the Creative Commons Attribution 4.0 License.
the Creative Commons Attribution 4.0 License.
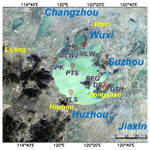
A dataset of microclimate and radiation and energy fluxes from the Lake Taihu eddy flux network
Zhen Zhang
Mi Zhang
Chang Cao
Wei Wang
Wei Xiao
Chengyu Xie
Haoran Chu
Jiao Wang
Jiayu Zhao
Lei Jia
Qiang Liu
Wenjing Huang
Wenqing Zhang
Yang Lu
Yanhong Xie
Yi Wang
Yini Pu
Yongbo Hu
Zheng Chen
Zhihao Qin
Xuhui Lee
Eddy covariance data are widely used for the investigation of surface–air interactions. Although numerous datasets exist in public depositories for land ecosystems, few research groups have released eddy covariance data collected over lakes. In this paper, we describe a dataset from the Lake Taihu eddy flux network, a network consisting of seven lake sites and one land site. Lake Taihu is the third-largest freshwater lake (area of 2400 km2) in China, under the influence of subtropical climate. The dataset spans the period from June 2010 to December 2018. Data variables are saved as half-hourly averages and include micrometeorology (air temperature, humidity, wind speed, wind direction, rainfall, and water or soil temperature profile), the four components of surface radiation balance, friction velocity, and sensible and latent heat fluxes. Except for rainfall and wind direction, all other variables are gap-filled, with each data point marked by a quality flag. Several areas of research can potentially benefit from the publication of this dataset, including evaluation of mesoscale weather forecast models, development of lake–air flux parameterizations, investigation of climatic controls on lake evaporation, validation of remote-sensing surface data products and global synthesis on lake–air interactions. The dataset is publicly available at https://yncenter.sites.yale.edu/data-access (last access: 24 October 2020) and from the Harvard Dataverse (https://doi.org/10.7910/DVN/HEWCWM; Zhang et al., 2020).
- Article
(6246 KB) - Full-text XML
- BibTeX
- EndNote
Inland lakes and reservoirs are a vital freshwater resource for the society. Globally, there are more than 27 million water bodies with size greater than 0.01 km2, occupying a total of 3.5 % of the earth's land surface area (Downing et al., 2006; Verpoorter et al., 2014). Accurate observation of the lake microclimate and lake–air interactions will help to better manage this water resource and to better predict how it may be affected by environmental changes. Towards that end, an increasing number of studies have employed the eddy covariance (EC) methodology to monitor physical state (temperature, wind, humidity) and process variables (momentum flux and radiation and energy fluxes) in the lake environment (Vesala et al., 2006; Blanken et al., 2011; Nordbo et al., 2011; Wang et al., 2014; Li et al., 2015; Yusup and Liu, 2016; Du et al., 2018; Hamdani et al., 2018; Xiao et al., 2018; Wang et al., 2019). Unlike EC studies in land ecosystems, however, data from these lake studies are rarely published as data papers or are rarely archived in public data depositories accessible by the broader scientific community. For example, of the nearly 500 sites that have contributed EC and micrometeorological data to AmeriFlux, a public data depository (https://ameriflux.lbl.gov/data/data-availability/, last access: 24 October 2020), none are a lake site. Although a few scientific groups have provided data supplements to their scientific papers on lake–air fluxes (e.g., Charusombat et al., 2018; Franz et al., 2018; Zhao and Liu, 2018), we are not aware of a data paper devoted to systematic description and archival of EC lake observations.
In this paper, we describe the dataset from the Lake Taihu eddy flux network (Lee et al., 2014). Established in 2010, the network currently consists of six active lake sites, one inactive lake site and one active land site. Lake Taihu is the third-largest freshwater lake (area of 2400 km2) in China. Data variables are recorded at half-hourly intervals, and the measurement has continued for over 8 years. Several areas of research can potentially benefit from the publication of this dataset, including evaluation of mesoscale weather forecast models, development of lake–air flux parameterizations, investigation of climatic controls on lake evaporation, validation of remote-sensing surface data products and global synthesis on lake–air interactions.
This paper is organized as follows. Section 2 is a brief overview of the sites and the instruments used by the network. This is followed, in Sect. 3, with a description of data quality measures employed during the field monitoring. Section 4 provides the essential information about the dataset, including data variables, gap-filling methods and data quality flags. Results of postfield evaluation of the data quality are given in Sect. 5.
Users of this dataset may be interested in the relevant papers published by our group. Lee et al. (2014) gave an overview of the Lake Taihu eddy flux network. Using the data collected at a subset of the sites and during the early phase of the network. Wang et al. (2014) investigated the spatial variability of energy and momentum fluxes across the lake. Xiao et al. (2013) improved the bulk parameterizations of heat, water and momentum fluxes for shallow lakes. Deng et al. (2013) and Hu et al. (2017) modified the Community Land Model (CLM) lake simulator (Subin et al., 2012) to improve its prediction of the lake evaporation. Wang et al. (2017) and X. Zhang et al. (2019) evaluated the performance of two mesoscale models of the lake–land breeze. More recently, Xiao et al. (2020) investigated drivers of the interannual variability of the lake evaporation observed at one of the lake sites (Bifenggang). The value of the dataset is enhanced by these peer-reviewed publications because they have helped us to continuously improve our measurement and data-processing protocols. For example, we have used the locally calibrated bulk parameterizations of Xiao et al. (2013) to gap-fill the flux variables.
2.1 Sites and data periods
Table 1 shows the basic site information, and Fig. 1 is a map that gives the relative position of Lake Taihu in China and locations of the EC measurement sites. Also shown in Fig. 1 are World Meteorological Organization (WMO) baseline weather stations around the lake, whose data can be obtained from the National Meteorological Information Center in China (http://data.cma.cn/site/index.html, last access: 24 October 2020). The lake, located between the latitudinal range of 30∘5′40′′ to 31∘32′58′′ N and longitudinal range of 119∘52′32′′ to 120∘36′10′′ E, has a total area of 2400 km2 and an average depth of 1.9 m. The climate is subtropical monsoon, with an annual mean temperature of 16.2 ∘C and annual total precipitation of 1122 mm. The lake is ice-free throughout the year.
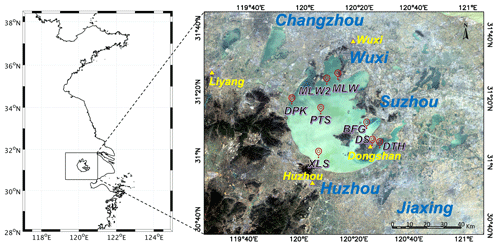
Figure 1Map showing locations of Lake Taihu, eddy covariance sites (red bubbles) and WMO weather stations (yellow triangles). City names are shown in blue. DS is a land site, and MLW, MLW2, DPK, PTS, XLS, BFG and DTH are lake sites. The background is a natural color image from Landsat 8 without correction for atmospheric interference.
The EC network consists of seven lake sites and one land site. The lake sites (Meiliangwan, MLW; Dapukou, DPK; Bifenggang, BFG; Xiaoleishan, XLS; Pingtaishan, PTS; Dongtaihu, DTH; Meiliangwan2, MLW2) are distributed according to biological characteristics and across eutrophication gradients of the lake. The MLW site, located in Meiliangwan Bay near the northern shore of Lake Taihu, was the first site in operation; the measurement began in June 2010 and was replaced by MLW2 in 2018, 10 km southwest of MLW. Both MLW and MLW2 sites are located in the lake eutrophic zone. BFG is located in the eastern part of Lake Taihu in relatively clean water inhabited by submerged vegetation with a growth season from April to November. DTH is located in the shallow water (mean depth of 1.3 m) in the southeastern part of the lake. After more than 20 years of crab aquaculture, this zone was returned to an unmanaged state in December 2018 in order to improve water quality. The observation at DTH enables the examination of lake–air exchange processes in the transition from human management to a natural state. PTS is situated in the middle of Lake Taihu, where occasional algal blooms occur, and no aquatic vegetation is present. DPK is located near the western shore, in a relatively deep (depth 2.5 m) super eutrophic zone due to heavy influence of agricultural and urban runoffs. XLS is located in the relatively clean and vegetation-free zone in the southeast. Finally, DS is a land site surrounded by rice agriculture, serving as a land reference for the lake sites. The MLW site is situated at a distance of 200 m from the northern shore of the lake. All the other lake sites in the lake are at a distance of more than 1 km away from the land.
Table 2Percent of data coverage. The percentage represents the proportion of high-quality original measurement.
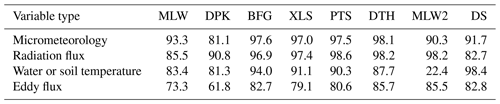
The lake water level is monitored daily by the Taihu Basin Authority at five locations around the lake (http://www.tba.gov.cn/, last access: 24 October 2020). Using the water level time series, we have constructed the water depth for our eddy covariance sites (Fig. 2).
2.2 Instrumentation
Each site is equipped with an EC system for long-term, continuous monitoring of the surface momentum, sensible heat, latent heat and carbon dioxide fluxes. The EC system consists of a sonic anemometer and thermometer (Model CSAT3A; Campbell Scientific, Logan, UT, USA) and a CO2∕H2O infrared gas analyzer (Model 7500A, LI-COR, Inc., Lincoln, NE, USA at DS, MLW, MLW2 and DPK; Model EC150, Campbell Scientific, at other sites). The EC system is at a height of 3.5 to 9.4 m above the water surface at the lake sites and at a height of 20 m above the ground at the land site. Other measurements include air humidity and air temperature (Model HMP45D/HMP155A; Vaisala, Inc, Helsinki, Finland) as well as wind speed and wind direction (Model 03002; R. M. Young Company, Traverse City, MI, USA) and four components of the net radiation (Model CNR4; Kipp & Zonen B.V., Delft, the Netherlands). At the lake sites, water temperature profile was measured with temperature probes (Model 109-L; Campbell Scientific) at water depths of 20, 50, 100 and 150 cm and in the sediment at about 5 cm below the bottom of the water column. The top four temperature sensors were tied to a nylon rope hanging from a buoy to ensure that they were at the designed depths regardless of water level fluctuations. At the DS land site, soil temperature profile was measured with the same type of probes at depths of 5, 10 and 20 cm. The MLW and the DS sites are supported by AC power, and other sites are powered by battery packs connected to solar panels. Measurements at the lake sites were made on fixed platforms. Readers are referred to Lee et al. (2014) and Xiao et al. (2017) for photographs of the platform and the instruments.
All the variables are reported as 30 min averages. The EC data are expressed in the natural coordinate system (Lee et al., 2004). In this coordinate system, the longitudinal coordinate axis is aligned with the 30 min mean velocity vector so that the 30 min mean lateral and vertical velocity components are 0, the magnitude of the mean velocity is equal to the mean longitudinal component, and the covariance between the lateral and the vertical velocity components is 0. Additionally, a small density correction has been applied to the water vapor flux according to Webb et al. (1980).
Every site in the Lake Taihu eddy flux network is equipped with a wireless transmission module for real-time monitoring and for data transmission. Time series of all 30 min variables are examined weekly, and abnormal behaviors are flagged for site operators. Each site is visited every 1 to 2 months to perform instrument repair and maintenance and to download 10 Hz EC data. The data coverage rates are summarized in Table 2, where the percentage values represent the proportions of data with quality flag 0, which indicates high-quality original measurement (Table 3).
The four-way net radiometers at MLW and XLS were compared in the field against a laboratory standard of the same type in the summer of 2018 to check their long-term stability (Fig. 3). These two sites were chosen because they have been in operation for more than 5 years. Additionally, the radiometer at MLW was relocated to MLW2 after MLW had been discontinued. The laboratory standard, which had been calibrated at the manufacturer prior to this performance evaluation, was mounted next to the field instrument for about 10 d at each site, covering overcast to clear-sky conditions. The mean bias error was smaller than 1 W m−2 for all the radiation components. It was −0.81, −0.81, 0.79 and −0.44 W m−2 for the downward shortwave, upward shortwave, downward longwave and upward longwave radiation flux at MLW, respectively. The corresponding values were 0.91, 0.40, 0.69 and 0.77 W m−2 for XLS. (Comparison experiments are being planned for the other sites.)
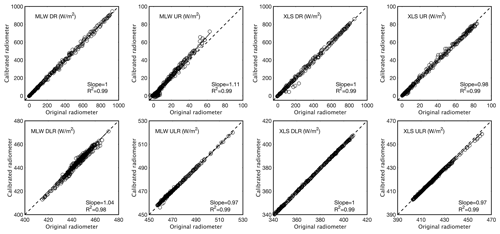
Figure 3Comparison of four components of the radiation balance between the original radiometer (horizontal axis) and a laboratory standard (vertical axis) at MLW and XLS. Refer to Table 4 for variable definitions.
The EC gas analyzers were calibrated every 1 to 2 years. The zero-point calibration was carried out with high-purity nitrogen gas, the CO2 span calibration was made with standard carbon dioxide gases (in the concentration range of 389 to 525 ppm) provided by the National Institute of Metrology of China (NIM) and certified to an accuracy of 1 %, and the H2O span calibration was made with a portable dew point generator (LI-610; LI-COR, Inc.).
We use a five-point moving average to screen outliers. If the deviation from the moving average is greater than 2 standard deviations, the data point is discarded. If a gap length is 30 min to 1 h, the gap is filled by linear interpolation. Larger gaps in meteorological variables, radiation components and water temperature are filled with linear regression involving observation of the same variable at another site. This spatial interpolation consists of three steps. First, linear correlation is calculated using the valid data at the target site and at all other sites for the month during which the data gap occurred. Second, the observation at the site with the highest linear correlation is used to establish a linear-regression equation. Third, the gap at the target site is filled with the linear regression and the observation at that site.
Radiation data gaps at the DS land site require special treatment. The radiometer at the DS eddy flux site ended in January 2013. Subsequent measurements of the radiation component are provided by a radiometer belonging to the Dongshan WMO weather station at a distance of 50 m from the eddy covariance tower (Fig. 1). While large gaps in meteorological variables (air temperature, relative humidity, wind speed and air pressure), downward solar radiation and downward longwave radiation are filled with the spatial interpolation method, large gaps in upward shortwave radiation and upward longwave radiation cannot be filled with data from other lake sites even with linear regression. In the case of the upward shortwave radiation, the data gaps were filled using the relationship between downward shortwave radiation and the monthly mean albedo. In the case of upward longwave radiation, the data gaps were filled by a regression equation between the upward longwave radiation and the fourth power of soil temperature at 5 cm depth. Compared to the original data, the gap-filled data do not capture the full diurnal variations because the 5 cm soil temperature has smaller diurnal amplitudes than the soil surface temperature, but the daily mean upward longwave radiation flux seems reasonable.
Large data gaps in the EC variables (sensible heat flux, latent heat flux and friction velocity) are filled with a hybrid method. First, if observations exist for the relevant state variable, the gap is filled with the bulk transfer relationship using a transfer coefficient tuned locally for each site (Xiao et al., 2013). For example, the relationship for filling gaps in the sensible heat flux is
where ρa is air density, cp is specific heat of air at constant pressure, CH is the transfer coefficient for sensible heat, Ta is air temperature, and Ts is water surface temperature. The transfer coefficient CH is determined from the observed H and the state variables (U, Ta and Ts) outside gap periods. The missing data on H are then filled with the above relationship using the tuned CH and the observed U, Ta and Ts. Second, if data for the state variables are missing, the spatial interpolation method is used to fill the gaps in these EC variables.
Table 4A list of data columns and variable definitions.
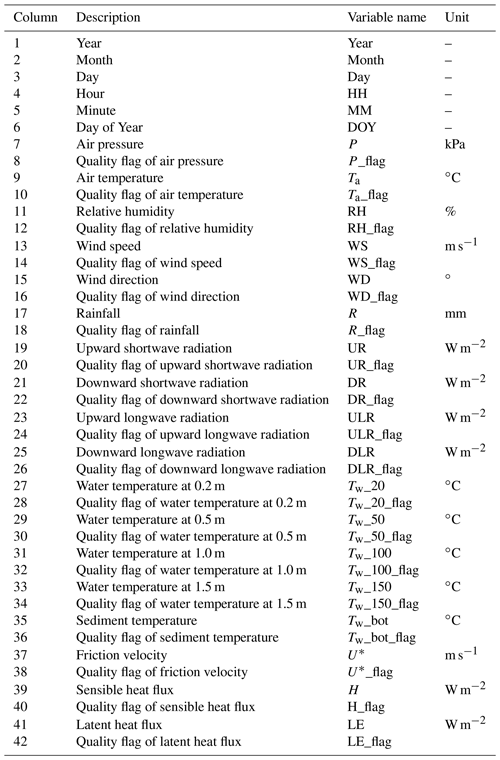
Notes: (1) time marks end of a half-hourly observation in Beijing time (UTC+8); (2) at the DS site, columns 27, 29 and 31 represent soil temperature at 5, 10 and 20 cm, respectively; column 33 represents soil heat flux G (W m−2) measured at 5 cm depth; and column 34 represents quality flag of soil heat flux.
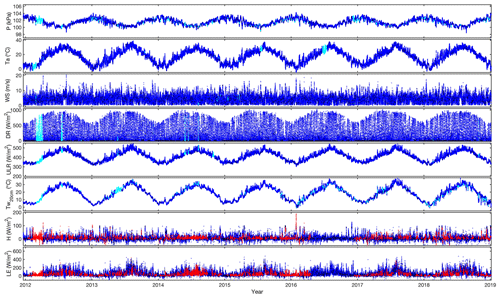
Figure 4Complete gap-filled time series for selected variables observed at BFG. Blue, black, cyan and red dots represent quality flag 0, 1, 2 and 3, respectively. Variable definitions are given in Table 4.
The spatial interpolation method described above occasionally causes a sudden jump at the beginning or end of a data gap. To harmonize the data, we apply a five-point moving average to the gap-filled time series. If a data point deviates by 2 times the standard deviation from the moving average, it is replaced by linear interpolation using the two adjacent data points.
Each data variable is assigned a quality flag to distinguish original measurements and gap-filled values and gap-filling methods (Table 3). The data flags employed here should not be confused with quality flags commonly assigned to the EC methodology in the literature. Specifically, Flag 0 indicates high-quality original data. Other flag values indicate gap-filled data or missing values. Flag 1 indicates that the data were filled by temporal interpolation. Flag 2 indicates that the data were filled by the spatial interpolation method. Flag 3 for the EC variables indicates that the data were filled by the bulk relationship. We also use Flag 3 to mark the upward shortwave and longwave radiation data filled with the albedo and the surface temperature relationship, respectively, for the DS land site. Missing values occur in some situations, which are marked with Flag 4. Figure 4 is an example showing the gap-filled time series of several variables at BFG along with the flag status.
Rainfall data have not been quantity-controlled or gap-filled. Because of the episodic nature of rainstorms and high spatial variability of rainfall, it is not appropriate to fill data gaps with the time interpolation or spatial-interpolation method. The total rain amount is likely biased low because no wind screens are used to protect the rain gages from the influence of wind which is much higher on the lake than on land (Fig. 5 below). On several site visits, the drain opening to the tipping bucket was found to be partially blocked by debris. Rain amount at a constant and low rate and excessively long rain duration are evidence of such blockage. The flag status of 0 for the rainfall variable simply indicates that the field measurement is available, but it does not guarantee high data quality.
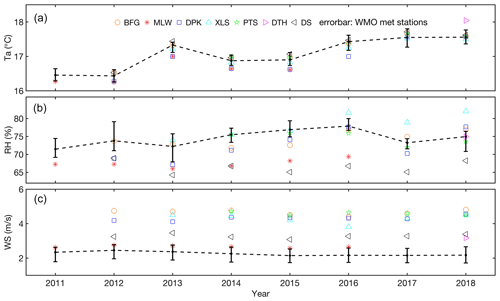
Figure 5Annual mean air temperature (a), relative humidity (b) and wind speed (c) observed at the eddy flux sites (symbols) and at the four WMO weather stations around the lake (line). Error bars represent the range of the annual means of the four WMO stations.
The data coverage begins from the start time of each site (Table 1) and ends in December 2018. The time resolution is 30 min. The dataset includes microclimate variables (air pressure, air temperature, relative humidity, wind speed, wind direction and rainfall); radiation fluxes (upward and downward shortwave radiation, upward and downward longwave radiation); water temperature at depths of 0.2, 0.5, 1.0 and 1.5 m and in the 5 cm sediment; and eddy fluxes (friction velocity, sensible heat and latent heat fluxes; Table 4). The time stamp is Beijing time (UTC+8), given by data columns 1 to 5 as year, month, day, hour and minute, and marks the end of the observation period. For example, time stamp “2012, 1, 1, 12, 00” indicates that the data acquisition period is from 11:30 to 12:00 UTC+8 on 1 January 2012.
Although the data table does not include the radiative surface temperature Ts, the user can easily calculate it from the two longwave radiation fluxes as
where σ is the Stefan–Boltzmann constant; ε is emissivity; and L↑and L↓ are upward and downward longwave radiation flux, respectively. We use a value of 0.97 for lake surface emissivity in this calculation (Deng et al., 2013; Wang et al., 2014).
Figure 5 compares the annual mean air temperature, relative humidity and wind speed at the Taihu eddy flux sites with those at the four WMO weather stations (Wuxi, Liyang, Huzhou and Dongshan) around the lake (Fig. 1). The error bars represent the maximum and minimum values among the four WMO stations, and the lines represent the mean values of the four station measurements. The annual mean air temperature at DTH is 0.3 ∘C higher than the station mean. At other sites, air temperature is in close agreement with the weather station data in terms of both magnitude and interannual variability. The annual mean wind speed at MLW, a site near the shoreline, is comparable with the station data. At other more exposed sites, the wind speed is much higher than observed at the WMO stations. The annual mean relative humidity (RH) shows a larger spread among the eddy flux sites than among the WMO stations partly because the measurement height at the eddy flux sites is not standardized (Table 1). The upward trends in RH over time at DPK and XLS seem to be related more to aging of the sensor than to a real interannual variability. We have not fully investigated this aging problem, but it is possible to rectify it by doing a detailed regression analysis against the station data.
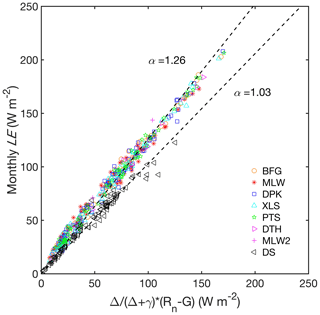
Figure 6Comparison of observed monthly latent heat flux with Priestley–Taylor model prediction using the original α coefficient of 1.26 and a modified coefficient of 1.03. Here Rn is net radiation, G is heat storage in the water column, Δ is the slope of the saturation vapor pressure curve, and γ is the psychrometric constant.
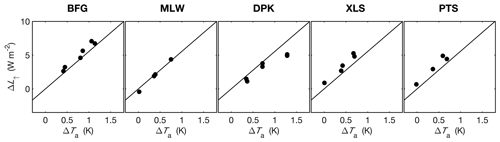
Figure 7The relationship between changes in observed annual mean upward longwave radiation flux and annual mean air temperature (dots). Solid lines represent the prediction of the Stefan–Boltzmann law.
Consistency of the energy flux variables can be evaluated with the energy balance closure. Using observations made at a subset of the sites in the earlier years of the flux network, Wang et al. (2014) reported a closure rate of 70 % to 110 % on a monthly basis, meaning that the sum of the measured monthly sensible and the latent heat flux H+λE is 70 % to 110 % of the monthly available energy Rn−G, where Rn is net radiation, and G is heat storage in the water column. By selecting days without data gaps, we found that the daily energy balance closure is in the range between 66 % and 78 % for all the lake sites and all the years. Such closure rates are typical of eddy covariance observations (Tanny et al., 2008; Wilson et al., 2002).
We have shown that the monthly latent heat flux at the lake sites MLW, BFG and DPK during July 2010 to August 2012 follows the Priestley–Taylor (PT) model prediction with the original PT constant α of 1.26 and that at the DS land site it is in agreement with the PT model if the constant is lowered to 1.0 (Lee et al., 2014). Figure 6 demonstrates that the same relationships hold for all the sites and all the observational months, indicating the overall stability of our measurement systems and the robustness of our gap-filling procedure. The reader is reminded that the monthly latent heat flux in Fig. 6 has been adjusted to force energy closure following the method recommended by Barr et al. (1994), Blanken et al. (1997) and Twine et al. (2000). (The half-hourly flux data in the data archive have not been adjusted for energy balance.)
Table 5Uncertainty of key measurement variables at half-hourly intervals. Instrument uncertainty is provided by the manufacturers. Performance uncertainty is 1 standard deviation of the difference between measurements made by the field instrument and the validation instrument. Environmental uncertainty is the spatial standard deviation of the variable measured at the lake sites.
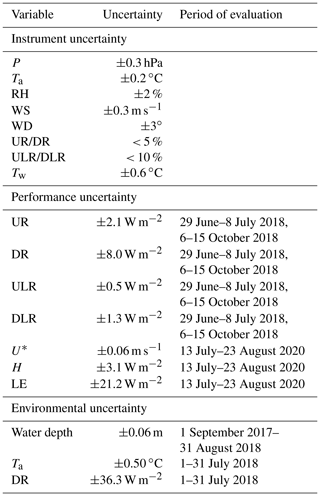
The Stefan–Boltzmann law offers another way for checking data consistency. Because the lake surface emits longwave radiation like a blackbody and because the annual mean air temperature and the surface water temperature are nearly identical at this lake (Wang et al., 2014), the change in the annual upward longwave radiation ΔL↑ can be expressed as
where Ta is annual mean air temperature, and Δ is the difference between the target year and the year with the lowest air temperature observed at the site. All the five long-term lake sites show good consistency between the longwave radiation and the air temperature observations (Fig. 7).
Table 5 is a summary of the uncertainty of key measurement variables at half-hourly intervals. The performance uncertainty is 1 standard deviation of difference in a variable measured by the field instrument and the same variable measured by a validation instrument (the closed-path EC in the case of eddy fluxes and the laboratory standard radiometer in the case of the radiation fluxes). The environmental uncertainty is 1 standard deviation of spatial variation in a variable measured at multiple lake sites.
All data are open-access and are available online for download and use at https://yncenter.sites.yale.edu/data-access (last access: 24 October 2020) and from the Harvard Dataverse (https://doi.org/10.7910/DVN/HEWCWM; Zhang et al., 2020).
The dataset described here consists of microclimate variables (air temperature, air humidity, wind speed, wind direction, water or soil temperature profile, and rainfall), four components of the radiation balance, friction velocity, and sensible and latent heat fluxes observed at seven lake sites and one land site. The period of coverage is from June 2010 to December 2018. The observation interval is 30 min. Except for rainfall and wind direction, all other variables have been gap-filled. Every data point is tagged with a data quality flag to help the user determine how to best use the data.
XL, WX and MZ directed the field program; ZZ performed data gap-filling and prepared the data for public release; CC, WW, CX, HC, JW, JZ, LJ, QL, WH, WZ, YL, YX, YW, YP, YH, ZC and ZQ participated in field data collection; and ZZ, XL and MZ wrote the manuscript.
The authors declare that they have no conflict of interest.
This research has been supported by the National Key R&D Program of China (grant no. 2019YFA0607202), the National Natural Science Foundation of China (grant nos. 41575147, 41801093, 41475141), and the Priority Academic Program Development (PAPD) of Jiangsu Higher Education Institutions.
This paper was edited by David Carlson and reviewed by two anonymous referees.
Barr, A. G., King, K. M., Gillespie, T. J., Den Hartog, G., and Neumann, H. H.: A comparison of Bowen ratio and eddy correlation sensible and latent heat flux measurements above deciduous forest, Bound.-Lay. Meteorol., 71, 21–41, 1994.
Blanken, P. D., Black, T. A., Yang, P. C., Neumann, H. H., Nesic, Z., Staebler, R., Den Hartog, G., Novak, M. D., and Lee, X.: Energy balance and canopy conductance of a boreal aspen forest: partitioning overstory and understory components, J. Geophys. Res.-Atmos., 102, 28915–28927, 1997.
Blanken, P. D., Spence, C., Hedstrom, N., and Lenters, J. D.: Evaporation from Lake Superior: 1. Physical controls and processes, J. Great Lakes Res., 37, 707–716, https://doi.org/10.1016/j.jglr.2011.08.009, 2011.
Charusombat, U., Fujisaki-Manome, A., Gronewold, A. D., Lofgren, B. M., Anderson, E. J., Blanken, P. D., Spence, C., Lenters, J. D., Xiao, C., Fitzpatrick, L. E., and Cutrell, G.: Evaluating and improving modeled turbulent heat fluxes across the North American Great Lakes, Hydrol. Earth Syst. Sci., 22, 5559–5578, https://doi.org/10.5194/hess-22-5559-2018, 2018.
Deng, B., Liu, S., Xiao, W., Wang, W., Jin, J., and Lee, X.: Evaluation of the CLM4 lake model at a large and shallow freshwater lake, J. Hydrometeorol., 14, 636–649, https://doi.org/10.1175/JHM-D-12-067.1, 2013.
Downing, J. A., Prairie, Y. T., Cole, J. J., Duarte, C. M., Tranvik, L. J., Striegl, R. G., McDowell, W. H., Kortelainen, P., Caraco, N. F., Melack, J. M., and Middelburg, J. J.: The global abundance and size distribution of lakes, ponds, and impoundments, Limnol. Oceanogr., 51, 2388–2397, https://doi.org/10.4319/lo.2006.51.5.2388, 2006.
Du, Q., Liu, H., Xu, L., Liu, Y., and Wang, L.: The monsoon effect on energy and carbon exchange processes over a highland lake in the southwest of China, Atmos. Chem. Phys., 18, 15087–15104, https://doi.org/10.5194/acp-18-15087-2018, 2018.
Franz, D., Mammarella, I., Boike, J., Kirillin, G., Vesala, T., Bornemann, N., Larmanou, E., Langer, M., and Sachs, T.: Lake-atmosphere heat flux dynamics of a thermokarst lake in arctic Siberia, J. Geophys. Res.-Atmos., 123, 5222–5239, 2018.
Franz, D., Mammarella, I., Boike, J., Kirillin, G., Vesala, T., Bornemann, N., Larmanou, E., Langer, M., and Sachs, T.: Lake-atmosphere heat flux dynamics of a thermokarst lake in arctic Siberia, J. Geophys. Res.-Atmos., 123, 5222–5239, 2018.
Hamdani, I., Assouline, S., Tanny, J., Lensky, I. M., Gertman, I., Mor, Z., and Lensky, N. G.: Seasonal and diurnal evaporation from a deep hypersaline lake: the Dead Sea as a case study, J. Hydrol., 562, 155–167, 2018.
Hu, C., Wang, Y., Wang, W., Liu, S., Piao, M., Xiao, W., and Lee, X.: Trends in evaporation of a large subtropical lake, Theor. Appl. Climatol., 129, 159–170, https://doi.org/10.1007/s00704-016-1768-z, 2017.
Lee, X., Massman, W., and Law, B.: Handbook of micrometeorology: a guide for surface flux measurement and analysis, Springer Science & Business Media, 2004.
Lee, X., Liu, S., Xiao, W., Wang, W., Gao, Z., Cao, C., Hu, C., Hu, Z., Shen, S., Wang, Y., Wen, X., Xiao, Q., Xu, J., Yang, J., and Zhang, M.: The taihu eddy flux network: An observational program on energy, water, and greenhouse gas fluxes of a large freshwater lake, B. Am. Meteorol. Soc., 95, 1583–1594, https://doi.org/10.1175/BAMS-D-13-00136.1, 2014.
Li, Z., Lyu, S., Ao, Y., Wen, L., Zhao, L., and Wang, S.: Long-term energy flux and radiation balance observations over Lake Ngoring, Tibetan Plateau, Atmos. Res., 155, 13–25, 2015.
Nordbo, A., Launiainen, S., Mammarella, I., Leppäranta, M., Huotari, J., Ojala, A., and Vesala, T.: Long-term energy flux measurements and energy balance over a small boreal lake using eddy covariance technique, J. Geophys. Res.-Atmos., 116, D02119, https://doi.org/10.1029/2010JD014542, 2011.
Subin, Z. M., Riley, W. J., and Mironov, D.: An improved lake model for climate simulations: Model structure, evaluation, and sensitivity analyses in CESM1, J. Adv. Model. Earth Sy., 4, 1–27, https://doi.org/10.1029/2011MS000072, 2012.
Tanny, J., Cohen, S., Assouline, S., Lange, F., Grava, A., Berger, D., Teltch, B., and Parlange, M. B.: Evaporation from a small water reservoir: Direct measurements and estimates, J. Hydrol., 351, 218–229, 2008.
Twine, T. E., Kustas, W. P., Norman, J. M., Cook, D. R., Houser, Pr., Meyers, T. P., Prueger, J. H., Starks, P. J., and Wesely, M. L.: Correcting eddy-covariance flux underestimates over a grassland, Agr. For. Meteorol., 103, 279–300, 2000.
Verpoorter, C., Kutser, T., Seekell, D. A., and Tranvik, L. J.: A global inventory of lakes based on high-resolution satellite imagery, Geophys. Res. Lett., 41, 6396–6402, https://doi.org/10.1002/2014GL060641, 2014.
Vesala, T., Huotari, J., Rannik, Ü., Suni, T., Smolander, S., Sogachev, A., Launiainen, S., and Ojala, A.: Eddy covariance measurements of carbon exchange and latent and sensible heat fluxes over a boreal lake for a full open-water period, J. Geophys. Res.-Atmos., 111, 1–12, https://doi.org/10.1029/2005JD006365, 2006.
Wang, B., Ma, Y., Wang, Y., Su, Z., and Ma, W.: Significant differences exist in lake-atmosphere interactions and the evaporation rates of high-elevation small and large lakes, J. Hydrol., 573, 220–234, 2019.
Wang, W., Xiao, W., Cao, C., Gao, Z., Hu, Z., Liu, S., Shen, S., Wang, L., Xiao, Q., Xu, J., Yang, D., and Lee, X.: Temporal and spatial variations in radiation and energy balance across a large freshwater lake in China, J. Hydrol., 511, 811–824, https://doi.org/10.1016/j.jhydrol.2014.02.012, 2014.
Wang, Y., Gao, Y., Qin, H., Huang, J., Liu, C., Hu, C., Wang, W., Liu, S., and Lee, X.: Spatiotemporal Characteristics of Lake Breezes over Lake Taihu, China, J. Appl. Meteorol. Climatol., 56, 2053–2065, 2017.
Webb, E. K., Pearman, G. I., and Leuning, R.: Correction of flux measurements for density effects due to heat and water vapour transfer, Q. J. R. Meteorol. Soc., 106, 85–100, 1980.
Wilson, K., Goldstein, A., Falge, E., Aubinet, M., Baldocchi, D., Berbigier, P., Bernhofer, C., Ceulemans, R., Dolman, H., and Field, C.: Energy balance closure at FLUXNET sites, Agr. For. Meteorol., 113, 223–243, 2002.
Xiao, K., Griffis, T. J., Baker, J. M., Bolstad, P. V, Erickson, M. D., Lee, X., Wood, J. D., Hu, C., and Nieber, J. L.: Evaporation from a temperate closed-basin lake and its impact on present, past, and future water level, J. Hydrol., 561, 59–75, 2018.
Xiao, W., Liu, S., Wang, W., Yang, D., Xu, J., Cao, C., Li, H., and Lee, X.: Transfer Coefficients of Momentum, Heat and Water Vapour in the Atmospheric Surface Layer of a Large Freshwater Lake, Bound.-Lay. Meteorol., 148, 479–494, https://doi.org/10.1007/s10546-013-9827-9, 2013.
Xiao, W., Zhang, Z., Wang, W., Zhang, M., Liu, Q., Hu, Y., Huang, W., Liu, S., and Lee, X.: Radiation Controls the Interannual Variability of Evaporation of a Subtropical Lake, J. Geophys. Res.-Atmos., 125, p.e2019JD031264, https://doi.org/10.1029/2019JD031264, 2020.
Yusup, Y. and Liu, H.: Effects of Atmospheric Surface Layer Stability on Turbulent Fluxes of Heat and Water Vapor across the Water–Atmosphere Interface, J. Hydrometeorol., 17, 2835–2851, 2016.
Zhang, X., Huang, J., Li, G., Wang, Y., Liu, C., Zhao, K., Tao, X., Hu, X.-M., and Lee, X.: Improving Lake-Breeze Simulation with WRF Nested LES and Lake Model over a Large Shallow Lake, J. Appl. Meteorol. Climatol., 58, 1689–1708, 2019.
Zhang, Z., Zhang, M., Cao, C., Wang, W., Xiao, W., Xie, C., Chu, H., Wang, J., Zhao, jiayu, Jia, L., Liu, Q., Huang, W., Zhang, W., Lu, Y., Xie, Y., Wang, Y., Pu, Y., Hu, Y., Chen, Z., Qin, Z., and Lee, X.: A dataset of microclimate and radiation and energy fluxes from the Lake Taihu Eddy Flux Network, Harvard Dataverse, https://doi.org/10.7910/DVN/HEWCWM, 2020.
Zhao, X. and Liu, Y.: Variability of surface heat fluxes and its driving forces at different time scales over a large ephemeral lake in China, J. Geophys. Res.-Atmos., 123, 4939–4957, 2018.