the Creative Commons Attribution 4.0 License.
the Creative Commons Attribution 4.0 License.
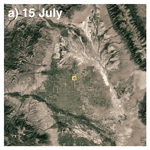
Measurements from mobile surface vehicles during the Lower Atmospheric Profiling Studies at Elevation – a Remotely-piloted Aircraft Team Experiment (LAPSE-RATE)
Sean Waugh
Alexander Erwin
Steven Borenstein
Cory Dixon
Wafa'a Shanti
Adam Houston
Brian Argrow
Between 14 and 20 July 2018, small unmanned aircraft systems (UASs) were deployed to the San Luis Valley of Colorado (USA) alongside surface-based remote sensors, in situ sensors, and radiosonde systems as part of the Lower Atmospheric Profiling Studies at Elevation – a Remotely-piloted Aircraft Team Experiment (LAPSE-RATE). The measurements collected as part of LAPSE-RATE targeted quantities related to enhancing our understanding of boundary layer structure, cloud and aerosol properties and surface–atmosphere exchange and provide detailed information to support model evaluation and improvement work. Additionally, intensive intercomparison between the different unmanned aircraft platforms was completed. The current paper describes the observations obtained using three different types of surface-based mobile observing vehicles. These included the University of Colorado Mobile UAS Research Collaboratory (MURC), the National Oceanic and Atmospheric Administration National Severe Storms Laboratory Mobile Mesonet, and two University of Nebraska Combined Mesonet and Tracker (CoMeT) vehicles. Over the 1-week campaign, a total of 143 h of data were collected using this combination of vehicles. The data from these coordinated activities provide detailed perspectives on the spatial variability of atmospheric state parameters (air temperature, humidity, pressure, and wind) throughout the northern half of the San Luis Valley. These datasets have been checked for quality and published to the Zenodo data archive under a specific “community” setup for LAPSE-RATE (https://zenodo.org/communities/lapse-rate/, last access: 21 January 2021) and are accessible at no cost by all registered users. The primary dataset DOIs are https://doi.org/10.5281/zenodo.3814765 (CU MURC measurements; de Boer et al., 2020d), https://doi.org/10.5281/zenodo.3738175 (NSSL MM measurements; Waugh, 2020), and https://doi.org/10.5281/zenodo.3838724 (UNL CoMeT measurements; Houston and Erwin, 2020).
- Article
(12471 KB) - Full-text XML
- BibTeX
- EndNote
In July 2018, a collection of atmospheric scientists and engineers from around the globe converged on the San Luis Valley (SLV) of Colorado (USA) to take part in the LAPSE-RATE (Lower Atmospheric Profiling Studies at Elevation – a Remotely-piloted Aircraft Team Experiment) field campaign (de Boer et al., 2020a, b). This campaign was focused on demonstrating the utility of unmanned aircraft systems (UASs) for atmospheric research and collecting scientifically interesting datasets to conduct targeted studies on specific topics of interest related to boundary layer processes. Connected to the annual meeting of the International Society for Atmospheric Research using Remotely-piloted Aircraft (ISARRA, de Boer et al., 2019), LAPSE-RATE included over 100 scientists and engineers, who together conducted nearly 1300 research flights and captured over 250 flight hours of data using UASs.
Information on the different UASs and profilers deployed during LAPSE-RATE, numerical simulations completed for the campaign, and an overview of the campaign itself is distributed in a range of articles, many of which are associated with this Earth System Science Data special issue and will not be revisited here (de Boer et al., 2020b, c; Bell et al., 2020; Pillar-Little et al., 2020; Bailey et al., 2020; Natalie et al., 2021; Islam et al., 2020; Brus et al., 2020; Pinto et al., 2020). The current paper is focused on describing datasets collected using mobile surface observing systems during the LAPSE-RATE campaign.
The general concept behind mobile surface-observing vehicles is to provide a full mobile platform from which accurate observations of atmospheric parameters can be made, thereby offering opportunities to position (and reposition) in situ surface meteorological instrumentation precisely to capture highly localized gradients and target locations that are thought to be critical for the development of phenomena of interest. To accomplish this, rack- or mast-mounted instrumentation is set up to measure quantities such as pressure, wind speed and direction, temperature, and relative humidity. In the case of vehicles set up for truly mobile measurements (i.e., measuring while driving) instruments are mounted far enough away from the vehicle structure to minimize direct influence to the observations from the vehicle itself. Such mobile mesonet systems have been deployed for atmospheric research for over two decades, and details on the original mobile mesonets can be found in Straka et al. (1996). These systems have generally been used to evaluate atmospheric conditions supporting the development of tornadic supercells (e.g., Markowski, 2002; Pietrycha and Rasmussen, 2004), though deployments to observe land-falling hurricanes have also been conducted (e.g., Caban et al., 2019). Additionally, mobile mesonet systems have been used in conjunction with airborne systems (manned or unmanned) to capture measurements along four-dimensional transects (e.g., Riganti and Houston, 2017).
During LAPSE-RATE, atmosphere-observing surface vehicles provided critical insight into gradients in state variables across the SLV, including information on temperature, pressure, humidity, and winds. This includes both single-site sampling and measurements covering extended transects conducted throughout the northern half of the SLV. The latter were particularly interesting given that the SLV features widely varying surface types (ranging from irrigated cropland to dry shrublands), significant topography, and terrain-induced flows. The following section describes the vehicles deployed and instrumentation that they carried, while Sect. 3 provides an overview of measurement locations and sampling strategies. Section 4 provides details on data processing and quality control (QC), while Sect. 5 offers information on dataset availability.
The three mobile surface-based platforms used to collect data during LAPSE-RATE and described in this paper include the Mobile UAS Research Collaboratory (MURC), operated by the University of Colorado Boulder (CU), a mobile mesonet (MM) vehicle operated by the NOAA National Severe Storms Laboratory (NSSL), and a pair of Combined Mesonet and Tracker (CoMeT) vehicles operated by the University of Nebraska Lincoln (UNL). As documented below, these three vehicles provided complementary measurements, including details on atmospheric temperature, pressure, humidity, and winds. Each operated in slightly different modes over the duration of the campaign, though all three vehicles were at the same location for limited times.
2.1 CU MURC
The CU MURC (Fig. 1) is an instrumented van that was added to the Integrated Remote and In Situ Sensing (IRISS) program vehicle fleet in early 2018. This system was specifically developed to work in tandem with unmanned aircraft operations, serving as a mobile command station and surface measurement facility during field deployments. This centralized operations center provides a platform from which to oversee field teams and provide general situational awareness. The MURC is equipped with two workstations for lighter computing loads, including on-site processing of data, real-time communications with team members and the broader community through web-based systems, and possibly serving as UAS ground stations. Additionally, the MURC carries two servers for more intensive computing tasks, with one dedicated to graphics-intensive processes (such as processing of imagery for photogrammetry-centric missions) and the other dedicated to general computing and intensive real-time data processing.
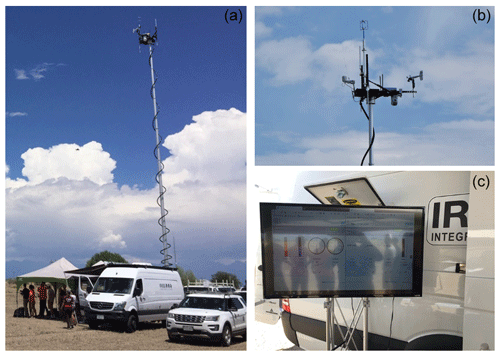
Figure 1The CU MURC vehicle, with mast extended (a), as deployed during LAPSE-RATE. The right hand panels show the instrument cluster mounted on the top of the MURC mast (b) and the MURC real-time data display (c).
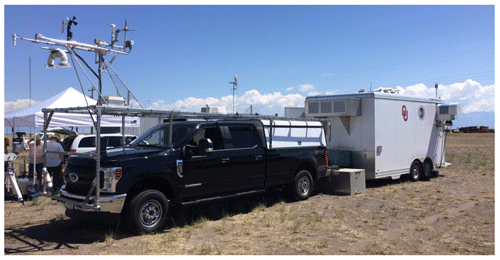
Figure 2The NSSL MM as deployed during the LAPSE-RATE project. The trailer is the University of Oklahoma CLAMPS system (see Bell et al., 2020, in this special issue).
From an observational perspective, the MURC is equipped with a 15 m extendable mast, atop which are mounted several meteorological sensors. This includes a Gill MetPak Pro Base Station that measures barometric pressure, air temperature, and relative humidity; a Gill WindMaster 3-D sonic anemometer for 3-D wind and fast temperature measurements; and an R.M. Young Wind Monitor (05103) propeller and vane anemometer which provides a redundant horizontal wind measurement and offers real-time situational awareness for nearby unmanned aircraft operators. An overview of the sensors and their projected accuracies is included in Table 1. The MURC is also equipped with a large communications suite that increases the range of UHF/VHF vehicle-to-vehicle radios used during field campaigns, increases cellular bandwidth for data transfer and communications, and improves the ground station to UAS communication link. While mobile, the MURC is set up to operate at a single location at any given time and does not collect measurements while traveling like other platforms described below. Data collected by the MURC were used to intercompare measurements from the different UASs deployed during LAPSE-RATE. The results of this intercomparison are documented in Barbieri et al. (2019).
2.2 NSSL mobile mesonet
In addition to the MURC, LAPSE-RATE included a deployment of the NOAA NSSL MM vehicle, a heavily modified version of the original MM created 25 years ago (Straka et al., 1996). The current generation of NSSL MM is built on a Ford F-250 extended-cab long-bed pickup truck. Instrumentation is located on a rack mounted forward and above the hood of the vehicle, in order to minimize atmospheric disturbances caused by the blunt forward edge of the truck (Fig. 2). Mounting the equipment rack over the roof of the vehicle (as was done with previous NSSL MM vehicles) was thought to result in observational biases due to the turbulent and accelerated airflow over the vehicle roof and thermal influence of the vehicle engine. The new setup requires the addition of a substantial structure to support the weight and drag of the instrument rack. This structure also allows for installation of a wire mesh hail cage to protect the windshield from hail strikes while operating in the vicinity of severe thunderstorms. In addition to the instrument rack, the MM can carry up to four helium tanks along with a Vaisala MW41 sounding system for mobile radiosonde launches.
For air temperature and relative humidity measurements, the NSSL MM deploys a Vaisala HMP155 sensor. While the HMP155 is highly accurate, the relative humidity sensor can be prone to contamination by atmospheric particles. To reduce this contamination the HMP155 requires integration of a membrane that allows water molecules to pass through while reducing the impact of contaminants. While this practice protects the RH observations from contamination, it also significantly delays the thermal response of the environment inside the membrane. In combination with a relatively slow time constant (testing on previous models such as the HMP35 and HMP45 have revealed time constants on the order of 10 min; Waugh, 2012), the impacts of this membrane result in very slow response to temperature measurements (hence the designation “slow temp” for the HMP155). To overcome this, a fast-responding Campbell Scientific T109SS temperature sensor is also installed on the NSSL MM (T109, a.k.a. “fast temp”). The HMP155 cannot be assumed to report a temperature and relative humidity that represents the true environmental conditions, only the conditions inside the membrane. However, while the HMP should not be used for temperature and relative humidity observations directly, dewpoint is conserved across the membrane allowing the HMP to be useful for observing the dewpoint (Richardson et al., 1998). This dewpoint observation is combined with data from the faster T109 temperature sensor to derive a relative humidity value that is representative of the true environmental value (Richardson et al., 1998). The thermodynamic observations are housed in a radiation shield to protect the sensors from direct solar radiation while maintaining adequate airflow from the real environment. This shield, known as the “U-tube”, was developed by NSSL to specifically accomplish this task (Waugh and Frederickson, 2010; Houston et al., 2016). For wind measurements, the NSSL MM deploys a standard propeller-vane combination anemometer from R.M. Young (Wind Monitor 05103) covering a wide range of wind speeds (0–100 m s−1). While the vehicle is stationary, ambient wind direction is derived using the vehicle-relative wind direction and vehicle heading from a KVH C100 magnetic compass. In combination, these allow for computation of the ambient wind vector. While in motion, the vehicle-relative wind vector is subtracted from GPS-obtained vehicle motion to produce the inertial environmental wind vector. The measurements from all of these sensors are logged at 1 Hz using a Campbell Scientific CR6 Wi-Fi-enabled data logger. A list of the sensors and their respective measurements, as well as general accuracies and response times are listed in Table 2.
2.3 UNL CoMeT
Finally, the UNL deployed two CoMeTs for LAPSE-RATE. These systems are built around Ford Explorers and feature a forward-mounted suite of meteorological sensors and a dual moonroof (see Fig. 3). The CoMeTs measure air temperature and relative humidity at ∼ 2 (above ground level) using a Vaisala HMP155A and also include a fast-response sensor (Campbell Scientific 109SS thermistor) for air temperature at ∼ 2 (same setup as the NOAA NSSL MM). Air pressure at ∼ 2.5 is measured using a Vaisala PTB210, while wind speed and direction are observed using an R.M. Young 05103 propeller-vane anemometer at approximately 3.5 The vehicle heading is tracked using a KVH Industries C-100 fluxgate compass. As on the NSSL MM, the HMP155A and 109SS thermistor are shielded and aspirated within a U-tube (Waugh and Frederickson, 2010; Houston et al., 2016). Manufacturer specifications for these instruments are provided in Hanft and Houston (2018) and are again listed in Table 2 of this paper. In addition to the measured variables, the CoMeT data loggers (Campbell Scientific CR6) along with a custom Python script use observed quantities to calculate dewpoint temperature (Td), mixing ratio (qv), potential temperature (θ), equivalent potential temperature (θe), virtual potential temperature (θv), and wind speed and direction. The equations used to compute these quantities are provided in Sect. 4.
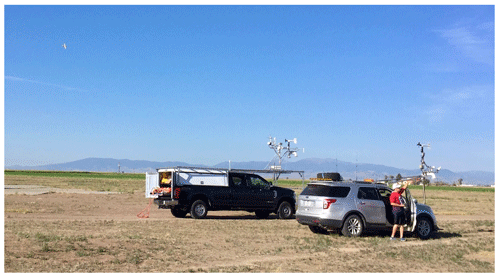
Figure 4The NSSL MM and UNL CoMeT deployed side-by-side for an on-site intercomparison. The CU MURC was also located on site, but out of the photograph, and a CU TTwistor UAS (see de Boer et al., 2020c, in this special issue) flies in the background.
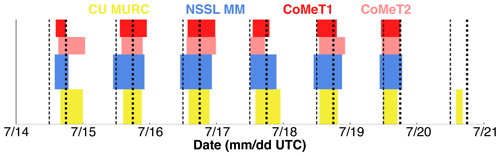
Figure 5Illustration of the uptime for the mobile surface vehicles deployed during LAPSE-RATE. Shown is the update for the CU MURC (yellow), the NSSL MM (blue), and the UNL CoMeTs (red and pink). The thin dashed black lines indicate 06:00 LT (Mountain Daylight Time), while the bold dashed black lines represent 12:00 LT for each day.
The vehicles described above covered a significant amount of ground over the course of the campaign. Each played a different role in addressing the primary objectives of the LAPSE-RATE campaign (see de Boer et al., 2020a, b). These vehicles were used to evaluate the performance of UAS sensors, in addition to intercomparison between different surface vehicles (see Fig. 4). Figure 5 provides an overview of the amount of time each vehicle spent making atmospheric measurements during the campaign. As shown, sampling primarily occurred in the morning and early afternoon (local time), with the NSSL MM generally starting the earliest in order to launch an early-morning radiosonde (see Bell et al., 2020). Sampling conducted on the afternoon of 14 July and the morning of 20 July by the CU MURC was in support of platform intercomparison efforts (Barbieri et al., 2019).
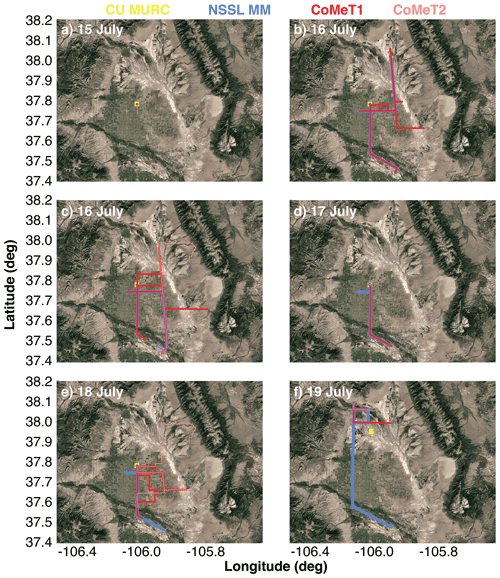
Figure 6Position data for the different platforms over the length of the campaign, broken down on a day-by-day basis. The CU MURC positions are indicated by the yellow square, while the NSSL mobile mesonet (blue) and UNL CoMeTs (red and pink) mobile datasets are shown by the lines. Background maps are from © Google through their API.
The primary role of the CU MURC was to provide daily measurements at a consistent location (Leach Airport) in the center of the sampling domain. In this role, the MURC acted as a meteorological tower that collected measurements in a similar manner from day to day, providing a baseline for putting other observations collected during the campaign into context. The only exception to this routine sampling took place on 19 July, when all platforms were focused on cold air drainage out of the smaller valleys on the northern end of the SLV. On 19 July, the MURC was positioned a bit farther to the north, as can be seen in Fig. 6f to help evaluate the timing and intensity of density currents flowing from Saguache (northwest corner of the SLV) and Villa Grove (northeast corner of the SLV). In total, the MURC operated for seven days, capturing a total of 45.5 h of data.
The NSSL MM filled multiple roles throughout the campaign. One important role included the launching of radiosondes from various locations around the SLV (Bell et al., 2020). This often included early-morning radiosondes from Leach Airport. In addition, the NSSL MM was leveraged as a mobile measurement platform to capture information on spatial variability throughout the broader valley. The first of these mobile measurement sorties took place on 15 July and included transects spanning the area between Alamosa, Colorado, and Moffatt, Colorado. These transects covered a variety of different surface types, ranging from irrigated cropland to dry desert-like areas on the eastern side of the SLV. On 16–18 July, the NSSL MM focused on the south-central portion of the SLV, with much of the measurement time spent at Leach Airport, and the transect between Leach Airport and the city of Alamosa. Finally, on 19 July, the NSSL MM covered an area from Alamosa to Saguache in the northwest corner of the SLV. Most of the time on that date was spent sampling the square shown in the northwest part of the SLV in Fig. 6f to help understand the spatial variability of the drainage flow exiting the Saguache Valley. In total, the NSSL MM collected a total of 55.4 h of data, in addition to the measurements from the radiosondes launched.
The two UNL CoMeT vehicles were deployed separately throughout the San Luis Valley during the majority of the LAPSE-RATE campaign. CoMeT-1 was principally focused on coordinated observations with the CU UAS team and involved both stationary data collection based at Leach Airport and transect data collection across the SLV. CoMeT-2 was principally focused on stationary data collection in coordination with the UNL UAS team based at a site on the eastern margins of the SLV in the northwest corner of the Great Sand Dunes National Park (henceforth referred to as observation site “Gamma”; Islam et al., 2019, 2020). As with the NSSL MM, the CoMeT data collection began on 15 July with CoMeT-1 operating transects based out of Leach Airport and CoMeT-2 collecting stationary observations at Gamma. Similar operations were executed on 16 and 18 July during which time CoMeT-1 performed extended east–west transects to the far eastern portion of the SLV to help understand the role of surface type gradients and sloping terrain on that side of the valley on convection initiation. On 18 July, following operations at Gamma, CoMeT-2 also executed a set of transects along the eastern margins of the irrigated region of the valley in an effort to explore whether surface flow parallel to this margin resulted in a coherent convergence boundary. On 17 July, both CoMeTs operated at Leach Airport. Finally, on 19 July, both CoMeTs joined the effort to capture the early-morning Saguache Valley cold-air drainage, with frequent transects along County Road X between Saguache and County Road 55. Over the course of the campaign, CoMeT-1 collected 50.4 h of data and CoMeT-2 collected 50.3 h of data.
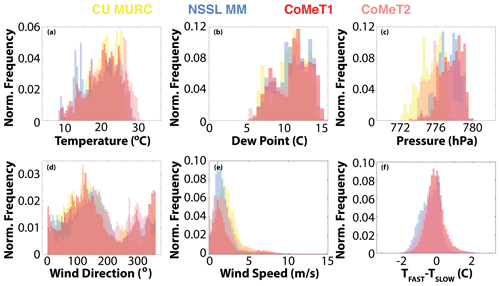
Figure 7Distributions of 1 min averages of data collected by the three platforms. Included are (a–f) distributions of air temperature, dew point temperature, pressure, wind direction, wind speed, and the difference between the fast and slow temperature sensors (where applicable). For all figures, CU MURC data are represented in yellow, NSSL MM data are represented in blue, and UNL CoMeT data are represented in red and pink.
Figure 7 provides a statistical overview of data collected by these three platforms over the duration of the LAPSE-RATE campaign. Included are normalized probability distributions of measured quantities, including temperature, relative humidity, air pressure, wind speed, wind direction, and (for the NSSL MM and UNL CoMeT datasets) the difference between the fast and slow temperature sensors. For all of these distributions, data were averaged to a moving 1 min equally weighted window. The distributions illustrate differences that are likely largely the result of instrument and platform location. For example, it is important to remember that while the NSSL MM and UNL CoMeT instruments were located close to (< 3 m) the ground, the CU MURC data were collected atop a 15.2 m mast. Therefore, it is not surprising that the CU MURC pressure measurements are found to be slightly lower than those measured by the other two platforms. Similarly, the CU MURC temperature and RH distributions lack the extremes, with measurements from the top of the mast likely missing the coldest temperatures in the early morning, and the warmest temperatures in the afternoon. Also attributable to this altitude difference is the slight but noticeable increase in wind speeds and counterclockwise shift in wind direction from the near-surface environment to the height of the mast. Finally, for both the NSSL MM and the UNL CoMeT data, the slow temperature sensor inside of the membrane (HMP155) was shown to have a warm bias relative to the fast sensor (T109 SS), and the UNL CoMeT difference distribution is shown to have an extended tail towards positive values. The mean difference (fast minus slow) of the NSSL MM temperature sensors was −0.328 ∘C, while the mean difference of the UNL CoMeT temperature sensors was −0.398 ∘C.
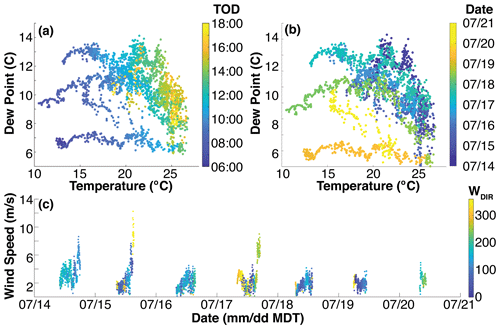
Figure 8Panels illustrating the temporal variability of the temperature (a, b), dew point temperature (a, b), and wind data (c) collected by the CU MURC. Panel (a) shows diurnal variability, while (b) shows the variability by date.
Figure 8 provides additional insight into the temporal variability of the recorded variables, both in terms of diurnal cycle and over the extent of the LAPSE-RATE campaign, based on measurements obtained by the CU MURC. Figure 8a shows that, as expected, temperatures were generally coldest in the early morning, with a gradual but notable warming over the course of the day. Along with this, relative humidity levels were generally highest in the morning and decreased significantly over the course of the day. Interestingly, the middle of the week did feature one day (17 July) where the MURC was sampling later into the afternoon, and temperatures were recorded dropping during that time period, decreasing from around 26 ∘C in the mid-afternoon to below 20 ∘C by the end of sampling around 17:00 MDT. Figure 8b provides insight into the variability occurring over the course of the field campaign. The earliest days (14–16 July) were consistently warm and relatively humid. The atmosphere became drier later in the campaign, with relative humidity values peaking at around 70 % on 19 July, despite temperatures that were slightly cooler than those recorded on 17 and 18 July, when relative humidity levels climbed above 90 % in the early-morning hours. In general, afternoons were illustrated to fall between 25–27 ∘C, and mornings between 10–15 ∘C (largely depending on the start time of sampling for a given date). Wind speed and direction measurements are shown in Fig. 8c and demonstrate that winds were generally quite light throughout the week, with values between 0–6 m s−1. An intensification of winds in the afternoon was seen on 14, 15, and 17 July, generally associated with convective systems developing over the valley and surrounding mountain peaks. Wind directions were generally from the south and east. With the stronger winds resulting in a more northerly and westerly component. The only sampling period with solidly westerly winds was the morning of 20 July.
4.1 CU MURC
Data available from the CU MURC have been processed in various ways to average the data, remove outliers, and correct the wind measurements from the sonic anemometer for platform pitch and roll. All data were averaged across a moving 1 s window. Any data points falling inside of the 1 s window were included in averaging, though no filter was implemented to ensure any particular number of samples within a given 1 s averaging window. Time periods where no data were collected are included as “NaN”. Screening for outliers was completed using the MATLAB “filloutliers” function, which detects and replaces outliers using a linear interpolation between points not deemed to be outliers. In the current application, outliers were defined as points falling more than three local standard deviations outside of a moving mean window encompassing 10 s worth of data. Note that this technique was applied to the measured zonal and meridional wind components only, and not to the wind speed and direction included in the dataset that are calculated using the components, given that such averages are not possible on the vector values.
Rotation of the CU MURC sonic anemometer data was completed using a standard three-axis rotation (Tropea et al., 2007), where the updated wind coordinates are calculated as follows:
Here, um, vm, and wm are the measured instantaneous velocity components as measured by the sonic anemometer; A is the rotation matrix; and uf, vf, and wf are the final velocity components. A can be approximated by combining multiple rotations to align the coordinate system using measured Euler angles. In this case, we assume the following:
where
and ψ, φ, and θ are the roll, pitch, and yaw rotation angles, respectively, as measured by the CU MURC operators. Note that these angles were only measured once after parking the vehicle and do not vary in time in between vehicle movements. Therefore, any swaying of the vehicle as a result of people getting in and out, wind, or for other reasons may impact the wind measurements from the sonic anemometer and may not be accounted for. Note that the rotations are applied in yaw, pitch, roll order, meaning that we step through the rotation as follows:
Note that these corrections are only applied to the wind measurements from the sonic anemometer. The influence of slight offsets in pitch and roll are negligible for the R.M. Young propeller-based wind instrument as the propeller follows a cosine response. For LAPSE-RATE, the sensor pitch and roll angles varied between −1.7 and 2.35∘ and −2.21 and 3.48∘, respectively. These angles correspond to a maximum error of 0.2 %, well below the uncertainty of the instrument. The sensor was aligned with magnetic north on a daily basis.
4.2 NSSL MM
For the NSSL MMs, a majority of the data processing and variable calculation is done in real time on the CR6 data logger. Most of the observations do not require much in the way of modification; the exceptions to that are derived ambient winds, vehicle heading, and the environmental RH. This is an advantage of the CR6 data logging system, as the onboard computing power is enough to handle the calculations in real time, making data display and recording easier.
For the derived winds, the measured wind speed and direction directly off the anemometer is a combination of the vehicle motion vector and the ambient wind vector (this combined vector is the vehicle relative vector), which need to be separated. To obtain the ambient wind vector, the vehicle motion must be subtracted from the vehicle relative vector in a process similar to that outlined in Sect. 4.1 for the CU MURC, though not as complex, as the wind monitor on the NSSL MM is only two-dimensional. The vehicle motion is obtained from the onboard GPS and broken into N–S and E–W components. The apparent wind vectors as measured by the anemometer directly are also broken into components but are first added to the vehicle heading to obtain a true directional vector rather than a vehicle relative vector. The apparent wind components are then subtracted from the vehicle motion components to obtain the ambient wind components. The final step of the process involves converting the ambient components back into vector form, which requires a tedious series of manual computations to determine the quadrant relative angle and its true meteorological heading. The vector wind speed is found simply with
where X and Y are the ambient wind components for the U and V directions, respectively. For the vector wind direction, the components must be examined to determine where on the meteorological coordinate system they lie and manually assembled in the correct direction. This is due to the fact that traditional use of sin, cos, and tangent (and their inverse functions) are referenced to a mathematical coordinate system which is reversed and offset by 90∘ from the meteorological coordinate system. To determine the wind direction, an offset to either 90 or 270∘ is found by taking ATAN(ABS(Y∕X)). This value is then added or subtracted from the appropriate reference angle depending on the quadrant. For example, if Y and X were both +15 m s−1, then ATAN(ABS(Y∕X)) = 45∘. Since both Y and X are positive values, the resulting angle should be in the first quadrant, or between 0 and 90∘; thus the ATAN value is subtracted from the 90∘ reference angle, obtaining an environmental wind direction of 45∘. A more detailed description of this process is forthcoming in a future paper. Note that if the vehicle is not moving, this component-based approach is not needed and the wind direction can be found by simply rotating the observed winds by the vehicle heading while stationary.
The vehicle heading is also corrected in real time for cases where the vehicle is not moving. In these situations, the heading of the vehicle is obtained via a magnetic compass which provides the magnetic bearing. This is used in cases where the vehicle motion is less than 1 m s−1. The magnetic heading differs from true north by an offset which is dependent on the coordinates of the observation location, called the magnetic declination angle. This angle is provided along with the GPS coordinates in real time and is used to correct the magnetic heading.
While the NSSL MM measures temperature and humidity, it does so with a set of sensors behind a protective membrane that significantly delays the response time as described in Sect. 2.2. With this filter in place, the measured RH is lagged behind the true environmental RH and must be re-derived. This process follows that of Richardson et al. (1998) where
where Tfast and TdC (the calculated dewpoint from the HMP155) are in degrees Celsius. The calculation for dewpoint is done with a built-in CR6 function for dewpoint, which uses the Tetens equation and the vapor pressure (Campbell Scientific, 2020).
As a final step to the process, after the data are collected and archived, each dataset is run through a QC procedure where the individual data files from a single operations period are combined, and a set of QC flags applied. The intent of these flags are not to remove data, but rather flag data that are potentially suspicious and should be examined manually. There are four QC flags, representing panel temperature excess, vehicle stationary periods, excessive changes to vehicle motion, and a general sanity check. More specific details of the QC flags are contained in the readme files that accompany the data; however, a brief description is presented here. The panel temperature flag identifies areas where the internal temperature of the CR6 data logger changes by a significant amount. This identifies periods where the logger may be having inconsistency issues or power supply problems, which manifest themselves in the internal temperature monitoring first. The second QC flag is meant to identify periods where the vehicle is stationary, which could increase the potential for bias in the observations, while the third flag looks for sharp changes to the speed or direction. The latter flag is meant to identify areas where there could be a discrepancy between the vehicle heading and the observed winds, such as in a sharp turn. The final QC flag simply examines all the observations for values that are well outside the normal operating range.
4.3 UNL CoMeTs
As mentioned in Sect. 2, the CoMeT data loggers and Python scripts are used to calculate key quantities of interest in real time. These quantities include corrected relative humidity, water vapor mixing ratio, dew point temperature, the potential temperature (θ), virtual potential temperature (θv), and equivalent potential temperature (θe).
For both CoMeT-1 and CoMeT-2, relative humidity is adjusted to the fast temperature following Richardson et al. (1998) and Houston et al. (2016): vapor pressure is calculated using slow temperature and relative humidity, saturation vapor pressure is calculated using fast temperature, and the ratio of the two is used to calculate the corrected (fast) relative humidity. In CoMeT-1, the calculations are done in the Python script using the following:
from Wexler (1976) and Bolton (1980), where e∗ is either vapor pressure or saturation vapor pressure and T∗ is dew point temperature (for vapor pressure) or fast temperature (for saturation vapor pressure). Dew point temperature is calculated using
where RH∗ is the uncorrected (slow) relative humidity and Tslow is the slow temperature. In contrast to CoMeT-1, the calculation of dew point temperature, vapor pressure, and saturation vapor pressure is done within the logger, and slightly different expressions are used. For dew point temperature
is used, where A1=0.61078, A2=17.558, and A3=241.88. The expression used in the logger for (saturation) vapor pressure is from Lowe (1977):
where B0=6.107799961, , , , , , and .
The water vapor mixing ratio is calculated using
and dew point temperature and vapor pressure as described above. Potential temperature for both CoMeTs is calculated using
Virtual potential temperature is calculated using
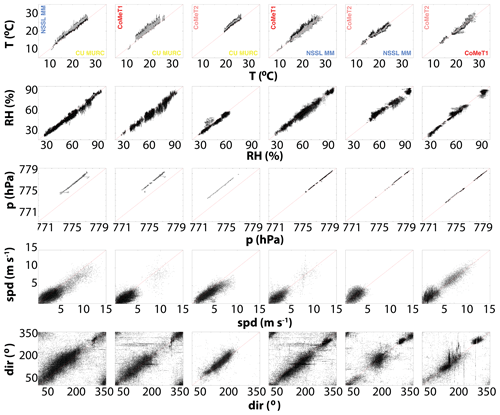
Figure 9Vehicle-to-vehicle comparisons for time periods when vehicles were within 300 m of one another. Variables evaluated include (top to bottom) temperature (∘C), relative humidity (%), pressure (hPa), wind speed (m s−1), and wind direction (∘). The top row includes labels to indicate which platform is on which axis, and these orientations are maintained through each column. For the temperature comparisons (top row), both the slow (dark dots) and fast (lighter dots) temperature sensors are evaluated.
Equivalent potential temperature is calculated in both CoMeTs following Bolton (1980):
Due to a hole in the pressure tube underneath the CoMeT-2 vehicle, it was found to have erroneously low air pressure measurements when the vehicle was in motion during LAPSE-RATE. To correct this error, observations from times when CoMeT-1 and CoMeT-2 were in motion and in close proximity were used to evaluate the level of inaccuracy of the CoMeT-2 measurement. Here “close proximity” was defined as any observations within 25 m of the same point, measured within 90 s of one another. The observations with the smallest distance between them were used, and duplicates were removed such that an observation from either vehicle was not used twice. The pressure difference and CoMeT-2 anemometer speed were then aligned with those from CoMeT-1 using a second-order polynomial. Anemometer speed was used instead of vehicle speed because vehicle speed was often a multiple of 5, which made it difficult to compute an accurate fit. The polynomial fit was used to calculate a pressure correction for all CoMeT-2 data obtained when the vehicle was in motion and the anemometer speed was greater than 10 m s−1. Other variables calculated using pressure (e.g., Td, qv, θ, θe, and θv) were recalculated using the corrected pressure.
Evaluation of data collected during the 14 July intercomparison along with an intercomparison conducted on 19 July revealed an approximately constant bias in slow temperature in the CoMeT-1 data. The magnitude of this bias was approximated through minimization of the root mean square error across the intercomparison datasets and analysis of the adjusted time series. The result was a −0.6 K correction applied to all CoMeT-1 slow temperature data.
Figure 9 illustrates comparisons between measurements from all of the mobile systems leveraged to produce this dataset. Using the GPS position, time periods where individual vehicles were within 300 m of one another were selected for this comparison. Each panel represents a comparison of the data points, interpolated to a common 1 s time grid, during these close encounters. Note that the times do not necessarily match from one inter-platform comparison to the next (i.e., the times change from column to column but are the same from one row to the next within a given column). General agreement is shown between the different platforms, with some notable (but predictable) differences. First, the CU MURC pressure is shown to be systematically lower than the pressure measured by the other vehicles, which as discussed above is consistent with the fact that the CU MURC pressure measurements were collected on from the top of the 15.2 m mast. As also discussed previously in relation to Fig. 7, this elevation difference additionally results in the MURC temperatures being slightly cooler than those observed at the surface, and the relative humidities being slightly higher. Again, wind speeds are also shown to be ever-so-slightly higher at the CU MURC mast height than at the surface. There is quite a bit of scatter in the wind direction comparison, particularly for those time periods with very light winds, though the majority of the points still fall close to the one-to-one line. A primary exception to this is for time periods when the wind was northerly, resulting in clusters of points in the upper-left- and lower-right-hand quadrants of the figures. For days with higher wind speeds, a tighter clustering around the one-to-one line is noticeable. Ultimately, this figure illustrates good consistency between the platforms, offering confidence to data users who plan to leverage a combination of these datasets.
The data files from the LAPSE-RATE project are generally being archived under a LAPSE-RATE community established at the Zenodo data archive (https://zenodo.org/communities/lapse-rate/, last access: 21 January 2021). From here, LAPSE-RATE observations are available for public download and use. Contributors were encouraged to provide files in NetCDF format, with self-describing metadata provided to the user inside the NetCDF file. To make it possible for scientists to cite LAPSE-RATE data in their publications, the organizers of the campaign recognized the value of digital object identifiers (DOIs). DOIs were automatically generated by the Zenodo archive at the data version and product level. Data from the different sources described above are posted as individual data streams on the archive, with each of the platforms described in the previous section having their own DOI. It is important to note that each platform may have several different levels of data available. Therefore, data products with different levels of processing and quality control may be provided with separate DOIs. This means the files and data described in this publication are spread across a variety of DOIs, and that additional DOIs could be created in the future that include LAPSE-RATE data, as additional data products are developed.
As of the writing of this paper, the CU MURC dataset (de Boer et al., 2020d) is available at the Zenodo website (https://zenodo.org/record/3814765#.XrSRdS-z1TY, last access: 21 January 2021) under the DOI https://doi.org/10.5281/zenodo.3814765. Data from the NSSL MM include two versions (Waugh, 2020). The original version contained files with incorrect QC flags. While the core data are correct, the QC flags can be useful for determining specific areas of interest or problems. After identifying this issue, the files were reprocessed to include the correct QC flags and were uploaded to the archive as version 2. Users should use version 2, which is available at Zenodo.org (https://zenodo.org/record/3738175#.XrNLkC-z1TY, last access: 21 January 2021) under DOI https://doi.org/10.5281/zenodo.37175. Finally, the UNL CoMeT datasets (Houston and Erwin, 2020) also include two versions, and users are encouraged to use version 2, which includes corrected GPS data for the vehicle locations. These data are also available at the Zenodo website (https://zenodo.org/record/3838724#.XvOMGi2z1TZ, last access: 21 January 2021) under the DOI https://doi.org/10.5281/zenodo.3838724.
This paper provides an overview of data collected by three types of mobile surface systems during the 2018 LAPSE-RATE campaign. These included the University of Colorado MURC, the NOAA National Severe Storms Laboratory Mobile Mesonet, and two University of Nebraska CoMeT vehicles. In combination, these vehicles collected over 140 h of meteorological data in the San Luis Valley of Colorado between 14–20 July 2018. Data from these vehicles are available for public download from the Zenodo website, and the previous sections describe processing conducted on this dataset before publication and provide information on the expected accuracy of the sensors deployed on these systems. The primary focus of the LAPSE-RATE campaign was to collect data from a fleet of unmanned aerial vehicles and surface in situ and remote-sensing systems, and to combine those data with high-resolution numerical simulations to gain understanding on boundary layer processes and phenomena. The primary measurement objectives of the vehicles discussed in the current paper are shared above, along with the locations of the operation of each throughout the campaign.
GB planned the LAPSE-RATE field campaign, constructed this paper, and conducted data processing of the CU MURC data. SB, CD, and BA contributed to the collection of the MURC data and were deployed to the field during LAPSE-RATE. AE, WS, and AH contributed to the collection of the UNL CoMeT data, processed and quality-controlled these data, and are the primary points of contact for this dataset. They additionally helped with the writing of this paper. SW was solely responsible for collection of the NSSL MM data and subsequent quality control of the resulting dataset. Additionally, he contributed to the writing of this paper.
Gijs de Boer is the lead editor for the special issue in which this paper appears. Additionally, Gijs de Boer works as a consultant for Black Swift Technologies, who participated in the LAPSE-RATE campaign.
This article is part of the special issue “Observational and model data from the 2018 Lower Atmospheric Process Studies at Elevation – a Remotely-piloted Aircraft Team Experiment (LAPSE-RATE) campaign”. It is a result of the International Society for Atmospheric Research using Remotely piloted Aircraft (ISARRA 2018) conference, Boulder, USA, 9–12 July 2018.
General support for salary and overhead associated with the collection of these datasets was provided by the NOAA Physical Sciences Division and the University of Colorado's Integrated Remote and In Situ Sensing (IRISS) grand challenge project. We would additionally like to recognize financial support for student participation and travel from the National Science Foundation (NSF AGS 1807199) and the US Department of Energy (DE-SC0018985). General support for the LAPSE-RATE campaign was provided by the International Society for Atmospheric Research using Remotely-piloted Aircraft (ISARRA). CoMeT-1 was funded through a grant from the Air Force Office of Scientific Research Defense University Research Instrumentation Program (FA2386-14-1-3010). CoMeT-2 was funded through an equipment allocation included in the NSF Research Infrastructure Improvement Program: Track-2 Focused EPSCoR Collaborations award (OIA-1539070). Funding for the NSSL MM and travel was provided for through internal NSSL funds, with sounding expendables donated by Oklahoma State University.
This research has been supported by the National Science Foundation, Division of Atmospheric and Geospace Sciences (grant no. AGS 1807199), and the US Department of Energy, Office of Science (grant no. DE-SC0018985).
This paper was edited by David Carlson and reviewed by Min Liu and one anonymous referee.
Bailey, S. C. C., Sama, M. P., Canter, C. A., Pampolini, L. F., Lippay, Z. S., Schuyler, T. J., Hamilton, J. D., MacPhee, S. B., Rowe, I. S., Sanders, C. D., Smith, V. G., Vezzi, C. N., Wight, H. M., Hoagg, J. B., Guzman, M. I., and Smith, S. W.: University of Kentucky measurements of wind, temperature, pressure and humidity in support of LAPSE-RATE using multisite fixed-wing and rotorcraft unmanned aerial systems, Earth Syst. Sci. Data, 12, 1759–1773, https://doi.org/10.5194/essd-12-1759-2020, 2020.
Barbieri, L., Kral, S. T., Bailey, S. C.C., Frazier, A. E., Jacob, J. D., Reuder, J., Brus, D., Chilson, P. B., Crick, C., Detweiler, C., Doddi, A., Elston, J., Foroutan, H., González-Rocha, J., Greene, B. R., Guzman, M. I., Houston, A. L., Islam, A., Kemppinen, O., Lawrence, D., Pillar-Little, E. A., Ross, S. D., Sama, M. P., Schmale, D. G., Schuyler, T. J., Shankar, A., Smith, S. W., Waugh, S., Dixon, C., Borenstein, S., and de Boer, G.: Intercomparison of Small Unmanned Aircraft System (sUAS) Measurements for Atmospheric Science during the LAPSE-RATE Campaign, Sensors, 19, 2179, https://doi.org/10.3390/s19092179, 2019.
Bell, T. M., Klein, P. M., Lundquist, J. K., and Waugh, S.: Remote sensing and radiosonde datasets collected in the San Luis Valley during the LAPSE-RATE campaign, Earth Syst. Sci. Data Discuss. [preprint], https://doi.org/10.5194/essd-2020-314, in review, 2020.
Bolton, D.: The computation of equivalent potential temperature, Mon. Weather Rev., 108, 1046–1053, 1980.
Brus, D., Gustafsson, J., Kempinen, O., de Boer, G., and Hirsikko, A.: Atmospheric aerosol, gases and meteorological parameters measured during the LAPSE-RATE campaign, Earth Syst. Sci. Data Discuss. [preprint], https://doi.org/10.5194/essd-2020-251, in review, 2020.
Campbell Scientific: CR Basic Help – CR Basic Editor, available at: https://help.campbellsci.com/crbasic/cr6/, last access: 16 May 2020.
de Boer, G., Argrow, B., Cassano, J., Cione, J., Frew, E., Lawrence, D., Wick, G., and Wolff, C.: Advancing unmanned aerial capabilities for atmospheric research, B. Am. Meteorol. Soc., 100, ES105–ES108, https://doi.org/10.1175/BAMS-D-18-0254.1, 2019.
de Boer, G., Diehl, C., Jacob, J., Houston, A., Smith, S. W., Chilson, P., Schmale III, D. G., Intrieri, J., Pinto, J., Elston, J., Brus, D., Kemppinen, O., Clark, A., Lawrence, D., Bailey, S. C.C., Sama, M. P., Frazier, A., Crick, C., Natalie, V., Pillar-Little, E. A., Klein, P., Waugh, S., Lundquist, J. K., Barbieri, L., Kral, S. T., Jensen, A. A., Dixon, C., Borenstein, S., Hesselius, D., Human, K., Hall, P., Argrow, B., Thornberry, T., Wright, R., and Kelly, J. T.: Development of community, capabilities and understanding through unmanned aircraft-based atmospheric research: The LAPSE-RATE campaign, B. Am. Meteorol. Soc., 101, E684–E699, https://doi.org/10.1175/BAMS-D-19-0050.1, 2020a.
de Boer, G., Houston, A., Jacob, J., Chilson, P. B., Smith, S. W., Argrow, B., Lawrence, D., Elston, J., Brus, D., Kemppinen, O., Klein, P., Lundquist, J. K., Waugh, S., Bailey, S. C. C., Frazier, A., Sama, M. P., Crick, C., Schmale III, D., Pinto, J., Pillar-Little, E. A., Natalie, V., and Jensen, A.: Data generated during the 2018 LAPSE-RATE campaign: an introduction and overview, Earth Syst. Sci. Data, 12, 3357–3366, https://doi.org/10.5194/essd-12-3357-2020, 2020b.
de Boer, G., Dixon, C., Borenstein, S., Lawrence, D. A., Elston, J., Hesselius, D., Stachura, M., Laurence III, R., Swenson, S., Choate, C. M., Doddi, A., Sesnic, A., Glasheen, K., Laouar, Z., Quinby, F., Frew, E., and Argrow, B. M.: University of Colorado and Black Swift Technologies RPAS-based measurements of the lower atmosphere during LAPSE-RATE, Earth Syst. Sci. Data Discuss. [preprint], https://doi.org/10.5194/essd-2020-333, in review, 2020c.
de Boer, G., Borenstein, S., Dixon, C., and Argrow, B.: University of Colorado MURC Observations from LAPSE-RATE [Data set], Zenodo, https://doi.org/10.5281/zenodo.3814765, 2020d.
Fernández-Cabán, P. L., Alford, A. A., Bell, M. J., Biggerstaff, M. I., Carrie, G. D., Hirth, B., Kosiba, K., Phillips, B. M., Schroeder, J. L., Waugh, S. M., Williford, E., Wurman, J., and Masters, F. J.: Observing Hurricane Harvey's Eyewall at Landfall, B. Am. Meteorol. Soc., 100, 759–775, https://doi.org/10.1175/BAMS-D-17-0237.1, 2019.
Hanft, W. and Houston, A. L.: An observational and modeling study of mesoscale air masses with high theta-e, Mon. Weather Rev., 146, 2503–2524, https://doi.org/10.1175/MWR-D-17-0389, 2018.
Houston, A. and Erwin, A.: University of Nebraska-Lincoln Mobile Mesonet files from LAPSE-RATE [Data set], Zenodo, https://doi.org/10.5281/zenodo.3709611, 2020.
Houston, A. L., Laurence III, R. J., Nichols, T. W., Waugh, S., Argrow, B., and Ziegler, C. L.: Intercomparison of unmanned aircraft-borne and mobile mesonet atmospheric sensors, J. Atmos. Ocean. Tech., 33, 1569–1582, https://doi.org/10.1175/JTECH-D-15-0178.1, 2016.
Islam, A., Houston, A. L., Shankar, A., and Detweiler, C.: Design and Evaluation of Sensor Housing for Boundary Layer Profiling Using Multirotors, Sensors, 19, 2481, https://doi.org/10.3390/s19112481, 2019.
Islam, A., Shankar, A., Houston, A., and Detweiler, C.: University of Nebraska UAS profiling during LAPSE-RATE, Earth Syst. Sci. Data Discuss. [preprint], https://doi.org/10.5194/essd-2020-374, in review, 2020.
Lowe, P. R.: An Approximating Polynomial for the Computation of Saturation Vapor Pressure, J. Appl. Meteorol. Clim., 16, 100–103, https://doi.org/10.1175/1520-0450(1977)016<0100:AAPFTC>2.0.CO;2, 1977.
Markowski, P. M.: Mobile Mesonet Observations on 3 May 1999, Weather Forecast., 17, 430–444, https://doi.org/10.1175/1520-0434(2002)017<0430:MMOOM>2.0.CO;2, 2002.
Natalie, V., Avery, A., Hemingway, B., Frazier, A., Crick, C., and Jacob, J.: Kinematic and thermodynamic atmospheric boundary layer observations in the San Luis Valley, CO via unmanned aircraft systems during the LAPSE-RATE field campaign, Earth Syst. Sci. Data Discuss., in preparation, 2021.
Pietrycha, A. E. and Rasmussen, E. N.: Finescale Surface Observations of the Dryline: A Mobile Mesonet Perspective, Weather Forecast., 19, 1075–1088, https://doi.org/10.1175/819.1, 2004.
Pillar-Little, E. A., Greene, B. R., Lappin, F. M., Bell, T. M., Segales, A. R., de Azevedo, G. B. H., Doyle, W., Kanneganti, S. T., Tripp, D. D., and Chilson, P. B.: Observations of the thermodynamic and kinematic state of the atmospheric boundary layer over the San Luis Valley, CO using remotely piloted aircraft systems during the LAPSE-RATE field campaign, Earth Syst. Sci. Data Discuss. [preprint], https://doi.org/10.5194/essd-2020-194, in review, 2020.
Pinto, J. O., Jensen, A. A., Jiménez, P. A., Hertneky, T., Muñoz-Esparza, D., Dumont, A., and Steiner, M.: Realtime WRF LES Simulations to Support UAS Flight Planning and Operations During 2018 LAPSE-RATE, Earth Syst. Sci. Data Discuss. [preprint], https://doi.org/10.5194/essd-2020-242, in review, 2020.
Richardson, S. J., Frederickson, S. E., Brock, F. V., and Brotzge, J. A.: Combination temperature and relative humidity probes: Avoiding large air temperature errors and associated relative humidity errors, Preprints, 10th Symp. On Meteorological Observations and Instrumentation, Phoenix, AZ, 11–16 January 1998, Amer. Meteor. Soc., 278–283, 1998.
Riganti, C. J. and Houston, A. L.: Rear-Flank Outflow Dynamics and Thermodynamics in the 10 June 2010 Last Chance, Colorado, Supercell, Mon. Weather Rev., 145, 2487–2504, https://doi.org/10.1175/MWR-D-16-0128.1, 2017.
Straka, J. M., Rasmussen, E. N., and Fredrickson, S. E.: A mobile mesonet for finescale meteorological observations, J. Atmos. Ocean. Tech., 13, 921–936, https://doi.org/10.1175/1520-0426(1996)013<0921:AMMFFM>2.0.CO;2, 1996.
Tropea, C., Yarin, A. L., and Foss, J. F. (Eds.): Springer Handbook of Experimental Fluid Mechanics, Berlin, Springer, https://doi.org/10.1007/978-3-540-30299-5, 2007.
Waugh, S.: The “U-tube”: An improved aspirate temperature system for mobile meteorological observations, especially in severe weather, MS thesis, Univ. of Oklahoma, 87 pp., 2012.
Waugh, S.: National Severe Storms Laboratory Mobile Mesonet data files from Lapse-Rate [Data set], Zenodo, https://doi.org/10.5281/zenodo.3738175, 2020.
Waugh, S. and Frederickson, S. E.: An improved aspirated temperature system for mobile meteorological observations, especially in severe weather, 25th Conf. on Severe Local Storms, Denver, CO, Amer. Meteor. Soc., P 5.2, available at: https://ams.confex.com/ams/25SLS/techprogram/paper_176205.htm (last access: 21 January 2021), 2010.
Wexler, A.: Vapor Pressure Formulation for Water in Range 0 to 100°C, A Revision, Journal of Research of the National Bureau of Standards, 80A, 775–785, 1976.
- Abstract
- Introduction
- Instrument and vehicle descriptions
- Description of measurement locations, deployment strategies, and sampling
- Data processing and quality control
- Data availability and file structure
- Summary
- Author contributions
- Competing interests
- Special issue statement
- Acknowledgements
- Financial support
- Review statement
- References
- Abstract
- Introduction
- Instrument and vehicle descriptions
- Description of measurement locations, deployment strategies, and sampling
- Data processing and quality control
- Data availability and file structure
- Summary
- Author contributions
- Competing interests
- Special issue statement
- Acknowledgements
- Financial support
- Review statement
- References