the Creative Commons Attribution 4.0 License.
the Creative Commons Attribution 4.0 License.
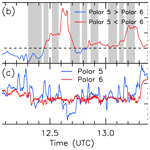
A comprehensive in situ and remote sensing data set from the Arctic CLoud Observations Using airborne measurements during polar Day (ACLOUD) campaign
Manfred Wendisch
Christof Lüpkes
Matthias Buschmann
Heiko Bozem
Dmitri Chechin
Hans-Christian Clemen
Régis Dupuy
Olliver Eppers
Jörg Hartmann
Andreas Herber
Evelyn Jäkel
Emma Järvinen
Olivier Jourdan
Udo Kästner
Leif-Leonard Kliesch
Franziska Köllner
Mario Mech
Stephan Mertes
Roland Neuber
Elena Ruiz-Donoso
Martin Schnaiter
Johannes Schneider
Johannes Stapf
Marco Zanatta
The Arctic CLoud Observations Using airborne measurements during polar Day (ACLOUD) campaign was carried out north-west of Svalbard (Norway) between 23 May and 6 June 2017. The objective of ACLOUD was to study Arctic boundary layer and mid-level clouds and their role in Arctic amplification. Two research aircraft (Polar 5 and 6) jointly performed 22 research flights over the transition zone between open ocean and closed sea ice. Both aircraft were equipped with identical instrumentation for measurements of basic meteorological parameters, as well as for turbulent and radiative energy fluxes. In addition, on Polar 5 active and passive remote sensing instruments were installed, while Polar 6 operated in situ instruments to characterize cloud and aerosol particles as well as trace gases. A detailed overview of the specifications, data processing, and data quality is provided here. It is shown that the scientific analysis of the ACLOUD data benefits from the coordinated operation of both aircraft. By combining the cloud remote sensing techniques operated on Polar 5, the synergy of multi-instrument cloud retrieval is illustrated. The remote sensing methods were validated using truly collocated in situ and remote sensing observations. The data of identical instruments operated on both aircraft were merged to extend the spatial coverage of mean atmospheric quantities and turbulent and radiative flux measurement. Therefore, the data set of the ACLOUD campaign provides comprehensive in situ and remote sensing observations characterizing the cloudy Arctic atmosphere. All processed, calibrated, and validated data are published in the World Data Center PANGAEA as instrument-separated data subsets (Ehrlich et al., 2019b, https://doi.org/10.1594/PANGAEA.902603).
- Article
(4959 KB) - Full-text XML
-
Supplement
(20953 KB) - BibTeX
- EndNote
The considerable increase in Arctic near-surface temperatures within the last 3 to 4 decades, a phenomenon commonly called Arctic amplification (Serreze and Barry, 2011), significantly exceeds the global warming and is associated with the decrease in Arctic sea ice. To improve the understanding and the ability to predict these changes, several international efforts, including joint model evaluations such as the Year of Polar Prediction within the Polar Prediction Project (Jung et al., 2016) and a series of observational field campaigns are underway. These observations obtained by land-based (Uttal et al., 2016), ship-based, and airborne activities (Wendisch et al., 2019) are essential to identify the dominant atmospheric processes and provide an observational basis for model and satellite data validations. Due to the diversity of instrumentation and required measurement strategies, these field campaigns often target specific components of the Arctic climate system.
In May and June 2017, two concerted field studies, the Arctic CLoud Observations Using airborne measurements during polar Day (ACLOUD) campaign and the Physical Feedbacks of Arctic Boundary Layer, Sea Ice, Cloud and Aerosol (PASCAL) ship cruise were performed to improve our understanding of the role of clouds and aerosol particles in Arctic amplification (Wendisch et al., 2019). Both campaigns were conducted within the framework of the “Arctic Amplification: Climate Relevant Atmospheric and Surface Processes, and Feedback Mechanisms (AC)3” project (Wendisch et al., 2017). During ACLOUD, two research aircraft, Polar 5 and Polar 6 (Wesche et al., 2016), were operated, which were stationed on Svalbard (Longyearbyen, Norway). For PASCAL the Research Vessel (R/V) Polarstern (Knust, 2017) entered the sea ice north of Svalbard, where an ice floe camp (including a tethered balloon, ground-based remote sensing, and in situ sampling of aerosol particles) was set up for 2 weeks (Macke and Flores, 2018). These observations were accompanied by permanent measurements at the joint research base AWIPEV at Ny-Ålesund on Svalbard (Neuber, 2006) operated by the Alfred Wegener Institute (AWI) and the French Polar Institute Paul-Émile Victor (IPEV; AWIPEV). The airborne operations during ACLOUD were coordinated with the ship-based (PASCAL) and ground-based activities (AWIPEV) and focused on the area north-west of Svalbard, linking the observations at AWIPEV and on Polarstern.
The general objectives of ACLOUD and PASCAL, the operated instrumentation, a summary of the measurement activities, and first highlights of the data analysis are presented by Wendisch et al. (2019), while the meteorological conditions during the observational period were analysed by Knudsen et al. (2018). In this paper, a detailed overview of the processed ACLOUD data set obtained on board both research aircraft is provided. The aim is to document the campaign-specific instrument operation, data processing, uncertainties of the derived quantities, and data availability to facilitate a widespread use of the data in a broad field of scientific analysis. To understand the aim and flight patterns of each research flight, in Sect. 2 an overview of the main scientific targets and the most common flight patterns is provided. The instrumentation, calibration, and data processing of measurements on Polar 5 and 6 are described in Sects. 3 and 4. Due to the operation of two identical aircraft (partly with identical instrumentation), several benefits arise for the data analysis. Coordinated observations from both aircraft flying in close collocation, e.g. remote sensing and in situ measurements, were combined as demonstrated in Sect. 5.1. In Sect. 5.2, the consistency of data from similar instruments operated on both aircraft is validated, which allows for merging observations from both aircraft into a single data set. The data availability, including links to the published data sets, is given in Sect. 6.
Table 1Overview of ACLOUD flights, including the takeoff and landing times of Polar 5 and 6 and the general scientific target of the flight. The objectives are categorized into cloud remote sensing (CRS), in situ cloud and aerosol particle and trace gas measurements (in situ), surface fluxes (SF), and flux profiles (FP). The remaining columns indicate if Polar 5 and 6 overflew Polarstern (PS) or Ny-Ålesund (NÅ), flew in collocated formation (Polar 5 above Polar 6), or were coordinated with an overpass of the NASA A-Train constellation.
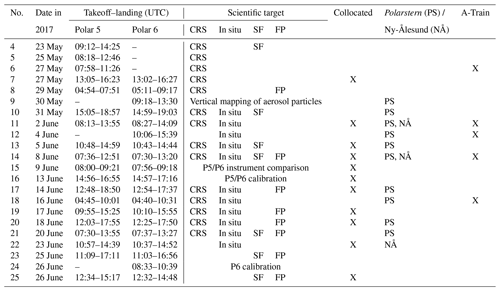
The ACLOUD aircraft campaign performed 22 research flights between 23 May and 26 June 2017, which are listed in Table 1 (flight numbers start with no. 4, neglecting the test and ferry flight nos. 1–3). In total, measurements were obtained in 165 flight hours distributed equally to both aircraft. A joint operation of Polar 5 and 6 was coordinated for 16 research flights. The general scientific goals of all ACLOUD flights are summarized by Wendisch et al. (2019). Most flights included different flight sections to address more than only one of the specific objectives. The dedicated missions and flight patterns can be categorized as follows.
-
Characterization of boundary layer clouds by remote sensing and in situ microphysical measurements. For this objective, 11 closely collocated flights with Polar 5 performing remote sensing in high altitudes (up to 4000 m) and Polar 6 sampling clouds below (down to 70 m above sea level) were conducted (column “collocated” in Table 1). The collocation of both aircraft aims to study the identical cloud section without horizontal or temporal mismatch. To obtain vertical profiles of cloud and aerosol particle properties and trace gases, horizontal legs in different altitudes were flown in double-triangle pattern, where Polar 6 changed altitude after each triangle and Polar 5 remained at high altitude. Longer straight flight sections crossing the marginal sea ice zone aim to study the contrast of clouds over open ocean and sea ice and release series of dropsondes. Table 1 indicates which flights include segments with cloud remote sensing (CRS) and in situ cloud and aerosol particle and trace gas measurements (in situ).
-
Satellite validation. Five research flights contain legs, which are time synchronized with overpasses of the NASA A-Train satellite constellation (Stephens et al., 2018, column “A-Train”) and flown parallel to their tracks. Within a certain time window, which depends on wind speed and cloud evolution, these data aim for a direct comparison of cloud structures observed from satellite and aircraft.
-
Comparison with ground-based observation. When possible, flight activities were coordinated with the PASCAL campaign of the research vessel Polarstern, which was met 10 times (column “Polarstern”), and with ground-based observations at Ny-Ålesund (column “Ny-Ålesund”), which was overpassed 4 times. To compare the ground-based and airborne observations in an area of comparable size, mostly double-triangle patterns were performed over the ground stations.
-
Near-surface turbulent and radiative fluxes. To quantify the turbulent and radiative fluxes at the surface (column “SF” in Table 1), long horizontal flight segments at low altitude were implemented in the research flights. In the case of cloudy conditions, a flight altitude below the cloud base was chosen.
-
Profiles of turbulent and radiative fluxes. Eight flights were partly dedicated to characterizing the vertical profiles of turbulent and radiative fluxes in the cloud-free and cloudy atmospheric boundary layer (column “FP”). For this mission, vertical stacks of short horizontal legs in different altitudes were flown across the main wind direction. During three flights, these patterns were flown jointly by both, horizontally separated from each other by 20–50 km.
-
Vertical mapping of aerosol particles. One single flight of Polar 6 aimed to map the vertical distribution of aerosol particles at two locations along the main wind direction. To do so, at each location horizontal legs in different altitudes were flown across the wind direction.
-
Instrument calibration and comparison. Three flights were dedicated to comparing the measurements of both aircraft and calibrating different instruments. For the comparison a joint ascent with both aircraft separated by less than 100 m was flown. The calibrations required instrument-specific calibration flight patterns.
For each flight, a flight report was compiled summarizing the major information about the flight required to recapture the objectives and their implementation. The flight reports are provided in the Supplement. Coordinated flights of Polar 5 and Polar 6 are combined in a single report. The reports include the flight track, description of predicted and present weather conditions, instrument performance, photographs, and notes.
A comprehensive general overview of airborne instrumentation in general is given by Wendisch and Brenguier (2013). Many of the instruments installed on Polar 5 and 6 are described in detail in this reference. Polar 5 was primarily operated as a remote sensing aircraft. Active radar and lidar observations were combined with passive spectral solar and microwave sensors, including an imaging spectrometer, a fisheye camera, a microwave radiometer, and a Sun photometer. For measurements of turbulent and radiative energy flux densities, a nose boom and broadband solar and terrestrial radiation sensors (pyranometer and pyrgeometer) were installed. Profiles of meteorological parameters were collected by dropsondes. The instrumentation is listed in Table 2.
Table 2Overview of the instrumentation of Polar 5 and 6 and the measured quantities that are part of the database. λ is wavelength, ν is frequency, T is temperature, and p is atmospheric pressure. RH is relative humidity, FOV is field of view, PNSD is the particle number size distribution, rBC is refractory black, and Dp is the particle diameter.
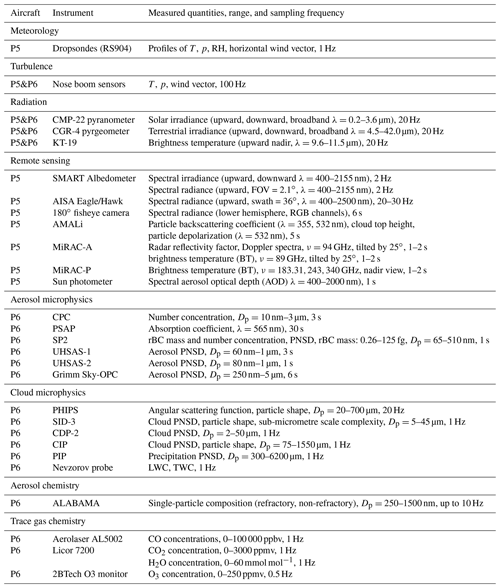
3.1 High-frequency wind vector, air temperature, and humidity
On both aircraft, identical sensors were installed in a nose boom for high-frequency measurements of the wind vector and the air temperature (Hartmann et al., 2018). The basic sensors are an Aventech five-hole probe placed at the tip of the nose boom and an open-wire Pt100 installed sidewards in a Rosemount housing. All data were recorded and published with a frequency of 100 Hz (Hartmann et al., 2019a, https://doi.org/10.1594/PANGAEA.900880). The response time of the sensors is below 0.01 s, well suited for atmospheric turbulence flux measurements (Lee, 1993). The five-hole probe is heated during the flight to prevent icing. It is equipped with a purging system to eject water that might have entered the central hole. Thus, measurements within clouds are reliable.
Pressure measurements in the five-hole probe are recorded by differential pressure transducers of type Setra 239 R for angle of attack, angle of sideslip and the dynamic pressure and by a Setra 278 for the static pressure. To convert the wind vector measured with respect to the aircraft frame into Earth-fixed coordinates, the position, movement, and attitude of the aircraft is measured with a combination of a high-precision global positioning system (GPS) receiver and an inertial navigation system (INS). The INS, a Honeywell Laseref V, provides longitude, latitude, ground speed, and angular rates and calculates the pitch, roll, and true heading angles with an accuracy of 0.1∘ (roll and pitch) and 0.4∘ (true heading). A Novatel GPS FlexPak6 receiver supports the calculation of the position and the velocity vector. Doppler-derived velocities (“Novatel bestvel”) are obtained with a precision of 0.03 m s−1. For the final data product, the INS and GPS data were merged by complementary filtering at a frequency of 0.1 Hz.
The wind vector was calculated by applying the procedure described by Hartmann et al. (2018). The method considers a careful calibration of the initial wind measurements, which is based on a combination of the differential measurement capabilities of the GPS and the high-accuracy INS. With the precise aircraft position and attitude, the horizontal wind components are derived with an absolute accuracy of 0.2 m s−1 for straight and level flight sections. The vertical wind can only be analysed as the deviation from the average vertical wind. To do so, the mean wind vector was averaged for flight sections of at least several kilometres length. For straight and level flight sections, the accuracy of the vertical wind speed relative to the average is about 0.05 m s−1.
The temperature measurements were corrected for the adiabatic heating of the air by the dynamic pressure. The absolute accuracy of the temperature measurements is 0.3 K with a resolution of 0.05 K. The lateral displacement between wind and temperature sensors (radial distance to the centre of the five-hole probe of 16 cm and an axial distance of 35 cm) was found to be not critical. For typical true air speeds of 60 m s−1, this axial distance corresponds to a time lag of about s, which is less than one sample at the recording frequency. Additionally, Polar 5 nose boom carried a closed-path LI-7200 gas analyser for CO2 and H2O concentration measurements. The performance of the analyser with respect to airborne humidity flux measurements has been tested, as described in detail by Lampert et al. (2018). For slow humidity measurements (frequency of 1 Hz), a Vaisala HMT-333, which includes a temperature and HUMICAP humidity sensor, was mounted in a Rosemount housing. Based on the temperature measurements (uncertainty of 0.1 K), the humidity data were corrected for adiabatic heating and reach an accuracy of 0.4 % (Hartmann et al., 2018). These measurements were merged into a reduced 1 Hz basic meteorological data set providing aircraft position, air pressure, temperature, relative humidity, and the horizontal wind vector (Hartmann et al., 2019b, https://doi.org/10.1594/PANGAEA.902849).
The achieved accuracy and temporal resolution of wind and temperature measurements are sufficient to derive turbulent fluxes of momentum and sensible heat in the atmospheric boundary layer with the eddy-covariance method (e.g. Busch, 1973). When using the 100 Hz data delivered to PANGAEA, note that the calibration of the five-hole probe is only valid for straight and level flights. The majority of measurements during ACLOUD were obtained over sea ice in slightly unstable or stable stratification where turbulent heat fluxes are rather small (heat fluxes in the order of a few W m−2). Such low flux conditions represent a challenge to instrumentation and measurement strategy and lead to less relative accuracy compared to turbulent fluxes derived in strong convective condition as, for example, cold air outbreaks.
3.2 Spectral solar radiation
Spectral solar radiation was measured by three different instruments on board Polar 5. The Spectral Modular Airborne Radiation measurement sysTem (SMART Albedometer) primarily measures upward and downward spectral solar irradiances in the wavelength range between 400 and 2155 nm (Wendisch et al., 2001; Ehrlich et al., 2008; Bierwirth et al., 2013). Additionally, upward radiances are obtained for wavelengths below 1000 nm with optical inlets covering a 2.1∘ field of view (FOV). All optical inlets are actively horizontally stabilized to correct for changes of the aircraft attitude of up to 6∘ with an accuracy of 0.2∘ (Wendisch et al., 2001). Two types of grating spectrometers are applied by the SMART Albedometer. At wavelengths below 920 nm, the spectrometers provide a 1 nm sampling resolution (520 spectral pixels) with a spectral resolution of 2–3 nm full width at half maximum (FWHM). Longer wavelengths, 920–2155 nm, 247 spectral pixels, the near-infrared spectrometers sample every 5 nm with a coarser spectral resolution of 12–15 nm. For these near-infrared spectrometers, the raw data were corrected for the dark signal using regular dark measurements with opto-mechanical shutters. The spectrometers measuring below 920 nm wavelength register the dark signal by integrated dark reference pixels. All quantities measured by the SMART Albedometer were merged and published in a combined data set (Jäkel et al., 2019, https://doi.org/10.1594/PANGAEA.899177).
The Airborne Imaging Spectrometer for Applications (AISA) Eagle/Hawk (two pushbroom hyperspectral imaging spectrometers operated in tandem) observes two-dimensional (2-D) fields of upward spectral solar radiance (Schäfer et al., 2013, 2015). Each of the two components consists of a single-line sensor with 1024 (AISA Eagle) and 384 (AISA Hawk) spatial pixels, respectively. The spatial resolution (cross-track pixel sizes) of the AISA Eagle/Hawk measurements is on the order of 4 m for a cloud situated 2 km below the aircraft. For each spatial pixel, the wavelength range of 400–2500 nm is spectrally resolved. The dark signal correction is obtained automatically by an integrated shutter. The measurements of AISA Eagle and AISA Hawk were filtered for straight flight legs and published separately to maintain the full spatial resolution of both sensors (Ruiz-Donoso et al., 2019, https://doi.org/10.1594/PANGAEA.902150).
A digital Canon camera equipped with a downward-looking 180∘ fisheye lens measured the directional distribution of upward radiance of the entire lower hemisphere every 6 s (Ehrlich et al., 2012). A complementary metal oxide semiconductor (CMOS) image sensor covers the three spectral channels (RGB) centred at wavelength of 591 nm (red), 530 nm (green), and 446 nm (blue) with about 80 nm full width at half maximum (FWHM) spectral resolution. The 3908×2600 pixels sensor provides an angular resolution of less than about 0.1∘. Images were recorded in raw data format to gain the full dynamic range (14 bit) of the camera sensor chip. The processing of the raw data was applied without white balance by setting the multipliers of all channels to 1 (Ehrlich et al., 2012). The dark signal of the images was quantified in the laboratory for different camera settings and does not exceed one digital unit of the 15 bit dynamic range. An identical digital camera system was installed on Polar 6. So far, only the measurements on Polar 5 were processed and published (Jäkel and Ehrlich, 2019, https://doi.org/10.1594/PANGAEA.901024).
All three systems were radiometrically, spectrally, and geometrically calibrated in the laboratory. A 1000 W standard calibration lamp (traceable to the standards of the National Institute of Standards and Technology, NIST) was applied for the irradiance measurements of the SMART Albedometer. All radiance measurements were calibrated with the same NIST traceable radiance source (integrating sphere). In-field calibrations with a secondary calibrated integrating sphere were used to track and correct systematic changes of the calibrations, which may appear during the integration on the aircraft.
The total uncertainties of the radiance measurements mostly originate from the radiometric calibration given by the uncertainty of the applied radiation source and the signal-to-noise ratio that differs with wavelength due to the sensitivity of the sensors. Assuming typical measurements above clouds or snow, the uncertainties of upward radiance measured by the SMART Albedometer range between 6 % at wavelengths below 1000 nm and 10 % for longer wavelengths. For the irradiance measurements of the SMART Albedometer, similar uncertainties are given by Bierwirth et al. (2009).
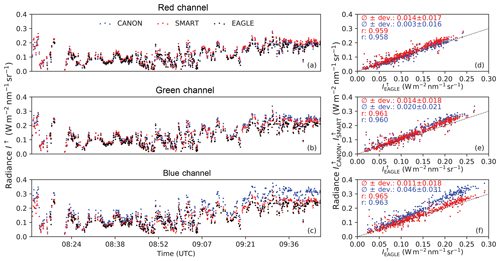
Figure 1Comparison of spectral radiance in the nadir direction I↑ measured by SMART, AISA Eagle/Hawk, and the Canon fisheye camera on 27 May 2017 (flight no. 6). All data are convolved to the three spectral bands of the fisheye camera. Time series for all bands (a) and scatter plots using the radiance of AISA Eagle/Hawk as reference (b) are shown. ∅ gives the mean and “Dev” the standard deviation of the differences between the data sets. r denotes the Pearson's correlation coefficient.
The calibration of all three systems was verified by comparing the upward radiances measured in the nadir direction. The spectrally higher-resolved measurements by the SMART Albedometer and the AISA Eagle/Hawk were convolved to the three spectral bands of the fisheye camera (Ehrlich et al., 2012). Figure 1 shows a time series of the three spectral bands for a 2 h flight section of 27 May 2017 (flight no. 6) and the corresponding scatter plots using AISA Eagle/Hawk as reference. To match the same 2.1∘ nadir spot of the SMART Albedometer, measurements of AISA Eagle/Hawk and the 180∘ fisheye camera were corrected for the aircraft attitude. For AISA Eagle/Hawk the 57 centre pixels were averaged over 10 time steps. For the 180∘ fisheye camera the 2.1∘ nadir spot is covered by 1177 spatial pixels. To avoid systematic effects due to the attitude correction, the comparison is limited to measurements, where the aircraft did not exceed a horizontal misalignment of more than 2∘ in roll or pitch angle. The time series covers clouds of different reflectivity and shows agreement between all three sensors in the observed dynamic range. The time series (Fig. 1a–c) show that all instruments captured the general cloud structure. Differences occur only on small temporal scales, likely due to the slightly different field of view and the different integration times, which range between 500 ms for the SMART Albedometer, 30 ms for AISA Eagle/Hawk, and 0.6 ms for the fisheye camera. The regression of the radiances of the SMART Albedometer and AISA Eagle/Hawk (red dots in Fig. 1d–f) shows an offset in the range of 10 %, which is similar to previous measurement campaigns (Bierwirth et al., 2013; Ehrlich et al., 2012). The red and green channel of the fisheye camera (blue dots in Fig. 1d–f) are comparable to the AISA Eagle/Hawk, while a significant difference of about 35 % on average is observed for the blue channel. This comparison of the three instruments was used to inter-calibrate the fisheye camera in order to provide a consistent data set.
3.3 Broadband solar and terrestrial radiation and surface brightness temperatures
Upward and downward broadband irradiances were measured by pairs of CMP 22 pyranometers and CGR4 pyrgeometers, covering the solar (0.2–3.6 µm) and thermal-infrared (4.5–42 µm) wavelength range, respectively. Both aircraft, Polar 5 and 6, were configured with an identical set of instruments and sampled with a frequency of 20 Hz. In stationary operation, the uncertainty of the sensors is less than 3 %, as characterized by the calibration of the manufacturer and evaluated by, e.g. Gröbner et al. (2014). For the airborne operation of the fixed mounted sensors, the misalignment of the aircraft was corrected by applying the approach by Bannehr and Schwiesow (1993) and Boers et al. (1998). This correction is valid only for the downward direct solar irradiance. Therefore, the relative fractions of direct and diffuse solar radiation were estimated using radiative transfer simulations (cloud free and cloud covered). The simulations were updated continuously based on available in-flight observations and consider the temperature and humidity profiles and the presence or absence of clouds. For the conditions during ACLOUD, a 5 % uncertainty of the simulated fraction of direct radiation amounts to less than 1 % uncertainty of the corrected downward irradiance. The upward solar radiation, as well as the upward and downward terrestrial radiation cannot be corrected for the aircraft attitude. However, these components are characterized by a nearly isotropic radiation field compared to the downward radiation and the effects of a misalignment are minimal for a nearly level sensor (Bucholtz et al., 2008). To limit the remaining uncertainties due to the aircraft movement, measurements with roll and pitch angles exceeding ±4∘ were removed from the data set.
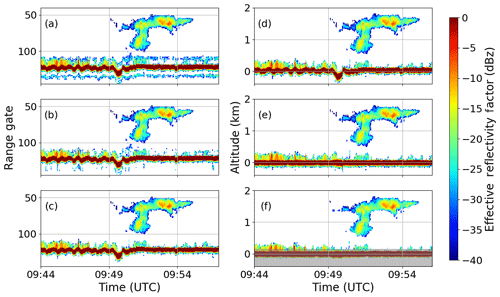
Figure 2Time series of radar reflectivity profiles measured on 25 May 2017 (flight no. 23) for different processing steps: (a) raw data; (b) after subtraction of mirror signal; (c) after speckle filter; (d) filtered data on a time–height grid; (e) corrected for sensor altitude, mounting position, and pitch and roll angle; (f) remapping onto a constant vertical grid. The grey shading indicates the range of surface contamination (≤ 150 m).
To account for the slow response of the pyranometer and pyrgeometer, a correction of the instrument inertia time following the approach by Ehrlich and Wendisch (2015) was applied. Response times of 2 and 6 s (e folding time), characterized in laboratory measurements, were applied for the pyranometer and pyrgeometer measurements. Assuming a typical ground speed of 60 m s−1 and a flight altitude of 100 m, the correction enables us to reconstruct horizontal fluctuations up to scales of 3 m.
During flights inside clouds, icing by super-cooled liquid water droplets might have affected the radiation measurements after ascents and descents through the clouds. Using on-board video camera observations, the data were screened for icing events when the solar downward irradiance appeared artificially reduced. As this detection of icing was not always reliable, uncertainties remain.
Surface brightness temperature was measured by a nadir-looking Kelvin infrared radiation Thermometer (KT-19). These measurements were converted into surface temperature values assuming an emissivity of 1. This is justified due to the small impact of atmospheric absorption in the wavelength range of 9.6 to 11.5 µm for which the KT-19 is sensitive (Hori et al., 2006). With a sampling frequency of 20 Hz, the KT-19 resolves small scales of the surface temperature heterogeneities, such as observed in the case of leads in sea ice (Haggerty et al., 2003). The processed data of the KT-19, pyranometer, and pyrgeometer were merged and published in a combined data set (Stapf et al., 2019, https://doi.org/10.1594/PANGAEA.900442).
3.4 Active and passive microwave remote sensing
The Microwave Radar/radiometer for Arctic Clouds (MiRAC; Mech et al., 2019) has been designed for operation on-board Polar 5. It consists of a single vertically polarized Frequency Modulated Continuous Wave (FMCW) cloud radar RPG-FMCW-94-SP, including a passive channel at 89 GHz (MiRAC-A) and a microwave radiometer (MiRAC-P) with six channels along the strong water vapour absorption line at 183.31 GHz and two window channels at 243 and 340 GHz. MiRAC-A is operated in a belly pod fixed below the aircraft fuselage pointing about 25∘ backwards off nadir, while MiRAC-P is integrated in the cabin-pointing nadir. The cloud radar of MiRAC-A provides vertically resolved profiles of the equivalent radar reflectivity. The vertical resolution depends on the chirp sequences and the temporal resolution, which varied between 1 and 2 s. During ACLOUD, three different settings with resolutions between 4 and 30 m were used. A multi-step processing of the radar data was performed to correct disturbances in radar signal due to the strong surface return and to convert them into geo-referenced data taking the sensor's mounting and the aircraft attitude into account (Mech et al., 2019). Figure 2 illustrates the effect of the processing steps, which finally lead to regularly gridded data, which become reliable 150 m above ground level. The passive channels receive microwave emission from the surface and the atmosphere. The 89 GHz channel is especially sensitive to the surface emission and the emission by liquid clouds. Over the open ocean, where the emissivity of the surface is low, this channel can be used to retrieve the liquid water path. The channels around the 183.31 GHz water vapour absorption line can be used to sense atmospheric moisture. The more the channels are displaced from the absorption line centre, the lower in the atmosphere the emitted radiation originates. The combination of all spectral channels, therefore, provides information about humidity from different layers. With increasing frequency, larger snow particles can lead to a brightness temperature depression due to scattering effects. The processed data of MiRAC-A and MiRAC-P were merged and published in a combined data set (Kliesch and Mech, 2019, https://doi.org/10.1594/PANGAEA.899565).
3.5 Remote sensing by lidar
The active microwave profiling by MiRAC was complemented by the Airborne Mobile Aerosol Lidar (AMALi) system (Stachlewska et al., 2010). This backscatter lidar has three channels: one unpolarized channel in the ultraviolet (UV) at 355 nm and two channels in the visible spectral range at 532 nm (perpendicular and a parallel polarized). The backscattered intensities can be converted into attenuated backscatter coefficients, depolarization ratio at 532 nm, and the colour ratio (532–355 nm) to analyse cloud and aerosol particles.
During ACLOUD, AMALi was installed pointing downwards (except on flight no. 10 where it pointed in the zenith direction) through a floor opening of Polar 5, thus probing the atmosphere between the flight level and the surface. For eye safety reasons, AMALi was operated at flight levels above 2700 m only. Overlap between the transmitted laser beam and the receiving telescope is achieved for ranges larger than 235 m (Stachlewska et al., 2010). Data are recorded with 7.5 m vertical and 1 s temporal resolution. For consistency with the radar profiles, the AMALi data were converted into “altitude above sea level” by using the GPS altitude. To improve the signal-to-noise ratio, the profiles were averaged for 5 s temporal resolution, which yields a horizontal resolution of 375 m for typical aircraft speeds over ground of 270 km h−1.
The data processing eliminated the background signal, which mainly results from scattered sunlight and electronic noise. Additionally, a drift of the so-called base line of each channel was corrected for. Neglecting aerosol extinction, the attenuated backscatter coefficients for each channel were calculated from the background-corrected signals by normalizing the measurements to a typical air density profile (Stachlewska et al., 2005). For the ACLOUD campaign, data from the AWIPEV station in Ny-Ålesund were used (Maturilli, 2017a, b).
The published data set provides cloud top height derived from the preliminary lidar profiles. Clouds below the aircraft were identified from the attenuated backscatter coefficients in the 532 nm parallel channel. Each height bin of the profile, which exceeds the backscatter coefficients of a reference cloud-free section by a factor of 5, was labelled as cloud. Cloud top height was then defined as the highest altitude, which meets the above criterion for consecutive altitude bins. In the published data set (Neuber et al., 2019, https://doi.org/10.1594/PANGAEA.899962), cloud tops close to the aircraft (less than 100 m below the flight level) and low clouds (below 30 m above the ground) are excluded. Profiles of attenuated backscatter coefficients and depolarization ratios are available on request and not yet included in the data set because the processing of the backscatter profiles needs special treatment depending on their specific application (clouds or aerosol).
3.6 Sun photometer
The airborne Sun photometer with an active tracking system (SPTA) was installed under a quartz dome of Polar 5 to derive the spectral aerosol optical depth (AOD). It operates a filter wheel with 10 selected wavelengths in the spectral range from 367 to 1024 nm. To measure the direct solar irradiance, the optics of the SPTA use an aperture with a field of view of 1∘. With knowledge of the extraterrestrial signal the spectral optical depth of the atmosphere as well as spectral optical depth of aerosol was derived. The algorithm applied for the SPTA is based on Herber et al. (2002). The extraterrestrial signal was calculated based on a Langley calibration, which are performed regularly in a high mountain area (Izana, Tenerife). The published data (Herber, 2019, https://doi.org/10.1594/PANGAEA.907097) were screened for contamination by clouds to minimize an artificial enhancement of the AOD by thin clouds. The cloud screening algorithm applied a threshold of measured irradiance and made use of the higher temporal and spatial variability of clouds compared to the rather smooth changes of aerosols properties (Stone et al., 2010).
3.7 Thermodynamic sounding
The Advanced Vertical Atmospheric Profiling System (AVAPS) was operated on Polar 5 to release dropsondes of type RS904 (Ikonen et al., 2010). The sondes measure vertical profiles of air temperature, humidity, pressure, and the horizontal wind vector between the typical flight altitude of 3–4 km and the surface. The vertical resolution of the profiles is about 5 m, determined by the fall velocity of about 10 m s−1 and the sampling frequency of 2 Hz. The Atmospheric Sounding Processing Environment (ASPEN, Version 3.3-543) software package was used to correct the raw data for the slow time response of the temperature sensor and to remove the known humidity bias (Voemel et al., 2016). Data close to the aircraft, where the sensors did not yet adjust to the outside temperature, and invalid measurements were removed by the quality check of ASPEN (configuration set “research dropsonde”). To resolve fast temperature and humidity changes at the cloud top, the time response of the sensors has been corrected by an alternative method following Miloshevich et al. (2004). A time response (e folding) of 4 s was applied to the temperature sensor and 5 s to the humidity sensor. Both data, processed by ASPEN and additionally corrected for the time response using the approach by Miloshevich et al. (2004), are included in the published data set (Ehrlich et al., 2019a, https://doi.org/10.1594/PANGAEA.900204).
Polar 6 was primarily equipped with in situ instruments characterizing aerosol particles, cloud droplets, ice crystals, and trace gases (Table 2). Cloud particles were sampled with five different optical array and scattering probes. Using a counterflow virtual impactor (CVI), the aerosol particles and cloud particle residuals were collected and characterized by the in situ aerosol instrumentation. The trace gas instrumentation measured concentrations of CO, CO2, O3, and water vapour. Meteorological properties, including turbulent and radiative fluxes, were measured with an instrumentation identical to that operated on Polar 5 (see Sect. 3.1).
4.1 Cloud particle in situ measurements
Four wing pylons are available on Polar 6, two on each wing. For ACLOUD five different probes were installed to sample cloud particle microphysical and optical properties: the Cloud Droplet Probe (CDP-2), the Cloud Imaging Probe (CIP), the Precipitation Imaging Probe (PIP), the Small Ice Detector Mark 3 (SID-3), and the Particle Habit Imaging and Polar Scattering probe (PHIPS). Two configurations were applied. The combination of PIP, CIP, SID-3, and PHIPS was operated during the first half of ACLOUD (flight nos. 8–15). In the second half (flight nos. 16–24), the PIP was replaced by the CDP-2 to improve the sampling of small cloud droplets, which dominated the rather warm clouds observed during ACLOUD. Bulk liquid and total water content (LWC, TWC) was measured on Polar 6 with a Nevzorov heated wire probe.
4.1.1 The Cloud Droplet Probe
The Cloud Droplet Probe (CDP-2) is a forward-scattering optical spectrometer (size range 2–50 µm) using a single-mode diode laser at a wavelength of 0.658 µm (Lance et al., 2010; Wendisch et al., 1996). It is operated with anti-shatter tips to reduce possible shattering artefacts (Korolev et al., 2011) and allows for the retrieval of particle by particle information. The instrument counts and sizes individual droplets by detecting pulses of light scattered from a laser beam in the near-forward direction (4–12∘). Sizes are accumulated in 30 bins with variable widths. For ACLOUD, a 1 µm bin width was chosen for small droplet sizes (2–14 µm), while larger cloud droplets (16–50 µm) were collected in 2 µm bins. The particle diameter was deduced from the measurement using a scattering cross section to diameter relationship based on the Mie theory. This relationship is a non-monotonic function, which can give multiple solutions for one scattering cross section measurement. Therefore, the particle number size distribution (PNSD) was obtained in two consecutive steps. First, the CDP-2 raw PNSD was computed by the probe manufacturer software, which applies the first solution of the Mie theory particle size determination. In the second step, raw PNSD has then been corrected using a Monte Carlo inversion method to ensure equiprobable values to all possible solutions of the Mie theory particle size determination. In order to do so, the particle counts (Nraw) from one raw size bin were uniformly distributed into a finer binning (Nfine) for a more precise particle size determination and a scattering cross section was computed for each Nfine. A diameter was then randomly attributed to each count of Nfine using the different solution given by the Mie theory with equiprobability, and these diameters were distributed into the same original size bins (Ncor).
The final calibrated PNSD are obtained by applying the calibrated sampling area and removing shattered particles, which are identified from the inter-arrival times. Prior to its use, the probe has been calibrated using glass beads for sizing and a single-droplet generator (Lance et al., 2010; Wendisch et al., 1996) for the sample area (0.32 mm2). Microphysical quantities such as LWC and effective droplet diameter Deff were derived from the PNSD.
4.1.2 The Cloud Imaging Probe and the Precipitation Imaging Probe
The Cloud Imaging Probe (CIP) and Precipitation Imaging Probe (PIP) measure the size and the shape of cloud particles (Baumgardner et al., 2011). Their measurement principle is based on that of Optical Array Probes (OAPs, Knollenberg, 1976), which use the linear array technique to acquire two-dimensional black and white images of particles. As the particles pass through the laser they cast a shadow, which is recorded on a photodiode array and analysed for particle dimension and shape. According to the resolution of the photodiode and their quantity, the CIP and PIP have nominal size ranges of 25–1550 µm (25 µm resolution and 64 diodes) and 100–6200 µm (100 µm resolution and 64 diodes), respectively. The particle size distribution of hydrometeors are computed from the OAP images. The assessment of the median mass diameter (MMD) and the ice water content (IWC) relies on the definition of the crystal diameter and its mass–diameter relationship. Two mass–diameter relationships were considered in the data set: Baker and Lawson (2006), denoted with BL06, and Brown and Francis (1995), labelled with BF95. Following the approach by Crosier et al. (2011), non-spherical ice crystals were separated from liquid droplets based on their circularity parameter (circularity larger than 1.25 and image area larger than 16 pixels). Only these non-spherical particle images were used for the computation of the “ice” phase. Possible contamination of shattering and splashing of ice and liquid particles on the instruments' tips have been identified and removed using inter-arrival time statistics and image processing (Field et al., 2006). Due to the large OAP measurement uncertainties for the smallest sizes, the first two PNSD size bins were removed. A complete description of the data processing, including a discussion of the applied mass–diameter relationships can be found in Leroy et al. (2016) and Mioche et al. (2017).
In the CDP-2, CIP, and PIP data set published in the PANGAEA database (Dupuy et al., 2019, https://doi.org/10.1594/PANGAEA.899074), the PNSDs of all instruments are stored separately. In order to retrieve the most statistically reliable PNSD, all particle images were used (suffix “ALL”). Truncated images were extrapolated in order to estimate the particle diameter following Korolev et al. (2000). However, the classification of non-spherical particles was based on complete images only (suffix “ALL-IN”). Depending on the application, different definitions of the particle diameters can be applied when calculating the PNSD. This is why three PNSDs are provided, each based on one of three different diameters (Dmax, Deq and Dcc), which are defined as follows.
-
Dmax or length is the maximum dimension originating from the image centre of gravity (see Leroy et al., 2016). It was used in previous studies in the region (Jourdan et al., 2010).
-
Deq or equivalent diameter is the diameter of the circle, which has the same surface as the particle image. Vaillant de Guélis et al. (2019) show that it is the least subjected to error in sizing due to out-of-focus deformation of the image. Also, as it represents a surface, its property is closer to the scattering cross section and thus more comparable to the CDP-2 measurements.
-
Dcc or circumpolar diameter is the diameter of the circle encompassing the particle image. This is the diameter used in the BF95 mass–diameter relationship.
4.1.3 The Small Ice Detector
The Small Ice Detector Mark 3 (SID-3) records the spatial distribution of the forward-scattered light from single cloud particles in the angular region of 5 to 26∘ as 2-D scattering patterns (Hirst et al., 2001). Cloud particles passing a laser beam (wavelength 532 nm) are detected using two nested trigger optics that have circular apertures with a half angle of 9.25∘ located at ±50∘ relative to the forward direction. The maximum camera acquisition rate is 30 Hz, whereas the trigger detector has a maximum acquisition rate of 11 kHz. The trigger signal is recorded as a histogram that can be used to retrieve the cloud particle size distribution using size calibration procedures described in Vochezer et al. (2016). The PNSD covers a size range of 5–45 µm divided into 16 size bins (2–5 µm resolution). From a subsample of the detected particles, a high-resolution 2-D scattering pattern is acquired. These scattering patterns were analysed for the particle shape and sphericity using methods described in Vochezer et al. (2016) or for the particle mesoscopic complexity using the methods described in Schnaiter et al. (2016). The particle shape is given in the form of nine Fourier coefficients yk (k=1…9) derived from the 2-D scattering pattern. Using these coefficients, the particles can be classified as columnar (maxima for y2 or y4) or hexagonal (maxima for y3, y6, or y9). In all other cases the particles are classified as irregular. The particle sphericity is given as a binary information, where all particles having sphericity of 1 are classified as spheres. The particle mesoscopic complexity is expressed with a complexity parameter ke that is an optical parameter varying roughly between 4 to 6. Discussion of the link between the complexity parameter and the actual particle complexity can be found in Schnaiter et al. (2016). The SID-3 data sets available in PANGAEA contain 1 Hz particle PNSD (Schnaiter and Järvinen, 2019a, https://doi.org/10.1594/PANGAEA.900261) and the analysis results of the individual 2-D scattering patterns (Schnaiter and Järvinen, 2019b, https://doi.org/10.1594/PANGAEA.900380). For each detected particle, information about the particle sphericity, shape, and mesoscopic crystal complexity are given.
4.1.4 The Particle Habit Imaging and Polar Scattering probe
The Particle Habit Imaging and Polar Scattering (PHIPS) probe is a combination of a polar nephelometer and a stereoscopic imager (Abdelmonem et al., 2016; Schnaiter et al., 2018) and analyses cloud particles in the size range 20–700 µm. The two parts of the instrument are combined by a trigger detector so that both imaging and scattering measurements are performed on the same single particle. The polar nephelometer has 20 channels from 18 to 170∘, with an angular resolution of 8∘ recording single-particle angular scattering functions. The stereomicroscopic imager consists of two camera and microscope assemblies with an angular viewing distance of 120∘ acquiring a bright field stereomicroscopic image. The magnification of the microscopes can be varied in the range from 1.4× to 9×, which corresponds to field of view dimensions ranging from 6.27×4.72 to 0.98×0.73 mm2, respectively. The optical resolution at the highest magnification setting is about 2.3 µm. During ACLOUD, two different magnifications of 6× and 8× were set for the two PHIPS microscopes of camera 1 and 2, respectively. The purpose of this setting is to capture a detailed view of the particle in camera 2 while ensuring that the same particle was completely captured by camera 1. Particles that were completely captured within the field of view of either camera were analysed for their size, sphericity, and position within the image, as explained in Schön et al. (2011). Furthermore, the images were manually assigned to different shape classes. The PHIPS data set available in PANGAEA contains separate image overviews for both cameras per flight (Schnaiter and Järvinen, 2019c, https://doi.org/10.1594/PANGAEA.902611). Further, it contains single-particle angular light-scattering data for each recorded particle. For a sub-sample of particles, the microphysical information derived from the image analysis was combined in a single ASCII file per flight.
4.1.5 Combined cloud particle number size distributions
When flown together (flight nos. 16–26), CDP-2, SID-3, and CIP data can be combined for merged PNSDs that cover a size range between 2 and 1550 µm. Figure 3 shows PNSD of all instruments averaged over the entire flight of 18 June 2017 (flight no. 20). Only data with liquid water content above 1 mg m−3 were included. For the CDP-2, the uncorrected PNSD produced by the manufacturer software was also included, which shows a significant overestimation of small droplets below 8 µm compared to the corrected version. The PNSD derived from SID-3 measurements agrees well with the CDP-2 and both match the smallest bins of the CIP. For CIP, all three options to calculate the particle diameter are presented. The choice of diameter definition mostly affects ice crystals larger than 200 µm where the equivalent diameter Deq gives the lowest ice crystal concentrations (assuming smaller crystals), and the circumpolar diameter Dcc gives the highest ice crystal concentrations (assuming larger crystals).
4.1.6 Bulk liquid water content
A standard Nevzorov heated wire probe (Korolev et al., 1998) was installed on the nose of Polar 6 to measure bulk liquid and total water content (LWC, TWC). The raw data were averaged over 1 s intervals and processed to compute the liquid water content based on the method described by (Korolev et al., 1998). For both sensors (total and liquid water), the collection efficiency is assumed to be equal to 1. The calculations require the true air speed, which was measured by the five-hole probe installed at the nose boom of Polar 6. Uncertainties of Nevzorov probes have been discussed by, e.g. Wendisch and Brenguier (2013) and Schwarzenboeck et al. (2009). The main uncertainty of the computed LWC and TWC is associated with the estimates of the dry-air output signal, which was determined manually right before and after the in-cloud segments of the flights. During the in-cloud segments, the dry-air signal is unknown and is obtained by linear interpolation of the before- and after-cloud values. The version of the Nevzorov probe installed on Polar 6 during ACLOUD requires manual balancing of the probe, which is done by an human operator during the flight. Some parts of the data could not be recovered when the balancing was not done on time by the operator. For the majority of clouds, the liquid water content values obtained from the LWC sensor of the Nevzorov probe are in close agreement with estimates obtained by integrating the droplet size distribution measured by the CDP-2. The ice water content calculated from the difference of TWC and LWC is highly uncertain in mixed-phase clouds due to the small amount of cloud ice in the majority of clouds observed during the ACLOUD campaign and, therefore, not included in the database (Chechin, 2019, https://doi.org/10.1594/PANGAEA.906658).
4.2 Aerosol particle measurements
Ambient aerosol particles and cloud particle residuals were collected by two inlets on-board Polar 6. Their microphysical and chemical properties were measured inside the cabin by a suite of aerosol sensors (Table 2). A third and fourth inlet provided ambient air for the in-cabin instrumentation of trace gas analysis. The characteristics and the handling of the different inlets is discussed below in Sect. 4.4.
4.2.1 Aerosol particle number concentration and number size distribution
All aerosol particle sizes measured during ACLOUD refer to dry aerosol because most particulate water evaporates in the sampling lines connecting the inlets and the instruments due to the higher temperature inside the aircraft cabin. Two ultra-high sensitivity aerosol spectrometers (UHSAS, Cai et al., 2008) were operated either at different inlets (for simultaneous measurements) or at the same inlet (for inter-comparison). The flow rate was set to 50 mL min−1. The UHSAS measures the number size distribution of particles with diameters between 60 and 1000 nm by detecting scattered laser light divided in 100 user-specified size bins of variable size (2–30 nm resolution). From these measurements, the mean particle diameter and the particle number concentration of a defined size range were derived. From the data evaluation it was inferred that the UHSAS-1 and the UHSAS-2 could reliably detect particles larger than 60 and 80 nm, respectively. During ACLOUD, the UHSAS-1 broke during flight no. 9 (17 June 2017, around 12:00 UTC), i.e. from this moment only the UHSAS-2 could be used for the scientific analysis.
The calibrations of both UHSAS were compared during flights when both instruments were connected to the same particle inlet. Figure 4 shows the total particle concentration (80–1000 nm) and averaged particle number size distributions from flight nos. 7, 14, and 18. The PNSDs of both instruments (Fig. 4b) match in the entire size range for all three flights. For flight nos. 14 and 18, the total particle concentration (Fig. 4a) of UHSAS-1 was found to be about 8 % higher than measured by UHSAS-2, while on flight no. 7 no significant difference could be observed.
In addition to the UHSAS, an optical particle counter (OPC Grimm 1.129) was operated to measure size distribution and number concentration of particles larger than 250 nm in diameter. Due to losses in the aerosol inlet and in the CVI sampling lines, the upper size limit of the OPC was estimated to about 5 µm. A condensation particle counter (CPC TSI-3010 Mertes et al., 1995) measured the total particle number concentration via a light-scattering technique after creating aerosol droplets inside the instrument large enough for detection. This way, number concentration of particles down to diameters of 10 nm and up to 3 µm (limited by the CPC) were measured at a sample flow of 1 L min−1. The measurements of the UHSAS-1, the CPC, and parameters of the CVI operation were merged and published in a combined data set (Mertes et al., 2019, https://doi.org/10.1594/PANGAEA.900403). To provide the full sampling frequencies, the UHSAS-2 data (Zanatta and Herber, 2019a, https://doi.org/10.1594/PANGAEA.900341) and the OPC measurements (Eppers and Schneider, 2019a, https://doi.org/10.1594/PANGAEA.901149) are published separately.
4.2.2 Light-absorbing particles
The absorption coefficient of the sampled particles was measured by a single-wavelength particle soot absorption photometer (PSAP, Bond et al., 1999; Springston, 2016) with a time resolution of 30 s. The PSAP uses the filter-based integrated plate technique in which the change in optical transmission caused by particle deposition is related to the optical absorption coefficient. To calculate the absorption coefficient, the correction given in Bond et al. (1999) Eq. (12) was applied. Only the correction term including the scattering coefficient was neglected because particle scattering was not measured. However, since the filters were changed when the transmittance was still high, the scattering correction is of minor importance. In order to calculate BC mass concentrations, a mass absorption cross section of 10 m2 g−1 was used. Assuming a mass absorption cross section, the absorption coefficient can be transferred into a mass concentration of equivalent black carbon (e.g. Mertes et al., 2004). All measurements of the PSAP are included in the CVI data set (Mertes et al., 2019, https://doi.org/10.1594/PANGAEA.900403).
The single-particle soot photometer (SP2, Stephens et al., 2003) was used to quantify the concentration and size distribution of refractory black carbon (rBC). Briefly, the SP2 is based on the laser-induced incandescence technique that allows for quantifying the mass of refractory BC particles despite the presence of other non-absorbing and non-refractory components. The calibration of the incandescence and scattering signal was performed using size selected fullerene soot particulate (Alfa Aesar, stock no. 40971, no. FS12S011) and polystyrene latex (Thermo Scientific). A complete description of the calibration set-up, standard materials, and operative principles is given by Moteki and Kondo (2010), Gysel et al. (2011), Baumgardner et al. (2012), and Laborde et al. (2012b, a). The number and mass size distribution and the number concentration and mass concentration of rBC particles were obtained for the rBC cores having a mass between 0.40 and 187 fg. The rBC core size is commonly expressed as rBC mass equivalent diameter (DrBC), calculated using a void-free material density of 1800 kg m−3 (Moteki et al., 2010), the resulting diameter detection range was 70–584 nm. Due to a failure of the scattering detector, the quantification of coating thickness was not possible during ACLOUD. The data set published in PANGAEA (Zanatta and Herber, 2019b, https://doi.org/10.1594/PANGAEA.899937) includes only number and mass concentration of rBC. The concentrations were too low to provide meaningful time series of the size distributions. Averaging of at least 3–4 min outside clouds was required but is still not sufficient in cloud measurements. Data can be requested by contacting the corresponding author of the data set.
4.2.3 Chemical particle composition
The aerosol particle and cloud particle residual compositions were measured by the Aircraft-based Laser ABlation Aerosol MAss spectrometer (ALABAMA, Brands et al., 2011; Köllner et al., 2017). In the depressurized part of the instrument, particles are detected by two detection lasers. The time of flight between the two laser beams is used to measure the velocity of the particles and to calculate their vacuum aerodynamic diameter. The detected particles are ablated and ionized by a single laser pulse and the formed ions are analysed by a bipolar time-of-flight mass spectrometer providing information on single-particle chemical composition. The analysed particle mass spectra (mass-to-charge ratio m∕z) were assigned to specific particle types by grouping similar mass spectra to clusters, using known marker ions, and comparing to reference mass spectra. Compared to previous missions, the inlet system of the ALABAMA was modified to extend the upper limit of the sampling range. During ACLOUD, 99 % of the analysed aerosol particles and cloud particle residuals ranged between 250 and 1500 nm. To provide the full ion information for each particle, only spectra with significant positive and negative ion signals were considered. During ACLOUD, 245 427 particles in total were chemically analysed by the ALABAMA (198 256 ambient aerosol particles and 47 171 cloud particle residuals). In a first step, the measured spectra were checked for frequent ion signal peaks and peak combinations. By comparison with known ion marker peaks from the literature (e.g. Köllner et al., 2017; Pratt and Prather, 2010), 11 different species were defined for the database in this study, which are listed in Table 3. Based on these marker peaks, external and internal mixtures of the different species were analysed and grouped into different particle types. The data set published in PANGAEA (Eppers and Schneider, 2019b, https://doi.org/10.1594/PANGAEA.901047) provides the chemical composition (particle species) of each individual particle. If available, the particle size defined by the vacuum aerodynamic diameter was added.
4.3 Trace gas measurements
Carbon monoxide (CO) was measured by the Aerolaser ultra-fast CO monitor model AL5002, which is based on vacuum ultraviolet (VUV) fluorimetry (Gerbig et al., 1999; Scharffe et al., 2012). The sensor makes use of the excitation of CO at 150 nm. UV radiation is emitted by a resonance lamp excited by radio frequency discharge. An optical filter consisting of two CaF2 lenses narrows the wavelength band of the emitted UV radiation to 150 nm. The fluorescence is captured at a right angle by means of a photomultiplier tube (PMT) with Suprasil optics. The instrument was modified to allow in situ calibrations during in-flight operations. During measurement flights of ACLOUD, regular calibrations were performed on 15 to 30 min time intervals using a NIST traceable calibration gas with a known CO concentration at atmospheric levels. Each calibration was followed by a zero measurement. This calibration procedure was used to determine and correct instrumental drifts. The precision of the calculated CO mixing ratio for ACLOUD is 1.5 ppbv. The remaining temporal stability of the CO concentration, which is mainly affected by temperature variations, is estimated with 4 ppbv. These values result in a total uncertainty for CO of 4.5 ppbv for all ACLOUD flights. Due to an instrumental failure on the 25 June 2017 no CO data are available for flight no. 23. For flight no. 24, the CO data are only available in a reduced time resolution.
Concentrations of carbon dioxide (CO2) and water vapour (H2O) were measured by the LI-7200 closed CO2∕H2O analyser from LI-COR Biosciences GmbH (Burba et al., 2010; Lampert et al., 2018). The simultaneous measurement of these two gases accounts for CO2-H2O-interference corrections. Infrared light emitted by an optical source passes a chopper filter wheel and then enters the sample path. Behind the sample path, a temperature-controlled lead selenide detector measures the remaining intensity from which the absorption is derived. The absorption ratio of CO2 and H2O in the sample path was then used to calculate the density and thus the mixing ratio of both gases. The LI-7200 instrument was combined with additional components for flow control and in situ calibrations during in-flight operations. Similar to CO, calibrations were performed in time intervals of 15 to 30 min using a NIST traceable calibration with a known carbon dioxide mixing ratio at atmospheric levels and water vapour close to zero. For ACLOUD, the precision of the instrument is given as 0.05 ppmv for CO2 and 3.7 ppmv for H2O. The temporal stability was calculated from the mean instrumental drift and was estimated with 0.39 ppmv for CO2 and 26.4 ppmv for H2O. Hence, the total uncertainty for CO2 and H2O amounts to 0.40 ppmv and 26.7 ppmv, respectively.
Ozone (O3) was measured by the 2B Technologies Dual Beam Ozone Monitor 205. The measurement principle is based on the attenuation of ultraviolet radiation (254 nm) due to O3 absorption. The UV light passes two separate 15 cm long absorption cells, which are flushed alternately with ozone-filtered and ozone-unfiltered air. The ozone mixing ratios were derived by measurement of the respective intensities. The total uncertainty of the ozone mixing ratios for ACLOUD is determined by instrumental precision and amounts to 1.21 ppbv. The time resolution for the O3 instrument is 0.5 Hz, whereas all other gaseous tracers are measured with 1 Hz resolution. For flight no. 14 on 8 June 2017, ozone data are only available from take-off to 12:58:36 UTC due to a failure of the data acquisition. All trace gas measurements were merged and published in a combined data set (Eppers et al., 2019, https://doi.org/10.1594/PANGAEA.901209).
4.4 Inlets
4.4.1 Counterflow virtual impactor
Cloud particle residues (CPR) are the dry particles that remain after the evaporation or sublimation of cloud droplets or ice particles, respectively. They are closely related to the cloud condensation nuclei (CCN) and ice-nucleating particles (INP) that form the clouds. Thus, their microphysical and chemical characterization provides important information about the aerosol properties, sources, and transportation pathways of atmospheric particles that formed clouds in the atmosphere.
To identify sources and transportation pathways of cloud condensation nuclei (CCN) and ice-nucleating particles (INP), the microphysical and chemical properties of cloud particle residues (CPR) were characterized by the aerosol instrumentation presented in Sect. 4.2. For that purpose, the CPR were sampled and distributed to the individual instruments. During ACLOUD, a counterflow virtual impactor (CVI) was applied, which on the one hand collects exclusively non-precipitating cloud particles (droplets, ice particles) inside the cloud and on the other hand releases their residual particles for aerosol analysis (Ogren et al., 1985; Twohy et al., 2003). The cloud particle collection is achieved by blowing a so-called counterflow out of the CVI inlet tip. As a consequence, interstitial gases are completely deflected from the inlet and smaller interstitial particles that are not activated to cloud droplets or did not nucleate ice particles are considerably decelerated, stopped, and blown out of the inlet. Only larger particles could overcome the counterflow and are sampled by the CVI. During ACLOUD, the clouds were dominated by liquid droplets with a rather low amount of cloud ice, which was almost not detectable by the CVI. Therefore, all sampled CPR can be considered to represent cloud droplet residuals (CDR).
The minimum cloud particle size that is collected by the CVI is determined by the air velocity at the inlet tip (true air speed of Polar 6) and the amount of the counterflow. Due to the rather low air speed of Polar 6, the adjustment of the counterflow to about 2 L min−1 could minimize the lower cut-off diameter to only 8 µm, which is slightly higher than reported in previous operation of the CVI inlet (Schwarzenboeck et al., 2000). Therefore, CDR could not be sampled for the complete cloud droplet population (Mertes et al., 2005). From time to time the counterflow was raised to 12 L min−1 in order to sample only the large hydrometeors in the cloud, which increased the lower cut-off size to 22–24 µm. After collection, the cloud particles are virtually impacted in a sampling line with warm, dry, and particle-free carrier air. The CDR become released and are distributed to the different aerosol sensors via evaporation of the liquid water and/or ice into the gas phase.
To calculate concentrations of the CDR relative to ambient cloud particle concentrations, the enrichment of the CVI needs to be considered. The enrichment factor is specified by the ratio of the air volume flows in front and within the CVI tip and can be expressed by a velocity ratio. The first velocity is identical to the true air speed of the Polar 6 and the second is calculated by the total sample flow. At typical in-cloud sampling conditions when all aerosol sensors were connected to the CVI (except the PSAP) the CVI enrichment was around a factor of 4.5. All particle concentrations measured behind the CVI were corrected by this factor accordingly. This has the positive effect on counting statistics of the connected instruments.
It needs to be considered that the operation of the CVI is designed for particles entering parallel to the inlet. In the case of a significant angle of sideslip (orientation of wind vector with respect to aircraft heading) not all droplets with diameters above the CVI lower cut-off size can be sampled. In that case, many droplets move on particle trajectories that have larger deviation angles with respect to the CVI inlet tip and are thus not collected. The extent of this effect, which was quantified by the aspiration efficiency, was inferred from the size-resolved cloud droplet number concentration measured by the cloud particle probes that are sensitive down to diameters of 5 µm (mainly CDP-2 and SID-3; see Sect. 4.1). For the ACLOUD measurement, the aspiration efficiency was estimated to vary between 0.2 and 0.8. During the first half of ACLOUD (flight no. 7–15), measurements by SID-3 were used to calculate the aspiration efficiency, while in the second half combined measurements by SID-3 and CDP-2 did provide a more accurate estimate. For flight nos. 8 and 10, when neither SID-3 nor CDP-2 were measuring, the aspiration efficiency of the previous flight was applied.
To convert the in-cabin measurements to ambient CDR properties, the CVI sampling efficiency needs to be characterized. It quantifies the ratio between number concentration of the sampled CDR and total number of cloud particles detected by the SID-3 or CDP-2 probe. The sampling efficiency is affected by the aspiration efficiency and depends on the shape of the cloud droplet size distribution, which can change within the cloud profile. In lower cloud levels, which are typically dominated by small cloud droplets (smaller than CVI cut-off diameter), the sampling efficiency is lower than in the upper cloud parts, where most of the cloud particles are larger than the CVI cut-off size. Thus, in the upper cloud layers the sampling efficiency was almost identical to the aspiration efficiency. Assuming that there were no differences between the CDR of sampled droplets and those of droplets larger than the CVI cut-off size, the derived sampling and aspiration efficiency were used to calculate ambient residual mass concentrations.
4.4.2 Aerosol inlet
The standard aerosol inlet on Polar 6 is a stainless steel inlet (Leaitch et al., 2016; Burkart et al., 2017) mounted on the front top of the aircraft, ahead of the engines. The inlet tip is a shrouded diffuser (0.35 cm diameter at intake point). Inside the cabin, the inlet was connected to a 1.9 cm stainless steel manifold of which sample lines were drawn to the various instrument racks using angled inserts. The manifold exhaust flowed freely into the back of the cabin, such that the intake flow varied with aircraft true airspeed. Due to the rather low flight speed, the manifold was not significantly over-pressured. For a true airspeed of 90 m s−1, the total flow at the intake point was approximately 55 L min−1, based on the sum of flows drawn by the instrumentation and bypass (13 L min−1) and the measured exhaust flow into the cabin (42 L min−1). Sampling speed in the inlet tip was approximately isokinetic for the airspeeds during ACLOUD, such that the particle transmission by the inlet was near unity for particles from 20 nm to about 1 µm and fell to 80 % at 5 µm and 30 % at 10 µm. Note that these transmission refers only to the main inlet (the tip and main 19 mm manifold without additional sampling lines) and not to the individual instruments, which have different particle size ranges (see Table 2).
4.4.3 Gas inlet
Two different inlets for trace gases were operated on Polar 6 (Leaitch et al., 2016). CO and O3 were sampled through an inlet designed with a Teflon tube of 0.40 cm outer diameter (OD). The air was passively pushed into the inlet by the aircraft forward motion in combination with a rear-facing exhaust Teflon line (0.95 cm OD) that reduced the line pressure. The sample flow was continuously recorded and remained almost stable at approximately 19 L min−1. For the sampling of CO2 and H2O, a separate gas inlet was used to avoid interaction of water vapour with the walls of the tubing. Therefore, this inlet is made of a stainless steel tube (0.40 cm OD). Similar to the Teflon inlet, the air flow was passively induced by the aircraft motion. For the typical true air speeds of Polar 6 flown during ACLOUD, a continuously flow of approximately 17 L min−1 was obtained.
4.4.4 Operation of CVI and aerosol inlet
The parallel operation of the aerosol and CVI inlet aims to characterize both ambient aerosol particles and CDR. Therefore, most aerosol instruments were connected to both inlets, which allows for switching between the inlets during flight. Table 4 summarized the configuration operated during ACLOUD. Unfortunately, the de-icing of the aerosol inlet did not always work properly. Flying in clouds with super-cooled liquid droplets, the inlet occasionally froze up. During these times, the aerosol inlet was clogged, and ambient aerosol particles were sampled through the CVI inlet operating without counterflow. To avoid the risk of losing data due to icing, the strategy of the inlet operation was changed during the campaign, connecting all instruments permanently through the CVI by switching off the counterflow when Polar 6 was clearly out of clouds (see Table 4).
Table 4Configuration of aerosol instruments and the inlet systems. Flight numbers indicate whether the instrument was switching during a flight between aerosol and CVI inlet or whether it remained connected to the CVI measuring ambient aerosol by switching off the CVI counterflow.

The operation of the CVI is illustrated in Fig. 5 for flight no. 11 (2 June 2017) by the CVI technical parameter (enrichment factor, cut-off diameter, sampling, and aspiration efficiency) and measured particle concentrations. The time series includes two descents (first and third cloud measurements) and one ascent through a cloud layer. In between, four legs in ambient conditions (two above and two below) were flown. The common procedure was to switch on/off the counterflow well before entering a cloud and well after leaving the cloud. The short outside cloud measurements were used to check the correct CVI operation indicated by zero CDR concentration measured behind the CVI (Fig. 5e). As soon as the counterflow was off, the CVI inlet was operated as second aerosol inlet measuring the ambient aerosol particles. In this sampling mode no enrichment exists, but the aspiration and sampling efficiencies are assumed to be 1, which was confirmed by comparison measurements at the standard aerosol inlet. Inside clouds, the CVI enrichment factor (between 4 and 5), the CVI counterflow (around 4 L min−1), and the CVI cut-off diameter (around 11 µm) did not significantly change over the whole flight, except for a short period at about 09:33 UTC. For this leg, the counterflow was substantially increased to obtain a higher cut-off diameter of about 22 µm and analyse larger cloud particles. Consequently, the CDR concentration dropped to almost zero, which indicates that only a small number of large particles were present.
To interpret the CVI sampling and aspiration efficiency, Fig. 5e shows the total cloud droplet concentration measured by SID-3. Additionally, the concentration of cloud droplets larger than the CVI cut-off diameter was calculated from the SID-3 measurements. For the cloud shown here, the cloud top is dominated by large droplets, while at the cloud base small droplets are in majority. Accordingly, the CVI aspiration and sampling efficiency are more or less equal at the cloud top. Towards cloud base, the sampling efficiency becomes smaller, while the aspiration efficiency remains rather constant.
During flight no. 18 (16 June 2017, 08:04 UTC), the CVI inlet heating broke and could not be repaired. In the following flights, this occasionally led the CVI inlet to freeze up when flying inside clouds. However, outside clouds the inlet could always be de-iced so that the majority of CDR measurements and all ambient aerosol particle measurements are valid. Measurements identified to be affected by inlet freezing were removed from the data sets of the connected aerosol instruments.
5.1 Combined Polar 5 and 6 flights
The identical flight performances of Polar 5 and 6 were used to coordinate the flight patterns of both aircraft in a way that measured data can be collocated or merged into a combined data set. Collocated flights aim at combining remote sensing and in situ observations. Similar flight patterns of Polar 5 and 6 at different locations were used to extend the data set of identical instruments installed on both aircraft.
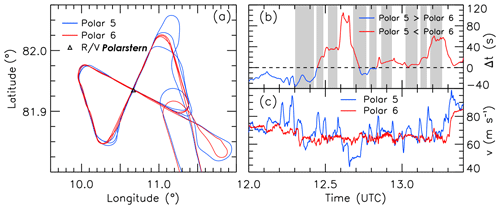
Figure 6Double-triangle flight track of Polar 5 and 6 on 5 June 2017 (flight no. 13) close to R/V Polarstern (a). Panel (b) shows the time difference between both aircraft along the flight path. For positive values (red) Polar 5 was ahead of Polar 6 and vice versa for negative values (blue). Grey-shaded areas indicate the straight flight legs of the double triangle where both aircraft were coordinated. Panel (c) shows the flight velocity of both aircraft.
5.1.1 Collocated remote sensing and in situ observations
Six flights were performed with Polar 5 and 6 flying a closely collocated flight track in different altitudes to characterize clouds (see Table 1). While Polar 5 was maintained at a high flight altitude of about 3000 m for the remote sensing of cloud properties, Polar 6 remained in, below, or a little above the cloud layer, measuring cloud and aerosol particle properties in situ. The close collocation allows for analysing the same clouds with observations from both aircraft. Figure 6 shows an example of a double-triangle flight pattern flown on 5 June 2017 (flight no. 13) close to the research vessel Polarstern. Along the two long straight legs of the double-triangle and the western short leg, both aircraft aimed to be horizontally collocated. To follow the same track with two aircraft is not difficult with modern navigation equipment. The task was to be at the same location within a short time difference to avoid changes of the cloud properties between remote sensing and in situ observations. Therefore, Polar 5 adjusted the flight speed as shown in Fig. 6c and, if needed, extended turns to reduce the distance between both aircraft. Figure 6b shows the time lag between Polar 5 and 6 along the flight track for the entire double-triangle pattern. When values are positive (red) Polar 5 was ahead of Polar 6 and vice versa for negative values (blue). Grey-shaded areas indicate the straight flight legs of the double triangle where both aircraft tracks were coordinated. During these legs, the time difference was mostly below 40 s. Only for the last leg did the separation exceed 50 s.
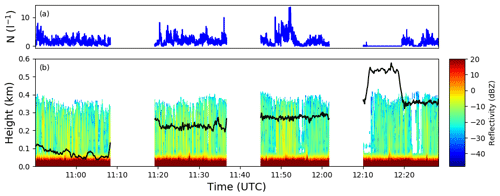
Figure 7Time series of radar reflectivity profiles (b) measured on 2 June 2017 (flight no. 13) during a double-triangle flight pattern (cf. Fig. 6). The flight altitude of Polar 6 operating in the cloud layer is indicated by the black line. Ice crystal number concentration of particle larger than 125 µm measured by the CIP instrument along this flight track are shown in (a). The data gaps result from extended turns of the aircraft when both aircraft were not well collocated, and these data were removed from the comparison.
Figure 7 shows a comparison of collocated remote sensing measurements obtained by MiRAC on Polar 5 and cloud in situ observations by the CIP on Polar 6. The data were obtained during a coordinated double-triangle pattern flown on 2 June 2017 (flight no. 11). Extended turns, when both aircraft were not well collocated, were excluded from the analysis. The radar reflectivity (Fig. 7b) shows a typical structure of Arctic mixed-phase boundary layer clouds with periodically occurring cloud rolls characterized by an enhanced radar reflectivity that is caused by the presence of ice crystals. Within the same cloud, Polar 6 was measuring at different altitudes, indicated by the flight altitude in Fig. 7b (black line). The ice crystal number concentration for particles larger than 125 µm, measured along this flight track by the CIP, is given in Fig. 7a. The concentration significantly varies between 0 and 10 m−3. These temporal (spatial) variations are clearly correlated with the changes of radar reflectivity. For example, the cloud roll structure identified by the radar in the first and second leg (10:50–11:10 and 11:20–11:35 UTC) is captured well by the variation in the ice crystal concentration measured by the CIP. Similarly, enhanced ice crystal concentrations were observed by both instruments for a longer period (larger cloud part) at around 11:50 UTC. These collocated remote sensing and in situ observations are of high value for further analysis aiming at validating the remote sensing methods and characterizing microphysical processes in Arctic mixed-phase clouds.
5.1.2 Series of vertical stacks
The combination of both aircraft allowed flying vertical stacks at a number of different locations along the mean wind direction. At each stack, profiles of mean variables and of turbulent fluxes can be derived. Depending on the structure of the boundary layer, horizontal legs in up to seven altitudes were flown. The typical length of these horizontal sections was at least 10 km, sufficient to apply the eddy covariance method to calculate turbulent fluxes (see Sect. 3.1). As demonstrated by an example of a single flux profile in Wendisch et al. (2019, Fig. 18), the derived profiles are in agreement with theory showing downward heat fluxes in stable environment and upward fluxes in a well-mixed surface forced convective layer. To study the change of flux profiles along the mean flow, series of vertical stacks were flown on 14, 20, and 25 June 2017 (flight nos. 17, 21, and 23). As an example the flight track of 25 June 2017 is illustrated in Fig. 8. Compared to a single-aircraft mission, the number of locations available for analysing flux profiles was increased by a factor of 2 without reducing the length of the horizontal legs or the number of flight levels. The seven locations of the vertical stacks (three to five legs, each 30 km in length) extend over a distance of 170 km with 28 km horizontal separation of the individual profiles. However, for the combined analysis, it needs to be assured that measurements of instruments on both aircraft can be merged into a single data set.
5.2 Merged Polar 5 and Polar 6 data
Data sets of identical instruments operated on both Polar 5 and Polar 6 can be merged to extend the scientific data analysis. To obtain a homogeneous combined data set, the data needs to agree within specific uncertainty ranges. To test the agreement, a coordinated flight with Polar 5 and 6, flying within a close distance of one another (about 100 m), was performed on 9 June 2017 (flight no. 15). The coordinated flight formation was remained for 1 h of flight time including a joint ascent and descent. Between about 1500 and 3100 m altitude, a cloud layer was present. Examples of the wind vector, air temperature, and broadband radiation during the comparison flight are presented in the following.
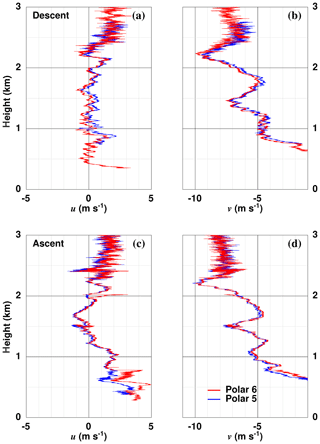
Figure 9Vertical profiles of the horizontal wind components u and v measured by Polar 5 and Polar 6 during the close-formation comparison flight on 9 June 2017. While (a) and (b) show the profiles obtained during a descent, data from the following ascent are given in (c) and (d). The horizontal distance between both aircraft was roughly 100 m, and the vertical distance was typically 10 m.
5.2.1 Horizontal wind vector
The horizontal wind vectors measured by Polar 5 and Polar 6 are shown in Fig. 9. The u and v wind velocity components are presented as vertical profiles separated into measurements during a subsequent ascent (Fig. 9c and d) and descent (Fig. 9a and b). The horizontal distance between both aircraft was roughly 100 m and the vertical distance typically 10 m.
For both wind components, the profiles measured on Polar 5 and 6 are in close agreement within ±1 m s−1 and are both able to reproduce even very small-scale variability down to vertical scales of about 20 m. Only for altitudes below 800 m of the ascent are the differences between the measurements larger, due to a larger vertical separation of both aircraft.
The agreement for both profiles, ascent and descent, indicates that the calibrations of the nose booms properly correct the effects of the dynamic pressure, which typically act differently during ascent and descent. High-frequency variability of the wind vector naturally differs due to the remaining horizontal separation of both aircraft. However, the measurements in the more turbulent cloud layer above 2200 m illustrate that the magnitude of the fluctuations is well captured by both nose booms, which is important for the calculation of turbulent fluxes. A similar quantitative agreement is obtained for the vertical velocity measured by both aircraft (not shown).
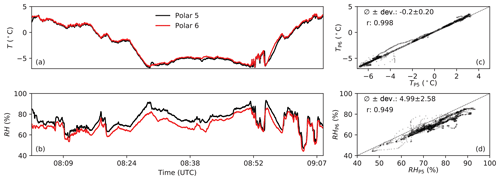
Figure 10Time series of air temperature T (a) and relative humidity RH (b) measured on Polar 5 (P5) and Polar 6 (P6) during a collocated flight section on 9 June 2017 (flight no. 15). Panels (c) and (d) show the scatter plot of Polar 5 versus Polar 6 measurements for both quantities. ∅ gives the mean, and “Dev” gives the standard deviation of the difference of T and RH measured on Polar 5 and 6. r denotes the Pearson's correlation coefficient.
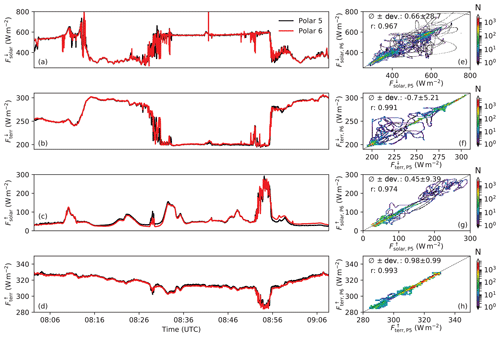
Figure 11Time series of upward and downward solar irradiance (a, c) and upward and downward terrestrial irradiance (b, d) measured on Polar 5 (P5) and Polar 6 (P5) during a collocated flight section on 9 June 2017 (flight no. 15). Panels (e–f) show the scatter plot of Polar 5 versus Polar 6 measurements for all four irradiances. The colour code indicates the number N of data points for each combination of values. ∅ gives the mean, and “Dev” gives the standard deviation of the difference of irradiances measured on Polar 5 and 6. r denotes the Pearson's correlation coefficient.
5.2.2 Air temperature and humidity
Figure 10a and b show time series of air temperature and relative humidity (over water) measured on Polar 5 and Polar 6 during the collocated flight section on 9 June 2017 (flight no. 15). The correlations between the instruments during this section are illustrated in Fig. 11c and d. The flight section includes an ascent and descent and, therefore, covers a significant range of atmospheric conditions with temperatures between −7 and 4 ∘C and relative humidity of 45 %–95 %.
For the entire time series, the Pt100 of Polar 5 shows slightly lower temperatures that are about 0.2 K below the measurements on Polar 6. However, the small-scale variability is reproduced by both aircraft indicated by the Pearson's correlation coefficient r=0.998 close to 1.0. Only in the inversion layer (08:55 UTC), characterized by the fast increase in temperature with height, were larger differences observed. These are likely caused by a slight vertical distance between both aircraft.
The humidity sensors also capture the atmospheric structures in very fine detail (r=0.948). However, a significant bias was observed between Polar 5 and 6 with higher humidity measured by Polar 5. On average, the bias is about 5 % relative humidity, but it obviously changes with time (little differences in the end of the flight section). These differences have to be taken into account when analysing microphysical properties within clouds where small differences of relative humidity may affect the formation of cloud particles. For this purpose, instruments measuring the absolute humidity such as the LiCOR integrated in the nose boom of Polar 5 need to be applied.
Table 6Overview of data availability and quality of all core instruments. The symbol ✓ indicates a complete and valid data set. Partly incomplete or defective data, which allow a limited analysis, are labelled (✓). The symbol ⊗ indicates completely missing data. Empty boxes show flights when the instrument was not operated.
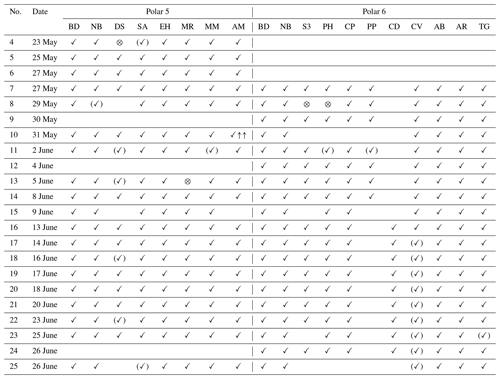
BD: basis data acquisition; NB: nose boom; DS: dropsondes; SA: SMART Albedometer; EH: AISA Eagle/Hawk; MR: MiRAC radar; MM: MiRAC microwave radiometer; AM: AMALi; S3: SID-3; PH: PHIPS; CP: CIP; PP: PIP; CD: CDP-2; CV: CVI; AB: ALABAMA; AR: aerosol rack; TG: trace gases
5.2.3 Broadband radiation
For the coordinated section of flight no. 15 (9 June 2017), Fig. 11a–d shows time series of all four components of the radiative energy budget, upward and downward irradiance for the solar and terrestrial spectral range. The correlations between the Polar 5 and 6 time series are given in Fig. 11e–g. The time series includes periods when stratiform clouds were present above the aircraft (08:14–08:31 UTC and after 08:55 UTC) and conditions with cloud-free sky (08:31–08:55 UTC). Before 08:14 UTC, cirrus was occasionally in front of the Sun. The downward solar and terrestrial irradiance, and agree well for both regimes, low and high agree in cloudy situations, and high and low agree in cloud-free situations. Differences occur when horizontally inhomogeneous clouds were above the aircraft (08:11 and 08:52 UTC), or during the ascent and descent through the mid-level cloud (08:30 and 08:55 UTC). In these cases, the small horizontal displacement of both aircraft is sufficient to measure different parts of the cloud and radiation field and explains the enhanced differences of and in the intermediate range of irradiances between cloud-free and cloudy measurements. However, the mean deviation is below 1 W m−2 for all quantities.
All data listed and described here are published in the World Data Center PANGAEA (Ehrlich et al., 2019b, https://doi.org/10.1594/PANGAEA.902603). Table 5 links each instrument to individual data sets and references. Within PANGAEA, these data are tagged with “ACLOUD” (https://www.pangaea.de/?q=keyword:"ACLOUD", last access: 26 November 2019) and “AC3” (https://www.pangaea.de/?q=project:label:AC3, last access: 26 November 2019) referring to the aircraft campaign and the overarching project (AC)3. Within (AC)3, other accompanying data such as long-term observations in Ny-Ålesund and measurements during the Polarstern cruise PASCAL are published in PANGAEA.
The data availability and quality of each data set are indicated in Table . A checkmark indicates a complete and valid data set. Partly incomplete or defective data that allow a limited analysis are labelled with a parenthesized checkmark. The crossed circle indicates completely missing data. Empty boxes show flights when the instrument was not operated (e.g. flight without clouds). Detailed information about the data quality is given in the metadata of each data set.
The ACLOUD campaign provides a comprehensive in situ and remote sensing observational data set characterizing the Arctic boundary layer and mid-level cloud. All data are published in the PANGAEA database by instrument-separated data subsets. This paper aims to give an overview of the instrument specification, data processing, and data quality. For detailed information, references are provided. It was highlighted how the scientific analysis of the ACLOUD data benefits from the operation of two identical aircraft. True collocated data of in situ and remote sensing observations have the potential to validate remote sensing methods, e.g. identify their sensitivities with respect to ice particles. Merging the data of identical instruments operated on both aircraft extends the spatial coverage of atmospheric quantities and turbulent and radiative energy flux measurements. The different cloud remote sensing techniques operated on Polar 5 can be combined to explore the synergy of multi-instrument cloud retrieval.
A series of ongoing studies have already made use of the ACLOUD data, concentrating on some of the highlights presented by Wendisch et al. (2019). These studies are collected in the inter-journal special issue of Atmospheric Chemistry and Physics and Atmospheric Measurement Techniques, “Arctic Mixed-Phase Clouds as Studied during the ACLOUD/PASCAL Campaigns in the Framework of (AC)3” (https://www.atmos-meas-tech.net/special_issue10_971.html, last access: 26 November 2019). However, the data set has a lot of further potential for detailed studies on cloud–aerosol interaction, satellite remote sensing comparison, validation of cloud resolving numerical models, and more. Further data products that are currently in development will be added to PANGAEA in future and will be linked to the current data set within PANGAEA via the tag “ACLOUD”.
In March and April 2019, most of the ACLOUD instrumentation (remote sensing instruments and part of the in situ cloud probes) was operated on Polar 5 during the Airborne measurements of radiative and turbulent FLUXes of energy and momentum in the Arctic boundary layer (AFLUX) campaign. In early spring and a late summer 2020 it is planned to repeat the coordinated operation of both Polar 5 and 6 using the ACLOUD instrument configuration during the Multidisciplinary drifting Observatory for the Study of Arctic Climate - Airborne observations in the Central Arctic (MOSAiC-ACA) campaign as part of the MOSAiC expedition within the framework of the (AC)3 project. These data will extend the ACLOUD observations in different seasons and in higher latitudes of the central Arctic and, therefore, will allow a statistically solid analysis of atmosphere, cloud, aerosol, trace gas, and sea ice properties.
The main part of the paper was prepared by AE, CL, and MW. Contributions to the section of the individual instruments were provided by CL, JH, and DC (nose boom); SC, LLK, and MM (MiRAC); HB and OE (trace gases); RD, EmJ, OJ, and MS (cloud probes); AH (Sun photometer); EvJ and ERD (spectral solar remote sensing); JSt (broadband radiation); SM, UK, OE, HCC, FK, JSc, and MZ (aerosol instrumentation); and RN (AMALi). MB summarized the data availability in PANGAEA. All authors discussed the results and contributed to the final writing of the paper.
The authors declare that they have no conflict of interest.
We gratefully acknowledge the funding by the Deutsche Forschungsgemeinschaft (DFG, German Research Foundation), project no. 268020496 – TRR 172, within the Transregional Collaborative Research Center: “ArctiC Amplification: Climate Relevant Atmospheric and SurfaCe Processes, and Feedback Mechanisms (AC)3”. The authors are grateful to AWI for providing and operating the two aircraft during the ACLOUD campaign. We thank the crews of the Polar 5 and Polar 6 and their technicians for the excellent technical and logistical support. The generous funding of the flight hours for ACLOUD by AWI is greatly appreciated.
This research has been supported by the Deutsche Forschungsgemeinschaft (grant no. 268020496 – TRR 172). The LaMP acknowledges the support of the Pollution in the Arctic System (PARCS) project funded by the Chantier Arctique of the Centre National de la Recherche Scientifique-Institut National des Sciences de l'Univers (CNRS-INSU).
This paper was edited by Alexander Kokhanovsky and reviewed by Sebastian Schmidt and one anonymous referee.
Abdelmonem, A., Järvinen, E., Duft, D., Hirst, E., Vogt, S., Leisner, T., and Schnaiter, M.: PHIPS–HALO: the airborne Particle Habit Imaging and Polar Scattering probe – Part 1: Design and operation, Atmos. Meas. Tech., 9, 3131–3144, https://doi.org/10.5194/amt-9-3131-2016, 2016. a
Baker, B. and Lawson, R. P.: Improvement in Determination of Ice Water Content from Two-Dimensional Particle Imagery. Part I: Image-to-Mass Relationships, J. Appl. Meteorol., 45, 1282–1290, https://doi.org/10.1175/JAM2398.1, 2006. a
Bannehr, L. and Schwiesow, R.: A Technique to Account for the Misalignment of Pyranometers Installed on Aircraft, J. Atmos. Ocean. Tech., 10, 774–777, https://doi.org/10.1175/1520-0426(1993)010<0774:ATTAFT>2.0.CO;2, 1993. a
Baumgardner, D., Brenguier, J.-L., Bucholtz, A., Coe, H., DeMott, P., Garrett, T., Gayet, J.-F., Hermann, M., Heymsfield, A., Korolev, A., Krämer, M., Petzold, A., Strapp, W., Pilewskie, P., Taylor, J., Twohy, C., and Wendisch, M.: Airborne instruments to measure atmospheric aerosol particles, clouds and radiation: A cook's tour of mature and emerging technology, Atmos. Res., 102, 10–29, https://doi.org/10.1016/j.atmosres.2011.06.021, 2011. a
Baumgardner, D., Popovicheva, O., Allan, J., Bernardoni, V., Cao, J., Cavalli, F., Cozic, J., Diapouli, E., Eleftheriadis, K., Genberg, P. J., Gonzalez, C., Gysel, M., John, A., Kirchstetter, T. W., Kuhlbusch, T. A. J., Laborde, M., Lack, D., Müller, T., Niessner, R., Petzold, A., Piazzalunga, A., Putaud, J. P., Schwarz, J., Sheridan, P., Subramanian, R., Swietlicki, E., Valli, G., Vecchi, R., and Viana, M.: Soot reference materials for instrument calibration and intercomparisons: a workshop summary with recommendations, Atmos. Meas. Tech., 5, 1869–1887, https://doi.org/10.5194/amt-5-1869-2012, 2012. a
Bierwirth, E., Wendisch, M., Ehrlich, A., Heese, B., Tesche, M., Althausen, D., Schladitz, A., Müller, D., Otto, S., Trautmann, T., Dinter, T., Hoyningen-Huene, W. V., and Kahn, R.: Spectral surface albedo over Morocco and its impact on radiative forcing of Saharan dust, Tellus B, 61, 252–269, https://doi.org/10.1111/j.1600-0889.2008.00395.x, 2009. a
Bierwirth, E., Ehrlich, A., Wendisch, M., Gayet, J.-F., Gourbeyre, C., Dupuy, R., Herber, A., Neuber, R., and Lampert, A.: Optical thickness and effective radius of Arctic boundary-layer clouds retrieved from airborne nadir and imaging spectrometry, Atmos. Meas. Tech., 6, 1189–1200, https://doi.org/10.5194/amt-6-1189-2013, 2013. a, b
Boers, R., Mitchell, R., and Krummel, P.: Correction of aircraft pyranometer measurements for diffuse radiance and alignment errors, J. Geophys. Res., 103, 16753–16758, https://doi.org/10.1029/98JD01431, 1998. a
Bond, T., Anderson, T., and Campbell, D.: Calibration and Intercomparison of Filter-Based Measurements of Visible Light Absorption by Aerosols, Aerosol Sci. Technol., 30, 582–600, https://doi.org/10.1080/027868299304435, 1999. a, b
Brands, M., Kamphus, M., Böttger, T., Schneider, J., Drewnick, F., Roth, A., Curtius, J., Voigt, C., Borbon, A., Beekmann, M., Bourdon, A., Perrin, T., and Borrmann, S.: Characterization of a newly developed aircraft–based laser ablation aerosol mass spectrometer (ALABAMA) and first field deployment in urban pollution plumes over Paris during MEGAPOLI 2009, Aerosol Sci. Technol., 45, 46–64, https://doi.org/10.1080/02786826.2010.517813, 2011. a
Brown, P. R. A. and Francis, P. N.: Improved measurements of the ice water content in cirrus using a total–water probe, J. Atmos. Ocean. Tech., 12, 410–414, https://doi.org/10.1175/1520-0426(1995)012<0410:IMOTIW>2.0.CO;2, 1995. a
Bucholtz, A., Bluth, R. T., Kelly, B., Taylor, S., Batson, K., Sarto, A. W., Tooman, T. P., and McCoy, R. F.: The Stabilized Radiometer Platform (STRAP)—An Actively Stabilized Horizontally Level Platform for Improved Aircraft Irradiance Measurements, J. Atmos. Ocean. Tech., 25, 2161–2175, https://doi.org/10.1175/2008JTECHA1085.1, 2008. a
Burba, G. G., McDermitt, D. K., Anderson, D. J., Furtaw, M. D., and Eckles, R.: Novel design of an enclosed CO2/H2O gas analyser for eddy covariance flux measurements, Tellus B, 62, 743–748, https://doi.org/10.1111/j.1600-0889.2010.00468.x, 2010. a
Burkart, J., Hodshire, A. L., Mungall, E. L., Pierce, J. R., Collins, D. B., Ladino, L. A., Lee, A. K. Y., Irish, V., Wentzell, J. J. B., Liggio, J., Papakyriakou, T., Murphy, J., and Abbatt, J.: Organic Condensation and Particle Growth to CCN Sizes in the Summertime Marine Arctic Is Driven by Materials More Semivolatile Than at Continental Sites, Geophys. Res. Lett., 44, 10725–10734, https://doi.org/10.1002/2017GL075671, 2017. a
Busch, N. E.: On the Mechanics of Atmospheric Turbulence, in: Workshop on Micrometeorology, edited by: Hangen, D. A., 1–65, American Meteorological Society, 1973. a
Cai, Y., Montague, D. C., Mooiweer-Bryan, W., and Deshler, T.: Performance characteristics of the ultra high sensitivity aerosol spectrometer for particles between 55 and 800 nm: Laboratory and field studies, J. Aerosol Sci., 39, 759–769, https://doi.org/10.1016/j.jaerosci.2008.04.007, 2008. a
Chechin, D.: Liquid water content measured by the Nevzorov probe during the aircraft ACLOUD campaign in the Arctic, Alfred Wegener Institute, Helmholtz Centre for Polar and Marine Research, Bremerhaven, PANGAEA, https://doi.org/10.1594/PANGAEA.906658, 2019. a, b
Crosier, J., Bower, K. N., Choularton, T. W., Westbrook, C. D., Connolly, P. J., Cui, Z. Q., Crawford, I. P., Capes, G. L., Coe, H., Dorsey, J. R., Williams, P. I., Illingworth, A. J., Gallagher, M. W., and Blyth, A. M.: Observations of ice multiplication in a weakly convective cell embedded in supercooled mid-level stratus, Atmos. Chem. Phys., 11, 257–273, https://doi.org/10.5194/acp-11-257-2011, 2011. a
Dupuy, R., Jourdan, O., Mioche, G., Gourbeyre, C., Leroy, D., and Schwarzenböck, A.: CDP, CIP and PIP In-situ arctic cloud microphysical properties observed during ACLOUD-AC3 campaign in June 2017, LAMP/CNRS/UCA/OPGC, PANGAEA, https://doi.org/10.1594/PANGAEA.899074, 2019. a, b
Ehrlich, A. and Wendisch, M.: Reconstruction of high-resolution time series from slow-response broadband terrestrial irradiance measurements by deconvolution, Atmos. Meas. Tech., 8, 3671–3684, https://doi.org/10.5194/amt-8-3671-2015, 2015. a
Ehrlich, A., Bierwirth, E., Wendisch, M., Gayet, J.-F., Mioche, G., Lampert, A., and Heintzenberg, J.: Cloud phase identification of Arctic boundary-layer clouds from airborne spectral reflection measurements: test of three approaches, Atmos. Chem. Phys., 8, 7493–7505, https://doi.org/10.5194/acp-8-7493-2008, 2008. a
Ehrlich, A., Bierwirth, E., Wendisch, M., Herber, A., and Gayet, J.-F.: Airborne hyperspectral observations of surface and cloud directional reflectivity using a commercial digital camera, Atmos. Chem. Phys., 12, 3493–3510, https://doi.org/10.5194/acp-12-3493-2012, 2012. a, b, c, d
Ehrlich, A., Wendisch, M., Lüpkes, C., Crewell, S., and Mech, M.: Master tracks in different resolutions during POLAR 5 campaign ACLOUD 2017, PANGAEA, https://doi.org/10.1594/PANGAEA.888173, 2018a. a
Ehrlich, A., Wendisch, M., Lüpkes, C., Crewell, S., and Mech, M.: Master tracks in different resolutions during POLAR 6 campaign ACLOUD 2017, PANGAEA, https://doi.org/10.1594/PANGAEA.888365, 2018b. a
Ehrlich, A., Stapf, J., Lüpkes, C., Mech, M., Crewell, S., and Wendisch, M.: Meteorological measurements by dropsondes released from POLAR 5 during ACLOUD 2017, PANGAEA, https://doi.org/10.1594/PANGAEA.900204, 2019a. a, b
Ehrlich, A., Wendisch, M., Lüpkes, C., Buschmann, M., Bozem, H., Chechin, D., Clemen, H.-C., Dupuy, R., Eppers, O., Hartmann, J., Herber, A., Jäkel, E., Järvinen, E., Jourdan, O., Kästner, U., Kliesch, L.-L., Köllner, F., Mech, M., Mertes, S., Neuber, R., Ruiz-Donoso, E., Schnaiter, M., Schneider, J., Stapf, J., and Zanatta, M.: Collection of data sources for the Arctic CLoud Observations Using airborne measurements during polar Day (ACLOUD) campaign, North-West of Svalbard between 23 May–26 June 2017, PANGAEA, https://doi.org/10.1594/PANGAEA.902603, 2019b. a, b
Eppers, O. and Schneider, J.: Airborne in-situ measurement of particle number concentration and size distribution using an optical particle counter during ACLOUD 2017, Institute for Atmospheric Physics, University of Mainz, PANGAEA, https://doi.org/10.1594/PANGAEA.901149, 2019a. a, b
Eppers, O. and Schneider, J.: Aircraft-based measurement of particle size and chemical composition for individual aerosol particles during the ACLOUD campaign 2017, Institute for Atmospheric Physics, University of Mainz, PANGAEA, https://doi.org/10.1594/PANGAEA.901047, 2019b. a, b
Eppers, O., Bozem, H., and Hoor, P.: Airborne in-situ measurement of carbon monoxide, carbon dioxide, water vapor and ozone during ACLOUD 2017, Institute for Atmospheric Physics, University of Mainz, PANGAEA, https://doi.org/10.1594/PANGAEA.901209, 2019. a, b
Field, P. R., Heymsfield, A. J., and Bansemer, A.: Shattering and Particle Interarrival Times Measured by Optical Array Probes in Ice Clouds, J. Atmos. Ocean. Tech., 23, 1357–1371, https://doi.org/10.1175/JTECH1922.1, 2006. a
Gerbig, C., Schmitgen, S., Kley, D., Volz-Thomas, A., Dewey, K., and Haaks, D.: An improved fast–response vacuum–UV resonance fluorescence CO instrument, J. Geophys Res., 104, 1699–1704, https://doi.org/10.1029/1998JD100031, 1999. a
Gröbner, J., Reda, I., Wacker, S., Nyeki, S., Behrens, K., and Gorman, J.: A new absolute reference for atmospheric longwave irradiance measurements with traceability to SI units, J. Geophys. Res., 119, 7083–7090, https://doi.org/10.1002/2014JD021630, 2014. a
Gysel, M., Laborde, M., Olfert, J. S., Subramanian, R., and Gröhn, A. J.: Effective density of Aquadag and fullerene soot black carbon reference materials used for SP2 calibration, Atmos. Meas. Tech., 4, 2851–2858, https://doi.org/10.5194/amt-4-2851-2011, 2011. a
Haggerty, J. A., Maslanik, J. A., and Curry, J. A.: Heterogeneity of sea ice surface temperature at SHEBA from aircraft measurements, J. Geophys. Res., 108, 8052, https://doi.org/10.1029/2000JC000560, 2003. a
Hartmann, J., Gehrmann, M., Kohnert, K., Metzger, S., and Sachs, T.: New calibration procedures for airborne turbulence measurements and accuracy of the methane fluxes during the AirMeth campaigns, Atmos. Meas. Tech., 11, 4567–4581, https://doi.org/10.5194/amt-11-4567-2018, 2018. a, b, c
Hartmann, J., Lüpkes, C., and Chechin, D.: High resolution aircraft measurements of wind and temperature during the ACLOUD campaign in 2017, Alfred Wegener Institute, Helmholtz Centre for Polar and Marine Research, Bremerhaven, PANGAEA, https://doi.org/10.1594/PANGAEA.900880, 2019a. a, b, c
Hartmann, J., Lüpkes, C., and Chechin, D.: 1Hz resolution aircraft measurements of wind and temperature during the ACLOUD campaign in 2017, Alfred Wegener Institute, Helmholtz Centre for Polar and Marine Research, Bremerhaven, PANGAEA, https://doi.org/10.1594/PANGAEA.902849, 2019b. a, b, c
Herber, A.: Aircraft measurements of AOD in the Arctic during the ACLOUD campaign 2017, Alfred Wegener Institute, Helmholtz Centre for Polar and Marine Research, Bremerhaven, PANGAEA, https://doi.org/10.1594/PANGAEA.907097, 2019. a, b
Herber, A., Thomason, L. W., Gernandt, H., Leiterer, U., Nagel, D., Schulz, K.-H., Kaptur, J., Albrecht, T., and Notholt, J.: Continuous day and night aerosol optical depth observations in the Arctic between 1991 and 1999, J. Geophys. Res., 107, AAC 6-1–AAC 6-13, https://doi.org/10.1029/2001JD000536, 2002. a
Hirst, E., Kaye, P. H., Greenaway, R. S., Field, P., and Johnson, D. W.: Discrimination of micrometre-sized ice and super-cooled droplets in mixed-phase cloud, Atmos. Environ., 35, 33–47, https://doi.org/10.1016/S1352-2310(00)00377-0, 2001. a
Hori, M., Aoki, T., Tanikawa, T., Motoyoshi, H., Hachikubo, A., Sugiura, K., Yasunari, T. J., Eide, H., Storvold, R., Nakajima, Y., and Takahashi, F.: In-situ measured spectral directional emissivity of snow and ice in the 8–14 µm atmospheric window, Remote Sens. Environ., 100, 486–502, https://doi.org/10.1016/j.rse.2005.11.001, 2006. a
Ikonen, I., Demetriades, N. W. S., and Holle, R.: Vaisala dropsondes: History, status, and applications, paper presented at 29th Conference on Hurricanes and Tropical Meteorology, SPONSOR, Tucson, Ariz., 10–14 May, 2010. a
Jäkel, E. and Ehrlich, A.: Radiance fields of clouds and the Arctic surface measured by a digital camera during ACLOUD 2017, Institute for Meteorology, Universität Leipzig, PANGAEA, https://doi.org/10.1594/PANGAEA.901024, 2019. a, b
Jäkel, E., Ehrlich, A., Schäfer, M., and Wendisch, M.: Aircraft measurements of spectral solar up- and downward irradiances in the Arctic during the ACLOUD campaign 2017, Universität Leipzig, PANGAEA, https://doi.org/10.1594/PANGAEA.899177, 2019. a, b
Jourdan, O., Mioche, G., Garrett, T. J., Schwarzenböck, A., Vidot, J., Xie, Y., Shcherbakov, V., Yang, P., and Gayet, J.-F.: Coupling of the microphysical and optical properties of an Arctic nimbostratus cloud during the ASTAR 2004 experiment: Implications for light-scattering modeling, J. Geophys. Res., 115, D23206, https://doi.org/10.1029/2010JD014016, 2010. a
Jung, T., Gordon, N. D., Bauer, P., Bromwich, D. H., Chevallier, M., Day, J. J., Dawson, J., Doblas-Reyes, F., Fairall, C., Goessling, H. F., Holland, M., Inoue, J., Iversen, T., Klebe, S., Lemke, P., Losch, M., Makshtas, A., Mills, B., Nurmi, P., Perovich, D., Reid, P., Renfrew, I. A., Smith, G., Svensson, G., Tolstykh, M., and Yang, Q.: Advancing Polar Prediction Capabilities on Daily to Seasonal Time Scales, B. Am. Meteorol. Soc., 97, 1631–1647, https://doi.org/10.1175/BAMS-D-14-00246.1, 2016. a
Kliesch, L.-L. and Mech, M.: Airborne radar reflectivity and brightness temperature measurements with POLAR 5 during ACLOUD in May and June 2017, PANGAEA, https://doi.org/10.1594/PANGAEA.899565, 2019. a, b
Knollenberg, R. G.: Three new instruments for cloud physics measurements, in: Preprints Int. Conf. Cloud Physics, 545–561, Boulder, Amer. Meteor. Soc., 1976. a
Knudsen, E. M., Heinold, B., Dahlke, S., Bozem, H., Crewell, S., Gorodetskaya, I. V., Heygster, G., Kunkel, D., Maturilli, M., Mech, M., Viceto, C., Rinke, A., Schmithüsen, H., Ehrlich, A., Macke, A., Lüpkes, C., and Wendisch, M.: Meteorological conditions during the ACLOUD/PASCAL field campaign near Svalbard in early summer 2017, Atmos. Chem. Phys., 18, 17995–18022, https://doi.org/10.5194/acp-18-17995-2018, 2018. a
Knust, R.: Polar Research and Supply Vessel POLARSTERN Operated by the Alfred-Wegener-Institute, J. Large-Scale Res. Facilities, 3, A119, https://doi.org/10.17815/jlsrf-3-163, 2017. a
Köllner, F., Schneider, J., Willis, M. D., Klimach, T., Helleis, F., Bozem, H., Kunkel, D., Hoor, P., Burkart, J., Leaitch, W. R., Aliabadi, A. A., Abbatt, J. P. D., Herber, A. B., and Borrmann, S.: Particulate trimethylamine in the summertime Canadian high Arctic lower troposphere, Atmos. Chem. Phys., 17, 13747–13766, https://doi.org/10.5194/acp-17-13747-2017, 2017. a, b
Korolev, A., Isaac, G. A., and Hallett, J.: Ice particle habits in stratiform clouds, Q. J. Roy. Meteor. Soc., 126, 2873–2902, https://doi.org/10.1002/qj.49712656913, 2000. a
Korolev, A. V., Strapp, J. W., Isaac, G. A., and Nevzorov, A. N.: The Nevzorov Airborne Hot-Wire LWC–TWC Probe: Principle of Operation and Performance Characteristics, J. Atmos. Ocean. Tech., 15, 1495–1510, https://doi.org/10.1175/1520-0426(1998)015<1495:TNAHWL>2.0.CO;2, 1998. a, b
Korolev, A. V., Emery, E. F., Strapp, J. W., Cober, S. G., Isaac, G. A., Wasey, M., and Marcotte, D.: Small Ice Particles in Tropospheric Clouds: Fact or Artifact? Airborne Icing Instrumentation Evaluation Experiment, B. Am. Meteorol. Soc., 92, 967–973, https://doi.org/10.1175/2010BAMS3141.1, 2011. a
Laborde, M., Mertes, P., Zieger, P., Dommen, J., Baltensperger, U., and Gysel, M.: Sensitivity of the Single Particle Soot Photometer to different black carbon types, Atmos. Meas. Tech., 5, 1031–1043, https://doi.org/10.5194/amt-5-1031-2012, 2012a. a
Laborde, M., Schnaiter, M., Linke, C., Saathoff, H., Naumann, K.-H., Möhler, O., Berlenz, S., Wagner, U., Taylor, J. W., Liu, D., Flynn, M., Allan, J. D., Coe, H., Heimerl, K., Dahlkötter, F., Weinzierl, B., Wollny, A. G., Zanatta, M., Cozic, J., Laj, P., Hitzenberger, R., Schwarz, J. P., and Gysel, M.: Single Particle Soot Photometer intercomparison at the AIDA chamber, Atmos. Meas. Tech., 5, 3077–3097, https://doi.org/10.5194/amt-5-3077-2012, 2012b. a
Lampert, A., Hartmann, J., Pätzold, F., Lobitz, L., Hecker, P., Kohnert, K., Larmanou, E., Serafimovich, A., and Sachs, T.: Comparison of Lyman-alpha and LI-COR infrared hygrometers for airborne measurement of turbulent fluctuations of water vapour, Atmos. Meas. Tech., 11, 2523–2536, https://doi.org/10.5194/amt-11-2523-2018, 2018. a, b
Lance, S., Brock, C. A., Rogers, D., and Gordon, J. A.: Water droplet calibration of the Cloud Droplet Probe (CDP) and in-flight performance in liquid, ice and mixed-phase clouds during ARCPAC, Atmos. Meas. Tech., 3, 1683–1706, https://doi.org/10.5194/amt-3-1683-2010, 2010. a, b
Leaitch, W. R., Korolev, A., Aliabadi, A. A., Burkart, J., Willis, M. D., Abbatt, J. P. D., Bozem, H., Hoor, P., Köllner, F., Schneider, J., Herber, A., Konrad, C., and Brauner, R.: Effects of 20–100 nm particles on liquid clouds in the clean summertime Arctic, Atmos. Chem. Phys., 16, 11107–11124, https://doi.org/10.5194/acp-16-11107-2016, 2016. a, b
Lee, M. F.: Dynamic response of pressure measuring systems, Technical Report 32, Department of Defence, Defence Science and Technology Organisation, Aeronautical Research Laboratory, Melbourne, Vic. Australia, 1993. a
Leroy, D., Fontaine, E., Schwarzenboeck, A., and Strapp, J. W.: Ice Crystal Sizes in High Ice Water Content Clouds. Part I: On the Computation of Median Mass Diameter from In Situ Measurements, J. Atmos. Ocean. Tech., 33, 2461–2476, https://doi.org/10.1175/JTECH-D-15-0151.1, 2016. a, b
Macke, A. and Flores, H.: The expeditions PS106/1 and 2 of the Research Vessel POLARSTERN to the Arctic Ocean in 2017, Reports on Polar and Marine Research, Bremerhaven, Alfred Wegener Institute for Polar and Marine Research, 719, 171 pp., ISSN 1866–3192, https://doi.org/10.2312/BzPM_0719_2018, 2018. a
Maturilli, M.: High resolution radiosonde measurements from station Ny-Ålesund (2017-06), PANGAEA, https://doi.org/10.1594/PANGAEA.879822, 2017a. a
Maturilli, M.: High resolution radiosonde measurements from station Ny-Ålesund (2017-05), PANGAEA, https://doi.org/10.1594/PANGAEA.879820, 2017b. a
Mech, M., Kliesch, L.-L., Anhäuser, A., Rose, T., Kollias, P., and Crewell, S.: Microwave Radar/radiometer for Arctic Clouds (MiRAC): first insights from the ACLOUD campaign, Atmos. Meas. Tech., 12, 5019–5037, https://doi.org/10.5194/amt-12-5019-2019, 2019. a, b
Mertes, S., Schröder, F., and Wiedensohler, A.: The Particle-detection Efficiency Curve of the Tsi-3010 Cpc As A Function of the Temperature Difference Between Saturator and Condenser, Aerosol. Sci. Technol., 23, 257–261, https://doi.org/10.1080/02786829508965310, 1995. a
Mertes, S., Dippel, B., and Schwarzenböck, A.: Quantification of graphitic carbon in atmospheric aerosol particles by Raman spectroscopy and first application for the determination of mass absorption efficiencies, J. Aerosol Sci., 35, 347–361, https://doi.org/10.1016/j.jaerosci.2003.10.002, 2004. a
Mertes, S., Lehmann, K., Nowak, A., Massling, A., and Wiedensohler, A.: Link between aerosol hygroscopic growth and droplet activation observed for hill-capped clouds at connected flow conditions during FEBUKO, Atmos. Environ., 39, 4247–4256, https://doi.org/10.1016/j.atmosenv.2005.02.010, 2005. a
Mertes, S., Kästner, U., and Macke, A.: Airborne in-situ measurements of the aerosol absorption coefficient, aerosol particle number concentration and size distribution of cloud particle residuals and ambient aerosol particles during the ACLOUD campaign in May and June 2017, Leibniz-Institut für Troposphärenforschung e.V., Leipzig, PANGAEA, https://doi.org/10.1594/PANGAEA.900403, 2019. a, b, c
Miloshevich, L., Paukkunen, A., Vomel, H., and Oltmans, S.: Development and validation of a time-lag correction for Vaisala radiosonde humidity measurements, J. Atmos. Ocean. Tech., 21, 1305–1327, https://doi.org/10.1175/1520-0426(2004)021<1305:DAVOAT>2.0.CO;2, 2004. a, b
Mioche, G., Jourdan, O., Delanoë, J., Gourbeyre, C., Febvre, G., Dupuy, R., Monier, M., Szczap, F., Schwarzenboeck, A., and Gayet, J.-F.: Vertical distribution of microphysical properties of Arctic springtime low-level mixed-phase clouds over the Greenland and Norwegian seas, Atmos. Chem. Phys., 17, 12845–12869, https://doi.org/10.5194/acp-17-12845-2017, 2017. a
Moteki, N. and Kondo, Y.: Dependence of Laser-Induced Incandescence on Physical Properties of Black Carbon Aerosols: Measurements and Theoretical Interpretation, Aerosol. Sci. Technol., 44, 663–675, https://doi.org/10.1080/02786826.2010.484450, 2010. a
Moteki, N., Kondo, Y., and Nakamura, S.: Method to measure refractive indices of small nonspherical particles: Application to black carbon particles, J. Aerosol Sci., 41, 513–521, https://doi.org/10.1016/j.jaerosci.2010.02.013, 2010. a
Neuber, R.: A multi-disciplinary Arctic research facility: From the Koldewey–Rabot–Corbel–Stations to the AWI-IPEV research base on Spitsbergen, Polarforschung, 23, 117–123, 2006. a
Neuber, R., Schmidt, L. V., Ritter, C., and Mech, M.: Cloud top altitudes observed with airborne lidar during the ACLOUD campaign, Alfred Wegener Institute – Research Unit Potsdam, PANGAEA, https://doi.org/10.1594/PANGAEA.899962, 2019. a, b
Ogren, J., Heintzenberg, J., and Charlson, R.: In situ sampling of clouds with a droplet to aerosol converter, Geophys. Res. Lett., 12, 121–124, https://doi.org/10.1029/GL012i003p00121, 1985. a
Pratt, K. A. and Prather, K. A.: Aircraft measurements of vertical profiles of aerosol mixing states, J. Geophys. Res., 115, D11305, https://doi.org/10.1029/2009JD013150, 2010. a
Ruiz-Donoso, E., Ehrlich, A., Schäfer, M., Jäkel, E., and Wendisch, M.: Spectral solar cloud top radiance measured by airborne spectral imaging during the ACLOUD campaign in 2017, Leipzig Institute for Meteorology, University of Leipzig, PANGAEA, https://doi.org/10.1594/PANGAEA.900880, 2019. a, b
Schäfer, M., Bierwirth, E., Ehrlich, A., Heyner, F., and Wendisch, M.: Retrieval of cirrus optical thickness and assessment of ice crystal shape from ground-based imaging spectrometry, Atmos. Meas. Tech., 6, 1855–1868, https://doi.org/10.5194/amt-6-1855-2013, 2013. a
Schäfer, M., Bierwirth, E., Ehrlich, A., Jäkel, E., and Wendisch, M.: Airborne observations and simulations of three-dimensional radiative interactions between Arctic boundary layer clouds and ice floes, Atmos. Chem. Phys., 15, 8147–8163, https://doi.org/10.5194/acp-15-8147-2015, 2015. a
Scharffe, D., Slemr, F., Brenninkmeijer, C. A. M., and Zahn, A.: Carbon monoxide measurements onboard the CARIBIC passenger aircraft using UV resonance fluorescence, Atmos. Meas. Tech., 5, 1753–1760, https://doi.org/10.5194/amt-5-1753-2012, 2012. a
Schnaiter, M. and Järvinen, E.: SID-3 1Hz size distribution of cloud particles during the ACLOUD campaign in 2017, Karlsruher Institut für Technologie, Institut für Meteorologie und Klimaforschung, Karlsruhe, PANGAEA, https://doi.org/10.1594/PANGAEA.900261, 2019a. a, b
Schnaiter, M. and Järvinen, E.: SID-3 analysis results for 2D scattering patterns during the ACLOUD campaign in 2017, Karlsruher Institut für Technologie, Institut für Meteorologie und Klimaforschung, Karlsruhe, PANGAEA, https://doi.org/10.1594/PANGAEA.900380, 2019b. a, b
Schnaiter, M. and Järvinen, E.: PHIPS particle-by-particle data for the ACLOUD campaign in 2017, Karlsruher Institut für Technologie, Institut für Meteorologie und Klimaforschung, Karlsruhe, PANGAEA, https://doi.org/10.1594/PANGAEA.902611, 2019c. a, b
Schnaiter, M., Järvinen, E., Vochezer, P., Abdelmonem, A., Wagner, R., Jourdan, O., Mioche, G., Shcherbakov, V. N., Schmitt, C. G., Tricoli, U., Ulanowski, Z., and Heymsfield, A. J.: Cloud chamber experiments on the origin of ice crystal complexity in cirrus clouds, Atmos. Chem. Phys., 16, 5091–5110, https://doi.org/10.5194/acp-16-5091-2016, 2016. a, b
Schnaiter, M., Järvinen, E., Abdelmonem, A., and Leisner, T.: PHIPS-HALO: the airborne particle habit imaging and polar scattering probe – Part 2: Characterization and first results, Atmos. Meas. Tech., 11, 341–357, https://doi.org/10.5194/amt-11-341-2018, 2018. a
Schön, R., Schnaiter, M., Ulanowski, Z., Schmitt, C., Benz, S., Möhler, O., Vogt, S., Wagner, R., and Schurath, U.: Particle Habit Imaging Using Incoherent Light: A First Step toward a Novel Instrument for Cloud Microphysics, J. Atmos. Ocean. Tech., 28, 493–512, https://doi.org/10.1175/2011JTECHA1445.1, 2011. a
Schwarzenboeck, A., Heintzenberg, J., and Mertes, S.: Incorporation of aerosol particles between 25 and 850 nm into cloud elements: measurements with a new complementary sampling system, Atmos. Res., 52, 241–260, https://doi.org/10.1016/S0169-8095(99)00034-4, 2000. a
Schwarzenboeck, A., Mioche, G., Armetta, A., Herber, A., and Gayet, J.-F.: Response of the Nevzorov hot wire probe in clouds dominated by droplet conditions in the drizzle size range, Atmos. Meas. Tech., 2, 779–788, https://doi.org/10.5194/amt-2-779-2009, 2009. a
Serreze, M. C. and Barry, R. G.: Processes and impacts of Arctic amplification: A research synthesis, Glob. Planet. Change, 77, 85–96, https://doi.org/10.1016/j.gloplacha.2011.03.004, 2011. a
Springston, S. R.: Radiance Research Particle Soot/Absorption Photometer Instrument Handbook, United States, DOE/SC-ARM-TR-176, https://doi.org/10.2172/1246162, 2016. a
Stachlewska, I. S., Ritter, C., and Neuber, R.: Application of the two-stream inversion algorithm for retrieval of extinction, backscatter, and lidar ratio for clean and polluted Arctic air, Proc. SPIE, 5984, https://doi.org/10.1117/12.629317, 2005. a
Stachlewska, I. S., Neuber, R., Lampert, A., Ritter, C., and Wehrle, G.: AMALi – the Airborne Mobile Aerosol Lidar for Arctic research, Atmos. Chem. Phys., 10, 2947–2963, https://doi.org/10.5194/acp-10-2947-2010, 2010. a, b
Stapf, J., Ehrlich, A., Jäkel, E., and Wendisch, M.: Aircraft measurements of broadband irradiance during the ACLOUD campaign in 2017, PANGAEA, https://doi.org/10.1594/PANGAEA.900442, 2019. a, b, c
Stephens, G., Winker, D., Pelon, J., Trepte, C., Vane, D., Yuhas, C., L’Ecuyer, T., and Lebsock, M.: CloudSat and CALIPSO within the A-Train: Ten Years of Actively Observing the Earth System, B. Am. Meteorol. Soc., 99, 569–581, https://doi.org/10.1175/BAMS-D-16-0324.1, 2018. a
Stephens, M., Turner, N., and Sandberg, J.: Particle identification by laser-induced incandescence in a solid-state laser cavity, Appl. Opt., 42, 3726–3736, https://doi.org/10.1364/AO.42.003726, 2003. a
Stone, R. S., Herber, A., Vitale, V., Mazzola, M., Lupi, A., Schnell, R. C., Dutton, E. G., Liu, P. S. K., Li, S.-M., Dethloff, K., Lampert, A., Ritter, C., Stock, M., Neuber, R., and Maturilli, M.: A three-dimensional characterization of Arctic aerosols from airborne Sun photometer observations: PAM-ARCMIP, April 2009, J. Geophys. Res., 115, D13203, https://doi.org/10.1029/2009JD013605, 2010. a
Twohy, C. H., Strapp, J. W., and Wendisch, M.: Performance of a Counterflow Virtual Impactor in the NASA Icing Research Tunnel, J. Atmos. Ocean. Tech., 20, 781–790, https://doi.org/10.1175/1520-0426(2003)020<0781:POACVI>2.0.CO;2, 2003. a
Uttal, T., Starkweather, S., Drummond, J. R., Vihma, T., Makshtas, A. P., Darby, L. S., Burkhart, J. F., Cox, C. J., Schmeisser, L. N., Haiden, T., Maturilli, M., Shupe, M. D., De Boer, G., Saha, A., Grachev, A. A., Crepinsek, S. M., Bruhwiler, L., Goodison, B., McArthur, B., Walden, V. P., Dlugokencky, E. J., Persson, P. O. G., Lesins, G., Laurila, T., Ogren, J. A., Stone, R., Long, C. N., Sharma, S., Massling, A., Turner, D. D., Stanitski, D. M., Asmi, E., Aurela, M., Skov, H., Eleftheriadis, K., Virkkula, A., Platt, A., Førland, E. J., Iijima, Y., Nielsen, I. E., Bergin, M. H., Candlish, L., Zimov, N. S., Zimov, S. A., O’Neill, N. T., Fogal, P. F., Kivi, R., Konopleva-Akish, E. A., Verlinde, J., Kustov, V. Y., Vasel, B., Ivakhov, V. M., Viisanen, Y., and Intrieri, J. M.: International Arctic Systems for Observing the Atmosphere: An International Polar Year Legacy Consortium, B. Am. Meteorol. Soc., 97, 1033–1056, https://doi.org/10.1175/BAMS-D-14-00145.1, 2016. a
Vaillant de Guélis, T., Schwarzenböck, A., Shcherbakov, V., Gourbeyre, C., Laurent, B., Dupuy, R., Coutris, P., and Duroure, C.: Study of the diffraction pattern of cloud particles and the respective responses of optical array probes, Atmos. Meas. Tech., 12, 2513–2529, https://doi.org/10.5194/amt-12-2513-2019, 2019. a
Vochezer, P., Järvinen, E., Wagner, R., Kupiszewski, P., Leisner, T., and Schnaiter, M.: In situ characterization of mixed phase clouds using the Small Ice Detector and the Particle Phase Discriminator, Atmos. Meas. Tech., 9, 159–177, https://doi.org/10.5194/amt-9-159-2016, 2016. a, b
Voemel, H., Young, K., and Hock, T. F.: NCAR GPS Dropsonde Humidity Dry Bias, NCAR Technical Note NCAR/TN-531+STR, 8 pp., https://doi.org/10.5065/D6XS5SGX, 2016. a
Wendisch, M. and Brenguier, J.-L.: Airborne Measurements for Environmental Research – Methods and Instruments, Wiley–VCH Verlag GmbH & Co. KGaA, Weinheim, Germany, Weinheim, Germany, ISBN: 978-3-527-40996-9, 2013. a, b
Wendisch, M., Keil, A., and Korolev, A.: FSSP characterization with monodisperse water droplets, J. Atmos. Ocean. Tech., 13, 1152–1165, https://doi.org/10.1175/1520-0426(1996)013<1152:FCWMWD>2.0.CO;2, 1996. a, b
Wendisch, M., Müller, D., Schell, D., and Heintzenberg, J.: An airborne spectral albedometer with active horizontal stabilization, J. Atmos. Ocean. Tech., 18, 1856–1866, https://doi.org/10.1175/1520-0426(2001)018<1856:AASAWA>2.0.CO;2, 2001. a, b
Wendisch, M., Brückner, M., Burrows, J. P., Crewell, S., Dethloff, K., Ebell, K., Lüpkes, C., Macke, A., Notholt, J., Quaas, J., Rinke, A., , and Tegen, I.: ArctiC Amplification: Climate Relevant Atmospheric and SurfaCe Processes, and Feedback Mechanisms: (AC)3, Eos, Trans. Amer. Geophys. Union, 98, https://doi.org/10.1029/2017EO064803, 2017. a
Wendisch, M., Macke, A., Ehrlich, A., Lüpkes, C., Mech, M., Chechin, D., Dethloff, K., Velasco, C. B., Bozem, H., Brückner, M., Clemen, H.-C., Crewell, S., Donth, T., Dupuy, R., Ebell, K., Egerer, U., Engelmann, R., Engler, C., Eppers, O., Gehrmann, M., Gong, X., Gottschalk, M., Gourbeyre, C., Griesche, H., Hartmann, J., Hartmann, M., Heinold, B., Herber, A., Herrmann, H., Heygster, G., Hoor, P., Jafariserajehlou, S., Jäkel, E., Järvinen, E., Jourdan, O., Kästner, U., Kecorius, S., Knudsen, E. M., Köllner, F., Kretzschmar, J., Lelli, L., Leroy, D., Maturilli, M., Mei, L., Mertes, S., Mioche, G., Neuber, R., Nicolaus, M., Nomokonova, T., Notholt, J., Palm, M., van Pinxteren, M., Quaas, J., Richter, P., Ruiz-Donoso, E., Schäfer, M., Schmieder, K., Schnaiter, M., Schneider, J., Schwarzenböck, A., Seifert, P., Shupe, M. D., Siebert, H., Spreen, G., Stapf, J., Stratmann, F., Vogl, T., Welti, A., Wex, H., Wiedensohler, A., Zanatta, M., and Zeppenfeld, S.: The Arctic Cloud Puzzle: Using ACLOUD/PASCAL Multiplatform Observations to Unravel the Role of Clouds and Aerosol Particles in Arctic Amplification, B. Am. Meteorol. Soc., 100, 841–871, https://doi.org/10.1175/BAMS-D-18-0072.1, 2019. a, b, c, d, e, f
Wesche, C., Steinhage, D., and Nixdorf, U.: Polar aircraft Polar5 and Polar6 operated by the Alfred Wegener Institute, J. Large-Scale Res. Facilities, 2, A87, https://doi.org/10.17815/jlsrf-2-153, 2016. a
Zanatta, M. and Herber, A.: Aircraft measurements of aerosol size distribution in the Arctic during the ACLOUD campaign 2017, Alfred Wegener Institute, Helmholtz Centre for Polar and Marine Research, Bremerhaven, PANGAEA, https://doi.org/10.1594/PANGAEA.900341, 2019a. a, b
Zanatta, M. and Herber, A.: Aircraft measurements of refractory black carbon in the Arctic during the ACLOUD campaign 2017, Alfred Wegener Institute, Helmholtz Centre for Polar and Marine Research, Bremerhaven, PANGAEA, https://doi.org/10.1594/PANGAEA.899937, 2019b. a, b
- Abstract
- Introduction
- Scientific targets of the research flights
- Instrumentation on Polar 5
- Instrumentation of Polar 6
- Coordinated flights and intercomparison
- Data availability
- Conclusions
- Author contributions
- Competing interests
- Acknowledgements
- Financial support
- Review statement
- References
- Supplement
- Abstract
- Introduction
- Scientific targets of the research flights
- Instrumentation on Polar 5
- Instrumentation of Polar 6
- Coordinated flights and intercomparison
- Data availability
- Conclusions
- Author contributions
- Competing interests
- Acknowledgements
- Financial support
- Review statement
- References
- Supplement