the Creative Commons Attribution 4.0 License.
the Creative Commons Attribution 4.0 License.
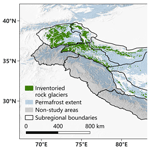
TPRoGI: a comprehensive rock glacier inventory for the Tibetan Plateau using deep learning
Zhangyu Sun
Adina Racoviteanu
Stephan Harrison
Xiaowen Wang
Jiaxin Cai
Xin Guo
Yujun He
Hailun Yuan
Rock glaciers – periglacial landforms commonly found in high-mountain systems – are of significant scientific value for inferring the presence of permafrost, understanding mountain hydrology, and assessing climate impacts on high-mountain environments. However, inventories remain patchy in many alpine regions, and as a result they are poorly understood for some areas of High Mountain Asia such as the Tibetan Plateau. To address this gap, we compiled a comprehensive inventory of rock glaciers for the Tibetan Plateau, i.e., TPRoGI (v1.0), developed using an innovative deep learning method. This inventory consists of a total of 44 273 rock glaciers, covering approximately 6000 km2, with a mean area of 0.14 km2. They are predominantly situated at elevations ranging from 4000 to 5500 m a.s.l., with a mean of 4729 m a.s.l. They tend to occur on slopes with gradients between 10 and 25°, with a mean of 17.7°. Across the plateau, rock glaciers are widespread in the northwestern and southeastern areas, with dense concentrations in the Western Pamir and Nyainqêntanglha, while they are sparsely distributed in the inner part. Our inventory serves as a benchmark dataset, which will be further maintained and updated in the future. This dataset constitutes a significant contribution towards understanding, future monitoring, and assessment of permafrost on the Tibetan Plateau in the context of climate change. The dataset is available at https://doi.org/10.5281/zenodo.10732042 (Sun et al., 2024a).
- Article
(10403 KB) - Full-text XML
-
Supplement
(22429 KB) - BibTeX
- EndNote
The Tibetan Plateau, the highest and largest plateau on Earth, is experiencing more pronounced warming than the global average. Currently, the warming rate on the plateau is 0.031 °C yr−1, higher than the global rate of 0.014 °C yr−1 since the 1960s (Zhang et al., 2020). Moreover, areas underlain by permafrost on the plateau have experienced an even higher warming rate of 0.05 °C yr−1 since 2004 (Zhao and Sheng, 2019). This accelerated warming trend has led to rapid degradation of permafrost, which is manifested as increasing ground temperature, decreasing permafrost area, thickening active layer, and increasing occurrence of thermokarst lakes and thaw slumps (Zhao et al., 2020; Mu et al., 2020). A valuable indicator of permafrost comes in the form of rock glaciers, defined as “debris landforms generated by the former or current creep of frozen ground (permafrost), detectable in the landscape with the following morphologies: front, lateral margins, and optionally ridge-and-furrow surface topography” (RGIK, 2023). These landforms are widespread across the plateau, especially in the mountainous regions.
Understanding of rock glaciers within the scientific community has been evolving since the publication of the initial article by Spencer (1900) on “a peculiar form of talus”. Over the past century, the identification of rock glaciers has been the subject of ongoing debate, and the criteria for identifying them have evolved with an increasing number of studies worldwide (Capps, 1910; Barsch, 1996; Haeberli et al., 2006; Berthling, 2011; Jones et al., 2019b; Janke and Bolch, 2021). In the last decade, the identification and compilation of rock glacier inventories has sparked heated debate due to the intricate nature of these landforms (Berthling, 2011; Brardinoni et al., 2019). In response to the challenge posed by inconsistencies in the identification and compilation of rock glaciers, the International Permafrost Association (IPA) Action Group Rock Glacier Inventories and Kinematics (RGIK) was established in 2018 with the aim of developing widely accepted guidelines for rock glacier inventorying, thereby fostering a globally consistent and comprehensive approach to rock glacier inventories (Delaloye et al., 2018; RGIK, 2023). Through the efforts of RGIK, the baseline and practical guidelines have been documented and updated in several versions, which greatly promote the global assemblage and uniform completion of rock glacier inventories (RGIK, 2023). This paper closely follows the RGIK guidelines in the conceptual definition of rock glaciers.
Rock glaciers are important to map and monitor for several reasons. First, they serve as visible indicators of frozen ground and provide essential information about the presence and extent of mountain permafrost (Barsch, 1996; Haeberli, 2006). Therefore, they are valuable for assessing the permafrost distribution (Boeckli et al., 2012; Schmid et al., 2015; Hassan et al., 2021; Li et al., 2024). Previous studies have used rock glacier inventories to construct permafrost maps in different regions. For instance, Boeckli et al. (2012) developed the Alpine Permafrost Index Map for the European Alps by calibrating a statistical model with rock glacier inventories. Schmid et al. (2015) used rock glaciers mapped from Google Earth to validate permafrost maps in the Hindu Kush Himalaya region. Similarly, Hassan et al. (2021) and Li et al. (2024) used rock glacier inventories to model the permafrost probability distribution in their study areas. Second, rock glaciers are an integral component of mountain hydrological system, especially in arid regions (Corte, 1976; Azócar and Brenning, 2010; Rangecroft et al., 2013, 2015; Munroe, 2018), which is a potentially significant water resource that remains poorly quantified. Jones et al. (2021a) estimated that 62.02 ± 12.40 Gt of water volume equivalent (WVEQ) is stored within rock glaciers globally. The ratio of rock-glacier-to-glacier WVEQ was estimated to be 1:618, which is expected to further increase with the ongoing melting of glaciers (Jones et al., 2021a). Given the arid conditions of much of the western Tibetan Plateau, the inventory of rock glaciers is critical for assessing potential water resources in these regions. Third, the kinematic behavior of rock glaciers is sensitive to changes in permafrost temperature and pore-water pressure, which are influenced by climate forcing such as air temperature and precipitation (e.g., Arenson et al., 2002; Arenson and Springman, 2005; Cicoira et al., 2019a, b). Numerous studies have demonstrated a decadal to multi-decadal acceleration trend in rock glacier velocity in many regions such as the European Alps (e.g., Delaloye et al., 2010; Marcer et al., 2021), northern Tien Shan (Kääb et al., 2021), and the Andes (Vivero et al., 2021). Based on these global trends, rock glacier velocity (RGV) has been added as a new product of the essential climate variable (ECV) permafrost by the Global Climate Observing System (Zemp et al., 2022). Fourth, rapid movement or destabilization of rock glaciers can trigger geohazards such as rockfalls, debris flows, and lake outbursts, posing a potential risk to nearby human infrastructure and facilities (Janke and Bolch, 2021; Marcer et al., 2021).
A full understanding of the role of rock glaciers on permafrost distribution, mountain hydrology, and hazards in regions such as the Tibetan Plateau is currently hampered by a lack of comprehensive and systematic inventories. Compiling a comprehensive inventory constitutes the first step towards monitoring the long-term evolution of rock glaciers and understanding the changes of mountain permafrost under climate change. In recent years, rock glacier inventories in several local areas on the Tibetan Plateau have been established by visually interpreting optical images of different sources, and in some cases, interferometric synthetic aperture radar (InSAR) maps were used (Jones et al., 2018, 2021b; Ran and Liu, 2018; Hassan et al., 2021; Reinosch et al., 2021; Cai et al., 2021; Zhang et al., 2021, 2022, 2023; Bolch et al., 2022; Hu et al., 2023, 2024; Li et al., 2024) (see Table S1 in the Supplement). However, the coverage remains patchy, and a plateau-wide open-access inventory compiled from a consistent set of images using a systematic methodology is currently still lacking – hence the purpose of this study.
However, the production of a rock glacier inventory through visual interpretation requires strong geomorphological expertise and is labor-intensive and time-consuming (Barsch, 1996; RGIK, 2023). Rock glaciers exhibit spectral properties similar to their surrounding environment, making it challenging to identify in optical remote sensing images (Robson et al., 2020). Moreover, in high-mountain environments, there are various landforms that resemble rock glaciers, such as debris-covered glaciers, rock avalanches, debris flows, and fluvial landforms (Haeberli et al., 2006; Robson et al., 2020). As a result, inexperienced analysts are prone to making erroneous judgments. With the development of artificial intelligence, deep learning models have become valuable tools for mapping complex landforms such as rock glaciers. Deep learning models are able to learn the visual patterns of objects and to identify features in previously unseen images with high accuracy (LeCun et al., 2015; Huang et al., 2020). In recent years, several studies have successfully employed deep learning techniques for the automatic detection of rock glaciers, yielding satisfactory results (Feng et al., 2019; Robson et al., 2020; Marcer, 2020; Xu et al., 2021; Erharter et al., 2022; Hu et al., 2023). However, the methods employed in previous studies are not systematic over large areas, leading to inconsistencies and patchy coverage.
In this study, we created the most extensive plateau-wide inventory of rock glaciers on the Tibetan Plateau, i.e., TPRoGI (v1.0), using a deep learning method based on the DeepLabv3+ model. It is expected that the benchmark dataset produced by this study will be maintained and updated in the future and will facilitate investigations into many scientific questions related to rock glaciers and mountain permafrost on the Tibetan Plateau.
The Tibetan Plateau is part of High Mountain Asia, covering an area of approximately 2.5×106 km2 with an average elevation of over 4500 m above sea level (Royden et al., 2008). The Tibetan Plateau has a continental climate dominated by the Indian monsoon, the East Asian monsoon, and the westerlies. The monsoon brings warm and moist air in summer, while the westerlies bring dry and cold air in winter. The interaction between monsoons and westerlies causes distinct seasonal climate variations and significant diurnal temperature differences on the Tibetan Plateau (Yao et al., 2012). Due to its high altitude and extreme weather conditions, the Tibetan Plateau has the largest cryosphere extent outside the Arctic and Antarctic regions and the largest area of permafrost terrain in the mid- and low-latitude regions (Zou et al., 2017).
Bolch et al. (2019) split High Mountain Asia into 22 subregions based on their topographical and climatological characteristics, of which 13 were situated on the Tibetan Plateau. We selected all 13 subregions as study areas for this work, thus covering most of the Tibetan Plateau (Fig. 1), as well as the Qaidam basin, which was not a subregion in the study by Bolch et al. (2019).
3.1 Planet Basemaps
We used a large amount of optical imagery from high-resolution satellite data, i.e., Planet Basemaps, as training images. Planet Labs Inc. generates the Planet Basemaps product using imagery and data from its fleet with over 200 Earth-imaging satellites (Nass et al., 2019). The three-band (red, green, blue) imagery contain well-processed, scientifically accurate, and analysis-ready mosaics with 4.77 m spatial resolution, visual consistency, and cloud mitigation (Nass et al., 2019). The visual consistency of Planet Basemaps is crucial for developing a comprehensive map of rock glaciers over broad regions. Furthermore, we chose images from a single sensor to ensure consistent quality and timestamp of the source images. To train the deep learning model and infer new rock glaciers, we mostly utilized the Planet Basemaps mosaics from the third quarter of 2021 (July–September 2021), supplemented with images from the fourth quarter of 2021 (October–December 2021) when needed to mitigate image quality problems in the third-quarter images, such as shadows and image distortion.
3.2 Existing rock glacier local inventories for training
To create a set of robust and diverse training data, we compiled existing rock glacier local inventories from multiple regions. Utilizing a multi-source approach helps increase the volume and diversity of the training dataset while mitigating the subjectivity and possible biases introduced by individual inventories. To incorporate more high-quality data, we included rock glaciers not only from the Tibetan Plateau but also from other regions, with a total of six local inventories comprising both intact and relict rock glaciers (Table 1).
Prior to generating the final training dataset, we performed a quality control to account for the various source images and compilation strategies employed among these inventories. As a result, we manually checked and modified rock glacier boundaries by overlaying and visually checking the previously inventoried rock glaciers on our Planet Basemaps images. For example, rock glaciers that were difficult to recognize at places where the image quality was poor or covered by shadows were removed; when we identified missing rock glaciers in previous inventories, these were manually added. Since the front is a critical feature of a rock glacier, we followed the RGIK guidelines to use the extended geomorphological footprints to delineate rock glacier training samples (RGIK, 2023). We finally collected 4085 rock glacier polygons as training samples.
3.3 Topo-climatic datasets
To analyze the patterns of rock glacier distribution and the associated environmental factors, we used several topo-climatic datasets, including (1) the 30 m resolution National Aeronautics and Space Administration Digital Elevation Model (NASADEM) (Crippen et al., 2016), (2) the 0.1°×0.1° monthly mean annual air temperature (MAAT) data from January 1982 to the present derived from the Noah 3.6.1 model in the Famine Early Warning Systems Network (FEWS NET) Land Data Assimilation System (FLDAS) (McNally and NASA/GSFC/HSL, 2018), (3) the mean annual ground temperature (MAGT) data from 2000 to 2016 at 1 km spatial resolution produced by Obu et al. (2018), and (4) the 0.1°×0.1° monthly precipitation data from 2001 to 2020 from the Integrated Multi-satellitE Retrievals for GPM (IMERG) (Huffman et al., 2023).
3.4 Auxiliary data
We also incorporated additional data sources including Google Earth images, ESRI base maps, information on glacier distributions, and information on permafrost distributions. Google Earth images and ESRI base maps were used as supplementary data to aid in the identification and validation of rock glaciers by using high-resolution images (Yu and Gong, 2012). For the clean glacier and debris-covered glacier data, we utilized the widely recognized Randolph Glacier Inventory (RGI v6.0), which provides global coverage of glacier outlines (Pfeffer et al., 2014). The RGI offers a valuable reference for distinguishing rock glaciers from adjacent glaciers. Regarding permafrost extent, we relied on the map for the Northern Hemisphere produced by Obu et al. (2018).
4.1 Deep-learning-based rock glacier mapping approach
We propose a systematic deep-learning-based approach for mapping rock glaciers on the Tibetan Plateau. The workflow of the mapping approach is illustrated in Fig. 2. The mapping process comprises two primary stages: (i) deep learning mapping and (ii) manual improvement, which will be elaborated on in the following subsections.
4.1.1 Deep learning mapping
DeepLabv3+, introduced by Chen et al. (2018), was selected as the neural network architecture for the deep learning model, with Xception71 serving as its backbone (Chollet, 2017). DeepLabv3+ is specifically designed for semantic segmentation tasks and has proven to excel in mapping permafrost landforms (Huang et al., 2020; Hu et al., 2023). Xception71 is a convolutional neural network architecture consisting of 71 layers and encompasses approximately 42 million parameters (Chollet, 2017).
Our deep learning model takes a three-channel image with red, green, and blue (RGB) bands as input and outputs a binary image indicating the occurrence of rock glaciers. The topographic information such as slope or elevation was not used as this model only accepts three image bands as input. For the model training, 70 % of rock glacier boundaries from the six local inventories were extracted, with the remaining 30 % kept for validation. The intersection over union (IoU) was employed as the accuracy metric of validation, which is defined as:
where A denotes the mapped polygon and B is the reference polygon. The IoU scores range from 0 to 1, and a higher value indicates a higher accuracy (Huang et al., 2020).
We supplemented our training dataset with negative samples, incorporating non-rock-glacier polygons to address potential misclassifications by the deep learning model, particularly when encountering landforms exhibiting similar characteristics to rock glaciers. These non-rock-glacier polygons encompass diverse features such as debris-covered glaciers, rock avalanches, and water bodies. To incorporate contextual information from the surrounding area of a rock glacier, we established a buffer area to extract a subset of Planet Basemaps images. A too small buffer area may fail to provide sufficient contextual information, resulting in an increased occurrence of false positives, while an overly large buffer area risks inundating the model with excessive background information, potentially compromising its ability to accurately detect rock glaciers (Huang et al., 2018, 2020). We conducted experiments with buffer area widths ranging from 500 to 2000 m. The results showed no significant increase in the IoU metric once the buffer area exceeded 1500 m. Therefore, we selected 1500 m as the buffer size. The subset of images was then subdivided into patches of 480×480 pixels with an overlap of 160 pixels. The binary label patches were created by rasterizing rock glacier polygons (Huang et al., 2020).
Once trained, the deep learning model was validated using images across the Tibetan Plateau. We determined true positives (TPs), false positives (FPs), and false negatives (FNs); then we calculated the precision, recall, and F1 score using the following equations (Huang et al., 2020):
Since the predicted polygons are subject to uncertainties due to varying qualities of imagery, training inventories, and model accuracy, initial results are referred to as “candidate rock glacier polygons”. These polygons did not constitute the final rock glacier inventory but rather served as the preliminary detection of rock glaciers, along with the locations and boundaries, which were then refined as described below.
4.1.2 Manual improvement and independent validation
To ensure the accuracy and reliability of the dataset, a manual checking and improving process was carried out on the candidate rock glacier polygons. By utilizing these polygons as a starting point, the subsequent manual compilation efforts were significantly streamlined. The manual improvement process followed the standard guidelines recommended by the IPA Action Group RGIK (RGIK, 2023). According to these guidelines, the mapped rock glaciers were visually checked based on specific geomorphological features, notably the visible accumulation of talus material at the front and the presence of a lateral extension of this talus material along the sides of the rock glacier. Additionally, certain rock glaciers may exhibit noticeable convex-downslope or longitudinal-surface undulations, creating a ridge-and-furrow topography. We considered the extended footprints of rock glaciers while restricting the horizontal distance between the upper front edge and the frontal talus base within 50 m to exclude the possible exaggerated front. Following the global glacier inventory standards and given the resolution limitations of Planet Basemaps (4.77 m), rock glaciers smaller than 10 000 m2 (0.01 km2) were excluded from the inventory (RGIK, 2023).
We proposed four “r” operations to manually check the rock glacier candidate polygons:
-
remain – no operation if the polygon accurately outlines the rock glacier,
-
remove – remove the polygon if it is not a rock glacier,
-
refine – modify the polygon if it was correctly identified as a rock glacier but the boundary was not correctly outlined,
-
retrieve – add a missing rock glacier and outline its boundary.
The “remove” operation is designed to exclude landforms that have been incorrectly identified as rock glaciers by the deep learning model. These misidentified landforms commonly include debris-covered glaciers and rock avalanches. Debris-covered glaciers are glaciers that are partially covered by variable layers of debris (from a few centimeters to 2 m) and are characterized by supraglacial features such as thermokarst features, supraglacial lakes, streams, and ice cliffs (Jones et al., 2019a; Racoviteanu et al., 2022; RGIK, 2023). Outlines from the RGI v6.0 inventory were used to visually remove polygons overlapping debris-covered glaciers. Rock avalanches, on the other hand, are composed of fragmented rocks that flow downhill, following large rock slope failures (Hungr et al., 2014). Unlike rock glaciers, rock avalanches typically lack any discernible pattern or order on the surface. The “refine” operation involves the manual editing of the deep-learning-predicted rock glacier outlines to ensure that the polygon boundaries closely matched the observed boundaries of rock glaciers in the images. The “retrieve” operation serves the purpose of adding missing rock glaciers to the inventory. Some rock glaciers can be overlooked by the deep learning model, due to either their subtle features or their low-quality image data. When missing rock glaciers were identified during the manual inspection of nearby candidate polygons, they were added to the inventory. Furthermore, in high-mountain environments, the convergence of multiple rock glacier units into a complex system is a frequent occurrence (RGIK, 2023). However, the deep learning model often tends to predict this system as a singular rock glacier. To anticipate such issues, we manually separated the system into smaller rock glacier units if their lateral boundaries were clearly observed in Planet Basemaps images.
Our team consisted of seven mappers and two independent reviewers. Each candidate rock glacier polygon was manually examined and refined by visual interpretation of Planet Basemaps images following the four “r” operations by each mapper. In cases where the features of rock glaciers were uncertain and not clearly observable in Planet Basemaps images, high-resolution Google Earth images and ESRI base maps were utilized for more accurate visual inspection and analysis. An extended footprint of each rock glacier was yielded, from which we generated the primary marker, which is a point identifying a unique rock glacier unit or system (RGIK, 2023).
We proceeded with an independent validation process to assess the quality of the revised inventory. Given the difficulty in accurately evaluating the delineated boundaries, our validation focused primarily on verifying the primary markers. To conduct this validation, we randomly selected 2110 samples (approximately 5 % of the primary markers). Two independent reviewers examined all the selected samples using Google Earth images. Based on their independent assessments, each reviewer provided one of four decisions: “yes”, indicating that the rock glaciers were correctly identified; “no”, suggesting an incorrect identification; “uncertain”, denoting a lack of certainty in the identification; or “undefined”, used when the examined rock glaciers could not be clearly observed due to factors such as heavy snow cover, shadows, or the unavailability of high-quality images.
4.2 Adding attributes of the final revised rock glaciers
According to the IPA RGIK guidelines, there are three mandatory attributes for a rock glacier unit (RGU): the primary ID, the associated rock glacier system (RGS), and the metadata (RGIK, 2023). In our inventory, the attribute “ID” is equivalent to the primary ID, which is formed by combining “RGU” with the WGS84 coordinates of the rock glacier, expressed in decimal degrees with four digits (RGIK, 2023). We were unable to provide the RGS information in our current inventory due to image resolution limitations and instance segmentation issues. We have included the metadata attribute, which contains information of source data, date of mapping, mapper's name, reviewer's name, and additional information, which are separately stored in SOUR_DATA, MAP_DATE, MAPPER, REVIEWER, and ADDI_INF attributes (RGIK, 2023). The ADDI_INF provides information on whether the rock glacier has been recognized as a false identification by the reviewers. Furthermore, we computed the geomorphic and climatic attributes of each inventoried rock glacier to analyze their spatial distribution characteristics and the associated topo-climatic conditions. We derived the rock glacier area based on the polygon extent. The NASADEM was used to calculate the elevation, slope, and aspect of the rock glaciers (Crippen et al., 2016). The climatic information, including MAAT, MAGT, and annual precipitation, of each rock glacier was extracted from the climatic data. We also calculated the annual potential incoming solar radiation (PISR) using the model described by Kumar et al. (1997). Table 2 lists all the attributes of the inventory.
Table 2Attribute data dictionary for the Tibetan Plateau rock glacier inventory shapefile.
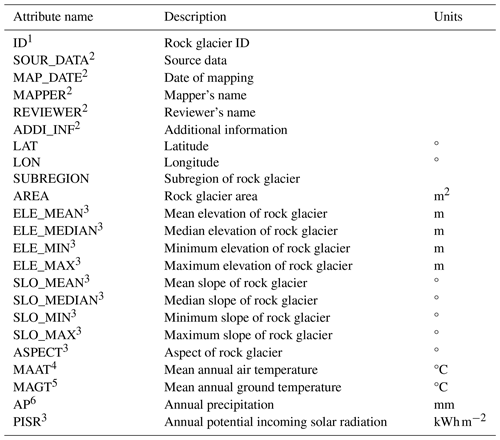
1 ID is identical to the primary ID attribute in the IPA RGIK guidelines.
2 SOUR_DATA, MAP_DATE, MAPPER, REVIEWER, and ADDI_INF contain the information of metadata attribute in the IPA RGIK guidelines.
3 ELE_MEAN, ELE_MEDIAN, ELE_MIN, ELE_MAX, SLO_MEAN, SLO_MEDIAN, SLO_MIN, SLO_MAX, ASPECT, and PISR are attributed based on the 30 m resolution National Aeronautics and Space Administration Digital Elevation Model (NASADEM) (Crippen et al., 2016) (https://search.earthdata.nasa.gov/search, last access: 3 December 2024).
4 MAAT is attributed based on the 0.1°×0.1° monthly mean annual air temperature (MAAT) data from January 1982 to the present derived from the Noah 3.6.1 model in the Famine Early Warning Systems Network (FEWS NET) Land Data Assimilation System (FLDAS) (McNally and NASA/GSFC/HSL, 2018) (https://disc.gsfc.nasa.gov/datasets/FLDAS_NOAH01_C_GL_M_001/summary?keywords=MERRA-2%20and%20CHIRPS, last access: 3 December 2024).
5 MAGT is attributed based on mean annual ground temperature (MAGT) data from 2000 to 2016 at 1 km spatial resolution produced by Obu et al. (2018) (https://apgc.awi.de/dataset/pex, last access: 3 December 2024).
6 AP is attributed based on the 0.1°×0.1° monthly precipitation data from 2001 to 2020 from the Integrated Multi-satellitE Retrievals for GPM (IMERG)
(https://doi.org/10.5067/GPM/IMERG/3B-MONTH/07, Huffman et al., 2023).
4.3 Spatial analysis of rock glaciers
To investigate the spatial distribution characteristics of rock glaciers on the Tibetan Plateau, we conducted statistical analyses of their geomorphic features within a 50 km×50 km grid cell. In each cell, we counted the number of rock glaciers and calculated the average values for their areas, minimum elevations, and slopes. We also analyzed the distribution patterns of their aspects in different subregions.
Across the entire study area, the deep learning model predicted a total of 48 767 candidate rock glacier polygons (Fig. S1 in the Supplement). After the manual improvement (see Sect. 4.1.2), we produced an inventory consisting of 44 273 rock glaciers (Fig. 5 and further described in Sect. 5.2 and 5.3). Below, we first present the validation of our results from three perspectives: (i) validation of the deep learning model based on training and validation datasets (Sect. 5.1.1), (ii) validation of the deep-learning-predicted rock glacier outlines based on manually improved rock glaciers used as our ground truth (Sect. 5.1.1), and (iii) independent validation of inventoried rock glaciers based on visual examination (Sect. 5.1.2).
5.1 Performance of deep-learning-based rock glacier mapping approach
5.1.1 Deep learning model performance and output
Figure 3a shows the IoU scores achieved by the deep learning model during the training and validating processes. Initially, both the training and validation IoU scores exhibit an upward trend, followed by a gradual stabilization. By the last iteration, the model achieved an IoU score of 0.76 on the training dataset and 0.70 on the validation dataset, indicating that the model learned effectively from the training data and generalized well to the validation data.
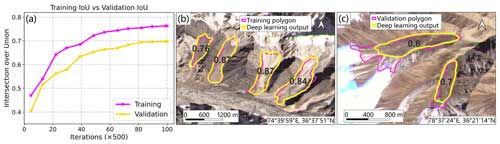
Figure 3(a) IoU scores during the training and validation processes. Examples of the candidate rock glaciers are shown in (b) training and (c) validation regions using the well-trained deep learning model. The IoU scores are labeled on the mapped rock glaciers.
To further evaluate the model performance, we applied the well-trained model to predict the rock glacier boundaries on both the training and validation datasets. The deep learning model accurately captured rock glacier characteristics within the training dataset, as evidenced by the close alignment between the predicted boundaries and the training polygons (Fig. 3b). Figure 3c further confirms that the model could generalize well to new datasets, with good agreement between predicted boundaries and validation polygons. However, difficulties in mapping rooting regions led to misalignment in those areas (Brardinoni et al., 2019).
Table 3 presents the calculated recall, precision, and F1 score of the deep learning mapped polygons for each subregion, as well as for the entire study area. Over the entire study area, the F1 score was 0.63, which we consider satisfactory for rock glacier mapping. The highest performance was for the Hengduan Shan (F1=0.76), with F1 scores of the Eastern Pamir, Karakoram, Nyainqêntanglha, Western Kunlun Shan, Western Pamir, and Hengduan Shan subregions above 0.6; lower F1 scores for the Altun Shan, Eastern Kunlun Shan, Eastern Tibetan Mountains, and Gangdise Mountains (0.27–0.36); and the lowest score for the Tibetan Interior Mountains subregion (0.16) (Table 3). This disparity arises from the scarcity of rock glaciers in certain subregions, where the deep learning model generated a large number of falsely detected polygons and subsequently produced high-false-positive values. Recall scores are generally higher than the precision scores, indicating that the false positives outweigh the false negatives in the model predictions. This finding suggests that the deep learning model possesses a strong capability for detecting rock glaciers.
Table 3Performance of deep-learning-mapped polygons in different subregions.
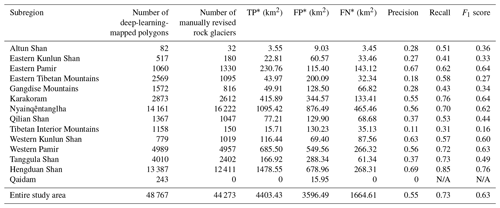
∗ TP (true positive), FP (false positive), and FN (false negative) are expressed as the total areas.
However, deep learning alone also generates numerous falsely detected polygons, highlighting the need for manual improvement. For example, Fig. 4 demonstrates the performance of the well-trained deep learning model in detecting and delineating rock glaciers in a new area – the Western Pamir, which was not included in the training process. As shown in Fig. 4a–c, there is good agreement between the deep learning output and the manually revised boundaries for a significant proportion of the rock glaciers in this area. However, Fig. 4 also illustrates some uncertainties associated with inaccurate boundary delineation, false detections, and missing identifications. For instance, as shown in Fig. 4d, a debris-covered glacier was falsely identified as a rock glacier, while Fig. 4e highlights several missing rock glaciers, possibly due to their poorly developed geomorphological features.
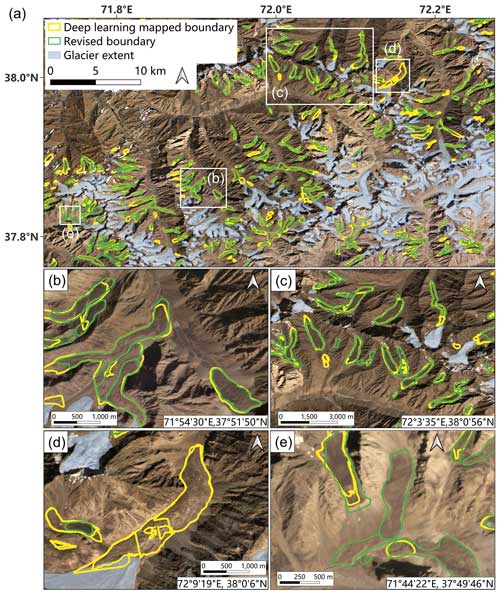
Figure 4(a) An example area in Western Pamir showing the deep learning outputs (in yellow) and manually revised rock glacier boundaries (in green). Clean and debris-covered glacier extents (light blue) are from the Randolph Glacier Inventory (RGI v.6) (Pfeffer et al., 2014); (b, c) enlarged views of the areas showing good agreement between deep learning outputs and revised boundaries; (d) enlarged view showing a false detection example in the center; (e) enlarged view showing multiple missing identifications.
5.1.2 Independent validation of the inventoried rock glaciers
Results of the independent review based on the 2110 rock glacier primary markers are presented in Table 4 and show that approximately 87 % of the primary markers were assigned as correctly identifying rock glaciers by both reviewers. This indicates that most of the sampled features met the criteria and characteristics of rock glaciers. Additionally, the evaluation process identified that only approximately 1 % and 6 % of the primary markers were assigned as false identifications by the first and second reviewers, respectively. This signifies that the occurrence of misclassifications or false positives within the inventory is relatively low (below 10 %). The discrepancy in the “no” decision numbers between the two reviewers can be attributed to the differences in the operators' judgments (Brardinoni et al., 2019).
5.2 Rock glacier inventory on the Tibetan Plateau: TPRoGI (v1.0)
After manual improvement, our plateau-wide inventory encompasses 44 273 rock glaciers, including both intact and relict types (Fig. 5). The inventoried rock glaciers cover a total area of approximately 6000 km2 (6 068 043 348 m2). The mean area is 0.14 km2. The largest rock glacier occupies 4.6 km2, whereas most of them (90.6 %) are smaller than 0.3 km2 (Fig. 6a). In terms of elevation, most rock glaciers (95.0 %) exhibit minimum elevations between 4000 and 5500 m above sea level (m a.s.l.), with an average value of 4729 m a.s.l. (Fig. 6b). The highest rock glacier is situated at an elevation of 5839 m a.s.l. in the Tibetan Interior Mountains, whereas the lowest lies at 2717 m a.s.l. in Western Pamir. Rock glaciers develop on slopes with varying gradients, and approximately 90 % of them occur on slopes between 10 and 25° with an average slope angle of 17.7° (Fig. 6c). Also, the compiled rock glaciers are distributed across various slope orientations with preferences at the north- and west-facing slopes (Fig. 6d).
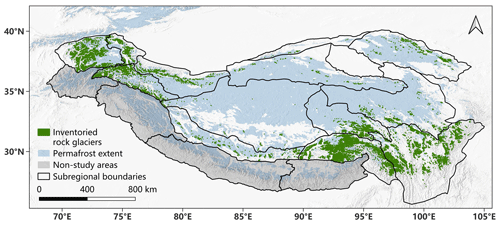
Figure 5Rock glacier inventory on the Tibetan Plateau (TPRoGI). The permafrost in the Hengduan Shan is overlapped by rock glaciers and thus is not visible on the map. The permafrost extent map is from Obu et al. (2018).
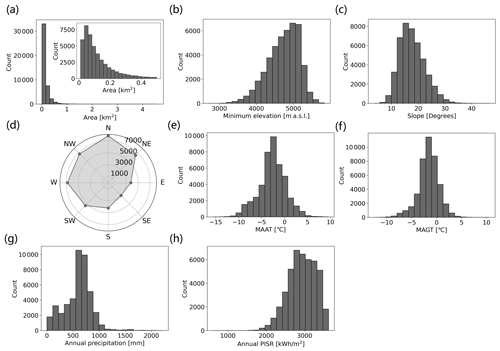
Figure 6Statistical summaries of the geomorphic and current climatic features of rock glaciers in the study region. (a) The areal histogram of all rock glaciers on the Tibetan Plateau. The inset shows the areas smaller than 0.5 km2. Panels (b)–(h) show histograms of the minimum elevations, slopes, aspects of the rock glaciers (with the radial axis representing the counts), mean annual air temperature (MAAT), mean annual ground temperature (MAGT), annual precipitation, and annual potential incoming solar radiation (PISR), respectively.
Rock glaciers predominantly occur in cold environments with temperatures at or slightly below freezing. A significant proportion of rock glaciers (66.3 %) thrive in areas where the MAAT ranges between −5 and 0 °C (Fig. 6e). Furthermore, 71.7 % of the rock glaciers exhibit MAGT between −5 and 0 °C (Fig. 6f). On average, the MAAT and MAGT for these rock glaciers are −2.7 and −1.6 °C, respectively. Approximately 82 % of the rock glaciers are situated in regions with annual precipitation ranging from 300 to 1000 mm, with an average of 597 mm (Fig. 6g). About 85 % of the rock glaciers receive incoming solar radiation (PISR) between 2500 and 3500 kWh m−2 annually, with a mean value of 2930 kWh m−2 (Fig. 6h).
5.3 Spatial distribution characteristics of rock glaciers
Figure 7 presents the spatial distribution and geomorphic characteristics of rock glaciers on the Tibetan Plateau within 50 km grid cells. Rock glaciers are widespread in the northwestern and southeastern plateau and densely distributed in the Western Pamir and Nyainqêntanglha, while they are scarce in the inner plateau (Fig. 7a). No rock glaciers were found in the Qaidam region, presumably due to the absence of permafrost and the occurrence of few mountains there. Rock glaciers in the western plateau have larger areas (mean=0.21 km2) than in the eastern plateau (mean=0.11 km2), as evident in Fig. 7b. Notably, a decreasing gradient is observed in minimum elevations of rock glaciers, with higher elevations in the Gangdise Mountains and lower elevations towards the east and west directions (Fig. 7c). The average slopes of rock glaciers are larger in northwestern Karakoram and southeastern plateau, suggesting a tendency for rock glaciers to develop on steeper slopes in these areas (Fig. 7d).
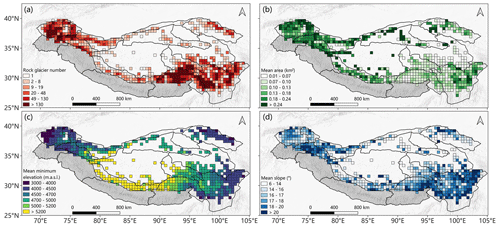
Figure 7Rock glacier (a) density, (b) area, (c) minimum elevation and (d) slope averaged over grid cells of 50 km×50 km.
Rock glacier aspects across different subregions are depicted in Fig. 8, characterized by a discernible west–east gradient and similarities between neighboring subregions. Specifically, the ones found in the western plateau (Western Kunlun Shan, Karakoram, Eastern Pamir, Western Pamir) display no distinct preference towards any specific orientation, whereas those situated in the central part of the plateau (Altun Shan, Eastern Kunlun Shan, Tibetan Interior Mountains, Gangdise Mountains) primarily face north. Conversely, rock glaciers in the eastern plateau (Qilian Shan, Eastern Tibetan Mountains, Tanggula Shan, Hengduan Shan, Nyainqêntanglha) exhibit a prevalent preference for north and west orientations.
6.1 Limitations of the deep-learning-based mapping approach
6.1.1 Limitations of Planet Basemaps imagery
The quality of the source images plays a crucial role in the uncertainty of the predicted results as the deep learning model accuracy heavily relies on high-quality input images. However, rock glaciers are frequently found in regions characterized by poor image quality due to factors associated with cloud cover, shadows, and distortions, which are common in mountainous areas. These challenges have a substantial impact on the accuracy of predictions. Consequently, when the deep learning model is input with images suffering severe quality issues, it may fail to identify rock glaciers within that region (Fig. S5 in the Supplement).
6.1.2 Limitations of the deep learning model
The mapped results generated by the deep learning model still have significant uncertainties associated with inaccurately predicted boundaries, false detections, and missing identifications (Fig. 4). Despite utilizing the powerful neural network DeepLabv3+ as the model structure, the training and validation IoU scores fall below 0.8 (Fig. 3). When applied to the entire study area, the uncertainty increases further, with a precision of 0.55, a recall of 0.73, and a F1 score of 0.63 (Table 3). These results are comparable to the results by Robson et al. (2020), which obtained a precision of 63.9 % to 68.9 % and a recall of 75 % to 75.4 %. Both results highlight the challenges of using deep learning to fully automatically map rock glaciers in high-mountain environments.
A key limitation of the current deep learning model is the restricted number of input bands. Our model only utilizes RGB bands, while inherently excluding crucial topographic information such as slope and elevation. As rock glacier occurrence is closely related to topography and underlying geology, the absence of morphometric inputs like terrain roughness and slope, as well as lithological data, may hinder the model performance.
Furthermore, the learning performance of the model can be hindered by limited and biased training samples. Our training samples were derived from six local inventories, encompassing 4085 rock glacier polygons. Due to the limited quantity of our training dataset, the model may struggle to fully capture the complexity and diversity of the training samples. Consequently, its generalization ability and accuracy may be compromised when presented with unfamiliar images (Rice et al., 2020). Additionally, the six local inventories were compiled by different operators from various institutes. The divergent knowledge and expertise among these operators can introduce inconsistencies in judgments, resulting in subjectivity and bias within the training dataset. As a result, inconsistent and biased training samples can potentially confuse the model, thereby impairing its ability to accurately identify rock glaciers (Ren et al., 2018).
Additionally, it is important to note that the deep learning model can only map the areas of rock glaciers and is not capable of performing instance segmentation, which would accurately segment individual rock glacier units (Erharter et al., 2022). Consequently, the model tends to predict the entire rock glacier system, composed of several adjacent rock glacier units, as a single entity.
6.1.3 Limitations of manual improvement
The manual examination and refinement were assigned by multiple individuals with varying levels of experience, which inevitably introduced subjectivity, human errors, and potential inconsistencies (Brardinoni et al., 2019). Moreover, accurately depicting the boundaries of rock glaciers via manual delineation can be challenging due to the 4.77 m resolution of the interpreted images; thus, the mapped rock glaciers inherently contain uncertainties (Jones et al., 2018).
Furthermore, delineating the upper and lateral boundaries within rock glacier systems presents even greater uncertainties (Brardinoni et al., 2019). In comparison to the lower boundary in the front and lateral margin regions, the upper boundary in the rooting zone and the lateral boundary between rock glaciers within a system often lack pronounced geomorphological features and thereby require more precise interpretation of surface texture and color variations. As a result, the delineation of upper and lateral boundaries is inherently ambiguous and subjective (Schmid et al., 2015; Jones et al., 2018; Erharter et al., 2022). Due to the difficulty in delineating lateral boundaries and the limitations imposed by image resolution, the separation of rock glacier systems is uncertain. Therefore, some rock glacier systems, particularly the smaller ones lacking pronounced features of lateral boundaries, may not be effectively separated (Fig. S4 in the Supplement).
Additionally, the “retrieve” operation focused on areas where missing rock glaciers were observed near the polygons identified by the deep learning model. Consequently, some rock glaciers may have been missed without conducting an exhaustive examination of the entire study region.
6.2 Comparison with existing local inventories
We compared the number of inventoried rock glaciers in our study with existing local inventories on the plateau, including Daxue Shan (Ran and Liu, 2018; Cai et al., 2021), Nyainqêntanglha (Reinosch et al., 2021; Zhang et al., 2023; Li et al., 2024), Hunza Basin (Hassan et al., 2021), Gangdise Mountains (Zhang et al., 2022), Western Kunlun Shan (Hu et al., 2023), and Qilian Shan (Hu et al., 2024) as shown in Table 5. The number of inventoried rock glaciers in our study is generally comparable to those in Daxue Shan and Hunza Basin. However, our inventory has more rock glaciers than the inventories in Gangdise Mountains and Western Kunlun Shan and fewer rock glaciers than the inventories in Nyainqêntanglha and Qilian Shan.
These discrepancies can be explained by inherent sources of error within each dataset. As highlighted in the RGIK guidelines (RGIK, 2023), operator judgment in compiling rock glacier inventories can vary over time, leading to discrepancies between inventories created at different time periods. Even within the same time frame, differences in operator experience can result in significant variations in judgment (Brardinoni et al., 2019). For example, the delineation of the upper boundary of rock glaciers in rooting regions is challenging and can vary among different operators (Brardinoni et al., 2019). In the Hunza Basin, our delineated rock glaciers had lower upper boundaries compared to the results of Hassan et al. (2021) (Fig. S2 in the Supplement). Additionally, small rock glaciers can be difficult to recognize due to the lack of distinct characteristics. In the Nyainqêntanglha region, some small landforms were included as rock glaciers in the inventories by Reinosch et al. (2021) and Li et al. (2024) but were excluded from our inventory (Fig. S3 in the Supplement). Moreover, it is common in mountainous environments for several rock glacier units to merge into a complex system (RGIK, 2023). Some operators may delineate this system as a single polygon, while others may separate it into smaller polygons. This can be observed in the case of Daxue Shan, where some systems were delineated as single polygons in our inventory but were separated into smaller polygons in the inventories of Ran and Liu (2018) and Cai et al. (2021) (Fig. S4).
Another significant factor contributing to discrepancies in inventories is the use of different image sources. Images with varying types, resolutions, and qualities can greatly influence the inventory results. The use of InSAR images, for example, is beneficial for detecting actively moving rock glaciers but may have poor performance in identifying slowly moving or relict rock glaciers (Liu et al., 2013; Hu et al., 2023). Moreover, images with low resolution used in some of the previous inventories may not clearly reveal the morphological characteristics of rock glaciers, increasing the probability of missing identifications. In the Western Kunlun Shan region, our inventory compiled more rock glaciers by using Planet Basemaps images (4.77 m resolution) compared to Hu et al. (2023), whose inventory was based on Sentinel-2 images (10 m resolution). Additionally, images with quality issues caused by clouds, snow, shadows, and image distortion can lead to missed identifications of rock glaciers. In some areas of Nyainqêntanglha, for instance, some rock glaciers were obscured by clouds in Planet Basemaps images and were missed in our inventory, but they were visible in Google Earth images and had been included in the inventories by Reinosch et al. (2021) and Li et al. (2024) (Fig. S5). Since the discrepancies between inventories can arise from various sources, conducting further quantitative comparisons on the accuracies of rock glacier locations and boundaries poses challenges.
6.3 Significance of the inventory and future work
To our knowledge, the creation of the new inventory on the Tibetan Plateau represents the most extensive collection of rock glaciers published worldwide. This large dataset offers exciting prospects for advancing various research areas related to rock glaciers, including permafrost distribution, mountain hydrology, climate impacts on the permafrost environment, and geohazards as introduced in Sect. 1.
First, our new inventory enables more accurate assessments of permafrost distribution, allowing researchers to refine existing permafrost maps and enhance our understanding of permafrost characteristics on the Tibetan Plateau (Schmid et al., 2015; Hassan et al., 2021; Zou et al., 2017; Li et al., 2024). We underline that the lack of comprehensive rock glacier information on the plateau had previously limited permafrost assessment studies in this region. Cao et al. (2021) found that a model driven by rock glacier observations led to an overestimation of permafrost extent by about 8.4 %–13.1 % on the Tibetan Plateau compared to a model using in situ measurements. Nevertheless, they used datasets from the Himalayan range as an alternative due to the limited availability of rock glacier observations on the plateau.
With respect to hydrology, Jones et al. (2021a) had estimated the global water contribution from rock glaciers and highlighted the lack of rock glacier data in certain regions, including the Tibetan Plateau. Our inventory fills the data gap in this critical region of High Mountain Asia, providing an opportunity to investigate the potential water storage available within rock glaciers (Corte, 1976; Azócar and Brenning, 2010; Jones et al., 2019b; Schaffer et al., 2019; Wagner et al., 2020, 2021) and the contribution of rock glacier meltwater to streamflow (Geiger et al., 2014; Wagner et al., 2016).
Moreover, our inventory serves as a guide for establishing rock glacier monitoring sites on the plateau, contributing to the study of the long-term evolution of rock glaciers and the impacts of climate change on mountain permafrost in this region. Systematic monitoring of rock glacier velocities has been established in the European Alps (e.g., Delaloye et al., 2010; Marcer et al., 2021), Northern Tien Shan (Kääb et al., 2021), and the Andes (Vivero et al., 2021). Currently, no such monitoring sites exist on the Tibetan Plateau due to the lack of information on rock glacier distribution.
Lastly, the new inventory developed in this study will contribute to the evaluation of rock glacier hazards and risks, providing important information for geohazard management and enabling informed decision-making regarding infrastructure planning on the Tibetan Plateau (Hassan et al., 2021; Janke and Bolch, 2021).
This benchmark dataset will be maintained and updated in the future. We will leverage multi-source datasets, including InSAR data, elevation change maps (from high-resolution DEM differencing), and high-resolution optical images (from Google Earth, ESRI base maps, and Bing maps), to validate and refine our inventory. The InSAR data will be used to attribute kinematic information (RGIK, 2023).
We will extend our inventory in the future by including the Himalayas, the Hindu Kush, and the Tien Shan regions to compile a comprehensive inventory for High Mountain Asia (Fig. S6 in the Supplement). Work is currently ongoing to evaluate the rock glacier kinematics on the Tibetan Plateau based on the developed inventory (Sun et al., 2024b), as well as to validate the deep learning rock glacier output for the Hindu Kush Himalaya regions (Racoviteanu et al., 2024).
The rock glacier inventory for the Tibetan Plateau can be accessed at https://doi.org/10.5281/zenodo.10732042 (Sun et al., 2024a).
In this study, we proposed a deep-learning-based approach for mapping rock glaciers and created the first plateau-wide inventory, i.e., TPRoGI (v1.0), encompassing 44 273 rock glaciers. This inventory fills the gap in the rock glacier data on the Tibetan Plateau and provides a baseline dataset for monitoring mountain permafrost in this region. Findings from the current study are summarized as follows: (1) the deep learning model demonstrates a promising capability in detecting and outlining rock glaciers and can serve as a valuable tool for inventorying rock glaciers across large regions; (2) rock glaciers are widespread in the northwestern and southeastern plateau and densely distributed in the Western Pamir and Nyainqêntanglha, while they are scarce in the inner plateau; (3) the majority of rock glaciers are situated at elevations from 4000 to 5500 m a.s.l. and on slopes between 10 and 25° with north and west preferences; (4) rock glaciers show a northwest preference in the eastern plateau, a north-only orientation in the central plateau, and no specific preference in the western plateau; (5) rock glaciers on the Tibetan Plateau cover a total area of 6000 km2 with a mean area of 0.14 km2, with rock glaciers in the western plateau exhibiting larger areas compared to those in other areas. However, there remain uncertainties in the current inventory due to limitations inherent in the imagery, the deep learning model, and the manual revisions. The inventory will continue to be maintained and updated in the future. We expect that the benchmark dataset produced in this study will facilitate investigations into many scientific questions related to rock glaciers and mountain permafrost on the Tibetan Plateau.
The supplement related to this article is available online at: https://doi.org/10.5194/essd-16-5703-2024-supplement.
YaH, LL, and SH designed the study with funding from the Hong Kong Research Grants Council and the CUHK–Exeter Joint Centre for Environmental Sustainability and Resilience. ZS and YaH performed the training and prediction of deep learning model. ZS, YaH, AR, JC, XG, YuH, and HY compiled the inventory. LL and XW reviewed and validated the inventory. ZS wrote the manuscript with input from YaH, AR, LL, and SH.
The contact author has declared that none of the authors has any competing interests.
Publisher's note: Copernicus Publications remains neutral with regard to jurisdictional claims made in the text, published maps, institutional affiliations, or any other geographical representation in this paper. While Copernicus Publications makes every effort to include appropriate place names, the final responsibility lies with the authors.
We would like to thank the Office National des Forêts (Departement Service Restauration des Terrains en Montagne) for providing the rock glacier inventory in the French Alps, Lingcao Huang for providing the deep learning codes, and Ho Ming Tsang for valuable assistance in the calculation of aspect angles.
This work has been supported by the Hong Kong Research Grants Council, University Grants Committee (grant nos. CUHK14302421, HKPFS PF20-42392), the The Chinese University of Hong Kong–Exeter Joint Centre for Environmental Sustainability and Resilience (ENSURE, grant no. 4930821), the CUHK Direct Grant for Research (grant no. 4053510 and 4053592), the National Natural Science Foundation of China (grant nos. 42371458, 42071410), and the “PHC PROCORE” program (project number: F-CUHK404/22), funded by the French Ministry for Europe and Foreign Affairs, the French Ministry for Higher Education and Research, and the Hong Kong Research Grants Council. Adina Racoviteanu's work is supported by the French National Research Institute for Sustainable Development (IRD).
This paper was edited by James Thornton and reviewed by Flavius Sirbu and two anonymous referees.
Arenson, L., Hoelzle, M., and Springman, S.: Borehole deformation measurements and internal structure of some rock glaciers in Switzerland, Permafrost Periglac., 13, 117–135, https://doi.org/10.1002/ppp.414, 2002.
Arenson, L. U. and Springman, S. M.: Mathematical descriptions for the behaviour of ice-rich frozen soils at temperatures close to 0 °C, Can. Geotech. J., 42, 431–442, https://doi.org/10.1139/t04-109, 2005.
Azócar, G. and Brenning, A.: Hydrological and geomorphological significance of rock glaciers in the dry Andes, Chile (27–33 S), Permafrost Periglac., 21, 42–53, https://doi.org/10.1002/ppp.669, 2010.
Barsch, D.: Rockglaciers: indicators for the present and former geoecology in high mountain environments, Springer-Verlag, Berlin, 331 pp., https://doi.org/10.1007/978-3-642-80093-1, 1996.
Berthling, I.: Beyond confusion: Rock glaciers as cryo-conditioned landforms, Geomorphology, 131, 98–106, https://doi.org/10.1016/j.geomorph.2011.05.002, 2011.
Boeckli, L., Brenning, A., Gruber, S., and Noetzli, J.: Permafrost distribution in the European Alps: calculation and evaluation of an index map and summary statistics, The Cryosphere, 6, 807–820, https://doi.org/10.5194/tc-6-807-2012, 2012.
Bolch, T., Shea, J. M., Liu, S., Azam, F. M., Gao, Y., Gruber, S., Immerzeel, W. W., Kulkarni, A., Li, H., Tahir, A. A., Zhang, G., and Zhang, Y.: Status and change of the cryosphere in the extended Hindu Kush Himalaya region, in: The Hindu Kush Himalaya Assessment, Mountains, Climate Change, Sustainability and People, edited by: Wester, P., Mishra, A., Mukherji, A., and Shrestha, A. B., Springer International Publishing, Cham, 209–255, https://doi.org//10.1007/978-3-319-92288-1_7, 2019.
Bolch, T., Yao, T., Bhattacharya, A., Hu, Y., King, O., Liu, L., Pronk, J. B., Rastner, P., and Zhang, G.: Earth observation to investigate occurrence, characteristics and changes of glaciers, glacial lakes and rock glaciers in the Poiqu river basin (central Himalaya), Remote Sens.-Basel, 14, 1927, https://doi.org/10.3390/rs14081927, 2022.
Brardinoni, F., Scotti, R., Sailer, R., and Mair, V.: Evaluating sources of uncertainty and variability in rock glacier inventories, Earth Surf. Proc. Land., 44, 2450–2466, https://doi.org/10.1002/esp.4674, 2019.
Cai, J., Wang, X., Liu, G., and Yu, B.: A comparative study of active rock glaciers mapped from geomorphic-and kinematic-based approaches in Daxue Shan, southeast Tibetan Plateau, Remote Sens.-Basel, 13, 4931, https://doi.org/10.3390/rs13234931, 2021.
Cao, B., Li, X., Feng, M., and Zheng, D.: Quantifying overestimated permafrost extent driven by rock glacier inventory, Geophys. Res. Lett., 48, e2021GL092476, https://doi.org/10.1029/2021GL092476, 2021.
Capps Jr., S. R.: Rock glaciers in Alaska, J. Geol., 18, 359–375, https://doi.org/10.1086/621746, 1910.
Chen, L.-C., Zhu, Y., Papandreou, G., Schroff, F., and Adam, H.: Encoder-decoder with atrous separable convolution for semantic image segmentation, in: Proceedings of the European conference on computer vision (ECCV), Munich, Germany, 8–14 September 2018, 801–818, https://doi.org/10.48550/arXiv.1802.02611, 2018.
Chollet, F.: Xception: Deep learning with depthwise separable convolutions, in: Proceedings of the IEEE conference on computer vision and pattern recognition, Honolulu, Hawaii, USA, 21–26 July 2017, 1251–1258, https://doi.org/10.48550/arXiv.1610.02357, 2017.
Cicoira, A., Beutel, J., Faillettaz, J., Gärtner-Roer, I., and Vieli, A.: Resolving the influence of temperature forcing through heat conduction on rock glacier dynamics: a numerical modelling approach, The Cryosphere, 13, 927–942, https://doi.org/10.5194/tc-13-927-2019, 2019a.
Cicoira, A., Beutel, J., Faillettaz, J., and Vieli, A.: Water controls the seasonal rhythm of rock glacier flow, Earth Planet. Sc. Lett., 528, 115844, https://doi.org/10.1016/j.epsl.2019.115844, 2019b.
Corte, A.: The hydrological significance of rock glaciers, J. Glaciol., 17, 157–158, https://doi.org/10.3189/S0022143000030859, 1976.
Crippen, R., Buckley, S., Agram, P., Belz, E., Gurrola, E., Hensley, S., Kobrick, M., Lavalle, M., Martin, J., Neumann, M., Nguyen, Q., Rosen, P., Shimada, J., Simard, M., and Tung, W.: NASADEM global elevation model: Methods and progress, Int. Arch. Photogramm., 41, 125–128, https://doi.org/10.5194/isprs-archives-XLI-B4-125-2016, 2016.
Delaloye, R., Lambiel, C., and Gärtner-Roer, I.: Overview of rock glacier kinematics research in the Swiss Alps, Geogr. Helv., 65, 135–145, https://doi.org/10.5194/gh-65-135-2010, 2010.
Delaloye, R., Barboux, C., Bodin, X., Brenning, A., Hartl, L., Hu, Y., Ikeda, A., Kaufmann, V., Kellerer-Pirklbauer, A., Lambiel, C., Liu, L., Marcer, M., Rick, B., Scotti, R., Takadema, H., Trombotto, D., Vivero, S., and Winterberger, M.: Rock glacier inventories and kinematics: A new IPA Action Group, in: Proceedings of the 5th European Conference on Permafrost, Chamonix-Mont Blanc, France, 23 June–1 July 2018, vol. 23, 392–393, https://bigweb.unifr.ch/Science/Geosciences/Geomorphology/Pub/Website/IPA/180628_EUCOP5_IPA_Action_Group_Delaloye.pdf (last access: 3 December 2024), 2018.
Erharter, G. H., Wagner, T., Winkler, G., and Marcher, T.: Machine learning – An approach for consistent rock glacier mapping and inventorying – Example of Austria, Applied Computing and Geosciences, 16, 100093, https://doi.org/10.1016/j.acags.2022.100093, 2022.
Feng, M., Xu, J., Wang, J., Ran, Y., and Li, X.: Identifying rock glacier in western China using deep learning and satellite data, in: AGU Fall Meeting Abstracts, San Francisco, USA, 9–13 December 2019, vol. 2019, GC53G–1249, https://ui.adsabs.harvard.edu/abs/2019AGUFMGC53G1249F/abstrac (last access: 3 December 2024), 2019.
Geiger, S. T., Daniels, J. M., Miller, S. N., and Nicholas, J. W.: Influence of rock glaciers on stream hydrology in the La Sal Mountains, Utah, Arct. Antarct. Alp. Res., 46, 645–658, https://doi.org/10.1657/1938-4246-46.3.645, 2014.
Haeberli, W., Hallet, B., Arenson, L., Elconin, R., Humlum, O., Kääb, A., Kaufmann, V., Ladanyi, B., Matsuoka, N., Springman, S., and Mühll, D. V.: Permafrost creep and rock glacier dynamics, Permafrost Periglac., 17, 189–214, https://doi.org/10.1002/ppp.561, 2006.
Hassan, J., Chen, X., Muhammad, S., and Bazai, N. A.: Rock glacier inventory, permafrost probability distribution modeling and associated hazards in the Hunza River Basin, Western Karakoram, Pakistan, Sci. Total Environ., 782, 146833, https://doi.org/10.1016/j.scitotenv.2021.146833, 2021.
Hu, Y., Liu, L., Huang, L., Zhao, L., Wu, T., Wang, X., and Cai, J.: Mapping and Characterizing Rock Glaciers in the Arid Western Kunlun Mountains Supported by InSAR and Deep Learning, J. Geophys. Res.-Earth, 128, e2023JF007206, https://doi.org/10.1029/2023JF007206, 2023.
Hu, Z., Yan, D., Feng, M., Xu, J., Liang, S., and Sheng, Y.: Enhancing mountainous permafrost mapping by leveraging a rock glacier inventory in northeastern Tibetan Plateau, Int. J. Digit. Earth, 17, 2304077, https://doi.org/10.1080/17538947.2024.2304077, 2024.
Huang, L., Liu, L., Jiang, L., and Zhang, T.: Automatic mapping of thermokarst landforms from remote sensing images using deep learning: A case study in the Northeastern Tibetan Plateau, Remote Sens.-Basel, 10, 2067, https://doi.org/10.3390/rs10122067, 2018.
Huang, L., Luo, J., Lin, Z., Niu, F., and Liu, L.: Using deep learning to map retrogressive thaw slumps in the Beiluhe region (Tibetan Plateau) from CubeSat images, Remote Sens. Environ., 237, 111534, https://doi.org/10.1016/j.rse.2019.111534, 2020.
Huffman, G. J., Stocker, E. F., Bolvin, D. T., Nelkin, E. J., Tan, J.: GPM IMERG Final Precipitation L3 1 month 0.1 degree x 0.1 degree V07, Greenbelt, MD, Goddard Earth Sciences Data and Information Services Center (GES DISC) [data set], https://doi.org/10.5067/GPM/IMERG/3B-MONTH/07, 2023.
Hungr, O., Leroueil, S., and Picarelli, L.: The Varnes classification of landslide types, an update, Landslides, 11, 167–194, https://doi.org/10.1007/s10346-013-0436-y, 2014.
Janke, J. and Bolch, T.: Rock glaciers, Treatise on Geomorphology, 2nd edn., vol. 4, Elsevier, https://doi.org/10.1016/B978-0-12-818234-5.00187-5, 2021.
Jones, D., Harrison, S., Anderson, K., Selley, H., Wood, J., and Betts, R.: The distribution and hydrological significance of rock glaciers in the Nepalese Himalaya, Global Planet. Change, 160, 123–142, https://doi.org/10.1016/j.gloplacha.2017.11.005, 2018.
Jones, D. B., Harrison, S., and Anderson, K.: Mountain glacier-to-rock glacier transition, Global Planet. Change, 181, 102999, https://doi.org/10.1016/j.gloplacha.2019.102999, 2019a.
Jones, D. B., Harrison, S., Anderson, K., and Whalley, W. B.: Rock glaciers and mountain hydrology: A review, Earth-Sci. Rev., 193, 66–90, https://doi.org/10.1016/j.earscirev.2019.04.001, 2019b.
Jones, D. B., Harrison, S., Anderson, K., and Betts, R.: Author Correction: Mountain rock glaciers contain globally significant water stores, Sci. Rep.-UK, 11, 23536, https://doi.org/10.1038/s41598-021-02401-0, 2021a.
Jones, D. B., Harrison, S., Anderson, K., Shannon, S., and Betts, R.: Rock glaciers represent hidden water stores in the Himalaya, Sci. Total Environ., 793, 145368, https://doi.org/10.1016/j.scitotenv.2021.145368, 2021b.
Kääb, A., Strozzi, T., Bolch, T., Caduff, R., Trefall, H., Stoffel, M., and Kokarev, A.: Inventory and changes of rock glacier creep speeds in Ile Alatau and Kungöy Ala-Too, northern Tien Shan, since the 1950s, The Cryosphere, 15, 927–949, https://doi.org/10.5194/tc-15-927-2021, 2021.
Kumar, L., Skidmore, A. K., and Knowles, E.: Modelling topographic variation in solar radiation in a GIS environment, Int. J. Geogr. Inf. Sci., 11, 475–497, https://doi.org/10.1080/136588197242266, 1997.
LeCun, Y., Bengio, Y., and Hinton, G.: Deep learning, Nature, 521, 436–444, https://doi.org/10.1038/nature14539, 2015.
Li, M., Yang, Y., Peng, Z., and Liu, G.: Assessment of rock glaciers and their water storage in Guokalariju, Tibetan Plateau, The Cryosphere, 18, 1–16, https://doi.org/10.5194/tc-18-1-2024, 2024.
Liu, L., Millar, C. I., Westfall, R. D., and Zebker, H. A.: Surface motion of active rock glaciers in the Sierra Nevada, California, USA: inventory and a case study using InSAR, The Cryosphere, 7, 1109–1119, https://doi.org/10.5194/tc-7-1109-2013, 2013.
Marcer, M.: Rock glaciers automatic mapping using optical imagery and convolutional neural networks, Permafrost Periglac., 31, 561–566, https://doi.org/10.1002/ppp.2076, 2020.
Marcer, M., Bodin, X., Brenning, A., Schoeneich, P., Charvet, R., and Gottardi, F.: Permafrost favorability index: spatial modeling in the French Alps using a rock glacier inventory, Front. Earth Sci., 5, 105, https://doi.org/10.3389/feart.2017.00105, 2017.
Marcer, M., Cicoira, A., Cusicanqui, D., Bodin, X., Echelard, T., Obregon, R., and Schoeneich, P.: Rock glaciers throughout the French Alps accelerated and destabilised since 1990 as air temperatures increased, Communications Earth & Environment, 2, 81, https://doi.org/10.1038/s43247-021-00150-6, 2021.
McNally, A. and NASA/GSFC/HSL: FLDAS Noah Land Surface Model L4 Global Monthly 0.1 x 0.1 degree (MERRA-2 and CHIRPS), Greenbelt, MD, USA, Goddard Earth Sciences Data and Information Services Center (GES DISC) [data set], https://doi.org/10.5067/5NHC22T9375G, 2018.
Mu, C., Abbott, B. W., Norris, A. J., Mu, M., Fan, C., Chen, X., Jia, L., Yang, R., Zhang, T., Wang, K., Peng, X., Wu, Q., Guggenberger, G., and Wu, X.: The status and stability of permafrost carbon on the Tibetan Plateau, Earth-Sci. Rev., 211, 103433, https://doi.org/10.1016/j.earscirev.2020.103433, 2020.
Munroe, J. S.: Distribution, evidence for internal ice, and possible hydrologic significance of rock glaciers in the Uinta Mountains, Utah, USA, Quaternary Res., 90, 50–65, https://doi.org/10.1017/qua.2018.24, 2018.
Nass, A., Manaud, N., van Gasselt, S., and Hare, T. M.: Towards a new face for Planetary Maps: Design and web-based Implementation of Planetary Basemaps, Advances in Cartography and GIScience of the ICA, 1, 15, https://doi.org/10.5194/ica-adv-1-15-2019, 2019.
Obu, J., Westermann, S., Kääb, A., and Bartsch, A.: Ground Temperature Map, 2000–2016, Northern Hemisphere Permafrost, Alfred Wegener Institute, Helmholtz Centre for Polar and Marine Research, Bremerhaven, PANGAEA, https://doi.org/10.1594/PANGAEA.888600, 2018.
Pfeffer, W. T., Arendt, A. A., Bliss, A., Bolch, T., Cogley, J. G., Gardner, A. S., Hagen, J.-O., Hock, R., Kaser, G., Kienholz, C., Miles, E. S., Moholdt, G., Mölg, N., Paul, F., Radić, V., Rastner, P., Raup, B. H., Rich, J., Sharp, M. J., and The Randolph Consortium: The Randolph Glacier Inventory: a globally complete inventory of glaciers, J. Glaciol., 60, 537–552, https://doi.org/10.3189/2014JoG13J176, 2014.
Racoviteanu, A., Nicholson, L., Glasser, N., Miles, E., Harrison, S., and Reynolds, J.: Debris-covered glacier systems and associated glacial lake outburst flood hazards: challenges and prospects, J. Geol. Soc. London, 179, jgs2021–084, https://doi.org/10.1144/jgs2021-084, 2022.
Racoviteanu, A., Sun, Z., Hu, Y., Liu, L., and Harrison, S.: Rock glacier inventorying and validation across the Hindu Kush Himalaya from deep learning and high-resolution images, EGU General Assembly 2024, Vienna, Austria, 14–19 Apr 2024, EGU24-16141, https://doi.org/10.5194/egusphere-egu24-16141, 2024.
Ran, Z. and Liu, G.: Rock glaciers in Daxue Shan, south-eastern Tibetan Plateau: an inventory, their distribution, and their environmental controls, The Cryosphere, 12, 2327–2340, https://doi.org/10.5194/tc-12-2327-2018, 2018.
Rangecroft, S., Harrison, S., Anderson, K., Magrath, J., Castel, A. P., and Pacheco, P.: Climate change and water resources in arid mountains: an example from the Bolivian Andes, Ambio, 42, 852–863, https://doi.org/10.1007/s13280-013-0430-6, 2013.
Rangecroft, S., Harrison, S., and Anderson, K.: Rock glaciers as water stores in the Bolivian Andes: an assessment of their hydrological importance, Arct. Antarct. Alp. Res., 47, 89–98, https://doi.org/10.1657/AAAR0014-029, 2015.
Reinosch, E., Gerke, M., Riedel, B., Schwalb, A., Ye, Q., and Buckel, J.: Rock glacier inventory of the western Nyainqêntanglha Range, Tibetan Plateau, supported by InSAR time series and automated classification, Permafrost Periglac., 32, 657–672, https://doi.org/10.1002/ppp.2117, 2021.
Ren, M., Zeng, W., Yang, B., and Urtasun, R.: Learning to reweight examples for robust deep learning, in: International Conference on Machine Learning, Stockholm, Sweden, 10–15 July 2018, PMLR, 4334–4343, https://proceedings.mlr.press/v80/ren18a.html (last access: 3 December 2024), 2018.
RGIK: Guidelines for inventorying rock glaciers: baseline and practical concepts (version 1.0), IPA Action Group Rock glacier inventories and kinematics, 25 pp., https://doi.org/10.51363/unifr.srr.2023.002, 2023.
Rice, L., Wong, E., and Kolter, Z.: Overfitting in adversarially robust deep learning, in: International Conference on Machine Learning, online, 12–18 July 2020, PMLR, 8093–8104, https://proceedings.mlr.press/v119/rice20a (last access: 3 December 2024), 2020.
Robson, B. A., Bolch, T., MacDonell, S., Hölbling, D., Rastner, P., and Schaffer, N.: Automated detection of rock glaciers using deep learning and object-based image analysis, Remote Sens. Environ., 250, 112033, https://doi.org/10.1016/j.rse.2020.112033, 2020.
Royden, L. H., Burchfiel, B. C., and van der Hilst, R. D.: The geological evolution of the Tibetan Plateau, Science, 321, 1054–1058, https://doi.org/10.1126/science.1155371, 2008.
Schaffer, N., MacDonell, S., Réveillet, M., Yáñez, E., and Valois, R.: Rock glaciers as a water resource in a changing climate in the semiarid Chilean Andes, Reg. Environ. Change, 19, 1263–1279, https://doi.org/10.1007/s10113-018-01459-3, 2019.
Schmid, M.-O., Baral, P., Gruber, S., Shahi, S., Shrestha, T., Stumm, D., and Wester, P.: Assessment of permafrost distribution maps in the Hindu Kush Himalayan region using rock glaciers mapped in Google Earth, The Cryosphere, 9, 2089–2099, https://doi.org/10.5194/tc-9-2089-2015, 2015.
Spencer, A.: A peculiar form of talus, Science, 11, 188–189, 1900.
Sun, Z., Hu, Y., Racoviteanu, A., Liu, L., Harrison, S., Wang, X., Cai, J., Guo, X., He, Y., and Yuan, H.: TPRoGI: a comprehensive rock glacier inventory for the Tibetan Plateau using deep learning (1.0), Zenodo [data set], https://doi.org/10.5281/zenodo.10732042, 2024a.
Sun, Z., Liu, L., Hu, Y., and Fan, C.: Assessing rock glacier velocities on the Tibetan Plateau using satellite SAR interferometry, EGU General Assembly 2024, Vienna, Austria, 14–19 Apr 2024, EGU24-3349, https://doi.org/10.5194/egusphere-egu24-3349, 2024b.
Vivero, S., Bodin, X., Farías-Barahona, D., MacDonell, S., Schaffer, N., Robson, B. A., and Lambiel, C.: Combination of aerial, satellite, and UAV photogrammetry for quantifying rock glacier kinematics in the Dry Andes of Chile (30 S) since the 1950s, Frontiers in Remote Sensing, 2, 784015, https://doi.org/10.3389/frsen.2021.784015, 2021.
Wagner, T., Pauritsch, M., and Winkler, G.: Impact of relict rock glaciers on spring and stream flow of alpine watersheds: Examples of the Niedere Tauern Range, Eastern Alps (Austria), Austrian J. Earth Sci., 109, 84–98, https://doi.org/10.17738/ajes.2016.0006, 2016.
Wagner, T., Brodacz, A., Krainer, K., and Winkler, G.: Active rock glaciers as shallow groundwater reservoirs, Austrian Alps, Grundwasser, 25, 215–230, https://doi.org/10.1007/s00767-020-00455-x, 2020.
Wagner, T., Kainz, S., Helfricht, K., Fischer, A., Avian, M., Krainer, K., and Winkler, G.: Assessment of liquid and solid water storage in rock glaciers versus glacier ice in the Austrian Alps, Sci. Total Environ., 800, 149593, https://doi.org/10.1016/j.scitotenv.2021.149593, 2021.
Xu, J., Feng, M., Wang, J., Ran, Y., Qi, Y., Yang, L., and Li, X.: Automatically Identifying Rock Glacier based on Gaofen Satellite Image and Deep Learning, Remote Sensing Technology and Application, 35, 1329–1336, 2021.
Yao, T., Thompson, L., Yang, W., Yu, W., Gao, Y., Guo, X., Yang, X., Duan, K., Zhao, H., Xu, B., Pu, J., Lu, A., Xiang, Y., Kattel, D. B., and Joswiak, D.: Different glacier status with atmospheric circulations in Tibetan Plateau and surroundings, Nat. Clim. Change, 2, 663–667, https://doi.org/10.1038/nclimate1580, 2012.
Yu, L. and Gong, P.: Google Earth as a virtual globe tool for Earth science applications at the global scale: progress and perspectives, Int. J. Remote Sens., 33, 3966–3986, https://doi.org/10.1080/01431161.2011.636081, 2012.
Zemp, M., Chao, Q., Han Dolman, A. J., Herold, M., Krug, T., Speich, S., Suda, K., Thorne, P., and Yu, W.: GCOS 2022 Implementation Plan, Global Climate Observing System GCOS, 85, https://doi.org/10.5167/uzh-224271, 2022.
Zhang, G., Yao, T., Xie, H., Yang, K., Zhu, L., Shum, C., Bolch, T., Yi, S., Allen, S., Jiang, L., Chen, W., and Ke, C.: Response of Tibetan Plateau lakes to climate change: Trends, patterns, and mechanisms, Earth-Sci. Rev., 208, 103269, https://doi.org/10.1016/j.earscirev.2020.103269, 2020.
Zhang, Q., Jia, N., Xu, H., Yi, C., Wang, N., and Zhang, L.: Rock glaciers in the Gangdise Mountains, southern Tibetan Plateau: Morphology and controlling factors, CATENA, 218, 106561, https://doi.org/10.1016/j.catena.2022.106561, 2022.
Zhang, X., Feng, M., Zhang, H., Wang, C., Tang, Y., Xu, J., Yan, D., and Wang, C.: Detecting rock glacier displacement in the central Himalayas using multi-temporal InSAR, Remote Sens.-Basel, 13, 4738, https://doi.org/10.3390/rs13234738, 2021.
Zhang, X., Feng, M., Xu, J., Yan, D., Wang, J., Zhou, X., Li, T., and Zhang, X.: Kinematic inventory of rock glaciers in the NyainqêntanglhaRange using the MT-InSAR method, Int. J. Digit. Earth, 16, 3923–3948, https://doi.org/10.1080/17538947.2023.2260778, 2023.
Zhao, L. and Sheng, Y.: Permafrost in the Qinghai-Tibet plateau and its changes, Science Press, Beijing, 356 pp., ISBN 7030581334, 2019.
Zhao, L., Zou, D., Hu, G., Du, E., Pang, Q., Xiao, Y., Li, R., Sheng, Y., Wu, X., Sun, Z., Wang, L., Wang, C., Ma, L., Zhou, H., and Liu, S.: Changing climate and the permafrost environment on the Qinghai–Tibet (Xizang) plateau, Permafrost Periglac., 31, 396–405, https://doi.org/10.1002/ppp.2056, 2020.
Zou, D., Zhao, L., Sheng, Y., Chen, J., Hu, G., Wu, T., Wu, J., Xie, C., Wu, X., Pang, Q., Wang, W., Du, E., Li, W., Liu, G., Li, J., Qin, Y., Qiao, Y., Wang, Z., Shi, J., and Cheng, G.: A new map of permafrost distribution on the Tibetan Plateau, The Cryosphere, 11, 2527–2542, https://doi.org/10.5194/tc-11-2527-2017, 2017.