the Creative Commons Attribution 4.0 License.
the Creative Commons Attribution 4.0 License.
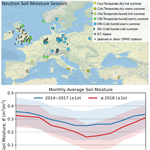
COSMOS-Europe: a European network of cosmic-ray neutron soil moisture sensors
Martin Schrön
Jannis Jakobi
Patrizia Ney
Steffen Zacharias
Mie Andreasen
Roland Baatz
David Boorman
Mustafa Berk Duygu
Miguel Angel Eguibar-Galán
Benjamin Fersch
Till Franke
Josie Geris
María González Sanchis
Yann Kerr
Tobias Korf
Zalalem Mengistu
Arnaud Mialon
Paolo Nasta
Jerzy Nitychoruk
Vassilios Pisinaras
Daniel Rasche
Rafael Rosolem
Hami Said
Paul Schattan
Marek Zreda
Stefan Achleitner
Eduardo Albentosa-Hernández
Zuhal Akyürek
Theresa Blume
Antonio del Campo
Davide Canone
Katya Dimitrova-Petrova
John G. Evans
Stefano Ferraris
Félix Frances
Davide Gisolo
Andreas Güntner
Frank Herrmann
Joost Iwema
Karsten H. Jensen
Harald Kunstmann
Antonio Lidón
Majken Caroline Looms
Sascha Oswald
Andreas Panagopoulos
Amol Patil
Daniel Power
Corinna Rebmann
Nunzio Romano
Lena Scheiffele
Sonia Seneviratne
Georg Weltin
Harry Vereecken
Climate change increases the occurrence and severity of droughts due to increasing temperatures, altered circulation patterns, and reduced snow occurrence. While Europe has suffered from drought events in the last decade unlike ever seen since the beginning of weather recordings, harmonized long-term datasets across the continent are needed to monitor change and support predictions. Here we present soil moisture data from 66 cosmic-ray neutron sensors (CRNSs) in Europe (COSMOS-Europe for short) covering recent drought events. The CRNS sites are distributed across Europe and cover all major land use types and climate zones in Europe. The raw neutron count data from the CRNS stations were provided by 24 research institutions and processed using state-of-the-art methods. The harmonized processing included correction of the raw neutron counts and a harmonized methodology for the conversion into soil moisture based on available in situ information. In addition, the uncertainty estimate is provided with the dataset, information that is particularly useful for remote sensing and modeling applications. This paper presents the current spatiotemporal coverage of CRNS stations in Europe and describes the protocols for data processing from raw measurements to consistent soil moisture products. The data of the presented COSMOS-Europe network open up a manifold of potential applications for environmental research, such as remote sensing data validation, trend analysis, or model assimilation. The dataset could be of particular importance for the analysis of extreme climatic events at the continental scale. Due its timely relevance in the scope of climate change in the recent years, we demonstrate this potential application with a brief analysis on the spatiotemporal soil moisture variability. The dataset, entitled “Dataset of COSMOS-Europe: A European network of Cosmic-Ray Neutron Soil Moisture Sensors”, is shared via Forschungszentrum Jülich: https://doi.org/10.34731/x9s3-kr48 (Bogena and Ney, 2021).
- Article
(7215 KB) - Full-text XML
- BibTeX
- EndNote
The years 2003, 2010, 2015, and 2018 are considered as the most notable years of the 21st century in Europe in terms of summer drought and also witnessed numerous heat-related deaths (Stott et al., 2004; Ionita et al., 2017; Laaha et al., 2017; Schuldt et al., 2020; Sutanto et al., 2020) and extensive forest fires (Fink et al., 2004; Grumm, 2011; Turco et al., 2017). This has stimulated a debate on how changes in the occurrence and characteristics of drought are related to climatic variability (e.g., Hanel et al., 2018; Hisdal et al., 2001; Seneviratne et al., 2012; Sheffield et al., 2012). During the most recent heat wave in 2018, daily temperature anomalies reached up to 14 ∘C in Scandinavia and central Europe and impacted the energy and carbon balance of European terrestrial ecosystems (Graf et al., 2020). This heat wave was exacerbated by a drought caused by a persistent circulation anomaly (Kornhuber et al., 2019), which additionally fostered unprecedented wildfires in Europe (e.g., Yiou et al., 2020). Recently, Humphrey et al. (2021) have shown that soil moisture variability explains 90 % of the interannual variability in global carbon uptake. The corresponding feedback between soil moisture and the atmosphere amplifies temperature and moisture anomalies and intensifies the direct effects of drought and soil water stress. In this respect, ground-based soil moisture measurements are indispensable to better understand the land-surface–atmosphere interactions leading to droughts and soil water stress.
Recent advances in measurement techniques, such as cosmic-ray neutron probes, allow continuous non-invasive soil moisture measurements that integrate over scales beyond the traditional point measurement (Zreda et al., 2012; Bogena et al., 2015; Andreasen et al., 2017b). In the 1950s it was discovered that neutron scattering could be used as a method of measuring soil moisture (e.g., Gardner and Kirkham, 1952), and this was to become the main means of quantifying water storage in soils for the next three decades. The neutron probe contains a radioactive source that generates fast neutrons that are decelerated by the hydrogen of the soil water to thermal neutrons, so that the detected thermal neutron count rate is closely related to the soil water content. Thanks to the pioneering work of Topp et al. (1980), from the 1980s the electromagnetic measurement technology became established for simple and continuous monitoring of soil moisture dynamics. As a result, neutron probes were hardly used anymore, and interest in neutron scattering in soils declined until the introduction of the cosmic-ray neutron measurement method (Zreda et al., 2008) generated renewed interest. Recently, neutron scattering is again considered one of the most promising soil moisture measurement techniques, as cosmic neutron sensors (CRNSs) provide non-invasive soil moisture at the field scale with an effective radius of 130 to 240 m and a penetration depth of 15 to 55 cm depending on soil wetness (Köhli et al., 2015; Schrön et al., 2017). In contrast to the classical active neutron probe, the CRNS is placed above ground and detects cosmogenic neutrons. The CRNS can be calibrated by comparing the neutron count rate with gravimetric soil moisture sampling data averaged over the CRNS footprint by a weighting function (Schrön et al., 2017). The CRNS shows excellent data acquisition reliability and can be applied also in vegetated areas with low to medium biomass such as cropped fields (Rivera Villarreyes et al., 2011; Franz et al., 2013) and forests (Bogena et al., 2013; Heidbüchel et al., 2016; Vather et al., 2020). During the last decade, several studies applied and progressed the CRNS technique both on stationary and mobile platforms up to the scale of square kilometers (Fersch et al., 2020; Schrön et al., 2018) and by monitoring stations installed in a broad variety of climate conditions, namely continental (e.g., Baatz et al., 2014), temperate (e.g., Evans et al., 2016), semi-arid (e.g., Zreda et al., 2012), and tropical (e.g., Hawdon et al., 2014). The advantages of the CRNS technique have promoted its application in various fields, such as hydrology (e.g., Dimitrova-Petrova et al., 2020a; Schattan et al., 2020), snow monitoring (e.g., Bogena et al., 2020; Schattan et al., 2017), precipitation monitoring (Franz et al., 2020), vegetation monitoring (e.g., Franz et al., 2013; Jakobi et al., 2018), validation of remote sensing products (e.g., Montzka et al., 2017; Duygu and Akyürek, 2019), land surface modeling (e.g., Shuttleworth et al., 2013; Baatz et al., 2017; Brunetti et al., 2019; Iwema et al., 2017, Patil et al., 2021), and agricultural management (e.g., Finkenbiner et al., 2018; Li et al., 2019).
According to Andreasen et al. (2017a), there are currently more than 200 stationary CRNSs operated worldwide, often as regional networks in hydrological observatories (e.g., Bogena et al., 2018; Kiese et al., 2018; Liu et al., 2018) or in entire countries (Zreda et al., 2012; Hawdon et al., 2014; Evans et al., 2016). This paper introduces the network of existing CRNS stations in Europe (COSMOS-Europe for short) and how the data are processed in a harmonized way. We present the current instrumentation and the protocols developed to process the raw measurements and how the CRNS stations have been recalibrated to derive soil moisture in a more consistent way. Based on the processed CRNS soil moisture time series, we then performed a brief analysis on the spatiotemporal occurrence of drought events in Europe.
For the COSMOS-Europe dataset presented here, CRNS data from 66 sites in 12 European countries (in alphabetical order: Austria, Denmark, France, Germany, Greece, Italy, Norway, Poland, Spain, Switzerland, Turkey, United Kingdom) were collected. The geographical distribution and location of the COSMOS-Europe sites is shown in Fig. 1. The key environmental and soil-related physical properties at the sites are given in Table 1. The key physical and soil-related site properties relevant to CRNS processing are summarized in Table 2.
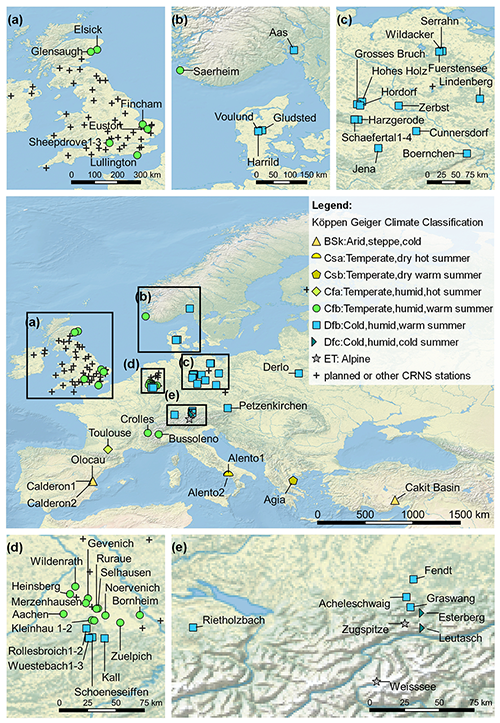
Figure 1Locations of the COSMOS-Europe sites (the symbols show the climatic zone to which they belong) as well as sites, which are currently under construction or sites whose data we could not use.
Table 1General information of the COSMOS-Europe sites (ordered based on latitude).
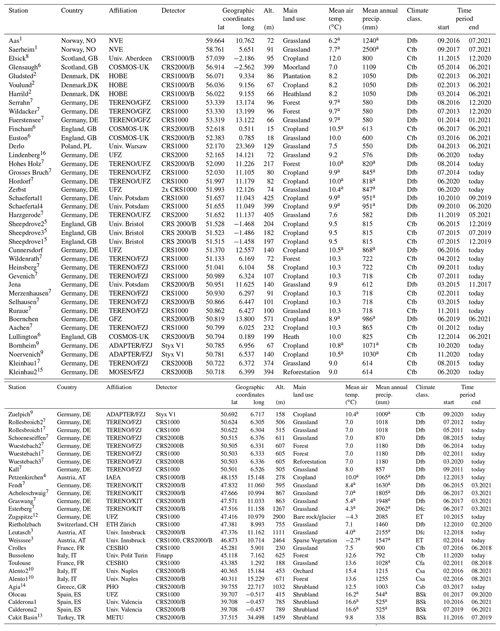
a Derived from ERA5 data. 1 Part of the groundwater and soil moisture monitoring network of the Norwegian water resources and energy directorate operated since 1998 and 1999, respectively. 2 Part of the Danish Hydrological Observatory, HOBE (http://www.hobe.dk/, last access: 2 March 2022). Data are partly published by Andreasen et al. (2019; 2020) with more information on the field sites. 3 Part of the Moosbeere network operated by the University of Innsbruck. The Weisssee station is located on rocky terrain and not suited for soil moisture analysis. 4 Part of the Hydrological Open Air Laboratory (HOAL, https://hoal.hydrology.at/the-hoal, last access: 2 March 2022), which is a cooperation project between the Federal Agency for Water Management (BAW Petzenkirchen) and the Vienna University of Technology (TU Wien). More information about the site can be found in Blöschl et al. (2016). 5 Located at the Sheepdrove Organic Farm in the UK. More information about the site can be found in Iwema et al. (2021), Schrön et al. (2017), and Berthelin et al. (2020). 6 Part of the COSMOS-UK network operated by the UK Centre for Ecology & Hydrology. Further information about the sites and COSMOS-UK is presented in Cooper et al. (2021). 7 Part of the German Terrestrial Environmental Observatories (TERENO) network (https://www.tereno.net/, last access: 2 March 2022). 8 The CRNS is placed at the corner of three adjacent agricultural fields. Further information about the site can be found Dimitrova-Petrova et al. (2020a; 2020b; 2021). 9 Part of the ADAPTER (ADAPT TERrestrial systems) project (https://www.adapter-projekt.de/, last access: 2 March 2022; Ney et al., 2021). 10 Part of Alento Hydrological Observatory (AHO) in southern Italy established in 2016 by the University of Naples in cooperation with Forschungszentrum Jülich GmbH (Romano et al., 2018; Nasta et al., 2020). 11 Due to a malfunction, the detector had to be replaced. To ensure a consistent time series, the neutron counts 1 year before and after the replacements were compared, and the time series after the replacement were adjusted accordingly. 12 The Zugspitze sensor is located in the Schneefernerhaus (UFS) observatory and surrounded by rocky mountain terrain and not suited for soil moisture analysis. 13 Operated by the Water Resources Laboratory of Middle East Technical University. 14 Part of the Pinios Hydrologic Observatory (PHO) in central Greece established in 2017 by the Soil and Water Resources Institute, Hellenic Agricultural Organization “DEMETER”, in cooperation with Forschungszentrum Jülich GmbH (Pisinaras et al., 2018; Bogena et al., 2020). 15 Part of the MOSES (Modular Observation Solutions for Earth Systems) project (https://www.ufz.de/moses/, last access: 2 March 2022). 16 DWD – Deutscher Wetterdienst (2021).
The COSMOS-Europe sites cover eight climatic zones (following the Köppen–Geiger climate classification; Beck et al., 2018), with the vast majority of stations located in the humid continental climate zone (n=34) and in the temperate oceanic climate zone (n=21). The remaining sites are located in six further climate zones. According to site owner information, the majority of COSMOS-Europe sites are managed grassland (n=23) and cropland (n=23), while the remaining sites are covered by forest (n=7), forest clear-cut (n=1), shrubland (n=5), heathland (n=2), orchard/plantation (n=2), bare rock/glacier (n=1), moorland (n=1), and sparse vegetation (n=1).
The soils of the COSMOS-Europe sites range from organic soils with a high organic matter content (max: 0.173 g g−1) to mineral soils with very low organic matter content (min: 0.004 g g−1). This variability is also reflected in the wide range of soil porosities ranging from 0.365 to 0.841. Two of the sites, Weisssee and Zugspitze, are located in rocky, alpine terrain. The Weisssee data only show limited and short snow-free periods where soil moisture data are available and with high uncertainties due to the difficult soil sampling in that area. The data from Zugspitze are not used for soil moisture analysis due to the absence of soil but offer great potential for other hydrological studies, such as snow water equivalent monitoring.
The measurements of neutron count rates and corresponding correction data (i.e., atmospheric pressure and air humidity) at the COSMOS-Europe sites cover very different periods of time (cf. Fig. 2 and Table 1). The shortest time series comes from the Zerbst site, which was put into operation in late 2020. The longest time series from the Wuestebach1 site spans a period of approximately 10 years (mid-2011 to present). The average length of the observation periods of all sites is 5.7 years (±2.78).
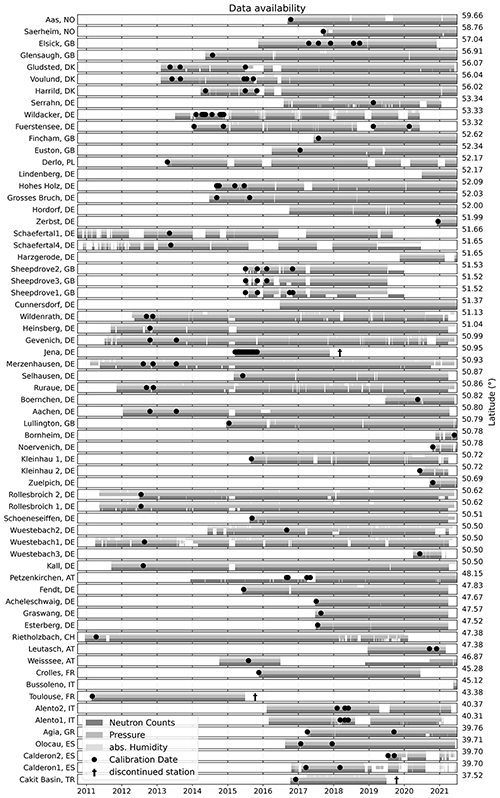
Figure 2Availability of atmospheric pressure, absolute humidity, and neutron count rates at the COSMOS-Europe sites (sorted by descending latitude). The dates of the local reference soil sampling for CRNS calibration are also shown. The timeline shown ends in June 2021, while data and calibration dates for some sensors extend to November 2021.
The geographic distribution of the COSMOS-Europe stations also reflects strong gradients of cutoff rigidities – a quantity describing the shielding of incoming cosmic-ray particles by Earth's geomagnetic field. Therefore, the dynamics and intensity of cosmic rays at stations in northern Europe are significantly higher than at stations further south. The cutoff rigidity ranges from 1.21 GeV for the Aas site in Norway to 8.37 for the Cakit Basin site in Turkey.
More than 50 additional sites are indicated in Fig. 1 (black cross) which are not specifically addressed in this paper. They either belong to other networks with dedicated data publications (e.g., COSMOS-UK or the intensive research experiment in Marquardt near Berlin), were installed just recently (e.g., Prague and northwest Germany), or refer to planned COSMOS locations in the near future (e.g., Finland and Ireland). There are even more stations across Europe that operate sub-snow cosmic-ray neutron detectors (Gugerli et al., 2019). Due to the slightly different measurement technique, the point-scale footprint, and the exclusive focus on snow monitoring, those sensors were not included in this paper and deserve dedicated articles.
Measured neutrons are a proxy for soil water content, but systematic factors and stochastic effects also influence the neutron signal. Research in the last decades has led to a profound understanding of these influencing factors and has facilitated a more accurate extraction of the soil moisture signal from the cosmic-ray neutron data. The processing framework is described below, while its technical implementation is supported by public tools and software libraries dedicated to CRNS research, e.g., Corny by Schrön (2021) and Crspy by Power et al. (2021).
3.1 Data pre-processing
In a first step, all datasets, i.e., raw neutron counts and supporting data, were aggregated to hourly time steps. Subsequently, following Zreda et al. (2008), a running 24 h average with a minimum of 12 measurements in the smoothing window was used to reduce the inherent noise of the raw neutron counts and to reduce the measurement uncertainty.
To ensure data consistency, the raw neutron counts were screened for data quality. Suspicious neutron count rates (Nraw) that fulfill one of the following conditions were flagged.
-
Extreme single outliers: Nraw<50 or Nraw>10 000 counts per hour (cts h−1).
-
Positive suspicious peaks: Nraw>24 h moving average +2 times the standard deviation of the 24 h rolling sum.
-
Negative suspicious peaks: Nraw<24 h moving average −2 times the standard deviation of the 24 h rolling sum.
Neutron count rates can be strongly affected by the presence of snow cover, resulting in inaccurate soil moisture measurements. Unfortunately, in most cases no additional snow measurements were available at the CRNS sites. Therefore, we used the ECMWF climate reanalysis data product ERA5-Land (Muñoz Sabater, 2019) to indicate snow cover events. For this, we flagged neutron count data when the 24 h moving average of the ERA5 SWE (snow water equivalent) product exceeded 1 mm.
To indicate unrealistically high values in the CRNS-derived soil moisture time series, we flagged values for soil moisture that were greater than local soil porosity. Because local measurements of soil porosity were not available, we estimated porosity using available information on bulk density and soil organic carbon content. We assumed that soil organic matter was 2 times the organic carbon content and assumed densities of 1.4 and 2.65 g cm−3 for the organic matter and the other soil minerals, respectively. Missing data on soil texture, porosity, and organic carbon were taken from the global raster-based soil dataset SoilGrids (Hengl et al., 2017).
It is important to note that the published dataset still includes the original and flagged data, while suspicious records were not included in the further data processing and analysis (see Fig. A5 for the used data flags). In this way, users can apply their own pre-processing techniques to the raw neutron count data. The final soil moisture product is cleaned from all negative influences to avoid inexperienced users using unrealistic soil moisture data.
The local air temperature, air humidity, and atmospheric pressure data needed for the correction of raw neutron counts often contained gaps due to measurement failure or due to removing suspicious data using max/min filters (see Fig. 2). These data gaps were filled with ERA5 data following the idea of Power et al. (2021). To ensure consistency of the data, linear regression models of the individual data time series were created to scale the ERA5 data to the local data prior to gap filling. Linear regression is necessary to compensate for differences in bias and slope, e.g., because due to the low spatial resolution of ERA5 (∼31 km), the average altitude, humidity, and atmospheric pressure for the ERA5 grid does not match those at the COSMOS-Europe site. These deviations occur especially in the high mountains due to strong elevation differences, e.g., for atmospheric pressure at the Leutasch site (see Fig. A2). The regression analysis showed that the ERA5 data mostly agreed well with the local measurements (Figs. A1 and A2), with mean correlations between ERA5 and local measurements of 0.95 for atmospheric pressure and 0.86 for absolute humidity. When the correlation coefficients for humidity and atmospheric pressure were less than 0.7 and 0.8, respectively, the local measurements were replaced entirely by ERA5 data to avoid inconsistencies in the gap-filled time series.
3.2 Correction of raw neutron counts
Variations of the incoming cosmic-ray intensity can have many causes, from galactic and solar disturbances to atmospheric and meteorological influences. Most of these anomalies are expected to change proportionally in every domain of the neutron energy spectrum and thus can be addressed by applying a set of correction factors,
The determination of the correction factors is explained in the following.
3.2.1 Atmospheric pressure correction
Since the cosmic-ray flux through the atmosphere is exponentially attenuated as a function of the traversed cumulative mass, measured neutron count rates can be normalized to standard atmospheric pressure by applying the standard pressure correction approach (Desilets and Zreda, 2003):
where Cp is atmospheric pressure correction factor, P0 is the reference atmospheric pressure (1013.25 hPa), P is the actual atmospheric pressure, and β=0.0076 is the barometric coefficient that is related to the local mass attenuation length of neutrons in air. We also tested the application of regionally variable values for β according to Desilets and Zreda (2003) and Desilets et al. (2006), but found only negligible variations over Europe. However, future work should further investigate the influence of local β variability on the atmospheric pressure correction.
3.2.2 Air humidity correction
We accounted for the effect of atmospheric water vapor fluctuations on neutron count rate using the approach of Rosolem et al. (2013):
with α=0.0054 and h the absolute humidity (g m−3) measured at 2 m height.
3.2.3 Incoming neutron correction
The galactic cosmic radiation, or incoming radiation I(t), that penetrates the upper atmosphere varies in time mainly due to the well-known 11-year cycle of the solar activity. At high solar power (the solar maximum), the stronger solar magnetic field deflects a larger proportion of galactic particles away from Earth and reduces I(t). Conversely, during low solar activity (the solar minimum) the weaker solar magnetic field allows more galactic protons to enter the atmosphere increasing I(t). Shorter-term fluctuations have a similar effect on I(t) but with lower amplitude. Changes in the shape of the geomagnetic field, which occur on timescales from years to decades, are of secondary importance compared to temporal fluctuations of I(t). These temporal variations are measured locally with so-called neutron monitors (NM), which are sensitive to high-energy secondary neutrons (>20 MeV) but insensitive to local environmental factors (Simpson, 2000). The incoming radiation varies also spatially with strong gradients from the pole to the Equator, corresponding to the cutoff rigidity of the Earth's magnetic field. A worldwide network of NM stations provides near-real-time access to incoming cosmic-ray data (https://www.nmdb.eu/, last access: 2 March 2022). Assuming that the incoming radiation along the rigidity lines is similar, a nearby NM should be able to provide representative data for other places on Earth with similar cutoff rigidity Rcut. The local Rcut can be estimated for individual CRNS stations using approaches provided by Bütikofer et al. (2007). Since every detector comes with an individual efficiency, the value I(t) could be normalized with an arbitrary but constant reference Iref, which we chose to be 150 cts h−1. However, NM stations are rare, representing only a few latitudes and often not providing continuous signals over long periods of time. The NM at Jungfraujoch (Switzerland) is one of the few stations that provides reliable long-term data that can be used for COSMOS stations in Europe due its central location. Hence, scaling of the Jungfraujoch signal is needed to match the widespread distribution of COSMOS stations in Europe. According to Schrön et al. (2016), the intensity correction factor can be calculated as follows:
in which I is the count rate of incoming cosmic-ray neutrons of a neutron monitoring station, Iref is the incoming count rate at an arbitrary time, and is an amplitude scaling factor to adjust for the mentioned geomagnetic effects. It depends on the cutoff rigidity of the local site and the neutron monitor used (see, e.g., Hawdon et al., 2014). For this paper, we use the approach from Hawdon et al. (2014) to bridge the regional difference of cutoff rigidities between the local site and the NM.
3.2.4 Biomass correction
Biomass can affect neutron count rates and should be considered when large temporal changes in biomass occur at a CRNS site. Therefore, we consider the biomass correction method proposed by Baatz et al. (2015) using the dry biomass B in kg m2:
This correction was applied at the Wuestebach1 site, where a large change in biomass had occurred in the CRNS footprint area due to clear-cutting of a forest. For the other sites, there were no strong biomass changes or no detailed information on biomass changes was available. As soon as changes in the biomass occur or information for a site is available, these can be taken into account.
3.3 Sensor calibration
3.3.1 In situ reference soil data
For the calibration, we used in most cases available information on gravimetrically measured soil moisture from soil samples taken within the CRNS footprint. The soil samples were weighted vertically according to Schrön et al. (2017); i.e., for each sample at depth d and penetration depth D, we evaluate the weight in the representative sample volume (d1 to d2) to generate the profile average soil moisture:
In addition, we applied horizontal weighting of the vertically averaged in situ soil moisture according to Schrön et al. (2017). For this, regions of equal contribution (annuluses of 20 % quantiles) to the neutron signal were defined depending on the local conditions (i.e., atmospheric pressure, air humidity, average soil moisture) that influence the spatial sensitivity of the CRNS. All sampling points that fall within an annulus A are arithmetically averaged and thus receive the same weights, which are calculated according to the weighting scheme of Schrön et al. (2017). More specifically, θhoriz is integrated over the entire domain to find the radii r1 and r2 that define the five annuluses A(r1, r2) within which all samples are equally averaged:
In particular, this method ensures that soil samples taken using the outdated COSMOS scheme (25, 75, 200 m), which assumed larger CRNS footprints, are not double weighted. Due to the long distances of the COSMOS sampling scheme, there may be no soil samples in one annulus. In this case, the samples in the next larger ring receive double the weight; i.e., the soil samples taken at 25 m distance are also representative for the soil moisture in the first annulus around the sensor. This problem does not arise for COSMOS-Europe sites sampled according to the revised weighting scheme of Schrön et al. (2017), as soil samples were also taken in the near field of the CRNS (i.e., 2–10 m distances). The in situ reference soil moisture of COSMOS-Europe sites and the weighted averages used for the CRNS soil moisture calibration are presented in Fig. A4.
3.3.2 Conversion of neutron count rate to soil moisture
To convert neutron count rates to soil moisture, we used the conventional relationship between neutrons and soil moisture initially introduced by Desilets et al. (2010). According to Köhli et al. (2021), it can be expressed in an equivalent but more unambiguous formulation with fewer parameters:
where Nmax is the maximum neutron flux under dry conditions which mainly depends on the individual detector sensitivity. Parameters , p1=0.346, and can be derived from the parameters used so far in the Desilets equation. Calibrated values for N0 of the COSMOS-Europe sites are given in Table 2.
Hydrogen in the organic matter as well as the lattice water content of soils affects how epithermal neutrons interact with the soil and thus affects the shape of the calibration function (Zreda et al., 2012). We accounted for this effect by fitting Nmax of the calibration to the total soil water, which is the sum of the water equivalents of lattice water and organic matter and the gravimetrically measured reference soil moisture. The volumetric soil moisture is then obtained by subtracting the lattice and organic matter water from CRNS total soil moisture multiplied with the soil density. We averaged the soil property values, in case multiple calibration dates were available. In order to increase the signal-to-noise ratio of the neutron counts we applied a moving average with a window size of 24 h for the Nmax calibration. It should be noted that a 24 h window is well suited for drought studies but may mask the signal from wetting events, which tend to occur much faster.
3.4 Soil moisture uncertainty
The statistical uncertainty of CRNS-derived soil moisture scales with the number of counts in a given period. However, this count rate is inversely related to soil moisture, so drier soils result in more accurate measurements (Desilets et al., 2010; Bogena et al., 2013). In addition, the size of the CRNS detector determines the count rate, i.e., a larger detector volume improves the count statistics and thus reduces the uncertainty of the soil moisture product (Weimar et al., 2020; Schrön et al., 2021). Different neutron detectors with different sizes and efficiencies are used in this study, so it is important to consider the CRNS-specific uncertainty (e.g., when using the data for validations). Due to the non-linearity of the neutron–soil-moisture relationship, the propagated uncertainty ±σθ is highly asymmetric (Iwema et al., 2021). For simplicity, it can be estimated by a symmetrical approximation approach suggested by Jakobi et al. (2020):
where the count rate N follows from the Desilets equation, is its Gaussian uncertainty, and p2=0.0752, p3=0.346. We provide both the symmetric and asymmetric uncertainty of the CRNS-based soil moisture products in order to facilitate applications where only one of the two options can be used. It is important to note that these stochastic uncertainty estimates do not account for other (systematic) uncertainties, e.g., due to unconsidered biomass effects (Avery et al., 2016), N0 calibration errors, and unconsidered variations in incoming neutron flux (Baroni et al., 2018), atmospheric pressure (Gugerli et al., 2019), and air humidity (Iwema et al., 2021). Please note that additional uncertainties may have occurred when filling gaps in the air pressure and humidity data with ERA5 data.
3.5 CRNS footprint radius and penetration depth
The footprint radius (i.e., R86) was obtained as the 86 % cumulative contribution quantile of the weighting functions from Schrön et al. (2017). For this, we integrated the weights up to 600 m distance considering the influences of soil moisture (as the sum of the CRNS soil moisture, lattice water, and organic carbon), air humidity, and pressure. Subsequently, we obtained the average penetration depth (i.e., D86) following Schrön et al. (2017), additionally considering the influence of soil bulk density.
3.6 Normalized quantiles of soil moisture
As suggested by Cooper et al. (2021), we use normalized quantiles to better indicate extreme soil moisture situations. First, the soil moisture values are normalized relative to the minimal and maximal observed soil moisture of the considered time series; i.e., the soil moisture values (θ) are scaled between 0 and 1 (p):
where θmin is the minimum observed soil moisture and θmax is the maximum observed soil moisture. Subsequently, p is used to obtain the quantile represented by each soil moisture value:
where θsort represents the soil moisture values sorted in increasing order and n is the total number of soil moisture observations. From Q the median of all soil moisture values (θmed) is subtracted, and the variance is scaled by dividing by the standard deviation (θSD) to obtain the normalized quantiles of soil moisture (Qnorm):
Each Qnorm is then plotted against an observed value of the CRNS estimated soil moisture.
3.7 Implementation of the data processing
The raw neutron counts and meteorological data were converted into a uniform data structure (Fig. A5b) and stored in a database within the decentralized data infrastructure TEODOOR (TEreno Online Data repOsitORy, Kunkel et al., 2013). The data pre-processing, corrections, calibration, and uncertainty estimation were implemented in the programming language Python. These scripts were applied to the raw data stored in the database using Node-RED, a graphical tool for deploying workflows (Node-RED, 2021). Node-RED offers the possibility to connect different data flows in a simple way, using so-called nodes. Each node has a defined and unique task. When data are transmitted to a node, the node can process these data and then transmit them to the next node. In this way, different corrections or other implementations in the data post-processing can be added or removed individually. As an interface for accessing the data in the TERENO database, the Sensor Observation Service (SOS) of Open Geospatial Consortium was used. Here, the data are processed by a separate proxy that forwards the requests to a virtual Python environment. In the last step, the processed data were written back directly to the database via email or SOS.
The raw data as well as the processed data are accessible via the TERENO Data Discovery Portal (DDP) at http://www.tereno.net (last access: 2 March 2022). The data portal enables the query, visualization, and access to data and metadata of the stations presented in this paper. Additionally, detailed information on each CRNS station is provided in the metadata (Fig. A5a) and can be retrieved from the data portal.
4.1 Spatiotemporal occurrence of drought events in Europe
The provision of a continental-scale dataset on soil moisture dynamics opens up numerous possibilities for analysis, especially with respect to large-scale climatic and hydrological applications. In the following, we present first analyses on the spatiotemporal occurrence of drought events in Europe based on the processed time series of CRNS soil moisture.
Figure 3 visualizes the results of the CRNS soil moisture processing for the COSMOS-Europe sites. The CRNS soil moisture (left subplot) shows strong temporal variations as well as large differences between the COSMOS-Europe sites. Due to these strong variations in CRNS soil moisture, similarities in the absolute values are difficult to discern, e.g., the impact of large-scale drought events on CRNS soil moisture. Therefore, following the approach of Cooper et al. (2021), Fig. 3 also presents the normalized quantiles of CRNS soil moisture (right subplot) to better indicate extreme soil moisture situations, i.e., to better distinguish between “normal low” soil moisture and “extremely low” soil moisture. In this way, the widespread impacts on the recent drought events of 2018, 2019, and 2020 on CRNS soil moisture in Europe become more apparent. The 2018 drought, in particular, is clearly visible with pronounced negative values in the normalized soil moisture quantiles across all latitudes, indicating that the whole of Europe was affected by the drought.
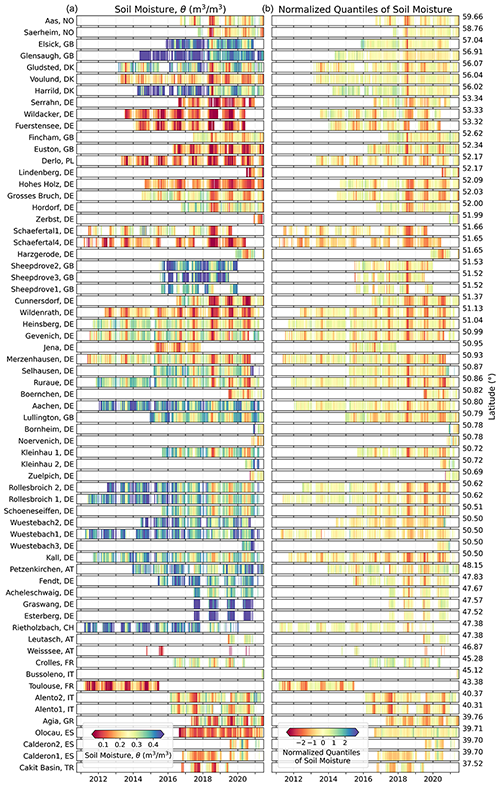
Figure 3Time series of CRNS soil moisture (a) and normalized quantiles of CRNS soil moisture (b) of the COSMOS-Europe sites ordered from north to south according to latitude (unrealistic soil moisture values are excluded, i.e., larger than porosity). The timeline shown ends in June 2021, while data and calibration dates for some sensors extend to November 2021.
In the following, we explore if CRNS soil moisture information can be a valuable basis for more accurate assessment of the uniqueness and potential impacts of drought events at regional to continental scales. In Fig. 4 the monthly mean CRNS soil moisture of all COSMOS-Europe sites since 2011 are presented, along with the spatial mean and SD (upper subplot).
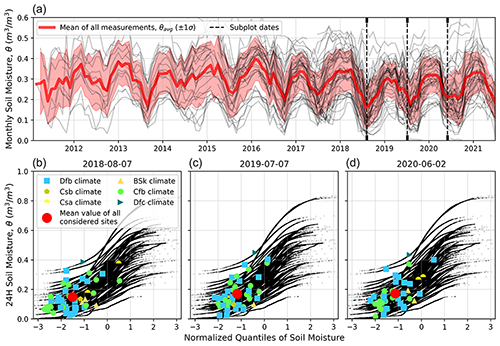
Figure 4Time series of monthly mean CRNS soil moisture (gray lines in panel a) and normalized quantiles of CRNS soil moisture of the COSMOS-Europe sites (black dots in panels b–d). For three exemplary days during recent drought periods in Europe (8 August 2018, 7 July 2019, and 2 June 2020), the normalized quantiles are highlighted and differentiated by climate zone. These days were selected as they exhibited the lowest hourly soil moisture during the drought events. The mean of normalized quantiles of CRNS soil moisture for these days is also shown.
Despite the different time series lengths, the seasonal variations in soil moisture can be clearly seen. We selected three drought events to examine differences in soil moisture between sites, with all data for the period of record presented as normalized quantiles of soil moisture for each site (Fig. 4, lower subplot). It is evident that sites even within the same climate zone with broadly similar weather patterns can have very different ranges and extremes of soil moisture.
This finding confirms results by Cooper et al. (2021) for the United Kingdom, who in particular suggested heterogeneity of soil properties as an explanation for the variabilities, and results of Dong and Ochsner (2018), who found that soil moisture at the regional scale is more controlled by soil texture than precipitation. However, when comparing the three events, it becomes evident that 2018 had more locations with pronounced extremes and that these occurred predominantly in the climate zone Cfb, while 2018 was not notably different from other drought years in the monthly soil moisture averages shown in Fig. 4 (upper subplot). This demonstrates again the advantage of normalized soil moisture quantiles for a more in-depth analysis of extreme events.
Finally, we investigated whether the CRNS data allow us to draw conclusions about longer-term trends in soil moisture in Europe. For this, we contrasted monthly mean soil moisture from 2014–2017 with monthly mean soil moisture from 2018 to 2021 in Fig. 5, using the 26 sites fully covering this period. From Fig. 5, it is evident that as of 2018, soil moisture was lower not only in the summer months but throughout the year. Although the considered soil moisture data cover only 7 years, they can be considered as an indicator of the magnitude and direction of the trend in soil water supply that Europe can expect as climate change progresses.
4.2 How representative and accessible is the soil moisture data?
The representativeness of the individual stations for the depicted land use type and geographical location is relevant, especially with regard to the validation of large-scale model applications of remote sensing products, which usually show coarser spatial resolutions and correspondingly “averaged” representation of site properties (e.g., Colliander et al., 2017; Montzka et al., 2020). In a few cases, the COSMOS-Europe sites represent larger site heterogeneity. This is reflected in particular in the resulting larger variability of soil moisture in the in situ calibration data measured for the individual sites (see Fig. A4). An example is the CRNS station at the Fuerstensee site in Germany (Rasche et al., 2021). The footprint represented by the CRNS measurement at this site includes a sand lens in the center of the footprint, which is surrounded by peat soils. The resulting heterogeneity, particularly of soil properties such as bulk density, soil organic matter content, and lattice water, challenges the harmonized data processing applied for COSMOS-Europe and leads to greater uncertainty in the derived soil moisture product, which is not easy to quantify.
While aspects such as soil heterogeneities or varying land use can be of great importance for local or CRNS methodological questions, this is rather an obstacle for large-scale questions. Especially with regard to the future development of the European network of CRNS stations, attention should be paid to the selection of sites that guarantee a high representativeness and homogeneity. With respect to the use of COSMOS-Europe data to derive conclusions about continental-scale trends in soil moisture, it is decisive that the network ensures the most representative coverage of key environmental and geographic gradients throughout Europe (e.g., altitude, climate, landforms, geology). This is currently the case only to a limited extent (see also Fig. 1). The clear majority of stations are concentrated in central Europe, while Scandinavia, eastern Europe, or the Mediterranean region in particular are covered by only very few stations. This limits the interpretability of the data, especially with regard to comparisons between different climate zones.
Another important question in this context is whether observations at a limited number of points can provide regional improvements in the prediction of hydrologic states and fluxes. For example, Baatz et al. (2017) assimilated measured soil moisture data from a CRNS network into the area-differentiated land surface model CLM 4.5 (Oleson et al., 2013) and showed that updating states and hydraulic parameters leads to better regional hydrologic predictions. This indicates that the COSMOS-Europe data could be beneficial for model applications at the continental scale despite the limited coverage in some areas of Europe, i.e., even though the measurements are not area-wide such as remote sensing data.
Furthermore, at present only a low number of CRNS stations are automatically transferring neutron count data to the TERENO database that hosts the COSMOS-Europe data. However, a near-real-time availability of the data would be necessary in particular for the use of the data for the improvement of flood models, e.g., in context of the European Flood Awareness System (EFAS, Smith et al., 2016). Efforts should be made in the future to equip more stations with automatic data transfer capabilities to enable rapid transfer of neutron counts to the TERENO database. Here, the implemented automated routines for data pre-processing, correction, and neutron counts to soil moisture allow for immediate provision of COSMOS-Europe data products.
Finally, we would like to point out that there are still alternative data products to some of the CRNS stations used here, which were processed using less comprehensive methods. Although our processing methods closely follow the commonly used method presented by Zreda et al. (2012), there are some important differences as we make use of the latest CRNS research findings from the last 10 years. For example, the US COSMOS database (http://cosmos.hwr.arizona.edu/, last access: 2 March 2022) does not contain corrections for air humidity, which were suggested by Rosolem et al. (2013) and Köhli et al. (2021). Another main difference of our processing scheme is the weighting of the in situ calibration data according to neutron transport theory (Schrön et al., 2017). We therefore expect soil moisture deviations that can be substantial depending on the date of previous processing schemes.
The dataset, entitled “Dataset of COSMOS-Europe: A European network of Cosmic-Ray Neutron Soil Moisture Sensors”, is stored in a common data format and shared via Forschungszentrum Jülich (https://teodoor.icg.kfa-juelich.de/ibg3butt/ibg.butt.download?FileIdentifier=519e0691-7eb3-4351-aff5-c0a0335933ab, last access: 24 September 2021); https://doi.org/10.34731/x9s3-kr48 (Bogena and Ney, 2021).
Potential users can also access the data of the individual CRNS stations in a dedicated section for COSMOS-Europe in the TERENO data portal TEODOOR at https://ddp.tereno.net/ddp/dispatch?searchparams=keywords-Cosmic%20Ray (last access: 2 March 2022). Here, metadata information about the stations (e.g., site owner) as well as the raw data and the processed data products can be accessed. Please note that downloads will only be made possible via a token and are provided with a disclaimer with the terms of use.
In this data paper, we present soil moisture data from 65 CRNS stations that are distributed across Europe and cover all major land use types and climate zones. The raw neutron count data from the CRNS stations were processed using state-of-the-art methods in a harmonized way including correction of the raw neutron counts and conversion into soil moisture based on available in situ information. In addition, information on the data uncertainty is added to the dataset – information that is particularly useful for remote sensing and modeling applications. It should be noted that the sites have individual heterogeneous conditions, which cannot always be adequately reflected by a standard processing scheme. In addition, the data processing used in this work represents the state of the art, but this may change as a result of future research. We therefore provide raw data and will update the published dataset with an incremental version number if new processing procedures become accepted in the future.
We show that the COSMOS-Europe dataset enables a good representation of the magnitude and distribution of drought events. However, so far, only the central part of Europe is particularly well covered by COSMOS-Europe, while there are still large gaps in the peripheral areas of Europe. The density of COSMOS stations in Europe is still not sufficient to completely represent soil moisture patterns across all parts of the continent. Thus, future efforts should invest in higher observational coverage. One emphasis in the further development of COSMOS-Europe must be to convince countries to put CRNS stations into operation that do not yet operate CRNS stations or hardly any. In addition, efforts should be made in the future to equip more stations with automatic data transfer capabilities to enable near-real-time accessibility of soil moisture information, e.g., to support flood forecasting. The data presented here can be used for a manifold of hydrological applications, such as drought assessment, flood risk assessment, and snow water estimation.
Similar to COSMOS-Europe, several other large-scale COSMOS networks already exist in the USA, Australia, and India. The obvious next step is to build on the methods developed in this study to create a global network of continental COSMOS networks, similar to the FLUXNET initiative for eddy covariance measurements of land–atmosphere exchange fluxes (FLUXNET, 2021) or the International Soil Moisture Network (ISMN) that provides in situ soil moisture data from 2842 stations worldwide (Dorigo et al., 2021). Initial networking efforts in this direction have already been undertaken.
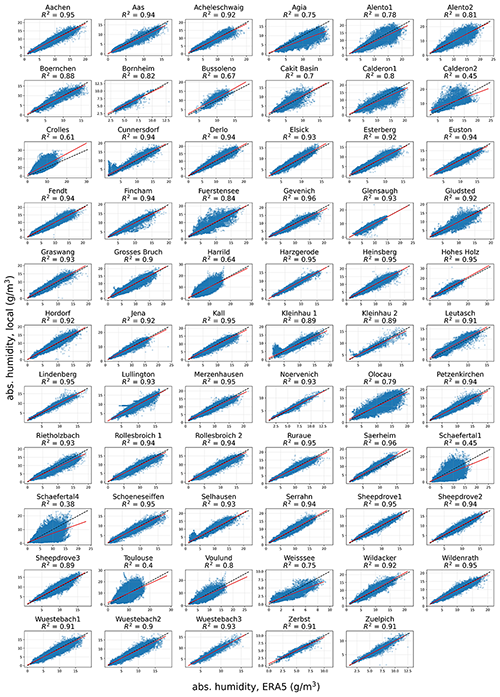
Figure A1The correlations between air humidity from local measurements and ERA5 for the COSMOS-Europe sites.
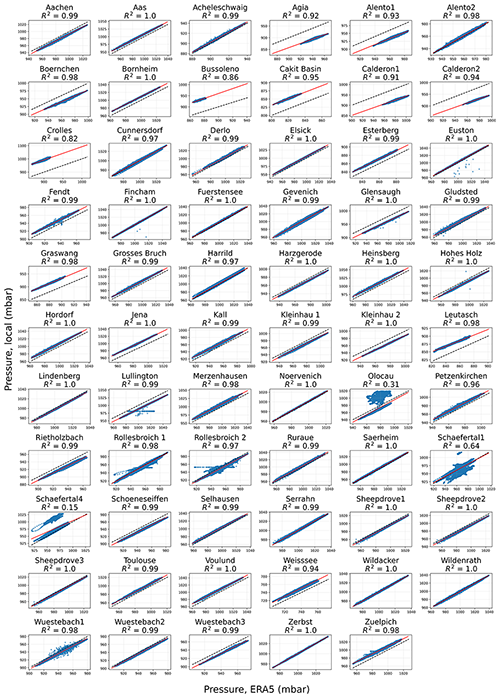
Figure A2The correlations between atmospheric pressure from local measurements and ERA5 for the COSMOS-Europe sites.
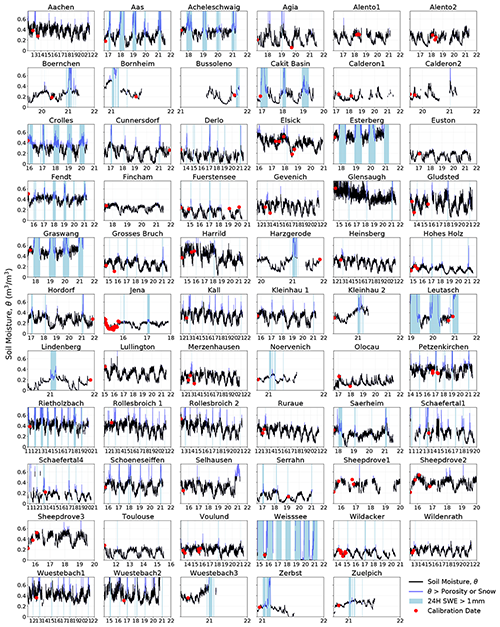
Figure A3Detected unrealistic CRNS soil moisture estimates due the presence of snow at the site (i.e., times of snow water equivalent from ERA5 larger than 1 mm) and soil moisture values exceeding the local soil porosity.
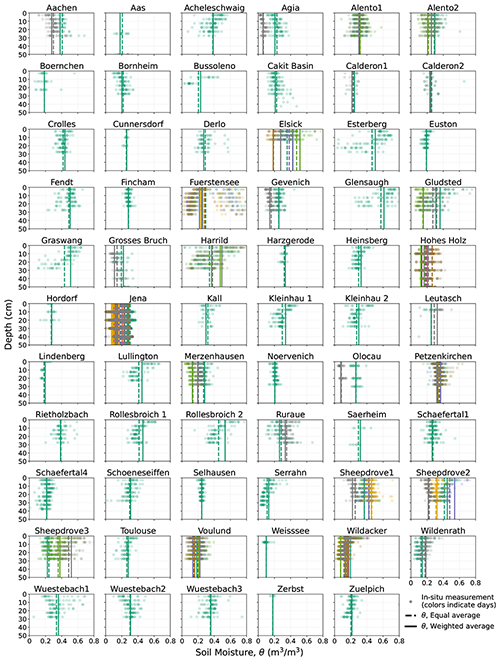
Figure A4Soil profiles of the in situ calibration data for the COSMOS-Europe sites. The weighted average soil moisture values are also shown. The varying colors indicate the different sampling dates.
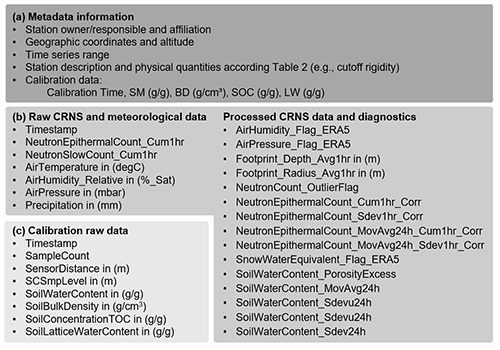
Figure A5Data structure in the TERENO Data Discovery Portal (DDP). Each station comprises metadata (a) with detailed site information and two time series. One time series contains the raw CRNS data, the meteorological data, and the processed data with the associated diagnostics (b). The second time series provides the raw calibration data (c).
HRB, MS, JJ, PNe, SZ, and HV conceptualized the study. HRB and MS led the data compilation and editing of the manuscript. Algorithm development was provided by HRB, MS, JJ, SZ, and PNe. JJ wrote the scripts for data pre-processing, corrections, calibration, and uncertainty estimation and performed the formal analysis. Programming and analysis have been supported by PNe and MS. PNe provided the map visualizations. PNe and TK developed the database within the decentralized data infrastructure TEODOOR. COSMOS-Europe stations were maintained and data were provided by HRB, MS, JJ, PNe, MA, RB, DB, MBD, MAEG, BF, TF, JG, MGS, YK, TK, ZM, AM, PNa, JN, VP, DR, RR, HS, PS, MZ, SA, EAH, ZA, TB, AdC, DC, KDP, JGE, SF, FF, DG, AG, FH, JI, KHJ, HK, AL, MCL, SO, CR, AnP, AmP, DP, NR, LMS, SS, and GW. HRB wrote the first manuscript draft. Further writing of the draft was performed by HRB, MS, JJ, PNe, and SZ. All authors contributed to editing the final version of the manuscript.
The contact author has declared that neither they nor their co-authors have any competing interests.
Publisher’s note: Copernicus Publications remains neutral with regard to jurisdictional claims in published maps and institutional affiliations.
We thank Peter Strauss and Gerhab Rab from the Institute for Land and Water Management Research, Federal Agency for Water Management Austria, Petzenkirchen, Austria. We thank Trenton Franz from the School of Natural Resources, University of Nebraska–Lincoln, Lincoln, NE, United States. We also thank Carmen Zengerle, Mandy Kasner, Felix Pohl, and Solveig Landmark, UFZ Leipzig, for supporting field calibration, lab analysis, and data processing. We furthermore thank Daniel Dolfus, Marius Schmidt, Ansgar Weuthen, and Bernd Schilling, Forschungszentrum Jülich, Germany. The COSMOS-UK project team is thanked for making its data available to COSMOS-Europe. Luca Stevanato is thanked for the technical details about the Finapp sensor. The stations at Cunnersdorf, Lindenberg, and Harzgerode have been supported by Falk Böttcher, Frank Beyrich, and Petra Fude, German Weather Service (DWD). The Zerbst site has been supported by Getec Green Energy GmbH and Jörg Kachelmann (Meteologix AG). The CESBIO sites have been supported by the CNES TOSCA program. The ERA5-Land data are provided by ECMWF (Muñoz Sabater, 2021). The Jena dataset was retrieved at the site of The Jena Experiment, operated by DFG research unit FOR 1451.
We thank TERENO (Terrestrial Environmental Observatories), funded by the Helmholtz-Gemeinschaft for the financing and maintenance of CRNS stations. We acknowledge financial support by the Deutsche Forschungsgemeinschaft (DFG, German Research Foundation) of the research unit FOR 2694 Cosmic Sense (grant no. 357874777) and by the German Federal Ministry of Education of the Research BioökonomieREVIER, Digitales Geosystem – Rheinisches Revier project (grant no. 031B0918A). COSMOS-UK has been supported financially by the UK’s Natural Environment Research Council (grant no. NE/R016429/1). The Olocau experimental watershed is partially supported by the Spanish Ministry of Science and Innovation through the research project TETISCHANGE (grant no. RTI2018-093717-B-I00). The Calderona experimental site is partially supported by the Spanish Ministry of Science and Innovation through the research projects CEHYRFO-MED (grant no. CGL2017-86839-C3-2-R) and SILVADAPT.NET (grant no. RED2018-102719-T) and the LIFE project RESILIENT FORESTS (grant no. LIFE17 CCA/ES/000063). The University of Bristol’s Sheepdrove sites have been supported by the UK’s Natural Environment Research Council through a number of projects (grant nos. NE/M003086/1, NE/R004897/1, and NE/T005645/1) and by the International Atomic Energy Agency of the United Nations (grant no. CRP D12014).
This paper was edited by Alexander Gruber and reviewed by three anonymous referees.
Andreasen, M., Jensen, K. H., Desilets, D., Franz, T., Zreda, M., Bogena, H., and Looms, M. C.: Status and perspectives of the cosmic-ray neutron method for soil moisture estimation and other environmental science applications, Vadose Zone J., 16, 1–11, https://doi.org/10.2136/vzj2017.04.0086, 2017a.
Andreasen, M., Jensen, K. H., Desilets, D., Zreda, M., Bogena, H. R., and Looms, M. C.: Cosmic-ray neutron transport at a forest field site: the sensitivity to various environmental conditions with focus on biomass and canopy interception, Hydrol. Earth Syst. Sci., 21, 1875–1894, https://doi.org/10.5194/hess-21-1875-2017, 2017b.
Andreasen, M., Looms, M. C., and Jensen, K. H.: Cosmic-ray neutron intensity and soil moisture estimates in the period 2013–2019 at three field sites located in the western part of Denmark, PANGAEA [data set], https://doi.org/10.1594/PANGAEA.909271, 2019.
Andreasen, M., Jensen, K. H., Bogena, H., Desilets, D., Zreda, M., and Looms, M. C.: Cosmic ray neutron soil moisture estimation using physically based site-specific conversion functions, Water Resour. Res., 56, e2019WR026588, https://doi.org/10.1029/2019WR026588, 2020.
Avery, W. A., Finkenbiner, C., Franz, T. E., Wang, T., Nguy-Robertson, A. L., Suyker, A., Arkebauer, T., and Muñoz-Arriola, F.: Incorporation of globally available datasets into the roving cosmic-ray neutron probe method for estimating field-scale soil water content, Hydrol. Earth Syst. Sci., 20, 3859–3872, https://doi.org/10.5194/hess-20-3859-2016, 2016.
Baatz, R., Bogena, H. R., Hendricks Franssen, H. J., Huisman, J. A., Qu, W., Montzka, C., and Vereecken, H.: Calibration of a catchment scale cosmic-ray probe network: A comparison of three parameterization methods. J. Hydrol., 516, 231–244, https://doi.org/10.1016/j.jhydrol.2014.02.026, 2014.
Baatz, R., Bogena, H. R., Hendricks Franssen, H.-J., Huisman, J. A., Montzka, C., and Vereecken, H.: An empirical vegetation correction for soil moisture content quantification using cosmic ray probes, Water Resour. Res., 51, 2030–2046, https://doi.org/10.1002/2014WR016443, 2015.
Baatz, R., Hendricks Franssen, H.-J., Han, X., Hoar, T., Bogena, H. R., and Vereecken, H.: Evaluation of a cosmic-ray neutron sensor network for improved land surface model prediction, Hydrol. Earth Syst. Sci., 21, 2509–2530, https://doi.org/10.5194/hess-21-2509-2017, 2017.
Baroni, G., Scheiffele, L. M., Schrön, M., Ingwersen, J., and Oswald, S. E.: Uncertainty, sensitivity and improvements in soil moisture estimation with cosmic-ray neutron sensing, J. Hydrol., 564, 873–887, https://doi.org/10.1016/j.jhydrol.2018.07.053, 2018.
Beck, H. E., Zimmermann, N. E., McVicar, T. R., Vergopolan, N., Berg, A., and Wood, E. F.: Present and future Köppen-Geiger climate classification maps at 1-km resolution, Sci. Data., 5, 180214, https://doi.org/10.1038/sdata.2018.214, 2018.
Berthelin, R., Rinderer, M., Andreo, B., Baker, A., Kilian, D., Leonhardt, G., Lotz, A., Lichtenwoehrer, K., Mudarra, M., Padilla, I. Y., Pantoja Agreda, F., Rosolem, R., Vale, A., and Hartmann, A.: A soil moisture monitoring network to characterize karstic recharge and evapotranspiration at five representative sites across the globe, Geosci. Instrum. Method. Data Syst., 9, 11–23, https://doi.org/10.5194/gi-9-11-2020, 2020.
Blöschl, G., Blaschke, A. P., Broer, M., Bucher, C., Carr, G., Chen, X., Eder, A., Exner-Kittridge, M., Farnleitner, A., Flores-Orozco, A., Haas, P., Hogan, P., Kazemi Amiri, A., Oismüller, M., Parajka, J., Silasari, R., Stadler, P., Strauss, P., Vreugdenhil, M., Wagner, W., and Zessner, M.: The Hydrological Open Air Laboratory (HOAL) in Petzenkirchen: a hypothesis-driven observatory, Hydrol. Earth Syst. Sci., 20, 227–255, https://doi.org/10.5194/hess-20-227-2016, 2016.
Bogena, H., and Ney, P.: COSMOS-Europe: A European network of Cosmic-Ray Neutron Soil Moisture Sensors, Forschungszentrum Jülich, https://doi.org/10.34731/x9s3-kr48, data available at: https://teodoor.icg.kfa-juelich.de/ibg3butt/ibg.butt.download?FileIdentifier=519e0691-7eb3-4351-aff5-c0a0335933ab or https://ddp.tereno.net/ddp/dispatch?searchparams=keywords-Cosmic%20Ray, last access: 24 September 2021.
Bogena, H. R., Huisman, J. A., Baatz, R., Hendricks Franssen, H.-J., and Vereecken, H.: Accuracy of the cosmic-ray soil water content probe in humid forest ecosystems: The worst case scenario, Water Resour. Res., 49, 1–14, https://doi.org/10.1002/wrcr.20463, 2013.
Bogena, H. R., Huisman, J. A., Güntner, A., Hübner, C., Kusche, J., Jonard, F., Vey, S., and Vereecken, H.: Emerging methods for noninvasive sensing of soil moisture dynamics from field to catchment scale: A review, WiRes Water, 2, 635–647, https://doi.org/10.1002/wat2.1097, 2015.
Bogena, H. R., Montzka, C., Huisman, J. A., Graf, A., Schmidt, M., Stockinger, M., von Hebel, C., Hendricks-Franssen, H. J., van der Kruk, J., Tappe, W., Lücke, A., Baatz, R., Bol, R., Groh, J., Pütz, T., Jakobi, J., Kunkel, R., Sorg, J., and Vereecken, H.: The Rur Hydrological Observatory: A multiscale multi-compartment research platform for the advancement of hydrological science, Vadose Zone J., 17, 180055, https://doi.org/10.2136/vzj2018.03.0055, 2018.
Bogena, H. R., Herrmann, F., Jakobi, J., Brogi, C., Ilias, A., Huisman, J. A., Panagopoulos, A., and Pisinaras, V.: Monitoring of Snowpack Dynamics With Cosmic-Ray Neutron Probes: A Comparison of Four Conversion Methods, Front. Water, 2, 19, https://doi.org/10.3389/frwa.2020.00019, 2020.
Brunetti, G., Šimůnek, J., Bogena, H., Baatz, R., Huisman, J. A., Dahlke, H., and Vereecken, H.: On the information content of cosmic-ray neutron data in the inverse estimation of soil hydraulic properties, Vadose Zone J., 18, 1–24, https://doi.org/10.2136/vzj2018.06.0123, 2019.
Bütikofer, R., Fluckiger, E. O., and Desorgher, L.: Characteristics of near real-time cutoff calculations on a local and global scale, in: Proceedings of the 30th International Cosmic Ray Conference, edited by: Caballero, R., D'Olivo, R. C., Medina-Tanco, G., Nellen, L., Sánchez, F. A. and Valdés-Galicia, J. F., Universidad Nacional Autónoma de México, Mexico City, Mexico, 2008, 1 (SH), 769-772, 2007.
Colliander, A., Jackson, T. J., Bindlish, R., Chan, S., Das, N., Kim, S. B., Cosh, M. H., Dunbar, R. S., Dang, L., Pashaian, L., Asanuma, J., Aida, K., Berg, A., Rowlandson, T., Bosch, D., Caldwell, T., Caylor, K., Goodrich, D., al Jassar, H., Lopez-Baeza, E., Martinez-Fernandez, J., Gonzalez-Zamora, A., Livingston, S., McNairn, H., Pacheco, A., Moghaddam, M., Montzka, C., Notarnicola, C., Niedrist, G., Pellarin, T., Prueger, J., Pulliainen, J., Rautiainen, K., Ramos, J., Seyfried, M., Starks, P., Su, Z., Zeng, Y., van der Velde, R., Thibeault, M., Dorigo, W., Vreugdenhil, M., Walker, J. P., Wu, X., Monerris, A., O'Neill, P. E., Entekhabi, D., Njoku, E. G., and Yueh, S.: Validation of SMAP surface soil moisture products with core validation sites, Remote Sens. Environ., 191, 215–231, 2017.
Cooper, H. M., Bennett, E., Blake, J., Blyth, E., Boorman, D., Cooper, E., Evans, J., Fry, M., Jenkins, A., Morrison, R., Rylett, D., Stanley, S., Szczykulska, M., Trill, E., Antoniou, V., Askquith-Ellis, A., Ball, L., Brooks, M., Clarke, M. A., Cowan, N., Cumming, A., Farrand, P., Hitt, O., Lord, W., Scarlett, P., Swain, O., Thornton, J., Warwick, A., and Winterbourn, B.: COSMOS-UK: national soil moisture and hydrometeorology data for environmental science research, Earth Syst. Sci. Data, 13, 1737–1757, https://doi.org/10.5194/essd-13-1737-2021, 2021.
Desilets, D. and Zreda, M.: Spatial and temporal distribution of secondary cosmic-ray nucleon intensities and applications to in situ cosmogenic dating, Earth Planet. Sci. Lett., 206, 21–42, 2003.
Desilets, D., Zreda, M., and Prabu, T.: Extended scaling factors for in situ cosmogenic nuclides: new measurements at low latitude, Earth Planet. Sc. Lett., 246, 265–276, https://doi.org/10.1016/j.epsl.2006.03.051, 2006.
Desilets, D., Zreda, M., and Ferré, T. P. A.: Nature's neutron probe: Land surface hydrology at an elusive scale with cosmic rays, Water Resour. Res., 46, W11505, https://doi.org/10.1029/2009WR008726, 2010.
Dimitrova-Petrova, K., Geris, J., Wilkinson, M. E., Rosolem, R., Verrot, L., Lilly, A., and Soulsby, C.: Opportunities and challenges in using catchment-scale storage estimates from cosmic ray neutron sensors for rainfall-runoff modelling, J. Hydrol., 586, 124878, https://doi.org/10.1016/j.jhydrol.2020.124878, 2020a.
Dimitrova-Petrova, K., Geris, J., Wilkinson, M. E., Lilly, A., and Soulsby, C.: Using isotopes to understand the evolution of water ages in disturbed mixed land-use catchments, Hydrol. Proc., 34, 972–990, https://doi.org/10.1002/hyp.13627, 2020b.
Dimitrova-Petrova, K., Rosolem, R., Soulsby, C., Wilkinson, M. E., Lilly, A., and Geris J.: Combining static and portable Cosmic Ray Neutron Sensor data to assess catchment scale heterogeneity in soil water storage and their integrated role in catchment runoff response, J. Hydrol., 601, 126659, https://doi.org/10.1016/j.jhydrol.2021.126659, 2021.
Dong, J. and Ochsner, T. E.: Soil texture often exerts a stronger influence than precipitation on mesoscale soil moisture patterns, Water Resour. Res., 54, 2199–2211, https://doi.org/10.1002/2017WR021692, 2018.
Dorigo, W., Himmelbauer, I., Aberer, D., Schremmer, L., Petrakovic, I., Zappa, L., Preimesberger, W., Xaver, A., Annor, F., Ardö, J., Baldocchi, D., Bitelli, M., Blöschl, G., Bogena, H., Brocca, L., Calvet, J.-C., Camarero, J. J., Capello, G., Choi, M., Cosh, M. C., van de Giesen, N., Hajdu, I., Ikonen, J., Jensen, K. H., Kanniah, K. D., de Kat, I., Kirchengast, G., Kumar Rai, P., Kyrouac, J., Larson, K., Liu, S., Loew, A., Moghaddam, M., Martínez Fernández, J., Mattar Bader, C., Morbidelli, R., Musial, J. P., Osenga, E., Palecki, M. A., Pellarin, T., Petropoulos, G. P., Pfeil, I., Powers, J., Robock, A., Rüdiger, C., Rummel, U., Strobel, M., Su, Z., Sullivan, R., Tagesson, T., Varlagin, A., Vreugdenhil, M., Walker, J., Wen, J., Wenger, F., Wigneron, J. P., Woods, M., Yang, K., Zeng, Y., Zhang, X., Zreda, M., Dietrich, S., Gruber, A., van Oevelen, P., Wagner, W., Scipal, K., Drusch, M., and Sabia, R.: The International Soil Moisture Network: serving Earth system science for over a decade, Hydrol. Earth Syst. Sci., 25, 5749–5804, https://doi.org/10.5194/hess-25-5749-2021, 2021.
Duygu, M. B. and Akyürek, Z.: Using cosmic-ray neutron probes in validating satellite soil moisture products and land surface models, Water, 11, 1362, https://doi.org/10.3390/w11071362, 2019.
DWD – Deutscher Wetterdienst: Lindenberg Meteorological Observatory – Richard Assmann Observatory, Lindenberg, Germany, https://www.dwd.de/SharedDocs/broschueren/EN/press/mo_lindenberg_en.pdf?__blob=publicationFile&v=3, last access: 10 September 2021.
Evans, J. G., Ward, H. C., Blake, J. R., Hewitt, E. J., Morrison, R., Fry, M., Ball, L. A., Doughty, L. C., Libre, J. W., Hitt, O. E., Rylett, D., Ellis, R. J., Warwick, A. C., Brooks, M., Parkes, M. A., Wright, G. M. H., Singer, A. C., Boorman, D. B., and Jenkins, A.: Soil water content in southern England derived from a cosmic ray soil moisture observing system: COSMOS-UK, Hydrol. Process., 30, 4987–4999, https://doi.org/10.1002/hyp.10929, 2016.
Fersch, B., Francke, T., Heistermann, M., Schrön, M., Döpper, V., Jakobi, J., Baroni, G., Blume, T., Bogena, H., Budach, C., Gränzig, T., Förster, M., Güntner, A., Hendricks Franssen, H.-J., Kasner, M., Köhli, M., Kleinschmit, B., Kunstmann, H., Patil, A., Rasche, D., Scheiffele, L., Schmidt, U., Szulc-Seyfried, S., Weimar, J., Zacharias, S., Zreda, M., Heber, B., Kiese, R., Mares, V., Mollenhauer, H., Völksch, I., and Oswald, S.: A dense network of cosmic-ray neutron sensors for soil moisture observation in a highly instrumented pre-Alpine headwater catchment in Germany, Earth Syst. Sci. Data, 12, 2289–2309, https://doi.org/10.5194/essd-12-2289-2020, 2020.
Fink, A. H., Brücher, T., Krüger, A., Leckebusch, G. C., Pinto, J. G., and Ulbrich, U.: The 2003 European summer heatwaves and drought-synoptic diagnosis and impacts, Weather, 59, 209–216, 2004.
Finkenbiner, C. E., Franz, T. E., Gibson, J., Heeren, D. M., and Luck, J.: Integration of hydrogeophysical datasets and empirical orthogonal functions for improved irrigation water management, Precis. Agric., 20, 78–100, https://doi.org/10.1007/s11119-018-9582-5, 2019.
Fischer, C., Tischer, J., Roscher, C., Eisenhauer, N., Ravenek, J., Gleixner, G., Attinger, S., Jensen, B., de Kroon, H., Mommer, L., Scheu, S., and Hildebrandt, A.: Plant species diversity affects infiltration capacity in an experimental grassland through changes in soil properties, Plant Soil, 397, 1–16, https://doi.org/10.1007/s11104-014-2373-5, 2015.
Fischer, C., Leimer, S., Roscher, C., Ravenek, J., de Kroon, H., Kreutziger, Y., Baade, J., Bessler, H., Eisenhauer, N., Weigelt, A., Mommer, L., Lange, M., Gleixner, G., Wilcke, W., Schroder, B., and Hildebrandt, A.: Plant species richness and functional groups have different effects on soil water content in a decade-long grassland experiment, J. Ecol., 107, 127–141, https://doi.org/10.1111/1365-2745.13046, 2019.
FLUXNET: The data portal serving the FLUXNET community, FLUXNET, https://fluxnet.org/, last access: 10 December 2021.
Franz, T. E., Zreda, M., Rosolem, R., and Ferre, T. P. A.: A universal calibration function for determination of soil moisture with cosmic-ray neutrons, Hydrol. Earth Syst. Sci., 17, 453–460, https://doi.org/10.5194/hess-17-453-2013, 2013.
Franz, T. E., Wahbi, A., Vreugdenhil, M., Weltin, G., Heng, L., Oismueller, M., Strauss, P., Dercon, G., and Desilets, D.: Using cosmic-ray neutron probes to monitor landscape scale soil water content in mixed land use agricultural systems, Appl. Environ. Soil Sci., 2016, 4323742, https://doi.org/10.1155/2016/4323742, 2016.
Franz, T. E., Wahbi, A., Zhang, J., Vreugdenhil, M., Heng, L., Dercon, G., and Wagner, W.: Practical data products from cosmic-ray neutron sensing for hydrological applications, Front. Water, 2, 1–13, https://doi.org/10.3389/frwa.2020.00009, 2020.
Franz, T. E., Wahbi, A., Zhang, J., Vreugdenhil, M., Heng, L., Dercon, G., Strauss, P., Brocca, L., and Wagner, W.: Practical Data Products From Cosmic-Ray Neutron Sensing for Hydrological Applications, Front. Water, 2, 9, https://doi.org/10.3389/frwa.2020.00009, 2020.
Gardner, W. and Kirkham, D.: Determination of soil moisture by neutron scattering, Soil Sci., 73, 391–402, 1952.
González-Sanchis, M., García-Soro, J. M., Molina, A. J., Lidón, A. L., Bautista, I., Rouzic, E., Bogena, H., Hendricks Franssen, H.-J. and del Campo, A. D.: Comparison of soil water estimates from cosmic-ray neutron and capacity sensors in a semi-arid pine forest: Which is able to better assess the role of environmental conditions and thinning?, Front. Water, 2, 39, https://doi.org/10.3389/frwa.2020.552508, 2020.
Graf, A., Klosterhalfen, A., Arriga, N., Bernhofer, C., Bogena, H., Bornet, F., Bruggemann, N., Brummer, C., Buchmann, N., Chi, J.S., Chipeaux, C., Cremonese, E., Cuntz, M., Dusek, J., El-Madany, T.S., Fares, S., Fischer, M., Foltynova, L., Gharun, M., Ghiasi, S., Gielen, B., Gottschalk, P., Grunwald, T., Heinemann, G., Heinesch, B., Heliasz, M., Holst, J., Hortnagl, L., Ibrom, A., Ingwersen, J., Jurasinski, G., Klatt, J., Knohl, A., Koebsch, F., Konopka, J., Korkiakoski, M., Kowalska, N., Kremer, P., Kruijt, B., Lafont, S., Leonard, J., De Ligne, A., Longdoz, B., Loustau, D., Magliulo, V., Mammarella, I., Manca, G., Mauder, M., Migliavacca, M., Molder, M., Neirynck, J., Ney, P., Nilsson, M., Paul-Limoges, E., Peichl, M., Pitacco, A., Poyda, A., Rebmann, C., Roland, M., Sachs, T., Schmidt, M., Schrader, F., Siebicke, L., Sigut, L., Tuittila, E. S., Varlagin, A., Vendrame, N., Vincke, C., Volksch, I., Weber, S., Wille, C., Wizemann, H. D., Zeeman, M., and Vereecken, H.: Altered energy partitioning across terrestrial ecosystems in the European drought year 2018, Philos. T. Roy. Soc. B, 375, 20190524, https://doi.org/10.1098/rstb.2019.0524, 2020.
Grumm, R. H.: The central European and Russian heat event of July–August 2010, Bull. Am. Meteorol. Soc., 92, 1285–1296, https://doi.org/10.1175/2011BAMS3174.1, 2011.
Gugerli, R., Salzmann, N., Huss, M., and Desilets, D.: Continuous and autonomous snow water equivalent measurements by a cosmic ray sensor on an alpine glacier, The Cryosphere, 13, 3413–3434, https://doi.org/10.5194/tc-13-3413-2019, 2019.
Hanel, M., Rakovec, O., Markonis, Y., Máca, P., Samaniego, L., Kyselý, J., and Kumar, R.: Revisiting the recent European droughts from a long-term perspective, Sci. Rep., 8, 9499, https://doi.org/10.1038/s41598-018-27464-4, 2018.
Hawdon, A., McJannet, D., and Wallace, J.: Calibration and correction procedures for cosmic-ray neutron soil moisture probes located across Australia, Water Resour. Res., 50, 5029–5043, https://doi.org/10.1002/2013WR015138, 2014.
Heidbüchel, I., Güntner, A., and Blume, T.: Use of cosmic-ray neutron sensors for soil moisture monitoring in forests, Hydrol. Earth Syst. Sci., 20, 1269–1288, https://doi.org/10.5194/hess-20-1269-2016, 2016.
Heinrich, I., Balanzategui, D., Bens, O., Blasch, G., Blume, T., Böttcher, F., Borg, E., Brademann, B., Brauer, A., Conrad, C., Dietze, E., Drager, N., Fiener, P., Gerke, H.H., Guntner, A., Heine, I., Helle, G., Herbrich, M., Harfenmeister, K., Heussner, K. U., Hohmann, C., Itzerott, S., Jurasinski, G., Kaiser, K., Kappler, C., Koebsch, F., Liebner, S., Lischeid, G., Merz, B., Missling, K. D., Morgner, M., Pinkerneil, S., Plessen, B., Raab, T., Ruhtz, T., Sachs, T., Sommer, M., Spengler, D., Stender, V., Stuve, P., and Wilken, F.: Interdisciplinary geo-ecological research across time scales in the Northeast German Lowland Observatory (TERENO-NE), Vadose Zone J., 17, 180116, https://doi.org/10.2136/vzj2018.06.0116, 2018.
Hengl, T., Mendes de Jesus, J., Heuvelink, G. B., Ruiperez Gonzalez, M., Kilibarda, M., Blagotić, A., Shangguan, W., Wright, M. N., Geng, X. Y., Bauer-Marschallinger, B., Guevara, M. A., Vargas, R., MacMillan, R. A., Batjes, N. H., Leenaars, J. G. B., Ribeiro, E., Wheeler, I., Mantel, S., and Kempen, B.: SoilGrids250m: Global gridded soil information based on machine learning. PLoS one, 12, e0169748, https://doi.org/10.1371/journal.pone.0169748, 2017.
Hisdal, H., Stahl, K., Tallaksen, L. M., and Demuth, S.: Have streamflow droughts in Europe become more severe or frequent?, Int. J. Climatol., 21, 317–333, https://doi.org/10.1002/joc.619, 2001.
Humphrey, V., Berg, A., Ciais, P. Gentine, P., Jung, M., Reichstein, M., Seneviratne, S. I., and Frankenberg, C.: Soil moisture – atmosphere feedback dominates land carbon uptake variability, Nature 592, 65–69, https://doi.org/10.1038/s41586-021-03325-5, 2021.
Ionita, M., Tallaksen, L. M., Kingston, D. G., Stagge, J. H., Laaha, G., Van Lanen, H. A. J., Scholz, P., Chelcea, S. M., and Haslinger, K.: The European 2015 drought from a climatological perspective, Hydrol. Earth Syst. Sci., 21, 1397–1419, https://doi.org/10.5194/hess-21-1397-2017, 2017.
Iwema, J.: Opportunities and Limitations of the Cosmic-Ray Neutron Soil Moisture Sensor under Humid Conditions, PhD-thesis, University of Bristol, UK, https://ethos.bl.uk/OrderDetails.do?uin=uk.bl.ethos.738236 (last access: 1 March 2022), 2017.
Iwema, J., Schrön, M., Koltermann Da Silva, J., Schweiser De Paiva Lopes, R., and Rosolem, R.: Accuracy and precision of the cosmic-ray neutron sensor for soil moisture estimation at humid environments, Hydrol. Proc., 35, e14419, https://doi.org/10.1002/hyp.14419, 2021.
Jakobi, J., Huisman, J. A., Vereecken, H., Diekkrüger, B., and Bogena H. R.: Cosmic-ray neutron sensing for simultaneous soil water content and biomass quantification in drought conditions, Water Resour. Res., 54, 7383–7402, https://doi.org/10.1029/2018WR022692, 2018.
Jakobi, J., Huisman, J. A., Schrön, M., Fiedler, J., Brogi, C., and Bogena, H.: Error estimation for soil moisture measurements from with cosmic-ray neutron sensing and with implications for rover surveys, Front. Water, 2, 10, https://doi.org/10.3389/frwa.2020.00010, 2020.
Kiese, R., Fersch, B., Baessler, C., Brosy, C., Butterbach-Bahl, K., Chwala, C., Dannenmann, M., Fu, J., Gasche, R., Grote, R., Jahn, C., Klatt, J., Kunstmann, H., Mauder, M., Roediger, T., Smiatek, G., Soltani, M., Steinbrecher, R., Voelksch, I., Werhahn, J., Wolf, B., Zeeman, M., and Schmid, H. P.: The TERENO Pre-Alpine Observatory: Integrating meteorological, hydrological and biogeochemical measurements and modeling, Vadose Zone J., 17, 180060, https://doi.org/10.2136/vzj2018.03.0060, 2018.
Köhli, M., Schrön, M., Zreda, M., Schmidt, U., Dietrich, P., and Zacharias, S.: Footprint characteristics revised for field-scale soil moisture monitoring with cosmic-ray neutrons, Water Resour. Res., 51, 5772–5790, https://doi.org/10.1002/2015WR017169, 2015.
Köhli, M., Weimar, J., Schrön, M., and Schmidt, U.: Soil moisture and air humidity dependence of the above-ground cosmic-ray neutron intensity, Front. Water, 2, 544847, https://doi.org/10.3389/frwa.2020.544847, 2020.
Köhli, M., Weimar, J., Schrön, M., Baatz, R., and Schmidt, U.: Soil moisture and air humidity dependence of the above-ground cosmic-ray neutron intensity, Front. Water, 2, 66, https://doi.org/10.3389/frwa.2020.544847, 2021.
Kornhuber, K., Osprey, S., Coumou, D., Petri, S., Petoukhov, V., Rahmstorf, S., and Gray, L.: Extreme weather events in early summer 2018 connected by a recurrent hemispheric wave-7 pattern, Environ. Res. Lett., 14, 054002, https://doi.org/10.1088/1748-9326/ab13bf, 2019.
Kunkel, R., Sorg, J., Eckardt, R., Kolditz, O., Rink, K., and Vereecken, H.: TEODOOR: a distributed geodata infrastructure for terrestrial observation data, Environ. Earth Sci., 2, 507–521, https://doi.org/10.1007/s12665-013-2370-7, 2013.
Laaha, G., Gauster, T., Tallaksen, L. M., Vidal, J.-P., Stahl, K., Prudhomme, C., Heudorfer, B., Vlnas, R., Ionita, M., Van Lanen, H. A. J., Adler, M.-J., Caillouet, L., Delus, C., Fendekova, M., Gailliez, S., Hannaford, J., Kingston, D., Van Loon, A. F., Mediero, L., Osuch, M., Romanowicz, R., Sauquet, E., Stagge, J. H., and Wong, W. K.: The European 2015 drought from a hydrological perspective, Hydrol. Earth Syst. Sci., 21, 3001–3024, https://doi.org/10.5194/hess-21-3001-2017, 2017.
Li, D., Schrön, M., Köhli, M., Bogena, H., Weimar, J., Bello, M. A. J., Han, X., Gimeno, M. A. M., Zacharias, S., Vereecken, H., and Hendricks Franssen, H.-J.: Can drip irrigation be scheduled with a cosmic-ray neutron sensor?, Vadose Zone J., 18, 190053. https://doi.org/10.2136/vzj2019.05.0053, 2019.
Liu, S., Li, X., Xu, Z., Che, T., Xiao, Q., Ma, M., Liu, Q., Jin, R., Guo, J., Wang, L., Wang, W., Qi, Y., Li, H., Xu, T., Ran, Y., Hu, X., Shi, S., Zhu, Z., Tan, J., Zhang, Y., and Ren, Z.: The Heihe Integrated Observatory Network: A basin-scale land surface processes observatory in China, Vadose Zone J. 17, 180072, https://doi.org/10.2136/vzj2018.04.0072, 2018.
Montzka, C., Bogena, H. R., Zreda, M., Monerris, A., Morrison, R., Muddu, S., and Vereecken, H.: Validation of spaceborne and modelled surface soil moisture products with cosmic-ray neutron probes, Remote Sens., 9, 103, https://doi.org/10.3390/rs9020103, 2017.
Montzka, C., Bogena, H. R., Herbst, M., Cosh, M. H., Jagdhuber, T., and Vereecken, H.: Estimating the number of reference sites necessary for the validation of global soil moisture products, IEEE Geosci. Remote Sens. Lett., 18, 1530–1534, https://doi.org/10.1109/LGRS.2020.3005730, 2020.
Muñoz Sabater, J.: ERA5-Land hourly data from 1950 to 1980, Copernicus Climate Change Service (C3S) Climate Data Store (CDS) [data set], https://doi.org/10.24381/cds.e2161bac, 2021.
Nasta, P., Bogena, H. R., Sica, B., Weuthen, A., Vereecken, H., and Romano, N.: Integrating Invasive and Non-invasive Monitoring Sensors to Detect Field-Scale Soil Hydrological Behavior, Front. Water, 2, 26, https://doi.org/10.3389/frwa.2020.00026, 2020.
Ney, P., Köhli, M., Bogena, H., and Goergen, K.: CRNS-based monitoring technologies for a weather and climate-resilient agriculture: realization by the ADAPTER project, 2021 IEEE Internat. Workshop Metrol. Agr. Forest. (MetroAgriFor), 203–208, https://doi.org/10.1109/MetroAgriFor52389.2021.9628766, 2021.
Node-RED: Node-RED user guide, https://nodered.org/docs/user-guide/, last access: 1 March 2022.
Lawrence, D., Fisher, R., Koven, C., Oleson, K., Swenson, S., Vertenstein, M., Andre, B., Bonan, G., Ghimire, B., and van Kampenhout, L.: Technical description of version 5.0 of the Community Land Model (CLM), Natl. Cent. Atmospheric Res. (NCAR), http://www.cesm.ucar.edu/models/cesm2/land/CLM50_Tech_Note.pdf (last access: 1 March 2022), 2018.
Patil, A., Fersch, B., Hendricks Franssen, H. J., and Kunstmann, H.: Assimilation of Cosmogenic Neutron Counts for Improved Soil Moisture Prediction in a Distributed Land Surface Model, Front. Water, 3, 115, https://doi.org/10.3389/frwa.2021.729592, 2021.
Pisinaras, V., Panagopoulos, A., Herrmann, F., Bogena, H. R., Doulgeris, C., Ilias, A., Tziritis, E., and Wendland, F.: Hydrologic and geochemical research at Pinios Hydrologic Observatory – Initial results, Vadose Zone J., 17, 180102, https://doi.org/10.2136/vzj2018.05.0102, 2018.
Power, D., Rico-Ramirez, M. A., Desilets, S., Desilets, D., and Rosolem, R.: Cosmic-Ray neutron Sensor PYthon tool (crspy 1.2.1): an open-source tool for the processing of cosmic-ray neutron and soil moisture data, Geosci. Model Dev., 14, 7287–7307, https://doi.org/10.5194/gmd-14-7287-2021, 2021.
Rasche, D., Köhli, M., Schrön, M., Blume, T., and Güntner, A.: Towards disentangling heterogeneous soil moisture patterns in cosmic-ray neutron sensor footprints, Hydrol. Earth Syst. Sci., 25, 6547–6566, https://doi.org/10.5194/hess-25-6547-2021, 2021.
Rivera Villarreyes, C. A., Baroni, G., and Oswald, S. E.: Integral quantification of seasonal soil moisture changes in farmland by cosmic-ray neutrons, Hydrol. Earth Syst. Sci., 15, 3843–3859, https://doi.org/10.5194/hess-15-3843-2011, 2011.
Romano, N., Nasta, P., Bogena, H., De Vita, P., Stellato, L., and Vereecken, H.: Monitoring hydrological processes for land and water resources management in a Mediterranean ecosystem: The Alento River Catchment Observatory, Vadose Zone J., 17, 1–12, 2018.
Rosolem, R., Shuttleworth, W. J., Zreda, M., Franz, T. E., Zeng, X., and Kurc, S. A.: The effect of atmospheric water vapor on neutron count in the cosmic-ray soil moisture observing system, J. Hydrometeorol., 14, 1659–1671, https://doi.org/10.1175/JHM-D-12-0120.1, 2013.
Schattan, P., Baroni, G., Oswald, S. E., Schöber, J., Fey, C., Kormann, C., Huttenlau, M., and Achleitner, S.: Continuous monitoring of snowpack dynamics in alpine terrain by aboveground neutron sensing, Water Resour. Res., 53, 3615–3634, https://doi.org/10.1002/2016WR020234, 2017.
Schattan, P., Köhli, M., Schrön, M., Baroni, G., and Oswald, S. E.: Sensing Area-Average Snow Water Equivalent with Cosmic-Ray Neutrons: The Influence of Fractional Snow Cover, Water Resour. Res., 5, 10796–10812, https://doi.org/10.1029/2019WR025647, 2019a.
Schattan, P., Baroni, G., and Oswald, S. E.: Cosmic-Ray Neutron Data at the Weisssee Snow Research Site, PANGAEA [data set], https://doi.org/10.1594/PANGAEA.900959, 2019b.
Schattan, P., Schwaizer, G., Schöber, J., and Achleitner, S.: The complementary value of cosmic-ray neutron sensing and snow covered area products for snow hydrological modelling, Remote Sens. Environ., 239, 111603, https://doi.org/10.1016/j.rse.2019.111603, 2020.
Schrön, M.: CORNish PASDy – COsmic-Ray Neutron flavored PASDy PASDy – Processing and Analysis of Sensor Data in pYthon [data set], https://git.ufz.de/CRNS/cornish_pasdy, last access: 16 December 2021.
Schrön, M., Zacharias, S., Köhli, M., Weimar, J. and Dietrich, P.: Monitoring environmental water with ground albedo neutrons and correction for incoming cosmic rays with neutron monitor data, in: The 34th International Cosmic Ray Conference, 30 July–6 August 2015, The Hague, The Netherlands (ICRC2015), 236231 (Proceedings of Science, 2016), https://doi.org/10.22323/1.236.0231, 2016.
Schrön, M., Köhli, M., Scheiffele, L., Iwema, J., Bogena, H. R., Lv, L., Martini, E., Baroni, G., Rosolem, R., Weimar, J., Mai, J., Cuntz, M., Rebmann, C., Oswald, S. E., Dietrich, P., Schmidt, U., and Zacharias, S.: Improving calibration and validation of cosmic-ray neutron sensors in the light of spatial sensitivity, Hydrol. Earth Syst. Sci., 21, 5009–5030, https://doi.org/10.5194/hess-21-5009-2017, 2017.
Schrön, M., Zacharias, S., Womack, G., Köhli, M., Desilets, D., Oswald, S. E., Bumberger, J., Mollenhauer, H., Kögler, S., Remmler, P., Kasner, M., Denk, A., and Dietrich, P.: Intercomparison of cosmic-ray neutron sensors and water balance monitoring in an urban environment, Geosci. Instrum. Method. Data Syst., 7, 83–99, https://doi.org/10.5194/gi-7-83-2018, 2018.
Schrön, M., Oswald, S. E., Zacharias, S., Kasner, M., Dietrich, P., and Attinger, S.: Neutrons on Rails–trans-regional monitoring of soil moisture and snow water equivalent, Geophys. Res. Lett., 48, e2021GL093924, https://doi.org/10.1029/2021gl093924, 2021.
Schuldt, B., Buras, A., Arend, M., Vitasse, Y., Beierkuhnlein, C., Damm, A., Gharun, M., Grams, T. E. E., Hauck, M., Hajek, P., Hartmann, H., Hiltbrunner, E., Hoch, G., Holloway-Phillips, M., Korner, C., Larysch, E., Lubbe, T., Nelson, D. B., Rammig, A., Rigling, A., Rose, L., Ruehr, N. K., Schumann, K., Weiser, F., Werner, C., Wohlgemuth, T., Zang, C. S., and Kahmen, A.: A first assessment of the impact of the extreme 2018 summer drought on Central European forests, Basic Appl. Ecol., 45, 86–103, 2020.
Seneviratne, S. I., Lehner, I., Gurtz, J., Teuling, A. J., Lang, H., Moser, U., Grebner, D., Menzel, L., Schroff, K., Vitvar, T., and Zappa, M.: Swiss prealpine Rietholzbach research catchment and lysimeter: 32 year time series and 2003 drought event, Water Resour. Res., 48, W06526, https://doi.org/10.1029/2011WR011749, 2012.
Sheffield, J., Wood, E. F., and Roderick, M. L.: Little change in global drought over the past 60 years, Nature, 491, 435–438, https://doi.org/10.1038/nature11575, 2012.
Shuttleworth, J., Rosolem, R., Zreda, M., and Franz, T.: The COsmic-ray Soil Moisture Interaction Code (COSMIC) for use in data assimilation, Hydrol. Earth Syst. Sci., 17, 3205–3217, https://doi.org/10.5194/hess-17-3205-2013, 2013.
Simpson, J. A.: The Cosmic Ray Nucleonic Component: The Invention and Scientific Uses of the Neutron Monitor, in: Cosmic Rays and Earth, edited by: Bieber, J. W., Eroshenko, E., Evenson, P., Flückiger, E. O., and Kallenbach, R., Space Sciences Series of ISSI, 10, 11-32, Springer, Dordrecht, https://doi.org/10.1007/978-94-017-1187-6_2, 2000.
Smith, P. J., Pappenberger, F., Wetterhall, F., Del Pozo, J. T., Krzeminski, B., Salamon, P., Muraro, D., Kalas, M., and Baugh, C.: On the operational implementation of the European Flood Awareness System (EFAS), in: Flood forecasting, 313–348, Academic Press, https://doi.org/10.1016/B978-0-12-801884-2.00011-6, 2016.
Stott, P. A., Stone, D. A., and Allen, M. R.: Human contribution to the European heatwave of 2003, Nature, 432, 610–614, https://doi.org/10.1038/nature03130, 2004.
Sutanto, S. J., Vitolo, C., Di Napoli, C., D'Andrea, M., and Van Lanen, H. A.: Heatwaves, droughts, and fires: exploring compound and cascading dry hazards at the pan-European scale, Environ. Int., 134, 105276, https://doi.org/10.1016/j.envint.2019.105276, 2020.
Topp, G. C., Davis, J. L., and Annan, A. P.: Electromagnetic determination of soil water content: Measurements in coaxial transmission lines, Water Resour. Res., 16, 574–582, 1980.
Turco, M., von Hardenberg, J., AghaKouchak, A., Carmen Llasat, M., Provenzale, A., and Trigo, R. M.: On the key role of droughts in the dynamics of summer fires in mediterranean Europe, Scient. Rep., 7, 81, https://doi.org/10.1038/s41598-017-00116-9, 2017.
Vather, T., Everson, C. S., and Franz, T. E.: The Applicability of the Cosmic Ray Neutron Sensor to Simultaneously Monitor Soil Water Content and Biomass in an Acacia mearnsii, Forest Hydrol., 7, 48, https://doi.org/10.3390/hydrology7030048, 2020.
Weimar, J., Köhli, M., Budach, C., and Schmidt, U.: Large-Scale Boron-Lined Neutron Detection Systems as a 3He Alternative for Cosmic Ray Neutron Sensing, Front. Water, 2, 16, https://doi.org/10.3389/frwa.2020.00016, 2020.
Wollschläger, U., Attinger, S., Borchardt, D., Brauns, M., Cuntz, M., Dietrich, P., Fleckenstein, J. H., Friese, K., Friesen, J., Harpke, A., Hildebrandt, A., Jaeckel, G., Kamjunke, N., Knoeller, K., Koegler, S., Kolditz, O., Krieg, R., Kumar, R., Lausch, A., Liess, M., Marx, A., Merz, R., Mueller, C., Musolff, A., Norf, H., Oswald, S. E., Rebmann, C., Reinstorf, F., Rode, M., Rink, K., Rinke, K., Samaniego, L., Vieweg, M., Vogel, H.-J., Weitere, M., Werban, U., Zink, M. and Zacharias, S.: The Bode hydrological observatory: a platform for integrated, interdisciplinary hydro-ecological research within the TERENO Harz/Central German Lowland Observatory, Environ. Earth Sci., 76, 1–25, https://doi.org/10.1007/s12665-016-6327-5, 2017.
Yiou, P., Cattiaux, J., Faranda, D., Kadygrov, N., Jézéquel, A., Naveau, P., Ribes, A., Robin, Y., Thao, S., van Oldenborgh, G. J., and Vrac, M.: Analyses of the Northern European summer heatwave of 2018, Bull. Am. Meteorol. Soc., 101, S35–S40, https://doi.org/10.1175/BAMS-D-19-0170.1, 2020.
Zreda, M., Desilets, D., Ferre, T. P. A., and Scott, R. L.: Measuring soil moisture content non-invasively at intermediate spatial scale using cosmic-ray neutrons, Geophys. Res. Lett., 35, L21402, https://doi.org/10.1029/2008GL035655, 2008.
Zreda, M., Shuttleworth, W. J., Zeng, X., Zweck, C., Desilets, D., Franz, T., and Rosolem, R.: COSMOS: the COsmic-ray Soil Moisture Observing System, Hydrol. Earth Syst. Sci., 16, 4079–4099, https://doi.org/10.5194/hess-16-4079-2012, 2012.
Zreda, M., Nitychoruk, J., Chodyka, M., Świerczewska-Pietras, K., and Zbucki, Ł.: New method for measuring soil moisture using cosmogenic neutrons, Prz. Geol., 63, 239–246, 2015.