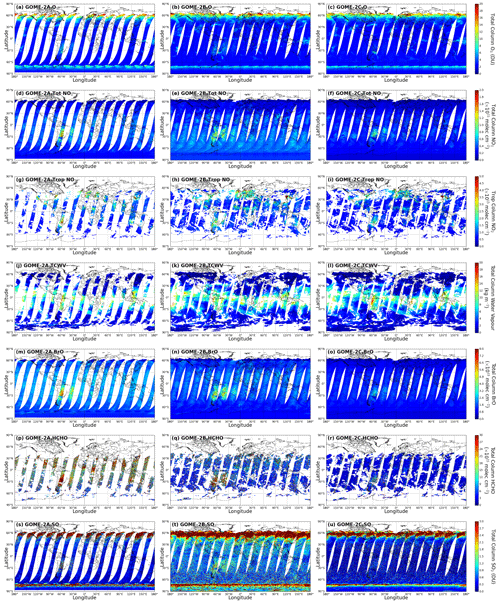
Figure 6Derived measurement errors in the daily level-3 product of GOME-2A (first column), GOME-2B (second column), and GOME-2C (third column) for 15 January 2020. Measurement errors of total column O3 (first row), total column NO2 (second row), tropospheric column NO2 (third row), total column water vapour (fourth row), total column BrO (fifth row), total column HCHO (sixth row), and total column SO2 (seventh row) are shown.
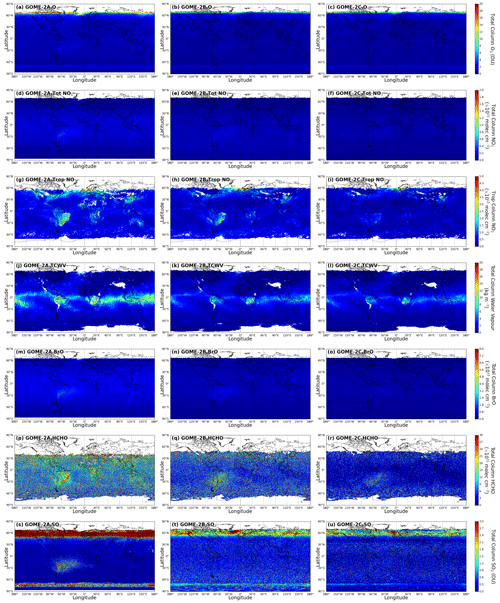
Figure 7Derived measurement errors in the monthly level-3 product of GOME-2A (first column), GOME-2B (second column), and GOME-2C (third column) for January 2020. Measurement errors of total column O3 (first row), total column NO2 (second row), tropospheric column NO2 (third row), total column water vapour (fourth row), total column BrO (fifth row), total column HCHO (sixth row), and total column SO2 (seventh row) are shown.
Figures 6 and 7 show examples of derived measurement errors in the daily and monthly level-3 products for all trace gases and all GOME-2 instruments. The measurement errors are in general related to the instrument noise, a priori assumption in the AMF calculations, and cloud effect and climatological input parameters, such as surface albedo and pressure profile. Some of the terms are multiplicable, such as AMFs, and therefore they are higher where the columns are higher. For measurement with a lower signal-to-noise ratio, such as HCHO and SO2, the measurement errors increase significantly in the polar regions, where the radiance intensities are low, as they are observed with higher solar zenith angles. The inclusion of measurement error in the level-3 products provides addition information on the measurement accuracy, and users can use it as an indicator to make additional filtering of the data.
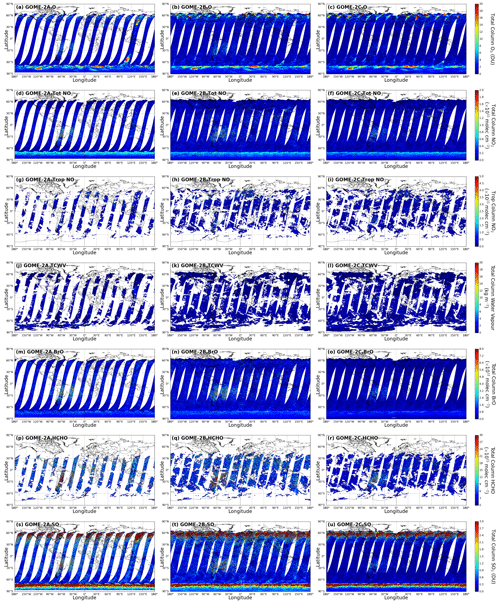
Figure 8Derived standard deviation in the daily level-3 product of GOME-2A (first column), GOME-2B (second column), and GOME-2C (third column) for 15 January 2020. Standard deviation of total column O3 (first row), total column NO2 (second row), tropospheric column NO2 (third row), total column water vapour (fourth row), total column BrO (fifth row), total column HCHO (sixth row), and total column SO2 (seventh row) are shown.
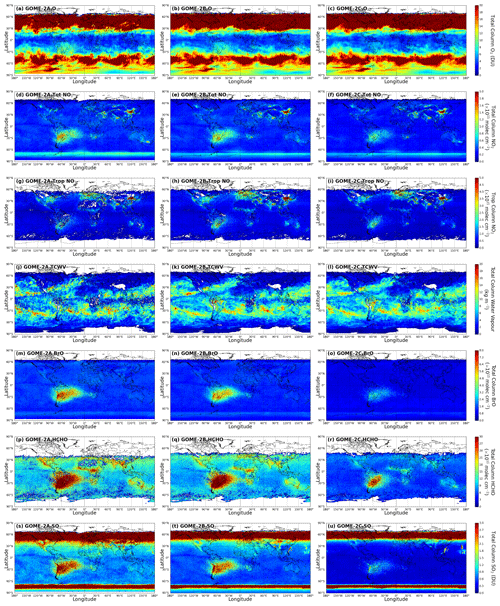
Figure 9Derived standard deviation in the monthly level-3 product of GOME-2A (first column), GOME-2B (second column), and GOME-2C (third column) for January 2020. Standard deviation of total column O3 (first row), total column NO2 (second row), tropospheric column NO2 (third row), total column water vapour (fourth row), total column BrO (fifth row), total column HCHO (sixth row), and total column SO2 (seventh row) are shown.
Figures 8 and 9 show examples of the standard deviation in the daily and monthly level-3 products for all trace gases and all GOME-2 instruments. The standard deviation in most areas (except the polar regions) in the daily products is expected to be close to 0, as there is only overlap with neighbouring pixels measured at roughly the same time of the day, while the standard deviation over the polar regions is expected to be higher as there are multiple measurements at different times of the day. The standard deviation in the monthly products reflects both the instrument/measurement noise and the natural variability. Higher standard deviations of SO2 columns over the polar regions are related to the low signal-to-noise ratio for observations taken with high solar zenith angles. In addition, the standard deviations of NO2, BrO, HCHO, and SO2 are higher over South America, mainly due to high cloudiness, while the standard deviations of O3 and water vapour columns are mainly driven by their natural variability. The standard deviation in the level-3 products provides additional information on the measurement precision and natural variability. Depending on the propose, users can use it to filter the data or study the variability.
In this section, we present validation results of the GOME-2 level-3 products. The GOME-2 level-3 products are first examined with respect to their cross-sensor consistency. In addition, level-3 products of each trace gas are compared to ground-based observations for validation.
5.1 Cross-sensor consistency
5.1.1 Average and bias
Figure 10 shows the global monthly mean time series of (a) total column O3, (b) total column NO2, (c) tropospheric column NO2, (d) total column water vapour, (e) total column BrO, (f) total column HCHO, and (g) total column SO2 for GOME-2A, GOME-2B, and GOME-2C. The error bars represent the 1σ standard deviation of variation. All species except for SO2 show pronounced seasonal variation patterns. The seasonal patterns are related to the natural variability and the variation of the coverage area of the GOME-2 measurements.
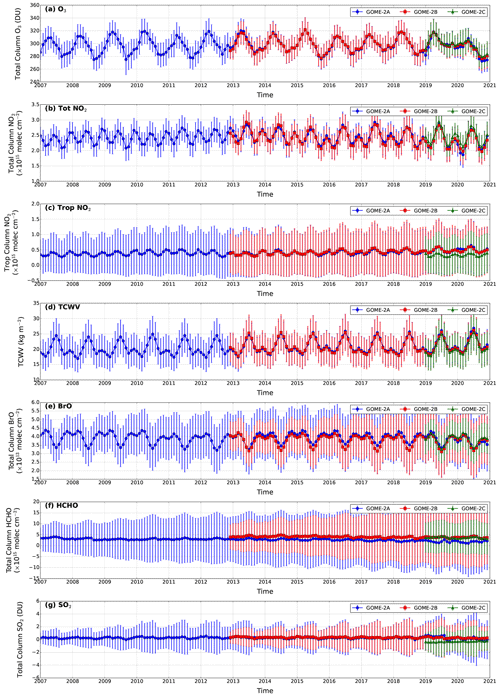
Figure 10Time series of global monthly mean (a) total column O3, (b) total column NO2, (c) tropospheric column NO2, (d) total column water vapour, (e) total column BrO, (f) total column HCHO, and (g) total column SO2 for GOME-2A (blue lines), GOME-2B (red lines), and GOME-2C (green lines). The error bars represent the 1σ standard deviation variation.
The global monthly mean total column O3 time series of GOME-2A, GOME-2B, and GOME-2C mostly overlap with each other, indicating the good agreement among the three sensors. However, GOME-2C reports a slightly higher (2–3 DU) value compared to GOME-2A and GOME-2B. This is likely related to the small difference in instrument characteristic, e.g. scan angle dependency and polarization sensitivity.
For total column NO2, observations from GOME-2A and GOME-2B show very good consistency, while GOME-2C data are about 1.2 × 1014 molec cm−2 higher than those of GOME-2A and GOME-2B. Tropospheric column NO2 from GOME-2A and GOME-2B is also in good agreement. However, GOME-2C observations are about 1.5 × 1014 molec cm−2 lower than GOME-2A and GOME-2B observations. The discrepancies in NO2 observations are likely related to the different processor versions (GDP 4.8 for GOME-2A and GOME-2B and GDP 4.9 for GOME-2C). The spectral-fitting band of NO2 is slightly different in different processor versions (see Sect. 2.2.2). A previous validation study shows that the NO2 slant columns retrieved from GOME-2C observations are slightly higher than those of GOME-2B (Pinardi et al., 2019), indicating the impact of the different spectral-fitting bands on the NO2 retrieval. In addition, the positive bias in the GOME-2C total column NO2 shows an impact on the tropospheric columns in the stratospheric and tropospheric separation process (Pinardi et al., 2019) and results in the discrepancies in the tropospheric columns.
Total column water vapour measurements from all three GOME-2 sensors also show very good consistency, with a bias smaller than 1 kg m−2.
For BrO observations, GOME-2B measurements show a negative bias of ∼ 1.0–1.5 × 1012 molec cm−2 compared to GOME-2A and GOME-2C. The discrepancies are partly related to the difference in the scanning swath width and the scan angle dependency (Merlaud et al., 2020). The impact of scan angle dependency on BrO measurements is more significant for GOME-2C compared to GOME-2B, which is likely linked to the polarization sensitivity of the GOME-2C instrument (Merlaud et al., 2020).
GOME-2A observations of total column HCHO are in general 1.5–1.9 × 1012 molec cm−2 lower than GOME-2B and GOME-2C measurements. Lower HCHO columns are observed by GOME-2A over the Amazon, central Africa, Southeast Asia, and Australia (see Fig. 5), thus resulting in slightly lower global averages. Similarly to BrO measurements, the scan angle dependency issue is also reported as significant for GOME-2C HCHO observations (Pinardi et al., 2020b). The scan angle dependency effect can also be seen in the BrO and HCHO daily level-3 product.
Total column SO2 observations from GOME-2C are in general 0.5 DU lower than GOME-2A and GOME-2B, resulting in a slightly negative global average. The higher global average of SO2 observed by GOME-2A and GOME-2B is related to the extreme values taken with a high solar zenith angle and thus low signal-to-noise ratio (see Figs. 4 and 5), while this effect is much less significant for GOME-2C. The overall bias and root mean square error among the GOME-2 sensors for each product are summarized in Table 2.
Table 2Bias and root mean square error of trace gas columns among the three GOME-2 sensors.
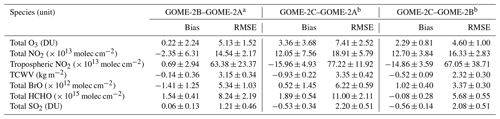
a For the period from 2013 to 2021. b For the period from 2019 to 2021.
Table 3Summary of the GOME-2 level-3 data comparison to ground-based measurements.

a Unit DU, b unit 1015 molec cm−2, c unit kg m−2, and d unit 1012 molec cm−2. e Regression is computed based on daily data, and f regression is computed based on monthly data. g Based on comparison at a single location. All biases are calculated based on daily data.
5.1.2 Zonal average
Each GOME-2 monthly averaged level-3 product derived from all three sensors is sorted by latitude and is plotted in Fig. 11.
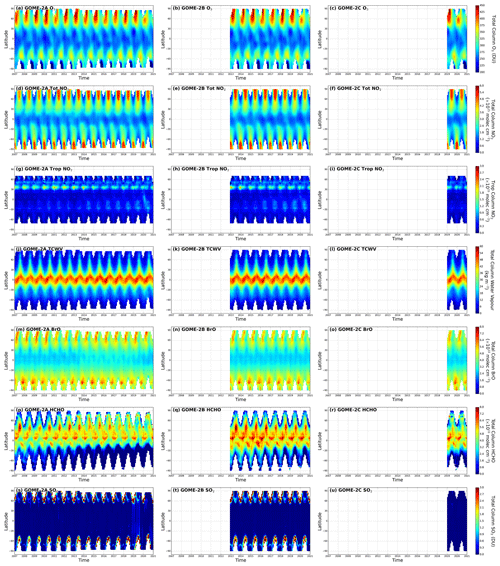
Figure 11Monthly zonal average of total column O3 (first row), total column NO2 (second row), tropospheric column NO2 (third row), total column water vapour (fourth row), total column BrO (fifth row), total column HCHO (sixth row), and total column SO2 (seventh row). Data from GOME-2A (first column from the left), GOME-2B (second column from the left), and GOME-2C (third column from the left) are shown.
All three GOME-2 sensors show consistent zonal and seasonal O3 patterns. Higher O3 columns are observed over high latitudes, and lower values are found over the tropics. Total column O3 over the Arctic shows a peak in February to March and a minimum in August to October, while Antarctica displays a reverted seasonal pattern.
Both total and tropospheric column NO2 from all three GOME-2 sensors shows good zonal and seasonal consistency. Elevated total column NO2 is observed in the polar regions during the warm months. This seasonal pattern is attributed to the stratospheric variation of NO2. Compared to total column NO2, tropospheric column NO2 shows a very different zonal and seasonal pattern. Tropospheric NO2 is mostly concentrated at the mid-latitudes of the Northern Hemisphere. This is because most of the population lives in this part of the world, and thus higher emissions occur at this latitude band. Tropospheric NO2 at mid-latitudes also shows a seasonal pattern with higher values over winter, which is related to higher energy consumption and a longer atmospheric lifetime of NO2 during the cold months. A significant increasing trend of tropospheric NO2 was observed by GOME-2A and GOME-2B over the sub-tropics and mid-latitudes of the Southern Hemisphere in recent years (see Fig. 11g and h). GOME-2C observed a much less significant enhancement of tropospheric NO2 in the Southern Hemisphere, which leads to lower global-average tropospheric NO2 measured by GOME-2C. This discrepancy is likely related to the difference in retrieval wavelength and the subsequent stratosphere and troposphere separation process.
Total column water vapour observations from all three GOME-2 sensors show consistent zonal and seasonal patterns, with higher values in the tropics and lower values at high latitudes. Total column water vapour is also higher during the warm months of the corresponding hemisphere.
All three GOME-2 sensors also show very similar zonal and seasonal patterns of total column BrO. However, GOME-2A total column BrO observations from 2014 to 2019 are slightly higher than those of GOME-2B at all latitude bands and result in a small bias of 1.41 × 1012 molec cm−2. However, when we look into the data from 2020 to 2021, the bias is smaller and results in a smaller bias of 0.52 × 1012 molec cm−2 with GOME-2C observations.
The total column HCHO from all three GOME-2 sensors shows higher values over the tropics and sub-tropics, while lower values appear at higher latitudes. Both GOME-2A and GOME-2B measurements show a significant decreasing trend of HCHO in the Southern Hemisphere. However, GOME-2A measurements are significantly lower than GOME-2B and GOME-2C, resulting in biases of −1.54 and −1.89 × 1015 molec cm−2 when compared to GOME-2B and GOME-2C observations. The discrepancy is related to the underestimation over HCHO-rich regions, e.g. the Amazon, Australia, Southeast Asia, China, and North America (see Fig. 5). This bias is probably related to the background correction process.
Total column SO2 observations from all three GOME-2 sensors show very low SO2 levels (very close to 0) around the globe, as expected. However, GOME-2A and GOME-2B measurements show significantly higher noise for measurement with a high solar zenith angle and result in a small overestimation under these extreme observation geometries, while this effect is much less significant for GOME-2C. Therefore, GOME-2C observations are in general about 0.5 DU lower than GOME-2A and GOME-2B. GOME-2A measurement is also about 0.5 DU higher in general compared to the years before and after.
5.2 Comparison to ground-based observations
In this section, all GOME-2 level-3 products are compared to the corresponding reference ground-based observations. We look into the scatter plot and histogram of the differences and sort the differences/biases by year, latitude band, or measurement site as a box plot and time series between GOME-2 and reference data sets to investigate the systematic bias/error.
5.2.1 Total column ozone
Daily and monthly GOME-2 level-3 total column ozone is compared to the co-located Brewer observations. Figure 12 shows the density scatter plots for the comparison of total column ozone between GOME-2 and ground-based Brewer observations. Comparisons of GOME-2A, GOME-2B, and GOME2-C data are shown in Fig. 12a, b, and c, respectively. Monthly data are also shown as black dots. Histograms of the differences between GOME-2 and Brewer observations are shown in Fig. 12d. Scatter plots show that GOME-2 monthly data are well in line with the daily data, and the agreement between GOME-2 and Brewer is in general very good, with a Pearson correlation coefficient (R) of ∼ 0.96 for all three GOME-2 sensors. The slopes of the total least-squares regression for the comparisons of all three instruments are very close to 1 (1.03 for GOME-2A, 1.01 for GOME-2B, and 0.99 for GOME-2C). The offsets of the total least-squares regression range between −5.1 and 4.3 DU. In general, the GOME-2 data sets show a small positive bias of 2.3 to 3.5 DU (0.72 %–1.07 %) compared to Brewer observations, with a standard deviation of 13.9 to 14.7 DU (4.03 %–4.48 %). The bias between all three GOME-2 sensors and the ground-based Brewer observations is below 1 %, which is within the uncertainty of Brewer measurements (Kerr et al., 1988) and fulfils the product requirements.
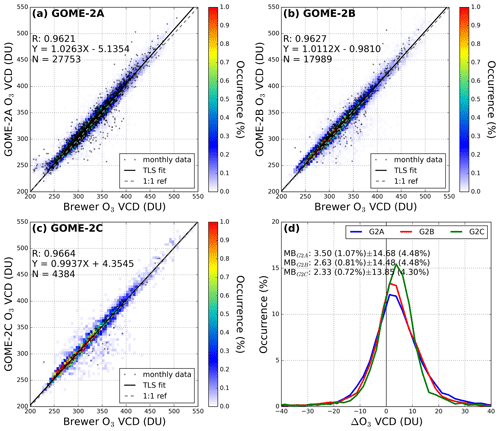
Figure 12Comparison of daily and monthly total column O3 measured by the ground-based Brewer instruments to (a) GOME-2A, (b) GOME-2B, and (c) GOME-2C. Histograms of the differences in total column O3 between GOME-2 and Brewer observations are shown in panel (d). Co-located daily and monthly averaged data are used in the comparison. Total least-squares regression is based on daily data.
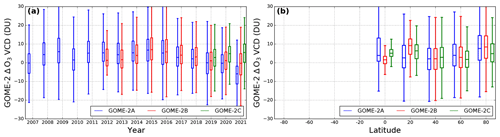
Figure 13Comparison of total column O3 between ground-based Brewer instruments and GOME-2 observations. Data are sorted by year in panel (a) and latitude band in panel (b).
Figure 13 shows box plots of the differences in total column ozone between the GOME-2 level-3 product and the co-located Brewer measurements. GOME-2 data are sorted by the measurement year (Fig. 13a) and latitude band (Fig. 13b). The box plot for the Southern Hemisphere is mostly empty due to an insufficient number of ground-based observations. The mean differences between GOME-2 and Brewer observations are within 5 DU for most of the years. However, we observed that there are years with a positive bias and some years with a negative bias. This is mostly related to the availability of ground-based data at different measurement sites, as some sites are biased high/low, and it will affect the statistic if they are not available for some years. On the other hand, the latitude-dependent analysis shows that GOME-2 observations are consistently higher than the ground-based Brewer measurements in the Northern Hemisphere and result in a positive bias of 2.3 to 3.5 DU on average. In addition, GOME-2C observations are about 2–3 DU higher than GOME-2A and GOME-2B, which is likely related to the instrumental issues which have been mentioned in Sect. 5.1.1.
5.2.2 Total column NO2
Daily and monthly GOME-2 level-3 total column NO2 is compared to the co-located ZSL-DOAS observations. Figure 14 shows the density scatter plots for the comparison of total column NO2 between GOME-2 and ground-based ZSL-DOAS observations. Comparisons of GOME-2A, GOME-2B, and GOME-2C data are shown in Fig. 14a, b, and c, respectively. Monthly data are also shown as black dots. Histograms of the differences between GOME-2 and ZSL-DOAS observations are shown in Fig. 14d. Scatter plots show that GOME-2 monthly data are well in line with the daily data. GOME-2 level-3 total column NO2 in general agrees well with ZSL-DOAS observations, with a Pearson correlation coefficient (R) of 0.85 to 0.88. However, GOME-2 observations are in general slightly lower than ZSL-DOAS observations. The slopes of the total least-squares fit for the comparisons of all three instruments vary from 0.84 to 0.88, with offsets ranging from 0.05 to 0.19 × 1015 molec cm−2. Overall, the GOME-2 level-3 total NO2 products are biased low by 0.24–0.29 × 1015 molec cm−2 (8 %–10 %) compared to ground-based ZSL-DOAS measurements. Considering that the uncertainty of satellite and ground-based measurements is about 10 %, the agreement between the two data sets is very satisfactory.
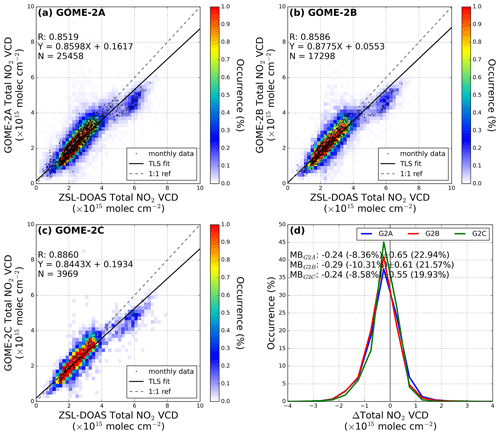
Figure 14Comparison of daily and monthly total column NO2 measured by the ground-based ZSL-DOAS to (a) GOME-2A, (b) GOME-2B, and (c) GOME-2C. Histograms of the difference in total column NO2 between GOME-2 and ZSL-DOAS observations are shown in panel (d). Co-located daily and monthly averaged data are used in the comparison. Total least-squares regression is based on daily data.
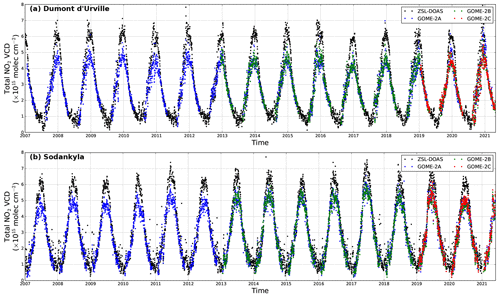
Figure 15Time series of total column NO2 measured by GOME-2A (blue), GOME-2B (green), GOME-2C (red), and ZSL-DOAS (black). Observations over (a) Dumont d'Urville, Antarctica, and (b) Sodankylä, Finland, are shown.
The scatter plots for all three instruments show a two-cluster characteristic. The major cluster of total column NO2 below 4 × 1015 molec cm−2 shows very good agreement between GOME-2 and ZSL-DOAS observations. The minor cluster at 5–6 × 1015 molec cm−2 shows significant underestimation of the NO2 column by 0.5–1.0 × 1015 molec cm−2, which is related to the measurement over the polar regions. Figure 15 shows the time series of total column NO2 measured at Dumont d'Urville, Antarctica, and Sodankylä, Finland. We observed that the total column NO2 measured by GOME-2 is significantly lower than the ground-based ZSL-DOAS observations during summer months. This is because of the multiple overpasses over the polar regions during summertime. Therefore, GOME-2 level-3 data represent the real “daily average”, while ZSL-DOAS only captures the morning values. Due to the diurnal variation of NO2, it is expected that ZSL-DOAS measurements in the morning will be higher than the daily averages. If we do not consider these two stations in the analysis, the minor cluster in the scatter plots would be removed. In addition, the underestimation would reduce to 0.13–0.21 × 1015 molec cm−2 (5 %–7 %).
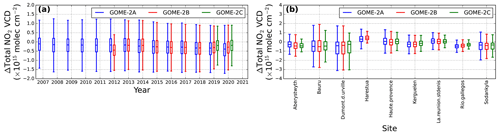
Figure 16Comparison of total column NO2 between ground-based ZSL-DOAS and GOME-2 observations. Data are sorted by year in panel (a) and measurement site in panel (b).
Figure 16 shows box plots of the differences in total column NO2 between the GOME-2 level-3 product and co-located ZSL-DOAS measurements. Data are sorted by the measurement year (Fig. 16a) and measurement site (Fig. 16b). The mean differences between GOME-2 and ZSL-DOAS observations are within 0.3 × 1015 molec cm−2 for most of the years, and this bias does not show significant temporal variation. Box plots for each measurement site show significant negative bias for some sites, i.e. Dumont d'Urville and Sodankylä. The reason for the negative bias has been explained above.
5.2.3 Tropospheric column NO2
Daily and monthly GOME-2 level-3 tropospheric column NO2 is compared to the co-located MAX-DOAS observations. Figure 17 shows the density scatter plots for the comparison of tropospheric column NO2 between GOME-2 and ground-based MAX-DOAS observations. Comparisons of GOME-2A, GOME-2B, and GOME-2C data are shown in Fig. 17a, b, and c, respectively. Monthly data are also shown as black dots. Histograms of the differences between GOME-2 and MAX-DOAS observations are shown in Fig. 17d. GOME-2 monthly tropospheric NO2 data are consistent with the daily data, and daily data show satisfactory correlation with ground-based MAX-DOAS observations, with a Pearson correlation coefficient (R) in the range of 0.68 to 0.75. However, GOME-2 tropospheric column NO2 is in general ∼ 30 % lower than MAX-DOAS observations. The slopes of the total least-squares fit for the comparisons of all three instruments vary from 0.61 to 0.74, with offsets ranging from −1.03 to 0.18 × 1015 molec cm−2. GOME-2 level-3 tropospheric NO2 products on average show a negative bias of 3.38–4.14 × 1015 molec cm−2 (37 %–39 %). The underestimation is mainly related to the a priori assigned too-low NO2 concentration at the lower troposphere and a spatial averaging effect over large satellite pixels. A previous study shows that using a better a priori vertical profile in GOME-2 retrieval reduces the underestimation of GOME-2 measurement by 15 %–20 % (Liu et al., 2019). The spatial averaging effect has also been estimated to result in an underestimation of 15 %–25 % in tropospheric column NO2 over pollution hotspots (Chen et al., 2009; Ma et al., 2013; Chan et al., 2020b; Pinardi et al., 2020a). Considering the sensitivity difference between satellite and ground-based MAX-DOAS measurements and the spatial averaging effect of large satellite footprints, the agreement between the two data sets is very satisfactory.
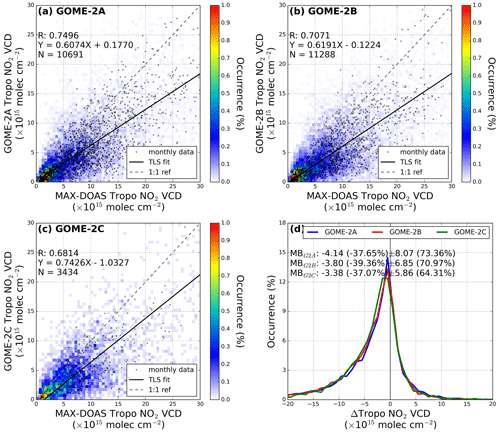
Figure 17Comparison of daily and monthly tropospheric column NO2 measured by the ground-based MAX-DOAS to (a) GOME-2A, (b) GOME-2B, and (c) GOME-2C. Histograms of the difference in tropospheric column NO2 between GOME-2 and MAX-DOAS are shown in panel (d). Co-located daily and monthly averaged data are used in the comparison. Total least-squares regression is based on daily data.
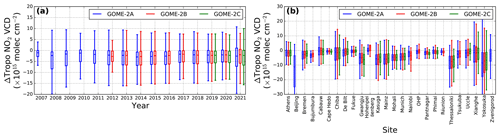
Figure 18Comparison of tropospheric column NO2 between ground-based MAX-DOAS and GOME-2 observations. Data are sorted by year in panel (a) and measurement site in panel (b).
Figure 18 shows box plots of the differences in tropospheric column NO2 between the GOME-2 level-3 product and co-located MAX-DOAS measurements. Data are sorted by the measurement year (Fig. 18a) and measurement site (Fig. 18b). The mean differences between GOME-2 and MAX-DOAS observations are ∼ 3 × 1015 molec cm−2 for most of the years, and this bias does not show significant temporal variation. Box plots for each measurement site show a significant negative bias for some polluted sites, i.e. Beijing (China), Thessaloniki (Greece), and Yokosuka (Japan). The reason for the negative bias has been explained above. The underestimation is significantly reduced over rural areas, e.g. Cape Hedo (Japan), Cabauw (Netherlands), and Phimai (Thailand). These results are in line with the level-2 data that GOME-2 in general underestimates tropospheric column NO2 over polluted areas.
5.2.4 Total column water vapour
Daily GOME-2 level-3 total column water vapour is compared to the co-located sun-photometer observations. Figure 19 shows the density scatter plots for the comparison of the total column water vapour between GOME-2 and ground-based sun-photometer observations. Comparisons of GOME-2A, GOME-2B, and GOME-2C data are shown in Fig. 14a, b, and c, respectively. Histograms of the differences between GOME-2 and MAX-DOAS observations are shown in Fig. 19d. Similar plots for monthly comparison are shown in Fig. 20. GOME-2 monthly total column water vapour data are in general consistent with the daily data. GOME-2 daily observations are in good agreement with sun-photometer observations, with a Pearson correlation coefficient (R) of ∼ 0.92 for all three instruments. Monthly comparison shows a higher correlation coefficient (R) of ∼ 0.94. The slopes of least-squares regression lines of daily comparison for all three GOME-2 sensors are very close to 1, while a small offset of 1.2–1.6 kg m−3 is observed. Monthly comparison shows a similar characteristic, with the slope of regression close to 1 and an offset of 1.1–1.6 kg m−3. GOME-2 level-3 total column water vapour in general shows a positive bias of 1.0–1.7 kg m−3 (6 %–9 %). Considering that sun-photometer measurements in general underestimate total column water vapour by 6 % (Pérez-Ramírez et al., 2014), the positive bias of 1.0–1.7 kg m−3 is reasonable.
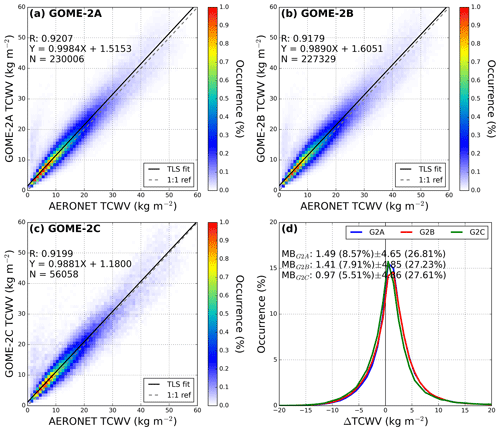
Figure 19Comparison of daily total column water vapour measured by the sun photometer to (a) GOME-2A, (b) GOME-2B, and (c) GOME-2C. Histograms of the difference between GOME-2 and the sun-photometer are shown in panel (d). Co-located daily averaged data are used in the comparison.
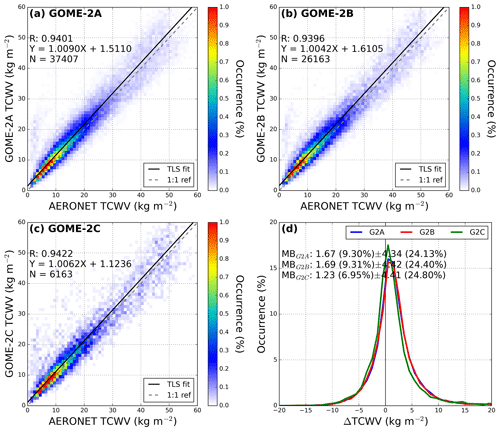
Figure 20Comparison of monthly total column water vapour measured by the sun photometer to (a) GOME-2A, (b) GOME-2B, and (c) GOME-2C. Histograms of the difference between GOME-2 and the sun photometer are shown in panel (d). Co-located monthly averaged data are used in the comparison.
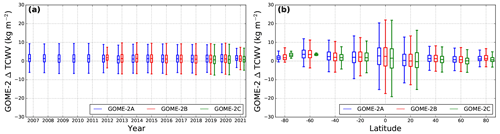
Figure 21Comparison of TCWV between the sun-photometer and GOME-2 observations. Data are sorted by year in panel (a) and latitude in panel (b).
Figure 21 shows box plots of the statistic of the differences in total column water vapour between the GOME-2 level-3 product and co-located sun-photometer measurements. Data are sorted by the measurement year (Fig. 21a) and latitude band (Fig. 21b). The bias between GOME-2 and sun-photometer observations is consistently at the level of 1–2 kg m−3 throughout the entire measurement period. The latitude dependency analysis shows larger variations in the tropics, while the variations are much smaller at higher latitudes. The absolute differences for measurements over the polar regions are slightly higher. This is mainly due to multiple overpasses over the polar regions during summer months and the resulting temporal mismatch.
5.2.5 Total column BrO
Co-located daily and monthly GOME-2 level-3 total column BrO is compared to ZSL-DOAS observations at Harestua, Norway. Figure 22 shows the density scatter plots for the comparison of total column BrO between GOME-2 and ZSL-DOAS observations. Comparisons of GOME-2A, GOME-2B, and GOME-2C data are shown in Fig. 22a, b, and c, respectively. Monthly data are also shown as black dots. Histograms of the differences between GOME-2 and ZSL-DOAS observations are shown in Fig. 22d. We can see from the scatter plots that both GOME-2 and ZSL-DOAS BrO measurements are quite noisy: this is mainly due to the low absorption of BrO and thus the low signal-to-noise ratio. Both daily and monthly GOME-2 level-3 data show quite good agreement with the ZSL-DOAS observations, with a Pearson correlation coefficient (R) ranging from 0.64 to 0.74. In general, GOME-2 observations underestimate the BrO column by 7.0–10.2 × 1012 molec cm−2 (16 %–26 %). Please note that the comparison only covered one site in Norway and that the numbers might vary in different parts of the globe.
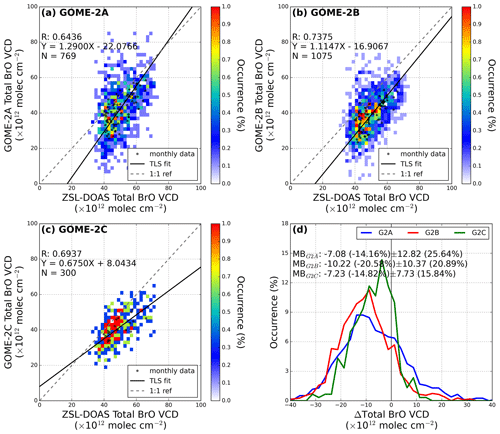
Figure 22Comparison of daily and monthly total column BrO measured by the ground-based ZSL-DOAS at Harestua, Norway, to (a) GOME-2A, (b) GOME-2B, and (c) GOME-2C. Histograms of the difference in total column BrO between GOME-2 and MAX-DOAS observations are shown in panel (d). Co-located daily and monthly averaged data are used in the comparison. Total least-squares regression is based on monthly data.
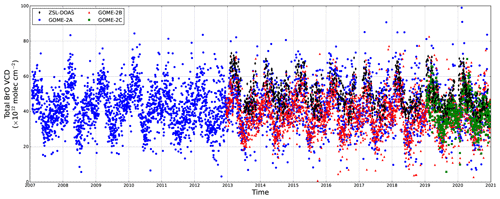
Figure 23Time series of total column BrO measured by GOME-2A (blue), GOME-2B (green), GOME-2C (red), and ZSL-DOAS (black) at Harestua, Norway.
Figure 23 shows the time series of total column BrO measured at Harestua, Norway. Measurements from all three GOME-2 sensors show a similar temporal variation trend with a higher BrO level during winter/early spring and a lower one in summer, which agrees with the ZSL-DOAS observations. However, GOME-2 observations are about 5–10 × 1012 molec cm−2 lower than the ZSL-DOAS data. This underestimation has also been given in the level-2 validation report (Theys et al., 2015a). Considering that the ZSL-DOAS data have been empirically corrected for the offset caused by the instrumental effect, the agreement between GOME-2 and ZSL-DOAS is deemed very satisfactory.
5.2.6 Total column HCHO
Daily and monthly GOME-2 level-3 total column HCHO is compared to the co-located MAX-DOAS observations. Figure 24 shows the density scatter plots for the comparison of total column HCHO between GOME-2 and ground-based MAX-DOAS observations. Comparisons of GOME-2A, GOME-2B, and GOME-2C data are shown in Fig. 24a, b, and c, respectively. Monthly data are also shown as black dots. Histograms of the differences between GOME-2 and MAX-DOAS observations are shown in Fig. 24d. We can see from the scatter plots that both daily GOME-2 and MAX-DOAS HCHO measurements are quite noisy: this is mainly due to the low absorption of HCHO and thus the low signal-to-noise ratio. However, when we look at the monthly averages, the GOME-2 level-3 data in general agree with the ground-based MAX-DOAS observations. The Pearson correlation coefficient (R) between monthly GOME-2 and MAX-DOAS data ranges from 0.68 to 0.78. However, GOME-2 observations in general underestimate total column HCHO by 20 %–25 %. The slope of the total least-squares regression line for the comparisons of all three instruments varies from 0.74 to 0.81, with the offset ranging from −1.61 to −1.14 × 1015 molec cm−2. GOME-2 level-3 total HCHO products on average show a small bias of −0.75 to 1.92 × 1015 molec cm−2 (−7 %–17 %), with a standard deviation of 8.8 to 11.4 × 1015 molec cm−2. The underestimation is partly related to the a priori profile used in GOME-2 retrieval and the difference in sensitivity between satellite and ground-based observations. The underestimation of the level-3 product is in line with the level-2 product. Previous studies show that the negative bias is significantly improved when the MAX-DOAS profiles are used for satellite column retrieval (De Smedt et al., 2015a, b). As the daily level-3 HCHO data are a bit noisy, it would be useful to further average the data spatially to reduce noise when looking into day-to-day variability or to use the temporally averaged product, i.e. the monthly product.
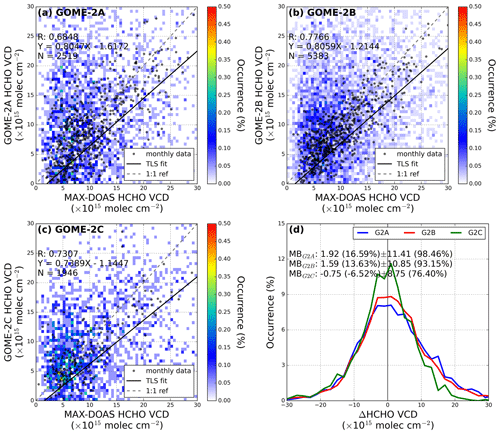
Figure 24Comparison of daily and monthly total column HCHO measured by the ground-based MAX-DOAS to (a) GOME-2A, (b) GOME-2B, and (c) GOME-2C. Histograms of the difference in total column HCHO between GOME-2 and MAX-DOAS are shown in panel (d). Co-located daily and monthly averaged data are used in the comparison. Total least-squares regression is based on monthly data.
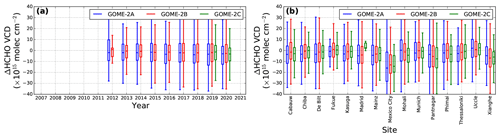
Figure 25Comparison of total column HCHO between ground-based MAX-DOAS and GOME-2 observations. Data are sorted by year in panel (a) and measurement site in panel (b).
Figure 25 shows box plots of the statistic of the differences in total column HCHO between the GOME-2 level-3 product and co-located MAX-DOAS measurements. Data are sorted by the measurement year (Fig. 25a) and measurement site (Fig. 25b). The mean differences between GOME-2 and MAX-DOAS observations are 1–2 × 1015 molec cm−2 for most of the years and do not show significant temporal variation. Box plots for each measurement site show that GOME-2 significantly underestimated the HCHO column over polluted areas, i.e. Mexico City (Mexico) and Xianghe (China). The underestimation is related to the difference in sensitivity, and this effect has been reported in previous level-2 validation studies for GOME-2 (De Smedt et al., 2015b; Pinardi et al., 2020b) as well as for other satellites (Chan et al., 2020b; De Smedt et al., 2021).
5.2.7 Total column SO2
The validation of volcanic SO2 is very difficult due to the differences in volcanic plume injection height for each eruption. Therefore, it is often done in a case study base, i.e. in previous validation reports (Theys et al., 2015b). In this study, we focus on the long-term statistic and stability and therefore look mainly into areas with significant SO2 sources. Co-located daily and monthly GOME-2 level-3 total column SO2 is compared to Pandora observations at Mexico City. Figure 26 shows the scatter plots for the comparison of total column SO2 between GOME-2 and Pandora observations. Comparisons of GOME-2A, GOME-2B, and GOME-2C data are shown in Fig. 26a, b, and c, respectively. Monthly data are also shown as black dots. Histograms of the differences between GOME-2 and Pandora observations are shown in Fig. 26d. Due to the low absorption and abundance of SO2, both GOME-2 and Pandora measurements are quite noisy. The histogram shows that GOME-2 underestimated total column SO2 by 0.25 to 0.48 DU (47 %–64 %). This underestimation is likely related to the a priori profiles used in AMF calculations. The product assumes an SO2 plume at 6 km. If an a priori SO2 located in the lower troposphere is used in the AMF calculations, it would increase the retrieved SO2 columns and reduce the underestimation. Please be aware that this is just a comparison over one site in Mexico and that the numbers might vary over different parts of the globe, especially due to the low concentration of SO2 in general.
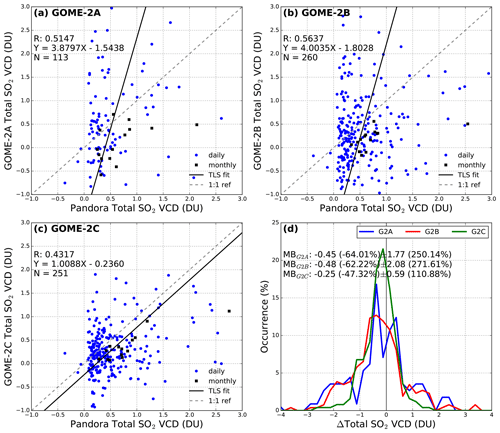
Figure 26Comparison of daily and monthly total column SO2 measured by the Pandora instrument in Mexico City to (a) GOME-2A, (b) GOME-2B, and (c) GOME-2C. Histograms of the difference in total column SO2 between GOME-2 and Pandora observations are shown in panel (d). Co-located daily and monthly averaged data are used in the comparison. Total least-squares regression is based on daily data.
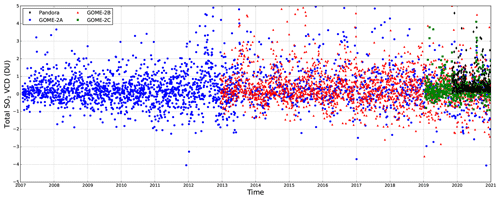
Figure 27Time series of total column SO2 measured by GOME-2A (blue), GOME-2B (green), GOME-2C (red), and Pandora (black) at Mexico City.
Figure 27 shows the time series of total column SO2 measured at Mexico City. All three GOME-2 sensors show similar SO2 columns. The overall averages are very close to 0 and do not show any significant trend. Due to the low abundance of SO2 and a low signal-to-noise ratio, there are a considerable number of negative values. On the other hand, due to the better signal-to-noise ratio, only a very few negative values were measured by Pandora. Considering the measurement noise of GOME-2, the agreement between the GOME-2 and Pandora data sets is reasonable.
The GOME-2 level-3 products described in this paper are available to the public via EUMETSAT’s Satellite Application Facility on Atmospheric Composition Monitoring (https://acsaf.org, last access: 15 March 2023). DOIs: daily products https://doi.org/10.15770/EUM_SAF_AC_0048 (AC SAF, 2023a); monthly products https://doi.org/10.15770/EUM_SAF_AC_0049 (AC SAF, 2023b).
We presented the new GOME-2 daily and monthly level-3 products, which include total column O3, total and tropospheric column NO2, total column water vapour, total column BrO, total column HCHO, and total column SO2. Compared to satellite observations from OMI and TROPOMI, which measure at noon or in the early afternoon, GOME-2 measurements in the morning provide addition information on the temporal/diurnal variation of these atmospheric trace gases. This additional information would be very useful for the studies of temporal/diurnal variations and photochemistry in the atmosphere. Details of the algorithm for level-2 to level-3 processing as well as the selection of an appropriate spatial resolution for the level-3 products are shown. Verification and validation of each GOME-2 level-3 product are achieved by investigating the consistency among the three GOME-2 sensors and comparison to ground-based reference measurements.
The overlapping area-weighting method is used for level-2 to level-3 processing. The spatial resolution of the GOME-2 level-3 products is selected based on the sensitivity study. The consistency among the three GOME-2 sensors is investigated through time series of global averages, zonal averages, and biases. Finally, the accuracy of the level-3 products is validated through the comparison to ground-based observations.
For the selection of an appropriate spatial resolution of the GOME-2 level-3 data, we have re-sampled GOME-2 level-2 data onto various spatial resolutions, i.e. 0.1∘ × 0.1∘, 0.25∘ × 0.25∘, and 0.5∘ × 0.5∘, and compared them to the original level-2 data. All the data sets show very similar spatial structures, and the absolute values are consistent with the level-2 products. As expected, level-3 data sampled at a higher spatial resolution (i.e. 0.1∘ × 0.1∘) better preserved the original GOME-2 instrument footprint. However, a lower resolution of 0.25∘ × 0.25∘ also preserves the spatial pattern of quickly varying tropospheric species, i.e. NO2, reasonably well, while a rather strong smoothing/averaging effect is observed from data gridded with a lower spatial resolution (i.e. 0.5∘ × 0.5∘). Therefore, we concluded that the spatial resolution of 0.25∘ × 0.25∘ is sufficient and appropriate for GOME-2 level-3 products.
The consistency of the level-3 product among the three GOME-2 sensors is investigated. Global-average time series plots show that total column ozone and water vapour products from all the GOME-2 sensors are consistent, with only a small bias of up to 3 DU (<1 %) for ozone and 0.9 kg m−2 (<5 %) for water vapour. For total and tropospheric column NO2 products, GOME-2A and GOME-2B measurements are consistent with each other, while GOME-2C data show significant discrepancy compared to the other two sensors. This is mainly due to the differences in processor versions (GDP 4.8 for GOME-2A and GOME-2B and GDP 4.9 for GOME-2C) and the spectral-fitting band of NO2. BrO observations from GOME-2B in general show a negative bias of 1.0–1.5 × 1012 molec cm−2 compared to GOME-2A and GOME-2C. GOME-2A HCHO columns are 1.5–1.9 × 1015 molec cm−2 lower than GOME-2B and GOME-2C measurements. This is due to the underestimation over the Amazon, Southeast Asia, and Australia. Total column SO2 observations from GOME-2C are on average 0.5 DU lower than GOME-2A and GOME-2B, resulting in a slightly negative global average. A slightly higher global average of SO2 measured by GOME-2A and GOME-2B is related to the high values taken under extreme viewing geometry, i.e. a high solar zenith angle. Please be reminded that BrO and SO2 products are only validated against measurements at specific locations, which might need to be further validated depending on the user applications.
For comparison of co-located GOME-2 level-3 data to ground-based observations, we found in general good agreement, and the results are consistent with previous level-2 validation studies. We summarize the statistical result in Table 3.
From the results above, we conclude that the daily and monthly GOME-2 level-3 products of total column O3, total and tropospheric column NO2, total column water vapour, total column BrO, total column HCHO, and total column SO2 for GOME-2A, GOME-2B, and GOME-2C are consistent and fulfil the product requirements.
KLC conceptualized the paper, devised the methodology, developed the algorithm, and validated the data sets. KLC and PV managed the project. KLC, KPH, RL, PH, and DL provided support on GOME-2 level-2 data. KLC, GP, MVR, FH, TW, VK, AB, AP, HI, YK, HT, YC, KP, JC, AC, UF, AR, JM, NB, RH, CRC, and MW provided ground-based reference data. KLC prepared the manuscript with contributions from all the co-authors.
The contact author has declared that none of the authors has any competing interests.
Publisher’s note: Copernicus Publications remains neutral with regard to jurisdictional claims in published maps and institutional affiliations.
We are grateful to EUMETSAT and AC SAF for the production of the GOME-2 level-2 and level-3 data. We thank WOUDC, NDACC, NIDFORVAL, and AERONET for harmonizing and managing the ground-based data sets used in this study. Work by Hitoshi Irie on ground-based MAX-DOAS observation data was supported by the Environment Research and Technology Development Fund (JPMEERF20215005) of the Environmental Restoration and Conservation Agency of Japan, JSPS KAKENHI (grant nos. JP20H04320, JP21K12227, JP22H03727, and JP22H05004), the JAXA 3rd Research Announcement on the Earth Observations (grant no. 19RT000351), and the Virtual Laboratory (VL) project of the Ministry of Education, Culture, Sports, Science and Technology (MEXT), Japan. The scientific results and conclusions, as well as any views or opinions expressed herein, are those of the author and do not necessarily reflect those of the NOAA, the Department of Commerce, or other data providers.
This research has been supported by the European Organization for the Exploitation of Meteorological Satellites (EUMETSAT) in the framework of the Satellite Application Facility on Atmospheric Composition Monitoring (AC SAF) Continuous Development and Operations Phase 3 (CDOP3) and corresponding Associated Scientist Project (agreement no. SAF/O3M/FMI/MGT/AGR/004).
This paper was edited by Jing Wei and reviewed by three anonymous referees.
AC SAF: Offline Level 3 GOME2 Daily Trace Gas columns – Metop, EUMETSAT SAF on Atmospheric Composition Monitoring, EUMETSAT [data set], https://doi.org/10.15770/EUM_SAF_AC_0048, 2023a. a, b
AC SAF: Offline L3 GOME2 Monthly Trace Gas columns – Metop, EUMETSAT SAF on Atmospheric Composition Monitoring, EUMETSAT [data set], https://doi.org/10.15770/EUM_SAF_AC_0049, 2023b. a, b
Alexandrov, M. D., Schmid, B., Turner, D. D., Cairns, B., Oinas, V., Lacis, A. A., Gutman, S. I., Westwater, E. R., Smirnov, A., and Eilers, J.: Columnar water vapor retrievals from multifilter rotating shadowband radiometer data, J. Geophys. Res.-Atmos., 114, D02306, https://doi.org/10.1029/2008JD010543, 2009. a, b
Anderson, G. P., Clough, S. A., Kneizys, F., Chetwynd, J. H., and Shettle, E. P.: AFGL atmospheric constituent profiles (0.120 km), Tech. Rep., Air Force Geophysics Lab Hanscom AFB MA, 1986. a
Antón, M., Loyola, D., López, M., Vilaplana, J. M., Bañón, M., Zimmer, W., and Serrano, A.: Comparison of GOME-2/MetOp total ozone data with Brewer spectroradiometer data over the Iberian Peninsula, Ann. Geophys., 27, 1377–1386, https://doi.org/10.5194/angeo-27-1377-2009, 2009. a
Balis, D., Kroon, M., Koukouli, M. E., Brinksma, E. J., Labow, G., Veefkind, J. P., and McPeters, R. D.: Validation of Ozone Monitoring Instrument total ozone column measurements using Brewer and Dobson spectrophotometer ground-based observations, J. Geophys. Res.-Atmos., 112, D24S46, https://doi.org/10.1029/2007JD008796, 2007a. a
Balis, D., Lambert, J.-C., Van Roozendael, M., Spurr, R., Loyola, D., Livschitz, Y., Valks, P., Amiridis, V., Gerard, P., Granville, J., and Zehner, C.: Ten years of GOME/ERS2 total ozone data – The new GOME data processor (GDP) version 4: 2. Ground-based validation and comparisons with TOMS V7/V8, J. Geophys. Res.-Atmos., 112, D07307, https://doi.org/10.1029/2005JD006376, 2007b. a
Bennouna, Y. S., Torres, B., Cachorro, V. E., Ortiz de Galisteo, J. P., and Toledano, C.: The evaluation of the integrated water vapour annual cycle over the Iberian Peninsula from EOS-MODIS against different ground-based techniques, Q. J. Roy. Meteor. Soc., 139, 1935–1956, https://doi.org/10.1002/qj.2080, 2013. a
Bovensmann, H., Burrows, J. P., Buchwitz, M., Frerick, J., Noël, S., Rozanov, V. V., Chance, K. V., and Goede, A. P. H.: SCIAMACHY: Mission Objectives and Measurement Modes, J. Atmos. Sci., 56, 127–150, https://doi.org/10.1175/1520-0469(1999)056<0127:SMOAMM>2.0.CO;2, 1999. a
Brinksma, E. J., Pinardi, G., Volten, H., Braak, R., Richter, A., Schönhardt, A., van Roozendael, M., Fayt, C., Hermans, C., Dirksen, R. J., Vlemmix, T., Berkhout, A. J. C., Swart, D. P. J., Oetjen, H., Wittrock, F., Wagner, T., Ibrahim, O. W., de Leeuw, G., Moerman, M., Curier, R. L., Celarier, E. A., Cede, A., Knap, W. H., Veefkind, J. P., Eskes, H. J., Allaart, M., Rothe, R., Piters, A. J. M., and Levelt, P. F.: The 2005 and 2006 DANDELIONS NO2 and aerosol intercomparison campaigns, J. Geophys. Res.-Atmos., 113, D16S46, https://doi.org/10.1029/2007JD008808, 2008. a
Bruns, M., Bovensmann, H., Richter, A., and Burrows, J. P.: A Stratospheric BrO Climatology for the GOME-2 instruments, O3M-SAF Visiting Scientist Report, IUP University of Bremen, https://atmos.eoc.dlr.de/app/docs/Bruns2003.pdf (last access: 15 March 2023) 2003. a
Burrows, J. P., Weber, M., Buchwitz, M., Rozanov, V., Ladstätter-Weißenmayer, A., Richter, A., DeBeek, R., Hoogen, R., Bramstedt, K., Eichmann, K.-U., Eisinger, M., and Perner, D.: The global ozone monitoring experiment (GOME): Mission concept and first scientific results, J. Atmos. Sci., 56, 151–175, https://doi.org/10.1175/1520-0469(1999)056<0151:TGOMEG>2.0.CO;2, 1999. a
Callies, J., Corpaccioli, E., Eisinger, M., Hahne, A., and Lefebvre, A.: GOME-2-Metop's second-generation sensor for operational ozone monitoring, ESA Bulletin, 102, 28–36, 2000. a, b
Celarier, E. A., Brinksma, E. J., Gleason, J. F., Veefkind, J. P., Cede, A., Herman, J. R., Ionov, D., Goutail, F., Pommereau, J.-P., Lambert, J.-C., van Roozendael, M., Pinardi, G., Wittrock, F., Schönhardt, A., Richter, A., Ibrahim, O. W., Wagner, T., Bojkov, B., Mount, G., Spinei, E., Chen, C. M., Pongetti, T. J., Sander, S. P., Bucsela, E. J., Wenig, M. O., Swart, D. P. J., Volten, H., Kroon, M., and Levelt, P. F.: Validation of Ozone Monitoring Instrument nitrogen dioxide columns, J. Geophys. Res.-Atmos., 113, D15S15, https://doi.org/10.1029/2007JD008908, 2008. a, b
Chan, K. L., Pöhler, D., Kuhlmann, G., Hartl, A., Platt, U., and Wenig, M. O.: NO2 measurements in Hong Kong using LED based long path differential optical absorption spectroscopy, Atmos. Meas. Tech., 5, 901–912, https://doi.org/10.5194/amt-5-901-2012, 2012. a
Chan, K. L., Hartl, A., Lam, Y. F., Xie, P. H., Liu, W. Q., Cheung, H. M., Lampel, J., Pöhler, D., Li, A., Xu, J., Zhou, H. J., Ning, Z., and Wenig, M.: Observations of tropospheric NO2 using ground based MAX-DOAS and OMI measurements during the Shanghai World Expo 2010, Atmos. Environ., 119, 45–58, https://doi.org/10.1016/j.atmosenv.2015.08.041, 2015. a
Chan, K. L., Wiegner, M., Wenig, M., and Pöhler, D.: Observations of tropospheric aerosols and NO2 in Hong Kong over 5 years using ground based MAX-DOAS, Sci. Total Environ., 619-620, 1545–1556, https://doi.org/10.1016/j.scitotenv.2017.10.153, 2018. a
Chan, K. L., Wang, Z., Ding, A., Heue, K.-P., Shen, Y., Wang, J., Zhang, F., Shi, Y., Hao, N., and Wenig, M.: MAX-DOAS measurements of tropospheric NO2 and HCHO in Nanjing and a comparison to ozone monitoring instrument observations, Atmos. Chem. Phys., 19, 10051–10071, https://doi.org/10.5194/acp-19-10051-2019, 2019. a, b
Chan, K. L., Valks, P., Slijkhuis, S., Köhler, C., and Loyola, D.: Total column water vapor retrieval for Global Ozone Monitoring Experience-2 (GOME-2) visible blue observations, Atmos. Meas. Tech., 13, 4169–4193, https://doi.org/10.5194/amt-13-4169-2020, 2020a. a
Chan, K. L., Wiegner, M., van Geffen, J., De Smedt, I., Alberti, C., Cheng, Z., Ye, S., and Wenig, M.: MAX-DOAS measurements of tropospheric NO2 and HCHO in Munich and the comparison to OMI and TROPOMI satellite observations, Atmos. Meas. Tech., 13, 4499–4520, https://doi.org/10.5194/amt-13-4499-2020, 2020b. a, b, c, d
Chen, D., Zhou, B., Beirle, S., Chen, L. M., and Wagner, T.: Tropospheric NO2 column densities deduced from zenith-sky DOAS measurements in Shanghai, China, and their application to satellite validation, Atmos. Chem. Phys., 9, 3641–3662, https://doi.org/10.5194/acp-9-3641-2009, 2009. a
Chipperfield, M. P.: Multiannual simulations with a three-dimensional chemical transport model, J. Geophys. Res.-Atmos., 104, 1781–1805, https://doi.org/10.1029/98JD02597, 1999. a
Clough, S. A. and Iacono, M. J.: Line-by-line calculation of atmospheric fluxes and cooling rates: 2. Application to carbon dioxide, ozone, methane, nitrous oxide and the halocarbons, J. Geophys. Res.-Atmos., 100, 16519–16535, https://doi.org/10.1029/95JD01386, 1995. a
Compernolle, S., Verhoelst, T., Pinardi, G., Granville, J., Hubert, D., Keppens, A., Niemeijer, S., Rino, B., Bais, A., Beirle, S., Boersma, F., Burrows, J. P., De Smedt, I., Eskes, H., Goutail, F., Hendrick, F., Lorente, A., Pazmino, A., Piters, A., Peters, E., Pommereau, J.-P., Remmers, J., Richter, A., van Geffen, J., Van Roozendael, M., Wagner, T., and Lambert, J.-C.: Validation of Aura-OMI QA4ECV NO2 climate data records with ground-based DOAS networks: the role of measurement and comparison uncertainties, Atmos. Chem. Phys., 20, 8017–8045, https://doi.org/10.5194/acp-20-8017-2020, 2020. a
De Smedt, I., Van Roozendael, M., Stavrakou, T., Müller, J.-F., Lerot, C., Theys, N., Valks, P., Hao, N., and van der A, R.: Improved retrieval of global tropospheric formaldehyde columns from GOME-2/MetOp-A addressing noise reduction and instrumental degradation issues, Atmos. Meas. Tech., 5, 2933–2949, https://doi.org/10.5194/amt-5-2933-2012, 2012. a, b
De Smedt, I., Pinardi, G., Yu, H., Hendrick, F., Gielen, C., Hao, N., Begoin, M., and Valks, P.: Validation report of GOME-2 NRT, offline and reprocessed GDP 4.8 HCHO column data for MetOp-A and B, https://acsaf.org/docs/vr/Validation_Report_NTO_OTO_DR_HCHO_GDP48_Oct_2015.pdf (last access: 15 March 2023), 2015a. a
De Smedt, I., Stavrakou, T., Hendrick, F., Danckaert, T., Vlemmix, T., Pinardi, G., Theys, N., Lerot, C., Gielen, C., Vigouroux, C., Hermans, C., Fayt, C., Veefkind, P., Müller, J.-F., and Van Roozendael, M.: Diurnal, seasonal and long-term variations of global formaldehyde columns inferred from combined OMI and GOME-2 observations, Atmos. Chem. Phys., 15, 12519–12545, https://doi.org/10.5194/acp-15-12519-2015, 2015b. a, b, c
De Smedt, I., Pinardi, G., Vigouroux, C., Compernolle, S., Bais, A., Benavent, N., Boersma, F., Chan, K.-L., Donner, S., Eichmann, K.-U., Hedelt, P., Hendrick, F., Irie, H., Kumar, V., Lambert, J.-C., Langerock, B., Lerot, C., Liu, C., Loyola, D., Piters, A., Richter, A., Rivera Cárdenas, C., Romahn, F., Ryan, R. G., Sinha, V., Theys, N., Vlietinck, J., Wagner, T., Wang, T., Yu, H., and Van Roozendael, M.: Comparative assessment of TROPOMI and OMI formaldehyde observations and validation against MAX-DOAS network column measurements, Atmos. Chem. Phys., 21, 12561–12593, https://doi.org/10.5194/acp-21-12561-2021, 2021. a, b
Diedrich, H., Preusker, R., Lindstrot, R., and Fischer, J.: Retrieval of daytime total columnar water vapour from MODIS measurements over land surfaces, Atmos. Meas. Tech., 8, 823–836, https://doi.org/10.5194/amt-8-823-2015, 2015. a
Drosoglou, T., Bais, A. F., Zyrichidou, I., Kouremeti, N., Poupkou, A., Liora, N., Giannaros, C., Koukouli, M. E., Balis, D., and Melas, D.: Comparisons of ground-based tropospheric NO2 MAX-DOAS measurements to satellite observations with the aid of an air quality model over the Thessaloniki area, Greece, Atmos. Chem. Phys., 17, 5829–5849, https://doi.org/10.5194/acp-17-5829-2017, 2017. a
Eleftheratos, K., Isaksen, I., Zerefos, C., Nastos, P., Tourpali, K., and Rognerud, B.: Ozone Variations Derived by a Chemical Transport Model, Water, Air, Soil Poll., 224, 1585, https://doi.org/10.1007/s11270-013-1585-2, 2013. a
Fried, A., Cantrell, C., Olson, J., Crawford, J. H., Weibring, P., Walega, J., Richter, D., Junkermann, W., Volkamer, R., Sinreich, R., Heikes, B. G., O'Sullivan, D., Blake, D. R., Blake, N., Meinardi, S., Apel, E., Weinheimer, A., Knapp, D., Perring, A., Cohen, R. C., Fuelberg, H., Shetter, R. E., Hall, S. R., Ullmann, K., Brune, W. H., Mao, J., Ren, X., Huey, L. G., Singh, H. B., Hair, J. W., Riemer, D., Diskin, G., and Sachse, G.: Detailed comparisons of airborne formaldehyde measurements with box models during the 2006 INTEX-B and MILAGRO campaigns: potential evidence for significant impacts of unmeasured and multi-generation volatile organic carbon compounds, Atmos. Chem. Phys., 11, 11867–11894, https://doi.org/10.5194/acp-11-11867-2011, 2011. a
Garane, K., Lerot, C., Coldewey-Egbers, M., Verhoelst, T., Koukouli, M. E., Zyrichidou, I., Balis, D. S., Danckaert, T., Goutail, F., Granville, J., Hubert, D., Keppens, A., Lambert, J.-C., Loyola, D., Pommereau, J.-P., Van Roozendael, M., and Zehner, C.: Quality assessment of the Ozone_cci Climate Research Data Package (release 2017) – Part 1: Ground-based validation of total ozone column data products, Atmos. Meas. Tech., 11, 1385–1402, https://doi.org/10.5194/amt-11-1385-2018, 2018. a
Garane, K., Koukouli, M.-E., Verhoelst, T., Lerot, C., Heue, K.-P., Fioletov, V., Balis, D., Bais, A., Bazureau, A., Dehn, A., Goutail, F., Granville, J., Griffin, D., Hubert, D., Keppens, A., Lambert, J.-C., Loyola, D., McLinden, C., Pazmino, A., Pommereau, J.-P., Redondas, A., Romahn, F., Valks, P., Van Roozendael, M., Xu, J., Zehner, C., Zerefos, C., and Zimmer, W.: TROPOMI/S5P total ozone column data: global ground-based validation and consistency with other satellite missions, Atmos. Meas. Tech., 12, 5263–5287, https://doi.org/10.5194/amt-12-5263-2019, 2019. a
Garane, K., Chan, K. L., Koukouli, M.-E., Loyola, D., and Balis, D.: TROPOMI/S5P Total Column Water Vapor validation against AERONET ground-based measurements, Atmos. Meas. Tech., 16, 57–74, https://doi.org/10.5194/amt-16-57-2023, 2023. a
Grossi, M., Valks, P., Loyola, D., Aberle, B., Slijkhuis, S., Wagner, T., Beirle, S., and Lang, R.: Total column water vapour measurements from GOME-2 MetOp-A and MetOp-B, Atmos. Meas. Tech., 8, 1111–1133, https://doi.org/10.5194/amt-8-1111-2015, 2015. a
Grzegorski, M.: Cloud retrieval from UV/VIS satellite instruments:(SCIAMACHY and GOME), PhD thesis, University of Heidelberg, https://doi.org/10.11588/heidok.00010423, 2009. a
Hao, N., Koukouli, M. E., Inness, A., Valks, P., Loyola, D. G., Zimmer, W., Balis, D. S., Zyrichidou, I., Van Roozendael, M., Lerot, C., and Spurr, R. J. D.: GOME-2 total ozone columns from MetOp-A/MetOp-B and assimilation in the MACC system, Atmos. Meas. Tech., 7, 2937–2951, https://doi.org/10.5194/amt-7-2937-2014, 2014. a
Hebestreit, K., Stutz, J., Rosen, D., Matveiv, V., Peleg, M., Luria, M., and Platt, U.: DOAS Measurements of Tropospheric Bromine Oxide in Mid-Latitudes, Science, 283, 55–57, https://doi.org/10.1126/science.283.5398.55, 1999. a
Hegglin, M. I., Fahey, D. W., McFarland, M., Montzka, S. A., and Nash, E. R.: Twenty Questions and Answers About the Ozone Layer: 2014 Update-Scientific Assessment of Ozone Depletion: 2014, https://oceanrep.geomar.de/id/eprint/34739/1/2014 Twenty Questions_Final.pdf (last access: 15 March 2023), 2015. a, b
Hendrick, F., Van Roozendael, M., Chipperfield, M. P., Dorf, M., Goutail, F., Yang, X., Fayt, C., Hermans, C., Pfeilsticker, K., Pommereau, J.-P., Pyle, J. A., Theys, N., and De Mazière, M.: Retrieval of stratospheric and tropospheric BrO profiles and columns using ground-based zenith-sky DOAS observations at Harestua, 60∘ N, Atmos. Chem. Phys., 7, 4869–4885, https://doi.org/10.5194/acp-7-4869-2007, 2007. a
Holben, B., Eck, T., Slutsker, I., Tanre, D., Buis, J., Setzer, A., Vermote, E., Reagan, J., Kaufman, Y., Nakajima, T., Lavenu, F., Jankowiak, I., and Smirnov, A.: AERONET – A Federated Instrument Network and Data Archive for Aerosol Characterization, Remote Sens. Environ., 66, 1–16, https://doi.org/10.1016/S0034-4257(98)00031-5, 1998. a
Holben, B. N., Tanre, D., Smirnov, A., Eck, T. F., Slutsker, I., Abuhassan, N., Newcomb, W. W., Schafer, J. S., Chatenet, B., Lavenu, F., Kaufman, Y. J., Castle, J. V., Setzer, A., Markham, B., Clark, D., Frouin, R., Halthore, R., Karneli, A., O'Neill, N. T., Pietras, C., Pinker, R. T., Voss, K., and Zibordi, G.: An emerging ground-based aerosol climatology: Aerosol optical depth from AERONET, J. Geophys. Res.-Atmos., 106, 12067–12097, https://doi.org/10.1029/2001JD900014, 2001. a
Hörmann, C., Sihler, H., Bobrowski, N., Beirle, S., Penning de Vries, M., Platt, U., and Wagner, T.: Systematic investigation of bromine monoxide in volcanic plumes from space by using the GOME-2 instrument, Atmos. Chem. Phys., 13, 4749–4781, https://doi.org/10.5194/acp-13-4749-2013, 2013. a
Hörmann, C., Sihler, H., Beirle, S., Penning de Vries, M., Platt, U., and Wagner, T.: Seasonal variation of tropospheric bromine monoxide over the Rann of Kutch salt marsh seen from space, Atmos. Chem. Phys., 16, 13015–13034, https://doi.org/10.5194/acp-16-13015-2016, 2016. a
Ionov, D. V., Timofeyev, Y. M., Sinyakov, V. P., Semenov, V. K., Goutail, F., Pommereau, J.-P., Bucsela, E. J., Celarier, E. A., and Kroon, M.: Ground-based validation of EOS-Aura OMI NO2 vertical column data in the midlatitude mountain ranges of Tien Shan (Kyrgyzstan) and Alps (France), J. Geophys. Res.-Atmos., 113, D15S08, https://doi.org/10.1029/2007JD008659, 2008. a
Irie, H., Kanaya, Y., Akimoto, H., Tanimoto, H., Wang, Z., Gleason, J. F., and Bucsela, E. J.: Validation of OMI tropospheric NO2 column data using MAX-DOAS measurements deep inside the North China Plain in June 2006: Mount Tai Experiment 2006, Atmos. Chem. Phys., 8, 6577–6586, https://doi.org/10.5194/acp-8-6577-2008, 2008. a
Irie, H., Kanaya, Y., Takashima, H., Gleason, J. F., and Wang, Z.: Characterization of OMI Tropospheric NO2 Measurements in East Asia Based on a Robust Validation Comparison, Scientific Online Letters on the Atmosphere, 5, 117–120, https://doi.org/10.2151/sola.2009-030, 2009. a
Irie, H., Boersma, K. F., Kanaya, Y., Takashima, H., Pan, X., and Wang, Z. F.: Quantitative bias estimates for tropospheric NO2 columns retrieved from SCIAMACHY, OMI, and GOME-2 using a common standard for East Asia, Atmos. Meas. Tech., 5, 2403–2411, https://doi.org/10.5194/amt-5-2403-2012, 2012. a
Irie, H., Muto, T., Itahashi, S., Kurokawa, J., and Uno, I.: Turnaround of Tropospheric Nitrogen Dioxide Pollution Trends in China, Japan, and South Korea, Scientific Online Letters on the Atmosphere, 12, 170–174, https://doi.org/10.2151/sola.2016-035, 2016. a
Kanaya, Y., Irie, H., Takashima, H., Iwabuchi, H., Akimoto, H., Sudo, K., Gu, M., Chong, J., Kim, Y. J., Lee, H., Li, A., Si, F., Xu, J., Xie, P.-H., Liu, W.-Q., Dzhola, A., Postylyakov, O., Ivanov, V., Grechko, E., Terpugova, S., and Panchenko, M.: Long-term MAX-DOAS network observations of NO2 in Russia and Asia (MADRAS) during the period 2007–2012: instrumentation, elucidation of climatology, and comparisons with OMI satellite observations and global model simulations, Atmos. Chem. Phys., 14, 7909–7927, https://doi.org/10.5194/acp-14-7909-2014, 2014. a
Kerr, J. B., Asbridge, I. A., and Evans, W. F. J.: Intercomparison of total ozone measured by the Brewer and Dobson spectrophotometers at Toronto, J. Geophys. Res.-Atmos., 93, 11129–11140, https://doi.org/10.1029/JD093iD09p11129, 1988. a
Khokhar, M., Frankenberg, C., Van Roozendael, M., Beirle, S., Kühl, S., Richter, A., Platt, U., and Wagner, T.: Satellite observations of atmospheric SO2 from volcanic eruptions during the time-period of 1996–2002, Adv. Space Res., 36, 879–887, https://doi.org/10.1016/j.asr.2005.04.114, 2005. a
Kiehl, J. T. and Trenberth, K. E.: Earth's Annual Global Mean Energy Budget, B. Am. Meteorol. Soc., 78, 197–208, https://doi.org/10.1175/1520-0477(1997)078<0197:EAGMEB>2.0.CO;2, 1997. a
Klaes, K. D., Cohen, M., Buhler, Y., Schlüssel, P., Munro, R., Luntama, J.-P., von Engeln, A., Clérigh, E. Ó., Bonekamp, H., Ackermann, J., and Schmetz, J.: An introduction to the EUMETSAT polar system, B. Am. Meteorol. Soc., 88, 1085–1096, https://doi.org/10.1175/BAMS-88-7-1085, 2007. a
Koelemeijer, R. B. A., de Haan, J. F., and Stammes, P.: A database of spectral surface reflectivity in the range 335–772 nm derived from 5.5 years of GOME observations, J. Geophys. Res.-Atmos., 108, 4070, https://doi.org/10.1029/2002JD002429, 2003. a
Koukouli, M. E., Balis, D. S., Loyola, D., Valks, P., Zimmer, W., Hao, N., Lambert, J.-C., Van Roozendael, M., Lerot, C., and Spurr, R. J. D.: Geophysical validation and long-term consistency between GOME-2/MetOp-A total ozone column and measurements from the sensors GOME/ERS-2, SCIAMACHY/ENVISAT and OMI/Aura, Atmos. Meas. Tech., 5, 2169–2181, https://doi.org/10.5194/amt-5-2169-2012, 2012. a
Koukouli, M. E., Lerot, C., Granville, J., Goutail, F., Lambert, J.-C., Pommereau, J.-P., Balis, D., Zyrichidou, I., Van Roozendael, M., Coldewey-Egbers, M., Loyola, D., Labow, G., Frith, S., Spurr, R., and Zehner, C.: Evaluating a new homogeneous total ozone climate data record from GOME/ERS-2, SCIAMACHY/Envisat, and GOME-2/MetOp-A, J. Geophys. Res.-Atmos., 120, 12296–12312, https://doi.org/10.1002/2015JD023699, 2015. a
Kuhlmann, G., Hartl, A., Cheung, H. M., Lam, Y. F., and Wenig, M. O.: A novel gridding algorithm to create regional trace gas maps from satellite observations, Atmos. Meas. Tech., 7, 451–467, https://doi.org/10.5194/amt-7-451-2014, 2014. a, b
Kumar, V., Beirle, S., Dörner, S., Mishra, A. K., Donner, S., Wang, Y., Sinha, V., and Wagner, T.: Long-term MAX-DOAS measurements of NO2, HCHO, and aerosols and evaluation of corresponding satellite data products over Mohali in the Indo-Gangetic Plain, Atmos. Chem. Phys., 20, 14183–14235, https://doi.org/10.5194/acp-20-14183-2020, 2020. a
Lang, R., Casadio, S., Maurellis, A. N., and Lawrence, M. G.: Evaluation of the GOME Water Vapor Climatology 1995–2002, J. Geophys. Res.-Atmos., 112, D17110, https://doi.org/10.1029/2006JD008246, 2007. a
Levelt, P., Van den Oord, G. H. J., Dobber, M., Malkki, A., Visser, H., de Vries, J., Stammes, P., Lundell, J., and Saari, H.: The ozone monitoring instrument, Geoscience and Remote Sensing, IEEE T. Geosci. Remote, 44, 1093–1101, https://doi.org/10.1109/TGRS.2006.872333, 2006. a
Li, X., Brauers, T., Hofzumahaus, A., Lu, K., Li, Y. P., Shao, M., Wagner, T., and Wahner, A.: MAX-DOAS measurements of NO2, HCHO and CHOCHO at a rural site in Southern China, Atmos. Chem. Phys., 13, 2133–2151, https://doi.org/10.5194/acp-13-2133-2013, 2013. a
Liu, S., Valks, P., Pinardi, G., De Smedt, I., Yu, H., Beirle, S., and Richter, A.: An improved total and tropospheric NO2 column retrieval for GOME-2, Atmos. Meas. Tech., 12, 1029–1057, https://doi.org/10.5194/amt-12-1029-2019, 2019. a
Liu, S., Valks, P., Pinardi, G., Xu, J., Argyrouli, A., Lutz, R., Tilstra, L. G., Huijnen, V., Hendrick, F., and Van Roozendael, M.: An improved air mass factor calculation for nitrogen dioxide measurements from the Global Ozone Monitoring Experiment-2 (GOME-2), Atmos. Meas. Tech., 13, 755–787, https://doi.org/10.5194/amt-13-755-2020, 2020. a
Loyola, D. G., Thomas, W., Livschitz, Y., Ruppert, T., Albert, P., and Hollmann, R.: Cloud Properties Derived From GOME/ERS-2 Backscatter Data for Trace Gas Retrieval, IEEE T. Geosci. Remote, 45, 2747–2758, https://doi.org/10.1109/TGRS.2007.901043, 2007. a, b
Loyola, D. G., Koukouli, M. E., Valks, P., Balis, D. S., Hao, N., Van Roozendael, M., Spurr, R. J. D., Zimmer, W., Kiemle, S., Lerot, C., and Lambert, J.-C.: The GOME-2 total column ozone product: Retrieval algorithm and ground-based validation, J. Geophys. Res.-Atmos., 116, D07302, https://doi.org/10.1029/2010JD014675, 2011. a, b, c
Loyola, D. G., Gimeno García, S., Lutz, R., Argyrouli, A., Romahn, F., Spurr, R. J. D., Pedergnana, M., Doicu, A., Molina García, V., and Schüssler, O.: The operational cloud retrieval algorithms from TROPOMI on board Sentinel-5 Precursor, Atmos. Meas. Tech., 11, 409–427, https://doi.org/10.5194/amt-11-409-2018, 2018. a
Lutz, R., Loyola, D., Gimeno García, S., and Romahn, F.: OCRA radiometric cloud fractions for GOME-2 on MetOp-A/B, Atmos. Meas. Tech., 9, 2357–2379, https://doi.org/10.5194/amt-9-2357-2016, 2016. a, b
Ma, J. Z., Beirle, S., Jin, J. L., Shaiganfar, R., Yan, P., and Wagner, T.: Tropospheric NO2 vertical column densities over Beijing: results of the first three years of ground-based MAX-DOAS measurements (2008–2011) and satellite validation, Atmos. Chem. Phys., 13, 1547–1567, https://doi.org/10.5194/acp-13-1547-2013, 2013. a, b
Martins, V. S., Lyapustin, A., Wang, Y., Giles, D. M., Smirnov, A., Slutsker, I., and Korkin, S.: Global validation of columnar water vapor derived from EOS MODIS-MAIAC algorithm against the ground-based AERONET observations, Atmos. Res., 225, 181–192, https://doi.org/10.1016/j.atmosres.2019.04.005, 2019. a
Merlaud, A., Theys, N., Hendrick, F., van Gent, J., Pinardi, G., Van Roozendael, M., Chan, K. L., Heue, K. P., and Valks, P.: Validation report of GOME-2 GDP 4.9 BrO column data for MetOp-C Operational Readiness Review, Tech. Rep., EUMETSAT AC SAF, https://acsaf.org/docs/vr/Validation_Report_OTO_BrO_May_2020.pdf (last access: 15 March 2023), 2020. a, b
Müller, J.-F. and Stavrakou, T.: Inversion of CO and NOx emissions using the adjoint of the IMAGES model, Atmos. Chem. Phys., 5, 1157–1186, https://doi.org/10.5194/acp-5-1157-2005, 2005. a
Munro, R., Lang, R., Klaes, D., Poli, G., Retscher, C., Lindstrot, R., Huckle, R., Lacan, A., Grzegorski, M., Holdak, A., Kokhanovsky, A., Livschitz, J., and Eisinger, M.: The GOME-2 instrument on the Metop series of satellites: instrument design, calibration, and level 1 data processing – an overview, Atmos. Meas. Tech., 9, 1279–1301, https://doi.org/10.5194/amt-9-1279-2016, 2016. a
Noël, S., Mieruch, S., Bovensmann, H., and Burrows, J. P.: Preliminary results of GOME-2 water vapour retrievals and first applications in polar regions, Atmos. Chem. Phys., 8, 1519–1529, https://doi.org/10.5194/acp-8-1519-2008, 2008. a
Palmer, P. I., Jacob, D. J., Chance, K., Martin, R. V., Spurr, R. J. D., Kurosu, T. P., Bey, I., Yantosca, R., Fiore, A., and Li, Q.: Air mass factor formulation for spectroscopic measurements from satellites: Application to formaldehyde retrievals from the Global Ozone Monitoring Experiment, J. Geophys. Res.-Atmos., 106, 14539–14550, https://doi.org/10.1029/2000JD900772, 2001. a
Pérez-Ramírez, D., Whiteman, D. N., Smirnov, A., Lyamani, H., Holben, B. N., Pinker, R., Andrade, M., and Alados-Arboledas, L.: Evaluation of AERONET precipitable water vapor versus microwave radiometry, GPS, and radiosondes at ARM sites, J. Geophys. Res.-Atmos., 119, 9596–9613, https://doi.org/10.1002/2014JD021730, 2014. a, b, c
Pinardi, G., Yu, H., Lambert, J.-C., Granville, J., van Gent, J., Van Roozendael, M., and Valks, P.: Validation report of GOME-2 GDP 4.9 NO2 column data for MetOp-C Operational Readiness Review, Tech. Rep., EUMETSAT AC SAF, https://acsaf.org/docs/vr/Validation_Report_NTO_OTO_NO2_Nov_2019.pdf (last access: 15 March 2023), 2019. a, b
Pinardi, G., Van Roozendael, M., Hendrick, F., Theys, N., Abuhassan, N., Bais, A., Boersma, F., Cede, A., Chong, J., Donner, S., Drosoglou, T., Dzhola, A., Eskes, H., Frieß, U., Granville, J., Herman, J. R., Holla, R., Hovila, J., Irie, H., Kanaya, Y., Karagkiozidis, D., Kouremeti, N., Lambert, J.-C., Ma, J., Peters, E., Piters, A., Postylyakov, O., Richter, A., Remmers, J., Takashima, H., Tiefengraber, M., Valks, P., Vlemmix, T., Wagner, T., and Wittrock, F.: Validation of tropospheric NO2 column measurements of GOME-2A and OMI using MAX-DOAS and direct sun network observations, Atmos. Meas. Tech., 13, 6141–6174, https://doi.org/10.5194/amt-13-6141-2020, 2020a. a, b
Pinardi, G., Yu, H., Van Roozendael, M., Van Gent, J., Chan, K. L., and Valks, P.: Validation report of GOME-2 GDP 4.9 HCHO column data for MetOp-C Operational Readiness Review, https://acsaf.org/docs/vr/Validation_Report_NTO_OTO_HCHO_May_2020.pdf (last access: 15 March 2023), 2020b. a, b
Platt, U. and Stutz, J.: Differential optical absorption spectroscopy – principles and applications, Springer, ISBN 978-3-540-21193-8, 2008. a
Platt, U. and Wagner, T.: Satellite mapping of enhanced BrO concentrations in the troposphere, Nature, 395, 486–490, https://doi.org/10.1038/26723, 1998. a
Richter, A., Wittrock, F., Eisinger, M., and Burrows, J. P.: GOME observations of tropospheric BrO in northern hemispheric spring and summer 1997, Geophys. Res. Lett., 25, 2683–2686, https://doi.org/10.1029/98GL52016, 1998. a
Richter, A., Wittrock, F., Ladstätter-Weißenmayer, A., and Burrows, J.: GOME measurements of stratospheric and tropospheric BrO, Adv. Space Res., 29, 1667–1672, https://doi.org/10.1016/S0273-1177(02)00123-0, 2002. a
Rix, M., Valks, P., Hao, N., van Geffen, J., Clerbaux, C., Clarisse, L., Coheur, P.-F., Loyola R., D. G., Erbertseder, T., Zimmer, W., and Emmadi, S.: Satellite Monitoring of Volcanic Sulfur Dioxide Emissions for Early Warning of Volcanic Hazards, IEEE J. Sel. Top. Appl., 2, 196–206, https://doi.org/10.1109/JSTARS.2009.2031120, 2009. a
Rix, M., Valks, P., Hao, N., Loyola, D., Schlager, H., Huntrieser, H., Flemming, J., Koehler, U., Schumann, U., and Inness, A.: Volcanic SO2, BrO and plume height estimations using GOME-2 satellite measurements during the eruption of Eyjafjallajökull in May 2010, J. Geophys. Res.-Atmos., 117, D00U19, https://doi.org/10.1029/2011JD016718, 2012. a
Shindell, D. T., Faluvegi, G., Koch, D. M., Schmidt, G. A., Unger, N., and Bauer, S. E.: Improved Attribution of Climate Forcing to Emissions, Science, 326, 716–718, https://doi.org/10.1126/science.1174760, 2009. a
Solomon, S., Schmeltekopf, A. L., and Sanders, R. W.: On the interpretation of zenith sky absorption measurements, J. Geophys. Res.-Atmos., 92, 8311–8319, https://doi.org/10.1029/JD092iD07p08311, 1987. a
Spurr, R.: LIDORT and VLIDORT: Linearized pseudo-spherical scalar and vector discrete ordinate radiative transfer models for use in remote sensing retrieval problems, Springer Berlin Heidelberg, Berlin, Heidelberg, 229–275, https://doi.org/10.1007/978-3-540-48546-9_7, 2008. a, b
Spurr, R. J.: VLIDORT: A linearized pseudo-spherical vector discrete ordinate radiative transfer code for forward model and retrieval studies in multilayer multiple scattering media, J. Quant. Spectrosc. Ra., 102, 316–342, https://doi.org/10.1016/j.jqsrt.2006.05.005, 2006. a
Theys, N., Van Roozendael, M., Dils, B., Hendrick, F., Hao, N., and De Mazière, M.: First satellite detection of volcanic bromine monoxide emission after the Kasatochi eruption, Geophys. Res. Lett., 36, L03809, https://doi.org/10.1029/2008GL036552, 2009. a
Theys, N., Van Roozendael, M., Hendrick, F., Yang, X., De Smedt, I., Richter, A., Begoin, M., Errera, Q., Johnston, P. V., Kreher, K., and De Mazière, M.: Global observations of tropospheric BrO columns using GOME-2 satellite data, Atmos. Chem. Phys., 11, 1791–1811, https://doi.org/10.5194/acp-11-1791-2011, 2011. a, b
Theys, N., Hendrick, F., Van Gent, J., Van Roozendael, M., Hao, N., and Valks, P.: Validation report of GOME-2 offline and reprocessed GDP 4.8 BrO total column data for MetOp-A and B, https://acsaf.org/docs/vr/Validation_Report_OTO_DR_BrO_GDP48_Dec_2015.pdf (last access: 15 March 2023), 2015a. a
Theys, N., Koukouli, M., Pinardi, G., Van Roozendael, M., Balis, D., Hedelt, H., and Valks, P.: Validation report of NRT, offline and reprocessed GOME-2 SO2 column data for MetOp-A and -B, https://acsaf.org/docs/vr/Validation_Report_NTO_OTO_DR_SO2_GDP48_Dec_2015.pdf (last access: 15 March 2023), 2015b. a
Valks, P., Pinardi, G., Richter, A., Lambert, J.-C., Hao, N., Loyola, D., Van Roozendael, M., and Emmadi, S.: Operational total and tropospheric NO2 column retrieval for GOME-2, Atmos. Meas. Tech., 4, 1491–1514, https://doi.org/10.5194/amt-4-1491-2011, 2011. a, b, c
Valks, P., Chan, K. L., Hedelt, P., Slijkhuis, S., , and Lutz, R.: Algorithm Theoretical Basis Document for GOME-2 Total Column Products of Ozone, NO2, BrO, SO2, H2O, HCHO, OClO and Cloud Properties (GDP 4.8/4.9), Tech. Rep., EUMETSAT AC SAF, https://acsaf.org/docs/atbd/Algorithm_Theoretical_Basis_Document_NTO_OTO_Nov_2019.pdf (last access: 15 March 2023), 2019. a
Van Roozendael, M., Loyola, D., Spurr, R., Balis, D., Lambert, J.-C., Livschitz, Y., Valks, P., Ruppert, T., Kenter, P., Fayt, C., and Zehner, C.: Ten years of GOME/ERS-2 total ozone data – The new GOME data processor (GDP) version 4: 1. Algorithm description, J. Geophys. Res.-Atmos., 111, D14311, https://doi.org/10.1029/2005JD006375, 2006. a
Vandaele, A. C., Hermans, C., Fally, S., Carleer, M., Colin, R., Merienne, M.-F., Jenouvrier, A., and Coquart, B.: High-resolution Fourier transform measurement of the NO2 visible and near-infrared absorption cross sections: Temperature and pressure effects, J. Geophys. Res.-Atmos., 107, 4348, https://doi.org/10.1029/2001JD000971, 2002. a
Veefkind, J., Aben, I., McMullan, K., Förster, H., de Vries, J., Otter, G., Claas, J., Eskes, H., de Haan, J., Kleipool, Q., van Weele, M., Hasekamp, O., Hoogeveen, R., Landgraf, J., Snel, R., Tol, P., Ingmann, P., Voors, R., Kruizinga, B., Vink, R., Visser, H., and Levelt, P.: TROPOMI on the ESA Sentinel-5 Precursor: A GMES mission for global observations of the atmospheric composition for climate, air quality and ozone layer applications, Remote Sens. Environ., 120, 70–83, https://doi.org/10.1016/j.rse.2011.09.027, 2012. a
Verhoelst, T., Compernolle, S., Pinardi, G., Lambert, J.-C., Eskes, H. J., Eichmann, K.-U., Fjæraa, A. M., Granville, J., Niemeijer, S., Cede, A., Tiefengraber, M., Hendrick, F., Pazmiño, A., Bais, A., Bazureau, A., Boersma, K. F., Bognar, K., Dehn, A., Donner, S., Elokhov, A., Gebetsberger, M., Goutail, F., Grutter de la Mora, M., Gruzdev, A., Gratsea, M., Hansen, G. H., Irie, H., Jepsen, N., Kanaya, Y., Karagkiozidis, D., Kivi, R., Kreher, K., Levelt, P. F., Liu, C., Müller, M., Navarro Comas, M., Piters, A. J. M., Pommereau, J.-P., Portafaix, T., Prados-Roman, C., Puentedura, O., Querel, R., Remmers, J., Richter, A., Rimmer, J., Rivera Cárdenas, C., Saavedra de Miguel, L., Sinyakov, V. P., Stremme, W., Strong, K., Van Roozendael, M., Veefkind, J. P., Wagner, T., Wittrock, F., Yela González, M., and Zehner, C.: Ground-based validation of the Copernicus Sentinel-5P TROPOMI NO2 measurements with the NDACC ZSL-DOAS, MAX-DOAS and Pandonia global networks, Atmos. Meas. Tech., 14, 481–510, https://doi.org/10.5194/amt-14-481-2021, 2021. a
Vigouroux, C., Hendrick, F., Stavrakou, T., Dils, B., De Smedt, I., Hermans, C., Merlaud, A., Scolas, F., Senten, C., Vanhaelewyn, G., Fally, S., Carleer, M., Metzger, J.-M., Müller, J.-F., Van Roozendael, M., and De Mazière, M.: Ground-based FTIR and MAX-DOAS observations of formaldehyde at Réunion Island and comparisons with satellite and model data, Atmos. Chem. Phys., 9, 9523–9544, https://doi.org/10.5194/acp-9-9523-2009, 2009. a
Wagner, T., Heland, J., Zöger, M., and Platt, U.: A fast H2O total column density product from GOME – Validation with in-situ aircraft measurements, Atmos. Chem. Phys., 3, 651–663, https://doi.org/10.5194/acp-3-651-2003, 2003. a
Wagner, T., Beirle, S., Grzegorski, M., and Platt, U.: Global trends (1996–2003) of total column precipitable water observed by Global Ozone Monitoring Experiment (GOME) on ERS-2 and their relation to near-surface temperature, J. Geophys. Res.-Atmos., 111, D12102, https://doi.org/10.1029/2005JD006523, 2006. a, b
Wagner, T., Beirle, S., Deutschmann, T., Grzegorski, M., and Platt, U.: Satellite monitoring of different vegetation types by differential optical absorption spectroscopy (DOAS) in the red spectral range, Atmos. Chem. Phys., 7, 69–79, https://doi.org/10.5194/acp-7-69-2007, 2007. a
Wang, Y., Beirle, S., Lampel, J., Koukouli, M., De Smedt, I., Theys, N., Li, A., Wu, D., Xie, P., Liu, C., Van Roozendael, M., Stavrakou, T., Müller, J.-F., and Wagner, T.: Validation of OMI, GOME-2A and GOME-2B tropospheric NO2, SO2 and HCHO products using MAX-DOAS observations from 2011 to 2014 in Wuxi, China: investigation of the effects of priori profiles and aerosols on the satellite products, Atmos. Chem. Phys., 17, 5007–5033, https://doi.org/10.5194/acp-17-5007-2017, 2017. a, b
Wenig, M., Kühl, S., Beirle, S., Bucsela, E., Jähne, B., Platt, U., Gleason, J., and Wagner, T.: Retrieval and analysis of stratospheric NO2 from the Global Ozone Monitoring Experiment, J. Geophys. Res.-Atmos., 109, D04315, https://doi.org/10.1029/2003JD003652, 2004. a
Wenig, M. O., Cede, A. M., Bucsela, E. J., Celarier, E. A., Boersma, K. F., Veefkind, J. P., Brinksma, E. J., Gleason, J. F., and Herman, J. R.: Validation of OMI tropospheric NO2 column densities using direct-Sun mode Brewer measurements at NASA Goddard Space Flight Center, J. Geophys. Res.-Atmos., 113, D16S45, https://doi.org/10.1029/2007JD008988, 2008. a
Wennberg, P. O., Cohen, R. C., Stimpfle, R. M., Koplow, J. P., Anderson, J. G., Salawitch, R. J., Fahey, D. W., Woodbridge, E. L., Keim, E. R., Gao, R. S., Webster, C. R., May, R. D., Toohey, D. W., Avallone, L. M., Proffitt, M. H., Loewenstein, M., Podolske, J. R., Chan, K. R., and Wofsy, S. C.: Removal of Stratospheric O3 by Radicals: In Situ Measurements of OH, HO2, NO, NO2, ClO, and BrO, Science, 266, 398–404, https://doi.org/10.1126/science.266.5184.398, 1994. a