the Creative Commons Attribution 4.0 License.
the Creative Commons Attribution 4.0 License.
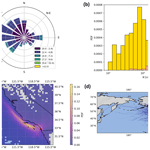
ARMTRAJ: a set of multipurpose trajectory datasets augmenting the Atmospheric Radiation Measurement (ARM) user facility measurements
Jennifer M. Comstock
Michael R. Kieburtz
Lynn M. Russell
Ground-based instruments offer unique capabilities such as detailed atmospheric, thermodynamic, cloud, and aerosol profiling at a high temporal sampling rate. The U.S. Department of Energy Atmospheric Radiation Measurement (ARM) user facility provides comprehensive datasets from key locations around the globe, facilitating long-term characterization and process-level understanding of clouds, aerosol, and aerosol–cloud interactions. However, as with other ground-based datasets, the fixed (Eulerian) nature of these measurements often introduces a knowledge gap in relating those observations with air-mass hysteresis. Here, we describe ARMTRAJ (https://doi.org/10.5439/2309851, Silber, 2024a; https://doi.org/10.5439/2309849, Silber, 2024b; https://doi.org/10.5439/2309850, Silber, 2024c; https://doi.org/10.5439/2309848, Silber, 2024d), a set of multipurpose trajectory datasets that helps close this gap in ARM deployments. Each dataset targets a different aspect of atmospheric research, including the analysis of surface, planetary boundary layer, distinct liquid-bearing cloud layers, and (primary) cloud decks. Trajectories are calculated using the Hybrid Single-Particle Lagrangian Integrated Trajectory (HYSPLIT) model informed by the European Centre for Medium-Range Weather Forecasts ERA5 reanalysis dataset at its highest spatial resolution (0.25°) and are initialized using ARM datasets. The trajectory datasets include information about air-mass coordinates and state variables extracted from ERA5 before and after the ARM site overpass. Ensemble runs generated for each model initialization enhance trajectory consistency, while ensemble variability serves as a valuable uncertainty metric for those reported air-mass coordinates and state variables. Following the description of dataset processing and structure, we demonstrate applications of ARMTRAJ to a case study and a few bulk analyses of observations collected during ARM's Eastern Pacific Cloud Aerosol Precipitation Experiment (EPCAPE) field deployment. ARMTRAJ will soon become a near real-time product accompanying new ARM deployments and an augmenting product to ongoing and previous deployments, promoting reaching science goals of research relying on ARM observations.
- Article
(4871 KB) - Full-text XML
- BibTeX
- EndNote
Synergistic use of ground-based, airborne, and satellite observations with continuously improving models promotes a better understanding of cloud and aerosol source and sink processes, aerosol–cloud interactions (ACIs), and cloud–climate feedbacks and helps refine climate projections. Nevertheless, even though high-resolution and Earth system models are becoming more sophisticated, our knowledge about some of these multiscale processes and their associate intensities and rates is still deficient; ergo, they remain the leading source of uncertainty in climate model predictions (Forster et al., 2021). Cutting-edge ground-based observations and their synthesis thereof provide opportunities to study cloud and aerosol processes in great detail. The U.S. Department of Energy Atmospheric Radiation Measurement (ARM) user facility operates multiple comprehensive suites of such instruments, which are deployed to key locations around the globe, including, for example, densely populated urban environments and high-interest regions such as the Southern Ocean, the Antarctic and Arctic, and the Atlantic and Pacific oceans' upwelling regions (Dorsey et al., 2024). ARM's mobile, fixed, and aerial facilities include, among other instruments, surface aerosol observing systems covering a wide range of sizes and properties (Uin and Smith, 2020), a range of profiling and scanning radars and lidars (e.g., Muradyan and Coulter, 2020; Widener et al., 2012a, b; Widener and Bharadwaj, 2012), and uncrewed aerial vehicles (Schmid and Ivey, 2016), which all promote atmospheric dynamic, thermodynamic, and radiative process research with a specific focus on clouds and aerosols.
In recent years, there has been a growing community recognition of the importance of Lagrangian considerations for acquiring causal understanding wherever atmospheric dynamics play a crucial role. This recognition is manifested in the integration of Lagrangian components in numerous aerosol and cloud, observational, and/or model simulation-based studies. For example, a comprehensive understanding of cloud life cycles often necessitates knowledge about the hysteresis and origin of cloudy air masses. Trajectory analyses support studies focused on warm, mixed-phase, and cold clouds, from low to high latitudes (e.g., Christensen et al., 2020; Ilotoviz et al., 2021; Mohrmann et al., 2019; Silber and Shupe, 2022; Svensson et al., 2023; Wernli et al., 2016). Back trajectories can inform on potential cloud formation mechanisms (e.g., Silber and Shupe, 2022; Svensson et al., 2023), be used to evaluate the influence of air-mass intrusions on cloud evolution (e.g., Christensen et al., 2020; Ilotoviz et al., 2021; Mohrmann et al., 2019), and generally support process understanding through modeling studies by providing boundary conditions and observationally based benchmarks (e.g., Neggers et al., 2019; Silber et al., 2019; Tornow et al., 2022).
Estimations of air-mass trajectories and origin are also highly valuable for understanding aerosol hysteresis and potential indirect effects. For example, back-trajectory analyses were previously used to examine dust ice-nucleating particle (INP) processing before entraining into a cloud (e.g., Wiacek and Peter, 2009), to quantify periods of chemical reactions experienced by aerosols prior to their transport to ground-based stations (e.g., Hawkins and Russell, 2010), to estimate source contribution functions and the similarity of source regions (e.g., Day et al., 2010; Liu et al., 2011), and to study the long-range transport of aerosols to surface sites (e.g., Zheng et al., 2020).
While ARM field deployments provide a high-end, unique, and comprehensive suite of measurements, most samples are collected at fixed sites, i.e., from an Eulerian perspective. Certain field campaigns include multiple deployment sites along climatological flow patterns (e.g., Geerts et al., 2022), yet a knowledge gap often still exists, which can be ameliorated using trajectory calculations. Here we describe ARMTRAJ, a set of Lagrangian trajectory data products for ARM fixed sites and mobile deployments, which can be used to close some of the gaps ensuing from the Eulerian nature of many ARM cloud, aerosol, and other atmospheric measurements, thereby enhancing the versatility of ARM datasets. Example include understanding the impact of pollution upwind of ARM deployment sites on measured aerosol properties versus clean upwind conditions; evaluating the effect of cloud-top aerosol entrainment on sink processes by using ARMTRAJ data and ARM measurements to initialize and force model simulations; and analyzing cloud life cycles before and after overpassing ARM sites by synthesizing ARM, satellite, and ARMTRAJ data. ARMTRAJ is based on the HYSPLIT model (Stein et al., 2015), informed by the European Centre for Medium-Range Weather Forecasts (ECMWF) ERA5 reanalysis (Hersbach et al., 2020) at its highest spatial resolution (0.25°, ∼ 31 km). ARMTRAJ datasets provide information about air-mass coordinates upwind and (in certain datasets) downwind, together with their thermodynamic properties and overpassed surface characteristics. Varying-size ensemble run results are also reported, facilitating the evaluation of trajectory consistency, robustness, and uncertainty while mitigating potential near-surface artifacts and errors. In Sect. 2, we describe ARMTRAJ's four dataset types, focusing on the surface, planetary boundary layer (PBL), and observed clouds over ARM sites. In Sect. 3, we demonstrate ARMTRAJ dataset applications using ARMTRAJ data for the ARM Eastern Pacific Cloud Aerosol Precipitation Experiment (EPCAPE; Russell et al., 2021), available on the ARM Data Discovery website (https://adc.arm.gov/discovery/, last access: 30 October 2024). Dataset availability is stated in Sect. 4. Conclusions and a short outlook are given in Sect. 5.
ARMTRAJ's four datasets include surface, PBL, liquid cloud layer, and primary cloud deck datasets, hereafter referred to as ARMTRAJ-SFC, ARMTRAJ-PBL, ARMTRAJ-CLD, and ARMTRAJ-ARSCL, respectively. Datasets are organized in daily files. Each file is in NetCDF format and follows ARM standards (see Palanisamy, 2016), including full metadata for each variable field. Each dataset contains sets of the following variables and properties extracted and derived from ERA5 data along each air-mass trajectory.
-
date and time;
-
air-mass coordinates: latitude, longitude, altitude above mean sea level (a.m.s.l.), and height above ground level (a.g.l.);
-
thermodynamic variables: air-mass pressure, temperature, potential temperature, equivalent potential temperature (excluding condensate from the calculation), virtual potential temperature, specific humidity, relative humidity (RH), and RH with respect to ice;
-
hourly-mean air-mass ascent rate (vertical motion);
-
PBL height (PBLH) in the air-mass column and air-mass height-to-PBLH ratio (greater than 1 when air mass is above the PBL and vice versa);
-
land–sea mask (land area fraction) and daily (00:00 UTC) sea-ice cover in the air-mass column (based on ERA5's associated ∼ 31 km native grid cell);
-
other surface properties in the air-mass column: terrain orientation and distortion in the horizontal plane, standard deviation and slope of orography within the ERA5 grid cell (using a minimum horizontal feature scale of 5 km), low and high vegetation type and cover, and soil type.
Trajectory calculations are performed with HYSPLIT, reading the same ERA5 global data files in pressure-level vertical coordinates supplemented with single-level reanalysis data fields such as PBLH and surface altitude, winds, and roughness length. Each ARMTRAJ dataset is initialized and configured differently to align with its purpose, potential use, and the characteristics of the ARM dataset required for initialization (see Table 1). ARMTRAJ datasets are discussed in detail below.
Table 1ARMTRAJ dataset summary. The dataset names ARMTRAJ-SFC, ARMTRAJ-PBL, ARMTRAJ-CLD, and ARMTRAJ-ARSCL refer to the surface, planetary boundary layer, liquid cloud layer, and primary cloud deck datasets, respectively.
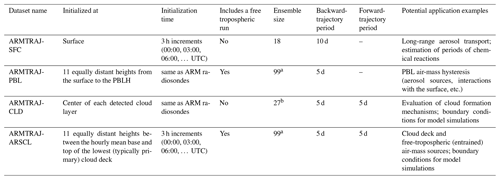
a Ensemble size of 9 in free-tropospheric runs (see Sect. 2.2). b Per detected liquid-bearing cloud layer (see Sect. 2.3).
2.1 Surface trajectory dataset (ARMTRAJ-SFC)
The ARMTRAJ-SFC dataset is designed to support research using ARM's surface measurements, with an emphasis on aerosol observations. While ground-based remote-sensing measurements and retrievals are made regularly and airborne in situ aerosol observations occur episodically during intensive observing periods, surface measurements are typically the most informative about the sampled aerosol chemical, morphological, microphysical, and radiative properties, given fewer limitations such as payload dimensions and weight. For a given day at a given ARM site, ARMTRAJ-SFC is initialized at the surface every 3 h. Each run includes a 10 d back trajectory. The 10 d period is sufficient to determine potential long-range aerosol transport sources and/or estimate periods of relevant chemical reactions (e.g., Hawkins and Russell, 2010; Lata et al., 2021; Zheng et al., 2020). While some studies examined back trajectories extending even 15 d, tests we performed using an ensemble approach (not shown) suggested that trajectory dispersion predominantly becomes so substantial that the air-mass information is no longer consistent nor robust. This dispersion is most likely driven by the propagation of errors stemming from multiple factors, such as the integration time step and the limited vertical resolution of the ERA5 pressure-level data used by HYSPLIT, especially near the surface.
In addition to the information for the trajectory initialized at the ARM site (surface level), the mean and standard deviation of ensemble results are reported for each of the variables listed above, except for the orographic, vegetation, and soil properties, the values of which are reported for the ensemble mean coordinates. The ensemble is initialized using 2 starting heights (surface and 50 m a.g.l.) and 9 starting horizontal locations (combinations of site coordinates ±7.5 km in the east–west and north–south directions, defining a 3 × 3 grid) for a total of 18 ensemble members. The fixed geodetic distance in metric units rather than in arc degrees is used to ensure ARMTRAJ's ensemble configuration consistency when initialized in different geographic regions, e.g., in ARM's North Slope of Alaska site (Verlinde et al., 2016), where a given longitudinal arc length translates to shorter geodetic distances relative to lower-latitude sites. The ensemble starting horizontal extent covers roughly half of the horizontal dimension of ERA5 grid cells, allowing for the evaluation of ensembles' physical variability yet keeping them initially constrained to the site vicinity. In practice, the ensemble results, specifically the standard deviation of reported ensemble variables, can be treated as a measure of trajectory-estimated uncertainty and potentially serve as tests for general trajectory robustness. We note that ARMTRAJ-SFC data files are supplemented with 1 h mean and standard deviation values (starting at trajectory initialization time) of surface observations from the corresponding ARM site Surface Meteorology System (Ritsche, 2011).
2.2 Planetary boundary layer trajectory dataset (ARMTRAJ-PBL)
ARMTRAJ-PBL, which could support PBL cloud and aerosol research in addition to other PBL research topics, includes 5 d back-trajectory calculations for the base (surface), middle, and top of the PBL (i.e., the PBLH). The PBLH used in HYSPLIT initialization is determined from ARM radiosonde measurements (Holdridge, 2020) using a bulk Richardson number method (Troen and Mahrt, 1986; Vogelezang and Holtslag, 1996) with a critical threshold value of 0.25, as reported in ARM's PBLH value-added product (VAP) (Sivaraman et al., 2013). Therefore, ARMTRAJ-PBL trajectories are initialized at radiosonde release times rounded to the nearest whole hour, resulting in two to four trajectory starting times for a given day, depending on sounding measurement availability.
There are other methods to determine the PBLH, the radiosonde-based retrievals of which are reported in ARM's VAP (see Sivaraman et al., 2013) and included in ARMTRAJ-PBL. However, the utilized bulk Richardson number method and its threshold value were evaluated by Seidel et al. (2012), who suggested they are suitable for both convective and stable PBLs, though we note that Zhang et al. (2022) recently suggested this method better compares to the ceilometer-based PBLH determination method under stable PBL conditions. Moreover, the same method and threshold values are consistent with the PBLH implementation in ERA5 diagnostics used here.
The ensemble in the ARMTRAJ-PBL dataset is much greater than ARMTRAJ-SFC's ensemble. It consists of 11 equally distant heights from the surface to the PBLH combined with a similar 3 × 3 grid, totaling 99 ensemble members. This extensive ensemble configuration ameliorates the lack of explicit mixing in the ECMWF Integrated Forecasting System (IFS) model used to drive ERA5 and the limited near-surface resolution (∼ 250 m) at the ERA5 pressure-level grid.
The PBL and its associated cloud and aerosol fields are known to interact with the free troposphere above the PBLH, with a potentially pronounced indirect impact on properties and processes such as cloud life cycles, aerosol scavenging, and the PBLH (e.g., Jiang et al., 2002; Raes, 1995; Raes et al., 2000; Sorooshian et al., 2020; Tornow et al., 2022). ARMTRAJ-PBL also includes free-tropospheric runs for each trajectory starting time, initialized 200 m above the reported PBLH to support and augment studies focusing on free-tropospheric entrainment effects. The free-tropospheric run results include all variables listed at the beginning of this section, as well as results for a small nine-member ensemble, initialized at the same height with the 3 × 3 grid as in the other ensembles.
2.3 Liquid-bearing cloud layer trajectory dataset (ARMTRAJ-CLD)
ARMTRAJ-CLD aims to augment liquid-bearing cloud studies from warm to mixed-phase clouds. The 5 d backward and forward trajectories reported in this dataset broadly cover the typical residence time of moisture in the atmosphere (see van der Ent and Tuinenburg, 2017; Läderach and Sodemann, 2016; Woods and Caballero, 2016) and can, therefore, promote the evaluation of cloud formation mechanisms and cloudy air-mass hysteresis. The dataset provides essential information for the configuration and initialization of modeling exercises (e.g., Silber et al., 2019; Tornow et al., 2022), and in some cases, the reported trajectories can even inform on other overpassed ground-based observational sites upwind or downwind (e.g., Ali and Pithan, 2020), further constraining modeling efforts.
Like ARMTRAJ-PBL, ARMTRAJ-CLD's initialization depends on ARM radiosonde data (see Table 1) for determining liquid-bearing cloud layers and, therefore, has the same starting times. A leading advantage of using radiosonde-based cloud detections for initialization is that we can examine full tropospheric profiles and are not confined to the first few to several optical depths, as in the case of lidars that are commonly used to detect liquid-bearing cloud layers from the bottom up in the case of ground-based observations (or from the top down in the case of satellite and aircraft observations). Liquid-bearing cloud layers are determined from radiosonde RH profiles using the following steps:
-
Set radiosonde samples as “cloud” if RH values exceed 96 %. This threshold value considers the radiosonde vendor's uncertainty (Holdridge, 2020) and was previously validated using lidar-based cloud layer detections (Silber et al., 2020, Fig. S1; e.g., Silber and Shupe, 2022; Stanford et al., 2023, Appendix D). We have also qualitatively examined detection consistency using higher RH thresholds against other remote-sensing measurements for different observed cases (not shown) and came to the same conclusion regarding the 96 % threshold value validity.
-
Concatenate “cloud” samples that are vertically distant by less than 50 m from each other.
-
Remove resulting layers if their total thickness (including the thickness of the cloud-top sample) is smaller than 25 m.
ARMTRAJ-CLD can report trajectories for up to 10 detected overlying liquid-bearing layers per initialization time step. The initialization height is set to the center of each detected cloud layer. In this case, the ensemble results are based on 27 members per detected cloud layer: 3 vertical starting heights (cloud layer center and center ±50 m) and 9 horizontal coordinates using the same 3 × 3 grid as in the other datasets. The detected liquid-bearing cloud layer boundaries and the utilized radiosonde thermodynamic and wind measurements are also reported in ARMTRAJ-CLD to further support cloud-related analysis.
2.4 Primary cloud deck trajectory dataset (ARMTRAJ-ARSCL)
Many studies, such as those on marine stratocumulus clouds, often focus on primary cloud decks, which in this context refer to the optically and geometrically thickest cloud decks in atmospheric columns. Those primary cloud decks typically produce a significant radiative effect, impacting the surface and atmospheric energy budgets. ARMTRAJ-ARSCL's objective is to support studies focusing on those cloud decks while still providing analysis flexibility by running the trajectory calculations 5 d backward and forward in time. The ARSCL suffix in the dataset's name refers to the Active Remote Sensing of CLouds (Clothiaux et al., 2001), a widely used ARM VAP, which combines data from ARM radars and lidars to produce an objective determination of cloud deck and hydrometeor vertical boundaries together with associated radar moments. In this context, a primary cloud deck can contain multiple liquid-bearing layers vertically connected by precipitation detectable by the profiling radar (e.g., Fig. 1).
The ARMTRAJ-ARSCL dataset is initialized every 3 h, similar to ARMTRAJ-SFC. The cloud deck base for trajectory initialization is determined as the 1 h mean (starting at the initialization timestamps) cloud base height (the “cloud base best estimate” field in ARSCL). This ARSCL field is processed using a ceilometer and micropulse lidar combination with a general tendency toward the ceilometer data product, which was previously evaluated against high-spectral-resolution lidar data and found to have a small positive bias typically under 50 m (Silber et al., 2018). This small bias should be, in most cases, insignificant, given that cloud deck geometrical depths are commonly significantly greater (e.g., Lu et al., 2021). The cloud deck top is set as the 1 h mean first radar top (first radar echo with an overlying clear-sky range gate), the samples of which are included in the averaging only if, at a given time step, they are above the cloud deck base. Among other similarities to ARMTRAJ-PBL, we also run the trajectory calculations for the free troposphere to address community interest in processes such as cloud-top entrainment. Because the cloud top can be fairly variable over a 1 h period, we set the free-tropospheric height as the sum of the 1 h mean cloud top, its 1 h standard deviation (using the same samples as in the first radar-top averaging), and 200 m. Figure 1 exemplifies sets of cloud deck base, top, and free-tropospheric heights used to initialize the ARMTRAJ-ARSCL trajectories over a 24 h period. Because a cloud deck was observed throughout the depicted day, the 3 h initialization interval translates to the eight illustrated sets. Note the consistency between the cloud base markers and the initialized cloud deck base height, as well as the variable distance between the cloud deck top and free-tropospheric height, depending on the cloud deck top's temporal variability.
Similar to ARMTRAJ-PBL, ARMTRAJ-ARSCL reports trajectory data for the cloud deck base, middle, and top and includes 99-member ensemble results using 11 equally distant heights between the cloud deck base and top combined with the same 3 × 3 grid as in the other datasets (the nine-member ensemble results are reported for the free troposphere). Hourly means of auxiliary data used and reported by ARSCL, such as hydrometeor field boundaries and liquid water path retrieved by ARM's microwave radiometer (Morris, 2006), are included in the ARMTRAJ-ARSCL data files.
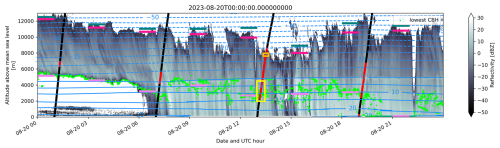
Figure 1ARSCL radar reflectivity (color scale) on 20 August 2023, depicting the landfall of tropical storm Hilary at the ARM EPCAPE deployment in La Jolla, San Diego, California. The green markers denote the ARSCL-reported cloud deck base. Blue contours represent temperatures (in degree Celsius) from the ARM interpolated-sounding VAP (Fairless et al., 2021). Slanted black lines illustrate sounding profiles, with the red sections delineating sounding-based liquid-bearing cloud layer detections. The violet, pink, and teal horizontal lines designate the cloud deck base, top, and free-tropospheric heights for ARMTRAJ-ARSCL's HYSPLIT trajectory initialization. The line lengths (a fixed 1 h) represent the ARSCL data averaging period. The yellow and orange rectangles highlight the liquid-bearing cloud layers analyzed in Sect. 3.1.
The ARMTRAJ datasets currently cover the full EPCAPE deployment, from February 2023 to February 2024 (ARMTRAJ-ARSCL starting from March 2023). Given the diverse potential usage options of ARMTRAJ, here we limit ourselves to four short analyses utilizing each of ARMTRAJ's datasets. We first describe a case study using ARMTRAJ-CLD where we exemplify the value of ARMTRAJ's ensemble runs in evaluating trajectory confidence and uncertainties. We then briefly present three bulk analyses of ARMTRAJ-SFC, ARMTRAJ-PBL, and ARMTRAJ-ARSCL.
3.1 Case study: mid- and upper-level liquid-bearing cloud layers in tropical storm Hilary
Hurricane Hilary was the first tropical cyclone to hit southern California as a tropical storm since 1939. By chance, this landfall occurred during the 1-year-long ARM EPCAPE deployment, most instruments of which were operating during the event. Profiling radar observations, for example, captured the cloud deck evolution over La Jolla from a cirrus-topped mixed-phase cloud to a heavily precipitating deep cloud deck with multiple embedded liquid-bearing layers, indicated by the sounding measurements (Fig. 1). Figure 2 depicts a 5 d back trajectory of a cloudy air mass detected using the 13:00 UTC radiosonde, which extended from an altitude of ∼ 2300 to 4250 m (yellow rectangle in Fig. 1). The trajectory for the cloud middle section starting at the ARM deployment coordinates appears largely colocated with the ensemble mean up to 4 d backward in time (Fig. 2, left). The trajectory consistency and relatively small variability in air-mass ensemble coordinates are indicative of the single-site coordinates' trajectory being representative in this specific scenario.
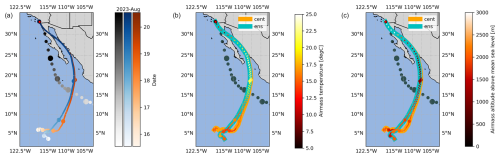
Figure 2ARMTRAJ-CLD 5 d back-trajectory properties of a cloudy layer detected on 20 August 2023, using the 13:00 UTC sounding measurements (see yellow rectangle in Fig. 1). (a) Air-mass trajectory using the ARM deployment coordinates (orange tints), ensemble-mean trajectory (blue shades), and 6-hourly markers of hurricane Hillary's track (transitioned to a tropical storm on 20 August, grey tints). (b) The same trajectories overlaid with hourly air-mass temperature and (c) altitude a.m.s.l. Larger markers denote 24 h increments from the trajectory initialization time. The red marker designates the ARM EPCAPE deployment site.
Examination of the trajectory timing against the center of Hilary (Fig. 2) suggests that the air mass entrained into the rear-right flank of the cyclone roughly 1–2 d prior to the EPCAPE overpass. Forced by the cyclone, the air mass strongly accelerated (increasing distance between large markers in Fig. 2) and gradually subsided and warmed until ∼ 18 h from the EPCAPE overpass (Fig. 2, middle and right). From that point, being much closer to the cyclone center, the air mass was lofted (Fig. 2, right); cooled, likely adiabatically (Fig. 2, middle); and eventually reached water vapor equilibrium, resulting in condensation.
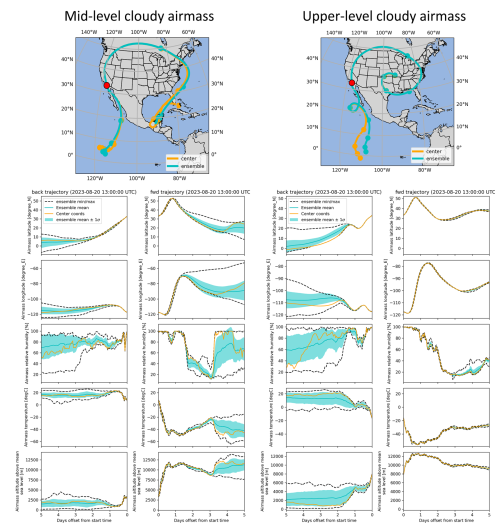
Figure 3Two sets of backward and forward trajectories of cloudy air masses detected on 20 August 2023 using the 13:00 UTC sounding. The top two panels depict trajectory maps (larger markers denote 24 h increments from initialization time), and the bottom panels illustrate (from top to bottom) time series of air-mass latitude and longitude coordinates, relative humidity, temperature, and altitude a.m.s.l. The back trajectory of the air mass in the left set of panels is the same as in Fig. 2, whereas the right set of panels represents a cloudy air mass detected over the EPCAPE deployment at a higher altitude (see orange rectangle in Fig. 1). Each time series plot shows the temporal evolution of air-mass parameters along trajectories initialized at the center coordinates together with the ensemble mean, minimum, maximum, and the mean ±1 standard deviation (σ) (see the legend).
The left set of panels in Fig. 3 expands the analysis of center coordinate trajectories versus ensemble results by depicting time series plots of both backward and forward trajectories of the same mid-level cloudy air mass discussed above. The right set of panels illustrates backward- and forward-trajectory calculations initialized for a thin (∼ 100 m deep) high-level supercooled cloud layer detected at ∼ 7800 m using the same 13:00 UTC EPCAPE sounding profile (orange rectangle in Fig. 1). In both cases, the air masses are forced upward by the cyclone on the first day following the EPCAPE overpass, thereby cooling, producing more condensate, and converting into a cirrus cloud, as suggested from the air-mass relative humidity, temperature, and altitude time series panels. Specifically, all ARMTRAJ ensemble members are consistently characterized by air-mass relative humidity remaining at ∼ 100 % and temperature decreasing and staying below −38 °C. During this significant ascent of the cloudy air masses to the tropopause region, they are entrained into the polar jet stream, which carries them several thousand kilometers (mostly eastward) in the following few days (see maps and the air-mass latitude and longitude panels).
In the case of the back trajectories for the mid-level cloudy air mass (left set of Fig. 3 panels), the air-mass parameters for the center coordinates and ensemble mean stay colocated for roughly 4 d, as noted above. However, the uncertainty of the air-mass origin and thermodynamic properties generally increases backward in time, evident by the increasing ensemble standard deviation and the range between the ensemble member minimum and maximum. Similarly, the uncertainty of the forward trajectory parameters significantly increases, starting roughly 1 d after the EPCAPE overpass. At the 5 d mark, the coordinate uncertainties are on the order of 10° in latitude and longitude, and the ensemble member range is on the order of several tens of degrees; relative humidity uncertainty is ∼ 20 % and temperature uncertainty is greater than 10 °C compared to ∼ 5 % and ∼ 2 °C at the 3 d mark, respectively. Taken together, these ensemble results suggest low confidence in the air-mass forward-trajectory properties, especially beyond 2–3 d, and somewhat higher confidence in the air-mass back-trajectory properties.
The back-trajectory ensemble spread in the right set of panels in Fig. 3, representing the upper-level cloudy air mass, is more extensive than the spread of the mid-level cloud layer discussed above. For example, 3 d prior to the EPCAPE overpass, this upper-level cloudy air mass exhibits relative humidity, temperature, and altitude uncertainties roughly double those of the mid-level cloudy air mass, with values of ∼ 27 %, ∼ 10 °C, and ∼ 1830 m compared to ∼ 14 %, 4 °C, and ∼ 850 m, respectively. However, given the ensemble temperature, relative humidity, and altitude largely monotonic tendencies, we can still deduce that this high-altitude air mass is most likely of warm and moist low-latitude low-level oceanic origin, forced upward by the cyclone, as also suggested by the spiraling movement depicted in the top-right panel. Unlike the mid-level layer back trajectories (left set of panels), in this case the center coordinates' air-mass trajectory is one of the ensemble extremes at certain times, even though the center coordinates are at the center of the ensemble latitude–longitude–height initialization mesh. As an additional contrast to the mid-level cloud case, the forward-trajectory ensemble remains consistent with very little variance throughout the 5 d period. The differences in ensemble spread between those somewhat similar trajectories calculated for cloudy air masses exemplify the case-specific nature of trajectory robustness and the value of ensemble data.
3.2 Bulk analysis of surface back trajectories
While the cloudy air mass observed during Hilary's EPCAPE overpass originated south to southeast of the deployment site, closer to the surface, the La Jolla region often experiences marine flow from the northwest directions (e.g., Liu et al., 2011). Here, we briefly examine the potential source origin and properties of air masses reaching the EPCAPE deployment. We focus on ensemble mean variables, which are more robust than single trajectories for the deployment site coordinates, and provide uncertainty estimates, though these are largely excluded from this analysis for brevity.
Analysis of hourly-mean winds, taken in 3 h increments per ARMTRAJ-SFC's structure (Sect. 2.1), indicates a westerly to northwesterly component dominance (Fig. 4a). A joint probability density function (PDF) of 12–36 h back-trajectory coordinates supports the surface measurement indications of marine air-mass sources, specifically of coastal origin (Fig. 4c). This proximity of air masses to densely populated regions could suggest that aerosol properties might be strongly influenced by the proximity to these more polluted regions, especially considering that more than 90 % of hourly air-mass samples in this 12 to 36 h period were within the PBL (when accounting for ensemble standard deviation of air-mass height).
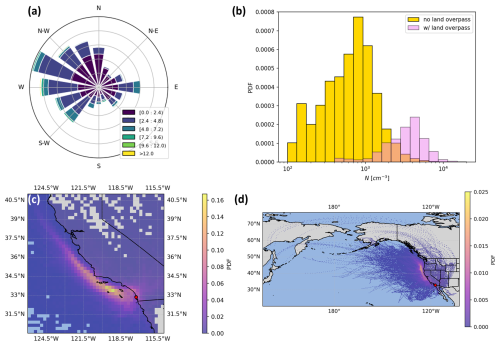
Figure 4ARMTRAJ-SFC bulk analysis: (a) wind rose based on hourly-mean data from the ARM Surface Meteorology System (legend values are in m s−1), (b) submicron total number concentration histogram (logarithmic bin width of 0.121) using SMPS data partitioned based on whether 12–36 h surface back trajectories had any land overpass (see the main text), (c) probability density function of 12–36 h back-trajectory samples (bin dimensions of 0.25 × 0.25°), and (d) the same but for 48–96 h back trajectories.
Indeed, the distribution of submicron aerosols (10–500 nm in diameter) measured by the ARM scanning mobility particle sizer (SMPS; Kuang, 2016) illustrates a distinct picture. When partitioned based on whether a given 12–36 h back trajectory overpassed a land surface grid cell (defined here as an overpass of an ERA5 grid cell with land area fraction exceeding 0.5 for at least 1 h), a clear separation is observed in total aerosol number concentration with cases of air masses overpassing land having notably higher concentrations (Fig. 4b). This number concentration separation was also distinct when only trajectories corresponding to westerly surface wind direction measurements were used and, to a lesser extent, when the partitioning was performed using earlier periods such as 72–96 h, 96–120 h, etc. (not shown). This sensitivity to land proximity, even in more distant periods in air-mass hysteresis, is supported by the general consistency of surface air masses sampled at EPCAPE to follow coastal flow patterns even several days before the arrival at the deployment site (Fig. 4d).
3.3 Potential PBL air-mass aerosol sources based on bulk statistics
We can further evaluate potential aerosol sources by examining the surface and vegetation types that air masses overpassed. Here, we consider that aerosols are continuously mixed within the PBL along their trajectory path until they are eventually sampled at the ground-based deployment site. We use the ARMTRAJ-PBL ensemble mean path and generate a pie chart of average surface and vegetation properties (implemented in the IFS model and reported in ARMTRAJ) along the trajectories during the 5 d preceding the arrival at EPCAPE's coordinates. We only count samples where the air-mass ensemble mean height minus the ensemble mean standard deviation is within the PBL (∼ 92 % of all ARMTRAJ-PBL samples). Vegetation and surface property samples are weighted based on their corresponding cover fraction. The resulting pie chart (Fig. 5) indicates the dominating marine sources. This result is generally expected, given the marine source dominance suggested in the ARMTRAJ-SFC combined with the median (average) PBLH of 180 (255) m calculated from the ARM surface measurements. On average, air masses are influenced more than 4 % of the time by evergreen shrubs and needleleaf trees, known to be significant sources of natural volatile organic compounds that can form secondary organic aerosols (SOAs) (see Guenther et al., 1995; Shrivastava et al., 2017). A detailed analysis beyond the scope of this study might be able to robustly characterize the influence of these air-mass overpasses on surface aerosol observations.
3.4 Bulk analysis of potential air-mass origin differences between cloud deck and free-tropospheric air masses
Aerosols such as SOA are commonly formed and transported within the PBL or other elevated mixed layers, serve as cloud condensation nuclei, and eventually influence cloud properties. Due to different hystereses (flow patterns, atmospheric residence time due to chemical reactions, scavenging, etc.), free-tropospheric aerosols often differ in their properties and source origin from mixed-layer aerosols in the same atmospheric column. This source origin difference can be demonstrated by analyzing the difference in potential source origin between cloudy and free-tropospheric air-mass trajectories in the ARMTRAJ-ARSCL dataset. Figure 6 qualitatively illustrates this in-cloud and above-cloud air-mass trajectory difference using 3–5 d ensemble mean coordinate PDFs. Some specific trajectories can be observed in the plot as distinct patterns. Similar to ARMTRAJ-SFC, in-cloud air masses tend to concentrate along the Pacific coast, though with greater spread. This spread is likely the result of variable flow patterns between cloud deck boundaries, which only partially overlap with the near-surface flow patterns or the PBL depth in general, as defined by the bulk Richardson number method. Unlike the in-cloud deck air masses, the above-cloud (or free-tropospheric) air masses tend to originate (within the 3–5 d range) in northeastern Pacific marine areas, generally west to southwest of the deployment site, as well as in inland regions along the Great Basin and Mojave Desert and the Californian part of the Sierra Nevada range. We can expect that aerosol properties of these in- and above-cloud deck air masses would be different. However, such detailed studies, likely involving airborne measurements such as those collected during the Southern California Interactions of Low cloud and Land Aerosol (SCILLA) experiment complementing EPCAPE, are beyond the scope of this study.
Current and future releases of ARMTRAJ datasets (https://doi.org/10.5439/2309851, Silber, 2024a; https://doi.org/10.5439/2309849, Silber, 2024b; https://doi.org/10.5439/2309850, Silber, 2024c; https://doi.org/10.5439/2309848, Silber, 2024d) are and will be available on the ARM Data Discovery website (https://adc.arm.gov/discovery/#/results/s::armtraj, last access: 30 October 2024). Sounding (https://doi.org/10.5439/1595321, Keeler et al., 2022), meteorological station (https://doi.org/10.5439/1786358, Kyrouac et al., 2021), PBLH (https://doi.org/10.5439/1991783, Zhang, 2021), interpolated sounding (https://doi.org/10.5439/1095316, Jensen et al., 2021), ARSCL (https://doi.org/10.5439/1393437, Johnson et al., 2014), and SMPS (https://doi.org/10.5439/1476898, Kuang et al., 2021) data from the ARM EPCAPE deployment are available on the ARM Data Discovery website (https://adc.arm.gov/discovery/, last access: 14 April 2024). Hurricane Hilary's tracking data (Kruk et al., 2010) are available on the International Best Track Archive for Climate Stewardship (IBTrACS) website (https://doi.org/10.25921/82ty-9e16, Gahtan et al., 2024).
ARMTRAJ datasets provide essential support for the utilization of ARM deployments. They mitigate the gap ensuing from the typically fixed nature of ground-based deployments, give context to collected measurements, enable better synthesis of ARM observations with satellite observations, and can provide boundary conditions for modeling studies constrained by ARM measurements. Here, we showcased only a limited number of analyses that can be performed by synergizing ARM measurements with ARMTRAJ datasets. The case study example emphasizes the value of ensemble statistics provided in ARMTRAJ datasets to evaluate uncertainties and the level of confidence in the trajectory model results. This case study also demonstrates that the level of confidence in trajectory calculations is case-specific but typically tends to decrease with the trajectory period and that conclusions drawn from a trajectory initialized at a single point can be misleading. We suggest that ensemble results should be preferred in most cases, especially when analyzing trajectories over periods of several days.
While we presented an analysis of ARMTRAJ datasets generated only for the recently completed EPCAPE field campaign, the implementation and application of ARMTRAJ will not end at that single site. ARMTRAJ recently reached an operational level such that datasets will begin production for past, ongoing, and future ARM deployments through the ARM infrastructure and will be continuously updated and made available via the ARM Data Discovery website, with near real-time production of fully annotated files.
Conceptualization, formal analysis, investigation, methodology, visualization, and original draft preparation: IS. Project administration: JMC. Data curation and validation: IS and MRK. Manuscript review and editing: IS, JMC, MRK, and LMR.
The contact author has declared that none of the authors has any competing interests.
Publisher’s note: Copernicus Publications remains neutral with regard to jurisdictional claims made in the text, published maps, institutional affiliations, or any other geographical representation in this paper. While Copernicus Publications makes every effort to include appropriate place names, the final responsibility lies with the authors.
The authors thank Krista Gaustad, Damao Zhang, John Shilling, Peng Wu, Jingjing Tian, and Scott Giangrande for valuable feedback. Data were obtained from the ARM user facility, a U.S. Department of Energy (DOE) Office of Science user facility managed by the Biological and Environmental Research (BER) program. The authors gratefully acknowledge the NOAA Air Resources Laboratory (ARL) for the provision of the HYSPLIT transport model used in this publication.
This research was supported by the ARM user facility, a U.S. Department of Energy (DOE) Office of Science user facility managed by the Biological and Environmental Research (BER) program. Pacific Northwest National Laboratory is operated for the U.S. Department of Energy by Battelle under contract DE-AC05-76RL01830.
This paper was edited by Luis Millan and reviewed by two anonymous referees.
Ali, S. M. and Pithan, F.: Following moist intrusions into the Arctic using SHEBA observations in a Lagrangian perspective, Q. J. Roy. Meteor. Soc., 146, 3522–3533, https://doi.org/10.1002/qj.3859, 2020.
Christensen, M. W., Jones, W. K., and Stier, P.: Aerosols enhance cloud lifetime and brightness along the stratus-to-cumulus transition, P. Natl. Acad. Sci. USA, 117, 17591–17598, https://doi.org/10.1073/pnas.1921231117, 2020.
Clothiaux, E. E., Miller, M. A., Perez, R. C., Turner, D. D., Moran, K. P., Martner, B. E., Ackerman, T. P., Mace, G. G., Marchand, R. T., Widener, K. B., Rodriguez, D. J., Uttal, T., Mather, J. H., Flynn, C. J., Gaustad, K. L., and Ermold, B.: The ARM Millimeter Wave Cloud Radars (MMCRs) and the Active Remote Sensing of Clouds (ARSCL) Value Added Product (VAP), ARM user facility, Pacific Northwest National Laboratory, Richland, WA, United States, https://doi.org/10.2172/1808567, 2001.
Day, D. A., Liu, S., Russell, L. M., and Ziemann, P. J.: Organonitrate group concentrations in submicron particles with high nitrate and organic fractions in coastal southern California, Atmos. Environ., 44, 1970–1979, https://doi.org/10.1016/j.atmosenv.2010.02.045, 2010.
Dorsey, K. S., Ireland, C. B., Mundy, M. E., Stafford, R. A., Palmer, K. K., Wasem, M. R., Prichard, M. E., Comstock, J. M., and Silber, I.: 2023 Atmospheric Radiation Measurement (ARM) Annual Report, United States, https://doi.org/10.2172/2281709, 2024.
Fairless, T., Jensen, M., Zhou, A., and Giangrande, S. E.: Interpolated Sounding and Gridded Sounding Value-Added Products, United States, https://doi.org/10.2172/1248938, 2021.
Forster, P., Storelvmo, T., Armour, K., Collins, W., Dufresne, J.-L., Frame, D., Lunt, D., Mauritsen, T., Palmer, M., Watanabe, M., Wild, M., and Zhang, H.: Chapter 7: The Earth's energy budget, climate feedbacks, and climate sensitivity, Victoria University of Wellington, https://doi.org/10.25455/wgtn.16869671.v1, 2021.
Gahtan, J., Knapp, K. R., Schreck III, C. J., Diamond, H. J., Kossin, J. P., and Kruk, M. C.: International Best Track Archive for Climate Stewardship (IBTrACS) Project, Version 4.01, NOAA National Centers for Environmental Information [data set], https://doi.org/10.25921/82ty-9e16, 2024.
Geerts, B., Giangrande, S. E., McFarquhar, G. M., Xue, L., Abel, S. J., Comstock, J. M., Crewell, S., DeMott, P. J., Ebell, K., Field, P., Hill, T. C. J., Hunzinger, A., Jensen, M. P., Johnson, K. L., Juliano, T. W., Kollias, P., Kosovic, B., Lackner, C., Luke, E., Lüpkes, C., Matthews, A. A., Neggers, R., Ovchinnikov, M., Powers, H., Shupe, M. D., Spengler, T., Swanson, B. E., Tjernström, M., Theisen, A. K., Wales, N. A., Wang, Y., Wendisch, M., and Wu, P.: The COMBLE Campaign: A Study of Marine Boundary Layer Clouds in Arctic Cold-Air Outbreaks, B. Am. Meteorol. Soc., 103, E1371–E1389, https://doi.org/10.1175/BAMS-D-21-0044.1, 2022.
Guenther, A., Hewitt, C. N., Erickson, D., Fall, R., Geron, C., Graedel, T., Harley, P., Klinger, L., Lerdau, M., Mckay, W. A., Pierce, T., Scholes, B., Steinbrecher, R., Tallamraju, R., Taylor, J., and Zimmerman, P.: A global model of natural volatile organic compound emissions, J. Geophys. Res.-Atmos., 100, 8873–8892, https://doi.org/10.1029/94JD02950, 1995.
Hawkins, L. N. and Russell, L. M.: Oxidation of ketone groups in transported biomass burning aerosol from the 2008 Northern California Lightning Series fires, Atmos. Environ., 44, 4142–4154, https://doi.org/10.1016/j.atmosenv.2010.07.036, 2010.
Hersbach, H., Bell, B., Berrisford, P., Hirahara, S., Horányi, A., Muñoz-Sabater, J., Nicolas, J., Peubey, C., Radu, R., Schepers, D., Simmons, A., Soci, C., Abdalla, S., Abellan, X., Balsamo, G., Bechtold, P., Biavati, G., Bidlot, J., Bonavita, M., Chiara, G. D., Dahlgren, P., Dee, D., Diamantakis, M., Dragani, R., Flemming, J., Forbes, R., Fuentes, M., Geer, A., Haimberger, L., Healy, S., Hogan, R. J., Hólm, E., Janisková, M., Keeley, S., Laloyaux, P., Lopez, P., Lupu, C., Radnoti, G., Rosnay, P. de, Rozum, I., Vamborg, F., Villaume, S., and Thépaut, J.-N.: The ERA5 global reanalysis, Q. J. Roy. Meteor. Soc., 146, 1999–2049, https://doi.org/10.1002/qj.3803, 2020.
Holdridge, D.: Balloon-Borne Sounding System (SONDE) Instrument Handbook, ARM-TR-029, DOE Office of Science, Office of Biological and Environmental Research, https://doi.org/10.2172/1020712, 2020.
Ilotoviz, E., Ghate, V. P., and Raveh-Rubin, S.: The Impact of Slantwise Descending Dry Intrusions on the Marine Boundary Layer and Air-Sea Interface Over the ARM Eastern North Atlantic Site, J. Geophys. Res.-Atmos., 126, e2020JD033879, https://doi.org/10.1029/2020JD033879, 2021.
Jensen, M., Giangrande, S., Fairless, T., and Zhou, A.: Interpolated Sonde (INTERPOLATEDSONDE), Atmospheric Radiat. Meas. ARM User Facil. [data set], https://doi.org/10.5439/1095316, 2021.
Jiang, H., Feingold, G., and Cotton, W. R.: Simulations of aerosol-cloud-dynamical feedbacks resulting from entrainment of aerosol into the marine boundary layer during the Atlantic Stratocumulus Transition Experiment, J. Geophys. Res.-Atmos., 107, AAC 20-1–AAC 20-11, https://doi.org/10.1029/2001JD001502, 2002.
Johnson, K., Giangrande, S., and Toto, T.: Active Remote Sensing of CLouds (ARSCL) product using Ka-band ARM Zenith Radars (ARSCLKAZR1KOLLIAS), Atmospheric Radiat. Meas. ARM User Facil. [data set], https://doi.org/10.5439/1393437, 2014.
Keeler, E., Burk, K., and Kyrouac, J.: Balloon-Borne Sounding System (SONDEWNPN), Atmospheric Radiat. Meas. ARM User Facil. [data set], https://doi.org/10.5439/1595321, 2022.
Kruk, M. C., Knapp, K. R., and Levinson, D. H.: A Technique for Combining Global Tropical Cyclone Best Track Data, J. Atmos. Ocean. Tech., 27, 680–692, https://doi.org/10.1175/2009JTECHA1267.1, 2010.
Kuang, C.: TSI Model 3936 Scanning Mobility Particle Spectrometer Instrument Handbook, United States, https://doi.org/10.2172/1245993, 2016.
Kuang, C., Singh, A., Howie, J., Salwen, C., and Hayes, C.: Scanning mobility particle sizer (AOSSMPS), Atmospheric Radiat. Meas. ARM User Facil. [data set], https://doi.org/10.5439/1476898, 2021.
Kyrouac, J., Shi, Y., and Tuftedal, M.: Surface Meteorological Instrumentation (MET), Atmospheric Radiat. Meas. ARM User Facil. [data set], https://doi.org/10.5439/1786358, 2021.
Läderach, A. and Sodemann, H.: A revised picture of the atmospheric moisture residence time, Geophys. Res. Lett., 43, 924–933, https://doi.org/10.1002/2015GL067449, 2016.
Lata, N. N., Zhang, B., Schum, S., Mazzoleni, L., Brimberry, R., Marcus, M. A., Cantrell, W. H., Fialho, P., Mazzoleni, C., and China, S.: Aerosol Composition, Mixing State, and Phase State of Free Tropospheric Particles and Their Role in Ice Cloud Formation, ACS Earth Space Chem., 5, 3499–3510, https://doi.org/10.1021/acsearthspacechem.1c00315, 2021.
Liu, S., Day, D. A., Shields, J. E., and Russell, L. M.: Ozone-driven daytime formation of secondary organic aerosol containing carboxylic acid groups and alkane groups, Atmos. Chem. Phys., 11, 8321–8341, https://doi.org/10.5194/acp-11-8321-2011, 2011.
Lu, X., Mao, F., Rosenfeld, D., Zhu, Y., Pan, Z., and Gong, W.: Satellite retrieval of cloud base height and geometric thickness of low-level cloud based on CALIPSO, Atmos. Chem. Phys., 21, 11979–12003, https://doi.org/10.5194/acp-21-11979-2021, 2021.
Mohrmann, J., Bretherton, C. S., McCoy, I. L., McGibbon, J., Wood, R., Ghate, V., Albrecht, B., Sarkar, M., Zuidema, P., and Palikonda, R.: Lagrangian Evolution of the Northeast Pacific Marine Boundary Layer Structure and Cloud during CSET, Mon. Weather Rev., 147, 4681–4700, 2019.
Morris, V. R.: Microwave radiometer (MWR) handbook, ARM-TR-016, DOE Office of Science, Office of Biological and Environmental Research, https://doi.org/10.2172/1020715, 2006.
Muradyan, P. and Coulter, R.: Micropulse Lidar (MPL) Handbook, PNNL, Richland, WA, https://doi.org/10.2172/1020714, 2020.
Neggers, R. A. J., Chylik, J., Egerer, U., Griesche, H., Schemann, V., Seifert, P., Siebert, H., and Macke, A.: Local and Remote Controls on Arctic Mixed-Layer Evolution, J. Adv. Model. Earth Sy., 11, 2214–2237, https://doi.org/10.1029/2019MS001671, 2019.
Palanisamy, G.: ARM Data File Standards Version 1.2, DOE ARM Climate Research Facility, Pacific Northwest National Laboratory, Richland, WA, United States, https://doi.org/10.2172/1253898, 2016.
Raes, F.: Entrainment of free tropospheric aerosols as a regulating mechanism for cloud condensation nuclei in the remote marine boundary layer, J. Geophys. Res.-Atmos., 100, 2893–2903, https://doi.org/10.1029/94JD02832, 1995.
Raes, F., Dingenen, R. V., Vignati, E., Wilson, J., Putaud, J.-P., Seinfeld, J. H., and Adams, P.: Formation and cycling of aerosols in the global troposphere, Atmos. Environ., 34, 4215–4240, https://doi.org/10.1016/S1352-2310(00)00239-9, 2000.
Ritsche, M.: ARM Surface Meteorology Systems Instrument Handbook, PNNL, Richland, WA, United States, https://doi.org/10.2172/1007926, 2011.
Russell, L. M., Lubin, D., Silber, I., Eloranta, E., Muelmenstaedt, J., Burrows, S., Aiken, A., Wang, D., Petters, M., Miller, M., Ackerman, A., Fridlind, A., Witte, M., Lebsock, M., Painemal, D., Chang, R., Liggio, J., and Wheeler, M.: Eastern Pacific Cloud Aerosol Precipitation Experiment (EPCAPE) Science Plan, United States, https://doi.org/10.2172/1804710, 2021.
Schmid, B. and Ivey, M.: ARM Unmanned Aerial Systems Implementation Plan, ARM Climate Research Facility, Pacific Northwest National Laboratory, Richland, WA, United States, report No. DOE/SC-ARM-16-054, https://www.osti.gov/servlets/purl/1332720 (last access: 30 October 2024), 2016.
Seidel, D. J., Zhang, Y., Beljaars, A., Golaz, J.-C., Jacobson, A. R., and Medeiros, B.: Climatology of the planetary boundary layer over the continental United States and Europe, J. Geophys. Res., 117, D17106, https://doi.org/10.1029/2012JD018143, 2012.
Shrivastava, M., Cappa, C. D., Fan, J., Goldstein, A. H., Guenther, A. B., Jimenez, J. L., Kuang, C., Laskin, A., Martin, S. T., Ng, N. L., Petaja, T., Pierce, J. R., Rasch, P. J., Roldin, P., Seinfeld, J. H., Shilling, J., Smith, J. N., Thornton, J. A., Volkamer, R., Wang, J., Worsnop, D. R., Zaveri, R. A., Zelenyuk, A., and Zhang, Q.: Recent advances in understanding secondary organic aerosol: Implications for global climate forcing, Rev. Geophys., 55, 509–559, https://doi.org/10.1002/2016RG000540, 2017.
Silber, I.: Back and forward trajectories for liquid-bearing cloud layers in full tropospheric profiles (ARMTRAJCLD), Atmospheric Radiat. Meas. ARM User Facil. [data set], https://doi.org/10.5439/2309851, 2024a.
Silber, I.: Back and forward trajectories for primary cloud decks based on ARSCL (ARMTRAJARSCL), Atmospheric Radiat. Meas. ARM User Facil. [data set], https://doi.org/10.5439/2309849, 2024b.
Silber, I.: Back trajectories for ARM surface deployments supporting aerosol studies (ARMTRAJSFC), Atmospheric Radiat. Meas. ARM User Facil. [data set], https://doi.org/10.5439/2309850, 2024c.
Silber, I.: Back trajectories for PBL and related aerosol and cloud studies (ARMTRAJPBL), Atmospheric Radiat. Meas. ARM User Facil. [data set], https://doi.org/10.5439/2309848, 2024d.
Silber, I. and Shupe, M. D.: Insights on sources and formation mechanisms of liquid-bearing clouds over MOSAiC examined from a Lagrangian framework, Elem. Sci. Anthr., 10, 000071, https://doi.org/10.1525/elementa.2021.000071, 2022.
Silber, I., Verlinde, J., Eloranta, E. W., Flynn, C. J., and Flynn, D. M.: Polar liquid cloud base detection algorithms for high spectral resolution or micropulse lidar data, J. Geophys. Res.-Atmos., 123, 4310–4322, https://doi.org/10.1029/2017JD027840, 2018.
Silber, I., Fridlind, A. M., Verlinde, J., Ackerman, A. S., Chen, Y.-S., Bromwich, D. H., Wang, S.-H., Cadeddu, M., and Eloranta, E. W.: Persistent Supercooled Drizzle at Temperatures below −25 °C Observed at McMurdo Station, Antarctica, J. Geophys. Res.-Atmos., 124, 10878–10895, https://doi.org/10.1029/2019JD030882, 2019.
Silber, I., Fridlind, A. M., Verlinde, J., Russell, L. M., and Ackerman, A. S.: Nonturbulent Liquid-Bearing Polar Clouds: Observed Frequency of Occurrence and Simulated Sensitivity to Gravity Waves, Geophys. Res. Lett., 47, e2020GL087099, https://doi.org/10.1029/2020GL087099, 2020.
Sivaraman, C., McFarlane, S., Chapman, E., Jensen, M., Toto (Fairless), T., Liu, S., and Fischer, M.: Planetary Boundary Layer Height (PBL) Value Added Product (VAP): Radiosonde Retrievals, ARM user facility, Pacific Northwest National Laboratory, Richland, WA, United States, https://doi.org/10.2172/1808688, 2013.
Sorooshian, A., Corral, A. F., Braun, R. A., Cairns, B., Crosbie, E., Ferrare, R., Hair, J., Kleb, M. M., Hossein Mardi, A., Maring, H., McComiskey, A., Moore, R., Painemal, D., Scarino, A. J., Schlosser, J., Shingler, T., Shook, M., Wang, H., Zeng, X., Ziemba, L., and Zuidema, P.: Atmospheric Research Over the Western North Atlantic Ocean Region and North American East Coast: A Review of Past Work and Challenges Ahead, J. Geophys. Res.-Atmos., 125, e2019JD031626, https://doi.org/10.1029/2019JD031626, 2020.
Stanford, M. W., Fridlind, A. M., Silber, I., Ackerman, A. S., Cesana, G., Mülmenstädt, J., Protat, A., Alexander, S., and McDonald, A.: Earth-system-model evaluation of cloud and precipitation occurrence for supercooled and warm clouds over the Southern Ocean's Macquarie Island, Atmos. Chem. Phys., 23, 9037–9069, https://doi.org/10.5194/acp-23-9037-2023, 2023.
Stein, A. F., Draxler, R. R., Rolph, G. D., Stunder, B. J. B., Cohen, M. D., and Ngan, F.: NOAA's HYSPLIT Atmospheric Transport and Dispersion Modeling System, B. Am. Meteorol. Soc., 96, 2059–2077, https://doi.org/10.1175/BAMS-D-14-00110.1, 2015.
Svensson, G., Murto, S., Shupe, M. D., Pithan, F., Magnusson, L., Day, J. J., Doyle, J. D., Renfrew, I. A., Spengler, T., and Vihma, T.: Warm air intrusions reaching the MOSAiC expedition in April 2020 – The YOPP targeted observing period (TOP), Elem. Sci. Anthr., 11, 00016, https://doi.org/10.1525/elementa.2023.00016, 2023.
Tornow, F., Ackerman, A. S., Fridlind, A. M., Cairns, B., Crosbie, E. C., Kirschler, S., Moore, R. H., Painemal, D., Robinson, C. E., Seethala, C., Shook, M. A., Voigt, C., Winstead, E. L., Ziemba, L. D., Zuidema, P., and Sorooshian, A.: Dilution of Boundary Layer Cloud Condensation Nucleus Concentrations by Free Tropospheric Entrainment During Marine Cold Air Outbreaks, Geophys. Res. Lett., 49, e2022GL098444, https://doi.org/10.1029/2022GL098444, 2022.
Troen, I. B. and Mahrt, L.: A simple model of the atmospheric boundary layer; sensitivity to surface evaporation, Bound.-Layer Meteorol., 37, 129–148, https://doi.org/10.1007/BF00122760, 1986.
Uin, J. and Smith, S.: Southern Great Plains (SGP) Aerosol Observing System (AOS) Instrument Handbook, United States, https://doi.org/10.2172/1756406, 2020.
van der Ent, R. J. and Tuinenburg, O. A.: The residence time of water in the atmosphere revisited, Hydrol. Earth Syst. Sci., 21, 779–790, https://doi.org/10.5194/hess-21-779-2017, 2017.
Verlinde, J., Zak, B. D., Shupe, M. D., Ivey, M. D., and Stamnes, K.: The ARM North Slope of Alaska (NSA) Sites, Meteorol. Monogr., 57, 8.1–8.13, https://doi.org/10.1175/AMSMONOGRAPHS-D-15-0023.1, 2016.
Vogelezang, D. H. P. and Holtslag, A. A. M.: Evaluation and model impacts of alternative boundary-layer height formulations, Bound.-Layer Meteorol., 81, 245–269, https://doi.org/10.1007/BF02430331, 1996.
Wernli, H., Boettcher, M., Joos, H., Miltenberger, A. K., and Spichtinger, P.: A trajectory-based classification of ERA-Interim ice clouds in the region of the North Atlantic storm track, Geophys. Res. Lett., 43, 6657–6664, https://doi.org/10.1002/2016GL068922, 2016.
Wiacek, A. and Peter, T.: On the availability of uncoated mineral dust ice nuclei in cold cloud regions, Geophys. Res. Lett., 36, L17801, https://doi.org/10.1029/2009GL039429, 2009.
Widener, K. and Bharadwaj, N.: C-Band Scanning ARM Precipitation Radar (C-SAPR) Handbook, ARM-TR-121, DOE Office of Science, Office of Biological and Environmental Research, https://doi.org/10.2172/1054629, 2012.
Widener, K. B., Bharadwaj, N., and Johnson, K.: Ka-Band ARM Zenith Radar (KAZR) Instrument Handbook, ARM-TR-106, DOE Office of Science, Office of Biological and Environmental Research, https://doi.org/10.2172/1035855, 2012a.
Widener, K. B., Bharadwaj, N., and Johnson, K.: Scanning ARM Cloud Radar (X/Ka/W-SACR), ARM-TR-113, DOE Office of Science, Office of Biological and Environmental Research, https://doi.org/10.2172/1043296, 2012b.
Woods, C. and Caballero, R.: The Role of Moist Intrusions in Winter Arctic Warming and Sea Ice Decline, J. Climate, 29, 4473–4485, https://doi.org/10.1175/JCLI-D-15-0773.1, 2016.
Zhang, D.: Planetary Boundary Layer Height (PBLHTSONDE1MCFARL), Atmospheric Radiat. Meas. ARM User Facil. [data set], https://doi.org/10.5439/1991783, 2021.
Zhang, D., Comstock, J., and Morris, V.: Comparison of planetary boundary layer height from ceilometer with ARM radiosonde data, Atmos. Meas. Tech., 15, 4735–4749, https://doi.org/10.5194/amt-15-4735-2022, 2022.
Zheng, G., Sedlacek, A. J., Aiken, A. C., Feng, Y., Watson, T. B., Raveh-Rubin, S., Uin, J., Lewis, E. R., and Wang, J.: Long-range transported North American wildfire aerosols observed in marine boundary layer of eastern North Atlantic, Environ. Int., 139, 105680, https://doi.org/10.1016/j.envint.2020.105680, 2020.