the Creative Commons Attribution 4.0 License.
the Creative Commons Attribution 4.0 License.
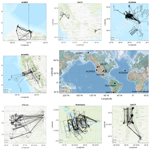
Atmospheric Radiation Measurement (ARM) airborne field campaign data products between 2013 and 2018
Jennifer M. Comstock
Mikhail S. Pekour
Jerome D. Fast
Krista L. Gaustad
Beat Schmid
Shuaiqi Tang
Damao Zhang
John E. Shilling
Jason M. Tomlinson
Adam C. Varble
Jian Wang
L. Ruby Leung
Lawrence Kleinman
Scot Martin
Sebastien C. Biraud
Brian D. Ermold
Kenneth W. Burk
Airborne measurements are pivotal for providing detailed, spatiotemporally resolved information about atmospheric parameters and aerosol and cloud properties, thereby enhancing our understanding of dynamic atmospheric processes. For 30 years, the US Department of Energy (DOE) Office of Science supported an instrumented Gulfstream 1 (G-1) aircraft for atmospheric field campaigns. Data from the final decade of G-1 operations were archived by the Atmospheric Radiation Measurement (ARM) Data Center and made publicly available at no cost to all registered users. To ensure a consistent data format and to improve the accessibility of the ARM airborne data, an integrated dataset was recently developed covering the final 6 years of G-1 operations (2013 to 2018, https://doi.org/10.5439/1999133; Mei and Gaustad, 2024). The integrated dataset includes data collected from 236 flights (766.4 h), which covered the Arctic, the US Southern Great Plains (SGP), the US West Coast, the eastern North Atlantic (ENA), the Amazon Basin in Brazil, and the Sierras de Córdoba range in Argentina. These comprehensive data streams provide much-needed insight into spatiotemporal variability in the thermodynamic quantities and aerosol and cloud properties for addressing essential science questions in Earth system process studies. This paper describes the DOE ARM merged G-1 datasets, including information on the acquisition, data collection challenges and future potentials, and quality control processes. It further illustrates the usage of this merged dataset to evaluate the Energy Exascale Earth System Model (E3SM) with the Earth System Model Aerosol–Cloud Diagnostics (ESMAC Diags) package.
- Article
(1906 KB) - Full-text XML
-
Supplement
(1073 KB) - BibTeX
- EndNote
The Earth's climate is changing due to human activities such as fossil fuel burning and deforestation. Atmospheric research is critical for understanding the causes and effects of climate change and for developing strategies to mitigate its impacts (IPCC, 2021). Airborne measurements provide a unique observational perspective within the broad objective of understanding atmospheric processes relevant to climate change. Aircraft are often the only platform from which in situ observations of chemical and physical parameters through the depth of the troposphere can be obtained. These platforms allow one to follow chemical and physical processes through the use of pseudo-Lagrangian flight patterns, their spatial coverage is usually greater than that obtained from surface sites, and their observations have a longer time duration than can be obtained from a (non-geostationary) satellite overpass. Moreover, vertical profiles can be measured, allowing researchers to obtain vertical profiles of atmospheric states, aerosols, clouds, and trace gas parameters with an accuracy that is not attainable with remote sensing. Such parameters are essential for understanding atmospheric physical and chemical processes. Compared with ground observatories, airborne measurements can provide greater spatiotemporal characterization in a limited time, contributing to a critical, otherwise missing context. This capability is particularly useful for studying rapidly evolving atmospheric phenomena like wildfires, hurricanes, and dust storms. Hence, airborne measurements are a valuable tool in atmospheric studies, as they offer several advantages over ground- or satellite-based measurements (Wendisch et al., 2013; Schumann et al., 2013; Petzold et al., 2013; McQuaid et al., 2013; McFarquhar et al., 2011; Krämer et al., 2013; Brenguier et al., 2013).
The US Department of Energy's Atmospheric Radiation Measurement (ARM) user facility (Mather and Voyles, 2013) has provided long-term measurements of atmospheric properties by operating ground-based observatories (fixed and mobile) as well as aerial facilities. Established in 2006, the ARM Aerial Facility (AAF) has led 14 field campaigns using state-of-the-art instruments aboard numerous aircraft (Schmid et al., 2014). Initially, the AAF data were archived individually for each instrument in the International Consortium for Atmospheric Research on Transport and Transformation (ICARTT) file format (Thornhill et al., 2011), which was developed to fulfill the data management need in 2004. A major strength of the ICARTT file format is its easy-to-use and standard approach to sharing airborne datasets to facilitate broad collaborative scientific research among airborne observation, atmospheric modeling, and satellite observation communities. However, shortcomings of the ICARTT file format have also long been identified: it is (1) not as efficient as binary formats for data collection and storage and (2) not suitable for extensive multidimensional data (https://www.earthdata.nasa.gov/esdis/esco/standards-and-practices/icartt-file-format, last access: 24 April 2023). ARM has started efforts to convert the historical field campaign data into Network Common Data Form (NetCDF) format (McCord and Voyles, 2016; Rew et al., 1997) – a widely used self-describing data format that supports creating, accessing and sharing array-oriented scientific data.
Additionally, researchers commonly use data analysis software (e.g., Python) that includes functions and library packages that make working with NetCDF files easier than text-like files. Using the NetCDF format, various airborne measurements can be easily combined to generate a merged dataset, thereby relieving end users of the burden of combining data from different data sources. The merged dataset can also aid the research community that uses the abundant ARM aerial data obtained from different field campaigns for diverse science objectives, as detailed in Table 1.
The demand for airborne observations continues to increase with the increase in weather and climate model complexity, as well as the increasing interest in small-scale physical and chemical processes. Such observations are needed to assess and validate process-level understanding. To provide a comprehensive view of atmospheric properties, it is desirable to integrate different types of data into a single file, thereby providing efficient data access for researchers to study the interactions among aerosols, clouds, and trace gases under various atmospheric conditions in order to understand effects on atmospheric processes and climate. Having all data in one file simplifies data management and reduces the number of files that need to be stored, shared, and accessed. It also minimizes the chances of data loss or errors during file transfers. In a single file, all measured variables are mapped into the same timestamp (e.g., 1 Hz for this study). More details are discussed in Sect. 4. These “merge files” are developed to assist researchers in performing more complex analyses, such as studying the relationships between different types of atmospheric data and carrying out more comprehensive studies with larger datasets. In addition, the merged data will hopefully encourage experimentalists and modelers to collaborate, thereby combining their expertise and resources to obtain a more complete understanding of the atmospheric phenomena. Thus, after standardizing the AAF data into a NetCDF file format for each field campaign, we used the ARM Data Integrator (ADI, https://github.com/ARM-DOE/ADI, last access: 24 April 2023) to retrieve and prepare data from each measurement and integrate them into a merged dataset (Gaustad et al., 2014).
This study provides an overview of the airborne datasets collected during seven field campaigns (listed in Table 1) between 2013 and 2018, an introduction to the integrated datasets, and a guide for users to access these datasets on the ARM data archive. Although airborne field campaigns can lead to highly significant scientific findings, they often have a lengthy timeline for generating research papers. Meanwhile, due to pressure to publish quickly and the limited length of funding cycles, researchers do not have enough time to fully leverage field campaign data. In this study, one of the objectives is to draw researchers' attention to these valuable field data, encouraging them to revisit underutilized datasets to reveal new insights. In Sect. 2, this paper provides an overview of the objectives, flight information, and measurements of the seven field campaigns that the AAF carried out with the G-1 aircraft between 2013 and 2018. Section 3 has three subsections: data quality is discussed by comparing the in situ measurements with other measurements; we briefly outline how to use the merged dataset to evaluate aerosol–cloud interactions represented in Earth system models; and we outline data collection challenges, lessons learned, and future potentials of these airborne data. Further detail on the data structure is given in Sect. 4. In addition, a summary section describes the potential of this merged dataset after explaining the data file structure and availability of the data.
2.1 AAF-supported field campaigns between 2013 and 2018
The ARM program is a US Department of Energy scientific user facility that aims to provide observation data to improve our understanding of the Earth's atmosphere and its interactions with the land surface and oceans. One of the key components of the ARM program is to conduct periodic field campaigns, which are intensive measurement periods at specific locations focused on specific scientific questions. These field campaigns involve deploying state-of-the-art instruments with both ground station and airborne platforms to collect measurements of atmospheric states, radiation, clouds, precipitation, aerosols, and trace gas variables, often including collaborations with other research programs and institutions. Table 1 shows the campaign locations, flight hours, and scientific objectives of each field study carried out by the AAF between 2013 and 2018. The “word cloud” depicted in Fig. S1 encapsulates the content of all AAF-supported field campaign publications, showing that the AAF has played a pivotal role in advancing research across various important atmospheric domains. The visual representation highlights a concentration of terms related to key atmospheric topics, including aerosols, clouds, precipitation properties, and the intricate processes governing their interactions. This observation underscores the multifaceted support provided by the AAF to the scientific community, contributing to the exploration and understanding of crucial atmospheric phenomena.
2.2 Description of the airborne platform and sensors
The ARM Aerial Facility (AAF) took over operations of the G-1 research aircraft in 2010. The G-1 is a medium-sized business aircraft that provided atmospheric measurements for various DOE programs from 1989 to 2018 (Fig. 2). The aircraft is powered by two turboprop engines, and it has a 1900 kg cabin payload and an operational range of approximately 4000 km for ferry flight at high altitudes and 800 km for boundary layer sampling, making it well-suited for deployments to remote locations. The G-1 can continuously fly for 3–4 h with a full payload (5 h with minimum payload) at a cruising speed of approximately 100 m s−1 up to a maximum altitude of approximately 7.6 km, which allows it to access a wide range of atmospheric conditions and altitudes for measurement purposes.
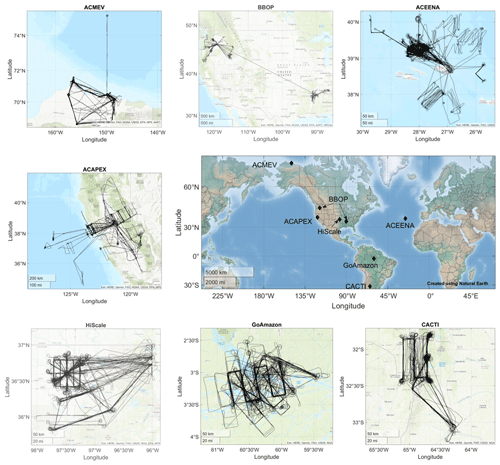
Figure 1Flight tracks overlaid with the campaign location map from seven AAF field campaigns between 2013 and 2018 using MATLAB®.
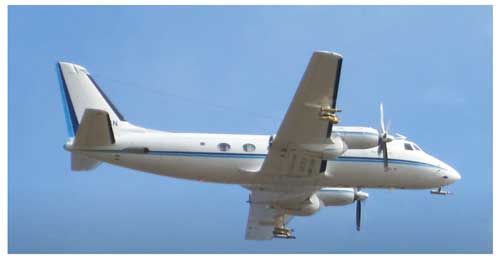
Figure 2The G-1 research aircraft flying over the ARM eastern North Atlantic (ENA) site (image courtesy of Ed Luke, Brookhaven National Laboratory).
The ARM G-1 is equipped with a suite of instruments and sensors for making measurements of atmospheric parameters (including temperature, humidity, pressure, winds, and turbulence); radiation; concentrations of atmospheric gases; and types, concentrations, and sizes of aerosol, cloud, and precipitation particles (as shown in Table 2). These instruments and sensors are mounted on the aircraft's exterior and inside the cabin. The measurement variables for individual data streams are listed in Table S1 and are used as input data for the merged data product. Note that some measurements, including those related to redundant cloud measurements and radiation, are not currently incorporated into the merged dataset described here due to the complexity of the existing data. However, these measurements remain under consideration for future inclusion, reflecting a commitment to expanding the dataset's scope as technology and analytical capabilities evolve. Additionally, photos, videos, and some guest instruments are nontraditional data types included in the ARM individual archive but not with these merged data. Driven by the various campaign objectives and payload limitations, the measurements available for each field campaign are listed in Table S2. The data collected by these instruments are used to improve our understanding of the Earth's climate system and to support the development of climate models and other tools for studying and predicting atmospheric and climate phenomena (Mei et al., 2020).
The AAF's airborne measurements are considered accurate and reliable because they are obtained directly from the atmosphere using well-calibrated instruments. Numerous prior studies have systematically assessed ARM data quality, employing methods such as laboratory evaluation based on community-accepted standards, comparing similar properties across different instruments, and conducting intercomparisons across diverse platforms (from ground to airborne or airborne to airborne) (Bond et al., 1999; Lance et al., 2010; Kassianov et al., 2015, 2018; Mei et al., 2020; Zawadowicz et al., 2021; Kulkarni et al., 2023; Tang et al., 2023; Zhang et al., 2023). Table S3 lists AAF measurements and uncertainties in the atmospheric properties, including temperature, humidity, aerosol concentrations, cloud particle sizes, and radiation levels (Mei et al., 2020).
The AAF airborne data are also often used as a benchmark or standard for other measurements, especially those from remote-sensing technologies such as satellites, ground-based radars, and lidars. Junghenn Noyes et al. (2020) validated remote-sensing retrievals with help from ground-based and airborne measurements. Their study enhanced the understanding of smoke particle behavior and its implications for remote sensing (Junghenn Noyes et al., 2020). Mech et al. (2020) showcased how integrating airborne data into the PAMTRA (Passive and Active Microwave TRAnsfer) validation process enhances the model's skill with respect to accurately simulating microwave measurements. The detailed comparison between simulated and observed data helps understand the model's performance under real-world conditions, leading to a more robust and reliable tool for atmospheric research (Mech et al., 2020). Yang et al. (2019) developed a new method to estimate supersaturation fluctuations in stratocumulus clouds using ground-based remote-sensing retrievals. Then, they used the airborne data to validate these estimations (Yang et al., 2019). Wu et al. (2020) retrieved profiles of marine boundary layer (MBL) cloud and drizzle microphysical properties from ground-based observations, validated by aircraft measurements over the Azores (Wu et al., 2020). Zhang et al. (2023) evaluated cloud droplet number concentrations using multiple ground-based methods validated through aircraft in situ measurements (Zhang et al., 2023).
Furthermore, research based on collected aircraft data led to advancements in the characterization and understanding of atmospheric processes (Martin et al., 2017; Fast et al., 2011, 2019a, b; Varble et al., 2021; Wang et al., 2022). For example, various studies have utilized aircraft measurements to characterize aerosol and cloud properties while also advancing the understanding of aerosol chemistry and cloud microphysical properties and processes, including investigations over the North Atlantic, Amazon Basin, and Southern Great Plains (Shrivastava et al., 2019; Shilling et al., 2018; Zawadowicz et al., 2021; Wang et al., 2023a; Fast et al., 2024) The airborne data have also been used to examine the vertical variability in the aerosol properties over the Southern Great Plains, contributing to a better understanding of the distribution and impact of aerosols at different atmospheric levels (Wang et al., 2016; Fast et al., 2022).
This study further demonstrates the comparison of AAF data with ground-based remote-sensing retrievals. The ARM G-1 aircraft was deployed above or near the eastern North Atlantic (ENA) and Southern Great Plains (SGP) sites during field campaigns like ACE-ENA and HI-SCALE. Various instruments employing diverse observational techniques measured atmospheric parameters, aerosol, and cloud properties from the ground and airborne perspectives. These coordinated deployments enable a thorough assessment of robustness and statistical representativeness across co-located measurements.
Comparing airborne and ground-based measurements involves evaluating data from two platforms that differ with respect to their spatial and temporal resolutions and measurement techniques. Thus, three potential biases exist in the measurements – spatial, temporal, and instrumental. The instrumental bias is typically due to the differences in sensors, calibration, and data-processing techniques between the two platforms. Airborne measurements usually provide in situ spatiotemporal data over leveled flight legs at different altitudes and can capture data over various, even difficult-to-access, terrains. Meanwhile, ground-based remote-sensing data usually provide continuous monitoring at a fixed location with limited spatial coverage or less vertical resolution. Our efforts focus on minimizing the temporal and spatial biases to ensure accurate and meaningful comparisons. We selected the comparison period by aligning the data acquisition times for both airborne and ground-based measurements as closely as possible. To ensure that both airborne and ground-based measurements are georeferenced accurately. For instance, ground-based remote sensing uses height or the altitude above ground level (a.g.l.) as the vertical geographic coordinate. In contrast, airborne data usually use the mean sea level (m.s.l.) altitude, which can be converted to the altitude above ground level. We then use interpolation techniques to match the spatial resolutions of airborne and ground-based data.
3.1 Airborne data quality control
3.1.1 Aircraft-integrated meteorological measurement system data
The aircraft platform velocity, position, and attitude were monitored by four redundant sensors: DSM, C-MIGITS, VectorNav, and AIMMS-20, as shown in Table 2. The DSM is the primary choice with respect to the data source for the merged dataset, followed by the C-MIGITS, AIMMS-20, and VectorNav. For static temperature measurement, the uncertainty in the field data is ±0.5 K. The static pressure has a measurement uncertainty of 0.5 hPa. The standard measurement uncertainties were ±2 K for the chilled-mirror hygrometer. The AIMMS-20 provides both meteorological measurements and wind vectors (https://aventech.com/products/aimms20.html, last access: 24 April 2023). The relative humidity sensor has an accuracy of 2 % in the operating range of 0 %–100 %. A calibration flight pattern was performed with each installation of the AIMMS-20 in each field campaign, improving the high-resolution wind data accuracy. The AIMMS-20 calibration uses two different procedures: first, an aerodynamic calibration maneuver helps to determine aerodynamic errors induced by the aircraft itself; then, the aircraft is operated to complete an inertial system calibration maneuver and capture minor alignment errors (i.e., cross-axis error) between the gyros, accelerometers, GPS antenna baseline, and the primary reference frame of the inertial measurement unit (IMU). Combining the measurements from the instruments that make up the AIMMS-20 (the air data probe – ADP; the GPS; and the IMU) provides the wind speed with an accuracy of 0.5 m s−1 for the north and east components and an accuracy of 0.75 m s−1 for the vertical wind component.
The AIMMS-20 wind parameters, such as wind speed and wind direction, were compared with the ground-based wind parameters retrieved using the ARM Doppler lidar (DL) (Newsom et al., 2019), as shown in Fig. 3. The ARM DL is an active remote-sensing instrument that provides temporally and range-resolved measurements (Newsom and Krishnamurthy, 2022). The DOE ARM user facility operates several scanning coherent Doppler lidar systems in the near-infrared (1.5 µm) at ARM's ground-based observatories and mobile facilities (Newsom et al., 2017). The Doppler lidar horizontal wind profiles value-added product (DLPROF-WIND VAP, https://www.arm.gov/capabilities/vaps/dlprof-wind, last access: 24 April 2023) provides accurate height-resolved measurements of wind speed and direction at a 15 min resolution (Newsom et al., 2019). Figure 3 compares G-1 aircraft winds to DL winds for level flight legs under cloudless conditions during the ACE-ENA field campaign. The aircraft data were averaged to match the DL time interval when the aircraft location was within a 3 km distance of the ARM ground site. Limited by frequent clouds and the DL data availability, only leveled flight legs from 12 flights between 26 June and 19 July 2017 were included in the comparison. We achieved a reasonably good comparison between the AIMMS-20 and DL wind parameters, especially for the wind direction. The wind speed plot exhibits increased dispersion, particularly at lower wind speeds (<5 m s−1), possibly due to the heterogeneous nature of wind speeds at different heights in the Doppler lidar data. Notably, data from a single aircraft flight path may not accurately reflect the values measured by the Doppler lidar. It should be noted that there are fundamental differences in aircraft-based wind measurements (in situ, spatial averages) and the Doppler lidar technique (single point, time/height averaged), which never allows perfect agreement between the two datasets.
3.1.2 Aerosol payload data
Aerosol measurements aboard the G-1 platform were performed downstream of an isokinetic inlet with a Nafion dryer to ensure a relative humidity below 40 % for the air sample. The isokinetic aerosol inlet was designed and built by Brechtel Manufacturing Inc. (Hayward, CA) and modified by the Pacific Northwest National Laboratory (PNNL). Previous closure studies have shown that the isokinetic inlet allowed sampling of the aerosols up to a 5 µm aerodynamic diameter, and the transmission efficiency is around 50 % at 1.5 µm (Kassianov et al., 2015, 2018; Mei et al., 2020; Kassianov et al., 2021). The best-estimate aerosol size distribution (BEASD) data product was created by merging the aerosol size distribution from several (up to four) aerosol and cloud sensors under dry conditions (<40 % RH). Two aerosol spectrometers were used as primary data sources: the fast integrated mobility spectrometer (FIMS; 10–600 nm size range; 30 log-spaced bins, 16.31 bins per decade) and the passive cavity aerosol spectrometer (PCASP, 0.095–2.9 µm size range). As shown in Fig. 4, we demonstrate a reasonable agreement between the integrated total number concentration from the BEASD and the total number concentration measured by the condensation particle counter (CPC, TSI, model 3772, >10 nm). This CPC was calibrated following the World Calibration Center for Aerosol Physics (WCCAP) guidelines. Compared with the laboratory standard CPC, the typical uncertainty is ∼10 % (Mei et al., 2020). Two cloud probes were used as a secondary source of aerosol measurements to cover the super-micron size range: the cloud aerosol spectrometer (CAS, 0.55–12.73 µm) and the fast cloud droplet probe (FCDP, 0.75–13.49 µm). Aerosol chemical composition, measured by the Aerodyne high-resolution time-of-flight aerosol mass spectrometer (HR-ToF-AMS), was used to estimate the aerosol refractive index (RI), which is necessary for the correction of the aerosol equivalent optical size into the equivalent geometric size (Li et al., 2023; Hand and Kreidenweis, 2002; Freedman et al., 2009).
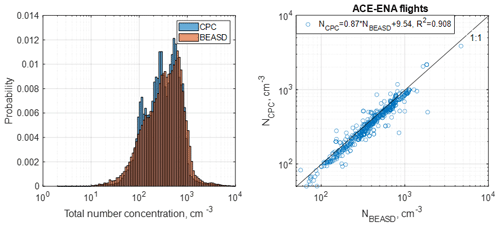
Figure 4Aerosol total number concentration comparison between the G-1 CPC 3772 and the best-estimate aerosol size distribution during the ACE-ENA flights.
The scattering and absorption coefficients are two essential parameters for understanding the optical properties of atmospheric aerosols, as they describe the scattering and attenuation of sunlight and the visibility of the atmosphere. The payload of the G-1 incorporated two aerosol optical instruments to measure these properties. A nephelometer (TSI, model 3563) was used to measure light scattering by aerosol particles at three wavelengths (Uin and Goldberger, 2020). It uses a light source to illuminate a sample of aerosol particles and measure the intensity of the total and backscattered light. The scattering coefficient from a nephelometer is defined as the ratio of the scattered light flux to the incident light flux and is typically expressed in units of inverse megameters (Mm−1). A particle soot absorption photometer (PSAP, Radiance Research) is used to estimate aerosol absorption by measuring the attenuation of a light beam passing through aerosols deposited on a filter (Springston, 2018). After correcting for the filter effect and scattering impacts on the absorption values, the amount of light absorbed by the particles for three wavelengths was recorded (Bond et al., 1999). Under dry conditions, the accuracies for the scattering and absorption coefficients are 25 % and 20 %, respectively (Rosati et al., 2016). The uncertainties in those airborne measurements might be even larger due to the complex field conditions. The sum of the absorption and scattering of aerosol particles (i.e., aerosol extinction) provides a measure of the effect of aerosols on radiant energy that passes through the atmosphere.
In addition, the aerosol extinction coefficient can be calculated from the aerosol size distribution and chemical composition using Mie theory with certain assumptions (Mie, 1908; Bohren and Huffman, 1998). Here, assuming spherical particles and a homogeneous composition in the estimated size range, we estimated the extinction coefficients of the aerosol particles and compared the values with the in situ measurements from the summation of the values from the PSAP and nephelometer in Fig. 5. The comparison data were only from level flight legs when aerosol sampling was under dry and isokinetic conditions. The BEASD estimation and the nephelometer and PSAP summation shown on the left-hand plot in Fig. 5 achieved a reasonably good agreement. The dots on the scatter plot shown on the right-hand side of Fig. 5 are color-coded by the relative humidity. This scatter plot indicates that the simple assumption, which ignores how the aerosol properties vary with particle size, shape, and refractive index and also neglects the ambient relative humidity effect, introduces uncertainty in the aerosol properties.
3.1.3 Cloud payload data
A variety of cloud optical sensors were deployed during the seven AAF campaigns. The cloud measurement methods can be separated into light scattering (FCDP) and shadow imaging (2D-S and HVPS), covering a wide range from small cloud droplets to precipitation elements. The FCDP measures cloud droplets in the size (diameter) range of 2–50 µm. Droplets are detected and sized depending on how much light they scatter in a specific angular range when illuminated by a focused laser beam (Lance et al., 2010). The 2D-S and HVPS are optical array probes that restore images from passing hydrometeors' shadows. Using two cameras positioned at different angles to create a stereo image of each cloud particle, 2D-S and HVPS provide information about the particle's size, shape, and orientation at different size ranges (Glienke and Mei, 2020, 2019). Based on previous studies, the undercounting bias of measured droplets between 3 and 20 µm diameter is around 20 %, whereas the uncertainty is up to 50 % for droplets with a diameter larger than 20 µm (Glienke et al., 2023; Mei et al., 2020). In addition, a merged size distribution based on the FCDP, 2D-S, and HVPS was created to cover the size range of cloud elements from 2 to 9075 µm.
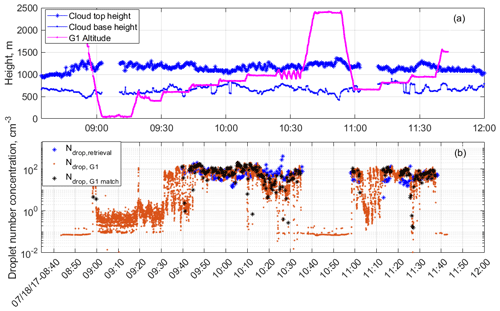
Figure 6Cloud droplet number concentration comparison between the integrated value from the merged cloud droplet size distribution and the ground-based Raman lidar retrieval value on 18 July 2017, during the ACE-ENA field campaign.
Figure 6a demonstrates one cloudy-day (stratocumulus cloud) case and shows three lines representing the cloud-top height, cloud-base height (retrieved from micropulse lidar), and aircraft G-1 altitude for the 18 July 2017 flight. The cloud extends from about 2 to 5 km altitude, with the cloud-top and cloud-base heights varying over time. The G-1 flew level through different cloud layers (09:40–10:35 and 11:00–11:40 UTC). The G-1 also porpoises in and out of the cloud between 10:27 and 10:33 UTC.
Figure 6b compares the time series of the total droplet concentration from three different sources. The blue stars depict the droplet concentration retrieved from the Raman lidar measurements, the brownish dots show the droplet concentration measured by the G-1 cloud probe, and the black stars show the droplet concentration by the G-1 measurements averaged to the lidar retrieval times. The cloud droplet number concentration (Ndrop, retrieval) is derived using the algorithms/methods developed by Snider et al. (2017), based on the ground-based Raman lidar particulate extinction profile from the Raman Lidar Vertical Profiles – Feature Detection and Extinction (RLPROF-FEX) value-added product (Chand et al., 2023). The Ndrop, retrieval achieves good results for stratocumulus cloud, while the cloud liquid water content profiles are closer to adiabatic/pseudo-adiabatic conditions. The lidar retrievals and the G-1 cloud probe measurements agree well, while the lidar-retrieved value has a lower droplet concentration near the cloud base between 9:40 and 9:55 UTC and between 11:10 and 11:40 UTC.
A statistical comparison of the total droplet number concentration (Ndrop) between the G-1 measurements and the lidar retrieval shows that the two measurements are highly correlated in the concentration ranges up to 200 cm−3, and the G-1 cloud probe measures a slightly higher droplet concentration than the lidar retrieval. Recent studies (Tang et al., 2022, 2023; Zhang et al., 2023) have also pointed out the need to develop appropriate criteria to quantify the cloud remote-sensing retrievals better using in situ measurement, as the lidar is looking straight up and measuring clouds passing over, while the aircraft is flying legs overhead and probing different cloud layers. The large discrepancy might be due to the cloud retrievals mainly capturing the cloud base, whereas the G-1 samples through a portion of the cloud.
3.2 Earth system model aerosol–cloud diagnostics package evaluation
An Earth system model (ESM) aerosol–cloud diagnostics package, known as ESMAC Diags (Tang et al., 2022, 2023), has been developed to facilitate the routine evaluation of aerosol, cloud, and aerosol–cloud interactions quantities simulated by the DOE's Energy Exascale Earth System Model (E3SM) using in situ surface, aircraft, and ship measurements. ESMAC Diags reads in datasets from selected field campaigns and model outputs with some processing and quality controls; it then generates a set of diagnostics plots and metrics, such as the mean, root-mean-square error, and correlation of aerosol and cloud variables. To run the simulation for comparison with aircraft field campaign data, we configure the model according to the Atmospheric Model Intercomparison Project protocol (Gates et al., 1999), using real-world initial conditions and nudging simulated winds toward Modern-Era Retrospective analysis for Research and Applications (MERRA-2) reanalysis data (Gelaro et al., 2017). Then, we save the hourly output for the field campaign area and utilize an “aircraft simulator” strategy (Fast et al., 2011) to extract the closest model grid and level-matching aircraft measurements. More details about the model configurations can be found in Tang et al. (2022).
With this diagnostic package, various types of diagnostics and evaluation metrics are performed for the aerosol number, size distribution, chemical composition, cloud condensation nuclei (CCN) concentration, and various meteorological quantities to assess how well the E3SM represents observed aerosol properties across spatial scales (Tang et al., 2022). Data from two ARM airborne field campaigns (HI-SCALE and ACE-ENA) have been included in the current version of the ESMAC Diags package. The integrated dataset discussed in this study provides a more consistent input data format than prior data files for ESMAC Diags. One example is shown in Fig. 7. During the intensive operation period (IOP) in the ACE-ENA field campaign (between 15 June and 20 July 2017), three aerosol number concentrations (for aerosol particles larger than 3, 10, and 100 nm) and the cloud condensation nuclei concentration (at 0.1 % supersaturation) were compared using an E3SM model (version 2) simulation with the ESMAC Diags package. Although the E3SM qualitatively reproduced the observation, it overestimated accumulation-mode aerosols and CCN concentration over the ENA regions. In addition, larger discrepancies were observed at lower altitudes (<500 m a.g.l.) for the aerosol number concentrations (>3 and >10 nm), which might be due to the weak representation of nucleation-mode aerosol in the model. It did not capture the vertical variation in the CCN concentration, which indicates that process-level improvement is still needed for the E3SM over the Atlantic Ocean.
3.3 Data collection – challenges and future potential
We summarize the challenges and data collection limitations encountered during the seven field campaigns in Table 3. The lessons learned from these campaigns suggest that ensuring data quality and enhancing data collection variability require the following key strategies:
-
regular sensor calibration to maintain accuracy with scheduled, impromptu, and well-documented validation methods ensuring reliable measurements;
-
cross-validation and monitoring of sensor performance using redundancy, dataset fusion, and statistical techniques to identify inconsistencies and malfunctions;
-
diversified sampling strategies to ensure comprehensive data representation across varying conditions;
-
the leveraging of model simulations and statistical studies based on previous measurements to refine data collection methods and anticipate potential issues, leading to more effective and targeted data acquisition.
These combined approaches ensure robust data collection, improve measurement accuracy, and provide reliable data products for the community of users.
Future work can expand data collection and merge and facilitate further investigations into atmospheric chemistry, aerosol properties, aerosol–cloud interactions and their representation in Earth system models. To support future research needs, the ARM Data Center plans to work with the AAF instrument mentors and community experts to standardize this merged data product. Future deployments will use a new airborne platform (Challenger 850) and include more baseline airborne measurements (https://arm.gov/capabilities/instruments?type[0]=armobs&category[0]=Airborne Observations, last access: 24 April 2023). For example, we plan to add the liquid water content measurements from the Multi-Element Water Content System (https://arm.gov/capabilities/instruments/wcm-air, last access: 24 April 2023) and solar radiation measurements from multi-filter radiometers (https://arm.gov/capabilities/instruments/mfr-air, last access: 24 April 2023) into the future merged dataset.
In addition to the baseline measurements, we plan to offer data integration options through the ARM Data Integrator tool (details in Sect. 4), allowing campaign principal investigators or community users to flexibly incorporate additional data into the merged data product. This approach provides users with the ability to target specific science themes. For example, one proposed data product is to include additional aerosol optical properties with this AAF merged dataset. The atmospheric community can expand research on the evolution of aerosol particles from wildfires, particularly on how different combustion phases (flaming vs. smoldering) result in varying chemical compositions and quantities of emitted aerosols with additional chemical composition and gas-phase concentration data. This custom-built merged dataset is crucial for improving air quality models and understanding the climate impacts of biomass burning. Continuing to investigate the influence of urban pollution on natural aerosol formation, similar to the studies conducted in the Amazon during GoAmazon2014/5, will provide new insights into aerosol composition. Potential data for such a study could be non-airborne remote-sensing data. Combining airborne data with ground-based remote-sensing data allows the exploration of interactions between different aerosol types and evolving cloud and precipitation patterns. Quantifying these interactions can improve models and understanding, aiding the development of strategies for mitigating anthropogenic impacts on natural environments.
By strategically combining long-term ground-based remote-sensing measurements with high-resolution airborne data, researchers can achieve more robust analyses of atmospheric processes, leading to more accurate scientific findings and better-constrained models. For instance, ground-based sensors can continuously monitor a specific location, while targeted airborne missions can capture critical in situ measurements during specific events that are not retrievable by remote sensing to better study cloud evolution, pollutant transport, or extreme weather.
The ARM data management system treats all data from the G-1 airborne deployments as field campaign data streams, meaning the data were collected during the intensive observational periods rather than over long-term observation. The data (https://doi.org/10.5439/1999133; Mei and Gaustad, 2024) in this paper were produced following ARM data file standards and archived through the ARM data ingestion process (Prakash et al., 2016) under a Creative Commons license. In 2016, the ARM G-1 raw instrument data were directly “ingested” into NetCDF format and archived automatically during and after flight operations. To provide a dataset with a uniform data format, the G-1 payload scientists (ARM mentors) reprocessed data for field campaigns between 2013 and 2015 and converted the historical data from ICARTT to NetCDF. Note that a specific directory was created for reviewers to access the data at https://doi.org/10.5439/1999133 (Mei and Gaustad, 2024).
The ARM data system uses a multitiered data-processing approach (Prakash et al., 2016) that iteratively processes the instrument data to produce higher-level data products. Data are first processed from the instrument's raw data format (data level 00) to NetCDF format. During this initial processing, the conversion of geophysical units and the application of calibration factors are performed as appropriate (documented in a- and b-level data products). Quality controls can also be applied (creating b1-level data files). Additional processing can be added to further increase the level of these files with “higher value” to store as a data level “c1”. For example, mentor-edited data files with additional quality improvement calibration are usually considered the c-level data product.
The content of ARM data files is structured in three main sections: dimensions, variables, and global attributes. As time-series measurements, the time dimension of ARM products is considered “unlimited”. Per NetCDF-3 requirements, the unlimited dimension “time” is the first dimension of a variable that uses the time dimension. The variables encompass coordinate variables (reporting dimension values), primary measurements (recommended for scientific use), supporting measurements (e.g., diagnostics and quality), and location variables (detailing latitude, longitude, and altitude). Variables are equipped with supporting attributes to facilitate the user's understanding and interpretation. These include a “long_name” for unique descriptions, “units” conforming to unit conventions, and a “missing_value” to represent no data. A “standard_name” attribute, following the Climate Forecast (CF) standard, is assigned when applicable. The final section in ARM NetCDF files consists of global attributes containing information related to the platform's location, time interval, calibration procedures (if available), and contact information for instrument mentors or principal investigators.
A final merged product (aafmerged.c1) was created to provide users with all G-1 airborne measurements in a single file. This merged data product is produced using the ARM Data Integrator (ADI, https://doi.org/10.5439/1999133, Mei and Gaustad, 2024), a framework designed to automate data retrieval, integration, and the creation of time-series NetCDF data products. The ADI allows users to seamlessly combine data products, extract specific variables, and transform them into user-defined coordinate systems. The time dimension of the merged data product aligns with the input aafnaviwg.c1 data stream. All other instrument data were mapped onto this sampling period using ADI's nearest-neighbor transformation method.
All data products produced by the ARM data system (Prakash et al., 2016) adhere to ARM data standards and are made available to the user community via the ARM Data Center in files using the following naming convention (as detailed in Table 4): (sss)(inst)(qualifier)(temporal)(Fn).(dl).(yyyymmdd).
(hhmmss).nc.
For example, a NetCDF file produced for the G-1 airborne deployment at the ACE-ENA field campaign that includes quality-controlled data (in geophysical units) collected starting at 08:31:45 UTC on 18 July 2017, is named enaaafF1.b1.20170718.083145.nc.
All AAF data collected and ingested after 2016 align with the ARM standard data process and format described above. Due to the complex nature of various airborne measurements, some G-1 instrument data have been edited by the mentor (such as the cloud probe) to add additional value. These mentor-edited data are directly ingested from raw data into c1-level data products. For the historical campaign data before 2016, we reprocessed the AAF data following the standard process if the raw data were in a similar format; otherwise, the mentor-edited data were used as the input variables to the ADI process to create the aafmerged.c1 products. Details about individual data points included in the final merged data file for each field campaign are listed in Table S2, as are the primary measurements and their associated standard names used in the recommended aafmerged.c1 product. A “standard_name” is listed in Table S4 for the primary variables, which is consistent with the naming convention based on the CF standard.
ARM includes sufficient metadata in each NetCDF file to facilitate the user's understanding and interpretation. The public data usage rights are as follows: this work is licensed under the Creative Commons Attribution 4.0 International License. To view a copy of this license, visit https://creativecommons.org/licenses/by/4.0/ (last access: 19 January 2023).
This paper provides an overview of the platform, the aircraft instrumentation, flight tracks, and data collected during the ARM airborne field campaigns and introduces information on data quality control. While numerous studies based on AAF data have both directly and indirectly demonstrated the quality of the datasets, this paper further reinforces this by providing specific examples. It compares in situ measurements with other co-located observations, offering additional evidence to underscore the reliability of the AAF data. A merged dataset containing each flight's meteorological, aerosol, and cloud information was generated for seven AAF field campaigns between 2013 and 2018. The data from 766.4 h of research flights were collected over multiple continents and under various environmental conditions.
Four of the seven field campaigns were based in the USA. One campaign collected data from the wildfires in the US Pacific Northwest and agricultural burns in the lower Mississippi River valley as part of the BBOP in 2013. In 2015, ACAPEX provided data on atmospheric rivers and associated aerosol–cloud interactions that produce heavy precipitation on the US West Coast during the early spring. Research data from ACME V, collected during the summer of 2015, gave scientists insight into trends and variability in trace gases in the atmosphere over the North Slope of Alaska to improve Arctic climate models. In the early summer and autumn of 2016, HI-SCALE provided an extensive dataset geared toward coupled processes that affect the life cycle of shallow clouds through the interaction among aerosol, cloud, the land surface, and ecosystems.
In 2014 (March and October), the airborne sampling moved outside of the USA to Manus in the central Amazon, Brazil, where residential and industrial emissions were extensively characterized by G-1 flights. The GoAmazon2014/15 aircraft campaign data are being integrated with aquatic and terrestrial ecosystem measurements to quantify anthropogenic perturbations to a usually pristine tropical environment. Another international airborne mission was carried out in the eastern North Atlantic region. The ACE-ENA campaign saw the G-1 aircraft fly from Terceira Island in the Azores during the summer of 2017 and the winter of 2018. The campaign studied both seasons to measure key aerosol and cloud processes under various meteorological and cloud conditions with different aerosol sources. The G-1 was then deployed to the Sierras de Córdoba range in central Argentina from October to November 2018 to study orographic convective cloud interactions with their surrounding environment.
The combined observational data from these field campaigns facilitate the study of atmospheric processes, such as boundary layer processes, aerosol–cloud–precipitation interactions, and land–atmosphere–cloud interactions across a wide range of conditions. Although each field campaign faced different challenges and data collection limitations, many previous studies have benefited from the G-1 field campaign data (Gu et al., 2017; Creamean et al., 2018; Fast et al., 2019b; Shrivastava et al., 2019; Berg et al., 2020; Yeom et al., 2021; Zhang et al., 2021; Wang et al., 2023a, b; Zhang et al., 2023), and more manageable data access would further support new users in the research community. By incorporating data from multiple sources, these ARM datasets and open-source tools can provide more accurate and reliable information and assist the model simulation/prediction improvement. Overall, a merged airborne aerosol, cloud, and trace gas dataset covering seven field campaigns is a powerful tool for atmospheric scientists, supporting a more comprehensive understanding of atmosphere processes impacting the climate. We hope our efforts will encourage broader usage of the ARM data and enhance the collaboration between the ARM user facility and the atmospheric science community.
The supplement related to this article is available online at: https://doi.org/10.5194/essd-16-5429-2024-supplement.
FM led the formulation of this paper. FM and ST provided the figures. JMT, FM, MSP, JES, JMC, DZ, and BS participated in the data collection and preparation of the field campaigns. JDF, ACV, JW, SM, SCB, LRL, and LK led the field campaigns and provided revision comments on the manuscript. BDE, KLG, and KWB worked on the data formatting and merging processes. FM wrote the draft of the manuscript, and all coauthors provided editing suggestions.
The contact author has declared that none of the authors has any competing interests.
Publisher's note: Copernicus Publications remains neutral with regard to jurisdictional claims made in the text, published maps, institutional affiliations, or any other geographical representation in this paper. While Copernicus Publications makes every effort to include appropriate place names, the final responsibility lies with the authors.
This work has been supported by the Office of Biological and Environmental Research (OBER) of the US Department of Energy (DOE) as part of the Atmospheric Radiation Measurement (ARM) user facility and the Atmospheric System Research (ASR) program. ST and JDF were also supported by the Enabling Aerosol–cloud interactions at GLobal convection-permitting scalES (EAGLES) project (project no. 74358), funded by the US Department of Energy, Office of Science, Office of Biological and Environmental Research, Earth System Model Development (ESMD) program area. Battelle operates the Pacific Northwest National Laboratory (PNNL) for the DOE under contract no. DE-AC05-76RL0 1830. This work benefited from the assistance of OpenAI's ChatGPT for refining the language used in this document.
This research has been supported by the Biological and Environmental Research (grant no. DE-AC05-76RL0 1830).
This paper was edited by Jing Wei and reviewed by two anonymous referees.
Berg, L. K., Long, C. N., Kassianov, E. I., Chand, D., Tai, S.-L., Yang, Z., Riihimaki, L. D., Biraud, S. C., Tagestad, J., Matthews, A., Mendoza, A., Mei, F., Tomlinson, J., and Fast, J. D.: Fine-Scale Variability of Observed and Simulated Surface Albedo Over the Southern Great Plains, J. Geophys. Res.-Atmos., 125, e2019JD030559, https://doi.org/10.1029/2019jd030559, 2020.
Bohren, C. F. and Huffman, D. R.: Absorption and Scattering by a Sphere, in: Absorption and Scattering of Light by Small Particles, 82–129, https://doi.org/10.1002/9783527618156.ch4, 1998.
Bond, T. C., Anderson, T. L., and Campbell, D.: Calibration and Intercomparison of Filter-Based Measurements of Visible Light Absorption by Aerosols, Aerosol Sci. Technol., 30, 582–600, https://doi.org/10.1080/027868299304435, 1999.
Brenguier, J.-L., Bachalo, W. D., Chuang, P. Y., Esposito, B. M., Fugal, J., Garrett, T., Gayet, J.-F., Gerber, H., Heymsfield, A., Kokhanovsky, A., Korolev, A., Lawson, R. P., Rogers, D. C., Shaw, R. A., Strapp, W., and Wendisch, M.: In Situ Measurements of Cloud and Precipitation Particles, in: Airborne Measurements for Environmental Research, 225–301, https://doi.org/10.1002/9783527653218.ch5, 2013.
Chand, D., Newsom, R., Thorsen, T., Cromwell, E., Sivaraman, C., Flynn, C., Shilling, J., and Comstock, J. M.: Aerosol and Cloud Optical Properties from the ARM Raman Lidars: The Feature Detection and Extinction (FEX) Value-Added Product, ARM user facility, https://doi.org/10.2172/1560857, 2023.
Collier, S., Zhou, S., Onasch, T. B., Jaffe, D. A., Kleinman, L., Sedlacek, A. J., 3rd, Briggs, N. L., Hee, J., Fortner, E., Shilling, J. E., Worsnop, D., Yokelson, R. J., Parworth, C., Ge, X., Xu, J., Butterfield, Z., Chand, D., Dubey, M. K., Pekour, M. S., Springston, S., and Zhang, Q.: Regional Influence of Aerosol Emissions from Wildfires Driven by Combustion Efficiency: Insights from the BBOP Campaign, Environ. Sci. Technol., 50, 8613–8622, https://doi.org/10.1021/acs.est.6b01617, 2016.
Creamean, J. M., Maahn, M., de Boer, G., McComiskey, A., Sedlacek, A. J., and Feng, Y.: The influence of local oil exploration and regional wildfires on summer 2015 aerosol over the North Slope of Alaska, Atmos. Chem. Phys., 18, 555–570, https://doi.org/10.5194/acp-18-555-2018, 2018.
Fan, J., Rosenfeld, D., Zhang, Y., Giangrande, S. E., Li, Z., Machado, L. A. T., Martin, S. T., Yang, Y., Wang, J., Artaxo, P., Barbosa, H. M. J., Braga, R. C., Comstock, J. M., Feng, Z., Gao, W., Gomes, H. B., Mei, F., Pohlker, C., Pohlker, M. L., Poschl, U., and de Souza, R. A. F.: Substantial convection and precipitation enhancements by ultrafine aerosol particles, Science, 359, 411–418, https://doi.org/10.1126/science.aan8461, 2018.
Fast, J. D., Gustafson, W. I., Chapman, E. G., Easter, R. C., Rishel, J. P., Zaveri, R. A., Grell, G. A., and Barth, M. C.: The Aerosol Modeling Testbed: A Community Tool to Objectively Evaluate Aerosol Process Modules, B. Am. Meteorol. Soc., 92, 343–360, https://doi.org/10.1175/2010BAMS2868.1, 2011.
Fast, J. D., Berg, L. K., Alexander, L., Bell, D., D'Ambro, E., Hubbe, J., Kuang, C. A., Liu, J. M., Long, C., Matthews, A., Mei, F., Newsom, R., Pekour, M., Pinterich, T., Schmid, B., Schobesberger, S., Shilling, J., Smith, J. N., Springston, S., Suski, K., Thornton, J. A., Tomlinson, J., Wang, J., Xiao, H., and Zelenyuk, A.: Overview of the HI-SCALE Field Campaign: A New Perspective on Shallow Convective Clouds, B. Am. Meteorol. Soc., 100, 821–840, https://doi.org/10.1175/bams-d-18-0030.1, 2019a.
Fast, J. D., Berg, L. K., Feng, Z., Mei, F., Newsom, R., Sakaguchi, K., and Xiao, H.: The Impact of Variable Land-Atmosphere Coupling on Convective Cloud Populations Observed During the 2016 HI-SCALE Field Campaign, J. Adv. Model. Earth Syst., 11, 2629–2654, https://doi.org/10.1029/2019ms001727, 2019b.
Fast, J. D., Bell, D. M., Kulkarni, G., Liu, J., Mei, F., Saliba, G., Shilling, J. E., Suski, K., Tomlinson, J., Wang, J., Zaveri, R., and Zelenyuk, A.: Using aircraft measurements to characterize subgrid-scale variability of aerosol properties near the Atmospheric Radiation Measurement Southern Great Plains site, Atmos. Chem. Phys., 22, 11217–11238, https://doi.org/10.5194/acp-22-11217-2022, 2022.
Fast, J. D., Varble, A. C., Mei, F., Pekour, M., Tomlinson, J., Zelenyuk, A., Sedlacek III, A. J., Zawadowicz, M., and Emmons, L. K.: Large Spatiotemporal Variability in Aerosol Properties over Central Argentina during the CACTI Field Campaign, EGUsphere [preprint], https://doi.org/10.5194/egusphere-2024-1349, 2024.
Freedman, M. A., Hasenkopf, C. A., Beaver, M. R., and Tolbert, M. A.: Optical Properties of Internally Mixed Aerosol Particles Composed of Dicarboxylic Acids and Ammonium Sulfate, J. Phys. Chem. A, 113, 13584–13592, https://doi.org/10.1021/jp906240y, 2009.
Gates, W. L., Boyle, J. S., Covey, C., Dease, C. G., Doutriaux, C. M., Drach, R. S., Fiorino, M., Gleckler, P. J., Hnilo, J. J., Marlais, S. M., Phillips, T. J., Potter, G. L., Santer, B. D., Sperber, K. R., Taylor, K. E., and Williams, D. N.: An Overview of the Results of the Atmospheric Model Intercomparison Project (AMIP I), B. Am. Meteorol. Soc., 80, 29–56, https://doi.org/10.1175/1520-0477(1999)080<0029:AOOTRO>2.0.CO;2, 1999.
Gaustad, K., Shippert, T., Ermold, B., Beus, S., Daily, J., Borsholm, A., and Fox, K.: A scientific data processing framework for time series NetCDF data, Environ. Model. Softw., 60, 241–249, https://doi.org/10.1016/j.envsoft.2014.06.005, 2014.
Gelaro, R., McCarty, W., Suárez, M. J., Todling, R., Molod, A., Takacs, L., Randles, C. A., Darmenov, A., Bosilovich, M. G., Reichle, R., Wargan, K., Coy, L., Cullather, R., Draper, C., Akella, S., Buchard, V., Conaty, A., da Silva, A. M., Gu, W., Kim, G.-K., Koster, R., Lucchesi, R., Merkova, D., Nielsen, J. E., Partyka, G., Pawson, S., Putman, W., Rienecker, M., Schubert, S. D., Sienkiewicz, M., and Zhao, B.: The Modern-Era Retrospective Analysis for Research and Applications, Version 2 (MERRA-2), J. Climate, 30, 5419–5454, https://doi.org/10.1175/JCLI-D-16-0758.1, 2017.
Glienke, S. and Mei, F.: Two-Dimensional Stereo (2D-S) Probe Instrument Handbook, ARM user facility, https://doi.org/10.2172/1597436, 2019.
Glienke, S. and Mei, F.: High-Volume Precipitation Spectrometer (HVPS) Instrument Handbook, ARM user facility, https://doi.org/10.2172/1597643, 2020.
Glienke, S., Allwayin, N., Mei, F., Comstock, J. M., Shaw, R. A., Tomlinson, J., and Schmid, B.: Performance of optical sensors for cloud measurements deployed by the ARM Aerial Facility during ACE-ENA, Appl. Optics, 62, 5282–5293, https://doi.org/10.1364/AO.491401, 2023.
Gu, D., Guenther, A. B., Shilling, J. E., Yu, H., Huang, M., Zhao, C., Yang, Q., Martin, S. T., Artaxo, P., Kim, S., Seco, R., Stavrakou, T., Longo, K. M., Tota, J., Ferreira de Souza, R. A., Vega, O., Liu, Y., Shrivastava, M., Alves, E. G., Santos, F. C., Leng, G., and Hu, Z.: Airborne observations reveal elevational gradient in tropical forest isoprene emissions, Nat. Commun., 8, 15541, https://doi.org/10.1038/ncomms15541, 2017.
Hand, J. L. and Kreidenweis, S. M.: A New Method for Retrieving Particle Refractive Index and Effective Density from Aerosol Size Distribution Data, Aerosol Sci. Technol., 36, 1012–1026, https://doi.org/10.1080/02786820290092276, 2002.
IPCC: Climate Change 2021: The Physical Science Basis. Contribution of Working Group I to the Sixth Assessment Report of the Intergovernmental Panel on Climate Change, edited by: Masson-Delmotte, V., Zhai, P., Pirani, A., Connors, S. L., Péan, C., Berger, S., Caud, N., Chen, Y., Goldfarb, L., Gomis, M. I., Huang, M., Leitzell, K., Lonnoy, E., Matthews, J. B. R., Maycock, T. K., Waterfield, T., Yelekçi, O., Yu, R., and Zhou, B., Cambridge University Press, Cambridge, United Kingdom and New York, NY, USA, 2391 pp., https://doi.org/10.1017/9781009157896, 2021.
Junghenn Noyes, K., Kahn, R., Sedlacek, A., Kleinman, L., Limbacher, J., and Li, Z.: Wildfire Smoke Particle Properties and Evolution, from Space-Based Multi-Angle Imaging, Remote Sens., 12, 769, https://doi.org/10.3390/rs12050769, 2020.
Kassianov, E., Berg, L. K., Pekour, M., Barnard, J., Chand, D., Flynn, C., Ovchinnikov, M., Sedlacek, A., Schmid, B., Shilling, J., Tomlinson, J., and Fast, J.: Airborne Aerosol in Situ Measurements during TCAP: A Closure Study of Total Scattering, Atmosphere, 6, 1069–1101, 2015.
Kassianov, E., Berg, L. K., Pekour, M., Barnard, J., Chand, D., Comstock, J., Flynn, C., Sedlacek, A., Shilling, J., Telg, H., Tomlinson, J., Zelenyuk, A., and Fast, J.: A Closure Study of Total Scattering Using Airborne In Situ Measurements from the Winter Phase of TCAP, Atmosphere, 9, 228, https://doi.org/10.3390/atmos9060228, 2018.
Kassianov, E., Pekour, M., Barnard, J., Flynn, C. J., Mei, F., and Berg, L. K.: Estimation of Aerosol Columnar Size Distribution from Spectral Extinction Data in Coastal and Maritime Environment, Atmosphere, 12, 1412, https://doi.org/10.3390/atmos12111412, 2021.
Kleinman, L. I., Sedlacek III, A. J., Adachi, K., Buseck, P. R., Collier, S., Dubey, M. K., Hodshire, A. L., Lewis, E., Onasch, T. B., Pierce, J. R., Shilling, J., Springston, S. R., Wang, J., Zhang, Q., Zhou, S., and Yokelson, R. J.: Rapid evolution of aerosol particles and their optical properties downwind of wildfires in the western US, Atmos. Chem. Phys., 20, 13319–13341, https://doi.org/10.5194/acp-20-13319-2020, 2020.
Krämer, M., Twohy, C., Hermann, M., Afchine, A., Dhaniyala, S., and Korolev, A.: Aerosol and Cloud Particle Sampling, in: Airborne Measurements for Environmental Research, 303–341, https://doi.org/10.1002/9783527653218.ch6, 2013.
Kulkarni, G., Mei, F., Shilling, J. E., Wang, J., Reveggino, R. P., Flynn, C., Zelenyuk, A., and Fast, J.: Cloud Condensation Nuclei Closure Study Using Airborne Measurements Over the Southern Great Plains, J. Geophys. Res.-Atmos., 128, e2022JD037964, https://doi.org/10.1029/2022jd037964, 2023.
Lacher, L., DeMott, P. J., Levin, E. J. T., Suski, K. J., Boose, Y., Zipori, A., Herrmann, E., Bukowiecki, N., Steinbacher, M., Gute, E., Abbatt, J. P. D., Lohmann, U., and Kanji, Z. A.: Background Free-Tropospheric Ice Nucleating Particle Concentrations at Mixed-Phase Cloud Conditions, J. Geophys. Res.-Atmos., 123, 10506–10525, https://doi.org/10.1029/2018jd028338, 2018.
Lance, S., Brock, C. A., Rogers, D., and Gordon, J. A.: Water droplet calibration of the Cloud Droplet Probe (CDP) and in-flight performance in liquid, ice and mixed-phase clouds during ARCPAC, Atmos. Meas. Tech., 3, 1683–1706, https://doi.org/10.5194/amt-3-1683-2010, 2010.
Levin, E. J. T., DeMott, P. J., Suski, K. J., Boose, Y., Hill, T. C. J., McCluskey, C. S., Schill, G. P., Rocci, K., Al-Mashat, H., Kristensen, L. J., Cornwell, G., Prather, K., Tomlinson, J., Mei, F., Hubbe, J., Pekour, M., Sullivan, R., Leung, L. R., and Kreidenweis, S. M.: Characteristics of Ice Nucleating Particles in and Around California Winter Storms, J. Geophys. Res.-Atmos., 124, 11530–11551, https://doi.org/10.1029/2019jd030831, 2019.
Li, Y., Bai, B., Dykema, J., Shin, N., Lambe, A. T., Chen, Q., Kuwata, M., Ng, N. L., Keutsch, F. N., and Liu, P.: Predicting Real Refractive Index of Organic Aerosols From Elemental Composition, Geophys. Res. Lett., 50, e2023GL103446, https://doi.org/10.1029/2023GL103446, 2023.
Maahn, M., de Boer, G., Creamean, J. M., Feingold, G., McFarquhar, G. M., Wu, W., and Mei, F.: The observed influence of local anthropogenic pollution on northern Alaskan cloud properties, Atmos. Chem. Phys., 17, 14709–14726, https://doi.org/10.5194/acp-17-14709-2017, 2017.
Martin, S. T., Artaxo, P., Machado, L., Manzi, A. O., Souza, R. A. F., Schumacher, C., Wang, J., Biscaro, T., Brito, J., Calheiros, A., Jardine, K., Medeiros, A., Portela, B., de Sá, S. S., Adachi, K., Aiken, A. C., Albrecht, R., Alexander, L., Andreae, M. O., Barbosa, H. M. J., Buseck, P., Chand, D., Comstock, J. M., Day, D. A., Dubey, M., Fan, J., Fast, J., Fisch, G., Fortner, E., Giangrande, S., Gilles, M., Goldstein, A. H., Guenther, A., Hubbe, J., Jensen, M., Jimenez, J. L., Keutsch, F. N., Kim, S., Kuang, C., Laskin, A., McKinney, K., Mei, F., Miller, M., Nascimento, R., Pauliquevis, T., Pekour, M., Peres, J., Petäjä, T., Pöhlker, C., Pöschl, U., Rizzo, L., Schmid, B., Shilling, J. E., Dias, M. A. S., Smith, J. N., Tomlinson, J. M., Tóta, J., and Wendisch, M.: The Green Ocean Amazon Experiment (GoAmazon2014/5) Observes Pollution Affecting Gases, Aerosols, Clouds, and Rainfall over the Rain Forest, B. Am. Meteorol. Soc., 98, 981–997, https://doi.org/10.1175/bams-d-15-00221.1, 2017.
Mather, J. H. and Voyles, J. W.: The ARM Climate Research Facility: A review of structure and capabilities, B. Am. Meteorol. Soc., 94, 377–392, 2013.
McCord, R. and Voyles, J.: The ARM Data System and Archive, Meteorological Monographs, 57, 11.11–11.15, https://doi.org/10.1175/AMSMONOGRAPHS-D-15-0043.1, 2016.
McFarquhar, G., Schmid, B., Korolev, A., Ogren, J. A., Russell, P. B., Tomlinson, J., Turner, D. D., and Wiscombe, W.: Airborne Intrumentation Needs for Climate and Atmospheric Research, B. Am. Meteorol. Soc., 92, 1193–1196, https://doi.org/10.1175/2011BAMS3180.1, 2011.
McQuaid, J., Schlager, H., Andrés-Hernández, M. D., Ball, S., Borbon, A., Brown, S. S., Catoire, V., Di Carlo, P., Custer, T. G., von Hobe, M., Hopkins, J., Pfeilsticker, K., Röckmann, T., Roiger, A., Stroh, F., Williams, J., and Ziereis, H.: In Situ Trace Gas Measurements, in: Airborne Measurements for Environmental Research, 77–155, https://doi.org/10.1002/9783527653218.ch3, 2013.
Mech, M., Maahn, M., Kneifel, S., Ori, D., Orlandi, E., Kollias, P., Schemann, V., and Crewell, S.: PAMTRA 1.0: the Passive and Active Microwave radiative TRAnsfer tool for simulating radiometer and radar measurements of the cloudy atmosphere, Geosci. Model Dev., 13, 4229–4251, https://doi.org/10.5194/gmd-13-4229-2020, 2020.
Mei, F. and Gaustad, K.: ARM airborne field campaign data products aboard Gulfstream 159. Between 2013 and 2018, CACTI, ACE-ENA, HiScale, ACME-V, ACAPEX, GoAmazon, BBOP. Atmospheric Radiation Measurement (ARM) user facility, ARM Data Center: Oak Ridge, Tennessee, USA [data set], https://doi.org/10.5439/1999133 (last access: 9 January 2024), 2024, updated periodically.
Mei, F., Wang, J., Comstock, J. M., Weigel, R., Krämer, M., Mahnke, C., Shilling, J. E., Schneider, J., Schulz, C., Long, C. N., Wendisch, M., Machado, L. A. T., Schmid, B., Krisna, T., Pekour, M., Hubbe, J., Giez, A., Weinzierl, B., Zoeger, M., Pöhlker, M. L., Schlager, H., Cecchini, M. A., Andreae, M. O., Martin, S. T., de Sá, S. S., Fan, J., Tomlinson, J., Springston, S., Pöschl, U., Artaxo, P., Pöhlker, C., Klimach, T., Minikin, A., Afchine, A., and Borrmann, S.: Comparison of aircraft measurements during GoAmazon2014/5 and ACRIDICON-CHUVA, Atmos. Meas. Tech., 13, 661–684, https://doi.org/10.5194/amt-13-661-2020, 2020.
Mie, G.: Beiträge zur Optik trüber Medien, speziell kolloidaler Metallösungen, Annalen der Physik, 330, 377–445, https://doi.org/10.1002/andp.19083300302, 1908.
Newsom, R. K. and Krishnamurthy, R.: Doppler Lidar (DL) instrument handbook, No. DOE/SC-ARM/TR-101, DOE Office of Science Atmospheric Radiation Measurement (ARM) User Facility, 2022.
Newsom, R., Sivaraman, C., Shippert, T., and Riihimaki, L.: Doppler lidar wind value-added product, DOE ARM Climate Research Facility, Washington, DC (United States), 2019.
Newsom, R. K., Brewer, W. A., Wilczak, J. M., Wolfe, D. E., Oncley, S. P., and Lundquist, J. K.: Validating precision estimates in horizontal wind measurements from a Doppler lidar, Atmos. Meas. Tech., 10, 1229–1240, https://doi.org/10.5194/amt-10-1229-2017, 2017.
O'Donnell, S. E., Akherati, A., He, Y., Hodshire, A. L., Shilling, J. E., Kuang, C., Fast, J. D., Mei, F., Schobesberger, S., Thornton, J. A., Smith, J. N., Jathar, S. H., and Pierce, J. R.: Look Up: Probing the Vertical Profile of New Particle Formation and Growth in the Planetary Boundary Layer With Models and Observations, J. Geophys. Res.-Atmos., 128, e2022JD037525, https://doi.org/10.1029/2022jd037525, 2023.
Petzold, A., Formenti, P., Baumgardner, D., Bundke, U., Coe, H., Curtius, J., DeMott, P. J., Flagan, R. C., Fiebig, M., Hudson, J. G., McQuaid, J., Minikin, A., Roberts, G. C., and Wang, J.: In Situ Measurements of Aerosol Particles, in: Airborne Measurements for Environmental Research, 157–223, https://doi.org/10.1002/9783527653218.ch4, 2013.
Prakash, G., Kumar, J., Rush, E., Records, R., Clodfelter, A., and Voyles, J.: HPC infrastructure to support the next-generation ARM facility data operations, 2016 IEEE International Conference on Big Data (Big Data), 5–8 December 2016, Washington, DC, USA, 4026–4028, https://doi.org/10.1109/BigData.2016.7841098, 2016.
Rew, R. K., Davis, G. P., Emmerson, S., and Davies, H.: NetCDF User's Guide for C, An Interface for Data Access, Version 3, Unidata (UCAR), Boulder, 1997.
Rosati, B., Herrmann, E., Bucci, S., Fierli, F., Cairo, F., Gysel, M., Tillmann, R., Größ, J., Gobbi, G. P., Di Liberto, L., Di Donfrancesco, G., Wiedensohler, A., Weingartner, E., Virtanen, A., Mentel, T. F., and Baltensperger, U.: Studying the vertical aerosol extinction coefficient by comparing in situ airborne data and elastic backscatter lidar, Atmos. Chem. Phys., 16, 4539–4554, https://doi.org/10.5194/acp-16-4539-2016, 2016.
Schmid, B., Tomlinson, J. M., Hubbe, J. M., Comstock, J. M., Mei, F., Chand, D., Pekour, M. S., Kluzek, C. D., Andrews, E., Biraud, S. C., and McFarquhar, G. M.: The DOE ARM Aerial Facility, B. Am. Meteorol. Soc., 95, 723–742, https://doi.org/10.1175/BAMS-D-13-00040.1, 2014.
Schumann, U., Fahey, D. W., Wendisch, M., and Brenguier, J.-L.: Introduction to Airborne Measurements of the Earth Atmosphere and Surface, in: Airborne Measurements for Environmental Research, 1–5, https://doi.org/10.1002/9783527653218.ch1, 2013.
Shilling, J. E., Pekour, M. S., Fortner, E. C., Artaxo, P., de Sá, S., Hubbe, J. M., Longo, K. M., Machado, L. A. T., Martin, S. T., Springston, S. R., Tomlinson, J., and Wang, J.: Aircraft observations of the chemical composition and aging of aerosol in the Manaus urban plume during GoAmazon 2014/5, Atmos. Chem. Phys., 18, 10773–10797, https://doi.org/10.5194/acp-18-10773-2018, 2018.
Shrivastava, M., Andreae, M. O., Artaxo, P., Barbosa, H. M. J., Berg, L. K., Brito, J., Ching, J., Easter, R. C., Fan, J., Fast, J. D., Feng, Z., Fuentes, J. D., Glasius, M., Goldstein, A. H., Alves, E. G., Gomes, H., Gu, D., Guenther, A., Jathar, S. H., Kim, S., Liu, Y., Lou, S., Martin, S. T., McNeill, V. F., Medeiros, A., de Sá, S. S., Shilling, J. E., Springston, S. R., Souza, R. A. F., Thornton, J. A., Isaacman-VanWertz, G., Yee, L. D., Ynoue, R., Zaveri, R. A., Zelenyuk, A., and Zhao, C.: Urban pollution greatly enhances formation of natural aerosols over the Amazon rainforest, Nat. Commun., 10, 1046, https://doi.org/10.1038/s41467-019-08909-4, 2019.
Snider, J. R., Leon, D., and Wang, Z.: Droplet Concentration and Spectral Broadening in Southeast Pacific Stratocumulus Clouds, J. Atmos. Sci., 74, 719–749, https://doi.org/10.1175/JAS-D-16-0043.1, 2017.
Springston, S. R.: Particle Soot Absorption Photometer (PSAP) Instrument Handbook, ARM user facility, https://doi.org/10.2172/1246162, 2018.
Tadić, J. M., Miller, S., Yadav, V., and Biraud, S. C.: Greenhouse gas fluxes from Alaska's North Slope inferred from the Airborne Carbon Measurements campaign (ACME-V), Atmos. Environ., 248, 118239, https://doi.org/10.1016/j.atmosenv.2021.118239, 2021.
Tang, S., Fast, J. D., Zhang, K., Hardin, J. C., Varble, A. C., Shilling, J. E., Mei, F., Zawadowicz, M. A., and Ma, P.-L.: Earth System Model Aerosol–Cloud Diagnostics (ESMAC Diags) package, version 1: assessing E3SM aerosol predictions using aircraft, ship, and surface measurements, Geosci. Model Dev., 15, 4055–4076, https://doi.org/10.5194/gmd-15-4055-2022, 2022.
Tang, S., Varble, A. C., Fast, J. D., Zhang, K., Wu, P., Dong, X., Mei, F., Pekour, M., Hardin, J. C., and Ma, P.-L.: Earth System Model Aerosol–Cloud Diagnostics (ESMAC Diags) package, version 2: assessing aerosols, clouds, and aerosol–cloud interactions via field campaign and long-term observations, Geosci. Model Dev., 16, 6355–6376, https://doi.org/10.5194/gmd-16-6355-2023, 2023.
Thompson, D. R., McCubbin, I., Gao, B. C., Green, R. O., Matthews, A. A., Mei, F., Meyer, K. G., Platnick, S., Schmid, B., Tomlinson, J., and Wilcox, E.: Measuring cloud thermodynamic phase with shortwave infrared imaging spectroscopy, J. Geophys. Res.-Atmos., 121, 9174–9190, https://doi.org/10.1002/2016jd024999, 2016.
Thornhill, A., Brown, C., Aknan, A., Crawford, J., Chen, G., and Williams, E.: NASA Standard for Airborne Data: ICARTT Format ESDS-RFC-019, AGU Fall Meeting Abstracts, IN51B-1587, 5–9 December 2011, San Francisco, California, 2011.
Uin, J. and Goldberger, L.: Nephelometer Aboard Aircraft Instrument Handbook, edited by: Stafford, R., ARM user facility, DOE/SC-ARM-TR-248, 2020.
Varble, A. C., Nesbitt, S. W., Salio, P., Hardin, J. C., Bharadwaj, N., Borque, P., DeMott, P. J., Feng, Z., Hill, T. C. J., Marquis, J. N., Matthews, A., Mei, F., Öktem, R., Castro, V., Goldberger, L., Hunzinger, A., Barry, K. R., Kreidenweis, S. M., McFarquhar, G. M., McMurdie, L. A., Pekour, M., Powers, H., Romps, D. M., Saulo, C., Schmid, B., Tomlinson, J. M., van den Heever, S. C., Zelenyuk, A., Zhang, Z., and Zipser, E. J.: Utilizing a Storm-Generating Hotspot to Study Convective Cloud Transitions: The CACTI Experiment, B. Am. Meteorol. Soc., 102, E1597–E1620, https://doi.org/10.1175/bams-d-20-0030.1, 2021.
Veals, P. G., Varble, A. C., Russell, J. O. H., Hardin, J. C., and Zipser, E. J.: Indications of a Decrease in the Depth of Deep Convective Cores with Increasing Aerosol Concentration during the CACTI Campaign, J. Atmos. Sci., 79, 705–722, https://doi.org/10.1175/jas-d-21-0119.1, 2022.
Wang, J., Krejci, R., Giangrande, S., Kuang, C., Barbosa, H. M., Brito, J., Carbone, S., Chi, X., Comstock, J., Ditas, F., Lavric, J., Manninen, H. E., Mei, F., Moran-Zuloaga, D., Pohlker, C., Pohlker, M. L., Saturno, J., Schmid, B., Souza, R. A., Springston, S. R., Tomlinson, J. M., Toto, T., Walter, D., Wimmer, D., Smith, J. N., Kulmala, M., Machado, L. A., Artaxo, P., Andreae, M. O., Petaja, T., and Martin, S. T.: Amazon boundary layer aerosol concentration sustained by vertical transport during rainfall, Nature, 539, 416–419, https://doi.org/10.1038/nature19819, 2016.
Wang, J., Wood, R., Jensen, M. P., Chiu, J. C., Liu, Y., Lamer, K., Desai, N., Giangrande, S. E., Knopf, D. A., Kollias, P., Laskin, A., Liu, X., Lu, C., Mechem, D., Mei, F., Starzec, M., Tomlinson, J., Wang, Y., Yum, S. S., Zheng, G., Aiken, A. C., Azevedo, E. B., Blanchard, Y., China, S., Dong, X., Gallo, F., Gao, S., Ghate, V. P., Glienke, S., Goldberger, L., Hardin, J. C., Kuang, C., Luke, E. P., Matthews, A. A., Miller, M. A., Moffet, R., Pekour, M., Schmid, B., Sedlacek, A. J., Shaw, R. A., Shilling, J. E., Sullivan, A., Suski, K., Veghte, D. P., Weber, R., Wyant, M., Yeom, J., Zawadowicz, M., and Zhang, Z.: Aerosol and Cloud Experiments in the Eastern North Atlantic (ACE-ENA), B. Am. Meteorol. Soc., 103, E619–E641, https://doi.org/10.1175/bams-d-19-0220.1, 2022.
Wang, Y., Bagya Ramesh, C., Giangrande, S. E., Fast, J., Gong, X., Zhang, J., Tolga Odabasi, A., Oliveira, M. V. B., Matthews, A., Mei, F., Shilling, J. E., Tomlinson, J., Wang, D., and Wang, J.: Examining the vertical heterogeneity of aerosols over the Southern Great Plains, Atmos. Chem. Phys., 23, 15671–15691, https://doi.org/10.5194/acp-23-15671-2023, 2023a.
Wang, Y., Zheng, X., Dong, X., Xi, B., and Yung, Y. L.: Insights of warm-cloud biases in Community Atmospheric Model 5 and 6 from the single-column modeling framework and Aerosol and Cloud Experiments in the Eastern North Atlantic (ACE-ENA) observations, Atmos. Chem. Phys., 23, 8591–8605, https://doi.org/10.5194/acp-23-8591-2023, 2023b.
Wendisch, M., Pilewskie, P., Bohn, B., Bucholtz, A., Crewell, S., Harlow, C., Jäkel, E., Schmidt, K. S., Shetter, R., Taylor, J., Turner, D. D., and Zöger, M.: Atmospheric Radiation Measurements, in: Airborne Measurements for Environmental Research, 343–411, https://doi.org/10.1002/9783527653218.ch7, 2013.
Wu, P., Dong, X., Xi, B., Tian, J., and Ward, D. M.: Profiles of MBL Cloud and Drizzle Microphysical Properties Retrieved From Ground-Based Observations and Validated by Aircraft In Situ Measurements Over the Azores, J. Geophys. Res.-Atmos., 125, e2019JD032205, https://doi.org/10.1029/2019jd032205, 2020.
Yang, F., McGraw, R., Luke, E. P., Zhang, D., Kollias, P., and Vogelmann, A. M.: A new approach to estimate supersaturation fluctuations in stratocumulus cloud using ground-based remote-sensing measurements, Atmos. Meas. Tech., 12, 5817–5828, https://doi.org/10.5194/amt-12-5817-2019, 2019.
Yeom, J. M., Yum, S. S., Shaw, R. A., La, I., Wang, J., Lu, C., Liu, Y., Mei, F., Schmid, B., and Matthews, A.: Vertical Variations of Cloud Microphysical Relationships in Marine Stratocumulus Clouds Observed During the ACE-ENA Campaign, J. Geophys. Res.-Atmos., 126, e2021JD034700, https://doi.org/10.1029/2021JD034700, 2021.
Zawadowicz, M. A., Suski, K., Liu, J., Pekour, M., Fast, J., Mei, F., Sedlacek, A. J., Springston, S., Wang, Y., Zaveri, R. A., Wood, R., Wang, J., and Shilling, J. E.: Aircraft measurements of aerosol and trace gas chemistry in the eastern North Atlantic, Atmos. Chem. Phys., 21, 7983–8002, https://doi.org/10.5194/acp-21-7983-2021, 2021.
Zhang, D., Vogelmann, A. M., Yang, F., Luke, E., Kollias, P., Wang, Z., Wu, P., Gustafson Jr., W. I., Mei, F., Glienke, S., Tomlinson, J., and Desai, N.: Evaluation of four ground-based retrievals of cloud droplet number concentration in marine stratocumulus with aircraft in situ measurements, Atmos. Meas. Tech., 16, 5827–5846, https://doi.org/10.5194/amt-16-5827-2023, 2023.
Zhang, Q., Zhou, S., Collier, S., Jaffe, D., Onasch, T., Shilling, J., Kleinman, L., and Sedlacek, A.: Understanding Composition, Formation, and Aging of Organic Aerosols in Wildfire Emissions via Combined Mountain Top and Airborne Measurements, in: Multiphase Environmental Chemistry in the Atmosphere, ACS Symposium Series, American Chemical Society, Washington DC, 363–385, https://doi.org/10.1021/bk-2018-1299.ch018, 2018.
Zhang, Z., Song, Q., Mechem, D. B., Larson, V. E., Wang, J., Liu, Y., Witte, M. K., Dong, X., and Wu, P.: Vertical dependence of horizontal variation of cloud microphysics: observations from the ACE-ENA field campaign and implications for warm-rain simulation in climate models, Atmos. Chem. Phys., 21, 3103–3121, https://doi.org/10.5194/acp-21-3103-2021, 2021.