the Creative Commons Attribution 4.0 License.
the Creative Commons Attribution 4.0 License.
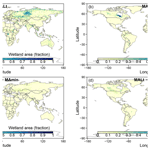
Development of the global dataset of Wetland Area and Dynamics for Methane Modeling (WAD2M)
Etienne Fluet-Chouinard
Katherine Jensen
Kyle McDonald
Gustaf Hugelius
Thomas Gumbricht
Mark Carroll
Catherine Prigent
Annett Bartsch
Benjamin Poulter
Seasonal and interannual variations in global wetland area are a strong driver of fluctuations in global methane (CH4) emissions. Current maps of global wetland extent vary in their wetland definition, causing substantial disagreement between and large uncertainty in estimates of wetland methane emissions. To reconcile these differences for large-scale wetland CH4 modeling, we developed the global Wetland Area and Dynamics for Methane Modeling (WAD2M) version 1.0 dataset at a ∼ 25 km resolution at the Equator (0.25∘) at a monthly time step for 2000–2018. WAD2M combines a time series of surface inundation based on active and passive microwave remote sensing at a coarse resolution with six static datasets that discriminate inland waters, agriculture, shoreline, and non-inundated wetlands. We excluded all permanent water bodies (e.g., lakes, ponds, rivers, and reservoirs), coastal wetlands (e.g., mangroves and sea grasses), and rice paddies to only represent spatiotemporal patterns of inundated and non-inundated vegetated wetlands. Globally, WAD2M estimates the long-term maximum wetland area at 13.0×106 km2 (13.0 Mkm2), which can be divided into three categories: mean annual minimum of inundated and non-inundated wetlands at 3.5 Mkm2, seasonally inundated wetlands at 4.0 Mkm2 (mean annual maximum minus mean annual minimum), and intermittently inundated wetlands at 5.5 Mkm2 (long-term maximum minus mean annual maximum). WAD2M shows good spatial agreements with independent wetland inventories for major wetland complexes, i.e., the Amazon Basin lowlands and West Siberian lowlands, with Cohen's kappa coefficient of 0.54 and 0.70 respectively among multiple wetland products. By evaluating the temporal variation in WAD2M against modeled prognostic inundation (i.e., TOPMODEL) and satellite observations of inundation and soil moisture, we show that it adequately represents interannual variation as well as the effect of El Niño–Southern Oscillation on global wetland extent. This wetland extent dataset will improve estimates of wetland CH4 fluxes for global-scale land surface modeling. The dataset can be found at https://doi.org/10.5281/zenodo.3998454 (Zhang et al., 2020).
- Article
(11516 KB) - Full-text XML
- BibTeX
- EndNote
Wetlands cover about 10 % of global land area (Davidson et al., 2018) and play an important role in regulating global climate via biogeochemical cycling of greenhouse gases (IPCC, 2013). Wetlands are highly productive ecosystems that store large amounts of soil carbon due to their waterlogged conditions inhibiting aerobic soil respiration. Flooded conditions alter the soil redox state for microbes to favor methanogenesis, and thus wetlands are the largest natural source of methane (CH4) to the atmosphere, contributing ∼ 20 %–30 % of the total annual global methane budget (Kirschke et al., 2013; Saunois et al., 2016, 2020). The spatial and temporal distribution of wetlands is one of the most important and yet uncertain factors determining the time and location of CH4 fluxes (Melton et al., 2013; Parker et al., 2018). Wetlands are at risk from human activities such as conversion to agricultural lands and land clearing and drainage and are also at risk from climate-change-caused drying or less predictable precipitation events (Davidson et al., 2018).
Because wetland definitions vary between science, applications, and policy objectives, a definition suitable for CH4 modeling is needed for comparative reasons and to avoid double counting. Since the first global wetland map of Matthews and Fung (Matthews and Fung, 1987), several additional global and regional wetland area datasets have been developed (Table A1). These datasets are characterized by differences in definition, data sources, methodologies, and time period covered. For example, the Ramsar Convention on Wetlands focusing on nature conservation uses an inclusive definition for wetlands as both vegetated and non-vegetated systems (i.e., rivers, lakes, ponds). However, the biogeochemistry and methane flux pathways from open water and vegetated wetlands differ substantially. Additionally, human-made water bodies (e.g., reservoirs, rice paddies, agricultural wastewater ponds, i.e., aquaculture; Grinham et al., 2018) are considered wetlands in the definition of the IPCC Guidelines for National Greenhouse Gas Inventories (Hiraishi et al., 2014). The biogeochemical processes in these kinds of intensely managed wetlands differ from those of natural wetlands, and generic modeling approaches are not applicable. Boreal taiga forests and tropical floodplains, which are considered CH4-emitting areas given their seasonally inundated states and significant CH4 transport pathway via tree stems (Barba et al., 2019; Pangala et al., 2017), are underestimated by many wetland mapping products (Junk, 2013) due to the lack of record in inventories and difficulty in detecting dense forest canopies in the satellite-based products. Broadly defined, wetland datasets available to this day fall into one of four types: (1) static maps based on a compilation of regional inventories based on geomorphic features and aerial photography (Finlayson et al., 1999; Hugelius et al., 2013; Lehner and Döll, 2004; Matthews and Fung, 1987; Wulder et al., 2018), (2) remote-sensing-derived products (Aires et al., 2017; Carroll et al., 2009; DeVries et al., 2017; Feng et al., 2016; Jensen and McDonald, 2019; Papa et al., 2010; Pekel et al., 2016; Poulter et al., 2017; Prigent et al., 2001, 2007; Schroeder et al., 2015; Yamazaki et al., 2015), (3) prognostic hydrological water-balance modeling using approaches like TOPMODEL (Kleinen et al., 2012; Ringeval et al., 2010; Stocker et al., 2014; Zhang et al., 2016), and (4) hybrid approaches that combine satellite observations with statistical modeling (Fluet-Chouinard et al., 2015; Gumbricht et al., 2017; Tootchi et al., 2019). These approaches differ in their representation of wetlands, ranging from long-term features of the landscape to area inundated at a given time.
Characterizing the seasonal and interannual variation in wetland extent is critical to improving global-scale wetland CH4 modeling. Contemporary evidence from remote sensing (Alsdorf et al., 2000, 2007; Hu et al., 2018; Lunt et al., 2019; Melack et al., 2004; Pandey et al., 2021; Prigent et al., 2007, 2012; Rodell et al., 2018) and field monitoring (Dunne and Aalto, 2013) suggests that global wetlands, especially tropical floodplains, have a significant seasonal cycle and interannual variability in spatial extent that depend on changes in water balance (i.e., precipitation, runoff, and evapotranspiration) and local topography. Despite the critical importance of spatial and temporal changes in wetland area, there are large discrepancies among the estimates of global wetland extent (Aires et al., 2018; Melton et al., 2013; Pham-Duc et al., 2017; Wania et al., 2013) and only a limited number of available global products characterize temporal dynamics in wetland extent (Gallant, 2015; Huang et al., 2014; Prigent et al., 2007, 2020).
Remotely sensed observations show potential for capturing spatiotemporal wetland patterns. While bottom-up inventories define wetlands based on a combination of soils, hydrology, and vegetation, satellite-based observations of surface inundation (i.e., water above the soil) capture areas that are permanently or seasonally wet. Microwave-sensor-based products (Jensen and McDonald, 2019; Papa et al., 2010; Prigent et al., 2020; Schroeder et al., 2015) can sense water below vegetated canopies and now provide a multi-decadal record, with weekly to monthly revisit times. Optical-sensor-based products using visible or infrared bands (Amani et al., 2019; Feng et al., 2016; Jones, 2019; Pekel et al., 2016; Wulder et al., 2018; Yamazaki et al., 2015) observe the open-water dynamics but have limited capacity to detect surface water beneath vegetation canopy. L-band (∼ 1 GHz) synthetic aperture radar (SAR) sensors are suitable for large-scale wetland mapping because of their ability to penetrate clouds and detect flooding beneath most woody vegetation (Melack et al., 2004) or sufficiently dense canopies with thickness on the same order as the wavelength. SAR is more successful at mapping forested wetlands than higher-temporal-frequency observations (e.g., < 1 week) such as optical or microwave products. These products separate inland-water types at a high spatial resolution but typically provide limited temporal coverage (e.g., > 1 month).
Data fusion approaches that merge remote sensing observations from multiple sources of sensors at different spatial resolutions present a feasible way to properly capture the dynamics of wetland extent. Despite recent progress in wetland mapping, developing long-term wetland dynamic datasets specifically suited for global CH4 studies (Poulter et al., 2017) is an area of active research. Further, recent work has shown significant differences between remote sensing wetland products (Pham-Duc et al., 2017). These discrepancies can be linked to methodological differences (including pre-processing), data sources, and definitions. This introduces large biases into the modeling of wetland CH4 emissions (Bohn et al., 2015) that can be traced to the following limitations: (1) higher-spatial-resolution optical sensors can only detect open water in the absence of clouds and vegetation, while SAR measurements can penetrate cloud and dense canopies but have limited temporal coverage; (2) available coarse-spatial-resolution microwave-based products are able to detect inundation only under conditions of low vegetation canopy cover; and (3) the intrinsic limitations in remote sensing include the difficulty in detecting inundation for high latitudes due to the satellite orbits or the low radiation in the winter. In addition, several recent studies (Fluet-Chouinard et al., 2015; Hess et al., 2015; Prigent et al., 2007; Reschke et al., 2012) suggest that the wetland mapping products at a coarse resolution tend to overlook small inundated areas. Some of the difficulty in merging these products arises from ambiguity in definitions of inundated versus open-water wetlands. Also, widely used descriptions of wetlands (shallow water with depth less than 2–2.5 m; Cowardin et al., 1979; Tiner et al., 2015) overlap with a vast array of lakes and small ponds – especially in permafrost peatlands and thermokarst regions (West and Plug, 2008). The confusion between wetlands and water bodies risks double counting CH4 emissions from high latitudes (Thornton et al., 2016). All these issues lead to biases and uncertainties in developing a global dataset of wetland extent.
The objective of this study is to develop a global dynamic wetland dataset with a data fusion approach using consistent definitions for use in wetland methane emission studies. Given the many wetland types used in the literature, we chose an operational definition of wetlands as all-natural vegetated forested and non-forested wetlands, excluding coastal wetlands; cultivated wetlands such as irrigated rice paddies; and open-water systems such as rivers, streams, lakes, ponds, and reservoirs. Estimates of the methane-producing area are used in all bottom-up CH4-flux methodologies: from upscaling fluxes measured by eddy covariance at an ecosystem scale (Knox et al., 2019; Peltola et al., 2019; Treat et al., 2018) to process-based modeling at a global scale (Bloom et al., 2010; Melton et al., 2013; Poulter et al., 2017).
The resulting dataset, named the Wetland Area and Dynamics for Methane Modeling (WAD2M), is designed to fuse multiple datasets including ground-based wetland inventories, remote sensing products of open waters, and surface inundation datasets based on optical and active and passive microwave satellite observations. Within this framework, the Surface Water Microwave Product Series (SWAMPS) is used as the basis for providing the temporal dynamics at a monthly time step and at a spatial resolution of 0.25∘ over a 19-year period (2000–2018). A set of wetland-related datasets at different spatial resolutions representing lakes, ponds, rivers and streams, rice paddies, and a coastal mask are applied to filter out non-vegetated and anthropogenic wetlands. Another set of static maps representing non-inundated wetlands, such as peatlands, are used to fill in the gaps of SWAMPS. Uncertainties are derived by comparing WAD2M with available benchmark products at regional and global scales.
2.1 Overview of data processing and wetland definition
Our data fusion approach begins with a time series of global, monthly surface inundation provided by SWAMPS v3.2 (Jensen and McDonald, 2019). The SWAMPS dataset is derived from a series of active and passive microwave remote sensing observations used to estimate total area of surface inundation including all natural and managed terrestrial (open to closed canopy vegetation) and open-water bodies, including coastal bodies, lakes, rivers, and ponds. All ancillary datasets (inventoried wetlands, remotely sensed inland waters, rice, ocean) were re-gridded to a 0.25∘ resolution to match SWAMPS and expressed as fractional areas. The following sections describe the data processing in the following steps (Fig. 1): the SWAMPS dataset was used to represent the temporal variation in wetland dynamics. For the wetland regions that were not captured or well-represented in SWAMPS mainly due to closed-canopy conditions, independent datasets of static wetland distributions were fused with SWAMPS. The merger was carried out in five steps: (1) by calculating the long-term maximum annual surface inundation from SWAMPS (fwmax), (2) on a per-pixel basis by comparing fwmax with the independent datasets of static wetland distributions (see Sect. 2.2), (3) by shifting fwmax to match the wetland maps for pixels where fwmax is less than the static distribution, (4) by imposing the SWAMPS seasonal cycle onto the corrected fwmax dataset, and (5) by removing inland water bodies, coastal waters, and rice agriculture.
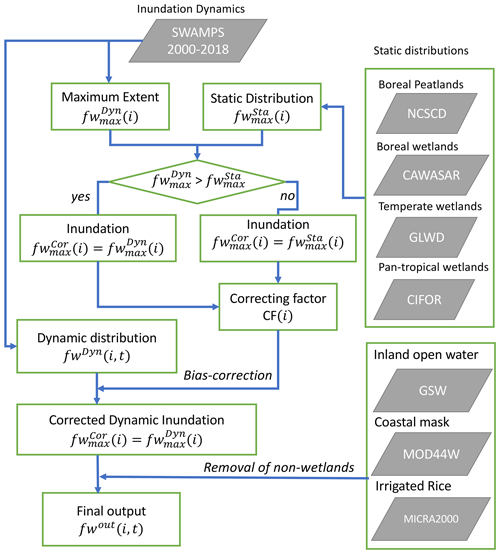
Figure 1Flow chart of the method describing the wetland extent estimate from SWAMPS and other water body datasets to consolidate our WAD2M product. The chart describes the step calculating fw in each 0.25∘ grid cell i at time (monthly) t.
We added missing wetlands to SWAMPS by fusing it with the best available maps and inventories of under-represented wetlands separately across three latitudinal bands. For northern wetland inventories, we used the Northern Circumpolar Soil Carbon Database (NCSCD; Hugelius et al., 2013) to map permafrost and non-permafrost peatlands (Histels and Histosols). Mineral soil wetlands were mapped from a SAR-based map by including occurrences of wetlands in the circum-Arctic (Widhalm et al., 2015) outside areas mapped as peatlands by the NCSCD. In the tropics, we used a 231 m resolution pan-tropical dataset based on the geomorphic classification approach (Gumbricht et al., 2017). For temperate regions not covered by either the boreal or tropical datasets, we used the 1 km Global Lakes and Wetlands Database (GLWD) Level 3 after removing Class 1–3 lakes and rivers (Lehner and Döll, 2004). A global dataset of monthly irrigated and rainfed crop areas (MIRCA2000) at a 10 km resolution was used to remove rice agriculture (Portmann et al., 2010). Lakes, ponds, rivers, and other permanent inland water bodies were removed using the Landsat Global Surface Water Explorer (GSW) dataset (Pekel et al., 2016). An ocean–coastline mask based on MOD44W Collection 6 (Carroll et al., 2009), a 250 m resolution annual product from the Moderate Resolution Imaging Spectroradiometer (MODIS) remote sensor, was used to remove ocean waters. The new SWAMPS v3.2 (Jensen and McDonald, 2019) is an updated version of SWAMPS v2.0 (Schroeder et al., 2015) that was used as input in the hybrid wetland product SWAMPS-GLWD (Poulter et al., 2017), the predecessor of WAD2M. The major differences between WAD2M and SWAMPS-GLWD are that (1) WAD2M uses an updated version, SWAMPS v3.2, with improved algorithm and ancillary datasets; (2) WAD2M uses multiple static wetland maps as mergers in the processing (while SWAMPS-GWLD only considers GLWD in the processing); (3) WAD2M includes removal of lakes, ponds, rivers, streams, and irrigated rice paddies; and (4) WAD2M uses a globally consistent ocean–land mask.
To characterize the temporal dynamics, three wetland statistics were computed: (1) mean annual minimum (MAmin), (2) mean annual maximum (MAmax), and (3) long-term annual maximum (MALt). For each 0.25∘ grid cell, the annual magnitude in wetland area can be calculated as the difference between MAmax and MAmin, while wetland areas that do not flood during the average year (i.e., intermittent wetlands) can be calculated as the difference between MALt and MAmin.
2.2 Datasets
2.2.1 Wetland dynamic dataset
The Surface Water Microwave Product Series v3.2 (SWAMPS) is a dataset of long-term, daily time series of the inundated area fraction derived from microwave remote sensing. The SWAMPS dataset provides estimates of terrestrial surface water dynamics, including for wetlands, rivers, lakes, ponds, reservoirs, rice paddies, and episodically inundated areas. SWAMPS provides estimates of the global inundated area fraction (fw) developed under the NASA Making Earth System Data Records for Use in Research Environments (MEaSUREs) program and is updated monthly. SWAMPS fw estimates are derived from a combination of passive microwave brightness temperature and active microwave radar backscatter from a variety of satellite sensors supplemented with a priori knowledge of land cover based on a static MODIS land cover product (Schroeder et al., 2015). The SWAMPS product includes daily gridded DMSP Special Sensor Microwave/Imager and Special Sensor Microwave Imager/Sounder (SSM/I-SSMIS) Pathfinder brightness temperature observations and active microwave backscatter from the NASA SeaWinds QuikSCAT Level-1B Sigma0 product and Advanced Scatterometer Level-1B (ASCAT) product, with ancillary snow water equivalent, land cover map, and normalized difference vegetation index (NDVI) from the Advanced Very High Resolution Radiometer (AVHRR) and MODIS for delineating snow cover and arid and semiarid areas. SWAMPS v3.2 is an update of v2.0 and includes a new cloud and snow mask, a quality control flag, a new land and ocean mask, freeze–thaw detection, and improved sensor intercalibration. For the purpose of this study, the SWAMPS v3.2 dataset, covering the years 2000 to 2018, was merged into a single monthly mean time series using samples flagged as “Valid Observations”. The sample sizes for the monthly mean range from 14–22 d globally with more measurements during the summer months and fewer measurements during winter months. For SWAMPS v3.2, the coastal zone was first filtered out using a Landsat-based 90 m mask of permanent ocean waters defined by the G3WBM global water body map dataset (Yamazaki et al., 2015) and then filtered using the MODIS MOD44W product to keep it consistent with the processing of the GSW dataset. The SWAMPS v3.2 data were remapped to WGS84 using bilinear interpolation at a 0.25∘ resolution with values aggregated from daily to monthly means.
2.2.2 Open water and land–ocean masks
The Global Surface Water Explorer (GSW) product is derived from 16 d Landsat thematic mapper imagery at a 30 m spatial resolution and identifies the presence or absence of water bodies over the period 1984–2016 (Pekel et al., 2016). We used this dataset to represent permanent water bodies which we define as those covered by open water for more than 50 % of the months during this time period. We used this as a permanent-water-body mask to avoid including temporary water bodies that are considered wetlands in our working definition. This distribution of long-term maximum permanent water was re-gridded to a 0.25∘ fractional area per grid cell and used for removing inland-water areas from SWAMPS v3.2. Because the coastal regions were masked out in the processing of SWAMPS, we used the MODIS product MOD44WC6 (Carroll et al., 2009) to generate an ocean mask in the processing of GSW to avoid over-deducting. The coastal region is defined as land areas along the coastline within 4 pixels (∼ 1 km) and was then intersected with the ocean-labeled pixels from MOD44WA1 to separate the ocean from inland water. The resulting ocean mask was then applied to remove coastal wetlands in GSW. The static long-term open-water area excluding coastal regions in GSW is 4.5 Mkm2, compared with the river and stream surface areas of 0.8 Mkm2 (Allen and Pavelsky, 2018).
2.2.3 Static wetland distributions
We used static wetland maps to fill gaps left by wetland types that are under-represented or missed by the SWAMPS dataset. However, most static maps do not have global coverage or tend to have lower accuracy compared to the regional products, leaving us to take a separate merging approach for each of the three latitudinal bands.
Many arctic wetlands, including peatlands, do not have surface inundation and thus are not captured by SWAMPS 3.2, but they still emit methane. We use the Northern Circumpolar Soil Carbon Database (NCSCD) to map permafrost and non-permafrost peatlands based on the Histel and Histosol soil orders (Hugelius et al., 2013). The NCSCD dataset is a digital polygon-based database compiled from harmonized regional soil classification maps in which data on soil order coverage have been linked to pedon data. In this study, the NCSCD wetland distribution is used as supplementary data for the latitudinal bands from 60–90∘ N. In this study we use a gridded version with a spatial resolution at 0.25∘. Permafrost and non-permafrost peatlands (Histels and Histosols, defined as > 40 cm surface peat) are mapped in the NCSCD from harmonized regional and national soil maps (Hugelius et al., 2013). However, these maps do not include occurrences of mineral soil tundra wetlands (with organic soil horizons of 0 to 40 cm) or smaller wetland complexes (Hugelius et al., 2020). To better include these types of wetlands, the NCSCD soil maps were combined with CircumArctic Wetlands based on Advanced Aperture Radar (CAWASAR) by Widhalm et al. (2015). The SAR data identify both organic and mineral wetland soils. They are based on Envisat advanced SAR data acquired in Global Monitoring mode (medium resolution) under frozen soil conditions; this represents surface roughness which can serve as a proxy for wetness levels in tundra. The wettest class was included as wetland. It corresponds to soils with > 25 kg C m2 in the top 100 cm (Bartsch et al., 2016). To avoid double counting of organic wetlands (peatlands) the datasets were overlaid so that any overlap between the datasets was removed, maintaining the NCSCD in the output data. The merged static map covers 2.3 Mkm2 for the high latitudes (> 60∘ N), including peatlands and mineral wetlands in the tundra biomes.
The distribution of tropical wetlands, including annually or seasonally waterlogged area and tropical peatlands, are derived from an expert-system mapping product (Gumbricht et al., 2017). We used the Center for International Forestry Research (CIFOR) wetland distribution for adjusting wetlands in the latitudinal bands from 60∘ S–40∘ N. This static map was generated by combining satellite images and topographic convergence indices (TCIs) by CIFOR. The TCI indices are calculated based on the Shuttle Radar Topography Mission (SRTM) digital elevation model (DEM) at a 250 m resolution with precipitation climatology from the WorldClim global dataset (Hijmans et al., 2005). A simplified hydrological model was used to estimate the local vertical water balance, runoff, and flood volumes. The topographic and hydrologic data are merged with MODIS (MCD43A4) images used for estimating the duration of wet and inundated soil conditions. The estimated areas of tropical peatlands and wetlands are ∼ 1.7 and ∼ 4.7 Mkm2 respectively. The estimated extent of CIFOR for the Cuvette Centrale tropical African peatland in the Congo Basin is 125 400 km2, which is in agreement with 145 500 km2 of a recent independent field investigation (Dargie et al., 2017).
The Global Lakes and Wetland Database (GLWD) (Lehner and Döll, 2004) is a global database of lakes, reservoirs, and wetlands based on the aggregation of aerial surveys, surveyor maps, and inventories at global and regional scales. While GLWD was generated from data sources now decades old, for some regions, it still represents the most complete wetland database available today. In this study, the GLWD wetland distribution is used to cover the temperate wetland only in the latitudinal band 40–60∘ N, outside the range of NCSCD and CIFOR. We used the Level-3 product, a global raster map that contains 12 classes of water bodies and wetlands at the 30 s resolution. We excluded the classes representing lakes, rivers, and reservoirs (1–3) and estimated the area of fractional wetland classes (9–12) as the midpoint from the range of each class. We then calculated the total fraction of wetland from all classes in 0.25∘ pixels. The estimated total wetland extent in GLWD is 8.7 Mkm2 for the globe and 2.7 Mkm2 for the 40–60∘ N bands.
2.2.4 Irrigated rice distributions
The distribution of rice paddies is derived from the global dataset of monthly irrigated and rainfed crop areas for the year ca. 2000 (MIRCA2000) (Portmann et al., 2010). The datasets used to develop MIRCA2000 are based on compiling census-based land use datasets downscaled to a grid-cell level and thus are generally consistent with subnational statistics collected by national institutions and by the FAO (Food and Agriculture Organization of the United Nations). For this study, we extracted the annual maximum area of irrigated rice paddies from its original resolution at 5 arcmin and remapped to a 0.25∘ resolutions. We did not consider rainfed rice as we could not reliably separate lowland from upland cropping practices, with only the latter seasonally contributing to surface inundation. The estimated rice paddies in MIRCA2000 (irrigated 0.64 Mkm2, rainfed 1.13 Mkm2) are largely consistent with census-based national and subnational statistics from the FAO (1.54 Mkm2 for total area at ca. 2000) and slightly lower than a remote sensing estimate for irrigated rice paddies (0.66 Mkm2) (Salmon et al., 2015). We thus apply the monthly rice cover from 2000 across the entire 2000–2018 time series. This assumption ignoring year-on-year change in rice paddy area is reasonable given that its area increased by < 1.6 % over 2000–2017 according to IRRI world rice statistics (http://ricestat.irri.org:8080/wrsv3/entrypoint.htm, last access: 6 May 2021).
2.3 WAD2M evaluation
WAD2M was evaluated against several, both static and dynamic, independent datasets of wetland area and surface inundation (Table A1). We used a set of satellite-based terrestrial water dynamics to evaluate the trends in temporal patterns of WAD2M, including (1) global soil moisture time series based on the ESA Soil Moisture and Ocean Salinity (SMOS) mission (Level 4; Kerr et al., 2012), (2) a global inundation time series from the Global Inundation Extent from Multi-Satellites (GIEMS version 2) (Prigent et al., 2020), (3) a global land water mass dynamics product from the Gravity Recovery and Climate Experiment (GRACE) mission (Landerer and Swenson, 2012), and (4) a global inundation dynamics from a prognostic run of a land surface model (LPJ) based on a topography-based hydrological model (TOPMODEL) using Climatic Research Unit (CRU) meteorological forcing (Zhang et al., 2018). We also compare WAD2M to a global static map from Tootchi et al. (2019) (regularly flooded wetlands plus groundwater-driven wetlands based on topographic index; hereafter denoted as Tootchi2019) and to regional static maps available over the West Siberian lowlands (Terentieva et al., 2016) and Amazon Basin (Hess et al., 2015). The similarity of WAD2M performance to these independent validation data is evaluated using the kappa index.
3.1 Effect of data processing on the results
Globally, WAD2M (MAmax) identifies 3.6 Mkm2 more wetlands compared to SWAMPS v3.2 (Table 1). On a continental scale, the wetland extent of SWAMPS v3.2 is in general agreement with inventories except for pronounced discrepancies for tropical wetlands (e.g., Amazon lowlands and tropical Africa), central Asia, and the Sahel regions. The lower area of tropical wetland in SWAMPS v3.2 is generally due to the influence of dense forest canopies. It should be noted that SWAMPS v3.2 detected more wetland area in India than in southeastern China, due to the inclusion of rice paddies in SWAMPS v3.2 that are masked out in WAD2M. Figure 2 shows the comparison of the latitudinal gradient between the original SWAMPS and WAD2M. Generally, WAD2M maintains the same latitudinal pattern as the original SWAMPS, where the peak of wetland area occurs in latitudinal bands of 40–50∘ N for boreal wetlands and there is another peak around the Equator for tropical wetlands.
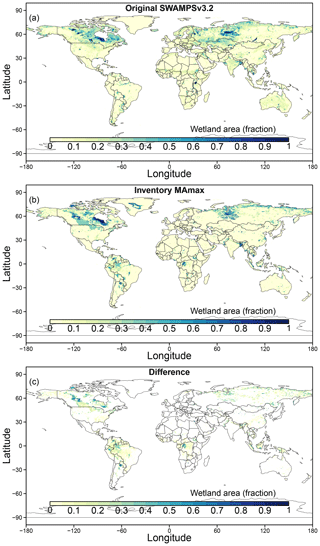
Figure 2Global maps of wetland fraction before and after processing for (a) global distribution of MAmax of SWAMPS; (b) global distribution of MAmax superimposed by NCSCD, GLWD, and CIFOR; (c) difference in MAmax between inventories and original SWAMPS (inventories minus SWAMPS). Here the positive difference is shown as only the regions with positive values after applying the upward correcting factors.
Table 1 quantifies the effect of the data processing steps on the continental and global estimates of wetland area. The total area including all water bodies such as rice paddies, rivers, streams, lakes, ponds, and reservoirs after fwmax correction is 17.0 Mkm2 for MALt. This number is close to the downscaled GIEMS-D15 (17.3 Mkm2), also produced through data merging, suggesting a good agreement between the two products. Applying the fwmax correction leads to a ca. 20 % increase for the three states of inundation relative to SWAMPS v3.2. As intended, the augmentation with inventories filled many missing or underestimated wetland areas of the SWAMPS dataset, which include the Congo floodplain, Amazon Basin lowlands, the Pantanal, Southeast Asia peatlands, and peatlands in high latitudes (i.e., Hudson Bay lowlands and West Siberian lowlands). The highest increase in wetland areas between SWAMPS v2.0 and SWAMPS v3.2 occurs in Asia, followed by North America in the fwmax correction step. However, when we subsequently removed open water and rice paddies in the last step, the increase in wetland area for Asia, North America, and Europe is eliminated. As a result, only South America has a higher wetland area in WAD2M than in SWAMPS v3.2.
3.2 Spatial distributions
3.2.1 Global distributions
Our estimated total annual maximum area of global vegetated wetlands (excluding Greenland and Antarctica) is ∼ 13.0 Mkm2 (Fig. 3a). This estimate consists of 3.5 Mkm2 of mean annual minimum wetlands (Fig. 3b), 4.0 Mkm2 of seasonally inundated wetlands (MAmax minus MAmin) (Fig. 3c), and 5.5 Mkm2 of intermittently inundated wetlands (MALt minus MAmax) (Fig. 3d). Our estimated global total wetland area is slightly higher than that of GIEMS2 (Table 2) but is lower than that of a high-resolution version of the GIEMS initial version GIEMS-D15, which reports a long-term maximum of 17.3 Mkm2 (Fluet-Chouinard et al., 2015). Considering that WAD2M conservatively excludes rice paddies (0.59 Mkm2), rivers, streams, and lakes and ponds (2.52 Mkm2) while GIEMS-D15 includes these water bodies, one possible conclusion is that WAD2M applies the upward mergers of CIFOR and NCSCD, which has lower wetland estimates than those of GLWD, causing a lower long-term maximum than in GIEMS-D15. In addition, our estimated total area for intermittently inundated wetlands is close to the 5.2 Mkm2 reported for similar wetlands by GIEMS-D15, suggesting a good agreement for temporarily inundated areas between two independently developed products. Other recent studies (Hu et al., 2017; Tootchi et al., 2019), however, have proposed much higher global wetland areas of 27–29 Mkm2, which are likely overestimations due to their approaches based on topographic wetness indices that do not take into account the location of surface water tables. This leads to an overestimation of the inundated area with shallow groundwater tables and large inundated areas in, e.g., central Asia and South America that are not matched by other wetland maps.
Table 2Summary of wetland areas (Mkm2) in WAD2M by latitudinal bands in comparison with the long-term maximum wetlands from merger datasets applied in the WAD2M processing and independent evaluation datasets.
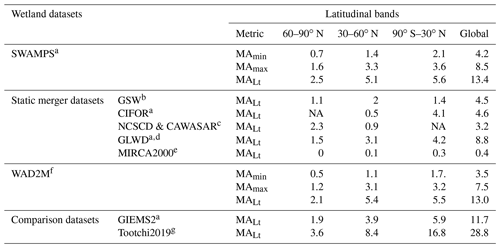
a Represents inundated area. b Represents open water. c Represents peatlands and mineral wetlands. d GLWD excludes rivers, lakes, and reservoirs; wetland classes interpreted to be in middle range. e Represents irrigated rice paddies. f Includes both inundated and non-inundated wetlands but excludes artificial inundation and lakes, ponds, and reservoirs. g For Tootchi et al. (2019), we use the composite wetland map based on the topography–climate wetness index (CW-TCI) version and exclude lake areas. NA – not available
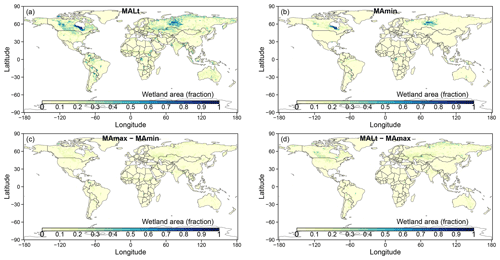
Figure 3Maps of wetland extent in WAD2M for (a) long-term maximum (MALt), (b) mean annual minimum (MAmin), (c) mean annual magnitude (MAmax minus MAmin), and (d) intermittent inundated (MALt minus MAmax).
Permanently inundated wetlands are located in well-documented wetland hotspots, including the Hudson Bay lowlands and West Siberian lowlands, where large extents of peat bogs and fens are not represented by SWAMPS v3.2. In tropical regions, key peatland areas along the Amazonian floodplain, in the Cuvette Centrale of the Congo (Dargie et al., 2017), and in the tropical peatlands in Indonesian Papua are all captured by WAD2M. The subtropical and boreal regions are the main contributors to the seasonally inundated wetlands. For the subtropical regions, the seasonal wetlands are largely located in Southeast Asia and the Sahel, where the variation in wetlands is mainly driven by the annual cycle of precipitation in monsoon regions. For the high latitudes, seasonal wetlands are primarily in the transition region from temperate to boreal across both North America and Eurasia. The high seasonality of inundation in these regions was also captured by the surface inundation retrievals of passive microwave observations from the Soil Moisture Active Passive (SMAP) mission (Du et al., 2018).
The latitudinal distribution of wetland area (Fig. 4) suggest that the Northern Hemisphere middle-to-high latitudes (> 45∘ N) have the highest coverage of wetland area with 45 ± 5 % of the total area of wetlands, followed by the equatorial region (10∘ S–10∘ N). A large portion of the intermittent wetlands are found in the northern middle–high latitudes, in regions that also have large areas of seasonal wetlands. The overall latitudinal pattern in WAD2M is similar to that of other estimates except for the Tootchi2019 one, which has the highest wetland area along the latitude gradient. The exception is over the mid-latitudes (20–40∘ N) where the wetland areas in GLWD are more extensive than that in WAD2M. The wetland areas in the arctic (> 60∘ N) in WAD2M have a lower wetland extent than in GLWD and NCSCD but higher than in GIEMS2. WAD2M shows a slightly higher wetland extent in the latitudinal band of 10–15∘ N compared to the other products, which we attribute to the higher intermittent wetlands in Southeast Asia detected by SWAMPS (Fig. 3d). The latitudinal gradient of the wetland area in WAD2M is similar to that of the previous version, SWAMPS-GLWD (Poulter et al., 2017), but with a reduced wetland area in the arctic (> 50∘ N) and at mid-latitudes (15–45∘ N), a consequence of the masking out the inland-water areas from GSW. Surface inundation products (GIEMS2 and SWAMPS) have limited observations in the high latitudes due to underestimates of wetland extent for unsaturated peatlands (Bohn et al., 2015) and due to the presence of snow and ice, and they are not reliable points of comparison in high latitudes.
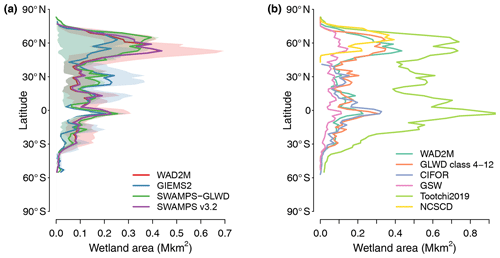
Figure 4Latitudinal gradient of WAD2M in comparison to existing wetland/inundation products. (a) Comparison with the dynamic inundation products GIEMS2 and SWAMPS-GLWD (previous version of WAD2M). The solid lines represent long-term mean annual maximum (MAmax) with upper (MALt) and lower (MAmin) range of inundated area marked as shaded area. (b) Comparison with static inventory datasets. To make it a fair comparison, lakes, rivers, and reservoirs (i.e., class 1–3 in GLWD) were excluded from GLWD. Lakes (code 4) were excluded from the topographic index (TCI) version of the global wetland composite map from Tootchi et al. (2019) (denoted as Tootchi2019). The wetland area is calculated by a 2∘ latitudinal band.
3.2.2 Regional comparison
We validated WAD2M against available independent fine-resolution datasets for the two methane-emitting hotspots, Amazon Basin lowlands (defined as the portion of the Amazon watershed below 500 m a.s.l.) and West Siberian lowlands. These two regions represent different wetland subtypes, vegetation compositions, and local hydrology, making them complementary for our validation.
The distribution of wetland area from WAD2M shows a similar spatial pattern for the Amazon Basin lowlands compared to the map based on JERS-1 SAR (Hess et al., 2015), which was used by Pangala et al. (2017) to estimate methane emissions. The distribution of WAD2M has a good similarity (κ= 0.54) to that of the independent, L-band synthetic aperture radar (SAR) map and is slightly lower than that of GIEMS2 (κ= 0.56; Fig. 6a) but higher than all other global products compared (range 0.1–0.2). WAD2M adequately captures the permanently inundated wetlands along with the Amazon Basin river channel network as well as temporarily flooded wetlands during the wet season (Fig. 5a). However, considerable spatial disagreements of the wetland location and extent were found among available datasets when compared with Hess et al. (2015). The disagreements captured in Fig. 6a are primarily related to the seasonally inundated Pantanal floodplains that have relatively flat terrains with dominant herbaceous/shrubland land cover (height < 5 m) and the Pastaza–Marañón wetland basin of the western Amazonia that have large areas of permanently inundated forested swamps with dense-canopy. The WAD2M-estimated wetland fraction exhibits a reasonably good agreement with independent SAR-based wetland products from Hess et al. (2015) for the Pantanal floodplains and the Ucayali–Marañón wetland basin. The WAD2M estimates for Pastaza–Marañón swamps are close to those of CIFOR and Hess et al. (2015) and are considerably lower than those of Tootchi2019. Over the Pantanal floodplains, WAD2M shows moderate-density wetlands, while Tootchi2019 suggest a widespread high-density area over the same extent. The CIFOR estimate is likely an underestimation given the limitations of its topographic-hydrology approach at estimating inundation over flat terrain like the Pantanal.
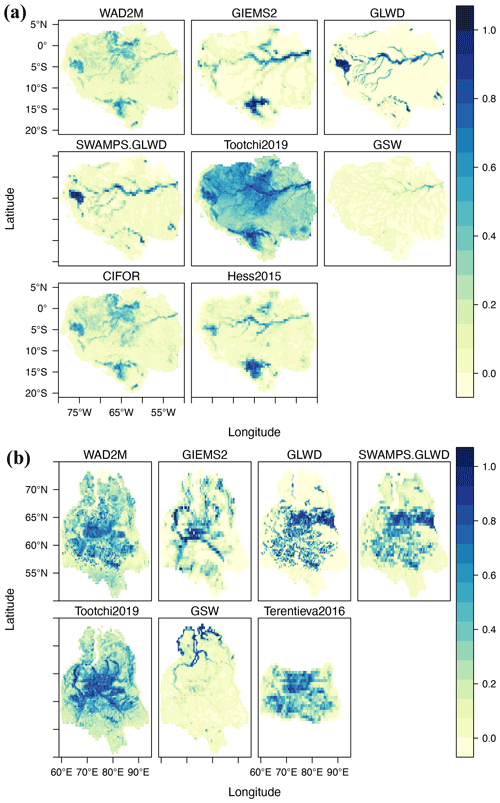
Figure 5Maps of fractional wetland area from WAD2M in comparison with benchmark datasets for (a) Amazon Basin lowlands and (b) West Siberian lowlands. The wetland maps from WAD2M, GIEMS2, GSW, and CIFOR represent long-term maximum, while the fractional inundation for Amazon lowlands from Hess et al. (2015) represents wetland during the period 1995–1996 for the high-water season. GLWD represents GLWD Level 3 that excludes lakes and reservoirs.
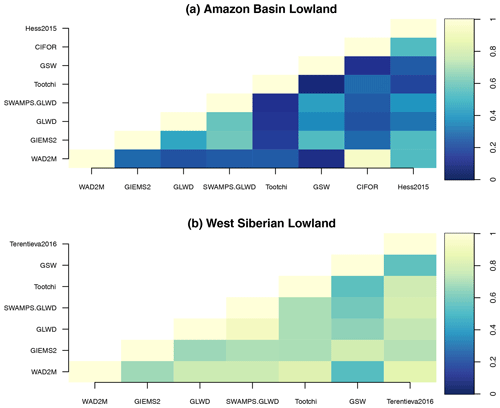
Figure 6Matrix of kappa coefficient for each pairwise comparison for (a) Amazon Basin lowlands and (b) West Siberian lowlands. The Hess et al. (2015) and Terentieva et al. (2016) data represent two independent regional datasets. All of the datasets in (b) were masked by the map of Terentieva et al. (2016), which is available for the taiga forest zone; hence the calculation of the kappa coefficient excludes the arctic tundra zone (latitude > 65∘ N).
The comparison of multiple wetland mapping products for the West Siberian lowlands (Fig. 5b) shows that WAD2M permanent wetlands capture the general spatial distribution represented by most other products. WAD2M shows a good agreement with the independent dataset from Terentieva et al. (2016) that combines field survey and satellite images, in particular for inundation peatlands (e.g., fens and bogs) from 55–65∘ N in the taiga forests. The kappa coefficient of the WAD2M wetland map with Terentieva et al. (2016) for the West Siberian lowlands is 0.70 (Fig. 6b), higher than the value of GIEMS2, GLWD, SWAMPS-GLWD, and Tootchi et al. (2019) (kappa coefficient of 0.18, 0.57, 0.54, and 0.43 respectively). Wetlands in high latitudes above 65∘ N are more intermittent, caused by thawing of permafrost, and thus related to interannual climate variations.
3.3 Temporal patterns
3.3.1 Seasonal cycle
Distinctive seasonal cycles in WAD2M can be observed across varying latitudinal bands (Fig. 7). The tropics (30∘ S–30∘ N) contribute 68 % of the global annual variation in wetland area, owing to the large wetting and drying cycles of tropical wetlands. Despite their large area of intermittent wetlands, the mid-latitudes have a less pronounced seasonal cycle with an average annual minimum of 0.9 Mkm2 and average annual maximum of 1.1 Mkm2 compared to the tropics and high latitudes. High-latitude wetlands again have a strong seasonal cycle with an average annual minimum of 0.24 Mkm2 and average annual maximum of 1.5 Mkm2. The seasonal cycle of WAD2M in mid-latitudes is small compared to that of GIEMS2 (Prigent et al., 2020), which is possibly due to different algorithms applied in SWAMPS and GIEMS2, especially in the way the vegetation contribution is accounted for. The seasonal cycle in the high latitudes is highest among the three regions, which is consistent with GIEMS2 and is mainly due to a significant annual freeze–thaw cycle.
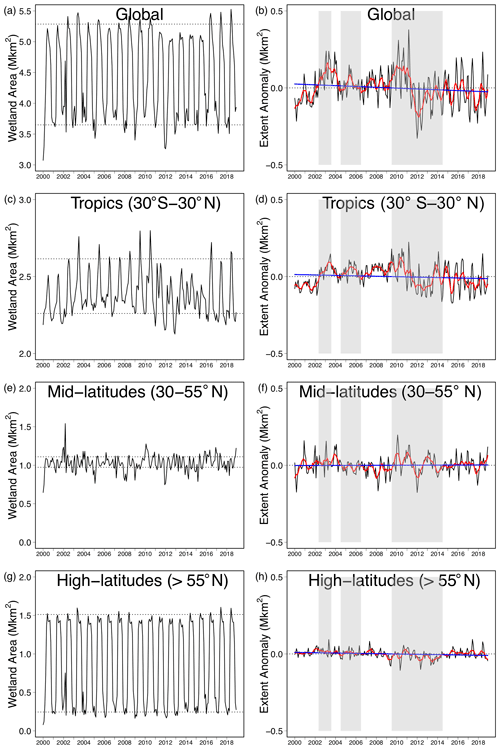
Figure 7The WAD2M wetland extent and their anomalies from 2000–2018. Monthly mean wetland extent (left column; a, c, e, g) for 2000–2018 in black, for the globe, the tropics (30∘ S–30∘ N), and mid-latitudes (30–55∘ N) and northern high latitudes (latitudes > 55∘ N). Horizontal dotted lines in the left column panels represent the mean annual maximum and the mean annual minimum. In the right column (b, d, f, h), the deseasonalized anomalies (black) with the 6-month running mean (red) and linearly fitted trends (blue) using least-squares regression are listed. Shaded areas represent the La Niña phase from the NOAA multivariate ENSO index.
Given that there is a surprising scarcity of independent wetland products with which to evaluate the seasonal patterns in middle and high latitudes, we only focus on the comparison of the seasonal cycle for the Amazon Basin, the largest regional contributor to the seasonal cycle of wetland extent. For the Amazon Basin lowlands, the estimates of wetland area exhibit a low seasonal pattern in WAD2M compared to the SAR-based high-resolution estimates from Hess et al. (2015). The flooded/inundated wetlands in WAD2M vary from 0.340 Mkm2 in the low-water season (October–November) to 0.401 Mkm2 in the high-water season (May–June). The satellite products based on passive microwave bands such as SWAMPS underestimate the seasonality and total wetland areas due to their limited ability to detect inundation outside of large wetlands and river floodplains. This indicates the need to improve the retrieval approach to account for the vegetation contribution in the processing of active and passive microwave signals.
3.3.2 Interannual variation
The interannual variations in WAD2M suggest the effect of climate variations on global wetland extent across varying latitudinal bands (Fig. 7). Monthly anomalies, calculated by subtracting the 19-year mean monthly value from the monthly time series, reveal the changes in global wetlands in response to global climate variability such as El Niño–Southern Oscillation (ENSO) (Fig. 7b). For instance, a strong positive response in wetland areal anomalies was captured by WAD2M during the strong 2010–2011 La Niña event that temporarily increases the terrestrial water storage via affecting precipitation patterns globally (Boening et al., 2012). The signal for the recovery captured by WAD2M, i.e., the decline during the late stage of La Niña, is consistent with the estimated terrestrial water storage from GRACE and the ESA CCI Soil Moisture product (Fig. 8). The linear fit of the pan-tropical wetland anomalies for WAD2M over 2000–2018 shows no significant change (p > 0.1) in the wetland extent for the entire period. There are no trends of wetland extent for mid-latitudes and high latitudes (p > 0.1) as was also found with Landsat imagery (Wulder et al., 2018).
In general, variation in surface water in the tropics is primarily driven by precipitation, and the agreement in the patterns of the surface water extent and precipitation gives confidence in the interannual variability in wetland area estimation. At high latitudes, surface water runoff from snowmelt, not from direct precipitation, contributes towards the lower correlation between inundation extent and precipitation. A strong decline in wetland area during the early stage of El Niño in 2015–2016 was captured by all of the products. The GIEMS2 dataset shows similar patterns to those of WAD2M in two aspects: (1) tropical wetlands contribute to over 50 % of the global total wetland areas and the decadal change in wetland extent is mostly confined to the tropics and (2) the temporal variations in WAD2M are consistent with those of GIEMS2, where a sharp decrease in the tropics was found for 2010–2012, followed by an upward trend from 2013–2014. Note that despite the decline in 2000–2006, the WAD2M estimate is followed by a slight recovery during 2007–2014.
For the interannual variations at a river basin scale (Lehner and Grill, 2013), there is a generally good agreement in the interannual variation in wetland extent between WAD2M and four surface water products that are based on different methodologies (Fig. 8). All the products, including WAD2M, suggest a declining trend in wetland extent in the Amazon Basin since 2012, with strong negative anomalies during 2015–2016 when the strong El Niño event occurred. The temporary increase in wetland extent in WAD2M responding to the strong La Nina event of 2010–2011 is supported by the satellite-based observation of water storage and surface soil moisture, where good agreements of a strong positive phase in terrestrial water were found for the Orinoco Basin and Paraná Basin in South America. The temporal variations in wetland extent for the Ganges, Nile, Yangtze, and Mekong also suggest a good agreement between WAD2M and other products, except for the period since 2015 where GRACE observations suggest a strong decline in the Ganges Basin and Yangtze Basin while WAD2M observations remain constant or slightly decline. This discrepancy could be due to changes in irrigated rice extent, suggesting that the wetland extent in these regions so far is less influenced by groundwater depletion caused by human activity (Rodell et al., 2018). This can be supported by the wetland extent estimate from the TOPMODEL-based prognostic hydrological approach (Zhang et al., 2018), which explicitly excludes influence of human activity and attributes the change to the enhanced tropical precipitation since 2014.
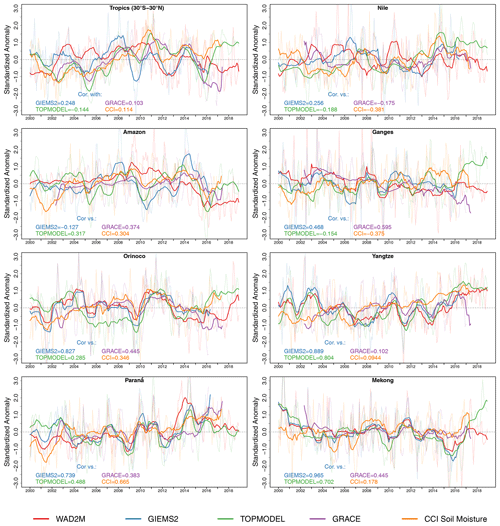
Figure 8Temporal variations in wetland anomalies in WAD2M in comparison with terrestrial water products from multiple sources. Monthly mean anomaly was calculated as total wetland area within a watershed and was then standardized using a Z score. The shaded lines and the solid lines represent monthly anomalies (standardized value) and trends (12-month running mean of the anomalies). The watershed boundaries are defined using the HydroBASINS dataset (Lehner and Grill, 2013). The correlations in the trend between WAD2M and other sources are listed.
3.4 Uncertainties in wetland areal estimation and future direction
Figure 9 shows the uncertainty range (1σ) of mean annual maximum wetland area across the six global and regional data sources applied in this study. The Amazon Basin lowlands and West Siberian lowlands are two regions with relatively more information compared to the rest of the world (Fig. 9b). There is considerable uncertainty in wetland hotspots such as Hudson Bay lowlands, West Siberian lowlands, and major tropical floodplain regions. The causes of the high uncertainty for the boreal and tropical wetlands differ. Mapping boreal wetlands requires discriminating between wetlands and small ponds, which are both considered wetlands in some inventories (e.g., GLWD) but inland waters in others (e.g., GSW). Thus, the removal of freshwater area is one reason that the boreal wetlands estimates in WAD2M are lower. The uncertainty over tropical floodplain systems is due to the temporal mismatches of the different data sources and to the large seasonal and interannual variability in inundated area. Further, densely vegetated forest canopies in tropical floodplains can lead to systematic underestimation of inundation from satellite-based products. Also, uncertainty in DEMs (from spatial resolution or from whether the measurements are “surface” or “soil”), which serve as the basis of the topographic index that is applied in the hybrid wetland mapping products (e.g., CIFOR; Tootchi et al., 2019), can lead to considerable uncertainty in estimation of wetland extent (Zhang et al., 2016), especially for the vegetated wetlands on complex-terrain surfaces (Su et al., 2015).
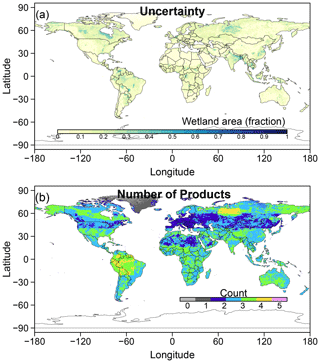
Figure 9Uncertainty (1σ) of annual maximum wetland area (MAmax) across data sources. The data sources used in the calculation are WAD2M, GIEMS2, GLWD, CIFOR and CAWASAR, NCSCD, Toochi2019, Terentieva et al. (2016), and Hess et al. (2015). (a) Spatial distribution of uncertainty in wetland fraction. (b) Number of products used in the calculation.
Our study has demonstrated that the spatial distributions of WAD2M based on the data fusion approach show reasonable improvements over existing wetland products and the temporal variations in WAD2M adequately represent interannual variation in response to the climate events such as El Niño–Southern Oscillation. However, the data fusion approach in this study largely relies on the quality of inventories to correct the regions which SWAMPS has limited capacity to detect, which could potentially inflate wetland area in WAD2M when inventories overestimate inundation extent. Due to the scarcity of ground-truth maps for representative regions, further work is needed to confirm the spatiotemporal distribution of inundation captured by WAD2M. In particular, the sensitivity to subcanopy inundation, the a priori knowledge of land cover applied in the retrieval algorithm, and the length of observations can affect the overall accuracy of SWAMPS and thus contribute to the uncertainty in WAD2M. For instance, WAD2M reports a vast inundated area in the Sahel region where validation of the SWAMPS retrieval algorithm is lacking due to sparsity of dynamic ground observations (Jensen and McDonald, 2019). In addition, the retrievals of SWAMPS tend to be affected by the low surface emissivity due to the outcrops of limestone deposits in semiarid and arid regions (Schroeder et al., 2015), which thus potentially causes overestimation in inundation in these regions. Moreover, the decadal trends of WAD2M are influenced by the intercalibration of brightness temperature across different microwave sensors, which could potentially introduce inconsistency between different time periods covered by the measurements. Thus, it is important to be cautious with the interpretation of the long-term trends based on WAD2M. Lastly, because the GSW and MIRCA2000 data sources are aggregated to a 0.25∘ spatial resolution in the processing of WAD2M, the potential overlapping between these two mergers at a fine spatial resolution is ignored, leading to unintentional double accounting when deducting open water and rice paddies from WAD2M.
Future refinements to WAD2M could come from (1) improvements to revisit spatial resolution, spectral range, and the signal-to-noise ratio of remotely sensed data input and (2) refinements to our fusion methodology to use uncertainties to generate ensemble maps. Several new or upcoming satellite missions may provide improved global wetland dynamics in the future version of WAD2M. The Cyclone Global Navigation Satellite System (CYGNSS) GNSS reflectometry (GNSS-R) (Nghiem et al., 2017) demonstrates its capabilities to detect inundation under different vegetation conditions, which is complementary to inventories for evaluation. The NASA Surface Water and Ocean Topography (SWOT), the Copernicus L-band SAR Radar Observing System for Europe (ROSE-L) (Pierdicca et al., 2019), and NASA-ISRO SAR (NISAR) missions will greatly increase our capacity to monitor the spatiotemporal dynamics of wetlands and floodplains at high spatial resolutions (< 50 m), making them immensely valuable resources in the future work of wetland dynamic mapping such as by WAD2M. Commercial satellites provide even higher spatial resolutions with daily revisits; i.e., the Planet Labs Dove constellation, which is intercalibrated, could go beyond providing static maps and provide time series of wetland data (Cooley et al., 2017).
For the methodology, combining products from different satellite sensors (e.g., optical and microwave) and inventories has been proved to be a feasible way to reduce the bias in the spatial distribution of wetlands and provide reliable estimates for the use of global wetland CH4 studies. However, the spatial resolution of WAD2M is dictated by the resolution of its input data on the wetland dynamic dataset unless a downscaling methodology is applied. Downscaling can also be used to improve spatial resolution using machine learning approaches (Alemohammad et al., 2018; Kratzert et al., 2018; Wu et al., 2017) or physically based hydrological models (Gumbricht, 2018), together with higher-resolution images (e.g., Landsat, ALOS, ALOS-2) which are better suited to capturing inundation features at fine scales. On the other hand, inventories at the regional and national scales are needed for some relatively under-examined wetlands (e.g., in Africa and Southeast Asia), which will help in reliable validation and evaluation for these regions in future quantitative studies of wetlands. Moreover, even with better sensors in the future, improvements on wetland maps from past and future satellite will be necessary for the backward extension of time series.
The global wetland dynamic dataset in NetCDF format is publicly available at https://doi.org/10.5281/zenodo.3998454 (Zhang et al., 2020).
The development of a global wetland product WAD2M has demonstrated the capability to produce maps of wetlands and inundation that are consistent with independent datasets. Combining temporal dynamics from the coarse-resolution product SWAMPS with complementary products and inventories was shown to be a practical means of tracking variations in global wetland extent over time. WAD2M represents the most reliable representation of global vegetated wetland distribution to date and will be useful to estimate wetland CH4 flux. WAD2M provides valuable information for a range of applications, ranging from understanding the role of floodplains to carbon modeling and general assessment of global response to climate change.
BP and ZZ conceived the work. All authors contributed to development of the wetland dataset, analysis of results, and writing of the manuscript.
The authors declare that they have no conflict of interest.
Katherine Jensen's effort was supported through an award from the NASA Earth and Space Science Fellowship Program under grant 80NSSC17K0387. Development of the SWAMPS dataset was supported by the NASA Making Earth System Data Records for Use in Research Environments Program under cooperative agreement NNX11AQ39G and cooperative agreement NNX11AP26A. Portions of the work were carried out at the Jet Propulsion Laboratory, California Institute of Technology, under contract to the National Aeronautics and Space Administration. Annett Bartsch's, Gustaf Hugelius', and Thomas Gumbricht's contributions are supported through the Nunataryuk project, funded under the European Union's Horizon 2020 Research and Innovation Programme under grant agreement no. 77342. The wetland mapping was partly ran in cooperation with the Center for International Forestry Research (CIFOR) and its SWAMP program (Sustainable Wetlands Adaptation and Mitigation Program), with support from US Agency for International Development (USAID) grant no. MTO069018. The development of global models for geomorphology and hydrology was partly carried out with support from the World Agroforestry Centre (ICRAF). We would also like to acknowledge the CGIAR research programs on Forests, Trees and Agroforestry (FTA) and Climate Change, Agriculture and Food Security (CCAFS).
This research has been supported by the Gordon and Betty Moore Foundation (grant no. GBMF5439) and the Strategic Priority Research Program of the Chinese Academy of Sciences (grant nos. XDA19040504 and XDA19070204).
This paper was edited by David Carlson and reviewed by Joe Melton and one anonymous referee.
Aires, F., Miolane, L., Prigent, C., Pham, B., Fluet-Chouinard, E., Lehner, B., and Papa, F.: A Global Dynamic Long-Term Inundation Extent Dataset at High Spatial Resolution Derived through Downscaling of Satellite Observations, J. Hydrometeorol., 18, 1305–1325, https://doi.org/10.1175/JHM-D-16-0155.1, 2017.
Aires, F., Prigent, C., Fluet-Chouinard, E., Yamazaki, D., Papa, F., and Lehner, B.: Comparison of visible and multi-satellite global inundation datasets at high-spatial resolution, Remote Sens. Environ., 216, 427–441, https://doi.org/10.1016/j.rse.2018.06.015, 2018.
Alemohammad, S. H., Kolassa, J., Prigent, C., Aires, F., and Gentine, P.: Global downscaling of remotely sensed soil moisture using neural networks, Hydrol. Earth Syst. Sci., 22, 5341–5356, https://doi.org/10.5194/hess-22-5341-2018, 2018.
Allen, G. H. and Pavelsky, T. M.: Global extent of rivers and streams, Science, 361, 585–588, https://doi.org/10.1126/science.aat0636, 2018.
Alsdorf, D. E., Melack, J. M., Dunne, T., Mertes, L. A. K., Hess, L. L., and Smith, L. C.: Interferometric radar measurements of water level changes on the Amazon flood plain, Nature, 404, 174–177, https://doi.org/10.1038/35004560, 2000.
Alsdorf, D. E., Rodríguez, E., and Lettenmaier, D. P.: Measuring surface water from space, Rev. Geophys., 45, RG2002, https://doi.org/10.1029/2006RG000197, 2007.
Amani, M., Mahdavi, S., Afshar, M., Brisco, B., Huang, W., Mohammad Javad Mirzadeh, S., White, L., Banks, S., Montgomery, J., and Hopkinson, C.: Canadian Wetland Inventory using Google Earth Engine: The First Map and Preliminary Results, Remote Sens.-Basel, 11, 842, https://doi.org/10.3390/rs11070842, 2019.
Barba, J., Bradford, M. A., Brewer, P. E., Bruhn, D., Covey, K., Haren, J., Megonigal, J. P., Mikkelsen, T. N., Pangala, S. R., Pihlatie, M., Poulter, B., Rivas-Ubach, A., Schadt, C. W., Terazawa, K., Warner, D. L., Zhang, Z., and Vargas, R.: Methane emissions from tree stems: a new frontier in the global carbon cycle, New Phytol., 222, 18–28, https://doi.org/10.1111/nph.15582, 2019.
Bartsch, A., Widhalm, B., Kuhry, P., Hugelius, G., Palmtag, J., and Siewert, M. B.: Can C-band synthetic aperture radar be used to estimate soil organic carbon storage in tundra?, Biogeosciences, 13, 5453–5470, https://doi.org/10.5194/bg-13-5453-2016, 2016.
Bloom, A. A., Palmer, P. I., Fraser, A., Reay, D. S., and Frankenberg, C.: Large-Scale Controls of Methanogenesis Inferred from Methane and Gravity Spaceborne Data, Science, 327, 322–325, https://doi.org/10.1126/science.1175176, 2010.
Boening, C., Willis, J. K., Landerer, F. W., Nerem, R. S., and Fasullo, J.: The 2011 La Niña: So strong, the oceans fell, Geophys. Res. Lett., 39, L19602, https://doi.org/10.1029/2012GL053055, 2012.
Bohn, T. J., Melton, J. R., Ito, A., Kleinen, T., Spahni, R., Stocker, B. D., Zhang, B., Zhu, X., Schroeder, R., Glagolev, M. V., Maksyutov, S., Brovkin, V., Chen, G., Denisov, S. N., Eliseev, A. V., Gallego-Sala, A., McDonald, K. C., Rawlins, M. A., Riley, W. J., Subin, Z. M., Tian, H., Zhuang, Q., and Kaplan, J. O.: WETCHIMP-WSL: intercomparison of wetland methane emissions models over West Siberia, Biogeosciences, 12, 3321–3349, https://doi.org/10.5194/bg-12-3321-2015, 2015.
Carroll, M. and Loboda, T.: Multi-Decadal Surface Water Dynamics in North American Tundra, Remote Sens.-Basel, 9, 497, https://doi.org/10.3390/rs9050497, 2017.
Carroll, M., Townshend, J., Dimiceli, C., Noojipady, P., and Sohlberg, R.: A new global raster water mask at 250 m resolution, Int. J. Digit. Earth, 2, 291–308, https://doi.org/10.1080/17538940902951401, 2009.
Cooley, S. W., Smith, L. C., Stepan, L., and Mascaro, J.: Tracking Dynamic Northern Surface Water Changes with High-Frequency Planet CubeSat Imagery, Remote Sens.-Basel, 9, 1306, https://doi.org/10.3390/rs9121306, 2017.
Cowardin, L. M., Carter, V., Golet, F. C., and LaRoe, E. T.: Classification of wetlands and deepwater habitats of the United States, U.S. Department of the Interior, Fish and Wildlife Service, Jamestown, Washington, D.C., USA, Northern Prairie Wildlife Research Center Online, (Version 04DEC1998), available at: https://www.fws.gov/wetlands/documents/classification-of-wetlands-and-deepwater-habitats-of-the-united-states.pdf (last access: 6 May 2021), 1979.
Dargie, G. C., Lewis, S. L., Lawson, I. T., Mitchard, E. T. A., Page, S. E., Bocko, Y. E., and Ifo, S. A.: Age, extent and carbon storage of the central Congo Basin peatland complex, Nature, 542, 86–90, https://doi.org/10.1038/nature21048, 2017.
Davidson, N. C., Fluet-Chouinard, E., and Finlayson, C. M.: Global extent and distribution of wetlands: trends and issues, Mar. Freshwater Res., 69, 620–627, https://doi.org/10.1071/MF17019, 2018.
DeVries, B., Huang, C., Lang, M., Jones, J., Huang, W., Creed, I., and Carroll, M.: Automated Quantification of Surface Water Inundation in Wetlands Using Optical Satellite Imagery, Remote Sens.-Basel, 9, 807, https://doi.org/10.3390/rs9080807, 2017.
Du, J., Kimball, J. S., Jones, L. A., and Watts, J. D.: Implementation of satellite based fractional water cover indices in the pan-Arctic region using AMSR-E and MODIS, Remote Sens. Environ., 184, 469–481, https://doi.org/10.1016/j.rse.2016.07.029, 2016.
Du, J., Kimball, J. S., Galantowicz, J., Kim, S.-B., Chan, S. K., Reichle, R., Jones, L. A., and Watts, J. D.: Assessing global surface water inundation dynamics using combined satellite information from SMAP, AMSR2 and Landsat, Remote Sens. Environ., 213, 1–17, https://doi.org/10.1016/j.rse.2018.04.054, 2018.
Dunne, T. and Aalto, R. E.: 9.32 Large River Floodplains, in: Treatise on Geomorphology, edited by: Shroder, J. F., Academic Press, San Diego, USA, 645–678, 2013.
Feng, M., Sexton, J. O., Channan, S., and Townshend, J. R.: A global, high-resolution (30 m) inland water body dataset for 2000: first results of a topographic-spectral classification algorithm, Int. J. Digit. Earth, 9, 113–133, https://doi.org/10.1080/17538947.2015.1026420, 2016.
Finlayson, C. M., Davidson, N. C., Spiers, A. G., and Stevenson, N. J.: Global wetland inventory – current status and future priorities, Mar. Freshwater Res., 50, 717–727, https://doi.org/10.1071/mf99098, 1999.
Fluet-Chouinard, E., Lehner, B., Rebelo, L.-M., Papa, F., and Hamilton, S. K.: Development of a global inundation map at high spatial resolution from topographic downscaling of coarse-scale remote sensing data, Remote Sens. Environ., 158, 348–361, https://doi.org/10.1016/j.rse.2014.10.015, 2015.
Gallant, A. L.: The Challenges of Remote Monitoring of Wetlands, Remote Sens.-Basel, 7, 10938–10950, https://doi.org/10.3390/rs70810938, 2015.
Grinham, A., Albert, S., Deering, N., Dunbabin, M., Bastviken, D., Sherman, B., Lovelock, C. E., and Evans, C. D.: The importance of small artificial water bodies as sources of methane emissions in Queensland, Australia, Hydrol. Earth Syst. Sci., 22, 5281–5298, https://doi.org/10.5194/hess-22-5281-2018, 2018.
Gumbricht, T.: Detecting Trends in Wetland Extent from MODIS Derived Soil Moisture Estimates, Remote Sens.-Basel, 10, 611, https://doi.org/10.3390/rs10040611, 2018.
Gumbricht, T., Roman-Cuesta, R. M., Verchot, L., Herold, M., Wittmann, F., Householder, E., Herold, N., and Murdiyarso, D.: An expert system model for mapping tropical wetlands and peatlands reveals South America as the largest contributor, Global Change Biol., 23, 3581–3599, https://doi.org/10.1111/gcb.13689, 2017.
Hess, L. L., Melack, J. M., Affonso, A. G., Barbosa, C., Gastil-Buhl, M., and Novo, E. M. L. M.: Wetlands of the Lowland Amazon Basin: Extent, Vegetative Cover, and Dual-season Inundated Area as Mapped with JERS-1 Synthetic Aperture Radar, Wetlands, 35, 745–756, https://doi.org/10.1007/s13157-015-0666-y, 2015.
Hijmans, R. J., Cameron, S. E., Parra, J. L., Jones, P. G., and Jarvis, A.: Very high resolution interpolated climate surfaces for global land areas, Int. J. Climatol., 25, 1965–1978, https://doi.org/10.1002/joc.1276, 2005.
Hiraishi, T., Krug, T., Tanabe, K., Srivastava, N., Baasansuren, J., Fukuda, M., and Troxler, T. G.: IPCC 2014, 2013 Supplement to the 2006 IPCC Guidelines for National Greenhouse Gas Inventories: Wetlands, IPCC, Switzerland, 2014.
Hu, H., Landgraf, J., Detmers, R., Borsdorff, T., Aan de Brugh, J., Aben, I., Butz, A., and Hasekamp, O.: Toward Global Mapping of Methane With TROPOMI: First Results and Intersatellite Comparison to GOSAT, Geophys. Res. Lett., 45, 3682–3689, https://doi.org/10.1002/2018GL077259, 2018.
Hu, S., Niu, Z., Chen, Y., Li, L., and Zhang, H.: Global wetlands: Potential distribution, wetland loss, and status, Sci. Total Environ., 586, 319–327, https://doi.org/10.1016/j.scitotenv.2017.02.001, 2017.
Huang, C., Peng, Y., Lang, M., Yeo, I.-Y., and McCarty, G.: Wetland inundation mapping and change monitoring using Landsat and airborne LiDAR data, Remote Sens. Environ., 141, 231–242, https://doi.org/10.1016/j.rse.2013.10.020, 2014.
Hugelius, G., Tarnocai, C., Broll, G., Canadell, J. G., Kuhry, P., and Swanson, D. K.: The Northern Circumpolar Soil Carbon Database: spatially distributed datasets of soil coverage and soil carbon storage in the northern permafrost regions, Earth Syst. Sci. Data, 5, 3–13, https://doi.org/10.5194/essd-5-3-2013, 2013.
Hugelius, G., Loisel, J., Chadburn, S., Jackson, R. B., Jones, M., MacDonald, G., Marushchak, M., Olefeldt, D., Packalen, M., Siewert, M. B., Treat, C., Turetsky, M., Voigt, C., and Yu, Z.: Large stocks of peatland carbon and nitrogen are vulnerable to permafrost thaw, P. Natl. Acad. Sci. USA, 117, 20438–20446, https://doi.org/10.1073/pnas.1916387117, 2020.
IPCC: Climate Change 2013: The Physical Science Basis, in: Contribution of Working Group I to the Fifth Assessment Report of the Intergovernmental Panel on Climate Change, Cambridge University Press, Cambridge, United Kingdom and New York, USA, available at: https://www.ipcc.ch/report/ar5/wg1/ (last access: 3 July 2019), 2013.
Jensen, K. and McDonald, K.: Surface Water Microwave Product Series Version 3: A Near-Real Time and 25-Year Historical Global Inundated Area Fraction Time Series From Active and Passive Microwave Remote Sensing, IEEE Geosci. Remote S., 16, 1402–1406, https://doi.org/10.1109/LGRS.2019.2898779, 2019.
Jin, H., Huang, C., Lang, M. W., Yeo, I.-Y., and Stehman, S. V.: Monitoring of wetland inundation dynamics in the Delmarva Peninsula using Landsat time-series imagery from 1985 to 2011, Remote Sens. Environ., 190, 26–41, https://doi.org/10.1016/j.rse.2016.12.001, 2017.
Jones, J. W.: Improved Automated Detection of Subpixel-Scale Inundation – Revised Dynamic Surface Water Extent (DSWE) Partial Surface Water Tests, Remote Sens.-Basel, 11, 374, https://doi.org/10.3390/rs11040374, 2019.
Junk, W. J.: Current state of knowledge regarding South America wetlands and their future under global climate change, Aqua. Sci., 75, 113–131, https://doi.org/10.1007/s00027-012-0253-8, 2013.
Kerr, Y., Philippe, W., Richaume, P., Wigneron, J.-P., Ferrazzoli, P., Mahmoodi, A., Al Bitar, A., Cabot, F., Gruhier, C., Juglea, S., Leroux, D., and Mialon, A.: The SMOS soil moisture retrieval algorithm, IEEE T. Geosci. Remote, 50, 1384–1403, https://doi.org/10.1109/TGRS.2012.2184548, 2012.
Kirschke, S., Bousquet, P., Ciais, P., Saunois, M., Canadell, J. G., Dlugokencky, E. J., Bergamaschi, P., Bergmann, D., Blake, D. R., Bruhwiler, L., Cameron-Smith, P., Castaldi, S., Chevallier, F., Feng, L., Fraser, A., Heimann, M., Hodson, E. L., Houweling, S., Josse, B., Fraser, P. J., Krummel, P. B., Lamarque, J.-F., Langenfelds, R. L., Le Quéré, C., Naik, V., O'Doherty, S., Palmer, P. I., Pison, I., Plummer, D., Poulter, B., Prinn, R. G., Rigby, M., Ringeval, B., Santini, M., Schmidt, M., Shindell, D. T., Simpson, I. J., Spahni, R., Steele, L. P., Strode, S. A., Sudo, K., Szopa, S., van der Werf, G. R., Voulgarakis, A., van Weele, M., Weiss, R. F., Williams, J. E., and Zeng, G.: Three decades of global methane sources and sinks, Nat. Geosci., 6, 813–823, https://doi.org/10.1038/ngeo1955, 2013.
Kleinen, T., Brovkin, V., and Schuldt, R. J.: A dynamic model of wetland extent and peat accumulation: results for the Holocene, Biogeosciences, 9, 235–248, https://doi.org/10.5194/bg-9-235-2012, 2012.
Knox, S. H., Jackson, R. B., Poulter, B., McNicol, G., Fluet-Chouinard, E., Zhang, Z., Hugelius, G., Bousquet, P., Canadell, J. G., Saunois, M., Papale, D., Chu, H., Keenan, T. F., Baldocchi, D., Torn, M. S., Mammarella, I., Trotta, C., Aurela, M., Bohrer, G., Campbell, D. I., Cescatti, A., Chamberlain, S., Chen, J., Chen, W., Dengel, S., Desai, A. R., Euskirchen, E., Friborg, T., Gasbarra, D., Goded, I., Goeckede, M., Heimann, M., Helbig, M., Hirano, T., Hollinger, D. Y., Iwata, H., Kang, M., Klatt, J., Krauss, K. W., Kutzbach, L., Lohila, A., Mitra, B., Morin, T. H., Nilsson, M. B., Niu, S., Noormets, A., Oechel, W. C., Peichl, M., Peltola, O., Reba, M. L., Richardson, A. D., Runkle, B. R. K., Ryu, Y., Sachs, T., Schäfer, K. V. R., Schmid, H. P., Shurpali, N., Sonnentag, O., Tang, A. C. I., Ueyama, M., Vargas, R., Vesala, T., Ward, E. J., Windham-Myers, L., Wohlfahrt, G., and Zona, D.: FLUXNET-CH4 Synthesis Activity: Objectives, Observations, and Future Directions, B. Am. Meteorol. Soc., 100, 2607–2632, https://doi.org/10.1175/BAMS-D-18-0268.1, 2019.
Kratzert, F., Klotz, D., Brenner, C., Schulz, K., and Herrnegger, M.: Rainfall–runoff modelling using Long Short-Term Memory (LSTM) networks, Hydrol. Earth Syst. Sci., 22, 6005–6022, https://doi.org/10.5194/hess-22-6005-2018, 2018.
Landerer, F. W. and Swenson, S. C.: Accuracy of scaled GRACE terrestrial water storage estimates, Water Resour. Res., 48, W04531, https://doi.org/10.1029/2011WR011453, 2012.
Lehner, B. and Döll, P.: Development and validation of a global database of lakes, reservoirs and wetlands, J. Hydrol., 296, 1–22, https://doi.org/10.1016/j.jhydrol.2004.03.028, 2004.
Lehner, B. and Grill, G.: Global river hydrography and network routing: baseline data and new approaches to study the world's large river systems: global river hydrography and network routing, Hydrol. Process., 27, 2171–2186, https://doi.org/10.1002/hyp.9740, 2013.
Li, L., Skidmore, A., Vrieling, A., and Wang, T.: A new dense 18-year time series of surface water fraction estimates from MODIS for the Mediterranean region, Hydrol. Earth Syst. Sci., 23, 3037–3056, https://doi.org/10.5194/hess-23-3037-2019, 2019.
Lunt, M. F., Palmer, P. I., Feng, L., Taylor, C. M., Boesch, H., and Parker, R. J.: An increase in methane emissions from tropical Africa between 2010 and 2016 inferred from satellite data, Atmos. Chem. Phys., 19, 14721–14740, https://doi.org/10.5194/acp-19-14721-2019, 2019.
Matthews, E. and Fung, I.: Methane emission from natural wetlands: Global distribution, area, and environmental characteristics of sources, Global Biogeochem. Cy., 1, 61–86, https://doi.org/10.1029/GB001i001p00061, 1987.
Mahdianpari, M., Salehi, B., Mohammadimanesh, F., Brisco, B., Homayouni, S., Gill, E., DeLancey, E. R., and Bourgeau-Chavez, L.: Big Data for a Big Country: The First Generation of Canadian Wetland Inventory Map at a Spatial Resolution of 10 m Using Sentinel-1 and Sentinel-2 Data on the Google Earth Engine Cloud Computing Platform, Can. J. Remote Sens., 46, 15–33, https://doi.org/10.1080/07038992.2019.1711366, 2020.
Melack, J. M., Hess, L. L., Gastil, M., Forsberg, B. R., Hamilton, S. K., Lima, I. B. T., and Novo, E. M. L. M.: Regionalization of methane emissions in the Amazon Basin with microwave remote sensing, Global Change Biol., 10, 530–544, https://doi.org/10.1111/j.1365-2486.2004.00763.x, 2004.
Melton, J. R., Wania, R., Hodson, E. L., Poulter, B., Ringeval, B., Spahni, R., Bohn, T., Avis, C. A., Beerling, D. J., Chen, G., Eliseev, A. V., Denisov, S. N., Hopcroft, P. O., Lettenmaier, D. P., Riley, W. J., Singarayer, J. S., Subin, Z. M., Tian, H., Zürcher, S., Brovkin, V., van Bodegom, P. M., Kleinen, T., Yu, Z. C., and Kaplan, J. O.: Present state of global wetland extent and wetland methane modelling: conclusions from a model inter-comparison project (WETCHIMP), Biogeosciences, 10, 753–788, https://doi.org/10.5194/bg-10-753-2013, 2013.
Messager, M. L., Lehner, B., Grill, G., Nedeva, I., and Schmitt, O.: Estimating the volume and age of water stored in global lakes using a geo-statistical approach, Nat. Commun., 7, 13603, https://doi.org/10.1038/ncomms13603, 2016.
Muster, S., Roth, K., Langer, M., Lange, S., Cresto Aleina, F., Bartsch, A., Morgenstern, A., Grosse, G., Jones, B., Sannel, A. B. K., Sjöberg, Y., Günther, F., Andresen, C., Veremeeva, A., Lindgren, P. R., Bouchard, F., Lara, M. J., Fortier, D., Charbonneau, S., Virtanen, T. A., Hugelius, G., Palmtag, J., Siewert, M. B., Riley, W. J., Koven, C. D., and Boike, J.: PeRL: a circum-Arctic Permafrost Region Pond and Lake database, Earth Syst. Sci. Data, 9, 317–348, https://doi.org/10.5194/essd-9-317-2017, 2017.
Nardi, F., Annis, A., Baldassarre, G. D., Vivoni, E. R., and Grimaldi, S.: GFPLAIN250m, a global high-resolution dataset of Earth's floodplains, Scientific Data, 6, 180309, https://doi.org/10.1038/sdata.2018.309, 2019.
Nghiem, S. V., Zuffada, C., Shah, R., Chew, C., Lowe, S. T., Mannucci, A. J., Cardellach, E., Brakenridge, G. R., Geller, G., and Rosenqvist, A.: Wetland monitoring with Global Navigation Satellite System reflectometry: Wetland Monitoring With GNSS-R, Earth Space Sci., 4, 16–39, https://doi.org/10.1002/2016EA000194, 2017.
Pandey, S., Houweling, S., Lorente, A., Borsdorff, T., Tsivlidou, M., Bloom, A. A., Poulter, B., Zhang, Z., and Aben, I.: Using satellite data to identify the methane emission controls of South Sudan's wetlands, Biogeosciences, 18, 557–572, https://doi.org/10.5194/bg-18-557-2021, 2021.
Pangala, S. R., Enrich-Prast, A., Basso, L. S., Peixoto, R. B., Bastviken, D., Hornibrook, E. R. C., Gatti, L. V., Marotta, H., Calazans, L. S. B., Sakuragui, C. M., Bastos, W. R., Malm, O., Gloor, E., Miller, J. B., and Gauci, V.: Large emissions from floodplain trees close the Amazon methane budget, Nature, 552, 230–234, https://doi.org/10.1038/nature24639, 2017.
Papa, F., Prigent, C., Aires, F., Jimenez, C., Rossow, W. B., and Matthews, E.: Interannual variability of surface water extent at the global scale, 1993–2004, J. Geophys. Res.-Atmos., 115, D12111, https://doi.org/10.1029/2009JD012674, 2010.
Parker, R. J., Boesch, H., McNorton, J., Comyn-Platt, E., Gloor, M., Wilson, C., Chipperfield, M. P., Hayman, G. D., and Bloom, A. A.: Evaluating year-to-year anomalies in tropical wetland methane emissions using satellite CH4 observations, Remote Sens. Environ., 211, 261–275, https://doi.org/10.1016/j.rse.2018.02.011, 2018.
Pekel, J.-F., Cottam, A., Gorelick, N., and Belward, A. S.: High-resolution mapping of global surface water and its long-term changes, Nature, 540, 418–422, https://doi.org/10.1038/nature20584, 2016.
Peltola, O., Vesala, T., Gao, Y., Räty, O., Alekseychik, P., Aurela, M., Chojnicki, B., Desai, A. R., Dolman, A. J., Euskirchen, E. S., Friborg, T., Göckede, M., Helbig, M., Humphreys, E., Jackson, R. B., Jocher, G., Joos, F., Klatt, J., Knox, S. H., Kowalska, N., Kutzbach, L., Lienert, S., Lohila, A., Mammarella, I., Nadeau, D. F., Nilsson, M. B., Oechel, W. C., Peichl, M., Pypker, T., Quinton, W., Rinne, J., Sachs, T., Samson, M., Schmid, H. P., Sonnentag, O., Wille, C., Zona, D., and Aalto, T.: Monthly gridded data product of northern wetland methane emissions based on upscaling eddy covariance observations, Earth Syst. Sci. Data, 11, 1263–1289, https://doi.org/10.5194/essd-11-1263-2019, 2019.
Pham-Duc, B., Prigent, C., Aires, F., and Papa, F.: Comparisons of Global Terrestrial Surface Water Datasets over 15 Years, J. Hydrometeorol., 18, 993–1007, https://doi.org/10.1175/JHM-D-16-0206.1, 2017.
Pickens, A. H., Hansen, M. C., Hancher, M., Stehman, S. V., Tyukavina, A., Potapov, P., Marroquin, B., and Sherani, Z.: Mapping and sampling to characterize global inland water dynamics from 1999 to 2018 with full Landsat time-series, Remote Sens. Environ., 243, 111792, https://doi.org/10.1016/j.rse.2020.111792, 2020.
Pierdicca, N., Davidson, M., Chini, M., Dierking, W., Djavidnia, S., Haarpaintner, J., Hajduch, G., Laurin, G. V., Lavalle, M., López-Martínez, C., Nagler, T., and Su, B.: The Copernicus L-band SAR mission ROSE-L (Radar Observing System for Europe), Conference Presentation, in: Active and Passive Microwave Remote Sensing for Environmental Monitoring III, International Society for Optics and Photonics, 2019, Strasbourg, France, 111540E, 2019.
Portmann, F. T., Siebert, S., and Döll, P.: MIRCA2000-Global monthly irrigated and rainfed crop areas around the year 2000: A new high-resolution data set for agricultural and hydrological modeling: Monthly irrigated and rainfed crop areas, Global Biogeochem. Cy., 24, GB1011, https://doi.org/10.1029/2008GB003435, 2010.
Poulter, B., Bousquet, P., Canadell, J. G., Ciais, P., Peregon, A., Saunois, M., Arora, V. K., Beerling, D. J., Brovkin, V., Jones, C. D., Joos, F., Gedney, N., Ito, A., Kleinen, T., Koven, C. D., McDonald, K., Melton, J. R., Peng, C., Peng, S., Prigent, C., Schroeder, R., Riley, W. J., Saito, M., Spahni, R., Tian, H., Taylor, L., Viovy, N., Wilton, D., Wiltshire, A., Xu, X., Zhang, B., Zhang, Z., and Zhu, Q.: Global wetland contribution to 2000–2012 atmospheric methane growth rate dynamics, Environ. Res. Lett., 12, 094013, https://doi.org/10.1088/1748-9326/aa8391, 2017.
Prigent, C., Matthews, E., Aires, F., and Rossow, W. B.: Remote sensing of global wetland dynamics with multiple satellite data sets, Geophys. Res. Lett., 28, 4631–4634, https://doi.org/10.1029/2001GL013263, 2001.
Prigent, C., Papa, F., Aires, F., Rossow, W. B., and Matthews, E.: Global inundation dynamics inferred from multiple satellite observations, 1993–2000, J. Geophys. Res.-Atmos., 112, D12107, https://doi.org/10.1029/2006JD007847, 2007.
Prigent, C., Papa, F., Aires, F., Jimenez, C., Rossow, W. B., and Matthews, E.: Changes in land surface water dynamics since the 1990's and relation to population pressure: Land surface water dynamics, Geophys. Res. Lett., 39, L08403, https://doi.org/10.1029/2012GL051276, 2012.
Prigent, C., Jimenez, C., and Bousquet, P.: Satellite-Derived Global Surface Water Extent and Dynamics Over the Last 25 Years (GIEMS-2), J. Geophys. Res.-Atmos., 125, e2019JD030711, https://doi.org/10.1029/2019JD030711, 2020.
Reichle, R.: SMAP L4 Global 3-hourly 9 km EASE-Grid Surface and Root Zone Soil Moisture Geophysical Data, Version 4, NASA National Snow and Ice Data Center Distributed Active Archive Center, Boulder, Colorado, USA, https://doi.org/10.5067/kpjnn2gi1dqr, 2018.
Reschke, J., Bartsch, A., Schlaffer, S., and Schepaschenko, D.: Capability of C-Band SAR for Operational Wetland Monitoring at High Latitudes, Remote Sens.-Basel, 4, 2923–2943, https://doi.org/10.3390/rs4102923, 2012.
Ringeval, B., de Noblet-Ducoudré, N., Ciais, P., Bousquet, P., Prigent, C., Papa, F., and Rossow, W. B.: An attempt to quantify the impact of changes in wetland extent on methane emissions on the seasonal and interannual time scales: Wetland extent's changes and CH4 emissions, Global Biogeochem. Cy., 24, GB2003, https://doi.org/10.1029/2008GB003354, 2010.
Rodell, M., Famiglietti, J. S., Wiese, D. N., Reager, J. T., Beaudoing, H. K., Landerer, F. W., and Lo, M.-H.: Emerging trends in global freshwater availability, Nature, 557, 651–659, https://doi.org/10.1038/s41586-018-0123-1, 2018.
Salmon, J. M., Friedl, M. A., Frolking, S., Wisser, D., and Douglas, E. M.: Global rain-fed, irrigated, and paddy croplands: A new high resolution map derived from remote sensing, crop inventories and climate data, Int. J. Appl. Earth Obs., 38, 321–334, https://doi.org/10.1016/j.jag.2015.01.014, 2015.
Saunois, M., Bousquet, P., Poulter, B., Peregon, A., Ciais, P., Canadell, J. G., Dlugokencky, E. J., Etiope, G., Bastviken, D., Houweling, S., Janssens-Maenhout, G., Tubiello, F. N., Castaldi, S., Jackson, R. B., Alexe, M., Arora, V. K., Beerling, D. J., Bergamaschi, P., Blake, D. R., Brailsford, G., Brovkin, V., Bruhwiler, L., Crevoisier, C., Crill, P., Covey, K., Curry, C., Frankenberg, C., Gedney, N., Höglund-Isaksson, L., Ishizawa, M., Ito, A., Joos, F., Kim, H.-S., Kleinen, T., Krummel, P., Lamarque, J.-F., Langenfelds, R., Locatelli, R., Machida, T., Maksyutov, S., McDonald, K. C., Marshall, J., Melton, J. R., Morino, I., Naik, V., O'Doherty, S., Parmentier, F.-J. W., Patra, P. K., Peng, C., Peng, S., Peters, G. P., Pison, I., Prigent, C., Prinn, R., Ramonet, M., Riley, W. J., Saito, M., Santini, M., Schroeder, R., Simpson, I. J., Spahni, R., Steele, P., Takizawa, A., Thornton, B. F., Tian, H., Tohjima, Y., Viovy, N., Voulgarakis, A., van Weele, M., van der Werf, G. R., Weiss, R., Wiedinmyer, C., Wilton, D. J., Wiltshire, A., Worthy, D., Wunch, D., Xu, X., Yoshida, Y., Zhang, B., Zhang, Z., and Zhu, Q.: The global methane budget 2000–2012, Earth Syst. Sci. Data, 8, 697–751, https://doi.org/10.5194/essd-8-697-2016, 2016.
Saunois, M., Stavert, A. R., Poulter, B., Bousquet, P., Canadell, J. G., Jackson, R. B., Raymond, P. A., Dlugokencky, E. J., Houweling, S., Patra, P. K., Ciais, P., Arora, V. K., Bastviken, D., Bergamaschi, P., Blake, D. R., Brailsford, G., Bruhwiler, L., Carlson, K. M., Carrol, M., Castaldi, S., Chandra, N., Crevoisier, C., Crill, P. M., Covey, K., Curry, C. L., Etiope, G., Frankenberg, C., Gedney, N., Hegglin, M. I., Höglund-Isaksson, L., Hugelius, G., Ishizawa, M., Ito, A., Janssens-Maenhout, G., Jensen, K. M., Joos, F., Kleinen, T., Krummel, P. B., Langenfelds, R. L., Laruelle, G. G., Liu, L., Machida, T., Maksyutov, S., McDonald, K. C., McNorton, J., Miller, P. A., Melton, J. R., Morino, I., Müller, J., Murguia-Flores, F., Naik, V., Niwa, Y., Noce, S., O'Doherty, S., Parker, R. J., Peng, C., Peng, S., Peters, G. P., Prigent, C., Prinn, R., Ramonet, M., Regnier, P., Riley, W. J., Rosentreter, J. A., Segers, A., Simpson, I. J., Shi, H., Smith, S. J., Steele, L. P., Thornton, B. F., Tian, H., Tohjima, Y., Tubiello, F. N., Tsuruta, A., Viovy, N., Voulgarakis, A., Weber, T. S., van Weele, M., van der Werf, G. R., Weiss, R. F., Worthy, D., Wunch, D., Yin, Y., Yoshida, Y., Zhang, W., Zhang, Z., Zhao, Y., Zheng, B., Zhu, Q., Zhu, Q., and Zhuang, Q.: The Global Methane Budget 2000–2017, Earth Syst. Sci. Data, 12, 1561–1623, https://doi.org/10.5194/essd-12-1561-2020, 2020.
Schroeder, R., McDonald, K., Chapman, B., Jensen, K., Podest, E., Tessler, Z., Bohn, T., and Zimmermann, R.: Development and Evaluation of a Multi-Year Fractional Surface Water Data Set Derived from Active/Passive Microwave Remote Sensing Data, Remote Sens.-Basel, 7, 16688–16732, https://doi.org/10.3390/rs71215843, 2015.
Stocker, B. D., Spahni, R., and Joos, F.: DYPTOP: a cost-efficient TOPMODEL implementation to simulate sub-grid spatio-temporal dynamics of global wetlands and peatlands, Geosci. Model Dev., 7, 3089–3110, https://doi.org/10.5194/gmd-7-3089-2014, 2014.
Su, Y., Guo, Q., Ma, Q., and Li, W.: SRTM DEM Correction in Vegetated Mountain Areas through the Integration of Spaceborne LiDAR, Airborne LiDAR, and Optical Imagery, Remote Sens.-Basel, 7, 11202–11225, https://doi.org/10.3390/rs70911202, 2015.
Terentieva, I. E., Glagolev, M. V., Lapshina, E. D., Sabrekov, A. F., and Maksyutov, S.: Mapping of West Siberian taiga wetland complexes using Landsat imagery: implications for methane emissions, Biogeosciences, 13, 4615–4626, https://doi.org/10.5194/bg-13-4615-2016, 2016.
Thornton, B. F., Wik, M., and Crill, P. M.: Double-counting challenges the accuracy of high-latitude methane inventories: Double-counting arctic methane, Geophys. Res. Lett., 43, 12569–12577, https://doi.org/10.1002/2016GL071772, 2016.
Tiner, R., Lang, M., Klemas, V., and Johnston, C. A.: Remote Sensing of Wetlands: Applications and Advances, Natural Resource Management Faculty Books, 2, CRC Press, available at: https://openprairie.sdstate.edu/nrm_book/2 (last access: 6 May 2021), 2015.
Tootchi, A., Jost, A., and Ducharne, A.: Multi-source global wetland maps combining surface water imagery and groundwater constraints, Earth Syst. Sci. Data, 11, 189–220, https://doi.org/10.5194/essd-11-189-2019, 2019.
Treat, C. C., Bloom, A. A., and Marushchak, M. E.: Nongrowing season methane emissions – a significant component of annual emissions across northern ecosystems, Global Change Biol., 24, 3331–3343, https://doi.org/10.1111/gcb.14137, 2018.
Verpoorter, C., Kutser, T., Seekell, D. A., and Tranvik, L. J.: A global inventory of lakes based on high-resolution satellite imagery, Geophys. Res. Lett., 41, 6396–6402, https://doi.org/10.1002/2014GL060641, 2014.
Wania, R., Melton, J. R., Hodson, E. L., Poulter, B., Ringeval, B., Spahni, R., Bohn, T., Avis, C. A., Chen, G., Eliseev, A. V., Hopcroft, P. O., Riley, W. J., Subin, Z. M., Tian, H., van Bodegom, P. M., Kleinen, T., Yu, Z. C., Singarayer, J. S., Zürcher, S., Lettenmaier, D. P., Beerling, D. J., Denisov, S. N., Prigent, C., Papa, F., and Kaplan, J. O.: Present state of global wetland extent and wetland methane modelling: methodology of a model inter-comparison project (WETCHIMP), Geosci. Model Dev., 6, 617–641, https://doi.org/10.5194/gmd-6-617-2013, 2013.
West, J. J. and Plug, L. J.: Time-dependent morphology of thaw lakes and taliks in deep and shallow ground ice, J. Geophys. Res.-Earth, 113, F01009, https://doi.org/10.1029/2006JF000696, 2008.
Widhalm, B., Bartsch, A., and Heim, B.: Circumarctic wetland dataset based on ENVISAT ASAR Global Monitoring Mode with links to geotiff image and NetCDF files (2005-01 to 2011-12), in: Supplement to: Widhalm, B. et al. (2015): A novel approach for the characterization of tundra wetland regions with C-band SAR satellite data, Int. J. Remote Sens., 36, 5537–5556, https://doi.org/10.1080/01431161.2015.1101505, PANGAEA, https://doi.org/10.1594/PANGAEA.840548, 2015.
Wu, Y., Chan, E., Melton, J. R., and Verseghy, D. L.: A map of global peatland distribution created using machine learning for use in terrestrial ecosystem and earth system models, Geosci. Model Dev. Discuss. [preprint], https://doi.org/10.5194/gmd-2017-152, 2017.
Wulder, M. A., Li, Z., Campbell, E. M., White, J. C., Hobart, G., Hermosilla, T.m and Coops, N. C.: A National Assessment of Wetland Status and Trends for Canada's Forested Ecosystems Using 33 Years of Earth Observation Satellite Data, Remote Sens.-Basel, 10, 1623, https://doi.org/10.3390/rs10101623, 2018.
Xu, J., Morris, P. J., Liu, J., and Holden, J.: PEATMAP: Refining estimates of global peatland distribution based on a meta-analysis, Catena, 160, 134–140, https://doi.org/10.1016/j.catena.2017.09.010, 2018.
Yamazaki, D., Trigg, M. A., and Ikeshima, D.: Development of a global ∼ 90 m water body map using multi-temporal Landsat images, Remote Sens. Environ., 171, 337–351, https://doi.org/10.1016/j.rse.2015.10.014, 2015.
Yan, F., Liu, X., Chen, J., Yu, L., Yang, C., Chang, L., Yang, J., and Zhang, S.: China's wetland databases based on remote sensing technology, Chinese Geogr. Sci., 27, 374–388, https://doi.org/10.1007/s11769-017-0872-z, 2017.
Zhang, Z., Zimmermann, N. E., Kaplan, J. O., and Poulter, B.: Modeling spatiotemporal dynamics of global wetlands: comprehensive evaluation of a new sub-grid TOPMODEL parameterization and uncertainties, Biogeosciences, 13, 1387–1408, https://doi.org/10.5194/bg-13-1387-2016, 2016.
Zhang, Z., Zimmermann, N. E., Calle, L., Hurtt, G., Chatterjee, A., and Poulter, B.: Enhanced response of global wetland methane emissions to the 2015–2016 El Niño-Southern Oscillation event, Environ. Res. Lett., 13, 074009, https://doi.org/10.1088/1748-9326/aac939, 2018.
Zhang, Z., Fluet-Chouinard, E., Jensen, K., McDonald, K., Hugelius, G., Gumbricht, T., Carroll, M., Prigent, C., Bartsch, A., and Poulter, B.: Development of a global dataset of Wetland Area and Dynamics for Methane Modeling (WAD2M), Zenodo, https://doi.org/10.5281/zenodo.3998454, 2020.