the Creative Commons Attribution 4.0 License.
the Creative Commons Attribution 4.0 License.
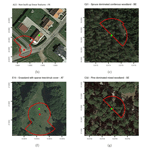
Advances in LUCAS Copernicus 2022: enhancing Earth observations with comprehensive in situ data on EU land cover and use
Raphaël d'Andrimont
Momchil Yordanov
Fernando Sedano
Astrid Verhegghen
Peter Strobl
Savvas Zachariadis
Flavia Camilleri
Alessandra Palmieri
Beatrice Eiselt
Jose Miguel Rubio Iglesias
Marijn van der Velde
The Land Use/Cover Area frame Survey (LUCAS) of the European Union (EU) presents a rich resource for detailed understanding of land cover and use, making it invaluable for Earth observation (EO) applications. This paper discusses the recent enhancements and improvements in the LUCAS Copernicus module, particularly the data collection process of 2022, its protocol simplifications, and geometry definitions compared to the 2018 survey and data. With approximately 150 000 polygons collected in 2022, an increase from 60 000 in 2018, the LUCAS Copernicus 2022 data provide a unique and comprehensive in situ dataset for EO applications. The protocol simplification also facilitates a faster and more efficient data collection process. In 2022, there were 137 966 polygons generated out of the original 149 408 LUCAS Copernicus points, which means that 92.3 % of the points were actually surveyed. The data have 82 land cover classes for the Copernicus module that map to 88 classes up to the LUCAS level-3 legend. For land use the data have 40 classes, along with 18 classes of land use types. The dataset is available for download (product IDentification – PID: http://data.europa.eu/89h/e3fe3cd0-44db-470e-8769-172a8b9e8874; European Commission, 2023). The paper elaborates further on the implications of these enhancements and the need for continuous harmonization to ensure semantic consistency and temporal usability of data across different periods. Moreover, it calls for additional studies exploring the potential of the collected data, especially in the context of remote sensing and computer vision. It ends with a discussion of future data usage and dissemination strategies.
- Article
(9517 KB) - Full-text XML
- Companion paper
- BibTeX
- EndNote
The importance of in situ data for Earth observation (EO) applications cannot be overstated. In situ observations provide ground-based reference data that are crucial for the production, validation, and calibration of remote sensing products derived from satellite or airborne observations. The two largest constraints on satellite-based model performance are training data and imagery (Burke et al., 2021). While imagery has become abundant, the scarcity and frequent unreliability of ground-based reference observation data make both training and validation of satellite-based models difficult. In particular, they are pivotal in assimilation practices to better inform Earth surface modeling and other EO endeavors (Balsamo et al., 2018). The Copernicus component of the European Union (EU) space program, known for its Earth observation capabilities, heavily relies on a vast array of in situ data. The cross-cutting coordination of Copernicus access to in situ data (https://insitu.copernicus.eu/, last access: 4 December 2024) provides support to entrusted entities in accessing such data for both the production and validation of Copernicus products.
Despite their significant value, the collection of low-uncertainty in situ data presents a myriad of challenges. The systematic collection of such data by humans is very resource-intensive, and ensuring the necessary quality and representativity for effective use in Earth observation applications further exacerbates the challenge (Teucher et al., 2022; Andries et al., 2022).
In the EU, a regularly surveyed sample of land cover (LC) and land use (LU) has been collected since 2006 in the framework of the Land Use/Cover Area frame Survey (LUCAS) (d'Andrimont et al., 2020). The data collected in this survey, especially the new LUCAS Copernicus module introduced in 2018, offer a remarkable source of in situ data. In 2018, this new LUCAS module (the Copernicus module) specifically tailored to EO was introduced (d'Andrimont et al., 2021a). A specific protocol was designed to collect in situ information with specific characteristics fitting EO processing requirements. As a result, a total of 58 428 polygons are provided with a level-3 land cover (66 specific classes including crop types) and land use (38 classes) information. This represents a unique set of in situ data, opening up the possibility of applications with higher thematic detail compared to previous LUCAS surveys, such as crop type mapping. This dataset has been used to generate continental mapping of crops with Sentinel-1 (d'Andrimont et al., 2021b) and Sentinel-2 (Ghassemi et al., 2022a; Luo et al., 2022) but also for land cover (Venter and Sydenham, 2021; Ghassemi et al., 2022b; Witjes et al., 2022), forest mapping (Bonannella et al., 2022), and land cover dynamics in watersheds (Beselly et al., 2021).
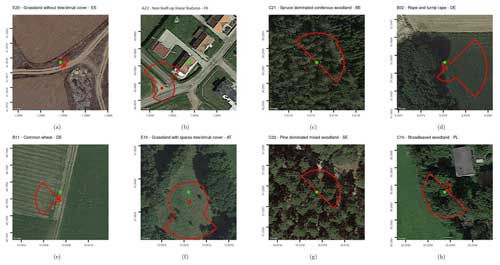
Figure 2Examples of LUCAS Copernicus polygons. The green point is the theoretical LUCAS point. The red point is the GPS location of the Copernicus surveyor. The polygons are built using distances in the northerly, easterly, southerly, and westerly directions collected on the ground. The background RGB imagery is obtained from map data from ©Google Maps 2023.
In 2022, a new survey and protocol were carried out. The advancements in the LUCAS Copernicus module, particularly the data collection process of 2022, its protocol simplifications, and geometry definitions compared to the 2018 survey and data, provide a substantial enhancement in the in situ dataset available for EO applications. This paper delves into these improvements and the implications thereof and discusses future data usage and dissemination strategies.
LUCAS is a two-phase sample survey. The LUCAS first-phase sample is a systematic selection of points on a grid with 2 km spacing in eastings and northings covering the whole of the EU's territory (Gallego and Bamps, 2008). Currently, it includes around 1.1 million points (Fig. 1) and is referred to as the master sample. Each point of the first-phase sample is classified into 1 of 10 land cover classes via visual interpretation of orthophotos or satellite images (ESTAT, 2018).
2.1 LUCAS 2018 survey
The LUCAS 2018 survey collected 97 variables at 337 854 points. Most of the points surveyed fall into a homogeneous area for which the minimum mapping unit is about 7 m2 (a circle with a 1.5 m radius). This homogeneity is first ascertained by way of orthophotos and affirmed or switched by a field survey afterwards. When the land cover is not homogeneous, e.g., when it is composed of trees or shrubs interspersed with grass, the scale of the observation is extended to classify it. In these cases, a systematic observation of the “environment” in the vicinity of the point, which in LUCAS is called the extended window of observation, has to be adopted. The extended window of observation expands to a radius of 20 m from the point (representing an area of 0.13 ha) for forest and shrublands. Detailed information about the survey can be found in Eurostat (2018a). The land cover surveyed is classified according to a harmonized three-level legend system (Eurostat, 2018b). In addition to the core variables collected, other specific modules were carried out on demand on a subset of points, such as (i) the topsoil module and (ii) the grassland module. The LUCAS Copernicus 2018 core data are available in a harmonized open database in d'Andrimont et al. (2020).
2.2 LUCAS Copernicus module
The LUCAS Copernicus 2018 module was applied to a subset of points from the 2018 survey to collect homogeneous land cover information up to an extent of 51 m until the land cover changed in the four cardinal directions around a point of observation. The exercise aimed to collect the area and shape of a pure and uninterrupted land cover, specifically multi-pixel in situ data compatible with the spatial resolution of high-resolution sensors (specifically Sentinel-1 and Sentinel-2; see d'Andrimont et al., 2021a, for the open ready-to-use dataset). The LUCAS Copernicus dataset contains 63 287 polygons that are supposed to represent the pure land cover at level 2 (genus). When filtering the data for which a level-3 (species) legend is available, 58 426 polygons with a level-3 land cover are available. Although the survey was designed with the idea of capturing pure land cover, every data collection exercise is prone to errors, such as wrong polygon labels, general geolocation errors or errors in extent in the cardinal directions. In order to arrive at a measure of homogeneity for a Copernicus polygon, one can weigh the pixel value by the fraction of the pixels that intersect the polygon (Meroni et al., 2021).
In 2022, the Copernicus module was simplified, as illustrated by the field form (Fig. A4). By removing some variables that were sampled in the 2018 protocol but that did not prove to be useful, the survey cost at each point could be reduced. Therefore, the total number of points could be increased to 150 000. The protocol requires the surveyor to register the LUCAS LC level 3 at the position they have reached. In contrast to the 2018 Copernicus module, this means that detailed information on, for example, permanent tree crops is also collected. The position reached can coincide with the LUCAS point or not due to physical, legal, or privacy barriers. Even when a surveyor cannot reach the LUCAS point or is even too far away to physically see it, they are normally able to collect Copernicus-relevant information via photointerpretation (PI), except when on a linear feature narrower than 3 m (e.g., tracks, grass margins, or similar), because this introduces excessive complexity. For most cases when doing PI in the field, it was generally possible to find a suitable location to carry out the survey.
An example of the data can be seen in Fig. 2, where the coverage of the polygons is visible on a recent Google basemap. The shapes of the polygons adequately capture the extent of the land cover. The small offsets between the positions of the theoretical and survey points can be explained by either GPS precision or the slight adjustments in the landscape by the surveyors. Of specific interest is Fig. 2b, which shows a point unreachable by the surveyor, as it is on private property. The surveyor in this case followed the Copernicus module protocol and completed the survey as it pertains to the physical location reached. We can see that the Copernicus land cover registered at this point ID (36782992) is A22 (“Non built-up linear features”), while the land cover of the theoretical point, as registered by the LC1 variable, is A11 (“Buildings with 1 to 3 floors”), which corresponds to the physical reality.
Table 1The number of LUCAS points at each step. The first column shows all the LUCAS 2022 points to be surveyed. The second column shows whether a point was originally selected as a LUCAS Copernicus point. The third column shows whether a Copernicus sample was actually taken (CANDO is the suffix of the column name). The final column shows the number of points for which it was possible to generate the radial geometry.

In the 2022 survey, 137 966 polygons were generated from validly collected survey data over EU-27 as shown in the map in Fig. 3. They were produced in varying amounts for 88 LC classes up to LUCAS legend level 3 as illustrated in Fig. 4. The clean version of these classes can be found in column lc1_code, whereas column survey_lc1 contains the original data, which we thought might also be interesting for users.
The mean size of the LUCAS Copernicus polygons is 0.35 ha, with the full distribution visible in Fig. 5. There are two tails at either end of the distribution – before the first quantile and after the third quantile. There is no obvious reason for the tails, meaning that no LC/LU class or country is overly represented within them, although many of the polygons larger than 0.8 ha are in the “Arable” land class, meaning used for agriculture. The parcels in the small ranges, specifically the 21 207 which are under 100 m2, are distributed throughout all the classes and member states. These should be used with caution and bearing in mind the ground-range resolution of the sensor used to extract the remote sensing data.
The crop samples of the survey are mapped in Fig. A2, and their distribution is in Fig. A3.
5.1 Comparison between 2018 and 2022
The changes between LUCAS Copernicus 2018 and 2022 are shown by land cover (Fig. 6a) and country (Fig. 6b). From Fig. 6a, we see that, for every LUCAS LC1, the number of samples has at least doubled. For some classes, like “Artificial Land” (A) and “Water” (G), there are many more samples – 91.6 % and 98.6 %, respectively.
A similar trend is observed for each country (NUTS0), where the number of samples has at least doubled and in some cases tripled (Belgium, Germany, Denmark, Greece, Ireland, Italy, the Netherlands, Slovenia, and Slovakia), except for the UK, which is no longer part of the survey. In essence, because of BREXIT, the quota of points available to the UK has been redistributed to the other member states.
5.2 Quadrilateral vs. radial polygon geometry
As shown in Fig. A1, in 2018 the polygons were erroneously generated using the distances noted by surveyors as the lengths of the line segments that make up the shapes of the irregular quadrilateral Copernicus polygons. In essence, this was a simplification of the actual survey design, which required the generation of radial quarter-arc slices in each cardinal direction that are to be merged together to form another irregular quasi-circular polygon shape. The difference between the two shapes obviously has an impact on the area of the polygon and hence on the number of Sentinel pixels that fall within it.
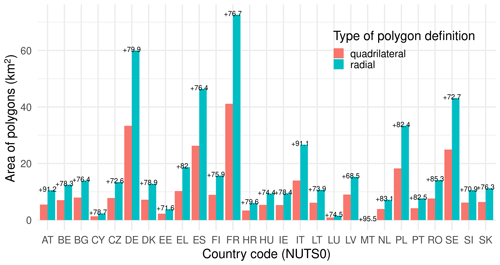
Figure 7Areas of LUCAS Copernicus polygons aggregated to the NUTS0 region, as derived based on the two types of polygon definitions. The number at the top of each bar group represents the increase (%) between the two.
The difference in area between the two types of polygon definitions (quadrilateral and radial) is shown in Fig. 7. The total area of the quadrilateral LUCAS Copernicus 2022 polygons is 274.6 km2, while for the radial definition the area is 489.4 km2, or an increase of 78.2 % for the entire EU-27. The maximum increase in area is registered in Malta (95.5 %) and the minimum in Latvia (68.5 %).
5.3 Assessment
A preliminary assessment of the dataset highlights the following key insights:
-
LUCAS Copernicus 2018 data proved themselves to be a valuable source of training and/or validation data for various operational programs, such as the Copernicus Land Monitoring Service (CLMS), Horizon 2020, Horizon Europe, and other associated activities. The improvements of the 2022 survey will further enhance this value by maximizing the pure land cover area irrespective of its size, having a more precise legend upon data collection, and sharing the data in a clear and open manner.
-
LUCAS point data can be used as a sampling and/or stratification scheme for selecting training and validation data that originate from elsewhere.
-
Sampling grid density, spatiotemporal coverage, temporal asynchrony from other CLMS products, geolocation precision issues during data collection, and legend-matching issues still prevent easy and straightforward use of LUCAS data for many applications (Schweitzer et al., 2023).
To produce the processing pipeline, the authors used both the R programming language, version 4.2.1, and PostgreSQL (13.0) with the PostGIS plugin (3.0.2). The code for producing the dataset from the raw LUCAS data and all the figures shown in the paper consists of four commented scripts – preprocessing, generating quadrilateral LUCAS polygons, generating radial LUCAS polygons, and producing figures and tables. The order in which these are executed is important, and they are numbered accordingly. The preprocessing includes the download of the micro data from the Eurostat website1. These, together with a README file, can be accessed here: https://jeodpp.jrc.ec.europa.eu/ftp/jrc-opendata/LUCAS/LUCAS_2022_Copernicus/ (d'Andrimont, 2024).
The produced dataset is provided in geopackage format and contains 121 relevant LUCAS attribute columns plus the radial geometry of the Copernicus polygons. The dataset is available here for download (Product IDentification – PID: http://data.europa.eu/89h/e3fe3cd0-44db-470e-8769-172a8b9e8874; European Commission, 2023). The data will also be available in Google Earth Engine after official publication. The connection between this dataset and previous LUCAS surveys or the harmonized product (d'Andrimont et al., 2020) is made via the “pointid” column. Although all the columns come from the data, the authors have added two additional columns – “survey_year” and “poly_area_sqm”. The first tracks which records come from which year of the survey, and the second is the calculated area in square meters.
A new simplified Copernicus protocol has been defined. Some notable improvements have been made, such as collecting the land cover level-3 data directly in the Copernicus polygons and increasing the pure land cover area. With 150 000 polygons collected in 2022, compared to 60 000 in 2018, the dataset provides unique in situ data for Earth observation applications. Because of some inherent differences between the survey years, whether in the form of the sampling design (Ballin et al., 2022) or differences in the classification of land cover or land use (Eurostat, 2022), further harmonization is needed in order to guarantee the semantic consistency of the coding and legend as well as the temporal inter-usability of both the 2018 and 2022 data. In the event of such a harmonization by the authors, they commit to publishing a new version of the data in a similar format and with all the relevant documentation. In terms of analysis, there is much to be done with the polygons themselves in the remote sensing context as well as the collection of ground photos that can be used for further computer vision work. From a data collection point of view, most issues with the surveys (FAQ from the surveyor) have been solved. Further discussions about the data use and diffusion are still needed.
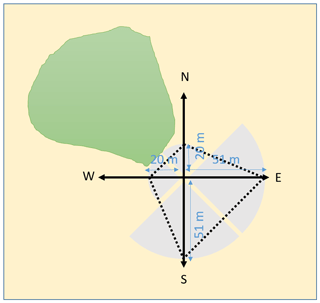
Figure A1Overview of how the polygons were built based on the radial distance collected on the ground. A distinction can be made between constructing a polygon based on quadrilateral distance, shown with dotted lines (as was done for the 2018 survey), and generating the radial quarter arcs, shown as gray polygons, corresponding to the field of view the observation should represent (as was done for the 2022 survey).
Rd'A, MvdV, and PS conceptualized the study. Rd'A and MY did the data curation, formal analysis and software and designed the methodology. FS, AV, and SZ helped with the investigation, resource allocation, and validation. FC, AP, BE, and JMRI provided much needed supervision for the peculiarities of the LUCAS study. All the authors contributed equally to the writing and review of the manuscript.
The contact author has declared that none of the authors has any competing interests.
The authors declare that the information and views set out in this article are theirs and do not necessarily reflect the official opinion of the institution that produced the data – Eurostat.
Publisher's note: Copernicus Publications remains neutral with regard to jurisdictional claims made in the text, published maps, institutional affiliations, or any other geographical representation in this paper. While Copernicus Publications makes every effort to include appropriate place names, the final responsibility lies with the authors.
The authors would like to acknowledge the invaluable efforts of the LUCAS field surveyors and the teams involved in the LUCAS database management at Eurostat. Their dedication and hard work have made the LUCAS Copernicus 2022 dataset possible. We would also like to thank the various project partners for their continuous support and feedback.
This paper was edited by Birgit Heim and reviewed by Kristof van Tricht and Žiga Malek.
Andries, A., Morse, S., Murphy, R. J., Lynch, J., and Woolliams, E. R.: Using data from earth observation to support sustainable development indicators: An analysis of the literature and challenges for the future, Sustainability, 14, 1191, https://doi.org/10.3390/su14031191, 2022. a
Ballin, M., Barcaroli, G., and Masselli, M.: New LUCAS 2022 Sample and Subsamples Design: Criticalities and Solutions, Publications Office of the European Union, Luxembourg, pp. 1–60, 2022. a
Balsamo, G., Agustí-Panareda, A., Albergel, C., Arduini, G., Beljaars, A., Bidlot, J., Blyth, E., Bousserez, N., Boussetta, S., Brown, A., Buizza, R., Buontempo, C., Chevallier, F., Choulga, M., Cloke, H. L., Cronin, M., Dahoui, M., de Rosnay, P., Dirmeyer, P. A., Drusch, M., Dutra, E., Ek, M., Gentine, P., Hewitt, H., Keeley, S. P. E., Kerr, Y. H., Kumar, S., Lupu, C., Mahfouf, J.-F., Mcnorton, J., Mogensen, K., Munoz-Sabater, J., Reichle, R., Orth, R., Mecklenburg, S., Rabier, F., Ruston, B., Pappenberger, F., Sandu, I., Seneviratne, S., Tietsche, S., Trigo, I. F., Uijlenhoet, R., Wedi, N., Woolway, R. I. I., and Zeng, X.: Satellite and in situ observations for advancing global Earth surface modelling: A Review, Remote Sens.-Basel, 10, 2038, https://doi.org/10.3390/rs10122038, 2018. a
Beselly, S., Lufira, R., and Andawayanti, U.: Seasonal Spatio-temporal Land Cover Dynamics in the Upper Brantas Watershed, IOP C. Ser. Earth Env., 930, 012021, https://doi.org/10.1088/1755-1315/930/1/012021, 2021. a
Bonannella, C., Hengl, T., Heisig, J., Parente, L., Wright, M. N., Herold, M., and De Bruin, S.: Forest tree species distribution for Europe 2000–2020: mapping potential and realized distributions using spatiotemporal machine learning, PeerJ, 10, e13728, https://doi.org/10.7717/peerj.13728, 2022. a
Burke, M., Driscoll, A., Lobell, D. B., and Ermon, S.: Using satellite imagery to understand and promote sustainable development, Science, 371, eabe8628, https://doi.org/10.1126/science.abe8628, 2021. a
d'Andrimont, R.: Processing pipeline for generating data, JRC [code], https://jeodpp.jrc.ec.europa.eu/ftp/jrc-opendata/LUCAS/LUCAS_2022_Copernicus/, last access: 5 December 2024. a
d'Andrimont, R., Yordanov, M., Martinez-Sanchez, L., Eiselt, B., Palmieri, A., Dominici, P., Gallego, J., Reuter, H. I., Joebges, C., Lemoine, G., and van der Velde, M.: Harmonised LUCAS in-situ land cover and use database for field surveys from 2006 to 2018 in the European Union, Sci. Data, 7, 1–15, 2020. a, b, c
d'Andrimont, R., Verhegghen, A., Meroni, M., Lemoine, G., Strobl, P., Eiselt, B., Yordanov, M., Martinez-Sanchez, L., and van der Velde, M.: LUCAS Copernicus 2018: Earth-observation-relevant in situ data on land cover and use throughout the European Union, Earth Syst. Sci. Data, 13, 1119–1133, https://doi.org/10.5194/essd-13-1119-2021, 2021a. a, b
d'Andrimont, R., Verhegghen, A., Lemoine, G., Kempeneers, P., Meroni, M., and Van der Velde, M.: From parcel to continental scale–A first European crop type map based on Sentinel-1 and LUCAS Copernicus in-situ observations, Remote Sens. Environ., 266, 112708, https://doi.org/10.1016/j.rse.2021.112708, 2021b. a
ESTAT: Technical reference document S1: Stratification Guidelines, Eurostat, https://ec.europa.eu/eurostat/documents/205002/8072634/LUCAS-2018-C1-Instructions.pdf (last access: 5 December 2024), 2018. a
European Commission, Joint Research Centre (JRC): LUCAS Copernicus 2022, European Commission, Joint Research Centre (JRC) [data set], http://data.europa.eu/89h/e3fe3cd0-44db-470e-8769-172a8b9e8874, 2023. a, b
Eurostat: Technical reference document C-1: Instructions for surveyors, Eurostat, https://ec.europa.eu/eurostat/documents/205002/8072634/LUCAS-2018-C1-Instructions.pdf (last access: 5 December 2024), 2018a. a
Eurostat: Technical reference document C-3: Classification, Eurostat, https://ec.europa.eu/eurostat/documents/205002/8072634/LUCAS2018-C3-Classification.pdf (last access: 5 December 2024), 2018b. a
Eurostat: Technical reference document C-3: Classification, Eurostat, https://ec.europa.eu/eurostat/documents/205002/13686460/C3-LUCAS-2022.pdf (last access: 5 December 2024), 2022. a
Gallego, J. and Bamps, C.: Using CORINE land cover and the point survey LUCAS for area estimation, Int. J. Appl. Earth Obs., 10, 467–475, 2008. a
Ghassemi, B., Dujakovic, A., Żółtak, M., Immitzer, M., Atzberger, C., and Vuolo, F.: Designing a european-wide crop type mapping approach based on machine learning algorithms using LUCAS field survey and sentinel-2 data, Remote Sens.-Basel, 14, 541, 2022a. a
Ghassemi, B., Immitzer, M., Atzberger, C., and Vuolo, F.: Evaluation of Accuracy Enhancement in European-Wide Crop Type Mapping by Combining Optical and Microwave Time Series, Land, 11, 1397, 2022b. a
Luo, Y., Zhang, Z., Zhang, L., Han, J., Cao, J., and Zhang, J.: Developing high-resolution crop maps for major crops in the european union based on transductive transfer learning and limited ground data, Remote Sens.-Basel, 14, 1809, 2022. a
Meroni, M., d'Andrimont, R., Vrieling, A., Fasbender, D., Lemoine, G., Rembold, F., Seguini, L., and Verhegghen, A.: Comparing land surface phenology of major European crops as derived from SAR and multispectral data of Sentinel-1 and-2, Remote Sens. Environ., 253, 112232, 2021. a
Schweitzer, K., Lindmayer, A., and Sorini, P.: LUCAS Assessment, Task B, Tech. Rep. 1.1, Space program of EU Copernicus, Frescatti, Rome, 2023. a
Teucher, M., Thürkow, D., Alb, P., and Conrad, C.: Digital In Situ Data Collection in Earth Observation, Monitoring and Agriculture—Progress towards Digital Agriculture, Remote Sens.-Basel, 14, 393, 2022. a
Venter, Z. S. and Sydenham, M. A.: Continental-scale land cover mapping at 10 m resolution over Europe (ELC10), Remote Sens.-Basel, 13, 2301, https://doi.org/10.3390/rs13122301, 2021. a
Witjes, M., Parente, L., van Diemen, C. J., Hengl, T., Landa, M., Brodskỳ, L., Halounova, L., Križan, J., Antonić, L., Ilie, C. M., Craciunescu, V., Kilibarda, M., Antonijević, O., and Glušica, L.: A spatiotemporal ensemble machine learning framework for generating land use/land cover time-series maps for Europe (2000–2019) based on LUCAS, CORINE and GLAD Landsat, PeerJ, 10, e13573, https://doi.org/10.7717/peerj.13573, 2022. a
The micro data were obtained from https://ec.europa.eu/eurostat/documents/205002/17561401/EU_LUCAS_2022.zip, last access: 1 November 2023. For the latest version of the data, please refer to the Eurostat website.