the Creative Commons Attribution 4.0 License.
the Creative Commons Attribution 4.0 License.
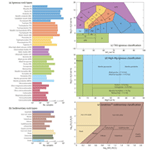
Global whole-rock geochemical database compilation
Matthew Gard
Derrick Hasterok
Jacqueline A. Halpin
Collation and dissemination of geochemical data are critical to promote rapid, creative, and accurate research and place new results in an appropriate global context. To this end, we have compiled a global whole-rock geochemical database, sourced from various existing databases and supplemented with an extensive list of individual publications. Currently the database stands at 1 022 092 samples with varying amounts of associated sample data, including major and trace element concentrations, isotopic ratios, and location information. Spatial and temporal distribution is heterogeneous; however, temporal distributions are enhanced over some previous database compilations, particularly in ages older than ∼ 1000 Ma. Also included are a range of geochemical indices, various naming schema, and physical property estimates computed on a major element normalized version of the geochemical data for quick reference. This compilation will be useful for geochemical studies requiring extensive data sets, in particular those wishing to investigate secular temporal trends. The addition of physical properties, estimated from sample chemistry, represents a unique contribution to otherwise similar geochemical databases. The data are published in .csv format for the purposes of simple distribution, but exist in a structure format acceptable for database management systems (e.g. SQL). One can either manipulate these data using conventional analysis tools such as MATLAB®, Microsoft® Excel, or R, or upload them to a relational database management system for easy querying and management of the data as unique keys already exist. The data set will continue to grow and be improved, and we encourage readers to contact us or other database compilations within about any data that are yet to be included. The data files described in this paper are available at https://doi.org/10.5281/zenodo.2592822 (Gard et al., 2019a).
- Article
(2434 KB) - Full-text XML
- BibTeX
- EndNote
Geochemical analyses in conjunction with other temporal, spatial, and physical property information have been vital sources of information for understanding the Earth and investigating both local and global geodynamic histories (e.g. Keller and Schoene, 2018). Effective collection, collation, and dissemination of this type of data are critical to promote rapid, creative and accurate research. Every year, the amount of data recorded globally increases, dispersed among many hundreds of individual publications. Since the 1960s and 1970s, broad element suites have been promptly accumulated due to the commercial availability of methods such as X-ray fluorescence (XRF) and inductively coupled plasma mass spectrometry (ICP-MS), and thus modern publications are swiftly expanding our cumulative global data records. However, due to the rate of new publications in conjunction with significant partitioning between different journals, these data are not always easy to find and can be incredibly time-consuming to collate. It is pertinent that this information be readily available for future studies, as all benefit from taking advantage of the full suite of data available to produce more robust models and constrained analyses.
Geochemical compilations have been used in a range of studies, from examining crustal magma reservoirs (e.g. Carbotte et al., 2013), proposing changes in mantle dynamics (e.g. Iwamori and Nakamura, 2015), looking at regional and global tectonic histories (e.g. Keller and Schoene, 2018), to examining the connections between life and the solid Earth (e.g. Cox et al., 2018). This information has implications not only for the scientific community, but also for issues such as environmental management, land use, and mineral resource development.
In this paper we present a global whole-rock geochemical database compilation consisting of modified whole-rock subsets from existing database compilations, in conjunction with significant supplementation from individual publications not yet included in these other collections. Additionally, we have generated naming schema, various geochemical indices, and other physical property estimates, including density, seismic velocity, and heat production for a range of the data contained within.
Many existing initiatives have worked to construct and maintain database compilations with great success, but often restrict themselves to certain tectonic environments or regimes, regions, or rock types. EarthChem (https://www.earthchem.org, last access: 25 March 2017) is currently the most notable general use geochemical data repository. It consists of many federated databases such as NAVDAT, PetDB, GEOROC, SedDB, MetPetDB, and the USGS National Geochemical Database, as well as other individually submitted publications. The constituent databases are mostly more specialized compilations, for example the following:
-
The North American Volcanic and Intrusive Rock Database (NAVDAT) has existed since 2002 and is primarily aimed at geochemical and isotopic data from Mesozoic and younger igneous samples of western North America (Walker et al., 2006) (http://www.navdat.org/, last access: 9 October 2019).
-
The Petrological Database of the Ocean Floor (PetDB) is the premier geochemical compilation suite for the igneous and metamorphic hosted data from mid-ocean ridges, back-arc basins, sea mounts, oceanic crust, and ophiolites (https://www.earthchem.org/petdb, last access: 9 October 2019).
-
Geochemistry of Rocks of the Oceans and Continents (GEOROC) is a more holistic compilation effort of chemical, isotope, and other data for igneous samples, including whole-rock, glass, minerals and inclusion analyses and metadata (http://georoc.mpch-mainz.gwdg.de, last access: 9 October 2019).
-
SedDB focuses on sedimentary samples, primarily from marine sediment cores. It has been static since 2014 and includes information such as major and trace element concentrations, isotopic ratios, and organic and inorganic components. (http://www.earthchem.org/seddb, last access: 25 March 2017).
-
MetPetDB is a database for metamorphic petrology, in a similar vein to PetDB and SedDB. This database also hosts large swathes of images collected through various methods such as X-ray maps and photomicrographs, although this information is not utilized in this paper (http://metpetdb.com/, last access: 3 June 2019).
-
The USGS National Geochemical Database archives geochemical information and its associated metadata from USGS studies and made available online
(https://www.usgs.gov/energy-and-minerals/mineral-resources-program/science/national-geochemical-database, last access: 9 October 2019).
Many other government initiatives and national databases exist, with notable examples including PETROCH from the Ontario Geological Survey (Haus and Pauk, 2010), New Zealand's national rock database (Petlab) (Strong et al., 2016), Australia's national whole-rock geochemical database (OZCHEM) (Champion et al., 2016), the Finnish lithogeochemical rock geochemistry database (RGDB) (Rasilainen et al., 2007), the Newfoundland and Labrador Geoscience Atlas (Newfoundland and Labrador Geological Survey, 2010), and the basement rock geochemical database of Japanese islands (DODAI) (Haraguchi et al., 2018).
While all of these are generally exceptional enterprises, we personally found that the variety of structures was cumbersome to reconcile or otherwise deficient in some respect for our own research. Some examples included databases being deficient in aged data (1000 Ma+) or lacking many recent publications. Some issues in certain existing databases were also evident; we found many samples missing information available in the original individual publications. It was quite common for age resolutions to be significantly larger than the values quoted within the paper itself, of the order of hundreds of millions of years in some cases or not included at all because they were not found in a table but within the text itself.
Thus, we sought to produce a database incorporating refined samples from previous databases and supplementing significantly from other, often recent, publications. Computed properties, naming schemes, and various geochemical indices have also been calculated where the data permit. Smaller subsets of previous iterations of this database have already been utilized for studies of heat production and phosphorus content (Hasterok and Webb, 2017; Hasterok et al., 2018; Cox et al., 2018; Gard et al., 2019b; Hasterok et al., 2019b), and this publication represents the totality of geochemical information gathered. As an ongoing process we have corrected some errors or omissions from previous databases as we have come across them, but we have not made a systematic effort to quality-check the prior compilations. We intend to continue updating the database in both additional entries and further clean-up when necessary.
While other database structures are incredibly efficient, some of the intricacies of the systems make it difficult to utilize the information contained within. For example, we had issues when seeking estimated or measured ages of rock samples. In order to examine temporal variations of chemistry and physical properties, an accurate and precise age is required. Under some of the present data management schemes it may be difficult to recover the desired data. Crystallization ages for older samples are often determined by U−Pb or Pb−Pb measurements from a suite of zircons. For a given sample, the individual zircon dates may be contained within the database and stored under mineral analyses. However, a search for rock chemistry may only return an estimated age (often a geologic timescale division). To get the crystallization age one would have to also download the individual mineral analyses, conduct an analysis on a concordia diagram (or similar), determine whether each individual analysis was valid, and then associate the result with the bulk chemistry. This process can be tedious and may be intractable. Had the estimated crystallization age been attributed to the sample directly, as often reported in the original study, much of this process could be shortened. Instead, our database attributes these estimated crystallization ages directly to the whole rock sample entry, which allows us to include estimated ages for the same unit or formation more readily. As a result the database presented here allows for a higher density of temporal sampling than other compilations.
The database is provided in two formats, the first as a compressed single spreadsheet for people unfamiliar with database management systems and the second as a mixed flat file and relational database structure. Codd (1970) was the first to propose a relational model for database management. A relational structure organizes data into multiple tables, with a unique key identifying each row of the sub-tables. These unique keys are used to link to other sub-tables. The main advantages of a relational database over a flat file format are that data are uniquely stored just once, eliminating data duplication as well as performance increases due to greater memory efficiency and easy filtering and rapid queries.
Rather than utilize an entirely relational database format, we have adopted some flat file formats for the sub-tables so as to reduce the number of total tables to an amount more manageable for someone unfamiliar with SQL database structure. This format raises storage memory due to data duplication in certain fields (e.g. repetition of certain string contents across multiple samples, such as rock name). However, we believe this is a reasonable trade-off for an easier-to-utilize structure for distribution and makes using these data for someone unfamiliar with SQL simpler. Ideally we would host a purely relational database structure online and be accessed via queries similar to the EarthChem Portal, but this is yet to be done.
PostgreSQL was utilized as the relational database management system (RDBMS) to update and administer the database. PostgreSQL contains many built-in features and useful addons, including the PostGIS geospatial database extender which we utilize, has a large open-source community, and runs on all major operating systems.
Python in conjunction with a PostgreSQL database adapter, Psycopg, is used to import new data efficiently. Data are copied into a .csv template directly from publications to reduce any chance of transcribing errors and dynamically uploaded to a temporary table in PostgreSQL. From here, the desired columns are automatically partitioned up and added to the database in their respective sub-tables. We iterate through a folder of new publications in this way and are able to add data rapidly as a result.
The database consists of 10 tables: trace elements, major elements, isotope ratios, sample information, rock group/origin/facies triplets, age information, reference information, methods, country, and computed properties. The inter-connectivity of these tables is depicted in Fig. 1, with tables linked via their respective id keys. A description of each of these tables is included in Table 1, and column names that require further details as well as computed property methods are detailed in Table 3. Individual sub-tables have been output as .csv files for use. We suggest inserting these into a RDBMS for efficient queries and extraction of desired data. However, we have exported these in .csv format in case people not familiar with database systems wish to work with them in other programs such as Microsoft®Excel, MATLAB®, or R. While technically inefficient, the largest sub-table currently stands at only 280 MB uncompressed, which we believe to be an acceptable size for data manipulation. The compressed merged spreadsheet is only 130 MB.
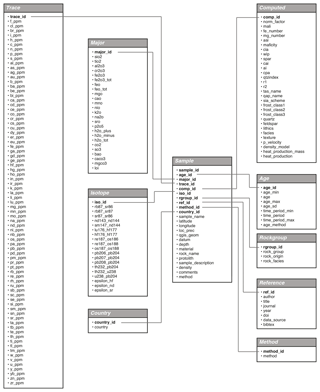
Figure 1Database relational structure. Sub-tables are linked through foreign id keys. Ambiguous field names are described in detail in the Supplement.
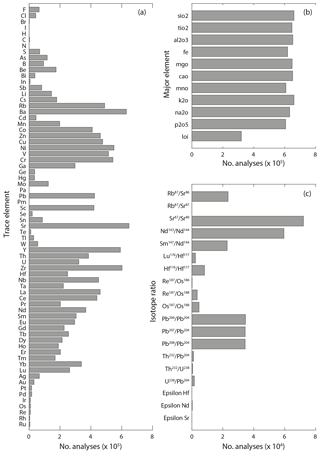
Figure 2Histogram of analyses. (a) Trace elements. (b) Major oxides. Fe denotes any one or more entries for feo, feo total, fe2o3, or fe2o3 total. (c) Isotope ratios and epsilon values.
Many samples include multiple geochemical analyses. These can vary from separate trace and major measurements with no overlap to duplicate element analyses using different methods. In the case of some subsets of these data we have chosen to merge these multiple analyses into a singular entry in the database. This methodology has both benefits and drawbacks. While it reduces the difficulty in selecting individual sample analyses, it means that lower-resolution geochemical methods are sometimes averaged with higher-precision ones. In the future we hope to prioritize these higher-precision methods where applicable (e.g. ICP-MS for many trace elements over XRF). Using a singular entry is simpler for many interdisciplinary scientists who do not wish to be slowed down by the complexity of managing duplicate samples and split analyses. We have generally kept track of this with the method field; where merging has occurred and both methods are known, we have concatenated the method in most cases.
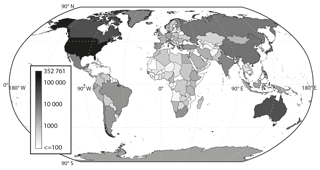
Figure 3Spatial distribution of geochemical samples. Countries are shaded based on the number of data points within the polygons.
4.1 Raw data
The largest existing database contributions to this database are listed in Table 2. Individual publication supplementation includes both new additions we have found in the literature as well as cleaned-up and modified entries from existing databases. The subsets of existing databases do not represent the entire collections for many of these programs as we have done pre-filtering to remove non-whole rock data or encountered issues with accessing the entire data set using online web forms.
Figure 2 denotes histograms of the various major, trace, and isotope analyses within the database. The majority of isotope data were recently sourced from the GEOROC database. Unsurprisingly, major element analyses in general dwarf the number of trace element measurements recorded.
Despite the heterogeneous nature of geochemical sampling, there is still reasonable spatial coverage around the world. However, there is a noticeable dominance of samples sourced from North America, and additionally Canada, Australia, and New Zealand (Fig. 3). The United States tops the list with 352 761 samples, including those from its non-contiguous states. The African continent suffers the most from lack of data with regards to the rest of the globe (Fig. 3).
Age distributions unsurprisingly show a significant dominance towards very recent samples (< 50 Ma), due largely to the oceanic subset (Fig. 4b). Age here is indicated as being an assumed crystallization age. Excluding major time-period-associated ages (e.g. a Paleoproterozoic age range of 2500–1600 Ma as the maximum and minimum ages of a sample), there are 355 467 samples with estimated crystallization age values. Of these, 282 147 have age uncertainty estimates, and observing the cumulative distribution function of these values indicates that ∼ 99 % of the age uncertainties fall below ∼ 150 Ma (Fig. 4a).
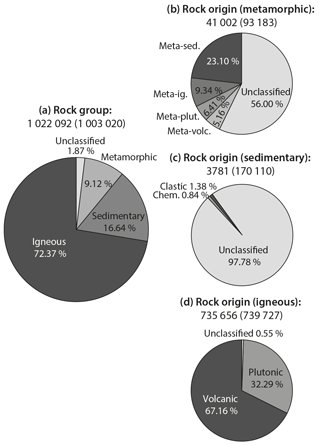
Figure 5Rock group partitioning. (a) Pie chart depicting distribution of samples containing a rock group. (b–d) denote the rock origin distributions of the rock group fields where rock origin is listed.
Rock group and rock origin are described in Table 3. There is a clear dominance towards igneous samples, making up 72.37 % of the data with known rock group information (Fig. 5). About 99 % of these igneous samples have a distinction noted as volcanic or plutonic in the rock origin field, with just over two-thirds of these being volcanic. Sedimentary samples are the next most common rock group; however, the vast majority of these have no classification in rock origin, and we aim to improve this in future updates. Finally, metamorphic rocks have ∼ 44 % of the samples with rock origin classifications. Metasedimentary origin is slightly more common than meta-igneous; however, meta-igneous includes two further subdivisions of meta-volcanic and meta-plutonic where known.
4.2 Naming schema – rock_type
Nomenclature varies significantly within geology, and unsurprisingly rock names within the database differ wildly as a result. Different properties such as texture, mineralogical assemblages, grain sizes, thermodynamic histories, and chemistry make up the majority of the basis for the various naming conventions utilized throughout, interspersed with author assumptions and/or inaccuracies. Thus, we sought a robust and consistent chemical classification scheme to assign rock names to the various samples of the database. This chemical basis classification scheme is stored in the computed table, within the rock_type field.
Differing naming work flows are applied to (meta-)igneous and (meta-)sedimentary samples. For igneous, meta-igneous, and unknown protolith origin metamorphic samples, we use a total alkali–silica (TAS) schema (Middlemost, 1994) modified to include additional fields for further classification of high-Mg volcanics (Le Bas and Streckeisen, 1991). See Fig. 6c and d for a partial visual description of the process. Furthermore, we classify igneous rocks as carbonatites when the CO2 concentration exceeds 20 wt. %. These entries are assigned either the plutonic or volcanic equivalent rock names depending on whether the sample is known to be of plutonic or volcanic origin.
For sedimentary and metasedimentary rocks, we first separate out carbonates and soils using ternary plot divisions of SiO2, Al2O3+Fe2O3, and CaO+MgO (Mason, 1952; Turekian, 1969). Additionally, we further partition clastic sediments using the SedClass™ classification method from Herron (1988). Quartzites are identified separately where SiO2 exceeds 0.9 in the ternary system. See Hasterok et al. (2018) for further discussion.
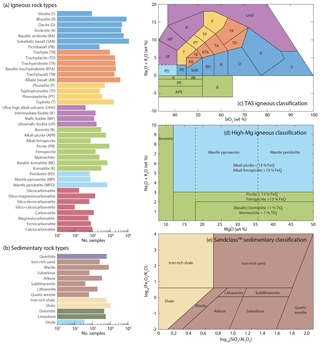
Figure 6Rock-type classification information. (a) Igneous and meta-igneous sample histograms of assigned rock names. (b) Sedimentary and metasedimentary sample histograms of assigned rock names. (c) TAS igneous classification (Middlemost, 1994). (d) High-Mg igneous classification. See Le Bas and Streckeisen (1991) for further information on classification methods. (e) Sedimentary classification, after Herron (1988) (Sandclass™).
A breakdown of the classification distributions is included in Fig. 6a and b. Sub-alkalic basalt/gabbro is a significantly large contribution to the volcanic samples, due to the extent of samples of oceanic nature.
4.3 Computed properties
In numerical models, rock types are often assigned physical property estimates that have been derived from limited data sets. We compute a number of properties and naming schema for a significant subset of the database, a new addition over many previous database compilations. This includes heat production, density, and P-wave velocity estimates, as well as various geochemical indices and descriptors such as modified TAS, QAPF, and SIA classifications. A full list of referenced methods and computed columns is given in Table 3.
Where computed values require major element concentrations, these properties and values have been calculated based on an LOI-free major element normalized version of the database, i.e. major element totals are normalized to 100, while preserving the relative proportions of each individual element's contribution to the total. This normalization occurs only on samples with major element totals between 85 wt. % and 120 wt. %. Totals lying outside this range are ignored, and properties requiring these values are not computed. The exact value of normalization for each sample is recorded in the computed table, within the norm_factor field. Figure 7a–c denote some property estimates calculated from the normalized analyses.
4.3.1 Density estimates
Density is an important input for a wide range of models, but only a small fraction of samples have measured density values associated with them. Contained within the database are a number of publications hosting density observations (e.g. Haus and Pauk, 2010; Barette et al., 2016; Slagstad, 2008). Following the method of Hasterok et al. (2018), we produce a set of simple oxide-based linear regression density models.
where Fe* is iron number, MALI is modified alkali-lime index, oxides are in weight percent, and ρ is density in kg m−3. Low-Mg, High-Mg, and Carb. (carbonated rocks) refer to the specific models for different rock groups. See Hasterok et al. (2018) for further discussion of the model fits. Density estimates peak at ∼ 2680 and ∼ 2946 kg m−3 due to mafic and felsic sample medians respectively.
4.3.2 Seismic velocity
We utilize the empirical model of Behn and Kelemen (2003) for estimating anhydrous P-wave seismic velocity. Their model was calibrated on ∼ 18 000 igneous rocks and validated against 139 high-quality laboratory measurements. However, this model does have limitations, as it was calibrated to anhydrous compositions only. Utilizing their three-oxide model, estimated uncertainty (1σ) is km s−1. P-wave velocity estimates depict maximums at ∼ 6.2 and ∼ 7.1 m s−1 (Fig. 7c). For further details or discussion, refer to Behn and Kelemen (2003) and Hasterok and Webb (2017).
where oxides are in weight percent and Vp is in m s−1.
4.3.3 Heat production
Heat production is computed by employing the relationship from Rybach (1988). Heat production estimates are resolved by a smoother distribution in log space than the dichotomous nature of the density and Vp estimates.
with concentrations of U, Th in ppm, K2O in weight percent and ρ in kg m−3. Heat production has a median value of ∼ 1.0 µW m−3, with first and third quartiles (25th and 75th percentiles) of 0.39 and 2.2 µW m−3 respectively.
5.1 Bibliographic information
Due to a high variety of sources and database formats, merging bibliographic information proved difficult. For individual publications and adjustments made manually, we have collated bibliographic information in higher detail. We hope to expand this .bib file as we continue to clean up the reference lists and make adjustments to other compilations. For other inherited bibliographic information from external databases, the exact format can vary. These details are contained within the reference .csv and are linked to each sample through the ref_id as seen in Fig. 1.
5.2 Ownership and accuracy
Although every effort is made to ensure accuracy, there are undoubtedly some errors, either inherited or introduced. We make no claims to the accuracy of database entries or reference information. It is up to the user to validate subsets for their own analyses, and ideally contact the original authors, previous database compilation sources, or ourselves to correct errors where they exist. We make no claim on ownership of these data; when utilizing this database, additionally cite the original authors and data sources.
The .bib file and .csv tables of this data set are available on Zenodo: https://doi.org/10.5281/zenodo.2592822 (last access: 9 October 2019) (Gard et al., 2019a). An associated set of software that can be used in MATLAB® to explore the database, including many of the individual methods cited above for the computed properties, is also available on github at https://github.com/dhasterok/global_geochemistry (Hasterok and Gard, 2019).
We have published portions of the database in the course of prior studies and will continue to expand this data set for our own research purposes. Small individual corrections have occurred incrementally with every version, and unfortunately we did not keep records of these improvements. Going forward, we plan to include a record of these corrections and forward them to the other database compilations as needed. We hope to work with existing compilation authors in the future to assist with new additions as well. This version of the database may be of use for these database initiatives to supplement their own records.
Utilizing this database, we have worked on methods for predicting protoliths of metamorphic rocks (Hasterok et al., 2019a). As over 57 % of the samples lack that information (Fig. 5), this methodology may be included in future database versions. We are also making progress on a geologic provinces map that captures tectonic terranes.
MG and DH worked on the processing codes, computed property estimates, and collated data sources. MG organized the database structure and framework codes and prepared the manuscript with contributions from all the co-authors. JAH collated the Antarctic geochemical set.
The authors declare that they have no conflict of interest.
We thank Bärbel Sarbas for supplying the GEOROC database in its entirety. We would also like to thank the following individuals for providing data sets and/or personal compilations: David Champion (GA), Dick Claeson (SGU), Trond Slagstad (NGU), Lorella Francalanci (UNIFI), Yuri Martynov (FEGI-RAS), Takeshi Hanyu (JAMSTEC), John Clemens (SUN), Harald Furness (UIB), Alex Burton-Johnson (BAS), and Marlina Elburg (UJ). Peter Johnson provided a collection of papers with data for the Arabian-Nubian Shield. Matthew Gard is supported by an Australian Government Research Training Program Scholarship. The views expressed herein are those of the authors and are not necessarily those of the Australian Government or Australian Research Council.
This research has been supported by the Australian Research Council's Discovery Projects funding scheme (grant no. DP180104074) and the Australian Research Council's Special Research Initiative for Antarctic Gateway Partnership (grant no. SR140300001).
This paper was edited by Attila Demény and reviewed by Juan Carlos Afonso, Kent Condie, and one anonymous referee.
Barette, F., Poppe, S., Smets, B., Benbakkar, M., and Kervyn, M.: Spatial variation of volcanic rock geochemistry in the Virunga Volcanic Province: Statistical analysis of an integrated database, J. Afr. Earth Sci., 134, 888–903, https://doi.org/10.1016/j.jafrearsci.2016.09.018, 2016. a
Barette, F., Poppe, S., Smets, B., Benbakkar, M., and Kervyn, M.: Spatial variation of volcanic rock geochemistry in the Virunga Volcanic Province: Statistical analysis of an integrated database, J. Afr. Earth Sci., 134, 888–903, https://doi.org/10.1016/j.jafrearsci.2016.09.018, 2017. a
Behn, M. and Kelemen, P. B.: Relationship between seismic P-wave velocity and the composition of anhydrous igneous and meta-igneous rocks, Geochem. Geophys. Geosyst., 4, 1041, https://doi.org/10.1029/2002GC000393, 2003. a, b, c
Buggle, B., Glaser, B., Hambach, U., Gerasimenko, N., and Marković, S.: An evaluation of geochemical weathering indices in loess–paleosol studies, Quatern. Int., 240, 12–21, https://doi.org/10.1016/j.quaint.2010.07.019, 2011. a
Carbotte, S. M., Marjanović, M., Carton, H., Mutter, J. C., Canales, J. P., Nedimović, M. R., Han, S., and Perfit, M. R.: Fine-scale segmentation of the crustal magma reservoir beneath the East Pacific Rise, Nat. Geosci., 6, 866–870, https://doi.org/10.1038/ngeo1933, 2013. a
Champion, D., Budd, A., Hazell, M., and Sedgmen, A.: OZCHEM National Whole Rock Geochemistry Dataset, Tech. rep., Geoscience Australia, available at: https://ecat.ga.gov.au/geonetwork/srv/eng/catalog.search?node=srv#/metadata/65464, last access: June 2016. a, b
Codd, E. F.: A Relational Model of Data for Large Shared Data Banks, Comm. ACM, 13, 377–387, https://doi.org/10.1145/362384.362685, 1970. a
Cox, G. M., Lyons, T. W., Mitchell, R. N., Hasterok, D., and Gard, M.: Linking the rise of atmospheric oxygen to growth in the continental phosphorus inventory, Earth Planet. Sci. Lett., 489, 28–36, https://doi.org/10.1016/j.epsl.2018.02.016, 2018. a, b
Debon, F. and Le Fort, P.: A chemical–mineralogical classification of common plutonic rocks and associations, T. Roy. Soc. Edin.-Earth, 73, 135–149, https://doi.org/10.1017/S0263593300010117, 1983. a, b
Frost, B. R., Barnes, C. G., Collins, W. J., Arculus, R. J., Ellis, D. J., and Frost, C. D.: A Geochemical Classification for Granitic Rocks, J. Petrol., 42, 2033–2048, https://doi.org/10.1093/petrology/42.11.2033, 2001. a, b, c, d, e, f, g, h, i
Gard, M., Hasterok, D., and Halpin, J.: Global whole-rock geochemical database compilation (Version 1.0.0), https://doi.org/10.5281/zenodo.2592823, 2019a. a, b
Gard, M., Hasterok, D., Hand, M., and Cox, G.: Variations in continental heat production from 4 Ga to the present – Evidence from geochemical data, Lithos, 342–343, 391–406, https://doi.org/10.1016/j.lithos.2019.05.034, 2019b. a
Geological Survey of Greenland: Ujarassiorit 1989–2011, Tech. rep., 2011. a
Haraguchi, S., Ueki, K., Yoshida, K., Kuwatani, T., Mohamed, M., Horiuchi, S., and Iwamori, H.: Geochemical database of Japanese islands for basement rocks: compilation of domestic article, J. Geol. Soc. Jpn., 124, 1049–1054, https://doi.org/10.5575/geosoc.2018.0027, 2018. a, b
Hasterok, D. and Gard, M.: A global geochemistry dataset for rocks, GitHub repository, available at: https://github.com/dhasterok/global_geochemistry, last acces: 8 October 2019.
Hasterok, D. and Webb, J.: On the radiogenic heat production of igneous rocks, Geosci. Front., 8, 919–940, https://doi.org/10.1016/j.gsf.2017.03.006, 2017. a, b, c
Hasterok, D., Gard, M., and Webb, J.: On the radiogenic heat production of metamorphic, igneous, and sedimentary rocks, Geosci. Front., 9, 1777–1794, https://doi.org/10.1016/j.gsf.2017.10.012, 2018. a, b, c, d, e, f
Hasterok, D., Gard, M., Bishop, C., and Kelsey, D.: Chemical identification of metamorphic protoliths using machine learning methods, Comput. Geosci., 132, 56–68, https://doi.org/10.1016/j.cageo.2019.07.004, 2019a. a
Hasterok, D., Gard, M., Cox, G., and Hand, M.: A 4 Ga record of granitic heat production - Implications for geodynamic evolution and crustal composition of the early Earth, Precambrian Res., 331, 105375, https://doi.org/10.1016/j.precamres.2019.105375, 2019b. a
Haus, M. and Pauk, T.: Data from the PETROCH lithogeochemical database, Miscellaneous release – data 250, Ontario Geol. Surv., 2010. a, b, c
Herron, M. M.: Geochemical Classification of Terrigenous Sands and Shales from Core or Log Data, SEPM Journal of Sedimentary Research, 58, 820–829, https://doi.org/10.1306/212f8e77-2b24-11d7-8648000102c1865d, 1988. a, b
Iwamori, H. and Nakamura, H.: Isotopic heterogeneity of oceanic, arc and continental basalts and its implications for mantle dynamics, Gondwana Res., 27, 1131–1152, https://doi.org/10.1016/j.gr.2014.09.003, 2015. a
Keller, B. and Schoene, B.: Plate tectonics and continental basaltic geochemistry throughout Earth history, Earth Planet. Sci. Lett., 481, 290–304, https://doi.org/10.1016/j.epsl.2017.10.031, 2018. a, b
la Roche, H. D., Leterrier, J., Grandclaude, P., and Marchal, M.: A classification of volcanic and plutonic rocks using R1R2-diagram and major-element analyses – Its relationships with current nomenclature, Chem. Geol., 29, 183–210, https://doi.org/10.1016/0009-2541(80)90020-0, 1980. a, b
Le Bas, M. and Streckeisen, A.: The IUGS systematics of igneous rocks, J. Geol. Soc., 148, 825–833, 1991. a, b
Lett, R. and Ronning, C.: BC rock geochemical database – British Columbia Geological Survey Geofile 2005–14, Tech. rep., British Columbia Ministry of Energy and Mines, 2005. a
Mamani, M., Worner, G., and Sempere, T.: Geochemical variations in igneous rocks of the Central Andean orocline (13 S to 18 S): Tracing crustal thickening and magma generation through time and space, Geol. Soc. Am. Bull., 122, 162–182, https://doi.org/10.1130/B26538.1, 2010. a
Mason, B.: Principles of Geochemistry, John Wiley & Sons, 1952. a, b, c, d
Middlemost, E. A.: Naming materials in the magma/igneous rock system, Earth Sci. Rev., 37, 215–224, https://doi.org/10.1016/0012-8252(94)90029-9, 1994. a, b
Nesbitt, H. W. and Young, G. M.: Formation and Diagenesis of Weathering Profiles, J. Geol., 97, 129–147, 1989. a
Newfoundland and Labrador Geological Survey: Newfoundland and Labrador GeoScience Atlas OnLine, Tech. rep., available at: http://geoatlas.gov.nl.ca (last access: June 2016), 2010. a, b
Parker, A.: An Index of Weathering for Silicate Rocks, Geol. Mag., 107, 501–504, https://doi.org/10.1017/S0016756800058581, 1970. a
Rasilainen, K., Lahtinen, R., and Bornhorst, T.: The Rock Geochemical Database of Finland Manual, Report of Investigation 164, Geol. Surv. Finland, 2007. a, b
Rybach, L.: Determination of heat production rate, in: Terrestrial Handbook of Heat-Flow Density Determination, edited by: Hänel, R., Rybach, L., and Stegena, I., chap. 4.2, 125–142, Kluwer Academic Publishers, Dordrecht, 1988. a, b, c
Slagstad, T.: Radiogenic heat production of Archean to Permian geological provinces in Norway, Norw. J. Geol., 88, 149–166, 2008. a
Strong, D., Turnbull, R., Haubrock, S., and Mortimer, N.: Petlab: New Zealand's national rock catalogue and geoanalytical database, New Zeal. J. Geol. Geophys., 53, 475–481, https://doi.org/10.1080/00288306.2016.1157086, 2016. a, b
Turekian, K.: The oceans, streams and atmosphere, in: Handbook of geochemistry, Springer-Verlag Berlin, Heidelberg, New York, 1, 297–323, 1969. a, b, c, d
Walker, J. D., Bowers, T. D., Black, R. A., Glazner, A. F., Lang Farmer, G., and Carlson, R. W.: A geochemical database for western North American volcanic and intrusive rocks (NAVDAT), in: Geoinformatics: Data to Knowledge, Geological Society of America, https://doi.org/10.1130/2006.2397(05), 2006. a