the Creative Commons Attribution 4.0 License.
the Creative Commons Attribution 4.0 License.
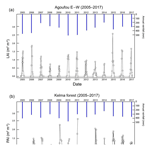
A leaf area index data set acquired in Sahelian rangelands of Gourma in Mali over the 2005–2017 period
Eric Mougin
Mamadou Oumar Diawara
Nogmana Soumaguel
Ali Amadou Maïga
Valérie Demarez
Pierre Hiernaux
Manuela Grippa
Véronique Chaffard
Abdramane Ba
The leaf area index of Sahelian rangelands and related variables such as the vegetation cover fraction, the fraction of absorbed photosynthetically active radiation and the clumping index were measured between 2005 and 2017 in the Gourma region of northern Mali. These variables, known as climate essential variables, were derived from the acquisition and the processing of hemispherical photographs taken along 1 km linear sampling transects for five contrasted canopies and one millet field. The same sampling protocol was applied in a seasonally inundated Acacia open forest, along a 0.5 km transect, by taking photographs of the understorey and the tree canopy. These observations collected over more than a decade, in a remote and not very accessible region, provide a relevant and unique data set that can be used for a better understanding of the Sahelian vegetation response to the current rainfall changes. The collected data can also be used for satellite product evaluation and land surface model development and validation. This paper aims to present the field work that was carried out during 13 successive rainy seasons, the measured vegetation variables, and the associated open database. Finally, a few examples of data use are shown. DOI of the referenced data set: https://doi.org/10.17178/AMMA-CATCH.CE.Veg_Gh.
- Article
(5509 KB) -
Supplement
(1217 KB) - BibTeX
- EndNote
The Global Climate Observing System, GCOS, identified the leaf area index, LAI, as one of the main terrestrial essential climate variables, ECVs, to be monitored from systematic long-term satellite and in situ measurements (GCOS, 2011; Bojinski et al., 2014). The LAI corresponds to one-half of the total green leaf area per unit horizontal ground surface area (Watson, 1947; Chen and Black, 1992). Similarly, the plant area index, PAI, corresponds here to the above-ground areal extent of green vegetation. LAI or PAI is related both to the fraction of absorbed photosynthetic active radiation (0.4–0.7 µm) by the green vegetation, fAPAR (Myneni and Williams, 1994; Fensholt et al., 2006), and to the canopy green cover fraction, fCover, i.e. the amount of green vegetation distributed in a horizontal plane (Carlson and Ripley, 1997). These ECVs drive the fundamental physiological processes at leaf and canopy levels such as photosynthesis and transpiration, as well as the energy and mass exchanges between the surface and the atmosphere (Sellers, 1985). The seasonal variation of the ECVs, i.e. changes in the vegetation phenology, plays an important role in the modulation of energy, water and gas exchanges (Jarlan et al., 2008; Boulain et al., 2009), surface properties such as surface albedo (Samain et al., 2008; Guichard et al., 2009), and evaporation–transpiration partitioning (Wang et al., 2014). As such, these ECVs are key variables required in most land surface and biogeochemical models (Running and Gower, 1991; Potter et al., 1993; Wang et al., 2007) or production efficiency models (Mougin et al., 1995; Running et al., 2004; Tracol et al., 2006; McCallum et al., 2009).
The Sahel is a vast ecoclimatic and biogeographic region extending south of the Sahara over 6000 km long and 400–600 km wide, from the Atlantic Ocean to the Red Sea (Le Houérou, 1989). The climate is arid to semi-arid tropical with a 1- to 4-month single rainy season centred in July–August, and controlled by the West African monsoon (WAM) (Nicholson et al., 1998; Lebel et al., 2003). Annual rainfall ranges between approximately 100 mm in the northern edge of the Sahel to approximately 600 mm at the southern edge with the humid Sudanian savannahs. The vegetation comprises a herbaceous layer almost exclusively composed of annual plants, among which grasses dominate, and scattered stands of shrubs and low trees (Hiernaux et al., 2009a). During the short rainy season, the grass layer develops rapidly following the first rains which occur between late May and mid-June, and the herbaceous production is achieved within a few weeks, peaking between late August and mid-September (Breman and Cissé, 1977; Penning de Vries and Djitèye, 1982). Afterwards, at the end of the rainy season in September, the herbaceous canopy suddenly dries out and, consequently, the amount of green vegetation decreases rapidly with plant wilting (Hiernaux and Le Houérou, 2006). Herbage production, and therefore LAI, fCover and fAPAR values are characterized by high spatial and inter-annual variations that are related to the variability of the WAM. After a humid period in the 1950s, the Sahel experienced 26 consecutive years of below average rainfall (Nicholson et al., 1998; Lebel et al., 2009) that was punctuated by major droughts in 1972–1973 and 1983–1984 with dramatic consequences on the natural resources and the population (Stige et al., 2006).
The Sahel is not a very accessible region and in situ measurements of LAI, fAPAR and fCover are scarce and were mainly performed in millet fields (Hanan and Bégué, 1995; Levy and Jarvis, 1999; Soegaard et al., 1999; Boulain et al., 2009), with a few data acquired in fallows (Boulain et al., 2009) and rangelands (Mougin et al., 2014). Since 1998, moderate spatial-resolution (typically 0.5 km to 1 km resolution) sensors such as the Moderate Resolution Imaging Resolution Spectroradiometer (MODIS), the SPOT-VEGETATION, and the PROBA-V (François et al., 2014) instruments have been used to provide continuous estimations of the ECV variables at a global scale (Myneni et al., 2002; Baret et al., 2013; Verger et al., 2015). However, the proposed products still need to be evaluated using reliable field estimates (Privette et al., 2002; Sea et al., 2011; Yan et al., 2016a, b; Xu et al., 2018a, b). In particular, several studies have pointed out the need to sample ecosystems like the semi-arid rangelands characterized by small, locally heterogeneous, and highly variable seasonally LAI values at the scale of moderate satellite resolution (e.g. Baret et al., 2006; Morisette et al., 2006). In 2000, the Sahelian Gourma sites have been integrated into the site network used in the Validation of Land European Remote Sensing Instruments (VALERI) project (Baret et al., 2005; http://www.avignon.ina.fr/valeri/, last access: 13 May 2019; Camacho et al., 2013) and the Committee on Earth Observation Satellites (CEOS) Land Product Validation (Morisette et al., 2006; http://lpvs.gsfc.nasa.gov/, last access: 13 May 2019) projects. Among the selected validation sites, the Gourma site stands out as the one with the lowest spatial heterogeneity at the moderate resolution scale (Garrigues et al., 2006). This is a major advantage for validation studies because of the ground geolocation uncertainties of satellite products (Garrigues et al., 2008). In 2010, the Gourma site was also selected as the Sahelian pilot site of West Africa for the international “Tropical Biomes in Transition” project (TROBIT) (http://www.geog.leeds.ac.uk/TROBIT/, last access: 13 May 2019).
The main objective of the current field monitoring is to document the seasonal, inter-annual and decadal variations of LAI and thus primary productivity in relation to the rainfall variability, at different spatio-temporal scales. More precisely, this monitoring aims to provide relevant data to investigate how the WAM and its spatio-temporal variability affect the vegetation cover in central Sahel. This data set can also be used in the interpretation of Sahelian surface processes that are mainly modulated by the vegetation cover such as the partition between latent and sensible energy fluxes (Timouk et al., 2009), and between dust and biogenic chemical emissions from soils (Pierre et al., 2012; Delon et al., 2015).
In the following, we describe the experimental protocol used for the estimation of vegetation variables, the associated open database, and show a few examples of data use.
The vegetation sites are located in north-east Mali, in the Gourma region which stretches from the loop of the Niger River southward down to the border with Burkina Faso. Since 2011, due to persistent security problems in the region, the monitored sites have been restricted to the vicinity of the town of Hombori (15.3∘ N, 1.5∘ W) within the 50 km × 50 km so-called super-site (Fig. 1) of the African Multidisciplinary Monsoon Analysis (AMMA) – Cycle Atmosphérique et Cycle Hydrologique (CATCH) observing system (Lebel et al., 2009, 2010; Mougin et al., 2009a; Gaillardet et al., 2018; Galle et al., 2018). At the national meteorological station of Hombori, the mean annual rainfall was 373 mm over the 1926–2017 period, and the mean annual temperature was 30.2 ∘C (1950–2010).
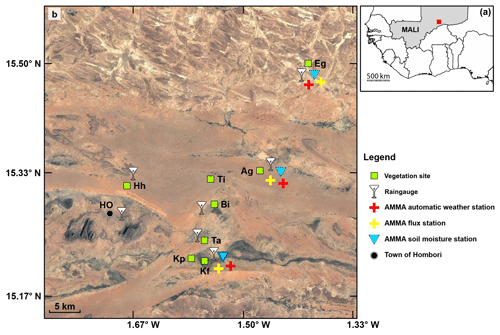
Figure 1The validation sites used in this study: (a) location of the 50 km × 50 km AMMA super-site in Mali, West Africa, and (b) map (on a Google Earth image, 31 December 2016) of the super-site showing the location of the eight vegetation canopies: Agoufou (no. 17, Ag), Timbadior (no. 18, Ti), Hombori Hondo (no. 19, Hh), Tara (no. 31, Ta), Kelma forest (no. 21, Kf) and Kelma plain (no. 21b, Kp), Eguerit (no. 40, Eg), Bilantao (no. 41, Bi), the national meteorological station at Hombori (HO) and the automatic meteorological, flux and soil moisture stations installed during the AMMA project during the 2005–2010 period. The vegetation sites refer to Hiernaux et al. (2009a).
The LAI measures were carried out at the sites previously installed in 1984 and monitored till 1994 by the International Livestock Centre for Africa (ILCA) and by the Institut d'Economie Rurale (IER, Bamako) (Hiernaux et al., 1984; Hiernaux et al., 2009a, b), and reactivated by the AMMA–CATCH observing system during the AMMA project (Redelsperger et al., 2006). These 1 km × 1 km sites were chosen within large and relatively homogeneous areas to sample the main vegetation types and canopies encountered within the super-site: herbaceous canopies on sandy soils, seasonally inundated Acacia open forest and its understorey herb layer in a plain of dominantly clay loam soils, and a seasonally inundated herbaceous canopy in a clayed loamy plain. In addition, a few data were acquired at an erosion surface site and in a crop millet field, for comparison purposes. Eventually, a total of eight sites on various soils, were retained, corresponding to nine vegetation canopies (Tables S1–S2 and Figs. S1–S2 in the Supplement). The selected vegetation canopies are as follows:
-
four herbaceous canopies on sandy soils: Agoufou (no. 17, Ag), Timbadior (no. 18, Ti), Hombori Hondo (no. 19, Hh) and Tara (no. 31, Ta),
-
one herbaceous canopy in a clay loam plain: Kelma plain (no. 21b, Kp),
-
one Acacia seyal open forest in a clay-loam plain: Kelma forest (no. 21, Kf),
-
one understorey herbaceous canopy in the Acacia seyal forest, Kelma herbs (no. 21, Kh),
-
one herbaceous canopy on an erosion surface: Eguerit (no. 40, Eg),
-
one millet field on sandy soil: Bilantao (no. 41, Bi).
Before the set-up of a seasonal and inter-annual survey, two preliminary field campaigns took place in 1999 and 2000, followed since 2005 by a systematic monitoring of eight 1 km × 1 km sites, during the wet season from June to September. The temporal variation of in situ LAI, λo, fCover and fAPAR were estimated through the analysis of digital hemispherical photographs (Weiss et al., 2004; Mougin et al., 2014). These photographs were collected during the growing season of herbaceous canopies or over the whole year for the forest canopy (Fig. 2a, c) and regularly taken along one or two perpendicular 1 km sampling transects crossing the site, perpendicular to the main orientation of the vegetation pattern that is perpendicular to the dune and interdune succession, usually in the north–south or the east–west directions (Table S3). Along the 1 km transects, this allows spatial heterogeneity to be taken into account. The choice of the sampling lines is described in Hiernaux et al. (2009a).
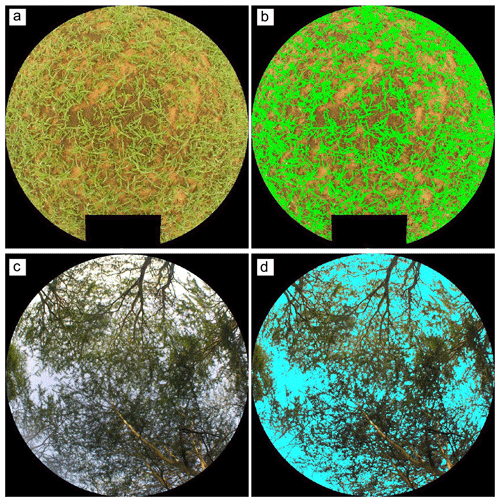
Figure 2Hemispherical photographs taken approximately at the maximum of green vegetation during the rainy season and the image classifications: (a) and (b) herbaceous canopy (Agoufou, 29 July 2007); (c) and (d) forest canopy (Kelma forest, 29 September 2016). Black zones on the (a) and (b) images correspond to masked areas. The processing of hemispherical images is restricted to 0–60∘ zenith angles to avoid mixed pixels. In the case of the forest canopy, note that the process could classify either vegetation elements like green herbaceous leaves (b) or non-vegetation elements like sky pixels (d).
At the seasonally inundated forest site, the unique field transect was limited to 0.5 km due to the difficulties associated with the field work in such an environment. At each site, the starting and ending points of the transects were georeferenced using global positioning systems (Garmin Trex©) to with approximately 10 m horizontal positional accuracy. At each sampling date, 100 or 50 hemispherical photographs were acquired at the 1 km herbaceous or 0.5 km forest sites, respectively, that is a picture taken every 10 m. At the forest site, photographs were acquired both in the upward and downward directions to sample the forest canopy and the herbaceous understorey. When the forest floor was inundated, which can occur during the rainy season, only the herbaceous vegetation component above the water surface was considered.
All the pictures were taken using a Nikon Coolpix 8400 (8 Mp) equipped with the fisheye FC-E9, viewing vertically downward above the herbaceous vegetation canopy with a distance between the top of the canopy and the lens of approximately 40 cm, providing a sampling area of approximately 1 m2 (Mougin et al., 2014). At the Acacia forest site, the camera was mounted on a levelled tripod, and orientated upward at a height of approximately 0.8 m above the forest floor, and maintained horizontally using a bubble level.
Prior to the field campaigns, the camera was calibrated using the method described in http://www.avignon.inra.fr/can_eye (last access: 13 May 2019) to compute the optical centre of the camera–fisheye lens system (Demarez et al., 2008). The collected hemispherical pictures were analysed using the image processing software CAN-EYE V5.0 which is dedicated to the classification of green and non-green vegetation elements and to series of photographs (Baret and Weiss, 2004). The CAN-EYE software provides LAI estimates from gap fraction measurements derived from the hemispherical photographs (Fig. 2b, d), and it has been proved to be efficient to derive the vegetation variables of herbaceous (Demarez et al., 2008; Mougin et al., 2014) and forest (Sea et al., 2011) canopies.
The main outputs of the CAN-EYE software are the LAI, the aggregation factor or clumping index λo, the vegetation cover, fCover, and the daily fraction of integrated absorbed photosynthetically active radiation, fAPAR (Table 1).
Finally, the estimated mean vegetation variables at the 1 km scale are computed by averaging all the 100 or 50 measurements acquired along the sampling transect for the herbaceous and forest canopy, respectively. The performance of the combined use of hemispherical photographs, and the CAN-EYE software for estimating LAI, λo, fAPAR and fCover at the 1 km transect scale has been analysed by Mougin et al. (2014) for a herbaceous canopy. These variables are estimated at a spatial scale which is compatible with remote-sensing observations performed by moderate resolution sensors, such as MODIS, SPOT-VEGETATION and PROBA-V. In particular, the clumping index brings valuable information on the temporal variation of the heterogeneity of grass cover, and can be retrieved from remote-sensing observations (Pisek et al., 2013; He et al., 2016). The accuracy associated to the field LAI estimates is approximately ±17.3 % for the herbaceous canopies (Mougin et al., 2014). Following the same approach and by taking into account the errors of classification and spatial sampling, the accuracy for the Acacia forest PAI is approximately ±36.5 % (this study).
Whenever possible, hemispherical photographs were taken approximately every 10 d during the growing seasons for the herbaceous canopies, whereas at the Kelma forest site, the monitoring took place approximately every 10 d during the leafy period, i.e. from July to January, and otherwise every month during the dry season. An illustration of the high seasonal temporal variation of LAI for five monitored herbaceous canopies, including the seasonally inundated herbaceous canopy, Kelma herbs, is given in Fig. 3 for the 2017 growing season. The strong temporal dynamics of the herbaceous cover justifies the 10 d resolution sampling to precisely characterize the seasonal vegetation cycle, particularly the maximum LAI, LAImax, which is usually observed towards the end of the rainy season. Afterwards, on sandy soils the herbaceous green canopy composed of annual plants, rapidly dried out. At the forest site, on clay soils temporarily flooded, the understorey did not dry as fast and is partly composed of a perennial grass Sporobolus helvolus. Large differences in LAImax values are observed among sites depending on soil fertility, soil water budget, intensity of grazing by livestock, and length of soil inundation in the clayed sites in depressions (no. 21 and no. 21b). In 2017, maximum LAI values, LAImax, ranged between 0.43 at the seasonally flooded Kelma site, and 1.58 at the Agoufou sandy site.
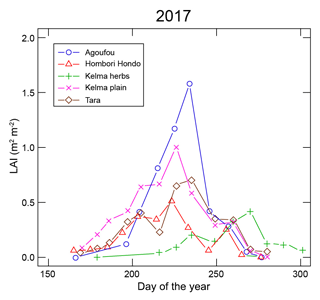
Figure 3Seasonal variations of the leaf area index (LAI) of herbaceous canopies observed in different sites from May to December 2017.
The magnitude of the inter-annual variability in LAI for herbaceous canopies observed over the 13-year monitoring period is quite high (see the example given in Fig. 4a), with temporal coefficients of variation higher than 40 % for the herbaceous sites, and up to approximately 100 % for the forest understorey (Table 2). Over the 2005–2017 period, the mean (SD) LAI value for the herbaceous sites on sandy soils was 0.96 (0.27).
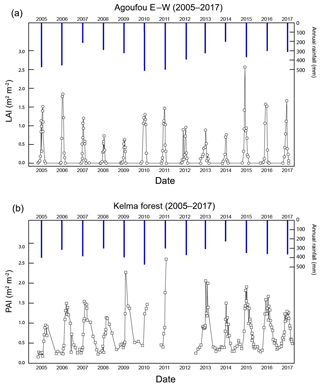
Figure 4Inter-annual variations of (a) the herbaceous leaf area index (LAI) at the Agoufou site, and (b) the plant area index (PAI) of the Kelma forest canopy recorded during the 2005–2017 period. Note that no data have been acquired from 29 September 2010 to 6 June 2011 and from 8 September 2011 to 4 March 2013 at the Kelma forest site, due to security issues. Total inter-annual precipitations are also shown.
Table 2Statistics of the maximum seasonal LAI, LAImax, of the herbaceous canopies over the 2005–2017 period. The number of monitored growing seasons (years) per site is also shown.
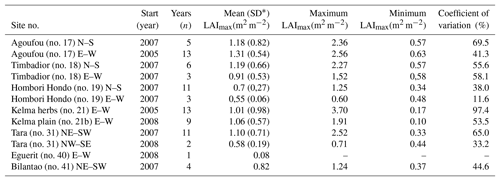
* Standard deviation.
Similarly, the observed PAI of the Kelma forest canopy exhibits large inter-annual variations, with maximum PAI values varying from 0.95 in 2005 to 2.6 in 2014. This corresponds to a foliage area index, LAI, of approximately 0.64 to 2.29 (Fig. 4b) once the wood area index, WAI, estimated at 0.31 by averaging PAI values during the leafless period, has been subtracted from the PAI estimates. Over the 2005–2017 period, the coefficient of variation of maximum PAI values of the Kelma forest is approximately 35.4 %, a value close to the 41.3 % estimated along the Agoufou E–W transect for the herbaceous LAImax.
The data set presented in this paper is part of the AMMA-CATCH observatory database (http://www.amma-catch.org, last access: 13 May 2019) and is identified by the permanent identifier https://doi.org/10.17178/AMMA-CATCH.CE.Veg_Gh (AMMA-CATCH, 2005), which describes its content with standardized metadata (DataCite1) and gives access instructions. Table 3 shows the data availability included in this data set: it covers the 2005–2017 period and is given for the 13 monitored transects. The dates of hemispherical photograph acquisitions are given in Table S4. Over the 13-year period, a total of 658 sampling transects have been monitored, and approximately 52 000 hemispherical images have been acquired, processed, and analysed. Associated data such as herbaceous aboveground masses, CO2, sensible and latent flux measurements, meteorological observations and soil moisture measurements collected at the Agoufou, Kelma and Eguerit sites can also be found in the AMMA-CATCH database.
Table 3Data availability (bold) for the different sites and transects sampled since the start of the monitoring in 2005. The number of transects performed per year and the total of transects sampled during the entire monitoring period are shown per site. The total of transects sampled during the 13-year monitoring period is 658.
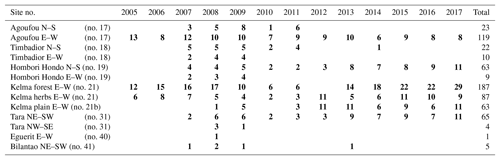
The data are available online, free of charge, via the web AMMA-CATCH database portal: http://bd.amma-catch.org (last access: 13 May 2019) and without any barriers except a registration. Registration is only required to download the data but not to browse the content of the data sets (metadata including variables names, spatial extent and coordinates, temporal extent, contacts, etc.), or to retrieve their content in a ISO 191152 standard file, or to localize on the map the acquisition sites. To access data to download, once connected to the portal, search for the data set by identifying it by its doi suffix (CE.Veg_Gh) or title among all the data sets contained in the database, and unfold the tab describing it. The database architecture also allows to extract a subset of the data at the spatio-temporal or variable level. The data files are provided in two formats: ASCII csv and NetCDF.
In addition to the documentation of the seasonal, inter-annual and decadal variations of vegetation variables in relation to rainfall variability, we present two examples of data use in validation exercises of remote-sensing products and surface models.
5.1 Validation of satellite products
Validation of existing LAI products is still a challenge (Xu et al., 2018a, b) and a homogeneous long-term LAI data set, based on an unchanged field protocol during more than a decade, is certainly of valuable interest with the objectives of validating satellite products (Fang et al., 2012). The spatial sampling along transects of 1 km, as performed during the present monitoring, has also been highly recommended for field-based validation of moderate resolution products (Baret et al., 2005; Sea et al., 2011). The temporal resolution of the sampling, i.e. 10 d, should allow LAI products to be accurately evaluated. Moreover, the corrections of the clumping effects performed using the estimated aggregation factor, enable another source of uncertainty to be considered, linked to the non-random green components distribution within the canopy (Chen et al., 1997; Kucharik et al., 1998).
Preliminary LAI and fCover 1 km estimates at the Timbadior site, with a different technique, have already been used in direct validation exercises using the SPOT-VEGETATION products within the frame of the VALERI project (Baret et al., 2005; Weiss et al., 2007; Fang et al., 2012), the MODIS products (Mougin et al., 2009b), and the Global LAnd Surface Satellite, GLASS, fCover products (Xiao et al., 2016). In this paper, we present two illustrations of a similar exercise using the LAI–fAPAR field data acquired during the 2005–2017 period, at the Agoufou savannah (Fig. 5a) and Kelma forest (Fig. 5b) sites, and the latest MODIS products (MOD15A2H, Collection 6) available since 2015 at a spatial resolution of 500 m (Myneni and Park, 2015). The Collection 6 benefited from improved surface reflectances and biome-type inputs (Wolfe et al., 2013) and provided more accurate products (Xu et al., 2018b).
5.2 Validation of land surface models
The LAI data set was also used to evaluate simulations by dynamic vegetation models, particularly those used in the AMMA Land Surface Models Intercomparison Exercise (ALMIP, Boone et al., 2009a, b; Grippa et al., 2017). This project was carried out to evaluate the capability of different state of the art land surface models to reproduce surface processes in West Africa. The second phase of ALMIP focused on the three mesoscale sites in Benin, Niger and Mali, instrumented by the AMMA-CATCH observatory (Galle et al., 2018) that provides specific data for forcing and evaluating model outputs. An example of the comparison between in situ LAI and simulated LAI by the Sahelian-Transpiration-Evaporation-Productivity model, STEP (Mougin et al., 1995; Pierre et al., 2016), for the ALMIP-2 runs is reported in Fig. 6. Both the seasonal and the inter-annual variability in LAI, including water stress events such as that happening in 2008, are well reproduced by STEP. However, the comparison with field data shows that the model response is a bit smoother than in the in situ data set.
A 13-year consistent LAI database was built for the 2005–2017 period, in a not easily accessible and weakly sampled semi-arid region, in central Sahel. The data set includes additional essential climatic variables such as the vegetation cover fraction, the fraction of absorbed photosynthetically active radiation, and the clumping index. All these variables were estimated from digital hemispherical photographs taken along 0.5 or 1 km transects crossing different sites in the Gourma region in north-east Mali. The same processing software, CAN-EYE, was used for image classification, gap fraction analysis and LAI derivation providing a homogeneous and consistent data set. Among others, this data set can be used to validate moderate-resolution satellite products, to establish relationships between satellite vegetation indices and vegetation variables, to evaluate land surface models, and to interpret surface fluxes. More specifically, this data set can be useful to interpret the current greening trend that has been observed widely over the Sahel since the 1980s drought.
Supplementary data sets related to AMMA-CATCH instruments, including meteorological, flux, soil moisture, herbaceous aboveground mass, tree species composition and woody plant population densities data can be downloaded from the AMMA-CATCH database (http://www.amma-catch.org/spip.php?rubrique63, last access: 13 May 2019). The supplement related to this article is available online at: https://doi.org/10.5194/essd-11-675-2019-supplement.
Data sets were collected by EM, MOD, NS, AM, VD and PH. Data sets were prepared by MOD and EM with the help of VC, who oversees the AMMA-CATCH database. All co-authors contributed to the criticizing of the individual data sets and contributed to the writing of the paper.
The authors declare that they have no conflict of interest.
The data are licensed under the Creative Common Attribution 4.0 International Licence3 (CC-BY 4.0) that sets the conditions of the data use. For any publication using AMMA-CATCH data, depending on the contribution of the data to the scientific results obtained, data users should either propose co-authorship to the principal investigators or at least acknowledge their contribution. Many thanks are expressed to the numerous people who participated in the field data acquisition: Tarawetti Ag Mostafa from Hombori, Hamma Maïga from Agoufou, Youssouf Maïga (deceased) from Gao, Alexis Berg, Marie-Noëlle Mulhaupt, Téri Massa-Mabilo and Yann Tracol. We thank Anne-Marie Cousin for her help with the figures. The two reviewers and the topical editor who provided helpful suggestions and comments on earlier versions of the paper are gratefully acknowledged.
This paper was edited by Falk Huettmann and reviewed by two anonymous referees.
AMMA-CATCH: Vegetation dataset (seasonal dynamics of herbaceous vegetation), within the Hombori site (2500 km2), Mali, IRD, CNRS-INSU, OSUG, OMP, OREME, https://doi.org/10.17178/AMMA-CATCH.CE.Veg_Gh, 2005.
Baret, F. and Weiss, M.: Can-Eye: processing digital photographs for canopy structure characterization, CAN EYE tutorial document, INRA-EMMAH UMR 114, Avignon, France, available at: https://www6.paca.inra.fr/can-eye (last access: 13 May 2019), 2004.
Baret, F., Weiss, M., Allard, D., Garrigue, S., Leroy, M., Jeanjean, H., Fernandes, R., Myneni, R., Privette, J., Morisette, J., Bohbot, H., Bosseno, R., Dedieu, G., Di Bella, C., Duchemin, B., Espana, M., Gond, V., Gu, X.F., Guyon, D., Lelong, C., Maisongrande, P., Mougin, E., Nilson, T., Veroustraete, F., and Vintilla, R.: VALERI: a network of sites and a methodology for the validation of medium spatial resolution land satellite products, doi:10.1.1.452, available at: https://www.academia.edu/ (last access: 13 May 2019), 2005.
Baret, F., Morissette, J. T., Fernandes, R. A., Champeaux, J. L., Myneni, Ranga B., Chen, J., Plummer, S., Weiss, M., Bacour, C., Garrigues, S., and Nickeson, J. E.: Evaluation of the Representativeness of Networks of Sites for the lobal Validation and Intercomparison of Land Biophysical Products: Proposition of the CEOS-BELMANIP, IEEE Trans. Geosci. Remote Sens., 44, 1794–1803, https://doi.org/10.1109/TGRS.2006.876030, 2006.
Baret, F., Weiss, M., Lacaze, R., Camacho, F., Makmara, H., Pacholczyk, P., and Smets, B.: GeoV1: LAI, FAPAR Essential Climate Variables and FCOVER global time series capitalizing over existing products. Part 1: Principles of development and production, Remote Sens. Environ., 137, 299–309, https://doi.org/10.1016/j.rse.2012.12.027, 2013.
Bojinski, S., Verstraete, M., Peterson, T. C., Richter, C., Simmons, A., and Zemp, M.: The concept of Essential Climate Variables in support of climate research, applications, and policy, B. Am. Meteorol. Soc., 95, 1431–1443, https://doi.org/10.1175/BAMS-D-13-00047.1, 2014.
Boone, A., de Rosnay, P., Balsamo, G., Beljaars, A., Chopin, F., Decharme, B., Delire, C., Ducharne, A., Gascoin, S., Guichard, F., Gusev, Y., Harris, P., Jarlan, L., Kergoat, L., Mougin, E., Nasonova, O., Norgaard, A., Orgeval, T., Ottlé, C., Poccard-Leclercq, I., Polcher, J., Sandholt, I., Saux-Picart, S., Taylor, C. M., and Xue, Y.: The AMMA Land Surface Model Intercomparison Project (ALMIP), B. Am. Meteorol. Soc., 90, 1865–1880, https://doi.org/10.1175/2009BAMS2786.1, 2009a.
Boone, A., Getirana, A. C. V., Demarty, J., Cappelaere, B., Galle, S., Grippa, M., Lebel, T., Mougin, E., Peugeot, C., and Vischel, T.: AMMA Land Surface Model Intercomparison Project Phase 2 (ALMIP-2), Gewex News, 9(4), International GEWEX Project Office, Silver Spring, MD, 9–10, 2009b.
Boulain, N., Cappelaere, B., Ramier, D., Issoufou, H. B. A., Halilou, O., Seghieri, J., Guillemin, F., Oi, M., Gignoux, J., and Timouk, F.: Towards an understanding of coupled physical and biological processes in the cultivated Sahel. 2. Vegetation and carbon dynamics, J. Hydrol., 375, 190–203, https://doi.org/10.1016/j.jhydrol.2008.11.045, 2009.
Breman, H. and Cissé, A. M.: Dynamics of sahelian pastures in relation to drought and grazing, Oecologia, 28, 301–315, https://doi.org/10.1007/BF00345986, 1977.
Camacho, F., Cernicharo, J., Lacaze, R., Baret, F., and Weiss, M.: GEOV1: LAI, FAPAR essential climate variables and FCOVER global time series capitalizing over existing products, Part 2: Validation and intercomparison with reference products, Remote Sens. Environ., 137, 310–329, https://doi.org/10.1016/j.rse.2013.02.030, 2013.
Carlson, T. N. and Ripley, D. A.: On the relation between NDVI, fractional vegetation cover, and Leaf Area Index, Remote Sens. Environ., 62, 241–252, https://doi.org/10.1016/S0034-4257(97)00104-1, 1997.
Chen, J. M. and Black, T. A.: Defining leaf area index for non-flat leaves, Plant Cell Environ., 15, 421–429, https://doi.org/10.1111/j.1365-3040.1992.tb00992.x, 1992.
Chen, J. M., Rich, P. M., Gower, S. T., Norman, J. M., and Plummer, S.: Leaf area index of boreal forests – Theory, techniques, and measurements, J. Geophys. Res., 102, 29429–29443, https://doi.org/10.1029/97JD01107, 1997.
Delon, C., Mougin, E., Serça, D., Grippa, M., Hiernaux, P., Diawara, M., Galy-Lacaux, C., and Kergoat, L.: Modelling the effect of soil moisture and organic matter degradation on biogenic NO emissions from soils in Sahel rangeland (Mali), Biogeosciences, 12, 3253–3272, https://doi.org/10.5194/bg-12-3253-2015, 2015.
Demarez, V., Dutoit, S., Baret, F., Weiss, M., and Dedieu, G.: Estimation of leaf area and clumping indexes of crops with hemispherical photographs, Agr. Forest Meteorol. 148, 644–655, https://doi.org/10.1016/j.agrformet.2007.11.015, 2008.
Fang, H., Wei, S., and Liang, S.: Validation of MODIS and CYCLOPES LAI products using global field measurement data, Remote Sens. Environ., 119, 43–54, https://doi.org/10.1016/j.rse.2011.12.006, 2012.
Fensholt, R., Sandholt, I., Rasmussen, M. S., Stisen, S., and Diouf, A.: Evaluation of satellite based primary production modeling in the semi-arid Sahel, Remote Sens. Environ., 105, 173–188, https://doi.org/10.1016/j.rse.2003.07.002, 2006.
François, M., Santandrea, S., Mellab, K., Davy Vrancken, D., and Versluys, J.: The PROBA-V mission: the space segment, Int. J.f Remote Sens., 35, 2548–2564, https://doi.org/10.1080/01431161.2014.883098, 2014.
Gaillardet, J., Braud, I., et al.: OZCAR: the French network of Critical Zone Observatories, Vadose Zone J., Special issue on Hydrological Observatories, 17, 180067, https://doi.org/10.2136/vzj2018.04.0067, 2018.
Galle, S., Grippa, M., Peugeot, C., Bouzou Moussa, I., Cappelaere, B., Demarty, J., Mougin, E., Panthou, G., Adjomayi, P., Agbossou, E. K., Ba, A., Boucher, M., Cohard, J.-M., Descloitres, M., Descroix, L., Diawara, M., Dossou, M., Favreau, G., Gangneron, F., Gosset, M., Hector, B., Hiernaux, P., Issoufou, B.-A., Kergoat, L., Lawin, E., Lebel, T., Malam Abdou, M., Malam-Issa, O., Mamadou, O., Nazoumou, Y., Pellarin, T., Quantin, G., Sambou, B., Seghieri, J., Séguis, L., Vandervaere, J-P., Vischel, T., Vouillamoz, J.-M., Wubda, M., Zannou, A., Alhassane, A., Afouda, S., Arjounin, M., Barral, H., Biron, R., Boubkraoui, S., Chaffard, V., Cazenave, F., Chazarin, J.-P., Guyard, H., Koné, A., Mainassara, I., Maman, A., Oi, M., Ouani, T., Soumaguel, N., Ago, E. E., Allé, C., Allies, A., Arpin-Pont, F., Awessou, B., Cassé, C., Charvet, G., Dardel, C., Depeyre, A., Diallo, B., Do, T., Fatras, C., Gal, L., Gascon, T., Gibon, F., Guiro, I., Ingatan, A., Kempf, J., Kotchoni, V., Lawson, F., Léauthaud, C., Louvet, S., Mason, E., Nguyen, C. C., Perrimond, B., Pierre, C., Richard, A., Robert, E., Roman, C., Velluet, C., and Wilcox, C.: AMMA-CATCH a critical zone observatory in West Africa monitoring a region in transition, in: Vadose Zone J., edited by: Jensen, K. H., Bogena, H., White, T., Li, X., and Bour, O., Special issue on Hydrological Observatories, https://doi.org/10.2136/vzj2018.03.0062, 2018.
Garrigues, S., Allard, D., Baret, F., and Weiss, M.: Influence of the spatial heterogeneity on the non linear estimation of Leaf Area Index from moderate resolution remote sensing data, Remote Sens. Environ., 106, 286–298, https://doi.org/10.1016/j.rse.2006.07.013, 2006.
Garrigues, S., Lacaze, R., Baret, F., Morisette, J. T., Weiss, M., Nickeson, J. E., Fernandes, R., Plummer, S., Shabanov, N. V., Myneni, R. B., Knyazikhin, Y., and Yang, W.: Validation and intercomparison of global leaf area index products derived from remote sensing data, J. Geophys. Res.-Biogeosci., 113, G02028, https://doi.org/10.1029/2007JG000635, 2008.
GCOS: Systematic Observation Requirements for Satellite-based Products for Climate, Supplemental Details to the Satellite-based Component of the Implementation Plan for the Global Observing System for Climate in Support of the UNFCCC (update, December), available at: https://library.wmo.int/index.php?lvl=notice_display&id=12907#.XNleX6Q6-Ul (last access: 13 May 2019), 2011.
Grippa, M., Kergoat, L., Boone, A., Peugeot, C., Demarty, J., Cappelaere, B., Gal, L., Hiernaux, P., Mougin, E., Ducharne, A., Dutra, E., Anderson, M., Hain, C., Ait-Mesbah, S., Balsamo, G., Bousetta, S., Chaffard, V., Chan, E., Cohard, J. M., Favot, F., Gascon, T., Galle, S., Gosset, M., Guichard, F., Gusev, Y., Harris, P., He, X., Hector, B., Kaptue, A., Kazuaki, Y., Kim, H., Kumar, S., Lebel, T., Lo, M.-H., Mahanama, S., Magand, C., Maignan, F., Mamadou, O., Nasonova, O., Oki, T., Ottlé, C., Pellarin, T., Pierre, C., Richard, A., Roujean, J.-L., Polcher, J., Quantin, G., Séguis, L., Shmakin, A., Shunji, K., Sokratov, V., Tanaka, K., Turkov, D., Velluet, C., Verseghy, D., Vischel, T., and Zin, I.: Modelling surface runoff and water fluxes over contrasted soils in pastoral Sahel: evaluation of the ALMIP-2 land surface models over the Gourma region in Mali, J. Hydrometeorol., ALMIP-2 special issue, 1847–1865, https://doi.org/10.1175/JHM-D-16-0170.1, 2017.
Guichard, F., Kergoat, L., Mougin, E., Timouk, F., Baup, F., and Lavenu, F.: Surface thermodynamics and radiative budget in the sahelian Gourma. Seasonal and diurnal cycles, J. Hydrol., AMMA CATCH special issue, 375, 161–177, https://doi.org/10.1016/j.jhydrol.2008.09.007, 2009.
Hanan, N. P. and Bégué, A.: A method to estimate instantaneous and daily intercepted photosynthetically active radiation using a hemispherical sensor, Agr. Forest Meteorol., 74, 155–168, https://doi.org/10.1016/0168-1923(94)02196-Q, 1995.
He, L., Liu, J., Chen, J. M., Croft, H., Wang, R., Sprintsin, M., Zheng, T., Ryu, Y., Pisek, J., Gonsamo, A., Deng, F., and Zhang, Y.: Inter-and intra-annual variations of clumping index derived from the MODIS BRDF product, Int. J. Appl. Earth Obs. Geoinformation, 44, 53–60, https://doi.org/10.1016/j.jag.2015.07.007, 2016.
Hiernaux, P. and Le Houérou, H. N.: Les parcours du Sahel, Sècheresse, 17, 51–71, 2006.
Hiernaux, P., Cissé, M. I., and Diarra, L.: Bilan d'une saison des pluies 1984 très déficitaire dans le Gourma (Sahel malien), Première campagne de suivi et télédétection expérimentale, Annexe: Fiches descriptives des sites, Addis Ababa, Ethiopia: ILCA, 90 p., 1984.
Hiernaux, P., Mougin, E., Diarra, L., Soumaguel, N., Lavenu, F., Tracol, Y., and Diawara, M.: Rangeland response to rainfall and grazing pressure over two decades: herbaceous growth pattern, production and species composition in the Gourma, Mali, J. Hydrol., 375, 114–127, https://doi.org/10.1016/j.jhydrol.2008.11.005, 2009a.
Hiernaux, P., Lassine, D., Trichon, V., Mougin, E., and Baup, F.: Woody plant population dynamics in response to climate changes from 1984 to 2006 in Sahel (Gourma, Mali), J. Hydrol., 375, 103–113, https://doi.org/10.1016/j.jhydrol.2009.01.043, 2009b.
Jarlan, L., Mangiarotti, S., Mougin, E., Hiernaux, P., Mazzega, P., and Le Dantec, V.: Assimilation of SPOT/VGT-NDVI data into a sahelian grassland model, Remote Sens. Environ., 112, 1381–1394, https://doi.org/10.1016/j.rse.2007.02.041, 2008.
Kucharik, C. J., Norman, J. M., and Gower, S. T.: Measurements of branch area and adjusting leaf area index indirect measurements, Agr. Forest Meteorol., 91, 69–88, https://doi.org/10.1016/S0168-1923(98)00064-1, 1998.
Lebel, T., Diedhiou, A., and Laurent, H.: Seasonal cycle and interannual variability of the Sahelian rainfall at hydrological scales, J. Geophys. Res., 108, 8389, https://doi.org/10.1029/2001JD001580, 2003.
Lebel, T., Cappelaere, B., Descroix, L., Galle, S., Hanan, N.P., Kergoat, L., Levis, S., Mougin, E., Peugeot, C., and Vieux, B.: AMMA-CATCH studies in the Sahelian region of West-Africa: an overview, J. Hydrol., 375, 3–13, https://doi.org/10.1016/j.jhydrol.2009.03.020, 2009.
Lebel, T., Parker, D. J., Flamant, C., Bourles, B., Marticorena, B., Mougin, E., Peugeot, C., Diedhiou, A., Haywood, J., Ngamini, J. B., Polcher, J., Redelsperger, J.-L., and Thorncroft, C. D.: The AMMA field campaigns: Multiscale and multidisciplinary observations in the West African region, Q. J. Roy. Meteorol. Soc., AMMA Special Issue, 136, 8–33, https://doi.org/10.1002/qj.486, 2010.
Le Houérou, H. N.: The grazing land ecosystems of the African Sahel. Ecological studies, 75, Springer-Verlag, Berlin, 282 pp., 1989.
Levy, P. E. and Jarvis, P. G.: Direct and indirect measurements of LAI in millet and fallow vegetation in HAPEX-Sahel, Agr. Forest Meteorol., 97, 199–212, https://doi.org/10.1016/S0168-1923(98)00092-6, 1999.
McCallum, I., Wagner, W., Schmullius, C., Shvidenko, A., Obersteiner, M., Fritz, S., and Nilsson, S.: Satellite-based terrestrial production efficiency modeling, Carbon Bal. Manage., 4, 8, https://doi.org/10.1186/1750-0680-4-8, 2009.
Morisette, J. T., Baret, F., Privette, J. L., Myneni, R. B., Nickeson, J. E., Garrigues, S., Shabanov, N. V., Weiss, M., Fernandes, R. A., Sylvain, G., Leblanc, S. G., Kalacska, M., Arturo Sánchez-Azofeifa, G., Chubey, M., Rivard, B., Stenberg, P., Rautiainen, M., Voipio, P., Manninen, T., Pilant, A. N., Lewis, T. E., Iiames, J. S., Colombo, R., Meroni, M., Busetto, L., Cohen, W. B., Turner, D. P., Warner, E. D., Petersen, G. W., Seufert, G., and Cook, R.: Validation of global moderate-resolution LAI products: A framework proposed within the CEOS land product validation subgroup, IEEE Trans. Geosci. Remote Sens., 44, 1804–1817, https://doi.org/10.1109/TGRS.2006.872529, 2006.
Mougin, E., Lo Seen, D., Rambal, S., Gaston, A., and Hiernaux, P.: A regional Sahelian grassland model to be coupled with multispectral satellite data. I. Description and validation, Remote Sens. Environ., 52, 181–193, https://doi.org/10.1016/0034-4257(94)00126-8, 1995.
Mougin, E., Hiernaux, P., Kergoat, L., Grippa, M., de Rosnay, P., Timouk, F., Le Dantec, V., Demarez, V., Lavenu, F., Arjounin, M., Lebel, T., Soumaguel, N., Ceschia, E., Mougenot, B., Baup, F., Frappart, F., Frison, P.L., Gardelle, J., Gruhier, C., Jarlan, L., Mangiarotti, S., Sanou, B., Tracol, Y., Guichard, F., Trichon, V., Diarra, L., Soumaré, A., Koité, M., Dembélé, F., Lloyd, C., Hanan, N.P., Damesin, C., Delon, C., Serça, D., Galy-Lacaux, C., Seghieri, J., Becerra, S., Dia, H., Gangneron, F., and Mazzega, P.: The AMMA Gourma observatory site in Mali: Relating climatic variations to changes in vegetation, surface hydrology, fluxes and natural resources, J. Hydrol., 375, 14–33, https://doi.org/10.1016/j.jhydrol.2009.06.045, 2009a.
Mougin, E., Demarez, V., Grippa, M., Hiernaux, P., Larouzière, L., Lion, C., Diawara, M., and Soumaguel, N.: Evaluation of MODIS LAI products in a Sahelian environment (Gourma, Mali), poster presented at the 4th Global Vegetation Workshop, Univ. of Mont., Missoula, 2009b.
Mougin, E., Demarez, V., Diawara, M., Hiernaux, P., Soumaguel, N., and Berg, A.: Estimation of LAI, fCover and fAPAR of Sahel rangelands (Gourma, Mali), Agr. Forest Meteorol., 198–199, 155–167, https://doi.org/10.1016/j.agrformet.2014.08.006, 2014.
Myneni, R. B. and Williams, D. L.: On the relationship between FAPAR and NDVI, Remote Sens. Envioron., 49, 200–211, https://doi.org/10.1016/0034-4257(94)90016-7, 1994.
Myneni, R. and Park, Y. K. T.: MOD15A2H MODIS/Terra Leaf Area Index/FPAR 8-Day L4 Global 500m SIN Grid V006, NASA EOSDIS Land Processes DAAC, https://doi.org/10.5067/MODIS/MOD15A2H.006, 2015.
Myneni, R. B., Hoffman, S., Knyazikhin, Y., Privette, J. L., Glassy, J., Tian, Y., Wang, Y. J., Song, X., Zhang, Y. M., Smith, G. R., Lotsch, A., Friedl, M., Morisette, J. T., Votava, P., Nemani, R. R., and Running, S. W.: Global products of vegetation leaf area and absorbed PAR from year one of MODIS data, Remote Sens. Environ., 83, 214–231, https://doi.org/10.1016/S0034-4257(02)00074-3, 2002.
Nicholson, S., Tucker, C. J., and Ba, M. B.: Desertification, Drought, and Surface Vegetation: An Example from the West African Sahel, B. Am. Meteorol. Soc., 79, 815–829, https://doi.org/10.1175/1520-0477(1998)079<0815:DDASVA>2.0.CO;2 , 1998.
Penning De Vries, F. w. T. and Djitèye, M. A. (Eds.): La productivité des paturages sahéliens, PUDOC Publ., Center for Agie. Puol and Docum., Wageningen, 525 pp., 1982.
Pierre, C., Bergametti, G., Marticorena, B., Mougin, E., Bouet, C., and Schmechtig, C.: Impact of vegetation and soil moisture seasonal dynamics on dust emissions over the Sahelian belt in West Africa, J. Geophys. Res., 117, D06115, https://doi.org/10.1029/2011JD016950, 2012.
Pierre, C., Grippa, M., Mougin, E., Guichard, F., and Kergoat, L.: Changes in Sahelian annual vegetation growth and phenology since 1960: a modeling approach, Global Planet. Change, 143, 162–174, https://doi.org/10.1016/j.gloplacha.2016.06.009, 2016.
Pisek, J., Ryu, Y., Sprintsin, M., He, L., Oliphant, A. J., Korhonen, L., Kuusk, J., Kuusk, A., Bergstrom, R., Verrelst, J., and Alikas, K.: Retrieving vegetation clumping index from multi-angle imaging SpectroRadiometer (MISR) data at 275 m resolution, Remote Sens. Environ., 138, 126–133, https://doi.org/10.1016/j.rse.2013.07.014, 2013.
Potter, C. S., Randerson, J. T., Field, C. B., Matson, P. A., Vitousek, P. M., Mooney, H. A., and Klooster, S. A.: Terrestrial ecosystem production: A process model based on global satellite and surface data, Global Biogeochem. Cy., 7, 811–841, https://doi.org/10.1029/93GB02725, 1993.
Privette, J. L., Myneni, R. B., Knyazikhin, Y., Mukelabai, M., Roberts, G., Tian, Y., Wang, Y., and Leblanc, S. G.: Early spatial and temporal validation of MODIS LAI product in the Southern Africa Kalahari, Remote Sens. Environ., 83, 232–243, https://doi.org/10.1016/S0034-4257(02)00075-5, 2002.
Redelsperger, J.-L., Thorncroft, C., Diedhiou, A., Lebel, T., Parker, D. J., and Polcher, J.: African Monsoon Multidisciplinary Analysis (AMMA): An international research project and field campaign, B. Am. Meteorol. Soc. 87, 1739–1746, https://doi.org/10.1175/BAMS-87-12-1739, 2006.
Running, S. W. and Gower, S. T.: FOREST-BGC, a general model of forest ecosystem processes for regional applications, II. Dynamic carbon allocation and nitrogen budgets, Tree Physiol., 9, 147–160, https://doi.org/10.1093/treephys/9.1-2.147, 1991.
Running, S. W., Nemani, R. R., Heinsch, F. A., Zhao, M. S., Reeves, M., and Hashimoto, A.: Continuous satellite-derived measure of global terrestrial primary production, Bioscience, 54, 547–560, https://doi.org/10.1641/0006-3568(2004)054[0547:ACSMOG]2.0.CO;2, 2004.
Samain, O., Kergoat, L., Hiernaux, P., Guichard, F., Mougin, E., Timouk, F., and Lavenu, F.: Analysis of the in-situ and MODIS albedo variability at multiple time scales in the Sahel, J. Geophys. Res.-Atmos., 113, D14119, https://doi.org/10.1029/2007JD009174, 2008.
Sea, W. B., Choler, P., Beringer, J., Weinmann, R. A., Hutley, L. B., and Leuning, R.: Documenting improvement in leaf area index estimates from MODIS using hemispherical photos for Australian savannas, Agr. Forest Meteorol., 151, 1453–1461, https://doi.org/10.1016/j.agrformet.2010.12.006, 2011.
Sellers, P. J.: Canopy reflectance, photosynthesis and transpiration, Int. J. Remote Sens., 6, 1335–1372, https://doi.org/10.1080/01431168508948283, 1985.
Soegaard, H., Lund, M. R., and Bolwig, S.: A comparison of modelled and measured millet production in northeastern Burkina Faso.Geografisk Tidsskrift, Danish J. Geogr., Special Issue, 2, 69–80, 1999.
Stige, L. C., Stave, J., Chan, K.-S., Ciannelli, L., Pettorelli, N., Glantz, M., and Stenseth, N. C.: The effect of climate variation on agro-pastoral production in Africa, P. Natl. Acad. Sci. USA, 103, 3049–3053, https://doi.org/10.1073/pnas.0600057103, 2006.
Timouk, F., Kergoat, L., Mougin, E., Lloyd, C., Ceschia, E., de Rosnay, P., Hiernaux, P., and Demarez, V.: Response of sensible heat flux to water regime and vegetation development in a central Sahelian landscape, J. Hydrol., AMMA CATCH special issue, 375, 178–189, https://doi.org/10.1016/j.jhydrol.2009.04.022, 2009.
Tracol, Y., Mougin, E., Jarlan, L., and Hiernaux, P.: Testing a sahelian grassland functioning model against herbage mass measurements, Ecol. Model., 193, 437–446, https://doi.org/10.1016/j.ecolmodel.2005.08.033, 2006.
Verger, A., Baret, F., Weiss, M., Filella, J., and Peñuelas, J.: GEOCLIM: A global climatology of LAI, FAPAR, and FCOVER from VEGETATION observations for 1999–2010, Remote Sens. Environ., 166, 126–137, https://doi.org/10.1016/j.rse.2015.05.027, 2015.
Wang, L., Good, S. P., and Caylor, K. K.: Global synthesis of vegetation control on evapotranspiration partitioning, Geophys. Res. Lett., 41, 6753–6757, https://doi.org/10.1002/2014GL061439, 2014.
Wang, Y. P., Houlton, B. Z., and Field, C. B.: A model of biogeochemical cycles of carbon, nitrogen, and phosphorus including symbiotic nitrogen fixation and phosphatase production, Global Biochem. Cy., 21, GB1018, https://doi.org/10.1029/2006GB002797, 2007.
Watson, D. J.: Comparative physiological studies in growth of field crops. I. Variation in net assimilation rate and leaf area between species and varieties, and within and between years, Ann. Bot., 11, 41–76, 1947.
Weiss, M., Baret, F., Smith, G. J., Jonckheere, I., and Coppin, P.: Review of methods for in situ leaf area index (LAI) determination. Part II. Estimation of LAI, errors and sampling, Agr. Forest Meteorol., 121, 37–53, https://doi.org/10.1016/j.agrformet.2003.08.001, 2004.
Weiss, M., Baret, F., Garrigues, S., and Lacaze, R.: LAI and FPAR CYCLOPES global products derived from VEGETATION. Part 2. Validation and comparison with MODIS collection 4 products, Remote Sens. Environ., 110, 317–331, https://doi.org/10.1016/j.rse.2007.03.001, 2007.
Wolfe, R. E., Devadiga, S., Masuoka, E. J., Running, S. W., Vermote, E., Giglio, L., Wan, Z., Riggs, G. A., Schaaf, C., Myneni, R. B., Friedl, M. A., Wang, Z., Sulla-menashe, D. J., and Zhao, M.: Improvements to the MODIS Land Products in Collection Version 6, Abstract id. B41D-0430, available at: http://adsabs.harvard.edu/abs/2013AGUFM.B41D0430W (last access: 13 May 2019), American Geophysical Union, Fall Meeting, 2013.
Xiao, Z., Wang, T., Liang, S., and Sun, R.: Estimating the Fractional Vegetation Cover from GLASS Leaf Area Index Product, Remote Sens., 8, 1–19, https://doi.org/10.3390/rs8040337, 2016.
Xu, B., Park, T., Yan, K., Chen, C., Zeng, Y., Song, W., Yin, G., Li, J., Liu, Q., Knyazikhin, Y., and Myneni, R. B.: Analysis of Global LAI/FPAR Products from VIIRS and MODIS Sensors for Spatio-Temporal Consistency and Uncertainty from 2012–2016, Forests, 9, 73, https://doi.org/10.3390/f9020073, 2018a.
Xu, B., Li, J., Park, T., Liu, G., Zeng, Y., Yin, G., Zhao, J., Fan, W., Yang, L., Knyazikhin, Y., and Myneni, R. B.: An integrated method for validating long-term leaf area index products using global networks of site-based measurements, Remote Sens. Environ., 209, 134–151, https://doi.org/10.1016/j.rse.2018.02.049, 2018b.
Yan, K., Park, T., Yan, G., Chen, C., Yang, B., Liu, Z., Nemani, R. R., Knyazikhin, Y., and Myneni, R. B.: Evaluation of MODIS LAI/FPAR Product Collection 6. Part 1: Consistency and Improvements, Remote Sens., 8, 359, https://doi.org/10.3390/rs8050359, 2016a.
Yan, K., Park, T., Yan, G., Chen, C., Yang, B., Liu, Z., Nemani, R. R., Knyazikhin, Y., and Myneni, R. B.: Evaluation of MODIS LAI/FPAR Product Collection 6. Part 2: Validation and intercomparison, Remote Sens., 8, 460, https://doi.org/10.3390/rs8060460, 2016b.
DataCite: Locate, identify, and cite research data, https://www.datacite.org/, last access: 13 May 2019
ISO 19115: geographic information – metadata standards, https://www.iso.org/standard/53798.html (last access: 13 May 2019)
The following sentence should appear in the acknowledgments of the publication: “The AMMA-CATCH regional observing system (http://www.amma-catch.org/?lang=fr, last access: 13 May 2019) was set up thanks to an incentive funding of the French Ministry of Research that allowed pooling together various pre-existing small-scale observing setups. The continuity and long-term perennity of the measurements are made possible by an undisrupted IRD funding since 1990 and by a continuous CNRS-INSU funding since 2005”.